- 1Department of Radiation Dosimetry, Nuclear Physics Institute, Czech Academy of Sciences, Prague, Czechia
- 2Institute of Radiation Medicine, Helmholtz Zentrum München—German Research Center for Environmental Health (GmbH), Neuherberg, Germany
- 3Radiation Biophysics and Radiobiology Group, Physics Department, University of Pavia, Pavia, Italy
Existing radiation codes for biomedical applications face the challenge of dealing with largely different spatial scales, from nanometer scales governing individual energy deposits to macroscopic scales of dose distributions in organs and tissues in radiotherapy. Event-by-event track-structure codes are needed to model energy deposition patterns at cellular and subcellular levels. In conjunction with DNA and chromatin models, they predict radiation-induced DNA damage and subsequent biological effects. Describing larger-scale effects is the realm of radiation transport codes and dose calculation algorithms. A coupling approach with a great potential consists in implementing into radiation transport codes the results of track-structure simulations captured by analytical formulas. This strategy allows extending existing transport codes to biologically relevant endpoints, without the need of developing dedicated modules and running new computationally expensive simulations. Depending on the codes used and questions addressed, alternative strategies can be adopted, reproducing DNA damage in dependence on different parameters extracted from the transport code, e.g., microdosimetric quantities, average linear energy transfer (LET), or particle energy. Recently, a comprehensive database on DNA damage induced by ions from hydrogen to neon, at energies from 0.5 GeV/u down to their stopping, has been created with PARTRAC biophysical simulations. The results have been captured as a function of average LET in the cell nucleus. However, the formulas are not applicable to slow particles beyond the Bragg peak, since these can have the same LET as faster particles but in narrower tracks, thus inducing different DNA damage patterns. Particle energy distinguishes these two cases. It is also more readily available than LET from some transport codes. Therefore, a set of new analytical functions are provided, describing how DNA damage depends on particle energy. The results complement the analysis of the PARTRAC database, widening its potential of application and use for implementation in transport codes.
Introduction
Radiation transport codes are a key tool for many research and practical applications dealing with radiation-matter interactions. Many codes exist, including FLUKA, Geant4, MCNP, PHITS or TOPAS [1–5]; recent reviews focused on radiotherapy applications can be found in [6, 7]. In most cases a common issue has to be faced: The radiation field and the resulting dose distribution have to be captured at a rather large spatial scale, e.g., in a human body, in a high-energy physics detector, or in a shielding layer in a space habitat. At the same time, however, radiation action proceeds through energy deposition events (mainly ionizations and excitations of target atoms) that are spatially distributed with a ∼nm spacing. Coupling these spatial scales and dealing with them in a common simulation framework is, without a doubt, a challenge. Two main approaches exist, namely the condensed-history approach, suitable for macroscopic simulations, and event-by-event track-structure approach, dedicated to microscopic and nanoscopic simulations. In the condensed-history approach, the radiation transport simulation algorithm deals with the cumulative effects of multiple particle collisions, approximated in a single “step.” The step is modelled as a path free of interactions, and an overall change in particle energy (using stopping power functions) and direction of motion is considered at its end. The actual free path length, energy lost and deflection are sampled stochastically. Nuclear reactions, leading to the production of different particles after the interaction has taken place, can also be simulated. Codes using such an approach are widely and successfully applied and allow accurate calculation of, e.g., radiation dose in targets of different sizes. On the contrary, track-structure codes [8, 9] are developed to simulate all single collisions with atomic electrons in the target in an event-by-event manner. The outcome of the simulation is the set of spatial coordinates of ionizations and excitations induced by the primary particle and its secondary electrons, and energy depositions in each event, forming the particle track. Concerning different target materials, radiation transport with a condensed-history approach mainly requires the knowledge of particle stopping power (as well as cross-section data for nuclear reactions), while an event-by-event simulation requires accurate knowledge of all interaction cross sections with atomic electrons [10]. Track-structure calculations are therefore mostly performed in water only.
For medical applications of ionizing radiation, but also in radiation protection, the macroscopic scale of interest is that of the whole radiation field impacting on the person. Accurate calculations of physical absorbed dose are needed in the organs and tissues of the exposed person, even for radiotherapy applications not only in the treatment volume but also in surrounding regions [11, 12]. Different elemental compositions and tissue inhomogeneities must be considered. At the same time, radiation effects in terms, e.g., of cancer cell killing or inducing genomic aberrations or mutations can be traced back to radiation interactions taking place at the scale of sensitive subcellular targets such as nuclear DNA or chromosomal domains. The description and, as far as possible, prediction of these effects requires simulation tools with a track-structure approach, coupled to a software replica of the target genomic content. Also, in addition to interactions with the DNA molecule (direct damage), it is necessary to consider that energy depositions in water in the cell can lead to water radiolysis, with the production of free radicals that can diffuse, chemically react with one another and damage DNA (indirect damage). Advanced track-structure codes such as PARTRAC [8] have been developed in this sense, currently allowing the full simulation of the chain of events from radiation traversal of a single-cell model (using liquid water as a surrogate material) to the induction of initial DNA damage (as well as, to some extent, damage evolution and repair).
Within this general context, efforts are currently ongoing to develop biophysical simulation tools that can provide at the same time a macroscopic description of the radiation field and its consequences in terms of damage at the cellular/subcellular level, e.g., the Geant4/Geant4-DNA project [13]. However, different strategies can be pursued to couple existing radiation transport codes using the condensed-history approach with track-structure codes. This would lead to a better exploitation of available tools, extending the applicability of transport codes towards biological endpoints, and of track-structure codes towards larger volumes and inclusion of secondary particles produced by nuclear reactions. A simple coupling approach with a great potential consists in the implementation in radiation transport codes of analytical formulas or look-up tables that capture the results of track-structure simulations on the induced DNA damage. Proceeding in this way brings a number of important advantages: Existing radiation transport codes either already provide the information needed for the coupling, or can be easily developed to do so; The coupling can also be performed off-line by the users, requiring no real development of the transport code; Implementation of formulas or tables in the transport code also leads to a significant saving of computational time with respect to running new simulations.
The question then arises as to which information is best suited to make the bridge between the output of the radiation transport and the track-structure code. A radiation transport code should be able to describe the characteristics of the radiation field with a wide choice of observables (particle types, energy distribution, LET distribution, direction of motion, etc.) in targets of varying sizes, ideally down to the step length used for calculations in the condensed-history approach. Of course, a full characterization of the field at a spatial scale that corresponds to the volume of a single cell is neither practical nor reasonable to achieve in terms of computational time when considering a macroscopic target. For instance, in the scenario of a voxelized target, the typical size of the cubic voxel is of the order of ∼1 mm, and averaging procedures can be used to extract parameters that describe the radiation field in the voxel. Interestingly, analytical formulas have been implemented, e.g., in the code PHITS, which allow calculation of the distribution of microdosimetric quantities in macroscopic target regions [5]. By definition, microdosimetric quantities as the lineal energy are built considering the stochasticity of energy depositions by radiation in sensitive sites of micrometric size. As such, it could be argued that they are ideal to characterize the radiation field in a single cell nucleus that can be assumed as a quasi-spherical volume with a linear dimension of ∼10 µm. These considerations are at the basis of our previous works, in which we proposed a full coupling between PHITS and PARTRAC to derive the RBE of neutrons of different energy [14, 15], as well as a full set of analytical functions [16] reproducing PARTRAC results on different types of DNA damage as a function of an LET estimate, when the cell nucleus is irradiated with a variety of light ions at radiotherapy-relevant energies down to stopping. Such LET estimate, obtained by dividing the dose delivered to the cell nucleus by particle fluence (with appropriate conversion of units), is indeed analogous to the concept of the dose-mean lineal energy in microdosimetry. However, some limitations exist for this coupling approach using LET-based functions: The dose-mean lineal energy (or a definition of LET that is coherent with that adopted in PARTRAC calculations) might not be readily available for all transport codes. Furthermore, the same LET value is crossed twice as the particle slows down, in its distal and proximal parts of the Bragg peak, but the track characteristics, including its biological effectiveness, are different. Consequently, when plotting DNA damage as a function of LET, hooks appear, and single-valued mathematical functions cannot reproduce the full set of results including the lowest particle energies. A representation of DNA damage as a function of particle energy can instead be proposed to solve this issue. It also has the advantage that particle energy distributions can be easily extracted from transport codes.
