- 1School of Science, Jimei University, Xiamen, Fujian, China
- 2State Key Laboratory of Modern Optical Instrumentation, College of Optical Science and Engineering, International Research Center for Advanced Photonics, Zhejiang University, Hangzhou, Zhejiang, China
- 3Intelligent Optics & Photonics Research Center, Jiaxing Research Institute, Zhejiang University, Jiaxing, China
During tumor resection, doctors use intraoperative biopsies to determine the tumor margin. However, the pathological procedures of traditional diagnostic methods, such as imprint cytology and frozen section analysis, are complicated and time-consuming. As this is not conducive to surgeries, their applications are limited to a large extent. Therefore, novel fast microscopy imaging technologies with resolutions comparable to those of pathological tissue sections are necessary. Stimulated Raman scattering (SRS), photoacoustic microscopy (PAM), multiphoton microscopy (MPM), and optical coherence microscopy (OCM) exhibit the advantages of high spatial resolution, large imaging depth, avoiding damage to biological tissues, label-free detection, and the availability of biochemical information of tissues. Additionally, they are superior to intraoperative biopsies owing to their fast imaging speeds. Therefore, they possess broad application prospects in tumor resection surgeries and the diagnosis of other diseases. This study briefly introduces the basic principles, structural characteristics, advantages and disadvantages, and the existing research status of SRS, PAM, MPM, and OCM in biomedicine. Furthermore, we propose a multi-mode hybrid detection technology that can be used for surgeries. The combination of the proposed technology with deep learning-based artificial intelligence can form the basis for intraoperative diagnosis in the future.
1 Introduction
Owing to the increase in the number of people diagnosed with cancer, the methods of treating cancer have diversified, among which surgical removal of tumors is an important method. During tumor resection, doctors often focus on the marginal part of the tumor, and the complete resection of the tumor is a key factor that affects the prognosis of the patient and positive outcomes. However, no real-time and effective standard intraoperative margin management method has been established thus far in tumor resection operations.
Common traditional intraoperative diagnostic methods include imprint cytology and frozen section analysis, which effectively increase the probability of marginal negative. However, estimating the distance between the tumor and surgical margin accurately is difficult using imprint cytology [14]. The cryosection technique requires the pathologist to undertake a heavy workload, and the production process is time-consuming and complex. As these shortcomings rendered further development of traditional methods challenging, researchers began to explore novel alternative methods. At present, stimulated Raman scattering (SRS), photoacoustic microscopy (PAM), multiphoton microscopy (MPM), and optical coherence microscopy (OCM) are four typical methods for microscopy imaging of biological tissues. Table 1 indicates that the methods exhibit lateral resolution at the cell or subcellular level with large imaging depth [15–17] while achieving non-destructive label-free imaging of biological tissues. In terms of imaging speed, the tissue can be directly scanned and imaged without processing, which significantly reduces the image acquisition time. In biological tissue imaging, each of the four microscopy imaging techniques exhibits its unique characteristics. SRS has achieved video-level imaging speed [18] and is molecularly specific, facilitating selective imaging of different chemical components in tissues. PAM uses endogenous optical absorption characteristics of the sample for imaging, which can aid in realizing both tissue structure imaging and functional imaging [19]. The spectral measurement and microscopy imaging technologies of MPM can obtain the microstructure of biological tissues and their spectral characteristics. OCM uses low-coherence interference technology to significantly suppress the light scattered from the focal plane, thereby achieving deep imaging of highly scattering interstitial materials. In summary, as the four microscopy imaging technologies are instrumental in obtaining micropathological information, they can be considered the emerging technologies in clinical surgery.
This study primarily reviews the four microscopy imaging technologies, namely SRS, PAM, MPM, and OCM, analyzes their basic principles, structural features, advantages and disadvantages, and summarizes their research status in biomedicine. Furthermore, a novel multi-mode hybrid detection technique is proposed. With the rapid development of deep learning-based artificial intelligence (AI) in biomedicine, the combination of the proposed technology and AI can provide a new direction for the future development of microscopy imaging technology in the clinical field.
2 Methods
2.1 Traditional pathological methods
Intraoperative imprint cytology and frozen section analysis are the two common methods of edge assessment used for treating cancer. Frozen section analysis involves removing the fresh cancerous tissue from the patient and completing it through a series of processes, such as quick freezing, fixation, sectioning, and staining. In the process of rapid freezing, embedding agents such as OCT agent, B-ultrasound coupling agent and common glue are needed. The reagents commonly used in the fixation process are formaldehyde, ethanol and other fixation solutions. Among them, the use of embedding agent should be appropriate, too much or too little will affect the frozen quality of the specimen, and the fixation time is generally about 2 days. Frozen sections stained with hematoxylin and eosin (H&E) can accurately identify the presence or absence of cancer cells and the type of cancerous tissue, which is crucial for intraoperative decision-making. However, owing to the complicated production process of frozen section analysis, professional specimen-cutting technicians and well-trained pathologists are required. Moreover, as the process is expensive and time-consuming, only a few hospitals incorporate this method. During imprint cytology, the tissue fluid or blood on the fresh sample is sucked clean using an absorbent paper, and a small amount of tissue obtained by pressing the specimen with a slide is then fixed and stained. As this method can identify only cancer cells and cannot be used for tissue morphology analysis, it cannot determine the type of cancer. However, the accuracy of imprint cytology is comparable to that of frozen sections. Additionally, it is highly suitable for development in primary hospitals as the method is fast, economical, and practical.
The daily work of pathology doctors is cumbersome and extremely error-prone. To improve work efficiency, AI algorithms based on deep learning are increasingly researched. In 2016, the DeepCare team conducted an experiment which allowed senior- and low-level pathologists to diagnose the same group of breast cancer lymphatic metastasis pathological sections. The results indicated that all doctors were correct for 30 normal sections. However, the diagnostic sensitivity of doctors with 10, 20, and 40 years of experience was 57.5, 67.5, and 97.5%, respectively, for 40 cancerous sections. The intelligent algorithm model developed by the DeepCare team attained an accuracy of 92.5%. In 2015-2016, ISBI held an international competition [20] to evaluate whether deep learning algorithms can improve the accuracy and efficiency of pathological diagnosis of axillary lymph node metastasis. Experimental results indicate that without time constraints, the area under the receiver operating characteristic curve (AUC) of the top five algorithms (0.960) is comparable to that reported by pathologists (0.966). When the time constraints are considered, the AUC of the best algorithm (0.944) is significantly better than that reported by pathologists (0.810).
These results verify that AI based on deep learning exhibits significant potential in pathological diagnosis. If AI algorithms can be combined with other emerging imaging technologies, the manual and financial resources can be reduced in the traditional pathological diagnosis process. Additionally, misdiagnosis caused by the lack of experience of pathologists can be avoided.
2.2 Emerging microscopy imaging methods
2.2.1 Stimulated Raman scattering
2.2.1.1 Principle of stimulated Raman scattering
Raman spectroscopy is a type of vibration spectroscopy based on Raman scattering [21]. Spontaneous Raman scattering microscopy is a microscope application of Raman spectroscopy. Spontaneous Raman scattering microscopy uses the difference in Raman characteristic peak signal intensity distributions of different molecules to perform selective imaging of specific chemical components of biological tissues based on the different molecular vibrations corresponding to different cell components. The extremely weak spontaneous Raman signal increases the data acquisition time [22], which significantly limits its application in biomedicine. Coherent anti-Stokes Raman scattering (CARS) and Stimulated Raman scattering (SRS) are coherent Raman scattering microscopy [23]. They enhance the signal through coherent excitation, considerably reducing data acquisition time. CARS generates non-resonant four-wave mixing signals [24], which cause a certain amount of spectrum distortion. Consequently, obtaining and processing accurate results become challenging. Unlike spontaneous Raman scattering and CARS microscopies, SRS microscopy is unaffected by non-resonant backgrounds and yields a spectrum that is substantially identical to spontaneous Raman microscopy. Moreover, SRS microscopy can acquire data at a faster rate [18], enabling real-time imaging of tumor boundaries during surgical procedures. The sensitivity of SRS exceeds that of spontaneous Raman microscopy by multiple orders of magnitude [25]. Therefore, SRS microscopy with considerably increased sensitivity can reduce the dwell time at a pixel to a few microseconds, achieving even video-rate imaging [25].
C.V. Raman proposed the Raman scattering effect in 1928 [26], which primarily refers to the frequency change after the laser is irradiated on the surface of an object; the changes in frequency rely on the characteristics of the scattering material. As the atomic groups of different substances exhibit different vibration modes, they generate scattered light of a specific frequency. SRS microscopy based on the Raman scattering effect is an emerging microscopy imaging technique, which was accidentally implemented in experiments by Woodbury and Ng in 1962 [27, 28]. In 2008, the research group of Harvard University involving Sunney Xie applied the technique to biological microscopy imaging [29]. SRS microscopy requires two lasers that satisfy the resonance conditions, namely pump light (Wp) and Stokes light (Ws), to excite the tissue sample. As depicted in Figure 1A, resonance coupling occurs when the frequency difference between the two beams of light equals the vibration frequency of the molecules in the sample. The molecules then transition from the ground state to the excited state, and the Raman signal is stimulated and amplified [29, 30]. Energy exchange occurs between the light and molecules during this process. Additionally, pumped photons are converted into Stokes photons via molecular vibration and energy level transitions. Thus, stimulated Raman loss (SRL) and stimulated Raman gain (SRG) occur in high-energy pump light and low-energy Stokes light, which can be used as a source of imaging contrast by detecting SRL and SRG, respectively [31].
