Combining Snow Depth From FY-3C and In Situ Data Over the Tibetan Plateau Using a Nonlinear Analysis Method
- 1Data Service Office, National Meteorological Information Center, China Meteorological Administration, Beijing, China
- 2China Meteorological Administration Training Center, China Meteorological Administration, Beijing, China
- 3Meteorological Disaster Risk Management Division, National Climate Center, China Meteorological Administration, Beijing, China
- 4College of Physics and Electronic Engineering, Changshu Institute of Technology, Changshu, China
- 5Laboratory for Climate Studies, National Climate Center, China Meteorological Administration, Beijing, China
- 6College of Physics Science and Technology, Yangzhou University, Yangzhou, China
Snow cover over the Tibetan Plateau plays a vital role in the regional and global climate system because it affects not only the climate but also the hydrological cycle and ecosystem. However, high-quality snow data are hindered due to the sparsity of observation networks and complex terrain in the region. In this study, a nonlinear time series analysis method called phase space reconstruction was used to obtain the Tibetan Plateau snow depth by combining the FY-3C satellite data and in situ data for the period 2014–2017. This method features making a time delay reconstruction of a phase space to view the dynamics. Both of the grids and their nearby in situ snow depth time series were reconstructed with two appropriate parameters called time delay and embedding dimension. The values of the snow depth for grids were averaged over the in situ observations and retrieval of the satellite if their two parameters were the same. That implies that the two trajectories of the time series had the same evolution trend. Otherwise, the snow depth values for grids were averaged over the in situ observation. If there were no in situ sites within the grids, the retrieval of the satellite remained. The results show that the integrated Tibetan Plateau snow depth (ITPSD) had an average bias of –1.35 cm and 1.14 cm, standard deviation of the bias of 3.96 cm and 5.67 cm, and root mean square error of 4.18 cm and 5.79 cm compared with the in situ data and FY-3C satellite data, respectively. ITPSD expressed the issue that snow depth is usually overestimated in mountain regions by satellites. This is due to the introduction of more station observations using a dynamical statistical method to correct the biases in the satellite data.
Introduction
Snow over the Tibetan Plateau plays a prominent role in the climate system, hydrological cycle, and biogeochemical cycle [1–5]. It is also a primary indicator of climate change and significantly impacts local and global climate, water resources, and economic and society development [1, 6, 7]. Long-term and high-resolution data are prerequisites for climate change monitoring and assessments, and climate forecast, especially for snow depth [1, 8, 9] because snow depth can provide quantitative information about the material and energy of snow. Thus, there is an urgent need for operations and research on climatology and hydrology.
Conventional snow measurement through in situ devices has a long history [10]. For China, systematic observations can be traced back to the 1950s. The measure is high in accuracy and usually used to validate satellite retrieval snow products and reanalysis products. However, observational networks suffer from low station density in complex terrains due to the Tibetan Plateau’s remoteness, high altitude, and harsh weather conditions, especially for the western and middle Tibetan Plateau. The installation and maintenance of stations in the Tibetan Plateau are the main challenges [11, 12].
The past four decades witnessed the development of passive microwave remote sensing to acquire large-scale snow datasets [13]. It has become an effective way to estimate snow depth for providing all-day and all-weather monitoring and spatially continuous information of snow depth variation derived from the Scanning Multichannel Microwave Radiometer (SMMR), the Special Sensor Microwave/Imager (SSM/I), the Advanced Microwave Sounding Unit (AMSU), the Advanced Microwave Scanning Radiometer for EOS (AMSR-E), and the Microwave Radiation Imager (MWRI). The MWRI is onboard the FY-3 series satellite. It is important for snow monitoring of the Tibetan Plateau. However, it usually overestimates the snow depth by mistaking the cold surface as snow cover in the retrieval algorithms in the Tibetan Plateau. This issue needs to be investigated.
One way is to combine the snow depth from the remote sensing data and station observations. There are two classical approaches to do this. One includes using a semiempirical snow emission model. In the model, the passive microwave brightness between 20 and 150 GHz is assimilated and the in situ snow depth values are used as input to estimate snow grain size at the station locations. The disadvantages of this method are as follows: the model is in the progress stage and forward modeling of the microwave brightness in the above frequency range exhibits large uncertainties [14]. Another approach is statistical interpolation taking both satellite and in situ snow depth increments and terrain-dependent error correlations of snow depth increments into consideration. However, currently, snow depth is not assimilated due to the perceived unreliability of satellite estimates [15].