Starting from these considerations, we provide in this work a set of new analytical functions exploring how PARTRAC results on DNA damage depend on particle energy, for light ions up to Ne, with energies per nucleon (specific energies, hereafter termed just energies) from 0.5 GeV/u down to stopping. The new formulas reproduce DNA damage yields per unit dose or per particle track, and complement previous results for damage yields as a function of particle LET. Overall, different choices are thus made possible for transport code users who want to implement such formulas in a radiation transport code. We further discuss how the availability of these formulas sets the basis of an efficient strategy to perform the coupling between radiation transport and track-structure codes. We discuss the limitations of such a strategy and how these can be addressed, in particular in relation to the issue of electronic equilibrium. Results of the full coupling between transport and track-structure codes for specific case studies shall be presented elsewhere, this work widening the potential of application of PARTRAC results and their use for an extension of existing transport codes to derive DNA-damage-based estimates of biological effectiveness.
Materials and Methods
PARTRAC Track-Structure Simulations
PARTRAC [8, 17–21] is a family of biophysical simulation tools for modelling the biological effects of ionizing radiation at subcellular and cellular scales. It possesses a modular structure that reflects the underpinning mechanisms and processes. Individual modules integrate knowledge from radiation physics, chemistry, biophysics of DNA and its radiation damage, and radiation biology. The modular structure of the tool facilitates model refinements, integration of novel features, as well as extensions to further endpoints.
The simulations start with a module that describes physical processes of radiation interaction with the traversed matter. The code is capable of handling photons, electrons, protons and light ions over a wide energy range as relevant for natural, medical and technical applications. Typically, liquid water is used as a surrogate for biological material. For photons, the user may specify a homogeneous medium with an arbitrary atomic composition. For each particle type, established cross-section data are implemented. The respective dataset is stochastically sampled to generate an in silico representation of the interaction pattern, in an event-by-event mode, i.e., simulating individual ionizations, excitations and further processes (except nuclear reactions). The primary particle is followed until stopping or until leaving the given volume of interest, which typically surrounds a cell. Also, all secondary electrons liberated by the primary particles are tracked, as well as all higher-order particles. Each such generated track is different, but they share some global features, e.g., dense core regions in ion tracks surrounded by penumbras with sparse secondary electron tracks.
In a subsequent module that represents pre-chemical and chemical processes of track development, individual ionization and excitation events are converted to reactive species or relaxed to water molecules. Branching ratios, yields of diverse species, and their diffusive properties are implemented. Diffusion of species is followed together with their mutual reactions, in a step-by-step mode. In addition to mutual reactions, the species are removed upon attacking cellular DNA, histones, or in non-specific reactions governed by their lifetimes.
Multi-scale DNA and chromatin models are implemented. They range from an atomic model of DNA double-helix, over its binding to nucleosomes, formation of ∼30 nm chromatin fiber, its loops and domains to chromosome territories within cell nuclei. Two such multi-scale models are available in PARTRAC at present, a spherical one for human lymphocytes and an elliptical one for human fibroblasts.
By overlaying these DNA and chromatin models with radiation tracks in terms of energy deposition patterns (so-called direct radiation effects) and attacks of reactive species (indirect effects), DNA damage is scored. Energy deposits within the sugar-phosphate backbone as well as attacks of hydroxyl radicals are in a stochastic manner converted to DNA strand breaks. Breaks on both strands within a genomic distance of 10 base pairs (bp) are scored as double-strand breaks (DSBs). Even DSBs may cluster, especially for high-LET particles. DSB clusters are scored whenever two or more DSBs appear within 25 bp. DSB clusters are an example of clustered lesions (locally multiply damaged sites) that likely pose critical challenges to cellular repair systems, and may represent initial damage finally leading to chromosome aberrations and cell killing. The term DSB sites includes both isolated DSBs and their clusters: an isolated DSB forms a DSB site, and a cluster of, e.g., three DSBs separated by less than 25 bp from each other is scored as a single DSB site as well. Beyond this simple classification scheme, PARTRAC includes another module that allows the user to score DNA fractionation patterns on numerous scales ranging from very short fragments (tens of bp) up to fragments of chromosomes (hundreds of Mbp).
Finally, much attention has been devoted in the last decade to the development of the repair module in PARTRAC [17, 18, 22–25]. It represents the non-homologous end-joining pathway of DSB repair, the dominant DSB repair in human cells in G0 and G1 cell-cycle phases. The module explicitly accounts for the action of repair enzymes as well as for the mobility of chromatin breaks. It has been extended to chromosome aberrations too. Promising preliminary results have been obtained for the endpoint of cell killing as well.
PARTRAC results have been validated against available large experimental datasets within radiation physics, chemistry, and biology, cf. [8] and Refs. therein. The tool has served as a benchmark for other codes. Its recent applications included a model that provided mechanistic interpretation for the biological effectiveness of neutrons [14, 15], a model of radiation-induced bystander effects [26–30], or a study on radiation effects on mitochondrial DNA [31].
In this work, we have analyzed the previously published comprehensive database of PARTRAC simulations on DNA damage induced by light ions [20, 21]. Model cell nuclei were irradiated by 1H, 4He, 7Li, 9Be, 11B, 12C, 14N, 16O or 20Ne ions at starting energies of 0.25, 0.5, 1, 2, 4, 8, 16, 32, 64, 128, 256 or 512 MeV/u. The ions were started fully stripped of electrons. The ion source was an 80 µm2 circle, placed tangentially to a model lymphocyte nucleus (sphere with 10 µm diameter, containing 6.6 Gbp DNA in 23 chromosome pairs). Interactions were scored in a spherical “world” region of interest with the diameter of 14.22 µm, concentric with the nucleus model. Per ion type and energy, at least 1,280 particles were simulated (up to 3.2 million high-energy protons). The simulations were divided into 256–8,192 runs with 5–3,200 particles per run. Statistical uncertainties are reported as ± 1.96 times the standard deviation, hence covering 95% confidence intervals on the simulated DNA damage yields, calculated based on Poisson distributions.
DSBs, DSB clusters and DSB sites were scored. From absolute numbers of the lesions and the deposited dose, damage yields per Gy per Gbp were calculated. In this work, this is referred to as a dose-based approach. Alternatively, damage yields were considered per track per Gbp, which may serve as a basis for a fluence-based approach. These two approaches can both be used to couple radiation transport to track-structure simulations using different strategies. It is up to the transport code user to select the appropriate one, depending on the availability of radiation transport results and on the specific case under study. This is addressed later in the discussion.
