- 1School of Mathematics and Statistics, North China University of Water Resources and Electric Power, Zhengzhou, China
- 2School of Management and Economics, North China University of Water Resources and Electric Power, Zhengzhou, China
Exploring the mechanism of tacit knowledge dissemination is the basis of tacit knowledge management. This paper explores the mechanism of tacit knowledge dissemination in Newman-Watts small-world networks using the SIR model. The introduction of diffusion in the traditional diffusion model does not change the stability of the system. Therefore, It cannot explain the dissemination process. We replace the diffusion term with the adjacency matrix of the network to achieve dissemination. The results indicate that the diffusion of tacit knowledge subjects and the entropy of the network (NSE) play a crucial role in generating periodic and local dissemination behaviors. This model can guide significance for effectively accelerating tacit knowledge dissemination and improving the core competitiveness of the enterprise.
1 Introduction
Tacit knowledge management is an integral part of enterprise knowledge management [1]. It is a core element of tacit knowledge management to make tacit knowledge spread rapidly and widely in enterprise alliance [2]. Most of the researches on tacit knowledge dissemination have focused on the influencing factors. They are distributed at different levels of individuals, organizations and teams, as well as in two different dimensions of facilitation and inhibition [3]. The tacit knowledge dissemination system is a nonlinear and complex system [4]. Strong nonlinear dynamics characteristics are reflected in the internal movement of the system, subjects multilevel, the knowledge complexity and knowledge diffusion environment complexity [5]. At the same time, the tacit knowledge dissemination by different subjects has different effects on the system dynamics. Exploring the dynamics of tacit knowledge dissemination is the basis for analyzing the factors affecting its dissemination. Due to the characteristics of tacit knowledge and the dependence of dissemination process on social relations [6], it is necessary to study the network dynamics behavior of the tacit knowledge dissemination system.
The tacit knowledge dissemination model is usually based on the classical SIR model. Kerssens and Cooke introduced the SIR model to describe the internal dissemination mechanism of knowledge [7]. The SIR model is also used in various fields, such as disease communication [8], information communication [9] and knowledge communication [10]. Del Valle Rafo et al. summarized how to model the spread of diseases in social networks using mean-field models[11]. Bajiya et al. used graph theory techniques and Lyapunov functions to establish the global stability of disease-free equilibrium points and endemic equilibrium points [12]. By constructing an interactive model of disease transmission and information transmission, Zhan et al. illustrated that complex network transmission and information transmission processes are highly correlated[13]. The system dynamics model [14] and knowledge dissemination model [15] and fluid dynamics model [16] have made significant contributions to study tacit knowledge dissemination. The above researches reveal the law of tacit knowledge dissemination in organizations. They provide a better theoretical basis for the knowledge management practice of enterprises. However, due to the characteristics of tacit knowledge and the dependence of dissemination process on social relationship, we will introduce social networks into the tacit knowledge dissemination model.
The studies of tacit knowledge dissemination from network perspective are increasing. Some studies analyze the thresholds and influencing factors of knowledge dissemination by extending the SIR model and combining it with complex network theory [17,18]. Liu et al. proposed that knowledge dissemination model driven by interaction frequency in social networks. The model have faster than traditional models [19]. The epidemic transmission models are commonly used to describe knowledge dissemination processes. However, forgetting mechanism is an essential element affecting tacit knowledge dissemination [20]. The forgetting mechanism is introduced into the SIR model to analyze the presence of a threshold for rumor propagation [21]. Cao et al. conclude that better cultural climate increases the knowledge enriched population combined the forgetting hierarchy [22]. The influence of network structure on tacit knowledge dissemination cannot be ignored [23]. Wang et al. studied the relationship between network structure and knowledge dissemination [24,25].
The tacit knowledge dissemination system is a diffusion system. Diffusion system application covers many disciplines, such as, predator-prey models of ecosystems [26], the spread of infectious diseases [27]. In addition, the absorptive capacity of tacit knowledge subjects affects tacit knowledge dissemination efficiency. Wei constructed networks from the interpersonal construct level, and found that the level of absorptive capacity affects the choice of network type [28]. Referring to [29], the absorption capacity of the subjects is determined to be less than 0.5 in this study. The above literatures have discussed factors influencing knowledge dissemination from different levels. How each factor and the tacit knowledge subjects diffusion affect the efficiency of knowledge dissemination will be our concern.
This study constructs a tacit knowledge dissemination model of network organization with SIR model and tacit knowledge subjects diffusion network. The effects of network structure and knowledge subjects diffusion are investigated through network dynamics analysis. The study explains internal dynamic mechanism of tacit knowledge dissemination and management mechanism.
2 The model of tacit knowledge dissemination
The employees of enterprise alliance can be divided into three categories according to whether they have tacit knowledge, i.e., knowledge learners, knowledge disseminators and knowledge immunes. According to the theory of SIR model, knowledge disseminators are identical to infected people carrying viruses; knowledge learners are equivalent to susceptible people; knowledge immunes are equal to infected people who recover health after treatment and removal. We set that each person has the same ability to spread knowledge. Knowledge dissemination occurs between knowledge learners and tacit knowledge disseminators in the process of work and learning. Generally, the employees of enterprise alliance will be stable under non-special circumstances. In the meanwhile, the competition among enterprises may lead to the loss of employees and their transfer. Tacit knowledge has the risk of degradation. It is necessary to fully explore employees tacit knowledge to be transferred to other employees, and then continuously circulated and flowed, and then rise to the tacit knowledge of the whole organizational structure layer. At the same time, due to forgetting mechanism, knowledge disseminators receive tacit knowledge and forget it. Because they do not apply it in time in work and study, or it has no value. The forgetting factor needs to be considered in the process of tacit knowledge dissemination. According to the basic assumptions of the tacit knowledge dissemination model, the six assumptions are given as follows.
Hypothesis 1: Firstly, we consider three types of individual in the enterprise alliance. A kind of individual who do not have some tacit knowledge is called knowledge learners. It assumes that the number of such individual is S(t). The second type of individual who have some tacit knowledge is called knowledge disseminators. This type of individual is the main body of disseminating tacit knowledge. It assumes that the number of disseminators is I(t). The third type of individual is knowledge immunes. Such individual possess tacit knowledge. However, they consider tacit knowledge is useless for their work. On the other hand, they are not interested in the tacit knowledge and do not disseminate it anymore. It assumes that the number of knowledge immunes is R(t).
Hypothesis 2: The total number of individual in enterprise alliance is N at time t. The time is measured in days.
Hypothesis 3: We consider the transfer rate and resignation rate of employees in the enterprise alliance. It assumes that the transfer rate of new employees is μ. The resignation rate of employees is β. We believe that the transferred new employees do not have tacit knowledge.
Hypothesis 4: When knowledge learners come into contact with knowledge disseminators, knowledge learners may be potentially influenced and transformed into knowledge disseminators with the possibility of η.
Hypothesis 5: Since the disseminators may think that knowledge dissemination does more disadvantage than advantage or is not interest in knowledge, they stop spreading tacit knowledge. Disseminators may be transformed into immunes with the possibility of γ.