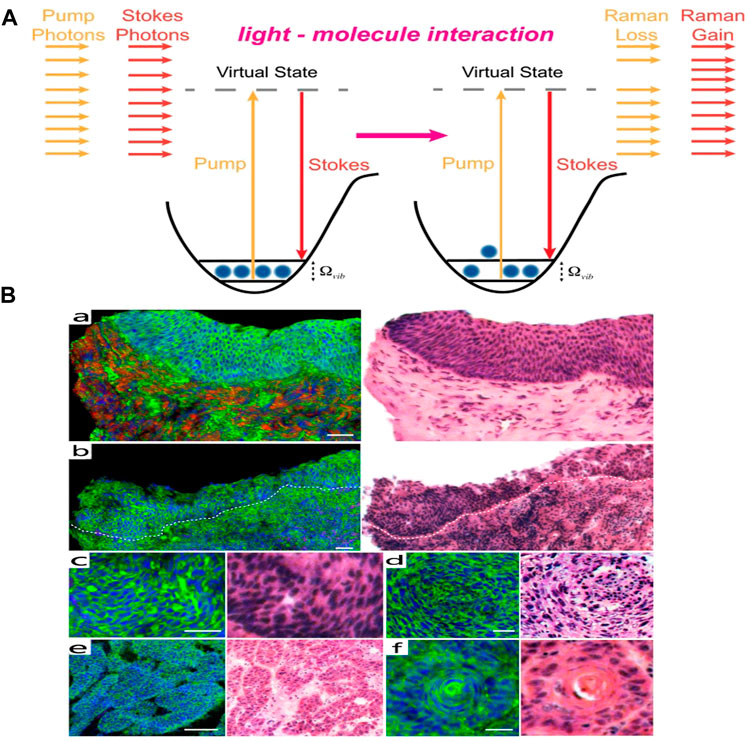
FIGURE 1. (A) Schematic of the stimulated Raman scattering (SRS) [30] and (B) SRS and hematoxylin and eosin (H&E) images of frozen sections obtained from laryngeal squamous cell carcinoma tissues. a: Laryngeal squamous cell carcinoma in situ; b: Invasive squamous cell carcinoma; c: Cytological atypia; d: Cytologicalatypia accompanied with lymphocytes and architectural neoplasm; e: Cancer nests; and f: A typical keratin pearl. Scale bars: 100 µm (b and e), 30 µm (a, c, d, and f) [40].
2.2.1.2 Research status of stimulated Raman scattering microscopy in biomedicine
As a label-free nonlinear optical microscopy imaging technique, SRS microscopy has a spatial resolution of up to 350 nm [32]. Additionally, the method is non-invasive, highly sensitive, and exhibits a fast imaging speed. This technique can achieve non-invasive high-resolution imaging of living biological tissues, providing important pathological information for doctors during intraoperative tumor resection. Studies have demonstrated that SRS imaging of tissue at different frequencies can map protein and lipid profiles with high vibrational contrast [3]. In 2016, Lu et al. [33] observed features in fresh brain tumor samples undetected by conventional methods. This improves the distinction between damaged and healthy tissues. Carbon-hydrogen (CH) vibrationalstretching is important for SRS microscopy [4]. Among the various CH stretching modes, the methyl (CH3) mode contributes to the vibrational signals of proteins and lipids. The methylene (CH2) mode is dominant in aliphatic lipids and can be utilized to map protein and lipid density.163 Therefore, SRS is sensitive to dense keratin [4].
As an emerging biomedical microscopy imaging technology, SRS has been widely used for rapid microscopy imaging of various cancer tissue samples, quantification, distribution, and metabolism of lipids [34–36], and drug delivery [37]. Experimental studies report that SRS microscopy can be comparable to standard H&E [38, 33].
In 2013, Mittal et al. [4] studied squamous cell carcinoma (SCC) in human skin using SRS microscopy technology. The obtained images indicate that keratinocytes with different morphologies exist in the base membrane and exhibit a tendency to expand to the dermis. Keratinization is an important feature of SCC, and SRS is highly sensitive to dense keratin proteins. Therefore, keratinocytes can be easily localized, which is convenient for doctors to formulate related treatment plans. Observing the tumor margin with clarity is extremely essential during brain tumor surgeries. In 2015, Ji et al. [39] of Harvard University used SRS microscopy to reveal the infiltration of gliomas successfully, which provided critical diagnostic information that was comparable to H&E. In 2017, Orringer et al. [17] used an in-house designed portable fiber laser microscope to perform rapid microscopy imaging of untreated brain tumor samples, pioneering the application of SRS microscopy in the operating room. The lateral resolution of SRS microscopy imaging technology is 360 nm and the axial resolution is 1.8 µm, which verifies that SRS microscopy serves as a simple and effective alternative to traditional histology. The portable fiber laser system facilitates the applications of SRS microscopy imaging technology in other clinical fields. In 2019, Zhang et al. [40] of Fudan University performed rapid SRS microscopy on laryngeal squamous cell carcinoma tissue sections; the images captured were nearly identical to those obtained using standard H&E sections in morphology. As depicted in Figure 1B, cancer nests, cancer cells, and keratin pearl are clearly visible. Based on this, they constructed a deep learning model capable of rapidly dividing the tissue samples into normal and cancerous, which is extremely essential for tumor resection during surgeries. As the naked eye cannot always distinguish the boundary between cancerous and normal tissues, incomplete resection of the diseased tissue and poor prognosis may occur without the use of special imaging equipment.
2.2.2 Photoacoustic microscopy
2.2.2.1 Principle of photoacoustic imaging
Photoacoustic imaging based on photoacoustic effects is a recently emerged non-invasive and non-destructive biomedical imaging technology; the basic principle can be summarized as follows. The optical signal generated by the nano-pulse laser is applied to biological tissues. The absorption of this optical signal by the biological tissue causes its interior to radiate photo-induced ultrasonic signals owing to the changes in energy. After the photo-induced ultrasound signal is received by the ultrasound transducer, a tissue image with characteristic information is obtained using the imaging algorithm [41]. Photoacoustic imaging exhibits the characteristics of high contrast, high resolution of optical imaging, and deep penetration of ultrasonic imaging. For AR-PAM systems, the lateral resolution and imaging depth can reach 45–120 µm and 3 mm, respectively [8]. The OR-PAM system has a lateral resolution and imaging depth of 5 µm [5] and 1.3 mm [6], respectively. Additionally, it exhibits the unparalleled advantages of pure optical and acoustic imaging [42].
PAM is an important branch of photoacoustic imaging [43], which generally adopts the methods of focused ultrasonic detection and incident light. The resolution of the image is determined by the smaller values in the ultrasonic and optical focuses [44]. PAM imaging can be divided into optical-resolution photoacoustic microscopy (OR-PAM) [5] and acoustic-resolution photoacoustic microscopy (AR-PAM) [8]. Figure 3A [7] and 2A [45] depict the structural diagrams of OR-PAM and AR-PAM, respectively. In OR-PAM system, the pulsed laser beam emitted by the laser source at 532nm is focused on the optical diffraction limit spot to irradiate the sample for excitation. A probe is then used to detect time-resolved photoacoustic signals in the sample [7]. In AR-PAM system, a tunable pulsed laser system is used to provide the light source, and the beam is weakly focused into the free space at the focal point by an optical condenser. The laser energy deposited on the surface is carefully monitored, and the image is acquired in each direction [45]. Typically, the OR-PAM system uses a focused or unfocused ultrasonic detector. The incident light operates in the focused mode, wherein the focal diameter of the light is less than tens of micrometers. The lateral resolution of the system is determined using the diameter of the light focal point, and its imaging depth is close to an average transmission free path. The AR-PAM system uses a weakly focused incident light and an ultrasonic transducer with a high frequency and large numerical aperture. The resolution of the system is determined by the ultrasonic transducer, and the imaging depth is greater than an average transmission free path, rendering it suitable for deep imaging of biological tissues [46]. Based on the mode of laser irradiation, AR-PAM can be divided into dark and bright field illuminations. When the dark field illumination is adopted, a large imaging depth and high signal-to-noise ratio (SNR) can be obtained. Moreover, the photoacoustic signal on the sample surface does not interfere with the internal signal, which improves the image quality. However, samples receive more laser energy when the bright field illumination is used.
2.2.2.2 Research status of photoacoustic microscopy in biomedicine
PAM is widely applied to the exploration of hemoglobin [9], melanin [45, 47], and lipids, which exhibit strong optical absorption properties. Furthermore, it is a powerful tool for studying cells, microvessels [45, 9], the brain [48], eyes [49], skin [45] and other tissues [50].
