- Institut de Physique des 2 Infinis de Lyon, Université Claude Bernard Lyon 1, Villeurbanne, France
Introduction: NanOx is a theoretical framework developed to predict cell survival to ionizing radiation in the context of radiotherapy. Based on statistical physics, NanOx takes the stochastic nature of radiation at different spatial scales fully into account. It extends concepts from microdosimetry to nanodosimetry, and considers as well the primary oxidative stress. This article presents in detail the general formalism behind NanOx.
Methods: Cell death induction in NanOx is modeled through two types of biological events: the local lethal events, modeled by the inactivation of nanometric sensitive targets, and the global events, represented by the toxic accumulation of oxidative stress and sublethal lesions. The model is structured into general premises and postulates, the theoretical bases compliant with radiation physics and chemistry, and into simplifications and approximations, which are required for its practical implementation.
Results: Calculations performed with NanOx showed that the energy deposited in the penumbra of ion tracks may be neglected for the low-energy ions encountered in some radiotherapy techniques, such as targeted radionuclide therapy. On the other hand, the hydroxyl radical concentration induced by ions was shown to be larger for low-LET ions and to decrease faster with time compared to photons. Starting from the general formalism of the NanOx model, an expression was derived for the cell survival to local lethal events in the track-segment approximation.
Discussion: The NanOx model combines premises of existing biophysical models with fully innovative features to consider the stochastic effects of radiation at all levels in order to estimate cell survival and the relative biological effectiveness of ions. The details about the NanOx model formalism given in this paper allow anyone to implement the model and modify it by introducing different approximations and simplifications to improve it, or even adapt it to other medical applications.
1 Introduction
Innovative radiotherapy techniques such as hadrontherapy, boron neutron capture therapy (BNCT) and targeted radionuclide therapy (TRT) exploit the advantages of ions for an improved irradiation of tumors.
From the radiobiological point of view ions have, due to their track structure, an enhanced efficacy in killing tumor cells, quantified through the relative biological effectiveness (RBE). The RBE associated with a radiation quality is defined as the ratio of the absorbed dose delivered by a reference radiation to the one delivered by a particle or by a given mixed field of different particles resulting in the same biological effect [1,2]. The RBE is a complex function which depends on physical parameters like the dose, the radiation type and energy, and on biological parameters such as the cell line, the cell cycle phase and the cell environment. It is normally assumed to be 1.1 along the whole path for protons [3], while for heavier ions it may be predicted with a biophysical model or via an empirical approach, e.g., from irradiation experiments with different cell lines [4,5].
Biophysical models are important tools often used to evaluate the biological effects of ionizing radiation in radiotherapy. In particular, two different biophysical models are currently implemented in the treatment planning systems (TPS) of hadrontherapy facilities all over the world: the first version of the local effect model (LEM) [6] and the modified microdosimetric kinetic model (mMKM) [7,8].
Improved versions of those models have been developed to solve some of their shortcomings [9–12]. Furthermore, other biophysical models have emerged as well in recent years proposing alternative frameworks to describe the radio-induced biological effects at different scales and using a variable number of free parameters [13–17].
In this context, we developed and published in 2017 [13] the NanOx biophysical model, a theoretical framework which gathered and combined the premises of some of the models proposed in the literature, while considering the stochastic nature of energy deposition down to the nanometric scale. The latter leads to a rigorous framework to describe radiation effects without, for instance, the need of non-Poissonian corrections.
Among the ideas that inspired us from the literature we can mention for instance the concept of local events defined in the three first versions of the LEM [6,9,10]. This notion was reused to describe processes leading to cell death due to energy deposition at the nanometric scale. However, as it was shown that the magnitude of fluctuations of such energy deposition cannot be disregarded [18], the local dose was replaced in NanOx by a stochastic quantity representing the physico-chemical mechanisms. This is in contrast to the latest version of the LEM [11,19], where the biological effect of ions is primarily linked to the initial distribution of DNA double-strand breaks (DSB) induced by radiation rather than the local dose itself. In addition, the LEM IV still applies the radial dose with an amorphous track model to describe the energy depositions by ions, without taking into account the significant stochastic effects in ion tracks. The LEM IV also introduces a second-order of spatiality (micrometer scale) beyond the nanometric scale, showing the relevance of accounting for both in comparison to experiments [20].
Moreover, as explained in [21,22], local lethal events alone do not allow to reproduce the shoulders in cell survival curves. For this reason, we introduced non-local lethal events in the NanOx model. The combination of “local” and “non-local” lethal events was inspired by the incorporation of the “ion-kill” and “γ-kill” components in the model developed by Katz et al. [23,24]. On the whole, NanOx is a framework that allows to predict cell survival to ionizing radiation consistently with the stochastic nature of the latter down to a nanometric scale. Since it takes into account not only the physical processes, but also the chemical ones, its name refers to the concepts of NANodosimetry and OXidative stress. Some predictions of this model for monoenergetic irradiations have already been published [13,25–28] and showed good agreement with experimental data.