Hypothesis 6: With the evolution of time, tacit knowledge may be forgotten by the knowledge subject. Due to forgetting, the disseminator may transform into immunes with the possibility of δ.
With the above assumptions, the schematic diagram of tacit knowledge dissemination is shown in Figure 1.
The model can be represent as Eq. 1.
To find the equilibrium point of the above model, we make the right-hand side of the equation equal to zero. We can get two equilibrium points of the equation
Meanwhile, the basic reproduction number R0 as an important parameter in the initial stage of tacit knowledge dissemination. From system Eq. 1, we get
From the equilibrium point, we know that the number of disseminators is zero at P1. If the system stabilizes at P1 as time evolves, the dissemination of tacit knowledge will disappear. Knowledge dissemination occurs if the system stabilizes at P2. Therefore, we can obtain the following theorem.
Theorem 1: The equilibrium point P1 of system Eq. 1 is stable when μη ≤ β2 + βγ + βδ, then the dissemination of tacit knowledge disappears. The equilibrium point P2 of system Eq. 1 is stable when μη ≥ β2 + βγ + βδ, then the dissemination of tacit knowledge exists.
Proof: Jacobian matrix of system Eq. 1 at the equilibrium point is
The characteristic equation at the equilibrium point is. A = |B− λE| =(−β − λ)[(−β − ηI∗ − λ) (ηS∗ − β − γ − δ − λ) + η2S∗I∗] = (λ + β)[(λ + β + ηI∗) (λ − ηS∗ + β + γ + δ) + η2S∗I∗] = 0.
For P1, there is.
For P2, there is. A (P2) = (−β − λ)[(−β − ηI∗ − λ) (ηS∗ − β − γ − δ − λ) + η2S∗I∗] = 0.
According to the Routh-Hurwitz theorem [30], system Eq. 1 is stable at P1 when μη ≤ β2 + βγ + βδ. Therefore, tacit knowledge dissemination will not take place. System Eq. 1 is stable at P2 if μη ≥ β2 + βγ + βδ. The tacit knowledge will spread steadily.
Theorem 2: When μη ≥ β2 + βγ + βδ, the stable number of knowledge disseminators I* is an increasing function of the employee transfer rate μ and the tacit knowledge dissemination rate η.
Proof: When μη ≥ β2 + βγ + βδ.
Then, I∗ is an increasing function of μ and η.
Theorem 1 gives the parameter conditions for the stable dissemination of tacit knowledge in a enterprise alliance. Theorem 2 illustrates that the number of knowledge disseminators can be adjusted by increasing or decreasing μ or η when the resignation rate of employees satisfies certain conditions.
The above theorems give the parameter conditions for the dissemination of tacit knowledge. The tacit knowledge has the characteristics of complexity and implicit[31]. It is not transmitted through data, but through employees contact. Therefore, the contact of knowledge subjects plays an important role in accelerating the spread of tacit knowledge. Therefore, we consider introducing diffusion into the model. Currently, considering the diffusion process of knowledge learners and knowledge disseminators, system Eq. 1 can be written as
Where d1 and d2 are diffusion coefficients. d1 > 0, d2 > 0.
Theorem 3: The addition of the diffusion does not change the stability of the system when the equilibrium point of the system is stable.
Proof: The Jacobian matrix for system Eq. 3 can be written as
First, P1 and P2 are stable for system Eq. 1.
The characteristic equation of system Eq. 3 at the equilibrium point is A = |B− λE| =(−β − λ)[(−β − ηI∗ − λ − d1k2) (ηS∗ − β − γ − δ − λ − d2k2) + η2S∗I∗] = 0.
For the P1,
System Eq. 3 is stable.
For the P2,
By the Routh-Hurwitz theorem, system Eq. 3 is stable.
Therefore, Theorem 3 illustrates that diffusion does not change the stability of the system for the traditional model. Therefore, it cannot reveal the mechanism of tacit knowledge diffusion. In the following, we consider the mechanism of tacit knowledge dissemination in NW networks.
3 The model of tacit knowledge dissemination in network
3.1 Network construction
The traditional diffusion theory can no longer explain the effect of diffusion of employee on the spread of tacit knowledge. We try to construct the tacit knowledge dissemination model on the complex network. In a social network, the friends of employees in a enterprise alliance are colleagues who live together or work in the same company. On the other hand, there may be multinational companies in the alliance. The friends of employees are located in a foreign country. With the popularity network technology, they can communicate remotely though the internet. The enterprise alliance shows a typical small-world nature. Remote communication by the internet corresponds to the remote connection generated by increasing the connected edges in the NW model. The subjects of tacit knowledge dissemination in enterprise alliance are employees. It is very appropriate to use NW networks to simulate the relationship among employees of enterprise alliance. Therefore, we can construct tacit knowledge dissemination model in the NW network to explore the dissemination of tacit knowledge.
Based on small characteristic path length and significant clustering coefficient of the NW network, Newman and Watts further proposed that a critical value of characteristic path length occurs, i.e. it possesses a continuous phase transition in the limit where the density of shortcuts tends to zero [32]. NW network starts from a ring with N nodes [33]. Each node represents an employee in the enterprise alliance. It only influences its connected nodes. An edge is added between a randomly selected pair of nodes with probability p. No self-loop or bound edge is allowed. The NW network is obtained by establishing new connections to unbounded nodes without changing the original relationship of nodes, or introducing shortcuts to strengthen connections between networks.
Figure 2 shows the network of 100 nodes of 5 enterprises with different reconnection probabilities p. The probability p represents the probability of adding an edge between any two nodes which do not have adjacent edges. The p can be viewed as the probability of communication among employees who do not know each other. When p is small, the corresponding network is sparse by the definition of network density
3.2 Tacit knowledge dissemination in NW network
The dissemination behavior of tacit knowledge is related to the dynamical behavior of SIR model and the NW network. We combine the system dynamics of the SIR model and the NW network to study the tacit knowledge dissemination. We can get a symmetric matrix showing the employees communication in the enterprise alliance and derive a new model.
Where A = (Aij) denotes the adjacency matrix. The eigenvalues of A may be positive, negative, or zero due to the coupling of the network nodes.
The stability of system Eq. 5 is closely related to the eigenvalues of the adjacency matrix. Now, we discuss the stability of tacit knowledge dissemination system. The Jacobian matrix of system Eq. 5 at the zero-equilibrium point is
Where Λi denotes the eigenvalues of the adjacency matrix A. The eigenvalues of the system can be written as
According to stability theory [34], it is known that system Eq. 5 is unstable when d1Λi > β or
Sometimes it is difficult to obtain the eigenvalues. We try to simplify tacit knowledge dissemination the model of network organization by introducing the maximum of the eigenvalues of the adjacency matrix. System Eq. 7 can be rewritten as
Where Λmax = max{Λi, i = 1, 2, 3, ⋅⋅⋅, N}, and
As long as there is an eigenvalue greater than zero, the zero-equilibrium point of system Eq. 8 will become unstable. I∗ > 0 when the system is unstable at the zero-equilibrium point. Then, tacit knowledge will spread. The maximum eigenvalue of the adjacency matrix plays a crucial role in tacit knowledge dissemination system. To study the rich dynamical behavior of the system, the following two Lemma are introduced:
Lemma 1[35]: For an n-dimensional linear system
Lemma 2[36]: For an n-dimensional ODE system (n ≥ 3), if there is a saddle-node bifurcation and a Jacobian matrix at the saddle-node bifurcation has a zero eigenvalue, and there exists a nu eigenvalue with Reλj > 0 and an ns eigenvalue with Reλj < 0. Then a saddle-node with ns. nu > 0, ns + nu + 1 = n has at least two homoclinic orbits. At the same time, the vanishing of a saddle point can produce an infinite number of periodic orbits.