Analytical Representation of DNA Damage Yields
To enable the conversion from the dose-based approach to the fluence-based one, the simulation results on the dose deposited in the nucleus per track Dtrack (in Gy) were fitted as a function of the ion starting energy E (in MeV/u) by:
The selection of this functional form was motivated by the shape of the simulation results in a log-log scale (cf. the Results section). This function combines two power-law terms,
The yields Y of DSBs, DSB clusters and DSB sites (expressed per Gy per Gbp) were fitted as a function of the ion starting energy E (expressed in MeV/u) by:
These functions combine three power-law terms with logistic-like transitions in-between. For parameters p > 0, the first and the last term decrease, while the second one increases with increasing energy. Parameters p2, p4 and p7 govern the position of these logistic power-law functions; p3, p5 and p8 their slopes; p1 and p6 the magnitude of the effects; and p0 depicts the high-energy asymptotic behavior. The high-energy (i.e., low-LET) behavior was taken from Ref. 16, using the following values: p0 = 6.8 DSB, 0.07 DSB clusters, and 6.8 DSB sites per Gy per Gbp.
Parameters of these test functions were fitted to the results of track-structure simulations using non-linear model fitting in Matlab (The MathWorks Inc., United States). This was done for each damage type and each ion separately, and for per-track doses as well. Some terms or parameters were not needed in some cases, and were thus not included (cf. Results). A few parameter values were fixed manually (in particular the parameter p8 for DSBs and their sites, cf. Results) in order to help avoid overfitting, especially to avoid local minima or maxima that otherwise might occur as mere artifacts of the fitting procedure, with no support by the simulation results. Damage yields per track per Gbp were then obtained by the product of formula (2) for the yields per Gy per Gbp and formula (1) for the dose per track.
Amorphous Track-Structure Calculations
With the given setup of PARTRAC track-structure simulations, only ions were considered whose track core regions hit the nucleus. Ions passing nearby and hitting the nucleus only by their penumbra regions were neglected. This approach was selected to limit the computational costs of track-structure simulations for high-energy ions that produce very wide but low-density penumbra regions, e.g., 512MeV/u protons liberating secondary electrons with ranges in water and hence track radius of a few millimetres but total LET (including the track core) of about 0.2 keV/μm only. To assess the limitations of this approach, additional calculations were performed using an amorphous track structure model [32] which assumes a radial dose distribution:
where the track radius rtr (in µm) is given by rtr = 0.062 E1.7, with E (MeV/u) denoting the ion energy [32, 33], and λ is an integration constant proportional to the LET of the track. The fraction of LET deposited in the inner part of the track (up to a specific distance R from the track axis, e.g., the radius of cell nucleus) was then calculated by:
where ln denotes natural logarithm. The average LET fraction deposited to the spherical cell nucleus by tracks that hit the nucleus by their core regions (and alternatively by nearby tracks) was calculated in Matlab (The MathWorks Inc., United States) by numerically integrating the radial dose distribution overlapping with the cell nucleus and averaging over the impact parameters (i.e., the distance of the track core from the cell nucleus centre).
Results
Mean doses deposited per track to the spherical cell nucleus are presented vs particle starting energy in Figure 1. The results in Figure 1 do not include tracks whose cores do not intersect the nucleus but whose penumbra may still deposit energy there. The results of PARTRAC event-by-event track-structure simulations are depicted by symbols. Lines show their fits using formula (1), with parameters listed in Table 1. As the doses deposited per particle cover a very wide range, from 0.44 mGy for 512 MeV protons (H ions) to 3.03 Gy for 1 MeV/u Ne ions, we show the plot in both logarithmic and linear scales. Except for 512 MeV/u, the analytical formulas nicely reproduce the simulation results. In particular, they capture the overall power-law increase of deposited dose with decreasing energy, down to about 0.5–1 MeV/u. Also the decrease of deposited dose with further decreasing energy is reproduced. Note that the plotted doses are mean values over all simulated tracks. The actual energy deposit by a track depends on its path length within the nucleus, and hence hugely differs from tracks whose cores hit the nucleus centrally to those hitting it only peripherally. Even larger variations would be obtained if also tracks were considered that overlap with the nucleus by their penumbras only (cf. Discussion). The stochastic nature of individual interactions plays a minor role only, due to the high numbers of events per track.
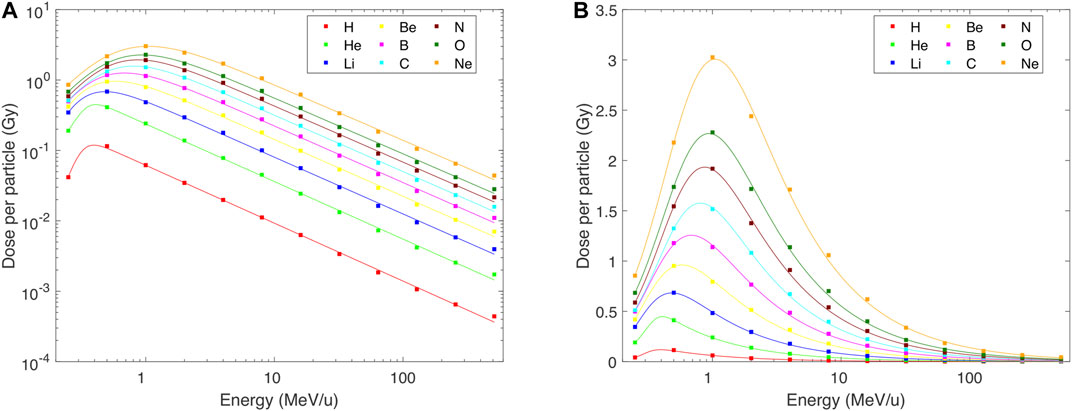
FIGURE 1. Mean dose deposited to the lymphocyte nucleus per single track of H–Ne ions with starting energies from 0.25 to 512 MeV/u, as simulated with PARTRAC event-by-event track-structure simulations (symbols) and fitted by analytical formulas Eq. 1 (lines) with parameters listed in Table 1. Panel (A) in logarithmic scale to highlight the wide range of values and the quality of fits; panel (B) in linear scale.
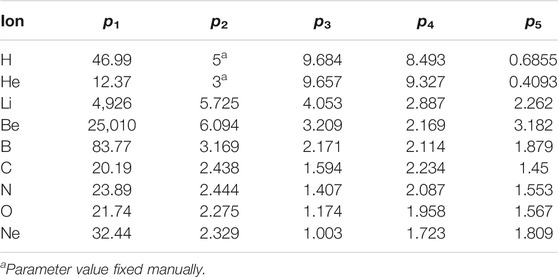
TABLE 1. Parameters of analytical functions (Eq. 1) describing the dose deposited to the cell nucleus (in Gy) per single started particle as a function of its starting energy (in MeV/u).
DNA damage patterns largely but not fully follow the pattern of the dose per track. Indeed, when the yields of DSBs, DSB clusters or DSB sites are plotted per track (Figures 2–4 panels A and B), the overall patterns resemble those in Figure 1: The induction of each of these damage classes increases with decreasing energy, approximately according to a power law down to about 0.5–1 MeV/u, and then decreases. However, a finer structure is revealed when damage yields are analyzed per unit dose: Total DSB yields (Figure 2C) increase from about 6.8 DSB per Gy per Gbp at high energies to about three times higher values at around 1 MeV/u for light ions, and saturate at around 16 DSB per Gy per Gbp for B–Ne ions below about 10 MeV/u. There is a systematic indication for a wavy behavior at these low energies for relatively heavy ions. Much more pronounced variations are seen for DSB clusters: Their yields (Figures 3C,D) increase from values as low as 0.07 per Gy per Gbp at high energies to about 1 for H, 2.6 for He, and 1.9–2.8 per Gy per Gbp for heavier ions at energies of 1–10 MeV/u. Similarly to DSBs, also for clusters the simulations predict a wavy behavior with local minima around 1 MeV/u for ions from C to Ne. The yields of DSB sites (Figure 4C) at high energies are about equal to total DSBs, as the vast majority of DSBs are formed as isolated ones. At low energies, however, the yields of DSB sites drop down, for the heaviest studied ions getting close to or even below the high-energy values. This happens as an increasing fraction of DSBs form clusters, which are counted as single sites only but as multiple DSBs. The simulations suggest that cluster yields may further increase at energies <0.5 MeV/u. For all damage classes, the results of track-structure simulations are nicely reproduced by the present analytical formulas. This holds not only for the damage yields per Gy (panels C) that were fitted by the formulas, but also for the yields per track (panels A and B) which were not fitted directly. Even the wide ranges of DSB cluster yields are represented properly (cf. the log-log plot in Figure 3D).