While a short description of the NanOx main principles and postulates was given in [13], no exhaustive and complete presentation of the model had been published until now. Moreover, this model was initially developed for hadrontherapy [13,28]. The present paper provides the general theoretical framework so it can be applied to any radiotherapy technique, including BNCT and TRT. It is structured as follows. Section 2 presents the basic concepts and the detailed mathematical formalism behind the NanOx model. In Section 3, we report some relevant results derived from the NanOx model formalism, as well as an example of application for computing the cell survival probability to local lethal events within the range of validity of the track-segment approximation. Section 4 discusses the positioning of NanOx in respect to other biophysical models proposed in the literature. Finally, conclusions are drawn in Section 5.
2 Materials and methods
The formalism behind the NanOx model is detailed in this section. Our discussion follows a top-down approach, i.e., the general premises and main postulates of the model are presented first (Sections 2.1 and Section 2.2); then, the simplifications and approximations necessary for the practical implementation of the model are introduced (Section 2.3). Lastly, a summary of the parameters required for NanOx calculations is provided in Section 2.4.
2.1 General premises of the NanOx model
The main premise of the NanOx model is that cell survival is the result of cell response to two types of biological events that take place in the sequence of an irradiation and occur at different spatial scales: the “local” and “non-local” lethal events.
The NanOx model relies on a completely stochastic theory, according to which cell survival is averaged over all irradiation configurations cK. Depending on the application field, cK may account for the set of parameters characterizing the nature of irradiation, such as the type of particles, their initial position, direction and energy. Each configuration consists of a stochastic number K of radiation tracks with a stochastic distribution of energy transfer points and radical species. A radiation track may correspond, for instance, to a track generated by an ion or an electron; to the interactions of photons or neutrons that lead to the ejection of secondary particles (electrons or ions); or to the different particles emitted in a radioactive decay. Radiation tracks are produced by means of Monte Carlo (MC) simulations of particle transport in matter (with a nanometric resolution), including physico-chemical and chemical processes [29].
As Figure 1 shows, the spatial distributions of energy transfer points and of primary radical species constitute the main input for the NanOx model. The spatial distribution of energy transfer points is used to compute the restricted specific energy (see Definition 2, Section 2.2.2) in nanometric targets associated with local lethal events. On the other hand, the distribution of primary radicals is used to model the effects induced by global events (a particular case of non-local lethal events) via the chemical specific energy (see Definition 5, Section 2.3.3.3).
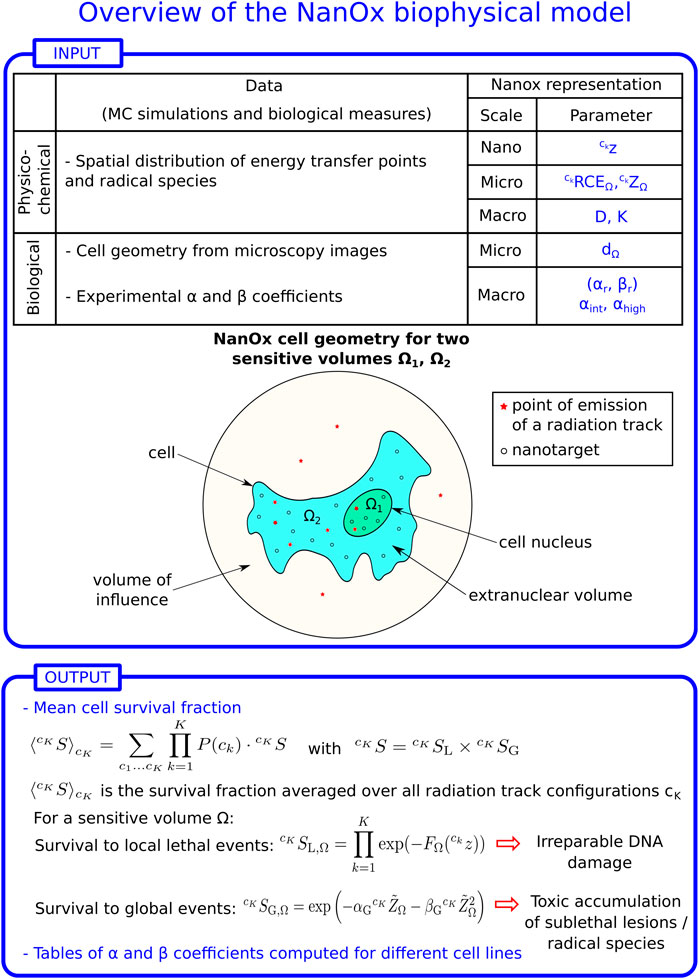
FIGURE 1. Overview of the NanOx biophysical model. z: restricted specific energy distribution in local targets; ZΩ: restricted specific energy distribution in the sensitive volume Ω; RCEΩ: relative chemical effectiveness; D: macroscopic dose; K: number of radiation tracks in the volume of influence (see Definition 1). Each radiation track k may correspond to a track generated by an ion or an electron, to the interactions of photons or neutrons that lead to the ejection of secondary particles, or to the particles emitted in a radioactive decay; P (ck): probability of a radiation configuration ck; dΩ: diameter of the sensitive volume Ω; αr, βr: linear-quadratic (LQ) coefficients for reference radiation; αint, αhigh: linear coefficient for intermediate- and high-LET radiation, respectively; FΩ: effective local lethal function for a sensitive volume Ω;
The procedure required for the calculation of the cell survival fraction, illustrated schematically in Figure 1, may be easily generalized to any mixed field.