In order to study the influence of network structure, the concept of entropy will be introduced. The entropy of network structure is defined to quantitatively study the heterogeneity of complex networks[37]. The connectivity of nodes determines the importance of the nodes in the network. We give the definition of node importance. Let the degree of node i in the network be ki and define
is the standard form of the entropy of the network structure, where 0 < E < 1. Here, the higher the entropy of the network structure, the more homogeneous the network is.
From the above analysis, the following results can be obtained:
Theorem 4: For system Eq. 5, if all eigenvalues at the equilibrium point have negative real parts, the system is stable. Otherwise, it is unstable.
Proof: system Eq. 5 can be viewed as a system of 3N equations. Its linearized equations can also be written as
Theorem 4 shows that the system stability changes when the relationships between network nodes are introduced. The eigenvalues are obtained to satisfy certain conditions. System instability leads to the dissemination of tacit knowledge. The mechanism of tacit knowledge dissemination is shown from the perspective of system dynamics.
Theorem 5: For system Eq. 5, if the eigenvalue Λi (i = 1, 2, 3 ⋅⋅⋅ N) of the linearized matrix A causes saddle bifurcation and ns. nu > 0, ns + nu + 1 = N, periodic orbits will be generated for system Eq. 5.
Proof: The eigenvalue Λi of the linearized matrix A can be regarded as a control parameter of the system. According to Lemma 2 and Theorem 4, periodic orbits will appear in system Eq. 5.
Theorem 5 proves that saddle node bifurcation occurs in the system when the eigenvalue satisfies certain conditions. The disappearance of saddle points generates infinitely many circular trajectories, which proves the cycle dissemination of tacit knowledge.
Corollary 1: For system Eq. 8, if the eigenvalue Λmax of the linearized matrix A causes saddle bifurcation and ns. nu > 0, ns + nu + 1 = N, periodic orbits will be generated for system Eq. 8.
Proof: If Λmax causes saddle-node bifurcation, let Λi = Λmax, then according to Theorem 4, system Eq. 8 can generate periodic orbits.
Theorem 6: In the homogenous network, In the case of stability analysis, the stability of system Eq. 5 and system Eq. 7 is consistent.
Proof: In a homogeneous network, the eigenvalues of the matrix A are meaningful when the coupling strength of the network reaches a certain value. Meanwhile, Λmax = max{Λi, i = 1, 2, 3, ⋅⋅⋅, N}. Thus, whenever λ1max, λ2max, λ3max has a negative real part. Therefore, there must be λ1i, λ2i, λ3i that all have negative real parts.
Theorem 6 shows that in the case of homogeneous networks, the stability of the network organization model is consistent with the original model through stability analysis.
4 Numerical results and discussion
We verify our theoretical results by numerical simulation and set the appropriate parameters μ = 0.1, β = 0.01, η = 0.3, γ = 0.2, δ = 0.5.
Based on Theorem 1, Figure 3 demonstrates that the system stabilizes at a positive equilibrium point when the parameter conditions are satisfied μη ≥ β2 + βγ + βδ. A suitable initial value condition is selected. The system stabilizes at a zero equilibrium point when the parameter conditions are satisfied μη ≤ β2 + βγ + βδ. According to Theorem 2 and comparing Figure 3 with Figure 4, it can be seen that the number of disseminators gradually decreases and finally disappears when the transfer rate decreases from 0.1 to 0.01 and certain parameters are satisfied. At the same time, the number of disseminators in enterprise alliance also decreases with the decrease of the tacit knowledge dissemination rate. Figures 4B,D give the critical values of the parameters for tacit knowledge dissemination, i.e., the bifurcation points of the system. Figure 4B shows that the system bifurcates when μ = 0.3, i.e., the transfer rate needs to reach 0.3 for the stable dissemination of tacit knowledge within the enterprise alliance. Similarly, Figure 4D provides a reference for the setting of the transmission rate. Therefore, enterprise alliance need to implement interventions to ensure the stable dissemination of tacit knowledge.
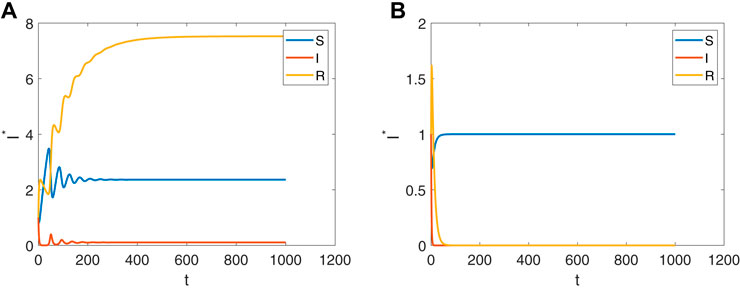
FIGURE 3. (A) The equilibrium point P2 is stable when the parameters μ = 0.1, β = 0.01, η = 0.3, γ = 0.2, δ = 0.5. (B) The equilibrium point P1 is stable when the parametersμ = 0.1, β = 0.1, η = 0.3, γ = 0.2, δ = 0.5. the initial values are (1,1,1).
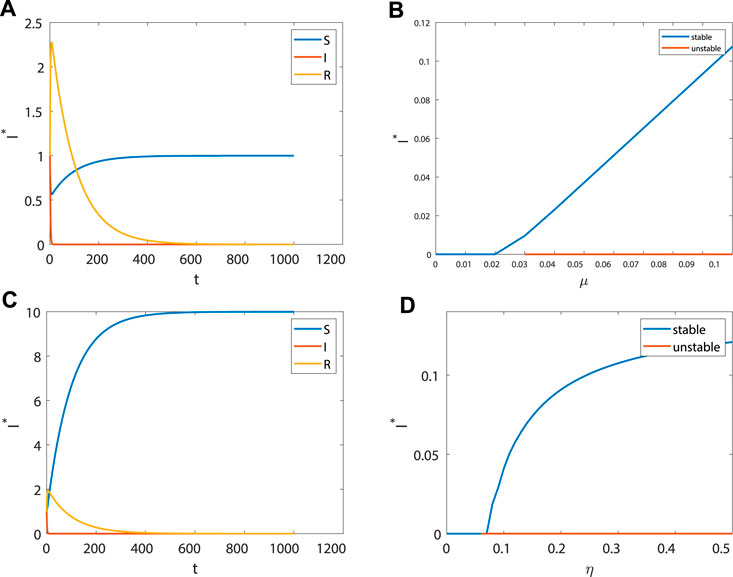
FIGURE 4. (A) The P1 is stable when μ = 0.01, β = 0.01, η = 0.3, γ = 0.2, δ = 0.5. (B) The bifurcation diagram of system Eq. 1 about μ. (C) The P1 is stable when μ = 0.1, β = 0.01, η = 0.05, γ = 0.2, δ = 0.5. (D) The bifurcation diagram of system Eq. 1 about η.
