- 1Section for Biomedical Physics, Department of Radiation Oncology, University Hospital and Medical Faculty, Eberhard Karls University Tübingen, Tübingen, Germany
- 2TheraPanacea, Paris, France
- 3CentraleSupelec, University of Paris-Saclay, Gif-sur-Yvette, France
- 4Department of Radiation Oncology, University Hospital and Medical Faculty, Eberhard Karls University Tübingen, Tübingen, Germany
- 5German Cancer Consortium (DKTK), Partner Site Tübingen, and German Cancer Research Center (DKFZ), Heidelberg, Germany
Purpose: Magnetic resonance imaging guided radiotherapy (MRgRT) allows treatment plan adaptation on the MRI of the day. For dose calculations, a structure-specific bulk relative electron density (RED) overwrite derived from a planning computed tomography (CT) poses as one possible treatment workflow. However, this approach introduces uncertainties due to assignment of mean densities and requires a planning CT. The aim of this study was to investigate the uncertainty of the used patient-specific (PSCT) dose calculation in contrast to the correct calculation on a CT and compare to MR-only workflows using population-based bulk ED (PBCT) and artificial intelligence–based pseudo-CTs (AICT).
Methods: Twenty primary prostate cancer patients treated on the 1.5 T MR-Linac were chosen from the clinical database, based on best visual congruence between the planning CT and daily MRI. CT-based reference dose distribution was compared to different pseudo-CT approaches. 1) For PSCT, mean REDs for the femur, pelvis, sacrum, rectum, bladder, and patient were assigned based on individual mean CT densities. 2) Population-based mean REDs were derived based on 50 previous, independent patients and assigned to the structures for the PBCT approach. 3) An AI model for pseudo-CT generation was trained using end-to-end ensembled self-supervised GANs and used to create AICTs from T2w-MRIs. For comparison, the CT was registered to the MRI, structures rigidly propagated, and treatment plans recalculated. Differences of DVH parameters were analyzed, and dose distributions were compared using gamma analysis.
Results: All approaches were able to reproduce the dose distribution accurately, according to a gamma criterion of 3%/3 mm, with pass rates greater than 98%. Applying a 2%/2 mm criterion, the median gamma pass rates for PSCT, PBCT, and AICT resulted in 98.6%, 98.2%, and 99.0%, respectively. The median differences for PTV D98% resulted in 0.13 Gy for AICT, −0.31 Gy for PBCT, and −0.32 Gy for PSCT. The OAR-related DVH parameter showed similar results between the three investigated methods.
Conclusion: In this study, a detailed analysis of uncertainties of MR-only treatment planning concepts for pelvic MRgRT was performed. Both a PBCT and an AICT approach, which bypass the need for a planning CT, may be considered clinically acceptable while reducing imaging dose and registration issues.
Introduction
Over the last years, magnetic resonance imaging guided adaptive radiotherapy (MRgRT) has been clinically introduced on most treated tumor sites [1–6]. Especially in the abdominal region, the additional soft tissue contrast of the magnetic resonance imaging (MRI) in combination with a daily plan optimization allows treatment improvements with the potential to extreme hypofractionation [7].
The increased imaging capabilities in MRgRT lead to improved annotation accuracy, which in combination with a daily optimization on relative dispositioning and deformation of organs at risk (OAR) might translate to a shrinkage of clinical target volume (CTV) to planning target volume (PTV) margins and may thus result in reduced OAR toxicities [8, 9]. In addition to the daily plan adaptation, sequential on-board imaging without additional radiation dose might allow for visual tumor tracking, accounting for shifts of prostate and seminal vesicles during the daily fraction [10, 11].
Although improved image quality of MRI allows for accurate anatomical and functional information, MRI also shows drawbacks in terms of spatial accuracy and correlation to photon cross-sections [12]. Due to this limited transferability of the proton distribution, imaged with the MRI, toward photon cross-sections, accurate calculation of dose distributions is only possible after postprocessing steps and assumptions. This introduces uncertainties in comparison to a conventional dose calculation on a calibrated computed tomography (CT) scan.
To translate the proton density information of an MRI into the electron density (ED) map required for accurate radiation dose calculation, different methods for MR-only radiotherapy planning (RTP) were recently developed in an offline setting, independent of the time pressure of online MRgRT. The existing concepts for MR-only RTP can be classified into voxel-based, atlas-based, and hybrid approaches [13]. Atlas-based methods use a correlation of the MRI to a database of CT patient data, followed by a deformable image registration. However, even though Chen et al. reported a gamma pass rate (2 mm/2%) of more than 98%, the required calculation time of 30 min does not make this approach suitable for online MRgRT [14]. In a further clinically introduced hybrid-based method, an mDixon MRI sequence was used to separate segments of the MRI into water, fat, and in-phase images [15]. These separated images were used as a basis for a classification algorithm in combination with an atlas for the generation of a pseudo-CT [16]. Recently, with the introduction of AI into clinical radiotherapy, Maspero et al. showed that this classification algorithm in combination with an atlas can be substituted by a deep learning–based cGAN, reducing the reconstruction time to less than 6 s [17]. However, this solution relies on diagnostic MRI quality and seems thus not directly transferable to the MR-Linac workflow. While the mDixon sequence clearly shows water and fat, the visualization of anatomical structures for plan adaptation within MRgRT is primarily carried out for prostate patients using a T2-weighted sequence [18]. Hence, this method would introduce an additional imaging sequence during each daily treatment fraction.
Therefore, the proposed pseudo-CT generation methods are either time-consuming or not adoptable in an MR-only workflow. Here, a fast voxel-based patient-specific relative electron density (RED) overwrite of structures overcomes these challenges and is considered clinically applicable.
In this method, the EDs of manually or semi-automatic annotated structures on the MRI of the day are overwritten with their respective patient- and structure-specific mean RED values, which are normalized to water, derived from a planning CT [19]. However, this additional step of a planning CT introduces additional dose depositions within the patients on a separate time slot. Due to uncertainties in registration resulting from different contrasts and anatomical changes between time points, this step of RED assignment is deemed one of the most critical during online adaptive MRgRT [20].