2.1.1 Locality and local lethal events
The notion of locality means that we can consider a volume small enough such that the probability that two or more particle tracks deposit a significant specific energy inside that volume is negligible at clinical doses. This is the case of biological targets of the order of tens of nanometers (
2.1.2 Non-local lethal events
Non-local lethal events constitute, by definition, the complementary class to that of local lethal events. They may be associated with physico-chemical mechanisms occurring at a larger scale than the local ones, but they may also correspond to a distribution of several local events, the single action of which being non-lethal. A common example of non-local lethal events used in microdosimetric models is the interaction between two sublethal cellular lesions separated by distances of the order of the micrometer. The combination of these events, by an effect of accumulation and/or interaction, may result in cell death by triggering apoptosis or via any other cell death pathway. In the current version of NanOx, we introduce “global” events to describe a phenomenon of toxic accumulation. This accumulation may refer to the generation of numerous sublethal lesions which become complex to manage for the cell, or refer to an imbalance between the amount of antioxidant defenses and reactive chemical species. This choice allows us to explore a mechanism that is usually not considered in other biophysical models reported in the literature. Moreover, this choice does not exclude the possibility of exploring other processes in the future, such as the radio-induced damage on cellular targets other than the nucleus (e.g., in the cell membrane or the mitochondria), the communications of type bystander, and the mechanisms underpinning the oxygen effect on tumor resistance to radiotherapy [34].
In a general way, we set X the quantity that represents the action that triggers global events. In this context, the production of primary reactive chemical species seemed to us of particular interest and we decided to take it as a parameter to account for all global events in the current version of NanOx. Indeed, radical species are responsible for causing oxidative stress and inducing a significant part of sublethal damage [35,36].
It should be noted that there is no straightforward correspondence between the direct effects of ionizing radiation and the local lethal events in the NanOx model; similarly, no one-to-one correspondence can be established between the indirect effects of ionizing radiation and the global events as defined in NanOx. As a matter of fact, both local and global events might have contributions of both direct and indirect effects.
2.2 Postulates of the NanOx model
This section requires the introduction of some definitions and notations, which are summarized in Table 1. The NanOx postulates, previously presented in [13], are listed; the equations derived from Postulate 3 are, however, more general here.
With NanOx, we look to perform a simulation close to reality. In that sense, to each cell in a population corresponds a unique radiation track configuration that represents the radiation impacts inside that cell, as well as the impacts taking place outside of it but which are able to induce energy deposition or ionizations in the cell.
Let us consider a large number N of cells irradiated with an absorbed dose D. Experimentally, the average cell survival for a cell population, ⟨Spop⟩, can be computed as:
Alternatively, we could consider a representative cell such that:
where the subscript IR denotes the set of N irradiation configurations impacting the N cells in the previous approach (Eq. 1). Ideally, N would be infinite to minimize statistical fluctuations.
The previous considerations lead us to the following simplification.
Simplification 1 (Representative cell associated with a cell population)We consider that the cell survival probability can be computed starting from a representative cell. The latter means that we calculate the average survival of the representative cell over many configurations of radiation tracks.he latter simplification implies that in NanOx we do not consider explicitly the effect of communications between cells. However, such processes are implicitly included in NanOx’s predictions because the model’s parameters are adjusted with experimental data, which clearly include all types of cell communications.
2.2.1 Sensitive volumes and volume of influence
Postulate 1 (Sensitive volumes associated with local and non-local lethal events)The cell survival fraction after an irradiation is characterized by the effect of such an irradiation on several sensitive volumes, some associated with local lethal events and some associated with non-local lethal events.