In 2005, Wang et al. [8] of the University of Washington designed and manufactured a reflective PAM imaging system based on dark field illumination, which was the first PAM imaging system. The system exhibited a lateral resolution of 45–120 µm and an imaging depth of 3 mm. They used the system to image the dorsal blood vessels of a dead rat and obtain a clearer picture of the blood vessel structure, paving the way for several PAM applications. In 2007, Wang and his research group [50] used the AR-PAM system to successfully realize tissue imaging up to 38 mm in chicken breasts using near-infrared laser pulses with a wavelength of 804 nm. In the same year, the group completed non-invasive imaging of changes in blood oxygen saturation of the subcutaneous microvasculature of living mice under hyperoxic, normoxic, and hypoxic conditions [51]. Animal experiments have demonstrated that the AR-PAM system can provide high-resolution images of microvessels. To demonstrate the clinical feasibility of the technology, some human experiments have been conducted. In 2011, Favazza et al. [45] used AR-PAM to image the microvascular system and a melanocytic nevus in human skin. The obtained images were used to analyze the skin microvascular circulation and pigmented lesions, which indicated the potential of using AR-PAM for pigmented diseases and microvascular systems. Figure 2B depicts the vascular system in a small piece of skin on the palm; the epidermis, cuticle, and epidermis–dermis boundary can be easily distinguished in the figure. Wang et al. [5] first proposed an optical resolution PAM imaging system in 2008 with an imaging depth of 0.7 mm; they achieved a lateral resolution of 5 µm via optical focusing. Owing to the strong optical absorption properties of hemoglobin, when the system was applied to the ears of living mice, the microvascular veins of the ears and single capillaries were clearly visible. In 2009, Wang et al. [9] used the OR-PAM system to demonstrate the quantification of hemoglobin concentration and oxygenation in a single microvessel below capillaries, which was a significant breakthrough in microhemodynamics. Their reports indicate that OR-PAM can be used to perform functional volume imaging of the vascular system microcirculation. As the existing OR-PAM is a complex desktop system that requires more space and lacks flexibility, it is difficult to extend it to clinical surgery for real-time observation. Therefore, Zemp and his research team [52] from the University of Alberta, Canada, developed a new type of handheld OR-PAM system through technical improvements in 2011. This probe weighs less than 0.5 kg with an area of 5 cm × 6 cm. Although no breakthrough exists in the lateral resolution and imaging degree of this system, the probe is sufficiently flexible to facilitate imaging of different body parts with potential clinical applications. Additionally, it forms the basis for a breakthrough in the research of OR-PAM. As respiration and heart movement of living animals produce certain artifacts, previous studies on tumor blood vessels using OR-PAM were performed in the ears of the experimental subjects. However, the ear is not an ideal site for tumor metastasis. Therefore, in 2015, Liang et al. of Shenzhen Institute of Advanced Technology [8] inoculated 4T1 tumors in the subcutaneous tissue on the backs of mice to observe the formation of tumor blood vessels. They used OR-PAM to perform the dynamic tracking and quantitative analysis of the tumor vessel density, curvature, and diameter on the 3rd, 5th, and seventh days of tumor growth, respectively. Figure 3B depicts the change in the tumor vessel diameter. This was the first study of OR-PAM on tumor blood vessels in the subcutaneous tissues on the backs of mice, indicating its broad application prospects in anti-tumor angiogenesis.
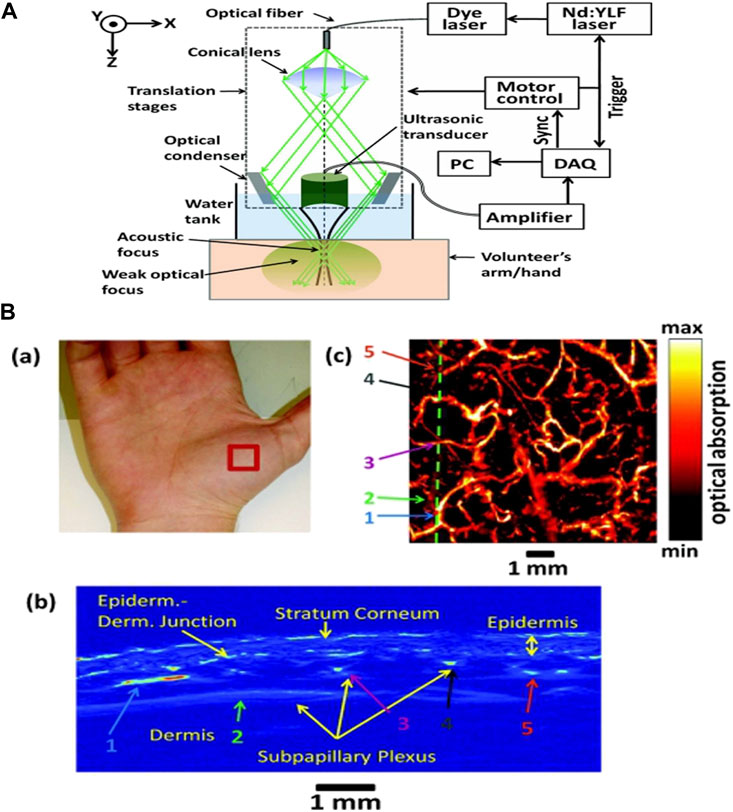
FIGURE 2. (A) Schematic of the acoustic-resolution photoacoustic microscopy (AR-PAM) system. (B) (a) A small region of the palm and (b) a cross-sectional photoacoustic image captured along the dashed line in (c). (c) A maximum amplitude projection photoacoustic image obtained from (a) [45].
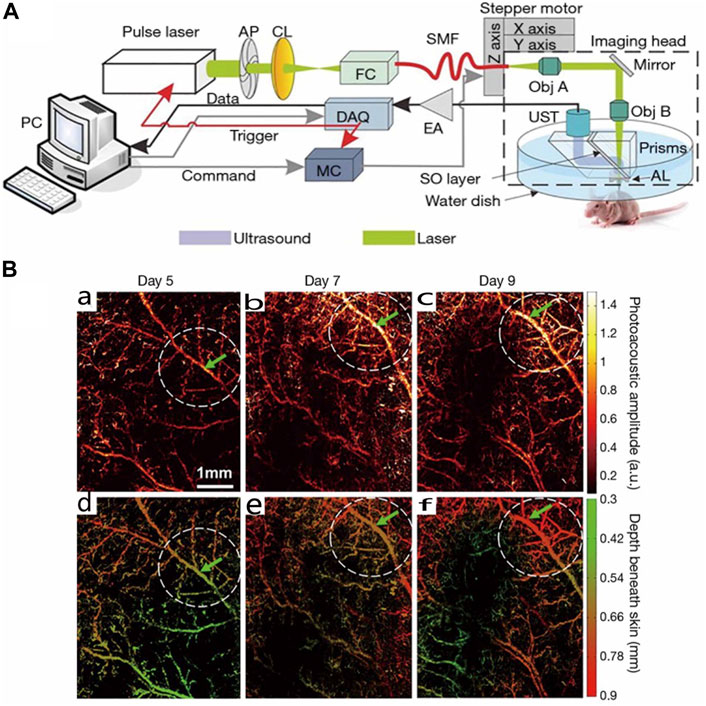
FIGURE 3. (A) Schematic of the optical-resolution photoacoustic microscopy (OR-PAM) system. (B) Maximum amplitude projection OR-PAM images of developing 4T1 tumor angiogenesis on (a) day 5, (b) day 7, and (c) day 9 post implantation. (d–f) Depth-encoded maximum amplitude projection corresponding to (a–c). The superficial and deep vessels are depicted in green and red, respectively [7].
2.2.3 Multiphoton microscopy
2.2.3.1 Principle of multiphoton microscopy
In MPM, two-photon excited fluorescence (TPEF) is collected and second harmonic generation (SHG) light signals are generated by the interaction between the femtosecond laser and endogenous substances in biological tissues to complete the non-destructive and label-free imaging of biological tissues [53, 54]. Hopper-Mayer proposed the TPEF theory in 1931 [55]. In 1990, Denk et al. of Cornell University in the United States first proposed the TPEF microscopy imaging technology and developed the first two-photon laser scanning microscope [56]. The second harmonic microscopy imaging technology was proposed in the 1980s and was applied to high-resolution microscopes only in the late 1990s. TPEF is a third-order nonlinear process, whereas SHG is a second-order nonlinear optical phenomenon. As depicted in Figure 4Aa, the fluorescent molecule transitions from the ground state to the excited state after absorbing two photons simultaneously. Subsequently, a long-wavelength photon is emitted back to the ground state after energy relaxation. During the two-photon excitation process, the frequency of the emitted photons is less than double the frequency. Moreover, the photons are broad-spectrum, non-directional, and non-coherent. SHG being a second-order nonlinear optical phenomenon [57] must satisfy two prerequisites; 1) the material must exhibit a non-centered symmetrical structure, and 2) the incident light should be a high-intensity coherent light. Figure 4Ab illustrates the occurrence of SHG. An electron in the ground state absorbs two photons with identical frequencies and attains the virtual energy state. Subsequently, a photon with a doubled frequency is emitted from the virtual energy state. In the SHG process, the outgoing light is directional and coherent. As SHG is associated with the second-order nonlinear polarizability of a material, it can be used as a sensitive index for the material properties of tissues.
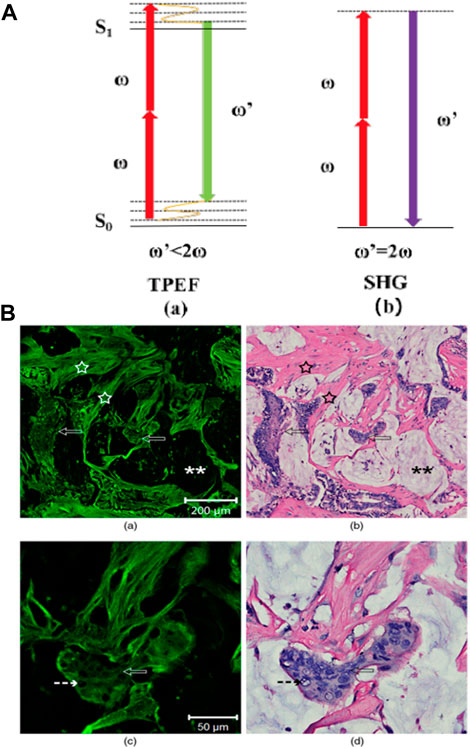
FIGURE 4. (A) Schematic of energy level transitions of (a) two-photon excited fluorescence (TPEF) and (b) second harmonic generation (SHG). (B) Comparison of representative multiphoton microscopy (MPM) and hematoxylin and eosin (H&E) images in pancreatic colloid carcinoma. Open arrows indicate the nest of cancerous tissue; pentagrams denote collagen fibers; asterisks represent mucous lakes; and dashed arrows indicate cancer cells [62, 63].