Therefore, the aim of this work was to develop CT-independent MR-only workflows for MRgRT on pelvic patients and assess their respective uncertainties with respect to a state-of-the-art dose calculation on a planning CT.
Methods
Patient Data and Imaging
All patients included into this study were treated at the University Hospital Tübingen between January 2019 and December 2021 under a phase 2 feasibility trial (NCT04172753) at the 1.5 Tesla MR-Linac (Unity MR-Linac, Elekta AB, Sweden). All patients gave written informed consent.
For all patients, the planning CT and MRI at the 1.5 Tesla MR-Linac were acquired with the same positioning devices. CT images were acquired with a Philips Brilliance BigBore CT scanner (Philips Healthcare, Best, the Netherlands), whereby the imaging parameters for the CT scans were as follows: 120 kV, 920–924 ms exposure time, 113–223 mA tube current, 512 × 512 pixels image size, 2 mm slice thickness, and 1.17 × 1.17 mm2 pixel spacing. Corresponding MRIs for the assessment of dose calculation uncertainty and AICT model generation were acquired using a T2-weighted MRI sequence with the following imaging parameters: echo time (TE) 277.82 ms, repetition time (TR) 1535 ms, acquisition time 116.66 s, flip angle 90°, pixel bandwidth 740 Hz/Px, voxel size 0.83 × 0.83 × 1 mm3, and reconstructed matrix 480 × 480 × 300 mm3.
Focus was put on anatomical congruence between the gold standard (GS) CT and MR-only approaches to ensure comparability. Therefore, from the developed approaches, the unseen database of patients with prostate cancer, treated at the MR-Linac between August 2020 and December 2021, was analyzed on the anatomical correspondence between the CT and MRI. Of this database, a testing dataset of n = 20 was defined based on the best alignment of femoral and pelvic bones, bladder, prostate, rectum, and skin surface between the CT and MRI. The accuracy of congruence was assessed by visual inspection in the treatment planning system (TPS) Monaco (v.5.40.1, Elekta AB, Stockholm, Sweden). Details of patient characteristics and treatment information for the tested dataset are presented in Table 1.
Treatment Plan Generation
MR-only workflows were investigated on their dosimetric impact in comparison to a gold standard (GS) CT-based dose calculation. In the first step, the uncertainty of the current clinical workflow at the Elekta MR-Linac system of assigning REDs from a corresponding patient-specific CT (PSCT) was evaluated as a baseline of clinical practice within MRgRT. In the second step, structure-specific REDs were derived out of a database of previously treated pelvic patients on the MR-Linac system and their corresponding mean densities used for a population-based bulk density (PBCT) approach. Finally, a generative adversarial neural network (GAN) for the generation of artificial intelligence–based pseudo-CTs was developed on the T2-weighted MRIs of the MR-Linac system and used for a predictive AI-based pseudo-CT (AICT) generation on the independent testing dataset.
Patient-Specific CT
In the first approach, the current workflow used at the 1.5 T MR-Linac system was evaluated. In this approach, bulk REDs in pelvis L/R, femur L/R, sacrum, rectum, bladder, and patient contours were assigned the mean REDs associated with these anatomical structures derived from the planning CT of each patient.
Population-Based CT
A population-based approach was developed using n = 50 from testing independent datasets of patients treated at the MR-Linac. Median and interquartile range (IQR) with respect to age were calculated for the database, defining the IQR by the 75th and 25th percentiles. The structure-specific averaged mean REDs were then assigned to the respective structures within the tested MRI. The same structures as for the PSCT approach were used for assignment of population-based REDs.
Artificial Intelligence–Based CT
In collaboration with TheraPanacea (TheraPanacea, Paris, France) a pseudo-CT AI-model was generated using end-to-end ensembled self-supervised GANs endowed with cycle consistency on a to the test patients’ unseen dataset of 42 patient with each one planning CTs and in average 5 T2w-MRIs. A two-phase learning pipeline involving three key steps was deployed: (i) cyclic generative adversarial deep learning–based unsupervised cross-modality image synthesis to generate pseudo-CT priors from MR images, (ii) alignment of CT to the MRI using weak priors via mono-modal multi-metric deformable registration with a combination of intensity-driven and intensity-agnostic metrics to generate paired data, (iii) pseudo-CT generation with the self-paired data using deep generative adversarial networks and image similarity metrics. Multiple networks were trained using different whole-body scans as reference space. Each of them relies on a different random separation between training (80%) and validation (20%) subsets. This developed AI model was then used for the generation of pseudo-CTs of T2w-MRIs of the MR-Linac on 4 Nvidia gtx 2080ti GPUS in parallel.
Dose Calculation
CT-based treatment plans were generated for all tested patients with a prescription of 20 × 3 Gy. All treatment plans consisted of 9–16 beam step-and-shoot IMRT plans on a voxel grid of 3 × 3 × 3 mm3. Dose distributions were calculated with the TPS-supported GPUMCD algorithm on a 1% statistical uncertainty. This clinical algorithm is a GPU-accelerated voxel-based Monte Carlo algorithm which includes the 1.5 T static magnetic field and accurately simulates the Lorentz force on charged electrons [21]. All optimized treatment plans were assessed for correctly calculated dose distribution and applicability with the clinically used secondary dose calculation system [22].
For all three planning approaches, the structures and isocenter position were propagated based on one rigid registration from the reference CT to the MRI.
Treatment plans were then, respectively, recalculated on every pseudo-CT approach using the same plan and beam configuration as on the primary planning CT. To minimize differences in skin surface, for the dose calculation only, the body contour intersection between the MRI and CT was considered relevant and tissue-only visible on one modality neglected [23].
Dosimetric Evaluation
For dosimetric evaluation, differences in the OAR-related dose–volume histogram (DVH) parameter to the planning CT were evaluated for representative DVH parameters according to the PRISM trial [24] presented in Table 2. Target-related DVH parameters were defined based on recommendations of the ICRU 83 [25]. Based on one rigid registration between the CT and the MRI, the reference structures were copied to the different pseudo-CTs and DVH parameters evaluated over the same volumes for all approaches. Differences were evaluated by subtraction of the reference CT-based value from the workflow method.