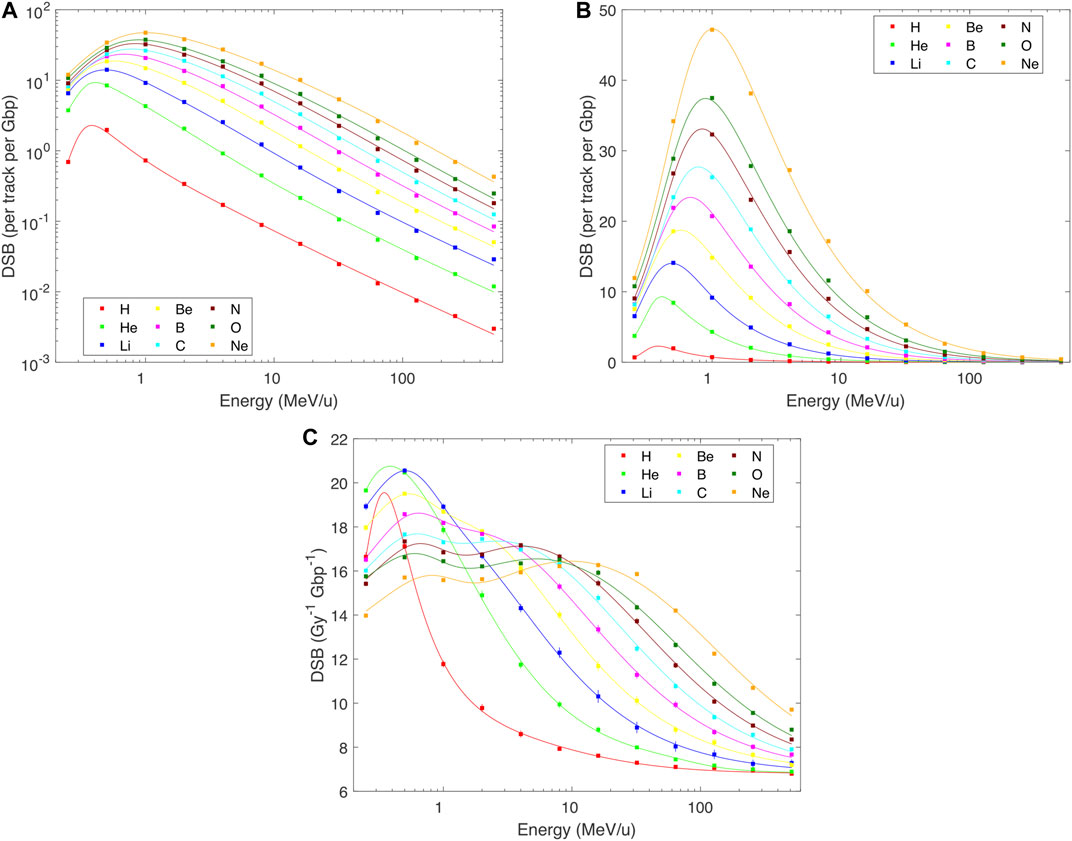
FIGURE 2. PARTRAC simulations of the yields of DNA double-strand breaks (DSBs) in human lymphocytes by H–Ne ions with starting energies from 0.25 to 512 MeV/u (symbols), fitted by analytical formulas (lines). Error bars depict estimated 95% confidence intervals of the simulated results. Where error bars are not visible, they are smaller than the symbols. Panels (A,B): yields per track per Gbp, in logarithmic and linear scales, calculated as a product of Eqs 1 and 2, details in the text; panel C: yield per Gy per Gbp, in linear scale, fitted by Eq. 2 with parameters in Table 2.
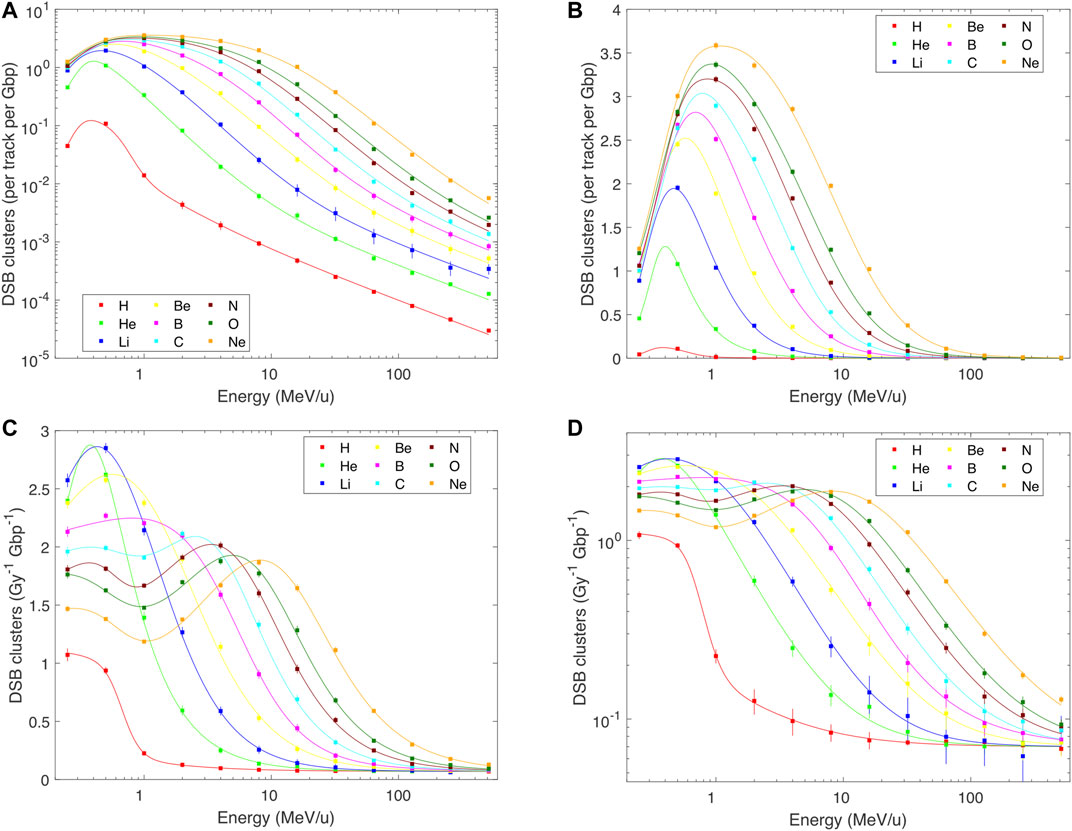
FIGURE 3. PARTRAC simulations (symbols) and their fits by analytical formulas (lines) for the yields of DNA double-strand break clusters (DSB clusters) in human lymphocytes by H–Ne ions with starting energies from 0.25 to 512 MeV/u. Error bars depict estimated 95% confidence intervals of the simulated results. Where error bars are not visible, they are smaller than the symbols. Panels (A,B): yields per track per Gbp, in logarithmic and linear scales, calculated as a product of Eqs 1 and 2, details in the text; panels (C,D): yield per Gy per Gbp, in linear and logarithmic scales, fitted by Eq. 2 with parameters in Table 2.