Figure 5 shows that the number of disseminators decreased gradually when δ increased from 0.1 to 1. Therefore, higher forgetting rate inhibits the spread of tacit knowledge. Therefore, employees should strengthen theoretical learning to reduce forgetting rate and promote the spread of tacit knowledge.
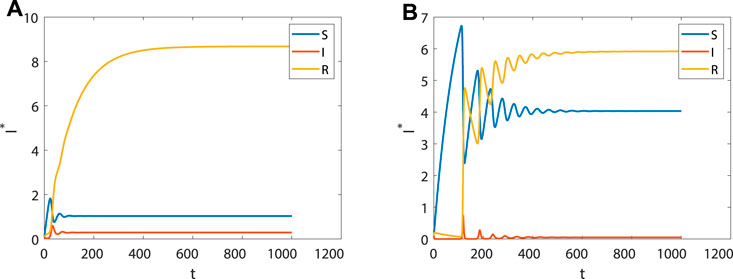
FIGURE 5. (A) The equilibrium point P2 is stable when μ = 0.1, β = 0.01, η = 0.3, γ = 0.2, δ = 0.1. (B) The equilibrium point P1 is stable when μ = 0.1, β = 0.1, η = 0.3, γ = 0.2, δ = 1 the initial values are (1,1,1).
Figure 6 shows that NSE increases and then stabilizes when k gradually increases. When the entropy of the network structure is larger, the network is more uniform and more conducive to the dissemination of tacit knowledge. Figure 7 illustrates that when k is small, λ1 < 0, system Eq. 5 is stable. When k increases, the system is unstable. At this time, λ1 = −0.1 + d1Λmax > 0. According to Theorem 4 and Corollary 1, the system Eq. 5 bifurcates (λ1 = −0.1 + d1Λmax = 0) and generates periodic orbits. The period of tacit knowledge dissemination will become shorter.
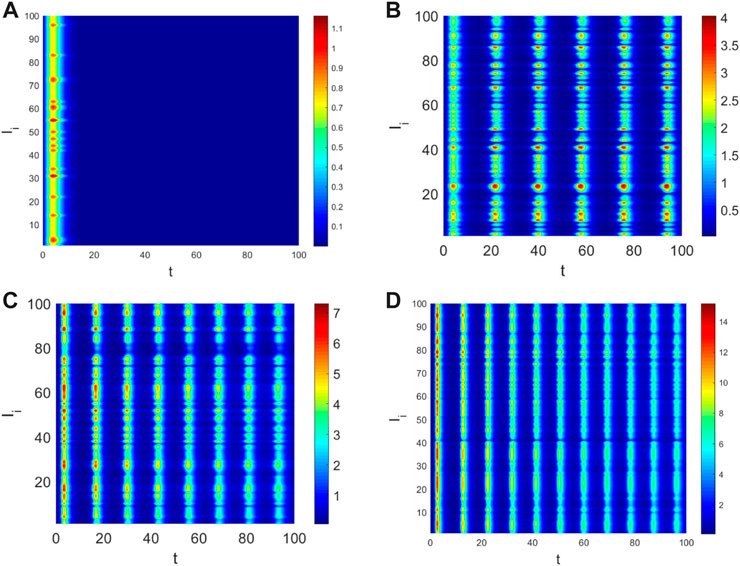
FIGURE 7. The pattern formation is given when d1 = 0.1, d2 = 0, μ = 0.1, β = 0.1, η = 0.3, γ = 0.2, δ = 0.5. Periodic dissemination behavior appears. The dissemination period becomes shorter. The blue region indicates that there is no disseminators in the system. At this time, the system is stable and the dissemination phenomenon does not exist. If the color gradually becomes shallow, then the system is unstable. Knowledge disseminators exist when the system is unstable. Tacit knowledge begins to spread (A) The system is stable when k = 10. (B) The system is unstable whenk = 100. (C) The system is unstable whenk = 200 (D) The system is unstable when k = 400.
We construct an NW network and study the influence of NSE on the knowledge dissemination process i.e., the impact of the number of connected edges of the network. NSE is an important index to measure the homogeneity of the network structure. When the NSE is large, employees in an enterprise alliance establish connections with other employees as much as possible. They seek to coordinate with multiple partners with complementary tacit knowledge, which corresponds to the network nodes being connected to other nodes as much as possible. The network is highly homogeneous. When the edges of the network are used as dissemination diffusion channels, the dissemination of information will be more convenient. Therefore, the degree of nodes (connected edges of the network) is particularly significant. When the network is highly homogeneous, the network nodes have a high degree of integration, i.e., the employees of the enterprise alliance will communicate more widely and deeply. The homogeneous network will be more conducive to the dissemination of tacit knowledge. In a heterogeneous network, the difference in degree between different nodes is large. The dissemination channel of the network is easily disturbed by external factors.
Figure 8, 9 shows that in addition to the periodic dissemination of tacit knowledge, there is a local dissemination behavior. The network connection is sparse when the NSE is small. Only a few nodes are connected, or isolated nodes exist. Some employees in the enterprise alliance may be unwilling to communicate with others because of their personality. From the above analysis, we can increase the NSE of the social network by increasing the value of k to promote the dissemination of tacit knowledge.
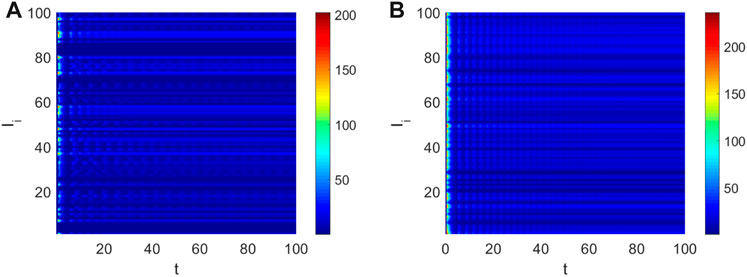
FIGURE 8. The pattern formation is given when d1 = 3, d2 = 0, μ = 0.1, β = 0.1, η = 0.3, γ = 0.2, δ = 0.5. (A) The system is unstable when k = 50. (B) The system is unstable when k = 100.
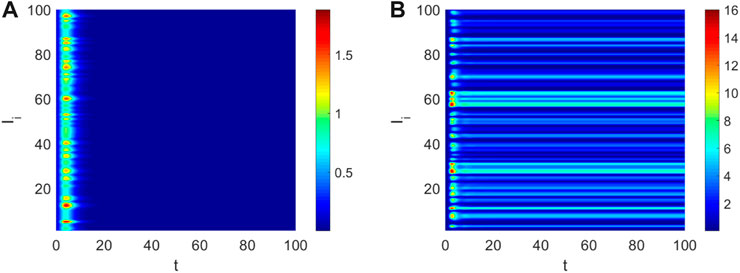
FIGURE 9. The pattern formation when k = 100, d2 = 0, μ = 0.1, β = 0.1, η = 0.3, γ = 0.2, δ = 0.5. (A) The system is stable when d1 = 0.1. (B) The system is unstable when d1 = 1.