Typically, a complete MPM system is composed of a laser light source, detection system, and scanning microscope system. Two-photon microscopy is a typical application of multiphoton microscopy; an example is presented to introduce this concept. The example used a titanium sapphire femtosecond laser to perform the two-photon experiments. The laser had extremely high peak power (10.3 PW) [58], and provided sufficient intensity for two-photon excitation. Furthermore, the light source used a long-wavelength near-infrared laser, which resulted in a large penetration depth for 150 µm [59] thick biological samples. The two-photon microscope used a high-energy mode-locked pulsed laser, which ensured that the outgoing laser exhibits lower average energy and minimizes cell damage. The laser confocal microscope of the LSM 880 system is equipped with 10x, 20x, 40x, and 63x objective lenses with different magnification capabilities. The focal point of the objective lens exhibits the highest photon density, and two-photon excitation occurs only at the focal point of the objective lens [60]. Therefore, two-photon microscopy imaging does not require confocal pinholes, which can reduce phototoxicity and improve SNR and fluorescence detection efficiency.
Inherent fluorophores are abundant in biological tissues. When external contrast agents are not used, different signals can be generated when the laser interacts with biological tissues. For instance, collagen fibers can generate SHG signals, elastic fibers, nicotinamide adenine dinucleotide, flavin adenine dinucleotide, oxidized flavoprotein (Fp), keratin, and tubulin can produce a TPEF signal [61]. This information provides details of the tissue structure, cell morphology, and function of the sample. Furthermore, the information obtained from multiphoton images and spectral measurements are complementary and corroborative to each other. Therefore, MPM technology exhibits significant development prospects in biomedical research.
2.2.3.2 Research status of multiphoton microscopy in biomedicine
Owing to its real-time, non-destructive, high-resolution imaging characteristics, MPM is considered uniquely advantageous in the study of digestive system tumors, skin diseases, and brains.
Yan et al. [62] investigated the tissue structure and cell morphology of Morris mouse hepatocellular carcinoma in situ and lung metastasis under the MPM system. They concluded that MPM can optically diagnose liver cancer and lung metastasis in real-time. Chen et al. [64] used the MPM system to image normal and tumoral pancreatic tissues. The comparison of the obtained multiphoton image with a standard H&E image (Figure 4B) indicates that the multiphoton image enables a clear observation of cancer cell nests, collagen fibers, a slime lake, and cancer cells. Therefore, MPM can serve as an efficient, environment-friendly, and sustainable alternative to H&E in the future. Liu et al. [65] observed a series of morphological characteristics of colonic mucinous adenocarcinoma through MPM and calculated the changes in the ratio of SHG to TPEF signals in normal and cancerous tissues. Finally, a fast Fourier transform chart was used to represent the degree of chaos between normal and cancerous tissue collagen. The aforementioned studies indicate that both qualitative and quantitative analyses can be performed using MPM. Kiss et al. [66] used the MPM technology to evaluate the skin of Ehlers–Danlos syndrome (EDS) and determined that although the healthy skin significantly differs from the EDS skin in terms of collagen fiber structure and content, their elastin contents are similar. Balu et al. [67] analyzed common skin diseases, such as vitiligo and melasma using MPM. They reported that the changes in melanin in patients with vitiligo under different disease states and the severe elastic deformation of melasma can be efficiently observed using MPM. This implies that MPM can serve as a guide for the clinical treatment of skin diseases.
2.2.4 Optical coherence microscopy
2.2.4.1 Principle of optical coherence microscopy
With the rapid development of biomedicine and the need for clinical surgery, understanding the internal microstructures of biological high-scattering deep tissues is extremely essential. However, laser confocal scanning and near-field optical microscopes can only image relatively transparent tissues and cannot obtain clear images of high-scattering deep tissues. In 1994, Izatt et al. [68] proposed OCM as a new technology developed by combining confocal microscopy and low-coherence interference techniques. The OCM system was designed to achieve microscopy of highly scattering deep biological tissues [69]; Figure 5A depicts the system [68]. As indicated in the figure, the system uses a single-mode fiber Michelson interferometer. The light emitted by the broadband light source, namely the 30-nm full-width half-maximum superluminescent diodes, is divided into two beams by a coupler. The beams are then passed through the reference and sample arms and reflected from the reference mirror and sample, respectively. Subsequently, the two reflected lights converge at the coupler. Interference occurs when the length of the optical path difference between the two reflected lights is less than the coherence length of the light source. The interference signal is received by a detector and output to a demodulator, which is collected by an analog-to-digital converter to complete the imaging process.
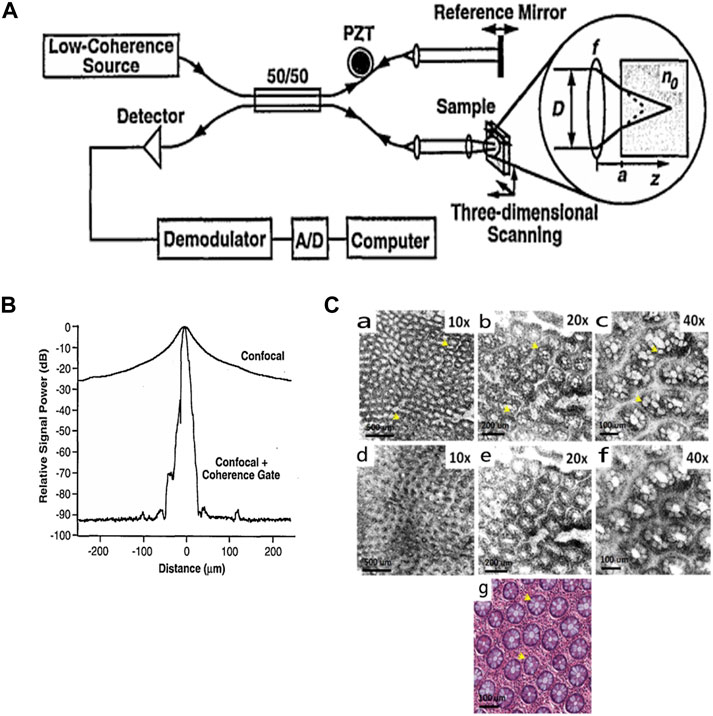
FIGURE 5. (A) Schematic of the optical coherence microscopy (OCM) system [70]. (B) Relative signal power of OCM and confocal microscopy as the displacement of the mirror changes. (NA = 0.4, 20x objective lens) [70] (C) (a–c) Ex vivo OCM images and the corresponding (d–f) simulated confocal images and (g) histology of fresh human colon specimen at different magnifications. Yellow arrows indicate goblet cells [16].
The lateral resolution of the OCM system is determined by the lateral resolution of the sample arm confocal microscope [70], whereas the axial resolution is determined by the autocorrelation product of the focusing objective lens and light source field. Confocal microscopy imaging is performed using point probe point scanning. Therefore, the detector does not receive stray light from outside the plane of the focal area, which significantly improves the image resolution. Confocal microscopy imaging cannot obtain clear images of thick scattering tissues as it fails to suppress scattered light that is longitudinally far from the focal region. The ability of an optical system to suppress scattered light outside the focal region can be expressed using a point spread function. The sharper the point spread function, the stronger the ability to suppress the scattered light. Figure 5B depicts the comparison of the point spread function between the OCM and confocal microscopy systems [68], with and without a coherent gate. The origin O in the figure is equivalent to the focal point. The sensitivity of the OCM with a coherent gate to the backscattered light decreases exponentially, whereas the sensitivity of the confocal microscopy to the backscattered light decreases gradually with respect to the distance from the focus [72]. Therefore, OCM can suppress scattered light substantially better than confocal microscopy [71].
2.2.4.2 Relationship between OCM and optical coherence tomography
In general, OCM can be regarded as a simple transformation of OCT. Although their imaging principle and structure are identical, the numerical aperture (NA) of the objective lens is different. When using a low-NA objective lens, the system exhibits a longer focal depth and lower lateral resolution, which serves as the OCT system. When a high-NA objective lens is used, the system exhibits a shorter focal depth and higher lateral resolution, serving as the OCM system [72]. In comparison with the OCT system, the OCM system enables the expansion of the imaging object from the tissue level to the cell level [73, 74].
As OCM systems with high-NA objective lenses limit the range of the focal depth, dynamic focusing technology must be used [35]; however, this in turn limits the speed of imaging. To improve the imaging speed, Dubois et al. proposed a full-field OCT system [75, 76] in 1998, which uses a high-NA objective lens to achieve higher lateral resolution and a heat source halogen lamp to improve the vertical resolution. In comparison with the fiber-type OCM, full-field OCM requires only a detector and wide-field illumination to perform a single parallel detection and complete an x–y plane imaging without horizontal scanning. Although full-field OCM imaging is theoretically faster, the use of phase shift algorithms and limitations of the detector sensitivity and light source power indicates that the full-field OCM system has no advantage in terms of imaging speed. A line-scan OCM system based on a broadband titanium sapphire laser and a line-scan charge-coupled device camera can satisfy high spatial resolution and achieve rapid imaging at the cell level in vivo [77].
2.2.4.3 Research status of optical coherence microscopy in biomedicine
As a imaging technique with high spatial resolution and imaging depth, OCM can image high scattering media and is significant for three-dimensional (3D) imaging of biological tissues [78].