Definition 1. (Volume of influence)The volume of influence is a volume large enough that the track of a particle outside this volume leads to a negligible transfer of energy into the sensitive volumes associated with local and non-local lethal events.
2.2.2 Restricted specific energy
Microdosimetry introduced the specific energy as the stochastic analogue of the dose; this quantity is in fact defined as the ratio of the energy imparted by one or more events in a site, to the mass of the site. We propose an alternative computation, disregarding the energy that simply causes the heating of the medium (e.g., molecular vibrations, interactions between electrons and water phonons, geminate recombinations).
Definition 2. (Restricted specific energy)The restricted specific energy z defined for a given target corresponds to the specific energy in that target obtained by considering only the energy transfers that may lead to events which are relevant for the biological effects of radiation (e.g., ionizations, excitations and attachments of electrons). For a sufficiently large target:
Where η ∼ 80% according to calculations with LQD [29] (see Section 3.1). The considered processes and their energies are listed in Table 2. Let us note that the value of η might depend on the set of interaction cross sections used in the simulations [37] and it is not measurable. On the other hand, the good agreement obtained for radical yields [38–40] and in the prediction of the mean ionization energy (or W-value, an indication of the fraction of energy related to ionization) [41,42] give confidence about the value of η reported here.
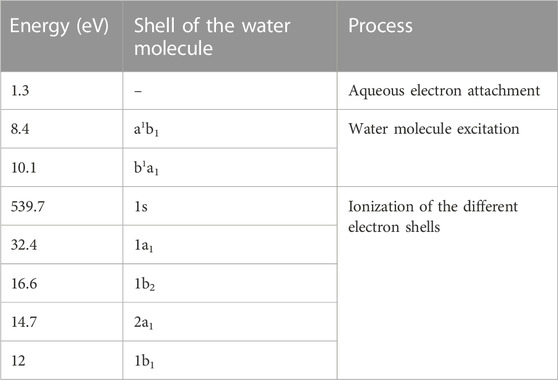
TABLE 2. Energy of the processes considered in the Monte Carlo simulations for NanOx [59].
2.2.3 Cell survival to local and non-local lethal events
Postulate 2 (Independence of local and non-local lethal events)Local and non-local lethal events are independent. Thus, the probability of cell survival to a configuration of radiation tracks cK (
Where
2.2.3.1 Cell survival to local lethal events
The modeling of cell survival probability to local lethal events is based on the single-hit, single-target theory [43]. Therefore cell killing is induced by the inactivation of one single target among N. The size of the latter is small enough (nanometric) to allow classifying this inactivation mechanism as a local lethal event.
Postulate 3 (Definition of the probability of cell survival to local lethal events)The probability of cell survival to local lethal events (
where
with NΩ the number of targets inside the sensitive volume Ω. For example, if the mitochondrion unit was considered as target, then the domain would correspond to the mitochondrial network. Considering that the size of the targets associated with local lethal events is defined so that the probability that two particle tracks deposit energy into the same target is negligible, the following approximation holds:
where ki is the index of the particle track responsible for the physical or chemical quantity associated with the target i. Obviously, if the target i does not suffer any physico-chemical process,
As it will be shown in Section 3.2, it is possible to write
2.2.3.2 Cell survival to non-local lethal events
In the present version of NanOx, we use the class of global events to describe a phenomenon of toxic accumulation, which may encompass the production of sublethal lesions that would saturate at a certain point the repair capacities of the cell, or the oxidative stress induced by reactive chemical species.
Postulate 4 (Non-local lethal events limited to global events)In this version of the NanOx model non-local lethal events are limited to the class of global events, for which a function representing the toxic accumulation of sublethal lesions or oxidative stress induced by reactive chemical species is introduced.
Postulate 5 (Probability of cell survival to global events)The probability of cell survival to global events
2.3 Implementation of NanOx
NanOx was defined in the previous sections, however its implementation requires some approximations and simplifications to drastically reduce the amount of computing resources required.
2.3.1 Core and penumbra for ion tracks
For ions we can consider a track segment of length L small enough to consider a straight trajectory over which the energy remains constant. For sufficiently high-energy ions, L may be equal or even larger than the size of the cell. The previous assumption is made basically to exploit the track-segment approximation in hadrontherapy applications. Let us note, however, that this approximation is not always verified, particularly when projectile or target fragmentation occurs. Indeed, it has been shown in the literature that even for proton beams target fragmentation modifies the biological dose calculation, especially in the entrance channel, while minor effects are expected in the Bragg peak region [45]. The impact of fragment species is currently not included in NanOx, but it may be investigated in future versions of the model along with low-energy ion irradiations.