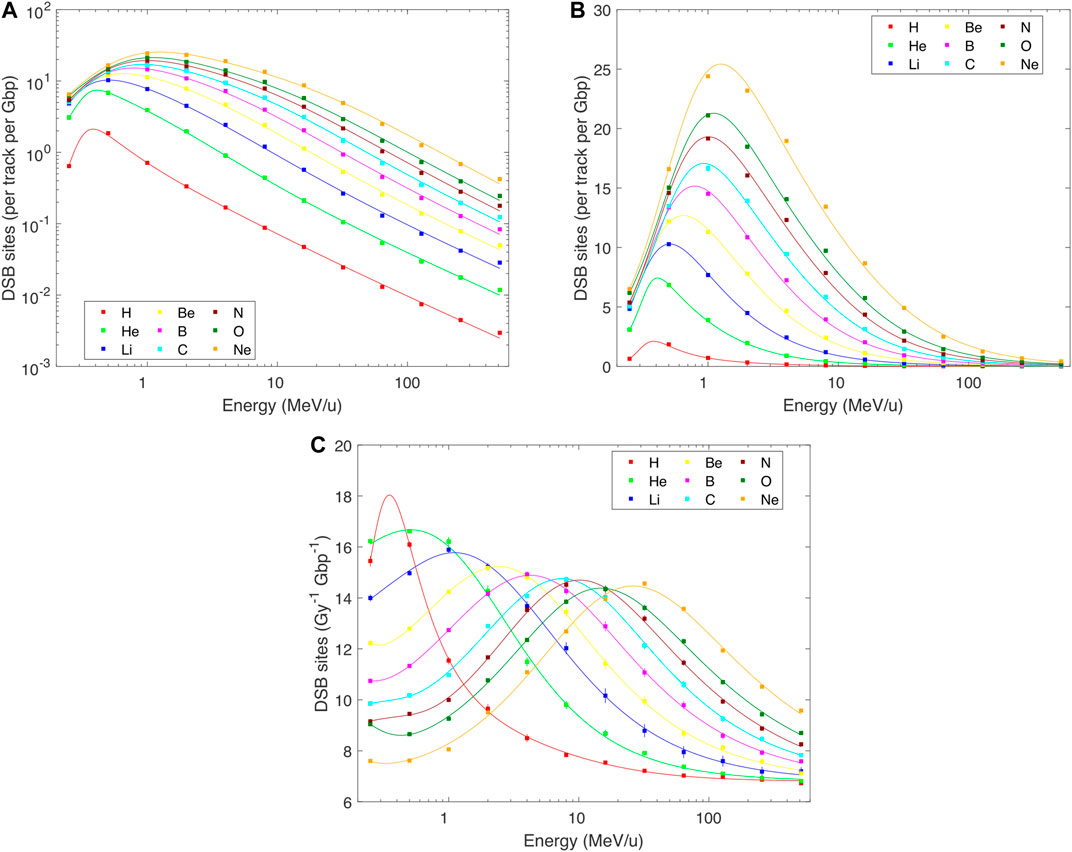
FIGURE 4. PARTRAC simulations on DNA double-strand break sites (DSB sites) induced in human lymphocytes by H–Ne ions with starting energies from 0.25 to 512 MeV/u (symbols), fitted by analytical formulas (lines). Error bars depict estimated 95% confidence intervals of the simulated results. Where error bars are not visible, they are smaller than the symbols. Panels (A,B): yields per track per Gbp, in logarithmic and linear scales, calculated as a product of Eqs 1 and 2, details in the text; panels (C): yield per Gy per Gbp, in linear scale, fitted by Eq. 2 with parameters in Table 2.
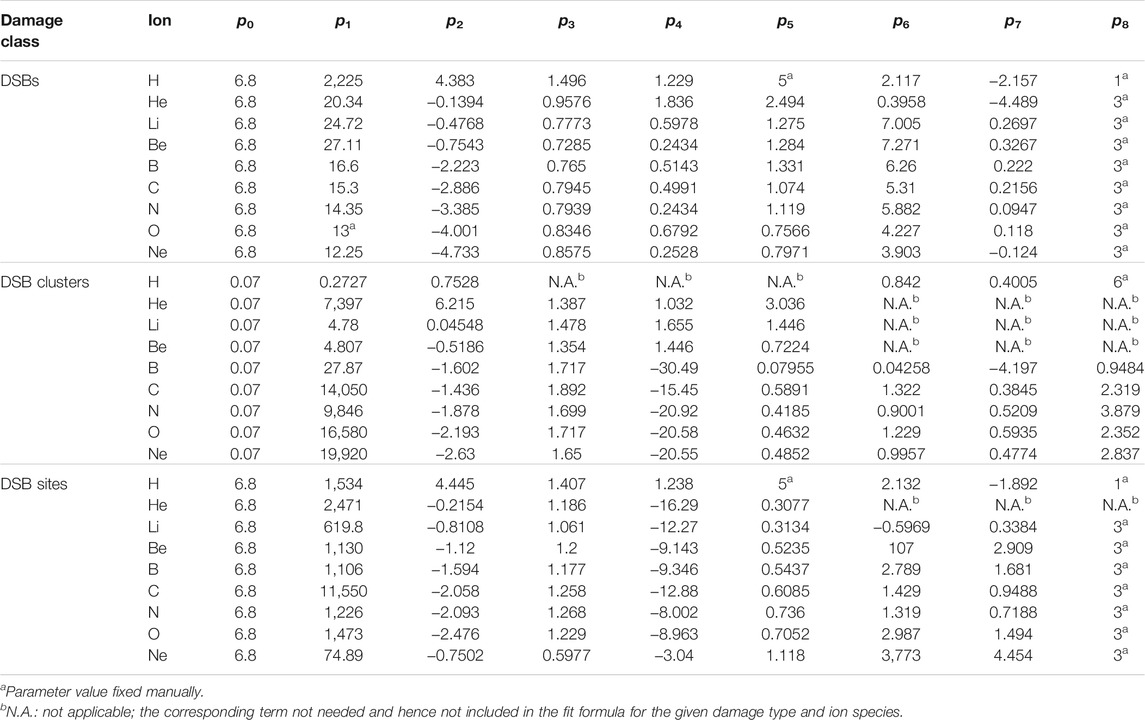
TABLE 2. Parameters of analytical functions (Eq. 2) describing the yields of DSBs, DSB clusters or DSB sites (expressed per Gy per Gbp) as a function of the particle starting energy (in MeV/u).