After the introduction of the OCM system in 1994, Izatt et al. [69] used the OCM system in 1996 for the first time to achieve cell-level microstructure imaging of high-scattering tissues, up to several hundred microns, in in vitro gastrointestinal structures. In 2010, Choi et al. [13] used a refractive index (RI) contrast imaging method to identify living cancer and normal cells, which was confirmed using a super-resolution full-field optical coherence microscope. As cancer cells exhibit higher RI values than normal cells, this method is suitable for the detection of precancerous lesions and invasive cancer changes. In 2011, Lee et al. [79] adopted the Gabor domain OCM system to complete the volume imaging of the epithelial cells on the skin of a human finger. The lateral and axial resolutions were 2 μm, and the imaging speed was 23K A-scans/s. The sensitivity decreased gradually with the increasing imaging depth, with the highest sensitivity reaching 96 dB. The imaging depth was up to 1 mm [24], which proved its potential in 3D imaging. In 2013, Ahsen et al. [16] used a frequency-scanning optical coherent microscopic imaging system to perform 3D imaging of the colon, thyroid, kidney, and other biological tissues. The measured lateral resolution was between 0.86 and 3.42 µm, axial resolution was 8.1 µm, and depth was less than 150 µm. Figure 5C depicts the human fresh colon tissue at different magnifications, where goblet cells are clearly visible. In 2015, Min et al. [80] used wide-field OCM to image brain slices of mice. By comparing OCM images with tissue slice images stained with Nissl and Luxol fast blue, they determined that the corpus callosum, caudoputamen, and cerebral peduncle regions exhibited better fiber bundle contrast. As the light scattering of myelin fibrous lipids is stronger than the surrounding tissue, wide-field OCM can be used to analyze the direction of fiber bundles in the brain, serving as a highly promising tool in neuroscience research. In 2019, Lichtenegger et al. [81] proposed a multimodal optical OCM and fluorescence imaging (FI) system to image intraoperative brain tumor biopsies, revealing the three-dimensional structure of brain parenchyma. In the same year, Tankam et al. [82] revealed changes in keratocytes size and reflectivity based on Gabor-domain optical coherence microscopy (GD-OCM) to determine the microstructure of the corneal layer during surgery.
3 Advantages and disadvantages of various microscopy techniques
Complete resection of tumors is a vital factor affecting the prognosis of patients with cancer. Ideally, the edge of the tumor should be entirely within the resected tumor and deeper than the edge of the operation. However, doctors may leave certain invisible cancer cells in the body during the actual surgery, which may lead to a high recurrence rate and poor prognosis for patients. Therefore, obtaining accurate intraoperative pathological information using professional tools is extremely essential for the success of tumor resection, which affects the formulation of surgical plans. At present, several detection tools exist for tumor diagnosis with their unique characteristics. They can be compared in terms of spatial resolution, penetration depth, biological tissue information, and the advantages and disadvantages of the detection tool itself.
SRS is a molecular vibration microscopy imaging technology, which does not require sample labeling and can avoid problems such as phototoxicity and photobleaching caused by fluorescent labeling. Additionally, SRS enhances the Raman signal via the excitation process, which significantly reduces the data acquisition time and even ensures video-level imaging speed [18]. Moreover, the intensity of the SRS signal is directly proportional to the number of chemical bonds detected in the biological tissue, which can be directly used for the quantitative analysis, reducing the time required for the information extraction process. At present, the spatial resolution of SRS has been limited to approximately 300 nm [6, 42], and the imaging depth is 0.5 mm [12]. As the detection signal of the SRS is identical to that of the laser wavelength, its intensity is only one ten-thousandth of the laser intensity or weaker. Therefore, the detection of the SRS signal is difficult under the background of a strong laser. In 2011, Freudiger et al. [83] proposed an imaging method based on the spectral modulation of a broadband pump beam at high frequencies (>1 MHz). This enables detection of narrowband Stokes beam with high sensitivity SRS signal. PAM is the combination of AR-PAM and OR-PAM. OR-PAM has a spatial resolution of 0.032 µm [84], and the imaging depth is approximately 1.3 mm [6]. Conversely, AR-PAM has a low spatial resolution of only 15 µm with an extremely deep imaging depth of up to approximately 3 mm [85, 86], which can provide more comprehensive information. AR-PAM system using the dark field illumination exhibits a high SNR when imaging biological tissues; consequently, image artifacts are not generated. AR-PAM and OR-PAM use the optical absorption characteristics of biological tissues as the source of contrast without requiring any staining. Moreover, they can achieve selective excitation of highly specific spectral tissues, which facilitates functional imaging and reflects the characteristics of the tissue structure. However, they require water for coupling during the imaging process, which inconveniences the operation. In 2016, Lee et al. [86] employed a self-made needle sensor to directly contact the coupling gel on the sample to obtain the PA signal. Thus, the coupling tank that previously hindered surgical procedures is eliminated, and the transducer contact area near the surgical field is minimized. MPM is a non-linear optical microscopy imaging technology with a spatial resolution of approximately 200 nm. The maximum imaging depth is approximately 1.6–2.1 mm [1], which can facilitate 3D imaging. The multi-photon excitation source of MPM is located in the near-infrared region, which is less cytotoxic and photobleached [89]. Furthermore, as multi-photon excitation is limited to the focal point, pinholes are not necessary to collect the scattered light, which improves both fluorescence detection efficiency and SNR [60]. Moreover, MPM can image the collagen fibers that are used to evaluate the development of diseases [89], whereas standard histopathology is not sufficient to observe the subtle changes in collagen fibers. Nevertheless, MPM exhibits certain disadvantages. For instance, as the imaging field of view is relatively small, MPM is weak in scanning large-area tumors. However, in 2020, Chen et al. [90] used MPM to image breast cancer cells and automatically spliced them through LSM software to form a large area image. Furthermore, multiphoton excitation requires expensive femtosecond lasers, which increases the cost of the system. OCM is an emerging microscopy imaging technology with a lateral resolution of approximately 1,300 nm and an imaging depth of approximately 2.3 mm [16]. The OCM system can effectively suppress the scattered light outside the focus area and obtain a clear image of thick scattering tissues. Additionally, a full-field OCM system with a halogen lamp as the thermal light source can suppress crosstalk and speckle. Owing to the wide bandwidth of the halogen lamp, the full-field OCM system can also provide ultra-high vertical resolution. However, common OCM systems exhibit slower imaging speeds, and achieving the purpose of real-time imaging becomes difficult. However, in 2010, Aguirre et al. [91] provided an excellent option for high-speed OCM imaging using either acousto-optic (AO) or electro-optic (EO) modulators.
A significant surgical objective for tumor resection is to ensure that no residual cancer cells remain in the surgical cavity. To this end, developing a microscopy imaging technology that can aid in real-time observations of the surgical cavity surface is crucial. However, the existing single-mode detection method cannot obtain comprehensive information about the organization. Therefore, multi-mode hybrid detection technology is the future development of surgery, which can detect tumor edges more effectively to better explain the biological tissue information and achieve improved results. Based on the analysis and comparison of the aforementioned four types of microscopy imaging technologies, we propose a novel multi-mode hybrid detection technology, namely the MPM/AR-PAM hybrid detection technology. In comparison with other imaging technologies, although AR-PAM exhibits low spatial resolution, its imaging depth is the largest (up to approximately 3 mm), which can provide comprehensive structural information. Additionally, although the imaging depth of MPM is limited because of the filtering effect [92, 93], its spatial resolution is close to the limit of optical diffraction; therefore, accurate biological information on tissues can be obtained. The combination of these two imaging technologies achieves complementary advantages, which enables large-scale observation and diagnosis of the tumor edge, thereby ensuring the complete resection of the tumor. Photoacoustic (PA) imaging can provide physiological information such as hemoglobin concentration, angiogenesis, and structural information [94]. MPM is used in the biomedical field to image tumor tissues found in the body, such as in the breast [89], colon [95], and stomach [96]. The wide wavelength tuning range (350–750 nm) of the PAM laser overlaps the MPM system range. This enables the acquisition of various functional information for targeted imaging [94]. Therefore, applying MPM/AR-PAM hybrid detection technology to the imaging of tumor tissue will further improve the imaging depth, enrich tissue information, and expand the clinical applicability. In addition to ensuring complete tumor resections during surgeries, the proposed multi-mode hybrid detection technology can significantly contribute to the investigation of the development process of tumors. In 2014, Rao et al. demonstrated for the first time the MPM/AR-PAM hybrid detection technique [97], providing a platform for future biological and medical discoveries. In 2019, Liu et al. [98] reported that the hybrid detection technique is effective for observing model organisms such as zebrafish, in vivo imaging of normal mouse ears, and an implanted xenograft tumor in mouse ears. It is also essential in the evaluation of oncology drugs, tumor angiogenesis, and medication resistance. Typically, once a single change occurs in a tumor, the blood vessels surrounding the tumor also change. As AR-PAM is highly sensitive to blood vessels with a large imaging depth, its combination with MPM for accurate localization and detailed observation can provide a better understanding of the development of tumors. Although both the detection tools, MPM and AR-PAM, are in the state of clinical application, the MPM/AR-PAM hybrid detection technology is expected to be the focus of tissue detection technology research in the future owing to its unique advantages.
At present, AI based on deep learning is rapidly developing in biomedicine, particularly in pathological diagnosis. Highly efficient AI algorithms can surpass even well-trained pathologists. SRS, PAM, MPM, and OCM are integrated with AI algorithms and can be applied to cancer diseases such as brain tumors [99], breast cancer [100], colorectal cancer [95], and oral cancer [101]. Because each optical imaging modality has fundamental capabilities and limitations, there are exciting opportunities to merge techniques into multimodal approaches. The capabilities of one modality can complement and overcome the limitations of the other [102]. During the operation of tumor resection, the multi-mode hybrid detection technology can be used for rapid and effective extraction of the tumor boundary image, and the AI algorithm [102] can be processed to obtain reliable information on the tumor boundary in time. This provides more information for the surgeon to make surgically appropriate decisions.
4 Conclusion
This study primarily outlines the four microscopy imaging technologies, namely SRS, PAM, MPM, and OCM, which are expected to be used for rapid intraoperative diagnosis in the future. Their existing research statuses in the biomedical field are also analyzed. Additionally, a multi-mode hybrid detection technology is proposed based on the characteristics of the four microscopy imaging technologies, referred to as the MPM/AR-PAM hybrid detection technology.