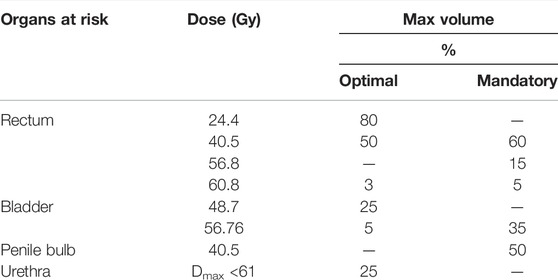
TABLE 2. Chosen OAR-related DVH parameters for the evaluation of PSCT, PBCT, and AICT approaches from the PRISM study.
In addition, a comparison of dose distribution with a gamma analysis was conducted with a global 2%/2 mm, 3%/2 mm, and 3%/3 mm criterion, considering all voxels with a dose value higher than 40% Dmax. The gamma comparison was performed using VeriSoft (v.8.0, PTW Freiburg, Freiburg, Germany).
Statistical testing of DVH parameters was executed with a paired Wilcoxon signed rank test in Python (v.3.8.5). The results were considered significant when p ≤ 0.05. Additionally, the resulting p values were corrected for multiple testing by the Bonferroni correction.
Results
Patient-Specific CT
The comparison of the GS dose calculation on CT in comparison to the PSCT approach showed a median (range) gamma pass rate of 98.6% (96.8–99.6%) for a 2%/2 mm criterion and 99.9% (98.9–100.0%) for a 3%/3 mm criterion. A detailed analysis is presented in Table 3. Within the DVH evaluation, the PSCT workflow reproduced the GS DVH parameter for every target constraint with a maximal median deviation of −0.4 Gy. The D2% values for PTV and CTV were reproduced with a median (range) deviation of −0.35 (−1.00 to 0.52) Gy and −0.38 (−1.02 to 0.58) Gy. The highest median deviation within the OAR parameters was observed for rectum V48.7Gy with 0.43%, and the highest range occurred for the penile bulb structure with −17.55% to 16.65%. A detailed analysis is shown in Table 4.
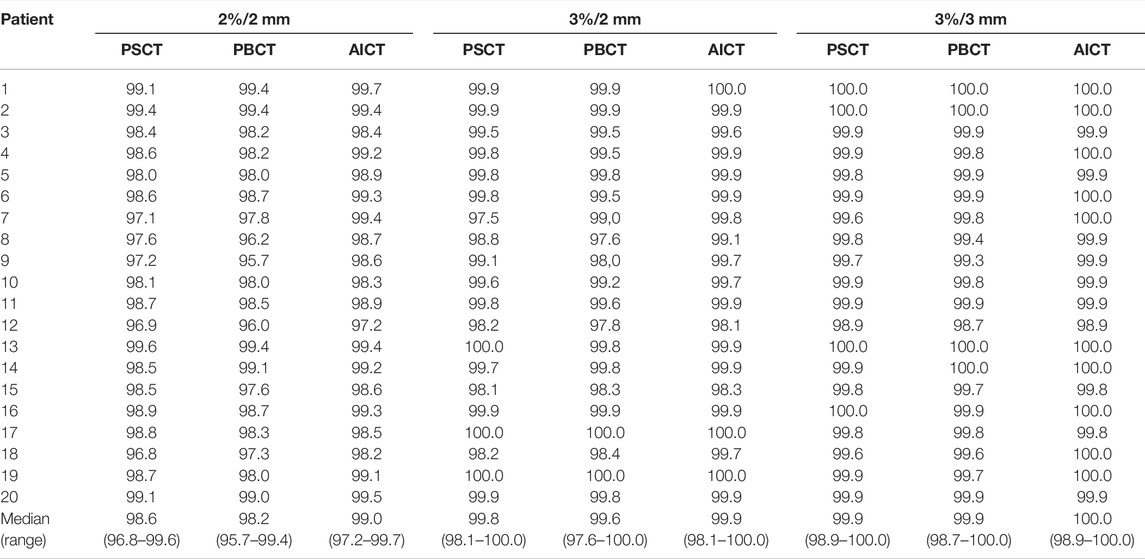
TABLE 3. Comparison of percentage pass rates for PSCT, PBCT, and AICT approaches using a 2 mm/2%, 2 mm/3%, and 3 mm/3% criterion, respectively, with a 40% lower dose threshold of the maximum dose. The bottom row depicts the median (range) pass rate over all patients.
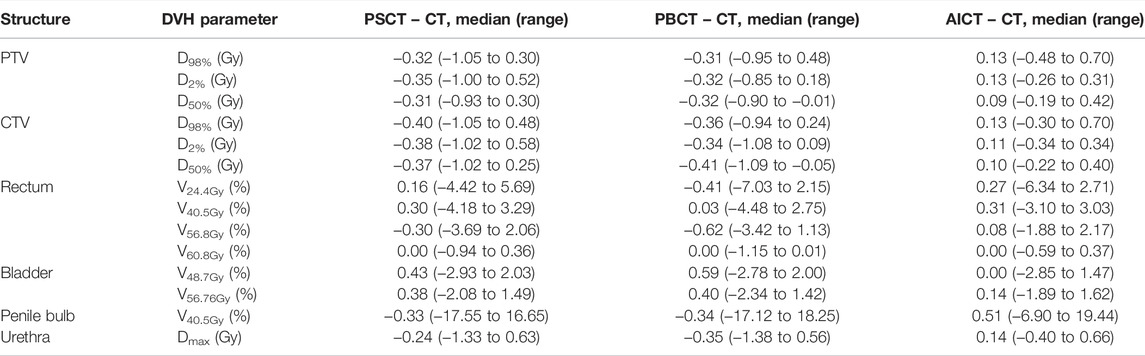
TABLE 4. Results on DVH parameters reporting the median (range) difference for each planning approach toward the gold standard CT-based plan for the considered DVH parameters based on the PRISM study.