Estimates of the deposited energy that are not reflected by the present track-structure simulations are shown in Figure 5, using the amorphous track-structure model. At high energies, only a part of the ion LET is actually deposited to the traversed nucleus (black circles). For instance, for 512 MeV/u ions this fraction drops to about 60%, so that the reported PARTRAC simulations can be expected to miss almost 40% of the total energy deposited by the track. However, this missing fraction rapidly decreases with decreasing ion energy, e.g., to 23% for 64 MeV/u tracks, and further diminishes (down to almost a zero fraction) for tracks narrower than the cell nucleus (energies below about 14 MeV/u). To reduce the missing fraction at a given energy, tracks passing in the vicinity of the nucleus would have to be included (coloured circles). However, the source and the simulation region would have to be largely increased, up to mm-sized regions for high-energy ions, with high computational costs of the track-structure simulations. Fortunately, the results of a simple formula, Eq. 4, for dose in the inner track part (black stars) provide a useful approximation of the fraction of energy deposited to the nucleus and hence also for the fraction that is missing in the track-structure simulations of high-energy ions, and can be used in conjunction with Eqs 1 and 2 in a fluence-based coupling strategy (cf. Discussion).
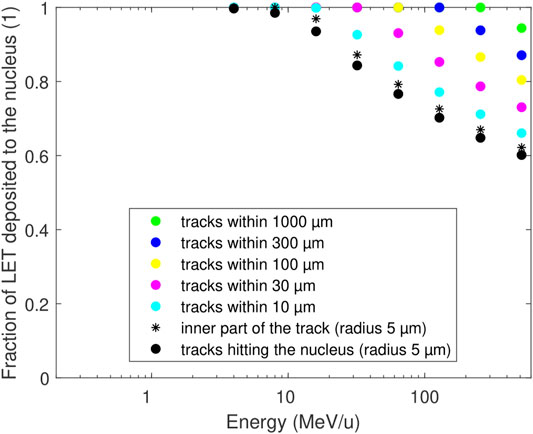
FIGURE 5. Energy deposited to a cell nucleus when only tracks are considered that directly hit the nucleus with their cores (black circles) as in the present track-structure simulations, or including the contribution from all tracks within a larger region (coloured symbols) at a given fluence. The deposited energy is expressed as the fraction of the particle LET (linear energy transfer). The mean deposit per track directly hitting the nucleus (black circles) can be approximated by the energy deposit by the inner part of the track with the same size, Eq. 4 (black stars). Calculations based on the amorphous track structure model.
Discussion
Analytical formulas have been provided, which represent the total yields of DNA double-strand breaks, their clusters, and DSB sites as a function of particle starting energy. It has to be made clear that the aim of this work was neither to provide a theoretical model of DNA damage by ion beams, nor to develop an optimal model to reproduce simulation data in terms of using a strictly limited number of parameters. The aim was solely to reproduce the simulation data in a phenomenological way, but with a high degree of accuracy, to facilitate their use within the framework of a radiation transport code.
Three classes of DNA damage were analyzed, in all cases considering the total damage, i.e., resulting from DNA lesions induced both directly (via direct energy deposition to DNA) and indirectly (mediated by free radicals). We have chosen to include in this study only selected classes of DNA damage, as justified in the following: DSBs are commonly considered as key initial lesions related to biological effects of ionizing radiation. However, even the majority of DSBs can be repaired. Clustered DNA lesions, in particular DSB clusters, likely pose critical challenges to the cellular repair system, and may typically lead to lethal events [34]. When patterns obtained for diverse classes of DNA damage are compared to those observed for cell killing in dependence on particle LET, a remarkably close match is seen for DSB sites [20], suggesting this endpoint is a good indicator of the outcome of the exposure in terms of inactivating cell replication.
The basic mechanistic assumption that underpins the present coupling strategy is that the analyzed DNA damage is additive, i.e., that the yields of the studied damage classes upon a mixed-field irradiation are given by a sum of yields from individual tracks. Due to the local nature of DSBs, their clusters and sites, this assumption is fulfilled up to doses of the order of several hundred Gy [16, 35]: The probability that, e.g., two DSBs induced by different primary particles would combine into a DSB cluster is negligible, since the two DSBs would have to occur within 25 bp. The same argument holds even stronger for a combination of two strand breaks into a DSB, which would have to take place within 10 bp. Note that deviations from additivity (and linearity) appear at much lower doses for larger-scale effects such as fragmentation on Mbp scales or chromosome aberrations, which typically happen over micrometer distances.
It is important to note that the setup used in the track-structure simulations that were performed to obtain the results fitted in this work does not provide electronic equilibrium conditions, both longitudinally and laterally. This is because the ions were started from a source directly adjacent to the cell nucleus and with an area as large as the nucleus section. This setup differs from the typical simulation with a transport code, where, except for the initial build-up region, the equilibrium conditions are fulfilled throughout the volume of interest. Fortunately, the longitudinal difference does not impose a serious limitation on the applicability of the present coupling strategy, at least in the dose-based scheme, as shown by pilot track-structure simulations with gradually increased source-to-nucleus distances [21]: Both the deposited dose and damage yields (as absolute numbers of lesions) are underestimated by present simulations compared with the case of electronic equilibrium, with differences that can reach 20% for high-energy ions. However, these effects almost cancel out in the yields of DSB classes per unit dose, which differ between the two setups by a few percent only. Similarly, also the lateral difference hardly affects the applicability of the dose-based scheme, since considerable fractions of energy deposited by a track are not reflected by the present setup only for high-energy, low-density tracks. The present setup with an ion source not larger than the cross section of a cell nucleus ignores energy deposited to the nucleus by tracks passing not directly through the nucleus but only in its vicinity (up to a few millimeters at the highest energies), i.e., tracks that overlap with the nucleus by their penumbras only. When the per-track dose and yields of DSB classes (fluence-based scheme) are considered, for high-energy ions one should add the contribution from electrons not included in the results reported here but present under the equilibrium conditions. Both longitudinally and laterally, this may correspond to spatial scales of a few millimeters. Laterally, although even high-energy tracks contain a core region with energy deposition density much higher than in the penumbra region (where it drops with the inverse square of the distance from track axis), notable fractions of energy may be deposited in large outer regions of the track. Importantly, energy depositions in the outer region are very sparse, and the resulting DNA damage corresponds to the low-LET limit of the present approach, i.e., to the yields of p0 = 6.8 DSB, 0.07 DSB clusters, and 6.8 DSB sites per Gy per Gbp. Yet the doses and damage yields should be added when using the fluence-based approach for the highest particle energies. Equation 4 used in conjunction with our p0 parameter values provides a first quick means for doing so. Further studies including the full simulation of tracks with PARTRAC, the inclusion of larger track-to-nucleus distances and the derivation of radial dose distribution profiles would offer the possibility of a test of validity and further refinement of this correction using the same approach.
The results presented in this work set the basis of a strategy to couple radiation transport and track-structure simulations, as discussed hereafter. The coupling could be performed in a dose-based or a fluence-based scheme, with the limitation just discussed. The main piece of information that needs to be extracted from the radiation transport code is the particle type and energy distributions at the site of interest. This means characterizing particle types and their energies at the entrance plane tangential to the cell nucleus. In most applications, the radiation field characteristics will hardly vary over several neighbouring cells, so that using a voxel size of ∼10 µm containing a single cell only would be unnecessarily detailed. However, typical voxel sizes in radiation transport codes of ∼1 mm may be too coarse. Finer voxelization may be needed in the regions of spatially highly varying field characteristics, such as narrow Bragg peak regions in active scanning techniques in hadron radiotherapy, or when dealing with short-range alpha particles from internal emitters or boron capture therapies. Compared with simulations of (physical) dose deposition only, this may involve some additional interpolation (or even extrapolation) steps or averaging procedures.