To simplify the implementation of the model and the calculations, we exploited a feature that characterizes ion tracks: the presence of two regions in which the energy deposition patterns are totally distinct. The inner core may be distinguished by a high concentration of energy transfer points around the ion’s path, while the remainder of the track, designated as penumbra, consists of sparser energy depositions issued from fast δ electrons; its extension is delimited by the farthest energy transfer points from the ion trajectory. The two regions may be easily identified in Figure 2, where the spatial pattern of energy depositions in water by a 2.6 MeV proton and a 12 MeV/u carbon ion is depicted.The delimitation of the two track components is not straightforward, since the typical extension of the inner region may vary according to the radiation quality. The interest in treating the track core and penumbra differently is to describe the physical and chemical events that compose the latter region in the same way as those induced by the reference radiation, i.e., photons (corresponding to LET values
Where
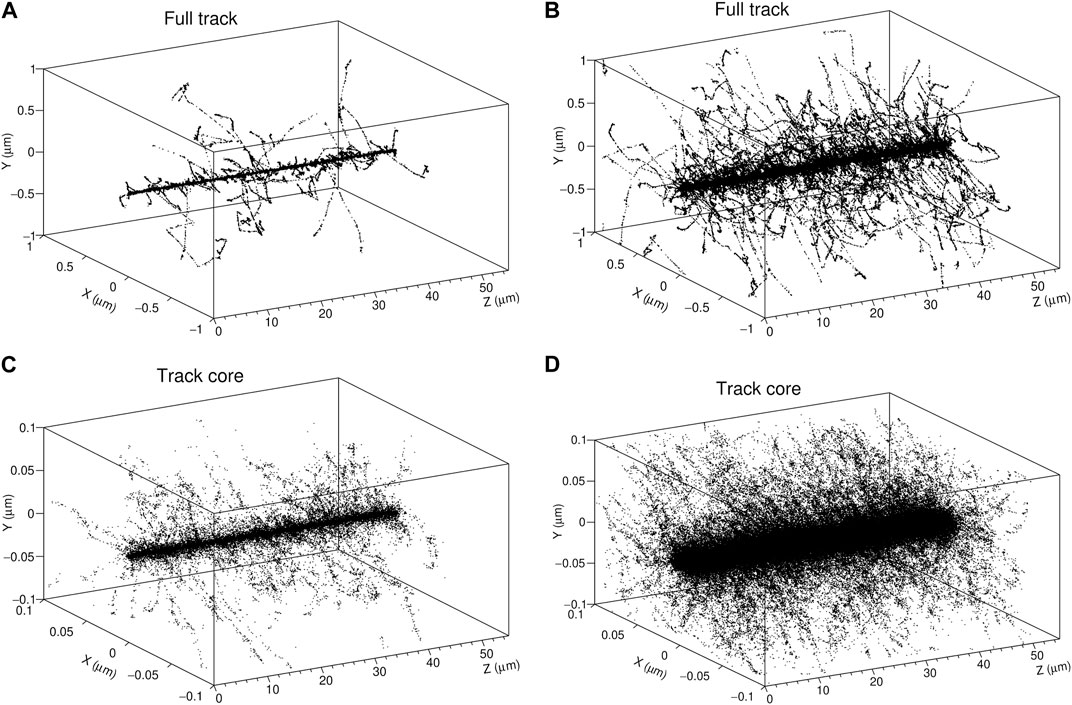
FIGURE 2. 3D plots of the tracks of a 2.6 MeV proton (A) and a 12 MeV/u carbon ion (B) with a LET in water of 13.5 and 151 keV/μm, respectively. Each point corresponds to an energy transfer point simulated with LQD (C, D) correspond to a zoom over the inner region of the tracks (core volume).
It is worth mentioning that for low-energy ions the track-segment approximation becomes more questionable than in hadrontherapy and therefore another strategy has to be developed. For instance, for sufficiently low energies, the penumbra may be neglected (see Section 3.3). This could be applied for instance to TRT with α-particle emitters, BNCT, or neutron irradiations in general. Ongoing work is devoted to extend the model to low-energy ion irradiations, which will allow to take into account as well the impact of fragmentation on NanOx predictions.
2.3.2 Local lethal events
2.3.2.1 Strategy: From nanoscale to microscale
In order to implement the modeling of cell survival to local lethal events, we present several approximations; the purpose is to disregard, when possible, the details at nanoscale in favor of some generalizations and to replace, when justified, some quantities with their mean values.