Population-Based CT
The evaluated database consisted of 50 patients with a median age of 72 (IQR 62–77). The analysis showed an average mean RED of 1.17/1.17 for the right and left pelvis, 1.22/1.21 for the right and left femur, 1.10 for the sacrum, 0.95 for the rectum, 1.01 for the bladder, and 0.98 for the surrounding patient. Additional information on the distribution is given in Figure 1 and Table 5. The evaluation with a gamma comparison against the GS showed a median (range) pass rate of 98.2% (95.7–99.4%) for the 2%/2 mm criterion and a median (range) pass rate of 99.9% (98.7–100.0%) at the 3%/3 mm criterion. Additional information on the gamma pass rates is given in Table 3.
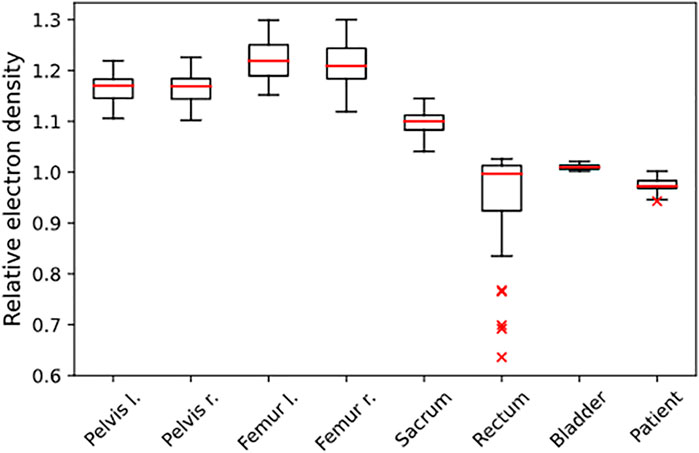
FIGURE 1. Distribution of REDs, determined from the independent population of n = 50 patients. The REDs are normalized to water, and the given structures were used for RED assignment in the PBCT approach.
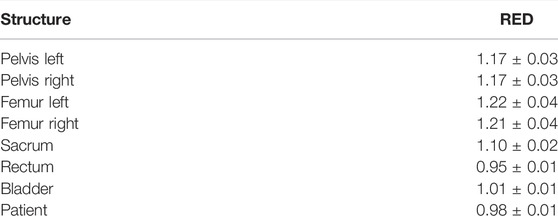
TABLE 5. Mean ± standard deviation of REDs, derived from the independent database of 50 patients for the structures the ED assignment was performed on for the PBCT approach.
The GS dose calculation was reproduced for the evaluated DVH criteria with a maximum median deviation of −0.41 Gy for the target parameters. The D98% for PTV and CTV showed a median (range) deviation of −0.31 (−0.95 to 0.48) Gy and −0.36 (−0.94 to 0.24) Gy, respectively. The highest median deviation to the GS was detected for rectum V56.8Gy with −0.62 (−3.42 to 1.13) Gy. The highest range deviation within the OARs was visible for the penile bulb with 35.37%. In addition to the detailed analysis in Table 4, a visual comparison of the different methods is presented in Figures 2 and 3.
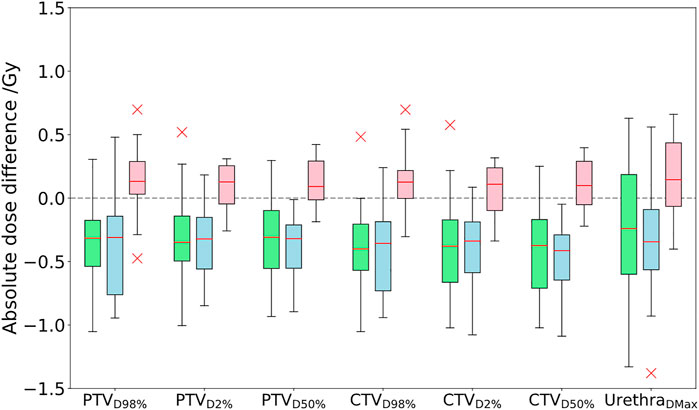
FIGURE 2. Comparison of absolute dose differences toward the GS CT plan for the three investigated approaches PSCT (green), PBCT (blue), and AICT (pink). DVH parameter differences are given by subtraction of the GS from the workflow method.
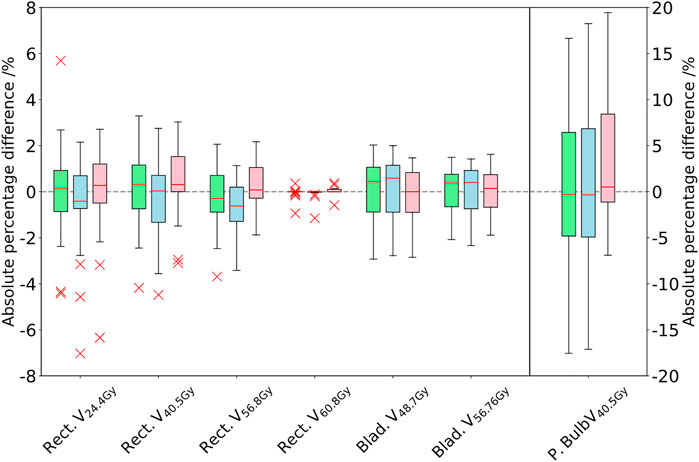
FIGURE 3. Comparison of the DVH parameters for the rectum, bladder, and penile bulb. The difference between the PSCT (green), PBCT (blue), and AICT (pink) approaches toward the gold standard CT is given in absolute percentage points of the structure volume by subtraction of the GS from the workflow method. The boxplot of the penile bulb is scaled on the y-axis of the right side.
The Wilcoxon signed rank test for the DVH evaluation detected no significant difference to the PSCT workflow based on every constraint, aside from the rectum (V24.4Gy: p = 0.011, V40.5Gy: p < 0.001, and V56.8Gy: p = 0.003, cf. Table 6).