In terms of imaging technology, the spatial resolutions of SRS, PAM (AR-PAM and OR-PAM), MPM, and OCM are of the order of micrometers or even sub-micrometers. Among them, the spatial resolution of MPM can attain the optical diffraction limit. The imaging depths of SRS, MPM, OCM, and OR-PAM typically do not exceed the “soft limit” of the traditional optical imaging depth of 1 mm. Although certain experiments of MPM, OCM, and OR-PAM have crossed the limit of 1 mm [6, 12, 11], AR-PAM exhibits an imaging depth of 3 mm [8]. To achieve more accurate tumor boundary exploration during the surgery, the advantages of MPM and AR-PAM can be combined to form the MPM/AR-PAM hybrid detection technology. Herein, AR-PAM scans the tissue to determine the approximate location of the tumor boundary [103], and MPM achieves precise positioning of tumor boundaries to ensure complete resection. In terms of applications, SRS, PAM (AR-PAM and OR-PAM), MPM, and OCM have been researched in terms of diseases in different body parts; several studies report that they can provide morphological, metabolic, and functional information at different stages of cancer treatment. For instance, PAM (AR-PAM and OR-PAM) is widely used in the detection of hemoglobin, melanin, and lipids with strong optical absorption characteristics. MPM is commonly used in the research on digestive system tumors, skin diseases, and brain tumors. At present, various imaging technologies are being developed in the direction of multi-mode, multi-function, and integration of diagnosis and treatment to satisfy the needs of biomedical applications. Additionally, various deep learning and AI algorithms are continuously optimized. However, several problems need to be addressed for them to be applied in clinical practices. For instance, the integration of two or more imaging modes should be improved to realize the miniaturization of equipment without affecting the system performance. Furthermore, obtaining real-time images with higher definition using image algorithms must be explored. Finally, improving the optimizer algorithm and reducing its running time should be investigated further.
With the development of various microscopy imaging technologies, deep learning, and AI, their role in the biomedical field has become prominent. Particularly, the multi-mode hybrid detection technology combined with AI is expected to be a novel technology that provides real-time pathological information for clinical surgeries, which can revolutionize biological imaging and clinical diagnoses.
Author contributions
HZ, and CS wrote the first draft of the manuscript. MX, TL, GW, GX, ZL, and SZ wrote sections of the manuscript. ZL, and SZ provide technical guidance.
Funding
This work was supported by the National Key Research and Development Program of China (2019YFE0113700), and the Joint Funds of Fujian Provincial Health and Education Research (2019-WJ-21).
Conflict of interest
The authors declare that the research was conducted in the absence of any commercial or financial relationships that could be construed as a potential conflict of interest.
Publisher’s note
All claims expressed in this article are solely those of the authors and do not necessarily represent those of their affiliated organizations, or those of the publisher, the editors and the reviewers. Any product that may be evaluated in this article, or claim that may be made by its manufacturer, is not guaranteed or endorsed by the publisher.
References
1. Bi Y Y, Yang C, Chen Y, Yan S, Yang G, Wu Y, et al. Near-resonance enhanced label-free stimulated Raman scattering microscopy with spatial resolution near 130 nm. Light Sci Appl (2018) 7(1):81–10. doi:10.1038/s41377-018-0082-1
2. Li J, Lin P, Tan Y, Cheng JX. Volumetric stimulated Raman scattering imaging of cleared tissues towards three-dimensional chemical histopathology. Biomed Opt Express (2019) 10(8):4329–39. doi:10.1364/boe.10.004329
3. Lu FK, Ji M, Fu D, Ni X, Freudiger CW, Holtom G, et al. Multicolor stimulated Raman scattering microscopy. Mol Phys (2012) 110:1927–32. doi:10.1080/00268976.2012.695028
4. Mittal R, Balu M, Krasieva T, Potma EO, Elkeeb L, Zachary CB, et al. Evaluation of stimulated Raman scattering microscopy for identifying squamous cell carcinoma in human skin. Lasers Surgery Med. (2013) 45 (8):496–502.
5. Maslov K, Zhang HF, Hu S, Wang LV. Optical-resolution photoacoustic microscopy for in vivo imaging of single capillaries. Opt Lett (2008) 33(9):929–31. doi:10.1364/ol.33.000929
6. Xing W, Wang L, Maslov K, Wang LV. Integrated optical-and acoustic-resolution photoacoustic microscopy based on an optical fiber bundle. Opt Lett (2013) 38(1):52–4. doi:10.1364/ol.38.000052
7. Lin R, Chen J, Wang H, Yan M, Zheng W, Song L. Longitudinal label-free optical-resolution photoacoustic microscopy of tumor angiogenesis in vivo. Quant Imaging Med Surg (2015) 5(1):23–9. doi:10.3978/j.issn.2223-4292.2014.11.08
8. Maslov K, Stoica G, Wang LV. In vivo dark-field reflection-mode photoacoustic microscopy. Opt Lett (2005) 30(6):625–7. doi:10.1364/ol.30.000625
9. Hu S, Maslov K, Wang LV. Noninvasive label-free imaging of microhemodynamics by optical-resolution photoacoustic microscopy. Opt Express (2009) 17(9):7688–93. doi:10.1364/oe.17.007688
10. Elagin V, Gubarkova E, Garanina O, Davydova D, Orlinskaya N, Matveev L, et al. In vivo multimodal optical imaging of dermoscopic equivocal melanocytic skin lesions. Sci Rep (2021) 11(1):1405–12. doi:10.1038/s41598-020-80744-w
11. Liu H, Deng X, Tong S, He C, Cheng H, Zhuang Z, et al. In vivo deep-brain structural and hemodynamic multiphoton microscopy enabled by quantum dots. Nano Lett (2019) 19(8):5260–5. doi:10.1021/acs.nanolett.9b01708
12. Yamanaka M, Hayakawa N, Nishizawa N. Signal-to-background ratio and lateral resolution in deep tissue imaging by optical coherence microscopy in the 1700 nm spectral band. Sci Rep (2019) 9(1):16041–8. doi:10.1038/s41598-019-52175-9
13. Choi WJ, Jeon DI, Ahn SG, Yoon JH, Kim S, Lee BH. Full-field optical coherence microscopy for identifying live cancer cells by quantitative measurement of refractive index distribution. Opt Express (2010) 18(22):23285–95. doi:10.1364/oe.18.023285
14. Bakhshandeh M, Tutuncuoglu SO, Fischer G, Masood S. Use of imprint cytology for assessment of surgical margins in lumpectomy specimens of breast cancer patients. Diagn Cytopathol (2007) 35(10):656–9. doi:10.1002/dc.20704
15. Hu S, Maslov K, Wang LV. Second-generation optical-resolution photoacoustic microscopy with improved sensitivity and speed. Opt Lett (2011) 36(7):1134. doi:10.1364/ol.36.001134
16. Ahsen OO, Tao YK, Potsaid BM, Sheikine Y, Jiang J, Grulkowski I, et al. Swept source optical coherence microscopy using a 1310 nm VCSEL light source. Opt Express (2013) 21(15):18021. doi:10.1364/oe.21.018021
17. Orringer DA, Pandian B, Niknafs YS, Hollon TC, Boyle J, Lewis S, et al. Rapid intraoperative histology of unprocessed surgical specimens via fibre-laser-based stimulated Raman scattering microscopy. Nat Biomed Eng (2017) 1(2):0027. doi:10.1038/s41551-016-0027
18. Evans CL, Potma EO, Puoris’ Haag M, Xie XS. Chemical imaging of tissue in vivo with video-rate coherent anti-Stokes Raman scattering microscopy. Proc Natl Acad Sci U S A (2005) 102(46):16807–12. doi:10.1073/pnas.0508282102
19. Wang PH, Luh JJ, Chen WS, Li ML. In vivo photoacoustic micro-imaging of microvascular changes for Achilles tendon injury on a mouse model. Biomed Opt Express (2011) 2(6):1462–9. doi:10.1364/boe.2.001462
20. Ehteshami Bejnordi B, Veta M, Johannes van Diest P, van Ginneken B, Karssemeijer N, Litjens G, et al. Diagnostic assessment of deep learning algorithms for detection of lymph node metastases in women with breast cancer. JAMA (2017) 318(22):2199. doi:10.1001/jama.2017.14585
21. Saar BG, Zeng Y, Freudiger CW, Liu YS, Himmel M, Xie X, et al. Label-free, real-time monitoring of biomass processing with stimulated Raman scattering microscopy. Angew Chem Int Ed Engl (2010) 49(32):5608–11. doi:10.1002/ange.201000900
22. Notingher I. Raman spectroscopy cell-based biosensors. Sensors (2007) 7(8):1343–58. doi:10.3390/s7081343
23. Müller M, Schins JM. Imaging the thermodynamic state of lipid membranes with multiplex CARS microscopy. J Phys Chem B (2002) 106(14):3715–23. doi:10.1021/jp014012y
24. Wang P, Liu B, Zhang D, Belew MY, Tissenbaum HA, Cheng JX. Imaging lipid metabolism in live Caenorhabditis elegans using fingerprint vibrations. Angew Chem Int Ed Engl (2014) 53(44):11981–6. doi:10.1002/ange.201406029
25. Cheng Q, Miao Y, Wild J, Min W, Yang Y. Emerging applications of stimulated Raman scattering microscopy in materials science. Matter (2021) 4(5):1460–83. doi:10.1016/j.matt.2021.02.013