A strategy to couple transport codes with track-structure simulations based on the use of a dose-based formalism would require the explicit calculation of the dose delivered to the target site per particle type with the radiation transport code. Ideally, one should then calculate the dose-weighted distribution of particle energies in the same site, and sum all damage yields corresponding to the different energy bins as calculated with the analytical formula of Eq. 2, to finally obtain the total damage. Another possibility would be to evaluate the first moment of the dose-weighted distribution of particle energy (basically, the energy value at which, on average, a given kind of particle is delivering dose) and apply Eq. 2 to this single energy value. This approximation is conceptually similar to the coupling performed with the application of LET-based formulas using the dose-average LET in the target site as a single LET value for a given species, as done in our previous work [14]. Its validity would have to be tested by dedicated simulations.
Within a fluence-based approach, both the dose to target nuclei (Eq. 1) and DNA damage induced per particle track (Eq. 1 multiplied by Eq. 2) are made available. A strategy based on the use of such an approach would then require the calculation of particle fluence (distribution in energy bins) for all particle species at the entrance of the target site. As discussed above, the full distribution of particle energies could possibly be replaced by a single average value, but also in this case the use of the dose-weighted average seems more suited than the fluence-weighted one. The extent to which the use of average energy values represents a good approximation should be investigated in the specific case. Generally speaking, the use of look-up tables with fluence-to-dose conversion factors is a common solution proposed by, e.g., ICRP publications for radiation protection applications. The fluence-based approach with analytical functions proposed in this work represents an analogous solution. It complements the fluence-to-dose conversion factors with fluence-to-damage ones that can eventually be used to apply a biological “weight” on top of the (physical) absorbed dose.
The present work complements the analysis of our previous study [16], where DNA damage yields were parameterized as a function of particle LET in the cell nucleus. Parameterizing DNA damage in terms of particle energy rather than LET has several advantages: First, particle energy is more readily available from transport codes than LET in the restricted sense as obtained from PARTRAC track-structure simulations, i.e., as energy deposited to the cell nucleus per unit track length. This LET estimate bears a large similarity to the microdosimetric lineal energy, but the availability of microdosimetric quantities is limited to a few transport codes such as PHITS. Second, the use of energy circumvents the issue of the same LET value that appears at two different energies during the particle slowing down. Thus the energy-based parameterization enables using a single mathematical function to describe the particle effectiveness in inducing DNA damage from fast down to stopping particles. Third, not only the most stable isotopes considered in this work but also other ones such as 2H, 3He or 11C are present in mixed fields generated when irradiating, e.g., with a 12C beam. DNA damage induction by diverse isotopes of the same species has not been explicitly addressed by PARTRAC simulations so far. However, one may foresee that, perhaps except for the Bragg peak region (energies below a few MeV/u), electronic interactions are very similar for isotopes with the same atomic number and charge at the same velocity, i.e., at the same energy per nucleon (specific energy). Their LET values, however, differ due to the differing masses. While a dedicated LET-based function depicting the effectiveness in inducing DNA damage would thus have to be used for each isotope, the energy parameterizations presented in this work should be largely generally applicable. Simulations directed at testing this hypothesis shall be performed in the future.
In conclusion, the present results provide a basis for coupling radiation transport codes to PARTRAC track-structure simulations. Such a coupling combines the strengths of both approaches. Transport codes bring the ability to deal with macroscopic scales, and track-structure simulations provide detailed representation of the underpinning mechanisms from energy deposition by radiation to the induction of DNA damage. The selection of the actual coupling strategy via a dose- or fluence-based approach and characterizing the radiation field by energy or LET distributions or weighted mean values depends on the particular transport code and specific situation studied. The provided analytical formulas represent a rather simple solution that opens the way to many future applications, including but not limited to modelling the biological effects of ion beam radiotherapy, proton boron capture therapy and boron neutron capture therapy.
Data Availability Statement
The original contributions presented in the study are included in the article/Supplementary Material, further inquiries can be directed to the corresponding authors.
Author Contributions
PK, WF, AO, and GB designed the research. WF, PK, and GB performed PARTRAC simulations. PK proposed the analytical formulas and performed the fits. PK, WF, and GB interpreted the results. PK and GB drafted the manuscript. All authors critically read and reviewed the manuscript.
Funding
The research leading to these results was supported by institutional funding of the Nuclear Physics Institute CAS, Helmholtz Zentrum München and University of Pavia, and partially by the Czech Science Foundation (project number 21-06451S).
Conflict of Interest
The authors declare that the research was conducted in the absence of any commercial or financial relationships that could be construed as a potential conflict of interest.
Publisher’s Note
All claims expressed in this article are solely those of the authors and do not necessarily represent those of their affiliated organizations, or those of the publisher, the editors and the reviewers. Any product that may be evaluated in this article, or claim that may be made by its manufacturer, is not guaranteed or endorsed by the publisher.
References
1. Battistoni G, Bauer J, Boehlen TT, Cerutti F, Chin MPW, Dos Santos Augusto R, et al. The FLUKA Code: an Accurate Simulation Tool for Particle Therapy. Front Oncol (2016) 6:116. doi:10.3389/fonc.2016.00116
2. Allison J, Amako K, Apostolakis J, Arce P, Asai M, Aso T, et al. Recent Developments in Geant4. Nucl Instr Methods Phys Res Section A: Acc Spectrometers, Detectors Associated Equipment (2016) 835:186–225. doi:10.1016/j.nima.2016.06.125
3. Werner CJ, Bull JS, Solomon CJ, Brown FB, McKinney GW, Rising ME, et al. MCNP Version 6.2 Release Notes. In: MCNP6.2 Release Notes. Los Alamos: Los Alamos National Laboratory, report LA-UR-18-20808 (2018). doi:10.2172/1419730
4. Faddegon B, Ramos-Méndez J, Schuemann J, McNamara A, Shin J, Perl J, et al. The TOPAS Tool for Particle Simulation, a Monte Carlo Simulation Tool for Physics, Biology and Clinical Research. Physica Med (2020) 72:114–21. doi:10.1016/j.ejmp.2020.03.019
5. Sato T, Iwamoto Y, Hashimoto S, Ogawa T, Furuta T, Abe S-i, et al. Features of Particle and Heavy Ion Transport Code System (PHITS) Version 3.02. J Nucl Sci Technol (2018) 55:684–90. doi:10.1080/00223131.2017.1419890
6. Brualla L, Rodriguez M, Lallena AM. Monte Carlo Systems Used for Treatment Planning and Dose Verification. Strahlenther Onkol (2017) 193:243–59. doi:10.1007/s00066-016-1075-8
7. Muraro S, Battistoni G, Kraan AC. Challenges in Monte Carlo Simulations as Clinical and Research Tool in Particle Therapy: a Review. Front Phys (2020) 8:567800. doi:10.3389/fphy.2020.567800
8. Friedland W, Dingfelder M, Kundrát P, Jacob P. Track Structures, DNA Targets and Radiation Effects in the Biophysical Monte Carlo Simulation Code PARTRAC. Mutat Research/Fundamental Mol Mech Mutagenesis (2011) 711:28–40. doi:10.1016/j.mrfmmm.2011.01.003
9. Nikjoo H, Emfietzoglou D, Liamsuwan T, Taleei R, Liljequist D, Uehara S. Radiation Track, DNA Damage and Response-A Review. Rep Prog Phys (2016) 79:116601. doi:10.1088/0034-4885/79/11/116601
10. Dingfelder M. Track-structure Simulations for Charged Particles. Health Phys (2012) 103:590–5. doi:10.1097/HP.0b013e3182621292
11. Hall EJ, Giaccia AJ. Radiobiology for the Radiologist. 8th ed. 9781496335418. Philadelphia: Wolters Kluwer (2019).
12. Friedland W, Kundrát P. Modeling of Radiation Effects in Cells and Tissues. In: Brahme A, editor. Comprehensive Biomedical Physics, Vol. 9. Amsterdam: Elsevier (2014). p. 105–42. doi:10.1016/b978-0-444-53632-7.00906-0
13.Geant4-DNA. Geant4-DNA: Extending the Geant4 Monte Carlo Simulation Toolkit for Radiobiology (2021). Available from: http://geant4-dna.org/(Accessed May 20, 2021).