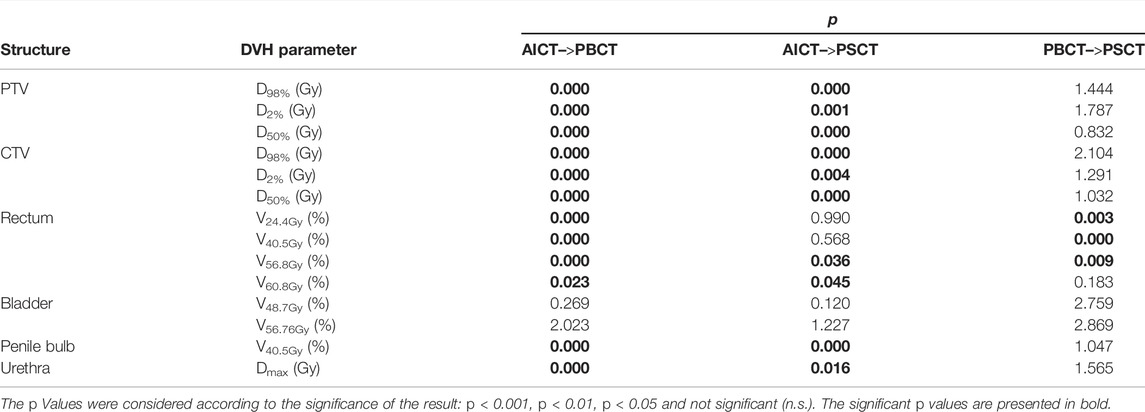
TABLE 6. Results of the Wilcoxon signed rank test for testing each planning approach against the others.
Artificial Intelligence–Based CT
AICTs were generated from the T2w-MRI images in a mean time of 30 s The gamma comparison with the GS dose distribution demonstrated a median (range) gamma pass rate of 99.0% (97.2–99.7%) and 100.0% (98.9–100.0%) for the 2%/2 mm and 3%/3 mm gamma criterion, respectively. A detailed summary of the pass rates is shown in Table 3. In Figure 4, a visual comparison for the case with minimal gamma pass rate of 97.2% at the 2 mm/2% criterion is presented.
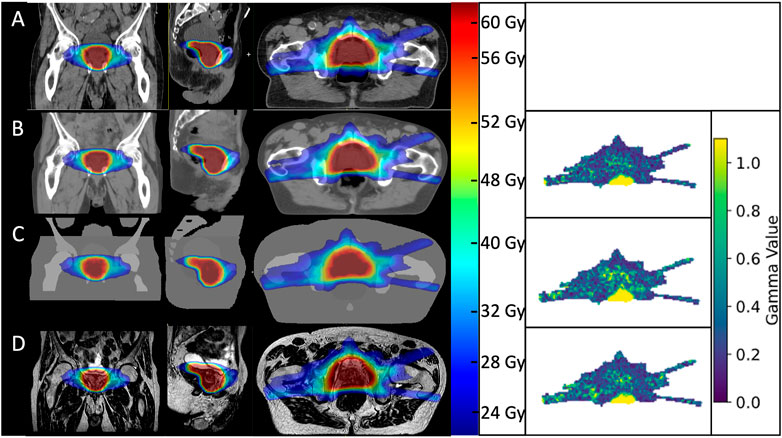
FIGURE 4. Visual comparison of dose distributions between the gold standard CT plan (A), AICT (B), PBCT (C), and PSCT (D) visualized over the used MRI for the patient with the lowest performing gamma pass rates. On the right side, the corresponding gamma maps for a 2 mm/2% criterion.
The DVH-based dosimetric evaluation showed, in comparison to the prior methods, increased target-related DVH parameters, with medians above zero for the absolute dose differences of the calculated dosages on the AICT (cf. Figure 2). All target parameters had a maximal median deviation smaller than 0.15 Gy to the GS, with a maximal median deviation of 0.13 (−0.3 to 0.70) Gy for the CTV D98%. For the OARs, the maximal median deviation was detected on the penile bulb with 0.51% (−6.90 to 19.44%). A detailed analysis is shown in Table 4.
Discussion
The aim of this study was to develop CT-independent MR-only workflows for the online MR-guided radiotherapy and assess the corresponding uncertainties in comparison to a state-of-the-art dose calculation on a CT. All approaches reproduced the GS dose calculation with a minimal gamma criterion of over 98.7% at 3%/3 mm, thus showing clinically acceptable deviation. Within the evaluation of DVH parameters, the AICT reproduced the target-related parameters with the smallest overall uncertainties, and all approaches reproduced the OAR-related parameters with a maximal median deviation of 0.6%.
The evaluation of REDs on an independent database of treated patients, as a basis for the PBCT approach, showed a maximal IQR range of 0.09 RED for the rectum structure due to differences in air and with a mean IQR of 0.036 RED over all other structures, with small deviations between mean RED. The dosimetric evaluation of the PBCT approach showed reasonably comparable agreement toward the GS dose calculation with a minimal median dose agreement of target structures for the ΔCTVD50% = −0.41 Gy (−0.7% for a prescription dose of 60 Gy).While the DVH parameters were comparable between the PSCT and PBCT approaches, detecting no significant differences in 11/14 Wilcoxon signed rank tests (Table 6), the analyzed gamma maps showed median increased pass rate of 98.6% versus 98.2% for the PSCT and PBCT approaches, respectively, at a 2%/2 mm criterion.
In comparison to a steady underestimation of the dose in both bulk density approaches, the dose calculation on the AICT presented an overestimation of parameters with a mean median DVH difference over all target parameters of 0.12 Gy. In the evaluation of rectal DVH constraints, the AICT showed the best median (range) results for the high-dose rectum parameters V60.8Gy = 0.0 (−0.59 to 0.37)] and V56.8Gy = 0.08 (−1.88 to 2.17)]. Within the gamma map evaluation, all three approaches presented a gamma pass rate of over 98% at 2%/2 mm, with a best median agreement over all patients for the AICT (pass rate = 99.0%).
The analyzed dosimetric differences of the PBCT workflow were comparable with the published data by Lambert et al. [26]. The authors investigated a bulk density approach, with a uniform density assignment to all parts of the bone and the resulting tissue as water equivalent. Based on this approach, they reported a mean bulk density for bone of 1.19 g/cm3 and a mean dose point deviation within the target of −1.4%. In contrast, differences in bone density were visible due to different anatomical structures being contoured individually within the MR-Linac workflow, to represent, with structure individual rotations and shift, the daily anatomy as accurately as possible. However, based on the correlation between density and RED incorporated in the TPS and published by Fippel et al., the detected mean RED over all bones of 1.17 translates into a density of 1.20 g/cm3 and replicates the Lambert et al. published values within 0.01 g/cm3 [27].