Phase space reconstruction is a nonlinear time series analysis method to reveal dynamic characteristics by expanding the time series to high dimensions, that is, a state space reconstruction method of delayed coordinates. This is a common method to predict the now and future state based on the past state in nonlinear time series analysis [7, 16]. It has achieved great success in climate prediction and analysis [17–22]. However, phase space reconstruction has rarely been applied to blend and analyze snow depth from satellites and in situ data. This study aimed to obtain accurate snow depth by integrating FY-3C satellite data and in situ data based on phase space reconstruction.
The remainder of the article is organized as follows. In Section 2, the study area and data used are described. The nonlinear analysis method—phase space reconstruction—and integrated Tibetan Plateau snow depth (ITPSD) bias correction model are introduced in Section 3. Section 4 presents the processes of combining snow depth observations from the FY-3C satellite and meteorological stations. In Section 5, the evaluation criteria of ITPSD are presented and the validation results are also provided in this section. The discussion and conclusion are presented in Section 6.
Study Area and Data
The study area is located at 25°N–40°N and 73°E–105°E and confines the Tibetan Plateau. It is about 2.6 million square kilometers in area. Most of it lies at an altitude of more than 4,000 m above sea level, making it peculiarly cold for its latitude—colder than anywhere else outside the polar regions, leading it to be known as the Third Pole [23]. It has an abundance of snow and ice cover. When they melt, the runoff from the region’s mountain feeds major rivers in Asia, such as the Yangtze, Yellow, Mekong, and Indus rivers. With the warming climate, snow cover is becoming even more important to gauge what is happening to the Tibetan Plateau and understand its potential impact on regional or global climate and water supply.
Due to the region’s remoteness, high altitude, and harsh weather conditions, there are only 340 meteorological stations which is much fewer than that in East China. The locations of the stations can be found in Figure 1. Some of the stations began operating in the 1950s. But they are insufficient to meet the needs for understanding the snow spatial variation of the Tibetan Plateau. The other common source of snow cover observation is remote sensing data. The Microwave Radiometer Imager (MWRI) is onboard the FY-3C satellite which was launched in 2014. Therefore, the study period was from 2014 to 2017. The MWRI daily product of snow depth and snow water equivalent was produced by the National Satellite Meteorological Center and is available at http://satellite.nsmc.org.cn/portalsite/default.aspx?currentculture=en-US#.
Methodology
A nonlinear prediction method called phase space reconstruction was used here to retrieve ITPSD. For nonlinear systems, it is a common method to view the dynamical factors of their evolution. For a grid point or an in situ site, its time series can be described by x1, x2, ..., xt, ..., xm, where m is the length of the record time. To reduce noise and view the dynamics of the time series, it was extended to three or even more dimensions, that is, a delayed coordinates phase space reconstruction (DCPSRC) was created. For a ƒ (n), the vector time series is given by the following equation:
where i denotes a grid point or an in situ site and τ is an appropriate time delay. Based on the decay of the auto-correlation function to 1/e, the appropriate time delay can be determined for each time series [24]. As for embedding dimension, the false nearest neighbor (FNN) approach was used to calculate the optimal embedding dimension [25].
In this study, to obtain ITPSD, DCPSRC was first applied to the time series of the in situ sites and grids from FY-3C, respectively. Then the optimal time delay (τ) and embedding dimension (n) for each site and grid were given and analyzed. Finally, ITPSD data were combined as per the following strategy. For a grid, if there were matched sites and its dynamic factors (i.e., the time delay and the embedding dimension) were similar to those of the sites, snow depth values of the grid were averaged over all the sources. If its dynamic factors were far from those of the sites, snow depth values of the grid were averaged over all the sites. If there were no matched sites, then its original values were referred to as the ITPSD.