For the tacit knowledge dissemination model of network organization, the diffusion of knowledge learners and knowledge disseminators are significant for disseminating tacit knowledge. In the following, we explore the effect of knowledge learner diffusion. At this point, let d2 = 0, we fix the parameters μ = 0.1, β = 0.1, η = 0.3, γ = 0.2, δ = 0.5. The eigenvalues of system Eqs. 6, 7 can be written as
Figure 10A presents that the system is stable when d1 = 0.01, d2 = 0, k = 150. As shown in Figure15, Λmax ≈ 4 when k = 150. Substituted d1 and Λmax into Eq. 10, we can get λ1max < 0. When d1 gradually increases from 0.01 to 1, we can get λ1max > 0. The phenomenon of periodic dissemination and local dissemination appears. The diffusion intensity of knowledge learners becomes larger. The knowledge learners frequently communicate with other employees to learn. The dissemination period becomes shorter. The local dissemination will become more and more obvious. Tacit knowledge can be circulated or disseminated within the company or within the innovation organizations in the alliance. By comparing (9) (B) and (11) (A), the system stability may be different even if the diffusion coefficients are the same when the coupling strength of the network changes. With the increase of the number of coupling nodes in NW network, the phenomenon of periodic and local dissemination coexist, which further improves the efficiency of tacit knowledge dissemination. Figure 11 illustrates that the sensitivity of tacit knowledge dissemination increases as d1 increases. Figures 12A,C give the bifurcation diagram of the system about d1, from which it can be seen that d1 ≈ 0.025 is the bifurcation point, corresponding to the eigenvalue λ1max (d1) = 0. (At this time, the system is stable when λ1max (d1) < 0; the system is unstable when λ1max (d1) > 0.) Therefore, the diffusion of knowledge learners is an important factor leading to the dissemination of tacit knowledge. It may not cause the dissemination of tacit knowledge when the communication frequency of knowledge learners is low. When strength of diffusion is relatively large, i.e., the communication frequency of knowledge learners is relatively high, then periodic dissemination will occur. From Figure 12B, the dissemination period may be shortened as d1 increases.
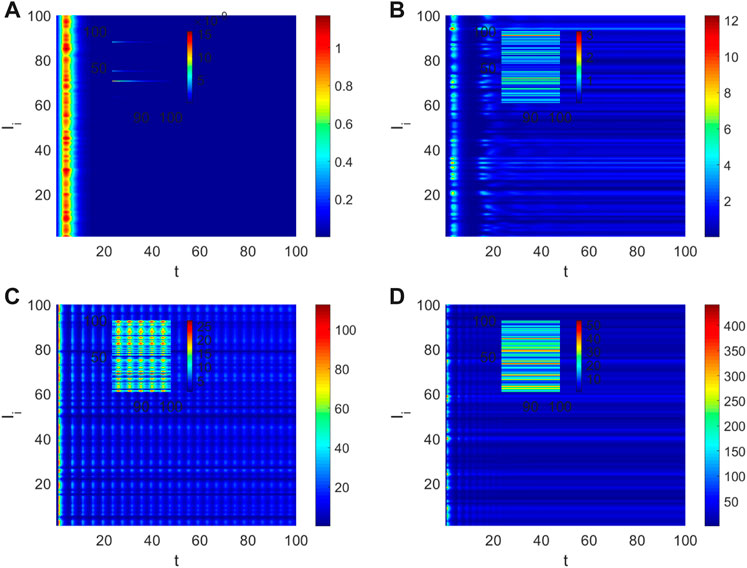
FIGURE 10. The pattern formation is given when k = 150, d2 = 0, μ = 0.1, β = 0.1, η = 0.3, γ = 0.2, δ = 0.5. The subfigure shows the stability of the system in the time interval (80–100). (A) The system is stable when d1 = 0.01 (B) The system is unstable when d1 = 0.1. (C) The system is unstable when d1 = 1. (D) The system is unstable d1 = 2.
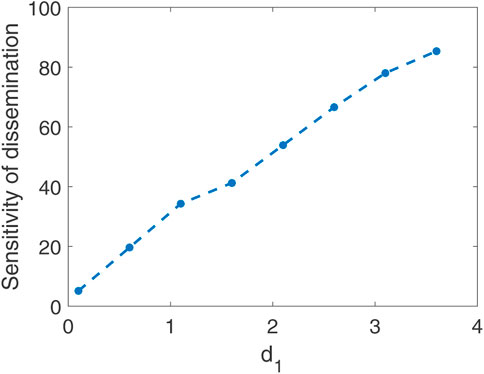
FIGURE 11. The relationship between dissemination sensitivity and d1 is given when μ = 0.1, β = 0.1, η = 0.3, γ = 0.2, δ = 0.5, k = 150, d2 = 0.
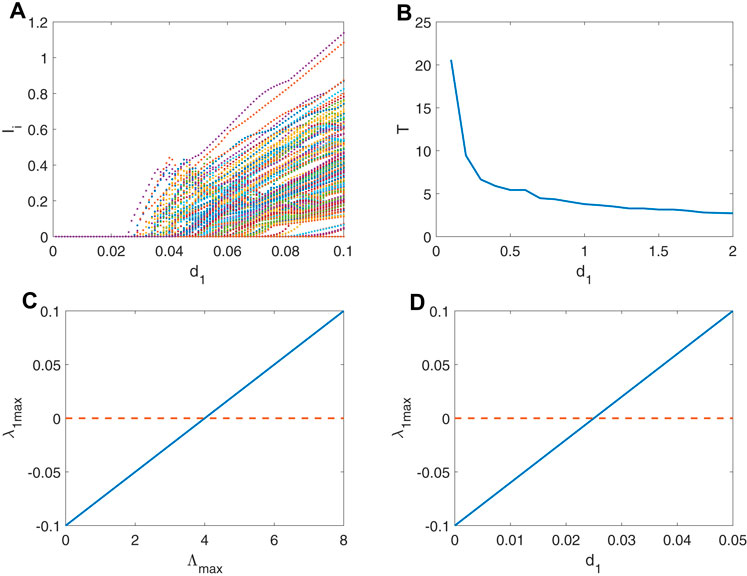
FIGURE 12. The bifurcation diagram is given when d1 = 0, μ = 0.1, β = 0.1, η = 0.3, γ = 0.2, δ = 0.5. (A) The bifurcation diagram of d1 is given. (B) The variation curve of dissemination period with d1 is given. (C) The variation curve of λ1max with Λmax is given. (D) The variation curve of λ1max with d1 is given.
We have studied the role of network coupling strength in tacit knowledge dissemination. The coupling strength of the network can be regarded as the frequency of communication among nodes or the sensitivity of tacit knowledge dissemination. Based on the above analysis, the diffusion of knowledge learners may increase the sensitivity of tacit knowledge dissemination. The dissemination period is shorten.