First, we provide a generic representation of local targets to treat them as N undifferentiated entities and neglect the specific features that each one may present. This will allow to introduce in Section 3.2 an “effective local lethal function” (ELLF), which characterizes the response of each cell line instead of the one of each target [46].
Second, for each radiation quality we establish a link between the effective number of local lethal events (ENLLE, written as n∗, see Section 3.2) and the restricted specific energy deposited in each sensitive volume Ω by K tracks (ZΩ). Indeed calculating the number of effective local lethal events for each configuration of targets and radiation tracks would be cumbersome, thus some simplifications are strongly recommended. For this reason, we introduce coefficient α defined generically as follows:
2.3.2.2 Characterization of the local targets
Approximation 1 (Definition of the number NΩ of local targets in the sensitive volume Ω)The number NΩ of local targets in the sensitive volume Ω is large enough so that the fluctuations in the ENLLE (Section 3.2) become negligible.
Simplification 2 (Identical local targets in the sensitive volume Ω)All local targets in a sensitive volume Ω have the same geometry and their response is characterized by the same law. We will use in the following the index
Simplification 3 (Homogeneous distribution of the targets associated with local lethal events)The targets associated with local lethal events are homogeneously distributed inside the sensitives volumes of the cell.
Simplification 4 (Characterization of the targets associated with local lethal events)The local targets are modeled as cylinders with length Lt = 10 nm and diameter dt = 20 nm, oriented along a single direction. For instance, the direction of an incident photon beam or the ion’s trajectory for track-segment conditions.The size of the local targets is independent of radiation quality and cell line. The chosen dimensions correspond roughly to the extension of a DSB, taking the diffusion of reactive chemical species into account. Indeed, a DSB may extend up to over 20 base pairs or 6 nm [9,21,47], while the reactive species can cause damage up to 4 nm away from the hit site [9,48].
2.3.2.3 Alpha coefficient for reference radiation
Definition 3. (Low-LET as reference radiation)The reference radiation corresponds to low-LET radiation (LET
Approximation 2 (α coefficient for reference radiation)The average ENLLE (see Section 3.2) induced in the sensitive volume Ω by reference radiation at clinical doses is proportional to the restricted specific energy deposited in the sensitive volume Ω:
where
2.3.3 Non-local lethal events
2.3.3.1 Cell survival probability to global events
Simplification 5 (The cell survival to global events has a linear-quadratic (LQ) shape)The probability of cell survival to global events computed over all sensitive volumes is written as:
where
with a and b representing cell line-dependent parameters. Indeed, to predict the shoulder seen in cell suvival curves it is necessary to have a quadratic term in the cell survival to global events.
Simplification 6 (Representative chemical species)Primary hydroxyl radicals (OH•) produced by water radiolysis at a time TRCE (where the subscript RCE refers to the relative chemical effectiveness defined in Section 2.3.3.3), elapsed after each radiation track, are chosen to represent the reactive chemical species. OH• radicals are indeed among the most effective reactive chemical species in causing cell damage [49].
2.3.3.2 Strategy: From nanoscale to microscale
2.3.3.3 Chemical specific energy
Definition 4. (Chemical yield)The chemical yield G is defined as the ratio between the concentration of a given reactive chemical species and the irradiation dose that induces such a concentration. More precisely, G represents the number of reactive chemical species generated per 100 eV.
Definition 5. (Chemical specific energy)The chemical specific energy
In Eq. 15,
and computed with the LQD/PHYCHEML/CHEM MC codes [29].