14. Baiocco G, Barbieri S, Babini G, Morini J, Alloni D, Friedland W, et al. The Origin of Neutron Biological Effectiveness as a Function of Energy. Sci Rep (2016) 6:34033. doi:10.1038/srep34033
15. Baiocco G, Barbieri S, Babini G, Morini J, Friedland W, Kundrát P, et al. At the Physics-Biology Interface: the Neutron Affair. Radiat Prot Dosimetry (2018) 180:278–81. doi:10.1093/rpd/ncx222
16. Kundrát P, Friedland W, Becker J, Eidemüller M, Ottolenghi A, Baiocco G. Analytical Formulas Representing Track-Structure Simulations on DNA Damage Induced by Protons and Light Ions at Radiotherapy-Relevant Energies. Sci Rep (2020) 10:15775. doi:10.1038/s41598-020-72857-z
17. Friedland W, Jacob P, Kundrát P. Stochastic Simulation of DNA Double-Strand Break Repair by Non-homologous End Joining Based on Track Structure Calculations. Radiat Res (2010) 173:677–88. doi:10.1667/RR1965.1
18. Friedland W, Kundrát P. Track Structure Based Modelling of Chromosome Aberrations after Photon and Alpha-Particle Irradiation. Mutat Res (2013) 756:213–23. doi:10.1016/j.mrgentox.2013.06.013
19. Schmitt E, Friedland W, Kundrát P, Dingfelder M, Ottolenghi A. Cross-section Scaling for Track Structure Simulations of Low-Energy Ions in Liquid Water. Radiat Prot Dosimetry (2015) 166:15–8. doi:10.1093/rpd/ncv302
20. Friedland W, Schmitt E, Kundrát P, Dingfelder M, Baiocco G, Barbieri S, et al. Comprehensive Track-Structure Based Evaluation of DNA Damage by Light Ions from Radiotherapy-Relevant Energies Down to Stopping. Sci Rep (2017) 7:45161. doi:10.1038/srep45161
21. Friedland W, Kundrát P, Schmitt E, Becker J, Li W. Modeling DNA Damage by Photons and Light Ions over Energy Ranges Used in Medical Applications. Radiat Prot Dosimetry (2019) 183:84–8. doi:10.1093/rpd/ncy245
22. Friedland W, Kundrát P, Schmitt E. Modelling Proton Bunches Focussed to Submicrometre Scales: Low-LET Radiation Damage in High-LET-like Spatial Structure. Radiat Prot Dosimetry (2015) 166:34–7. doi:10.1093/rpd/ncv146
23. Friedland W, Kundrát P. Chromosome Aberration Model Combining Radiation Tracks, Chromatin Structure, DSB Repair and Chromatin Mobility. Radiat Prot Dosimetry (2015) 166:71–4. doi:10.1093/rpd/ncv174
24. Schmid TE, Friedland W, Greubel C, Girst S, Reindl J, Siebenwirth C, et al. Sub-micrometer 20MeV Protons or 45MeV Lithium Spot Irradiation Enhances Yields of Dicentric Chromosomes Due to Clustering of DNA Double-Strand Breaks. Mutat Res Genet Toxicol Environ Mutagen (2015) 793:30–40. doi:10.1016/j.mrgentox.2015.07.015
25. Friedland W, Kundrát P, Schmitt E, Becker J, Ilicic K, Greubel C, et al. Modeling Studies on Dicentrics Induction after Sub-micrometer Focused Ion Beam Grid Irradiation. Radiat Prot Dosimetry (2019) 183:40–4. doi:10.1093/rpd/ncy266
26. Friedland W, Kundrát P, Jacob P. Track Structure Calculations on Hypothetical Subcellular Targets for the Release of Cell-Killing Signals in Bystander Experiments with Medium Transfer. Radiat Prot Dosimetry (2011) 143:325–9. doi:10.1093/rpd/ncq401
27. Kundrát P, Friedland W. Track Structure Calculations on Intracellular Targets Responsible for Signal Release in Bystander Experiments with Transfer of Irradiated Cell-Conditioned Medium. Int J Radiat Biol (2012) 88:98–102. doi:10.3109/09553002.2011.595874
28. Kundrát P, Friedland W. Non-linear Response of Cells to Signals Leads to Revised Characteristics of Bystander Effects Inferred from Their Modelling. Int J Radiat Biol (2012) 88:743–50. doi:10.3109/09553002.2012.698029
29. Kundrát P, Friedland W. Mechanistic Modelling of Radiation-Induced Bystander Effects. Radiat Prot Dosimetry (2015) 166:148–51. doi:10.1093/rpd/ncv170
30. Kundrát P, Friedland W. Enhanced Release of Primary Signals May Render Intercellular Signalling Ineffective Due to Spatial Aspects. Sci Rep (2016) 6:33214. doi:10.1038/srep33214
31. Friedland W, Schmitt E, Kundrát P, Baiocco G, Ottolenghi A. Track-structure Simulations of Energy Deposition Patterns to Mitochondria and Damage to Their DNA. Int J Radiat Biol (2019) 95:3–11. doi:10.1080/09553002.2018.1450532
32. Elsässer T, Cunrath R, Krämer M, Scholz M. Impact of Track Structure Calculations on Biological Treatment Planning in Ion Radiotherapy. New J Phys (2008) 10:075005. doi:10.1088/1367-2630/10/7/075005
33. Kiefer J, Straaten H. A Model of Ion Track Structure Based on Classical Collision Dynamics (Radiobiology Application). Phys Med Biol (1986) 31:1201–9. doi:10.1088/0031-9155/31/11/002
34. Nickoloff JA, Sharma N, Taylor L. Clustered DNA Double-Strand Breaks: Biological Effects and Relevance to Cancer Radiotherapy. Genes (2020) 11:99. doi:10.3390/genes11010099
Keywords: ionizing radiation, track structure, DNA damage, double strand breaks, clustered damage, light ions, radiation transport codes
Citation: Kundrát P, Friedland W, Ottolenghi A and Baiocco G (2021) Coupling Radiation Transport and Track-Structure Simulations: Strategy Based on Analytical Formulas Representing DNA Damage Yields. Front. Phys. 9:719682. doi: 10.3389/fphy.2021.719682
Received: 02 June 2021; Accepted: 25 August 2021;
Published: 08 September 2021.
Edited by:
Susanna Guatelli, University of Wollongong, AustraliaReviewed by:
Tuba Conka Yildiz, Türkisch-Deutsche Universität, TurkeyMario A. Bernal, State University of Campinas, Brazil
Ngoc Hoang Tran, Centre d’Etudes Nucleaires de Bordeaux Gradignan (CENBG), France
Copyright © 2021 Kundrát, Friedland, Ottolenghi and Baiocco. This is an open-access article distributed under the terms of the Creative Commons Attribution License (CC BY). The use, distribution or reproduction in other forums is permitted, provided the original author(s) and the copyright owner(s) are credited and that the original publication in this journal is cited, in accordance with accepted academic practice. No use, distribution or reproduction is permitted which does not comply with these terms.
*Correspondence: Pavel Kundrát, kundrat@ujf.cas.cz; Giorgio Baiocco, giorgio.baiocco@unipv.it