The main limitation of the PBCT approach is the necessity of a time-intense annotation of body surface, bones, rectum, and bladder. Hence, to eliminate these contouring steps, different groups have reported first concepts and results toward automatic segmentation of anatomical MRIs [28]. An additional drawback is the narrow distribution of patient age with a median value of 72 (IQR: 62–77) years in the database used for bulk density estimation (n = 50) and within the testing cohort of 74.5 (IQR: 71.5–78.25) years. Hence, the bone densities within the cohort are quite similar and do not differ due to age differences, which should result in higher REDs for younger and lower REDs for older patients [29]. However, dosimetric deviations due to the small, expected changes in RED over the course of life have not been investigated in this work and are outside the scope of this work.
A greater potential deviation to the CT-based dose calculation on a CT may result from air gaps in the anatomical region of interest. The mean RED of a dataset of patients represents the most common rectum filling with little amount of air. Therefore, the dose calculation would overestimate REDs on an MRI with a large air cavity inside the rectum, resulting in higher maximum dose values inside the rectum [30].
While this problem arises primarily in the PBCT, for the daily adaptive PSCT workflow, with MRI and CT on different days, that is, during fractionated daily adaptive MRgRT treatments, the same problem is visible and most conventionally counteracted by adjustment of the patient diet, fillings, repositioning, or medication to reduce air within the rectum [31, 32].
The bulk density assigned pseudo-CTs could not take these changes into account. On the contrary, the AICT did not only visualize anatomical regions with air gaps clearly but also showed better agreement for the high-dose rectum DVH parameters with a median rectum deviation of V56.8 Gy = 0.08 Gy. Even though the AICT showed the best agreement with the planning CT on the DVH parameter, the usage of an AI-based pseudo-CT model needs additional quality assurance and testing. In contrast to current commercial MR-only approaches, which are based on a physical detection of air, fat, and bone, the developed fast workflow is directly converting the anatomical T2w-MRI into density information [15]. Therefore, before clinical usage of such a system, limitations on patient eligibility, as for example, with respect to age, missing bone structures, or implants should be considered and an extensive check on the HU executed for each patient.
An advantage of the PBCT approach is that it can be directly used within the TPS, whereas the AICT must be predicted in a separate third-party software, which then has to be imported into the clinical workflow. However, as the currently supported MR-Linac system uses a mean RED assignment of structures in the online workflow, the AICT has the potential to replace a planning CT and therefore eliminate registration uncertainties, additional patient dose, and reduce cost by omitting an extra patient appointment. Furthermore, a sanity check of the AICT by the REDs used for the PBCT approach in the TPS would enable detecting extreme deviations.
This study is limited due to anatomical differences between the MRI and CT, as shown in Figure 4. Within this patient, a small variation of air gap between the planning CT and MRI resulted, for this slice, in a small gamma pass rate inside the rectal cavity. In addition, since we limited the anatomical differences by using an intersection of both modalities as a basis for dose calculation, we limited the information on behalf of the patient outline and corresponding spatial skin deviations. However, as the anatomical MRI sequence at the MR-Linac system is optimized for a high spectral bandwidth per pixel, the patient-induced distortions were considered neglectable. An additional challenge here is the dependence of image distortion as a function of the distance to the MRI isocenter. However, as Snyder et al. presented in their clinical experience on commissioning of the MR-Linac system, a distortion of below 1 mm at a diameter spherical volume of 350 mm was considered [33]. However, bigger patients limit the applicability of an MR-only workflow, when succeeding the field of view or the dimensions of the implemented distortion map of the MRI scanner. In this case, a correction for static magnetic field changes would not be possible. An additional limitation of this study was that we did not explicitly search for patients with extreme anatomical problems such as the highest possible amount of air within the rectum or a very small bladder. We as well did not consider patients with an artificial implant such as hip replacement or missing bony structures. Therefore, dose calculation based on a planning CT should still be performed for patients with unusual, extreme anatomies, which are difficult to model.
In conclusion, we presented in this article two developed approaches for the implementation of an MR-only workflow at the MR-Linac system. Both methods show clinically acceptable uncertainties, whereas the developed AICT supersedes the bulk density approaches in terms of accuracy. However, the PBCT approach could be quickly implemented within a clinical workflow, whereby the implementation of an artificial-based pseudo-CT needs additional quality assurance and extensive robustness testing of the system.
Data Availability Statement
The datasets presented in this article are not readily available due to data protection regulations as material and/or data transfer agreements (MTA/DTA) need to be set up. Requests to access the datasets should be directed to marcel.nachbar@med.uni-tuebingen.de.
Ethics Statement
The studies involving human participants were reviewed and approved by the Ethik-Kommission at the medical faculty of the Eberhard-Karls-Universität and Universitätsklinikum Tübingen, Gartenstraße 47, 72074 Tübingen. The patients/participants provided their written informed consent to participate in this study.
Author Contributions
CG, DZ, and DT were involved in the conception of study and patient selection. MN, IC, and DT created and analyzed the data and drafted the manuscript. KS, TR, and NP developed the AI model and supported in data analysis. All authors provided critical proofreading of the manuscript.
Funding
The research leading to these results was funded by the German Council (DFG, grant no. ZI 736/2-1), the Medical Faculty, the University Hospital of Tübingen, and the European Union’s Horizon 2020 research and innovation program under grant agreement no. 880314. We acknowledge support by the Open Access Publishing Fund of the University of Tübingen.
Conflict of Interest
Authors KS, TR and NP were employed by the company TheraPanacea.
The remaining authors declare that the research was conducted in the absence of any commercial or financial relationships that could be construed as a potential conflict of interest.
The Department of Radiation Oncology Tübingen receives financial and technical support by Elekta, Philips, Siemens, Kaiku Health, TheraPanacea, PTW and ITV in the context of research cooperations.