Property 1. (Tracks of primary reactive chemical species)As the chemical yields are computed for primary reactive chemical species, the tracks may be considered as independent:
At this stage, it is possible to express the probability of cell survival to global events (Eq. 13) as a function of the chemical specific energy. The concentration of primary reactive chemical species after a configuration of K radiation tracks may be expressed as:
The factor η, previously presented in Eq. 3, is due to the fact that we chose to discard the fraction of deposited energy leading exclusively to the heating of the medium in our calculations, while the definition of the yield relies on the standard notion of specific energy. By replacing the second term of Eq. 18 in Eq. 13, one obtains:
where the new coefficients read αG = a ⋅ Gr/η and
2.3.4 Calculation of cell survival for reference radiation
The αG and βG coefficients introduced in the previous section are determined by imposing that NanOx predictions reproduce a LQ expression for the survival of reference radiation. The calculation is detailed below. For the sake of simplicity we restrict the following discussion to the case of one sensitive volume, thus the index Ω will be omitted. For an irradiation with dose D, the average probability of cell survival corresponds to:
where Pr(K, D) is the probability that K radiation tracks are located inside the chosen volume of influence for a dose D delivered by the reference radiation and
Due to Approximation 2 and to the fact that for reference radiation RCEr = 1 and thus
where the Dirac delta function allowed for a reformulation of the same mathematical entity. Eq. 20 may, thus, be expressed as follows:
Finally, by renaming ∂PD/∂Z the weighting factor which represents the distribution of specific energy in the sensitive volume corresponding to a macroscopic dose D, one obtains:
Approximation 3 (The distribution of energy depositions of reference radiation at a microscopic scale is modeled with a Gaussian law)If the dose deposited by reference radiation in the sensitive volume is higher than a few cGy and lower than a few tens of Gy, i.e., belongs to a range in which the linear-quadratic approximation for cell survival is appropriate, ∂PD/∂Z corresponds to a Gaussian law:
with:
We remind that the factor η was mentioned in the description of the restricted specific energy (Definition 2), and its value corresponds almost to 80% (see also Section 3.1).Figure 3 reports, as an example, the specific energy distribution obtained after an irradiation with photons from a 60Co source depositing 0.1 Gy in cylinders of 4.89 µm radius and 1 µm thickness. The curve is a Gaussian peaked around 0.08 Gy, corresponding to the irradiation dose rescaled by the factor η.Once analyzed the factor ∂PD/∂Z, Eq. 24 may be used to derive the coefficients αG and βG, which have been introduced for the modeling of global events, and αrL for the description of local events. The term on the left represents the LQ description of cell survival probability to reference radiation in terms of the macroscopic dose D, by definition:
The term on the right of Eq. 24, on the other hand, corresponds to a convolution between a Gaussian and the NanOx representation of the cell survival probability to reference radiation as a function of the microscopic restricted specific energy. The latter equals:
where
The integral from 0 to infinity in Eq. 24 may be approximated to the one from −∞ to ∞ provided that D is sufficiently larger than 0 Gy. In this way, knowing the result of the Euler-Poisson integral, the convolution becomes:
Given that
In the framework of this approximation, the coefficients of cell survival at a microscopic scale for a low-LET irradiation are finally determined:
with the previous expressions simplifying to:
When σZ ≪⟨Z⟩ = ηD.Let’s recall that for our calculations we did not applied such approximations, and we defined the coefficient CL as:
to represent the fraction of the linear component of the cell survival probability at a microscopic scale due to local lethal events after reference radiation. CL is set to 1 in this version of the model, resulting in αG = 0 Gy−1, which allows for an independent adjustment of the local and the global events. We obtain then:
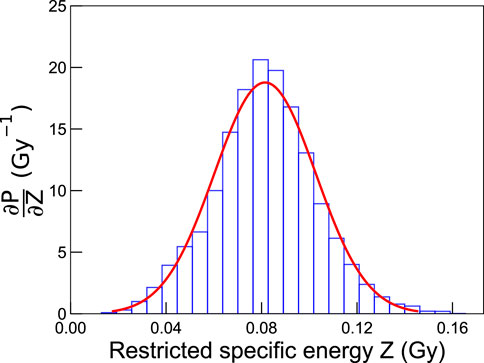
FIGURE 3. Probability distribution of specific energies in cylinders of 4.89 µm radius and 1 µm thickness for an irradiation of 0.1 Gy with photons from a 60Co source. The simulations were carried out with LQD, and the bin width was defined as 6 × 10−3 Gy.
2.4 Summary of the NanOx model parameters
To conclude the characterization of the model, which proceeded first via the presentation of the main postulates and then with the list of approximations and simplifications currently implemented, it is interesting to consider the parameters required for NanOx calculations. The main input parameters required for NanOx predictions are summarized in Table 3.
The modeling of local and non-local lethal events is based on the definition of critical cellular regions called “sensitive volumes”. The latter may correspond for instance to the cell nucleus and to the cytoplasm, if the modeling considers two sensitive volumes. For the sake of simplicity, and to reduce computing time, the sensitive volumes are modeled by default in NanOx simulations as cylinders. However, calculations may be extended to complex and more realistic cell geometries (e.g., obtained from microscopy images, see Figure 1).
The modeling of local lethal events relies on the inactivation of nanometric biological targets designated via their diameter dt and thickness Lt. A convenient representation of this process is given in terms of an “effective local lethal function” (ELLF), FΩ, expressing the response of the specific cell line instead of the one of each single local target (see Section 3.2). By initially defining FΩ as a linear combination of basis functions, it has been shown in [46] that this function presents a threshold and a saturation for the 3 cell lines (V79, CHO-K1 and HSG) considered in that study. In order to reproduce the function’s main features while reducing the number of parameters, an error-like function was then used with the three following parameters: h, the height of the response, z0, the restricted specific energy threshold and σ, the extent of the increase.