Next, we study the effect of diffusion of knowledge disseminators on the system. We assume d1 = 0. The eigenvalues of system (5) and system (7) can be written as
Figure 13A shows that there is no dissemination phenomenon when d2 = 0.1, k = 100. According to theorem 4, there is local dissemination phenomenon in Figure 13B as the diffusion coefficient increases. The dissemination strength increases with the coupling strength in Figure 13C. The dissemination of tacit knowledge disappears when the coupling strength is below a particular value (λ2max < 0). Comparing with each subplot of Figure 13, the diffusion of knowledge disseminators causes the local dissemination phenomenon. Therefore, the coupling strength of nodes and the diffusion of knowledge disseminators are fundamental to accelerate the dissemination of tacit knowledge. Next, we consider the bifurcation concerning d2, k, Λmax to explore the dynamical behavior of the system. Figure Figure14A shows that the system bifurcates when d2 = 0.15, corresponding to the bifurcation point in Figure 14B. The bifurcation diagram of the system for k and Λmax is given in Figure 14C.
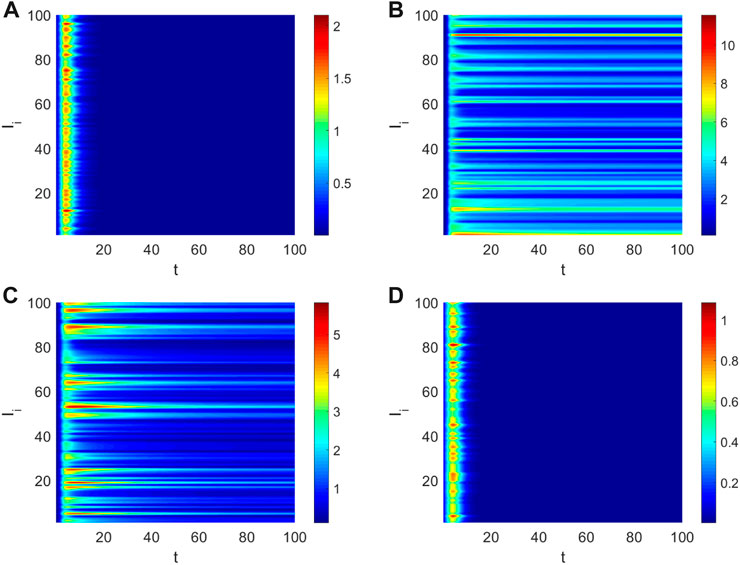
FIGURE 13. The pattern formation is given when μ = 0.1, β = 0.1, η = 0.3, γ = 0.2, δ = 0.5. (A) The system is stable when d1 = 0, d2 = 0.1, k = 100. (B) The system is unstable when d1 = 0, d2 = 0.2, k = 100. (C) The system is unstable whend1 = 0, d2 = 0.2, k = 130. (D) The system is stable when d1 = 0, d2 = 0.2, k = 50.
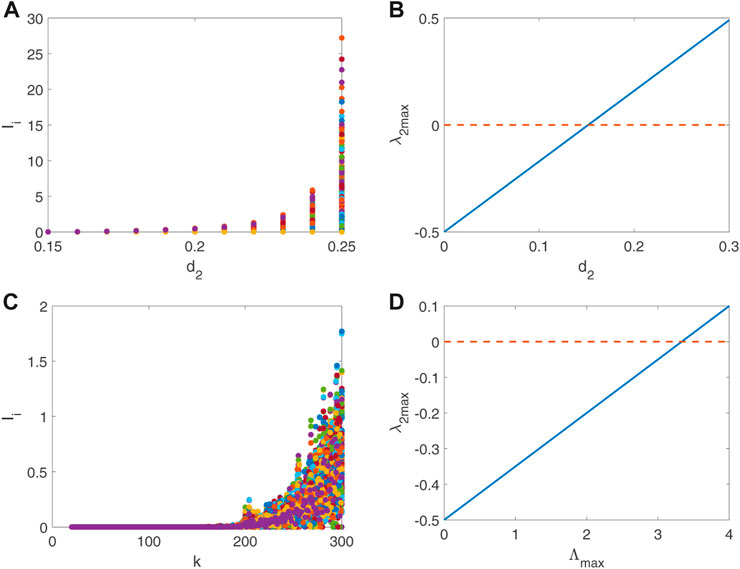
FIGURE 14. The bifurcation diagram is given when N = 100, d2 = 0.1, k = 150, μ = 0.1, β = 0.1, η = 0.3, γ = 0.2, δ = 0.5. (A) The bifurcation diagram of d2 is given. (B) The variation curve of Λmax with d2 is given. (C) The bifurcation diagram of k is given. (D) The variation curve of λ2max with Λmax is given.
From above, we investigated the effect of diffusion of a single type of individual in network organization tacit knowledge dissemination model. In the following, we study the combined effect of diffusion between knowledge learners and knowledge disseminators on the system dynamics behavior. At this point, the eigenvalues of system Eqs. 5, 7 can be written as
Figure 16 shows the pattern dynamics of the system when d1 = 0.1 is fixed and d2 gradually increases. At this time, From Figure 15, Λmax ≈ 4 when k = 150. Substituting d1 and Λmax into Eq. 14, we can see λ1max > 0. That’s why system Eq. 7 is always unstable in Figure 16.
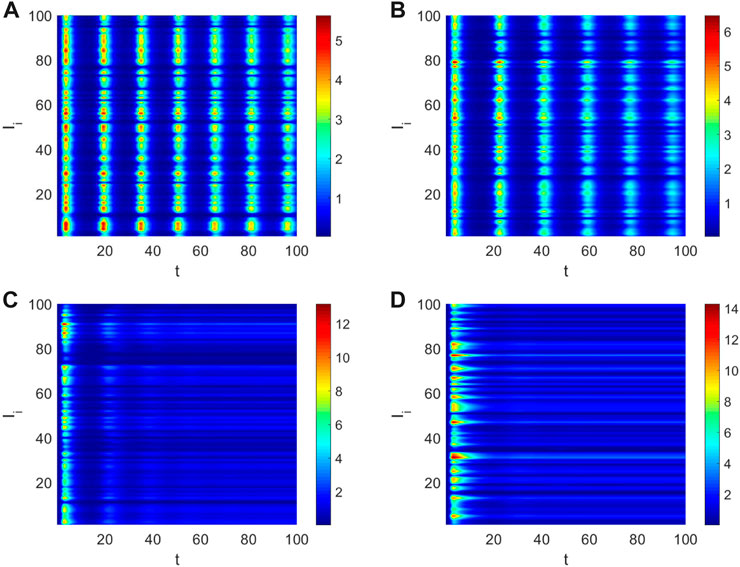
FIGURE 16. The pattern formation is given when d1 = 0.1, k = 150, μ = 0.1, β = 0.1, η = 0.3, γ = 0.2, δ = 0.5. (A) The system is unstable whend2 = 0.02. (B) The system is unstable when d2 = 0.05. (C) The system is unstable when d2 = 0.1. (D) The system is unstable d2 = 0.15.