Publisher’s Note
All claims expressed in this article are solely those of the authors and do not necessarily represent those of their affiliated organizations or those of the publisher, the editors, and the reviewers. Any product that may be evaluated in this article, or claim that may be made by its manufacturer, is not guaranteed or endorsed by the publisher.
References
1. Nachbar M, Mönnich D, Boeke S, Gani C, Weidner N, Heinrich V, et al. Partial Breast Irradiation with the 1.5 T MR-Linac: First Patient Treatment and Analysis of Electron Return and Stream Effects. Radiother Oncol (2020) 145:30–5. Epub 2019/12/25. doi:10.1016/j.radonc.2019.11.025
2. Bertelsen AS, Schytte T, Møller PK, Mahmood F, Riis HL, Gottlieb KL, et al. First Clinical Experiences with a High Field 1.5 T Mr Linac. Acta Oncologica (2019) 58(10):1352–7. Epub 2019/06/27. doi:10.1080/0284186x.2019.1627417
3. Tetar SU, Bruynzeel AME, Lagerwaard FJ, Slotman BJ, Bohoudi O, Palacios MAClinical Implementation of Magnetic Resonance Imaging Guided Adaptive Radiotherapy for Localized Prostate Cancer. Phys Imaging Radiat Oncol (2019) 9:69–76. doi:10.1016/j.phro.2019.02.002
4. Klüter STechnical Design and Concept of a 0.35 T Mr-Linac. Clin Translational Radiat Oncol (2019) 18:98–101. Epub 2019/07/26. doi:10.1016/j.ctro.2019.04.007
5. Lagendijk JJW, Raaymakers BW, Raaijmakers AJE, Overweg J, Brown KJ, Kerkhof EM, et al. Mri/Linac Integration. Radiother Oncol (2008) 86(1):25–9. Epub 2007/11/21. doi:10.1016/j.radonc.2007.10.034
6. Gani C, Boeke S, McNair H, Ehlers J, Nachbar M, Mönnich D, et al. Marker-Less Online Mr-Guided Stereotactic Body Radiotherapy of Liver Metastases at a 1.5 T Mr-Linac - Feasibility, Workflow Data and Patient Acceptance. Clin Translational Radiat Oncol (2021) 26:55–61. Epub 20201130. doi:10.1016/j.ctro.2020.11.014
7. Corradini S, Alongi F, Andratschke N, Belka C, Boldrini L, Cellini F, et al. Mr-Guidance in Clinical Reality: Current Treatment Challenges and Future Perspectives. Radiat Oncol (2019) 14(1):92. doi:10.1186/s13014-019-1308-y
8. Murray J, Tree ACProstate Cancer - Advantages and Disadvantages of Mr-Guided Rt. Clin Translational Radiat Oncol (2019) 18:68–73. Epub 20190401. doi:10.1016/j.ctro.2019.03.006
9. Christiansen RL, Dysager L, Hansen CR, Jensen HR, Schytte T, Nyborg CJ, et al. Online Adaptive Radiotherapy Potentially Reduces Toxicity for High-Risk Prostate Cancer Treatment. Radiother Oncol (2022) 167:165–71. Epub 20211216. doi:10.1016/j.radonc.2021.12.013
10. de Muinck Keizer DM, Pathmanathan AU, Andreychenko A, Kerkmeijer LGW, van der Voort van Zyp JRN, Tree AC, et al. Fiducial Marker Based Intra-fraction Motion Assessment on Cine-Mr for Mr-Linac Treatment of Prostate Cancer. Phys Med Biol (2019) 64(7):07NT02. Epub 2019/02/23. doi:10.1088/1361-6560/ab09a6)
11. Muinck Keizer DM, Willigenburg T, der Voort van Zyp JRN, Raaymakers BW, Lagendijk JJW, Boer JCJSeminal Vesicle Intrafraction Motion during the Delivery of Radiotherapy Sessions on a 1.5 T Mr-Linac. Radiother Oncol (2021) 162:162–9. doi:10.1016/j.radonc.2021.07.014
12. Fransson A, Andreo P, Pötter RAspects of Mr Image Distortions in Radiotherapy Treatment Planning. Strahlenther Onkol (2001) 177(2):59–73. doi:10.1007/PL00002385
13. Edmund JM, Nyholm TA Review of Substitute Ct Generation for Mri-Only Radiation Therapy. Radiat Oncol (2017) 12(1):28. Epub 20170126. doi:10.1186/s13014-016-0747-y
14. Chen S, Quan H, Qin A, Yee S, Yan DMr Image-Based Synthetic Ct for Imrt Prostate Treatment Planning and Cbct Image-Guided Localization. J Appl Clin Med Phys (2016) 17(3):236–45. Epub 20160508. doi:10.1120/jacmp.v17i3.6065
15. Tyagi N, Fontenla S, Zhang J, Cloutier M, Kadbi M, Mechalakos J, et al. Dosimetric and Workflow Evaluation of First Commercial Synthetic Ct Software for Clinical Use in Pelvis. Phys Med Biol (2017) 62(8):2961–75. Epub 20161216. doi:10.1088/1361-6560/aa5452
16. Tyagi N, Fontenla S, Zelefsky M, Chong-Ton M, Ostergren K, Shah N, et al. Clinical Workflow for Mr-Only Simulation and Planning in Prostate. Radiat Oncol (2017) 12(1):119. doi:10.1186/s13014-017-0854-4
17. Maspero M, Savenije MHF, Dinkla AM, Seevinck PR, Intven MPW, Jurgenliemk-Schulz IM, et al. Dose Evaluation of Fast Synthetic-Ct Generation Using a Generative Adversarial Network for General Pelvis Mr-Only Radiotherapy. Phys Med Biol (2018) 63(18):185001. doi:10.1088/1361-6560/aada6d
18. Yang J, Vedam S, Lee B, Castillo P, Sobremonte A, Hughes N, et al. Online Adaptive Planning for Prostate Stereotactic Body Radiotherapy Using a 1.5 Tesla Magnetic Resonance Imaging-Guided Linear Accelerator. Phys Imaging Radiat Oncol (2021) 17:20–4. doi:10.1016/j.phro.2020.12.001