26. Raman CV. A change of wave-length in light scattering. Nature (1928) 21(3051):619. doi:10.1038/121619b0
28. Eckhardt G, Hellwarth RW, McClung FJ, Schwarz SE, Weiner D, Woodbury EJ. Stimulated Raman scattering from organic liquids. Phys Rev Lett (1962) 9(11):455–7. doi:10.1103/physrevlett.9.455
29. Freudiger CW, Min W, Saar BG, Lu S, Holtom GR, He C, et al. Label-free biomedical imaging with high sensitivity by stimulated Raman scattering microscopy. Science (2008) 322(5909):1857–61. doi:10.1126/science.1165758
30. Wei L, Yu Y, Shen Y, Wang MC, Min W. Vibrational imaging of newly synthesized proteins in live cells by stimulated Raman scattering microscopy. Proc Natl Acad Sci U S A (2013) 110(28):11226–31. doi:10.1073/pnas.1303768110
31. Cheng JX, Xie XS. Vibrational spectroscopic imaging of living systems: An emerging platform for biology and medicine. Science (2015) 350(6264):aaa8870. doi:10.1126/science.aaa8870
32. Cheng JX, Xie XS. Coherent anti-Stokes Raman scattering microscopy: Instrumentation, theory, and applications. J Phys Chem B (2004) 108(3):827–40. doi:10.1021/jp035693v
33. Lu FK, Calligaris D, Olubiyi OI, Norton I, Yang W, Santagata S, et al. Labelfree neurosurgical pathology with stimulated Raman imaging. Cancer Res. (2016) 76(12):3451–3462. doi:10.1158/0008-5472.can-16-0270
34. Li J, Cheng JX. Direct visualization of de novo lipogenesis in single living cells. Sci Rep (2014) 4(1):6807–8. doi:10.1038/srep06807
35. Wang MC, Min W, Freudiger CW, Ruvkun G, Xie XS. RNAi screening for fat regulatory genes with SRS microscopy. Nat Methods (2011) 8(2):135–8. doi:10.1038/nmeth.1556
36. Shen Y, Zhao Z, Zhang L, Shi L, Shahriar S, Chan RB, et al. Metabolic activity induces membrane phase separation in endoplasmic reticulum. Proc Natl Acad Sci U S A (2017) 114(51):13394–9. doi:10.1073/pnas.1712555114
37. Fu D, Zhou J, Zhu WS, Manley PW, Wang YK, Hood T, et al. Imaging the intracellular distribution of tyrosine kinase inhibitors in living cells with quantitative hyperspectral stimulated Raman scattering. Nat Chem (2014) 6(7):614–22. doi:10.1038/nchem.1961
38. Freudiger CW, Pfannl R, Orringer DA, Saar BG, Ji M, Zeng Q, et al. Erratum: Multicolored stain-free histopathology with coherent Raman imaging. Lab Invest (2012) 92(11):1661. doi:10.1038/labinvest.2012.139
39. Ji M, Lewis S, Camelo-Piragua S, Ramkissoon SH, Snuderl M, Venneti S, et al. Detection of human brain tumor infiltration with quantitative stimulated Raman scattering microscopy. Sci Transl Med (2015) 7(309):309ra163. 309ra163-309ra163. doi:10.1126/scitranslmed.aab0195
40. Zhang L, Wu Y, Zheng B, Su L, Chen Y, Ma S, et al. Rapid histology of laryngeal squamous cell carcinoma with deep-learning based stimulated Raman scattering microscopy. Theranostics (2019) 9(9):2541–54. doi:10.7150/thno.32655
41. Yin B, Xing D, Wang Y, Zeng Y, Tan Y, Chen Q. Fast photoacoustic imaging system based on 320-element linear transducer array. Phys Med Biol (2004) 9(7):1339–46. doi:10.1088/0031-9155/49/7/019
42. Beard P. Biomedical photoacoustic imaging. Interf Focus (2011) 1(4):602–31. doi:10.1098/rsfs.2011.0028
43. Yoon TJ, Cho YS. Recent advances in photoacoustic endoscopy. World J Gastrointest Endosc (2013) 5(11):534–9. doi:10.4253/wjge.v5.i11.534
44. Wang LV, Hu S. Photoacoustic tomography: In vivo imaging from organelles to organs. Science (2012) 335(6075):1458–62. doi:10.1126/science.1216210
45. Favazza CP, Jassim O, Cornelius LA, Wang LV. In vivo photoacoustic microscopy of human cutaneous microvasculature and a nevus. J Biomed Opt (2011) 16(1):016015. doi:10.1117/1.3528661
46. Park S, Lee C, Kim J, Kim C. Acoustic resolution photoacoustic microscopy. Biomed Eng Lett (2014) 4(3):213–22. doi:10.1007/s13534-014-0153-z
47. Oh JT, Li ML, Zhang HF, Maslov K, Stoica G, Wang LV. Three-dimensional imaging of skin melanoma in vivo by dual-wavelength photoacoustic microscopy. J Biomed Opt (2006) 11(3):034032. doi:10.1117/1.2210907
48. Hu S, Maslov K, Tsytsarev V, Wang LV. Functional transcranial brain imaging by optical-resolution photoacoustic microscopy. J Biomed Opt (2009) 14(4):040503. doi:10.1117/1.3194136
49. Jiao S, Jiang M, Hu J, Fawzi A, Zhou Q, Shung KK, et al. Photoacoustic ophthalmoscopy for in vivo retinal imaging. Opt Express (2010) 18(4):3967–72. doi:10.1364/oe.18.003967
50. Song SH, Wang LV. Deep reflection-mode photoacoustic imaging of biological tissue. J Biomed Opt (2007) 12(6):060503. doi:10.1117/1.2818045
51. Zhang HF, Maslov K, Stoica G, Wang LV, Sivaramakrishnan M. Imaging of hemoglobin oxygen saturation variations in single vessels in vivo using photoacoustic microscopy. Appl Phys Lett (2007) 90(5):053901. doi:10.1063/1.2435697
52. Hajireza P, Shi W, Zemp RJ. Real-time handheld optical-resolution photoacoustic microscopy. Opt Express (2011) 19(21):20097–102. doi:10.1364/oe.19.020097
53. Paoli J, Smedh M, Wennberg AM, Ericson MB. Multiphoton laser scanning microscopy on non-melanoma skin cancer: Morphologic features for future non-invasive diagnostics. J Invest Dermatol (2008) 128(5):1248–55. doi:10.1038/sj.jid.5701139
54. Wang S, Chen J, Yang Y, Jiang W, Feng C, Guan G, et al. Assessment of tumor invasion depth in colorectal carcinoma using multiphoton microscopy. IEEE Photon J (2015) 7(2):1–8. doi:10.1109/jphot.2015.2420615
55. Göppert-Mayer M. Über elementarakte mit zwei quantensprüngen. Ann Phys (1931) 401(3):273–94. doi:10.1002/andp.19314010303
56. Denk W, Strickler JH, Web WW. Two-photon laser scanning fluorescence microscopy. Science (1990) 248(4951):73–6. doi:10.1126/science.2321027
57. Bloembergen N. Encounters in nonlinear optics: Selected papers of nicolaas bloembergen (with commentary). World Scientific Ser 20th Century PhysicsEncounters Nonlinear Opt (1996) 338–73.
58. Zhang Z, Wu F, Hu J, Yang X, Gui J, Ji P, et al. The laser beamline in SULF facility. High Pow Laser Sci Eng (2020) 8:e4. doi:10.1017/hpl.2020.3
59. Brandt C (2022). p. 13–9.Endoscopic MPM objective designed for depth scanning. Endoscopic Microsc XVII. Bellingham, Washington, United States: SPIERefstyled
60. Squirrell JM, Wokosin DL, White JG, Bavister BD. Long-term two-photon fluorescence imaging of mammalian embryos without compromising viability. Nat Biotechnol (1999) 17(8):763–7. doi:10.1038/11698
61. Yan J, Zhuo S, Chen G, Milsom JW, Zhang H, Lu J, et al. Real-time optical diagnosis for surgical margin in low rectal cancer using multiphoton microscopy. Surg Endosc (2014) 28(1):36–41. doi:10.1007/s00464-013-3153-7
62. Yan J, Zhuo S, Chen G, Tan C, Zhu W, Lu J, et al. Use of multiphoton microscopy to diagnose liver cancer and lung metastasis in an orthotopic rat model. Scanning (2012) 34(4):271–7. doi:10.1002/sca.21005
64. Chen Y, Chen J, Chen H, Hong Z, Zhu X, Zhuo S, et al. Multiphoton microscopy as a diagnostic imaging modality for pancreatic neoplasms without hematoxylin and eosin stains. J Biomed Opt (2014) 19(9):096008. doi:10.1117/1.jbo.19.9.096008
65. Liu N, Chen J, Xu R, Jiang S, Xu J, Chen R. Label-free imaging characteristics of colonic mucinous adenocarcinoma using multiphoton microscopy. Scanning (2013) 35(4):277–82. doi:10.1002/sca.21063
66. Kiss N, Haluszka D, Lorincz K, Kuroli E, Harsing J, Mayer B, et al. Ex vivo nonlinear microscopy imaging of Ehlers–Danlos syndrome-affected skin Arch Dermatol Res (2018) 310(5):463–73. doi:10.1007/s00403-018-1835-7
67. Balu M, Lentsch G, Williams JN, Saknite I, Koenig K, Ganesan A, et al. (2017). .Vivo multiphoton microscopy imaging of pigmentary skin disorders. OmM3D, 3. Washington, United States: Optical Society of AmericaRefstyled
68. Joseph A, Swanson EA, Fujimoto JG, Hee MR, Owen GM. Optical coherence microscopy in scattering media. Opt Lett (1994) 19(8):590–2. doi:10.1364/ol.19.000590
69. Izatt JA, Kulkarni M, Hsing-Wen Wang , Kobayashi K, Sivak M. Optical coherence tomography and microscopy in gastrointestinal tissues. IEEE J Sel Top Quan Electron (1996) 2(4):1017–28. doi:10.1109/2944.577331
70. Laaziz Y, Bennouna A, Elazhari M, Ramiro-Bargueno J, Outzourhit A, Chahboun N, et al. A method for monitoring the thickness of semiconductor and dielectric thin films: Application to the determination of large-area thickness profiles. Thin Solid Films (1997) 303(1-2):255–63. doi:10.1016/s0040-6090(97)00044-8
71. Kimura S, Wilson T. Confocal scanning optical microscope using single-mode fiber for signal detection. Appl Opt (1991) 30(16):2143–50. doi:10.1364/ao.30.002143
72. Dabarsyah B. Interferometric synthetic aperture microscopy (ISAM) reconstruction and characterization in a high numerical aperture system. Women S Hist Rev (2012) 20(4):555–67.