Processes of Combining Integrated Tibetan Plateau Snow Depth
The process of integrating data from multiple sources into the ITPSD dataset takes four steps: 1) choosing stations for whose snow depth is greater than zero and include records of more than 30 days, and match the snow depth from FY-3C; 2) applying DCPSRC to the time series of the stations and grids from FY-3C; 3) deriving all the dynamic factors of the stations and grids; and 4) combining the data from the in situ sites and FY-3C satellite according to the strategy in part 3 to form comprehensive records.
In the initial step, records that have snow fall for more than 30 days from stations and the satellite are considered to meet the statistics of applying DCPSRC. There are 5,461 records meeting the conditions in total. The statement of records of snow cover of the stations and FY-3C satellite are shown in Table 1: 1) the missing rate of snow cover in stations is low, accounting for only 0.19%, while that of the FY-3C satellite is much higher and accounts for 37.98%; 2) both rates of no snow cover for the two sources are very high and that of the stations is higher with the value of 97.2%; 3) for trace snow, the rate of station records is 0.63%. However, it is hard for the satellite to retrieve this kind of snow information and the rate of satellite records is 0; and 4) as for snow depth greater than 1 cm, the rates of the stations and satellite are 1.98% and 1.30%, which are closer to each other than in other situations. This type of snow is concentrated on in this study. The records chosen in the first step match the records of the stations and satellite of this type.
The second and third steps are key to determining how to integrate the station and satellite data in the final step. The second step is to construct the delayed phase space for all the data to find their dynamic factors. The third step is to give the time delay and the embedding dimension of the stations and the grids. Table 2 shows the site information of 18 stations and their dynamic factors. Meanwhile, these stations have matched satellite grids and these grids’ dynamic factors are identified with those of stations. Their time delay is between 3 and 4, and the embedding dimension is between 8 and 9.
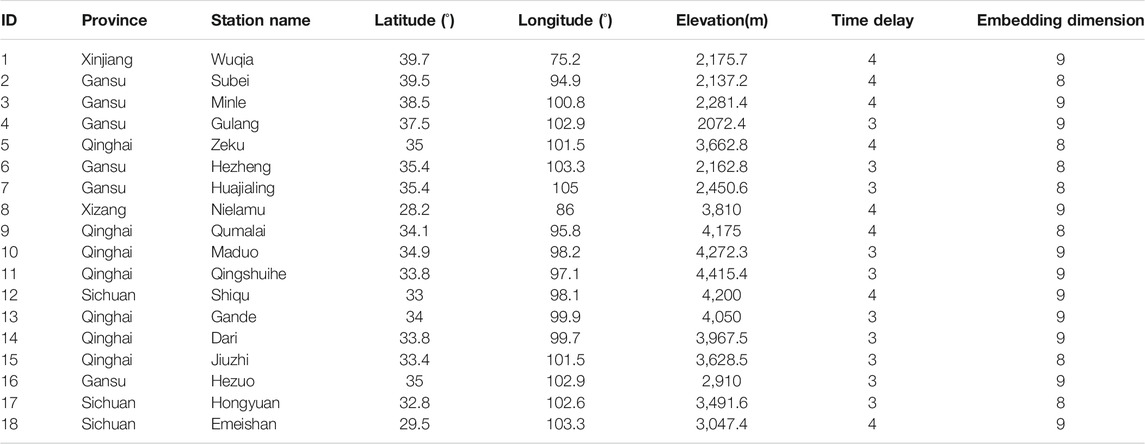
TABLE 2. Site information and dynamic factors of 18 stations which have the same dynamic factors as that of the satellite.
As shown in the Figure 2, the stations are Alpine stations and located at the edge of the Tibetan Plateau. This can be contributed to the fact that these sites have an abundance of snow cover and the atmosphere is clean due to less pollution. Therefore, the MWRI onboard the FY-3C satellite can penetrate the atmosphere more easily and reach the surface, and the bias correction effect is better.
Assessment and Results
Evaluation Matrices
To evaluate the data, four statistical accuracy measures were applied. The averaged bias (AB) was used for an assessment of the whole dataset bias between ITPSD, FY-3C satellite data, and in situ data. Standard deviation of the bias (SDB) evaluated the amount of variation or dispersion of the AB. Root mean square error (RMSE) measured their differences. Correlation coefficient (CC) assessed their relationships.