Figure 16 illustrates the combined effect of knowledge learner and knowledge disseminator diffusion on tacit knowledge dissemination. We can see a competition between the diffusion of knowledge learners and the diffusion of knowledge disseminators when d1 increase. The periodic dissemination behavior occurs, or the local dissemination behavior occurs. The periodic behavior occurs when d2 is small and d1 is fixed. The cycle length becomes larger as d2 increases. The local dissemination phenomenon occurs when d2 increases to some fixed value.
The same method was used. When d2 = 0.1 and k = 150 are fixed, substituting d2 and Λmax into Eq. 11, we obtain λ2max < 0. There is λ1max < 0 when d1 is small. Therefore, the system is stable. However, with the increase of d1, the periodic dissemination and local dissemination coexist. The pattern dynamics information of Figure 17 is obtained by changing d1. The dissemination intensity increases with d1. The diffusion of knowledge learners is the key factor of the periodical dissemination.
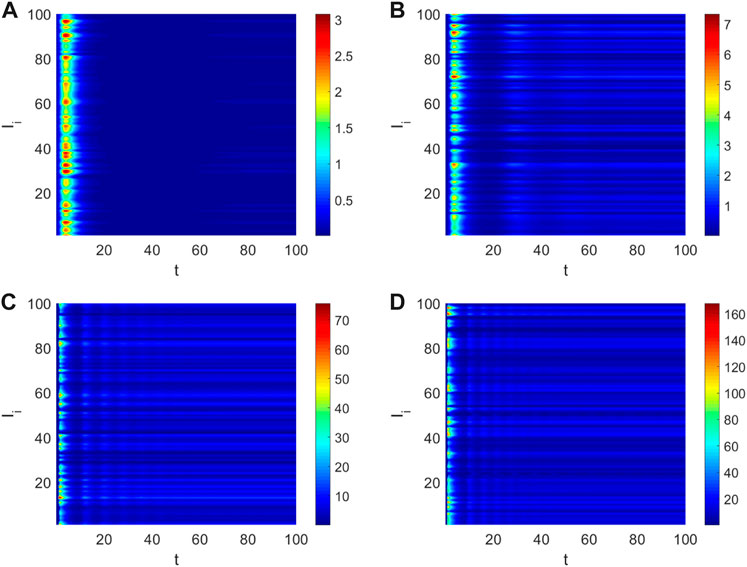
FIGURE 17. The pattern formation is given when d2 = 0.1, k = 150, μ = 0.1, β = 0.1, η = 0.3, γ = 0.2, δ = 0.5. (A) The system is unstable when d1 = 0.005. (B) The system is unstable when d1 = 0.05. (C) The system is unstable when d1 = 0.07. (D) The system is unstable when d1 = 0.1.
5 Conclusion and outlook
This study investigates the dynamic mechanism of the network organization tacit knowledge dissemination model. We transform network topology into adjacency matrix to study the influence of network structure on tacit knowledge dissemination. By using the methods of network dynamics and bifurcation theory, some valuable conclusions are obtained. Through stability analysis, the stable dissemination conditions of the system are obtained (Theorem 1). Tacit knowledge dissemination can be accelerated by adjusting corresponding parameters when certain parameters are satisfied (Theorem 2). The addition of diffusion does not change system stability (Theorem 3). The I* > 0 when the system becomes unstable at zero equilibrium. Therefore, tacit knowledge dissemination appears (Theorem 4). The effect of diffusion of knowledge subjects on the dynamical behavior system is explained by Turing instability. Meanwhile, this study demonstrates the effect of network topology on the dynamical behavior of the system through NSE. The results show that when the coupling strength of the network reaches a certain value, the system will show the phenomenon of period dissemination (Theorem 5 and Corollary 1). By introducing the maximum value of eigenvalues to simplify the model, the consistency of the stability of the two models is verified (Theorem 6). As the network coupling strength increases, the dissemination period will gradually become shorter. The local dissemination is generated. The joint diffusion of knowledge learners and knowledge disseminators can produce richer dynamical behavior.
The above analysis gives us some managerial implications. Firstly, in the enterprise alliance, the contact of employees is the main way of tacit knowledge dissemination. Therefore, alliance enterprises should establish smooth communication channels and enhance mutual trust to improve the speed of knowledge dissemination. Internal learning and external learning of enterprise alliance are equally important. Enterprises should strengthen communication among employees in different fields. Absorptive capacity and innovation capability are crucial for tacit knowledge dissemination and innovation of alliance enterprises. Alliance enterprises should establish learning organization. By guiding employees to improve their learning ability and carry out internal training, employees’ understanding and application of new knowledge are strengthened. In addition, enterprises should also introduce innovation incentive mechanism and strengthen the motivation for continuous innovation.
Data availability statement
The original contributions presented in the study are included in the article/Supplementary Material, further inquiries can be directed to the corresponding authors.
Author contributions
JS made contributions to the conception or dessign of mathematical model, supervision and funding acquistition. LG made contributions to supervision, the method and conception of Tacit knowledge dissemination. QL made contributions to writing, editing, original draft, formal analysis.
Funding
This work is supported by National Natural Science Foundation of China (11772291) and Basic Research Project of Universities in Henan Province (21zx009). Postgraduate Innovative Project of North China University of Water Resources and Electric Power(No.YK2021-114).
Conflict of interest
The authors declare that the research was conducted in the absence of any commercial or financial relationships that could be construed as a potential conflict of interest.
Publisher’s note
All claims expressed in this article are solely those of the authors and do not necessarily represent those of their affiliated organizations, or those of the publisher, the editors and the reviewers. Any product that may be evaluated in this article, or claim that may be made by its manufacturer, is not guaranteed or endorsed by the publisher.
References
1. Gubbins C, Dooley L. Delineating the tacit knowledge-seeking phase of knowledge sharing: The influence of relational social capital components. Hum Resource Dev Q (2021) 32:319–48. doi:10.1002/hrdq.21423
2. Brodbeck M, Polanyi M. Personal knowledge: Towards a post-critical philosophy. Am Sociological Rev (1960) 25:582. doi:10.2307/2092944
3. Long W, Guan L, Shen J, Song L, Cui L. A complex network for studying the transmission mechanisms in stock market. Physica A: Stat Mech its Appl (2017) 484:345–57. doi:10.1016/j.physa.2017.04.043
4. Jiang G, Xu Y. Tacit knowledge sharing in it r&d teams: Nonlinear evolutionary theoretical perspective. Inf Manag (2020) 57:103211. doi:10.1016/j.im.2019.103211
5. Ritala P, Huizingh E, Almpanopoulou A, Wijbenga P. Tensions in r&d networks: Implications for knowledge search and integration. Technol Forecast Soc Change (2017) 120:311–22. doi:10.1016/j.techfore.2016.12.020
6. Wenping W, Bing Z. Emergence characteristics of knowledge flow in knowledge networks under dynamic relationship strengths. J Manag Sci China (2013) 16:1–11.