73. Sticker M, Pircher M, Gotzinger E, Sattmann H, Fercher AF, Hitzenberger CK. En face imaging of single cell layers by differential phase-contrast optical coherence microscopy. Opt Lett (2002) 27(13):1126–8. doi:10.1364/ol.27.001126
74. Aguirre AD, Hsiung P, Ko TH, Hartl I, Fujimoto JG. High-resolution optical coherence microscopy for high-speed, in vivo cellular imaging. Opt Lett (2003) 28(21):2064–6. doi:10.1364/ol.28.002064
75. Schmitt JM. Optical coherence tomography (OCT): A review. IEEE J Sel Top Quan Electron (1999) 5(4):1205–15. doi:10.1109/2944.796348
76. Dubois A, Boccara AC, Lebec M, Blanchot L, Saint-Jalmes H. Full-field optical coherence microscopy. Opt Lett (1998) 23(4):244. doi:10.1364/ol.23.000244
77. Chen Y, Huang SW, Aguirre AD, Fujimoto JG. High-resolution line-scanning optical coherence microscopy. Opt Lett (2007) 32(14):1971–3. doi:10.1364/ol.32.001971
78. Zhou C, Wang Y, Aguirre AD, Tsai TH, Cohen DW, Connolly JL, et al. Ex vivo imaging of human thyroid pathology using integrated optical coherence tomography and optical coherence microscopy. J Biomed Opt (2010) 15(1):016001. doi:10.1117/1.3306696
79. Lee KS, Thompson KP, Meemon P, Rolland JP. Cellular resolution optical coherence microscopy with high acquisition speed for in-vivo human skin volumetric imaging. Opt Lett (2011) 36(12):2221–3. doi:10.1364/ol.36.002221
80. Eunjung M, Lee J, Vavilin A, Jung S, Shin S, Kim J, et al. Wide-field optical coherence microscopy of the mouse brain slice. Opt Lett (2015) 40(19):4420–3. doi:10.1364/ol.40.004420
81. Lichtenegger A, Gesperger J, Kiesel B, Muck M, Eugui P, Harper DJ, et al. Revealing brain pathologies with multimodal visible light optical coherence microscopy and fluorescence imaging. J Biomed Opt (2019) 24(6):1. doi:10.1117/1.jbo.24.6.066010
82. Tankam P, He Z, Thuret G, Hindman HB, Canavesi C, Escudero JC, et al. Capabilities of Gabor-domain optical coherence microscopy for the assessment of corneal disease. J Biomed Opt (2019) 24(4):1–17. doi:10.1117/1.JBO.24.4.046002
83. Freudiger CW, Min W, Holtom GR, Xu B, Dantus M, Sunney Xie X. Highly specific label-free molecular imaging with spectrally tailored excitation-stimulated Raman scattering (STE-SRS) microscopy. Nat Photon (2011) 5(2):103–9. doi:10.1038/nphoton.2010.294
84. Zhang HF, Wang J, Wei Q, Liu T, Jiao S, Puliafito CA. Collecting back-reflected photons in photoacoustic microscopy. Opt Express (2010) 18(2):1278–82. doi:10.1364/oe.18.001278
85. Song KH, Stein EW, Margenthaler JA, Wang LV. Noninvasive photoacoustic identification of sentinel lymph nodes containing methylene blue in vivo in a rat model. J Biomed Opt (2008) 13(5):054033. doi:10.1117/1.2976427
86. Lee D, Lee C, Kim S, Zhou Q, Kim J, Kim C. In vivo near infrared virtual intraoperative surgical photoacoustic optical coherence tomography. Sci Rep (2016) 6(1):35176–10. doi:10.1038/srep35176
87. Horton NG, Wang K, Kobat D, Clark CG, Wise FW, Schaffer CB, et al. In vivo three-photon microscopy of subcortical structures within an intact mouse brain. Nat Photon (2013) 7(3):205–9. doi:10.1038/nphoton.2012.336
88. König K. Multiphoton microscopy in life sciences. J Microsc (2000) 200(2):83–104. doi:10.1046/j.1365-2818.2000.00738.x
89. Hovhannisyan AZ, Hovhannisyan VA, Dong CY. Hypericin-mediated destruction of collagen fibers revealed by multiphoton microscopy. Int J Mod Phys Conf Ser (2012) 15:157–60. doi:10.1142/s201019451200709x
90. Chen Z. Label-free identification of early stages of breast ductal carcinoma via multiphoton microscopy. Scanning (2020):9670514.
91. Aguirre AD, Sawinski J, Huang SW, Zhou C, Denk W, Fujimoto JG. High speed optical coherence microscopy with autofocus adjustment and a miniaturized endoscopic imaging probe. Opt Express (2010) 18(5):4222–39. doi:10.1364/oe.18.004222
92. Lacomb R, Nadiarnykh O, Campagnola PJ. Quantitative second harmonic generation imaging of the diseased state osteogenesis imperfecta: Experiment and simulation. Biophysical J (2008) 94(11):4504–14. doi:10.1529/biophysj.107.114405
93. Lacomb R, Nadiarnykh O, Carey S, Campagnola PJ. Quantitative second harmonic generation imaging and modeling of the optical clearing mechanism in striated muscle and tendon. J Biomed Opt (2008) 13(2):021109. doi:10.1117/1.2907207
94. Kim J, Lee D, Jung U, Kim C. Photoacoustic imaging platforms for multimodal imaging. Ultrasonography (2015) 34(2):88–97. doi:10.14366/usg.14062
95. Zhuo S, Yan J, Chen G, Chen J, Liu Y, Lu J, et al. Label-free monitoring of colonic cancer progression using multiphoton microscopy. Biomed Opt Express (2011) 2(3):615–9. doi:10.1364/boe.2.000615
96. Yan J, Chen G, Chen J, Liu N, Zhuo S, Yu H, et al. A pilot study of using multiphoton microscopy to diagnose gastric cancer. Surg Endosc (2011) 25(5):1425–30. doi:10.1007/s00464-010-1409-z
97. Rao B, Soto F, Kerschensteiner D, Wang LV. Integrated photoacoustic, confocal, and two-photon microscope. J Biomed Opt (2014) 19(3):036002. doi:10.1117/1.jbo.19.3.036002
98. Liu C, Liao J, Chen L, Chen J, Ding R, Gong X, et al. The integrated high-resolution reflection-mode photoacoustic and fluorescence confocal microscopy. Photoacoustics (2019) 14:12–8. doi:10.1016/j.pacs.2019.02.001
99. Reinecke D, von Spreckelsen N, Mawrin C, Ion-Margineanu A, Furtjes G, Junger ST, et al. Novel rapid intraoperative qualitative tumor detection by a residual convolutional neural network using label-free stimulated Raman scattering microscopy. Acta Neuropathol Commun (2022) 10(1):109–13. doi:10.1186/s40478-022-01411-x
100. Balasundaram G, Krafft C, Zhang R, Dev K, Bi R, Moothanchery M, et al. Biophotonic technologies for assessment of breast tumor surgical margins—a review. J Biophotonics (2021) 14(1):e202000280. doi:10.1002/jbio.202000280
101. Ilhan B, Lin K, Guneri P, Wilder-Smith P. Improving oral cancer outcomes with imaging and artificial intelligence. J Dent Res (2020) 99(3):241–8. doi:10.1177/0022034520902128
102. Bishop KW, Maitland KC, Rajadhyaksha M, Liu JTC. In vivo microscopy as an adjunctive tool to guide detection, diagnosis, and treatment. J Biomed Opt (2022) 27(4):040601. doi:10.1117/1.jbo.27.4.040601
Keywords: intraoperative microscopy, stimulated Raman scattering, photoacoustic microscopy, multiphoton microscopy, optical coherence microscopy
Citation: Zhan H, Sun C, Xu M, Luo T, Wang G, Xi G, Liu Z and Zhuo S (2022) Analysis of intraoperative microscopy imaging techniques and their future applications. Front. Phys. 10:991279. doi: 10.3389/fphy.2022.991279
Received: 11 July 2022; Accepted: 23 September 2022;
Published: 10 October 2022.
Edited by:
Yuye Ling, Shanghai Jiao Tong University, ChinaReviewed by:
Shu-Chi A. Yeh, University of Rochester, United StatesZhongjiang Chen, Fujian Medical University, China
Copyright © 2022 Zhan, Sun, Xu, Luo, Wang, Xi, Liu and Zhuo. This is an open-access article distributed under the terms of the Creative Commons Attribution License (CC BY). The use, distribution or reproduction in other forums is permitted, provided the original author(s) and the copyright owner(s) are credited and that the original publication in this journal is cited, in accordance with accepted academic practice. No use, distribution or reproduction is permitted which does not comply with these terms.
*Correspondence: Zhiyi Liu, bGl1emhpeWkwN0B6anUuZWR1LmNu; Shuangmu Zhuo, c2h1YW5nbXV6aHVvQGptdS5lZHUuY24=
†These authors have contributed equally to this work