Here,
Assessment Results of Snow Depth
Blizzards and heavy snow were of great concern in the assessment (details just shown in Table 3), that is, when the snow depth was between 1 cm and 3 cm for blizzards and greater than 3 cm for heavy snow. The FY-3C satellite data and in situ data were not well correlated with negative values of CC around 0. The AB of them for heavy snow was rather low with a value of –0.13 cm, which meant that the FY-3C could easily identify heavy snow with little negative bias. The bias of blizzards between the FY-3C satellite data and in situ data was higher and the value was up to 7.4 cm. This indicates that blizzards were overestimated by the satellite, and blizzards also had low variation (SDB equaled 3.38 cm) but heavy snow had large variability (SDB was up to 12.87 cm). The RMSE was large both for blizzards and heavy snow.

TABLE 3. Comparison of different types of FY-3C satellite data and in situ data. The number of samples, CC, AB, SDB, and RMSE are listed.
The ITPSD and in situ data were highly correlated with the value of 0.93 for CC. While ITPSD and FY-3C satellite data were positively correlated, their CC was much lower with a value of 0.30 cm. The AB between the ITPSD and in situ data was –1.35 cm, while that between the ITPSD and FY-3C satellite data was 1.14 cm. The absolute value of them was around 1 cm, which meant that ITPSD had low bias both between the FY-3C satellite data and the in situ data. The variation of their bias was low with an SDB of 5.67 cm and 3.96 cm, respectively. The differences of the ITPSD between the FY-3C satellite data and in situ data were not apparently significant with an RMSE of 5.79 cm and 4.18 cm, respectively. Figure 3 shows the comparison of ITPSD and in situ data. The scatter plot is concentrated along two lines. And the slopes of them are about 1 and 0.3, respectively, as shown in Table 4. As for Figure 4, the ITPSD and FY-3C satellite data have moderate positive linear association with more outlier points.
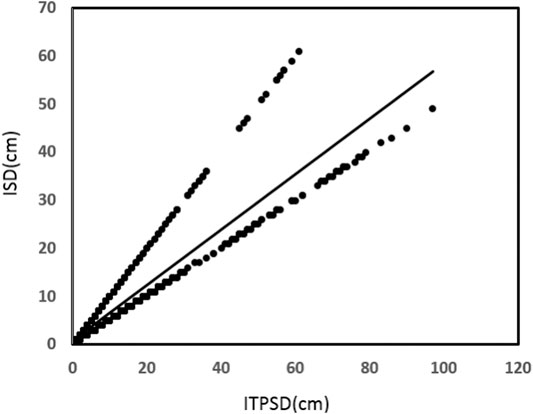
FIGURE 3. Scatter plots of ITPSD and in situ data. The corresponding RMSE and other statistics can be found in Table 4.

TABLE 4. Comparison of ITPSD with FY-3C satellite data and in situ data. The CC, AB, SDB, and RMSE are listed.
Discussion and Conclusion
In this study, a nonlinear time series analysis method called phase space reconstruction was introduced to improve the accuracy of the snow depth over the Tibetan Plateau by combining FY-3C satellite data and in situ data in the period 2014–2017. The results show that the method can integrate the FY-3C satellite data and in situ data effectively. This can be attributed to considering the evolution facts of snow with time to correct satellite bias and introducing more in situ observations. Other useful conclusions are as follows:
1) Eighteen stations and their matched FY-3C satellite grids were identified with the same dynamic factors (time delay and embedding dimension) of the method. The time delays were between 3 and 4 days.
This meant the snow depth time series had a short range of correlation. The embedding dimensions were between 8 and 9 indicating that in those dimensions the snow depth time series was ideal and noise free. The locations of the stations are at the edge of the Tibetan Plateau and they are Alpine stations. This can be attributed to the abundance of snow cover and the cleanness of the atmosphere in these stations. Therefore, the FY-3C satellite could retrieve snow data better.
2) A negative bias and a positive bias between the FY-3C snow depth and in situ snow depth for heavy snow and blizzards indicated that the FY-3C underestimated heavy snow but overestimated blizzards. For heavy snow, it was less underestimated with a value of –0.13 cm for the averaged bias. Blizzards were more likely to be overestimated with a value of 7.4 cm.