19. Winkel D, Bol GH, Kroon PS, van Asselen B, Hackett SS, Werensteijn-Honingh AM, et al. Adaptive Radiotherapy: The Elekta Unity Mr-Linac Concept. Clin Translational Radiat Oncol (2019) 18:54–9. Epub 2019/07/26. doi:10.1016/j.ctro.2019.04.001
20. Klüter S, Schrenk O, Renkamp CK, Gliessmann S, Kress M, Debus J, et al. A Practical Implementation of Risk Management for the Clinical Introduction of Online Adaptive Magnetic Resonance-Guided Radiotherapy. Phys Imaging Radiat Oncol (2021) 17:53–7. doi:10.1016/j.phro.2020.12.005
21. Hissoiny S, Ozell B, Bouchard H, Després PGpumcd: A New Gpu-Oriented Monte Carlo Dose Calculation Platform. Med Phys (2011) 38(2):754–64. doi:10.1118/1.3539725
22. Nachbar M, Mönnich D, Dohm O, Friedlein M, Zips D, Thorwarth DAutomatic 3d Monte-Carlo-Based Secondary Dose Calculation for Online Verification of 1.5 T Magnetic Resonance Imaging Guided Radiotherapy. Phys Imaging Radiat Oncol (2021) 19:6–12. doi:10.1016/j.phro.2021.05.002
23. Dinkla AM, Florkow MC, Maspero M, Savenije MHF, Zijlstra F, Doornaert PAH, et al. Dosimetric Evaluation of Synthetic CT for Head and Neck Radiotherapy Generated by a Patch‐based Three‐dimensional Convolutional Neural Network. Med Phys (2019) 46(9):4095–104. doi:10.1002/mp.13663
24. Dunlop A, Mitchell A, Tree A, Barnes H, Bower L, Chick J, et al. Daily Adaptive Radiotherapy for Patients with Prostate Cancer Using a High Field Mr-Linac: Initial Clinical Experiences and Assessment of Delivered Doses Compared to a C-Arm Linac. Clin Translational Radiat Oncol (2020) 23:35–42. doi:10.1016/j.ctro.2020.04.011
25.ICRUIcru Report Vol. 83. In: International Commission on Radiation Units and Measurements. Prescribing, Recording, and Reporting Photon-Beam Intensity-Modulated Radiation Therapy. Bethesda: Imrt (2010).
26. Lambert J, Greer PB, Menk F, Patterson J, Parker J, Dahl K, et al. Mri-Guided Prostate Radiation Therapy Planning: Investigation of Dosimetric Accuracy of Mri-Based Dose Planning. Radiother Oncol (2011) 98(3):330–4. doi:10.1016/j.radonc.2011.01.012
27. Fippel MFast Monte Carlo Dose Calculation for Photon Beams Based on the Vmc Electron Algorithm. Med Phys (1999) 26(8):1466–75. doi:10.1118/1.598676
28. Savenije MHF, Maspero M, Sikkes GG, van der Voort van Zyp JRN, T. J. Kotte AN, Bol GH, et al. Clinical Implementation of Mri-Based Organs-At-Risk Auto-Segmentation with Convolutional Networks for Prostate Radiotherapy. Radiat Oncol (2020) 15(1):104. Epub 20200511. doi:10.1186/s13014-020-01528-0
29. Telfer S, Brunnquell CL, Allen JD, Linnau KF, Zamora D, Kleweno CPThe Effect of Age and Sex on Pelvic Bone Density Measured Opportunistically in Clinical Ct Scans. J Orthop Res (2021) 39(3):485–92. doi:10.1002/jor.24792
30. Godoy Scripes P, Subashi E, Burleson S, Liang J, Romesser P, Crane C, et al. Impact of Varying Air Cavity on Planning Dosimetry for Rectum Patients Treated on a 1.5 T Hybrid MR‐linac System. J Appl Clin Med Phys (2020) 21(7):144–52. Epub 20200523. doi:10.1002/acm2.12903
31. Schaefer C, Zamboglou C, Volegova-Neher N, Martini C, Nicolay NH, Schmidt-Hegemann N-S, et al. Impact of a Low FODMAP Diet on the Amount of Rectal Gas and Rectal Volume during Radiotherapy in Patients with Prostate Cancer - a Prospective Pilot Study. Radiat Oncol (2020) 15(1):27. doi:10.1186/s13014-020-1474-y
32. Gani C, Lo Russo M, Boeke S, Wegener D, Gatidis S, Butzer S, et al. A Novel Approach for Radiotherapy Dose Escalation in Rectal Cancer Using Online Mr-Guidance and Rectal Ultrasound Gel Filling - Rationale and First in Human. Radiother Oncol (2021) 164:37–42. Epub 20210914. doi:10.1016/j.radonc.2021.09.002
Keywords: MRgRT, synthetic CT, artificial intelligence, MR-only, MR-Linac, bulk density, uncertainty
Citation: Coric I, Shreshtha K, Roque T, Paragios N, Gani C, Zips D, Thorwarth D and Nachbar M (2022) Dosimetric Evaluation of Dose Calculation Uncertainties for MR-Only Approaches in Prostate MR-Guided Radiotherapy. Front. Phys. 10:897710. doi: 10.3389/fphy.2022.897710
Received: 16 March 2022; Accepted: 21 June 2022;
Published: 12 August 2022.
Edited by:
Urszula Jelen, Genesis Care, AustraliaReviewed by:
James Chow, University of Toronto, CanadaShouliang Ding, Sun Yat-sen University Cancer Center (SYSUCC), China
Copyright © 2022 Coric, Shreshtha, Roque, Paragios, Gani, Zips, Thorwarth and Nachbar. This is an open-access article distributed under the terms of the Creative Commons Attribution License (CC BY). The use, distribution or reproduction in other forums is permitted, provided the original author(s) and the copyright owner(s) are credited and that the original publication in this journal is cited, in accordance with accepted academic practice. No use, distribution or reproduction is permitted which does not comply with these terms.
*Correspondence: Marcel Nachbar, TWFyY2VsLk5hY2hiYXJAbWVkLnVuaS10dWViaW5nZW4uZGU=