7. Kerssens-van Drongelen IC, Cooke A. Design principles for the development of measurement systems for research and development processes. R&D Manag (1997) 27:345–57. doi:10.1111/1467-9310.00070
8. Yu D, Pan T. Tracing knowledge diffusion of topsis: A historical perspective from citation network. Expert Syst Appl (2021) 168:114238. doi:10.1016/j.eswa.2020.114238
9. Starnini M, Machens A, Cattuto C, Barrat A, Pastor-Satorras R. Immunization strategies for epidemic processes in time-varying contact networks. J Theor Biol (2013) 337:89–100. doi:10.1016/j.jtbi.2013.07.004
10. Wang W, Liu Q-H, Cai S-M, Tang M, Braunstein LA, Stanley HE. Suppressing disease spreading by using information diffusion on multiplex networks. Sci Rep (2016) 6:29259–14. doi:10.1038/srep29259
11. del Valle Rafo M, Di Mauro JP, Aparicio JP. Disease dynamics and mean field models for clustered networks. J Theor Biol (2021) 526:110554. doi:10.1016/j.jtbi.2020.110554
12. Bajiya VP, Tripathi JP, Kakkar V, Wang J, Sun G. Global dynamics of a multi-group seir epidemic model with infection age. Chin Ann Math Ser B (2021) 42:833–60. doi:10.1007/s11401-021-0294-1
13. Zhan X-X, Liu C, Zhou G, Zhang Z-K, Sun G-Q, Zhu JJ, et al. Coupling dynamics of epidemic spreading and information diffusion on complex networks. Appl Math Comput (2018) 332:437–48. doi:10.1016/j.amc.2018.03.050
14. Liu Z, Han X. The process of organization and dynamic of evolution of tacit knowledge on innovation talents’ growth research. In: Advances in computational environment science. Charm: Springer (2012). p. 177–84.
15. Yunmeng L, Tiezhong L. Diffusion model for tacit knowledge of scientific cooperation network based on relevance: Case study of major sci-tech projects. Data Anal Knowledge Discov (2021) 5:10–20.
16. Muthuveloo R, Shanmugam N, Teoh AP. The impact of tacit knowledge management on organizational performance: Evidence from Malaysia. Asia Pac Manag Rev (2017) 22:192–201. doi:10.1016/j.apmrv.2017.07.010
17. Li J, Zhang Y, Man J, Zhou Y, Wu X. Sisl and sirl: Two knowledge dissemination models with leader nodes on cooperative learning networks. Physica A: Stat Mech its Appl (2017) 468:740–9. doi:10.1016/j.physa.2016.11.126
18. Maleszka M. Application of collective knowledge diffusion in a social network environment. Enterprise Inf Syst (2019) 13:1120–42. doi:10.1080/17517575.2018.1526325
19. Liu J-G, Zhou Q, Guo Q, Yang Z-H, Xie F, Han J-T. Knowledge diffusion of dynamical network in terms of interaction frequency. Sci Rep (2017) 7:10755–7. doi:10.1038/s41598-017-11057-8
20. Yu Z, Lu S, Wang D, Li Z. Modeling and analysis of rumor propagation in social networks. Inf Sci (2021) 580:857–73. doi:10.1016/j.ins.2021.09.012
21. Chen S, Zhao L, et al. Dynamic analysis of the rumor propagation model with consideration of the wise man and social reinforcement. Physica A: Stat Mech its Appl (2021) 571:125828. doi:10.1016/j.physa.2021.125828
22. Cao S-h. H, Bin and ZJ, Jin Z. Modeling of knowledge transmission by considering the level of forgetfulness in complex networks. Physica A: Stat Mech its Appl (2016) 451:277–87. doi:10.1016/j.physa.2015.12.137
23. Wang H, Wang J, Ding L, Wei W. Knowledge transmission model with consideration of self-learning mechanism in complex networks. Appl Math Comput (2017) 304:83–92. doi:10.1016/j.amc.2017.01.020
24. Wang H, Wang J, Small M, Moore JM. Review mechanism promotes knowledge transmission in complex networks. Appl Math Comput (2019) 340:113–25. doi:10.1016/j.amc.2018.07.051
25. Wang H, Wang J, Small M. Knowledge transmission model with differing initial transmission and retransmission process. Physica A: Stat Mech its Appl (2018) 507:478–88. doi:10.1016/j.physa.2018.05.041
26. Hu Q, Shen J. Turing instability of a modified reaction–diffusion holling–tanner model over a random network. Int J Bifurcation Chaos (2022) 32:2250049. doi:10.1142/s0218127422500493
27. Zheng Q, Shen J, Xu Y, Pandey V, Guan L. Pattern mechanism in stochastic sir networks with er connectivity. Physica A: Stat Mech its Appl (2022) 603:127765. doi:10.1016/j.physa.2022.127765
28. Wei Q. An analytical framework for the conflict coordination mechanism of knowledge network. In: International conference on management science and engineering management. Charm: Springer (2017). p. 166–79.
29. Dyer JH, Nobeoka K. Creating and managing a high-performance knowledge-sharing network: The toyota case. Strateg Manag J (2000) 21:345–67. doi:10.1002/(sici)1097-0266(200003)21:3<345:aid-smj96>3.0.co;2-n
30. Anagnost JJ, Desoer CA. An elementary proof of the Routh-hurwitz stability criterion. Circuits Syst Signal Process (1991) 10:101–14. doi:10.1007/bf01183243
32. Newman ME, Watts DJ. Renormalization group analysis of the small-world network model. Phys Lett A (1999) 263:341–6. doi:10.1016/s0375-9601(99)00757-4
33. Watts DJ, Strogatz SH. Collective dynamics of ‘small-world’networks. nature (1998) 393:440–2. doi:10.1038/30918
34. Wilson E, Farhoomand I, Bathe K. Nonlinear dynamic analysis of complex structures. Earthq Eng Struct Dyn (1972) 1:241–52. doi:10.1002/eqe.4290010305
35. Teschl G. Ordinary differential equations and dynamical systems. Graduate Stud Math (2000) 140:08854.
36. Kuznetsov YA. Saddle-node bifurcation. Scholarpedia (2006) 1:1859. doi:10.4249/scholarpedia.1859
Keywords: newman-watts network, network structure entropy, tacit knowledge, dynamical mechanism, local dissemination
Citation: Liang Q, Guo L and Shen J (2022) Dynamical mechanism of tacit knowledge dissemination based on newman-watts network. Front. Phys. 10:963640. doi: 10.3389/fphy.2022.963640
Received: 07 June 2022; Accepted: 02 September 2022;
Published: 29 September 2022.
Edited by:
Mahdi Jalili, RMIT University, AustraliaReviewed by:
Ankur Sensharma, University of Gour Banga, IndiaGui-Quan Sun, North University of China, China
Copyright © 2022 Liang, Guo and Shen. This is an open-access article distributed under the terms of the Creative Commons Attribution License (CC BY). The use, distribution or reproduction in other forums is permitted, provided the original author(s) and the copyright owner(s) are credited and that the original publication in this journal is cited, in accordance with accepted academic practice. No use, distribution or reproduction is permitted which does not comply with these terms.
*Correspondence: Lingling Guo, Z3VvbGluZ2xpbmdAbmN3dS5lZHU=; Jianwei Shen, eGNqd2hlbkBnbWFpbC5jb20=