3) Integrated Tibetan Plateau snow depth had a positive linear association with the FY-3C snow depth and in situ snow depth. This relationship was strong between the integrated Tibetan Plateau snow depth and in situ snow depth, while that between the integrated Tibetan Plateau snow depth and the FY-3C snow depth was moderate.
Although the integrated Tibetan Plateau snow depth dataset is much accurate than that of the in situ and FY-3C data, more work needs to be done to extend the time span and density of the dataset. Furthermore, more datasets should be included, such as the ERA5 reanalysis dataset, to increase the samples of the data on dynamic integration.
Data Availability Statement
The datasets presented in this article are not readily available because the data are only used for the operation in CMA. Requests to access the datasets should be directed to fengax@cma.gov.cn.
Author Contributions
QW and ZG designed the study. AF performed the study and wrote the manuscript. FG, AF, QZ, YS, GF, and YZ provided suggestions and took part in the discussion.
Funding
This work is supported by the National Natural Science Foundation of China under grant numbers 41775081, 41975100, 41901016, 41875100, and 41675107, the Climate Change Project of the China Meteorological Administration under grant numbers CCSF202042, and the National Key Research and Development Program of China under grant number 2018YFC1507702.
Conflict of Interest
The authors declare that the research was conducted in the absence of any commercial or financial relationships that could be construed as a potential conflict of interest.
Acknowledgments
We thank the National Satellite Meteorological Center for providing the MWRI daily product of snow depth retrieved from FY-3C.
References
Gao L, Bernhardt M, Schulz K. Elevation Correction of ERA-Interim Temperature Data in Complex Terrain. Hydrol Earth Syst Sci (2012) 16:4661–73. doi:10.5194/hess-16-4661-2012
Gao L, Hao L, Chen X-w. Evaluation of ERA-Interim Monthly Temperature Data over the Tibetan Plateau. J Mt Sci (2014) 11:1154–68. doi:10.1007/s11629-014-3013-5
Ma Y, Hong Y, Chen Y, Yang Y, Tang G, Yao Y, et al. Performance of Optimally Merged Multisatellite Precipitation Products Using the Dynamic Bayesian Model Averaging Scheme over the Tibetan Plateau. J Geophys Res Atmos (2018) 123:814–34. doi:10.1002/2017jd026648
Yang K, Wu H, Qin J, Lin C, Tang W, Chen Y. Recent Climate Changes over the Tibetan Plateau and Their Impacts on Energy and Water Cycle: A Review. Glob Planet Change (2014) 112:79–91. doi:10.1016/j.gloplacha.2013.12.001
Zhu Y, Ding Y. Influences of Snow Cover over Tibetan Plateau on Weather and Climate: Advances and Problems. Meteorol Sci Technol (2007) 35:1–8.
Gao L, Schulz K, Bernhardt M. Statistical Downscaling of ERA-Interim Forecast Precipitation Data in Complex Terrain Using LASSO Algorithm. Adv Meteorology (2014) 2014:1–16. doi:10.1155/2014/472741
Gao L, Deng H, Lei X, Wei J, Chen Y, Li Z, et al. Evidence for Elevation-dependent Warming from the Chinese Tianshan Mountains. The Cryosphere (2020). doi:10.5194/tc-2020-188
Gao L, Bernhardt M, Schulz K, Chen X, Chen Y, Liu M. A First Evaluation of ERA-20CM over China. Mon Weather Rev (2016) 144:45–57. doi:10.1175/mwr-d-15-0195.1
Gao L, Bernhardt M, Schulz K, Chen X. Elevation Correction of ERA-Interim Temperature Data in the Tibetan Plateau. Int J Climatol (2017) 37:3540–52. doi:10.1002/joc.4935
Rasmussen R, Baker B, Kochendorfer J, Meyers T, Landolt S, Fischer AP, et al. How Well Are We Measuring Snow: The NOAA/FAA/NCAR winter Precipitation Test Bed. Bull Amer Meteorol Soc. (2012) 93:811–29. doi:10.1175/bams-d-11-00052.1
Kunkel KE. Simple Procedures for Extrapolation of Humidity Variables in the Mountainous Western United States. J Clim (1989) 2:656–70. doi:10.1175/1520-0442(1989)002<0656:spfeoh>2.0.co;2
Rolland C. Spatial and Seasonal Variations of Air Temperature Lapse Rates in Alpine Regions. J Clim (2003) 16:1032–46. doi:10.1175/1520-0442(2003)016<1032:sasvoa>2.0.co;2
Rostosky P, Spreen G, Farrell SL, Frost T, Heygster G, Melsheimer C. Snow Depth Retrieval on Arctic Sea Ice from Passive Microwave Radiometers-Improvements and Extensions to Multiyear Ice Using Lower Frequencies. J Geophys Res Oceans (2018) 123:7120–38. doi:10.1029/2018jc014028
Grody N. Relationship between Snow Parameters and Microwave Satellite Measurements: Theory Compared with Advanced Microwave Sounding Unit Observations from 23 to 150 GHz. J Geophys Res (2008) 113:D22108. doi:10.1029/2007jd009685
Kongoli C, Key J, Smith TM. Mapping of Snow Depth by Blending Satellite and In-Situ Data Using Two-Dimensional Optimal Interpolation-Application to AMSR2. Remote sensing (2019) 11:3049. doi:10.3390/rs11243049
Feng G, Dong W, Gong Z, Hou W, Wan S, Zhi R. The Nonlinear Spatiotemporal Theory and Method of Observations. Beijing: China Meteorological Press (2006).
Feng G-L, Yang J, Zhi R, Zhao J-H, Gong Z-Q, Zheng Z-H, et al. Improved Prediction Model for Flood-Season Rainfall Based on a Nonlinear Dynamics-Statistic Combined Method. Chaos, Solitons & Fractals (2020) 140:110160. doi:10.1016/j.chaos.2020.110160
Feng A, Gong Z, Huang Y, Wang Q. Spatiotemporal Analysis of Information Entropy of the Global Temperatur. Acta Phys Sin (2011) 60:099204.
Feng A, Wang Q, Gong Z, Feng G. Vertical Structure of Predictability and Information Transport over the Northern Hemisphere. Chin Phys B (2014) 23(2):029202. doi:10.1088/1674-1056/23/2/029202
Tsonis AA, Elsner JB. Mapping the Channels of Communication between the Tropics and Higher Latitudes in the Atmosphere. Physica D: Nonlinear Phenomena (1996) 92:237–44. doi:10.1016/0167-2789(95)00265-0
Wang G, Yang P. A Compound Reconstructed Prediction Model for Nonstationary Climate Processes. Int J Climatol (2005) 25:1265–77. doi:10.1002/joc.1158
Wang G, Yang P, Bian J, Zhou X. A Novel Approach in Predicting Non-stationary Time Series by Combining External Forces. Chin Sci Bull (2011) 56:3053–6. doi:10.1007/s11434-011-4638-1
Keywords: snow depth, Tibetan Plateau, phase space reconstruction, FY-3C satellite, nonlinear analysis method
Citation: Feng A, Gao F, Wang Q, Feng A, Zhang Q, Shi Y, Gong Z, Feng G and Zhao Y (2021) Combining Snow Depth From FY-3C and In Situ Data Over the Tibetan Plateau Using a Nonlinear Analysis Method. Front. Phys. 9:672288. doi: 10.3389/fphy.2021.672288
Received: 25 February 2021; Accepted: 17 May 2021;
Published: 11 June 2021.
Edited by:
Yipeng Guo, Nanjing Normal University, ChinaReviewed by:
Hongxing Cao, Chinese Academy of Meteorological Sciences, ChinaAnna Carbone, Politecnico di Torino, Italy
Shixuan Zhang, Pacific Northwest National Laboratory (DOE), United States
Copyright © 2021 Feng, Gao, Wang, Feng, Zhang, Shi, Gong, Feng and Zhao. This is an open-access article distributed under the terms of the Creative Commons Attribution License (CC BY). The use, distribution or reproduction in other forums is permitted, provided the original author(s) and the copyright owner(s) are credited and that the original publication in this journal is cited, in accordance with accepted academic practice. No use, distribution or reproduction is permitted which does not comply with these terms.
*Correspondence: Qiguang Wang, photon316@163.com; Zhiqiang Gong, gzq0929@126.com