On the other hand, the modeling of global events, a particular case of non-local lethal events, requires the parameters αG, βG and TRCE. Nevertheless, an extensive study of the influence of NanOx parameters [26] demonstrated that the prediction of the biological effect of ions for a wide LET range may be based, when considering a single sensitive volume, on only five parameters characterizing a given cell line (dΩ, h, z0, σ, βG), while the other parameters can be fixed according to specific considerations.
3 Results
3.1 Computation of η
As mentioned in Section 2.2.2, the restricted specific energy z takes into account only the fraction of energy deposits that may contribute to biological damage. Such fraction, denoted as η in our model, is the ratio of the energy deposited in the medium to the total energy lost by radiation. We computed by means of the LQD MC code [29] the value of η for different radiation types and incident energies. The results are presented in Table 4. It can be seen that regardless of the radiation quality, η is approximately equal to 0.8 for all practical purposes.
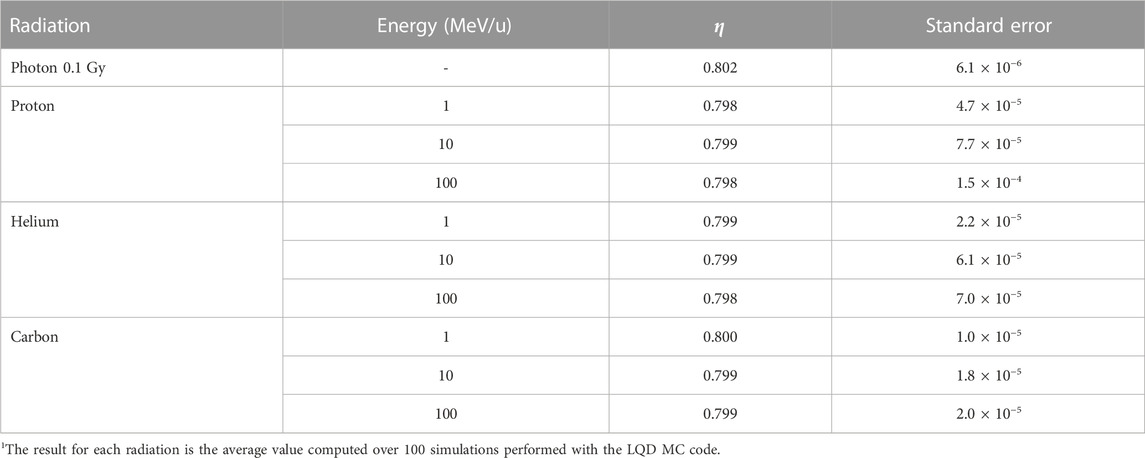
TABLE 4. Values for the factor η, i.e., the ratio of the energy deposited in the medium to the total energy lost by radiation1.
3.2 Effective number of local lethal events and effective local lethal function
The average number of local lethal events in a local target i belonging to the sensitive volume Ω, determined over the whole set of possible biological responses in the sequence of an irradiation leading to a quantity xi in the target i, is:
since ci ∈ Ω. We introduce here a quantity more convenient for the practical implementation of the model, which we designate as the average (over the whole set of possible biological responses) effective number of local lethal events (ENLLE) in the target i:
Given that, by definition, local lethal events are mutually independent, the average ENLLE induced by the track k in the sensitive volume Ω with NΩ targets is:
Or taking into account the total number of targets N in the sensitive volumes:
The cell survival fraction to local lethal events for a configuration of N targets and K particle tracks (Eq. 8) can then be written as:
At this stage it is possible to define the effective local lethal function FΩ(z) (ELLF) for a sensitive volume Ω:
with
and
3.3 Energy deposited in the core and penumbra of ion tracks
Table 5 shows the average ratio of the energy deposited in the penumbra (Ep) to the energy deposited in the core (Ec) of ion tracks for protons, helium and carbon ions with energies in the range from 1 MeV/u to 150 MeV/u. The values were computed with the LQD MC code considering a core volume defined as a parallelepiped with a square cross section of 200 nm edge. It can be seen that the ratio depends essentially on the ion’s energy, but not its type, and that a maximum value is reached for energies between 50 MeV/u and 100 MeV/u. The fact that the maximum value of the ratio does not correspond to the highest ion energy might be due to the enhanced forward emission (i.e., in the direction of the ion’s trajectory) of secondary electrons. The latter would increase the energy deposited in the core, thus explaining the overall decrease in the ratio. Furthermore, the results indicate that the energy deposited in the penumbra represents about 12% of the energy deposited in the core at 3 MeV/u, and only about 6% at 2 MeV/u. Thus, the contribution of the penumbra may be neglected for low-energy ions such as the ones used in BNCT and TRT with α-particle emitters.
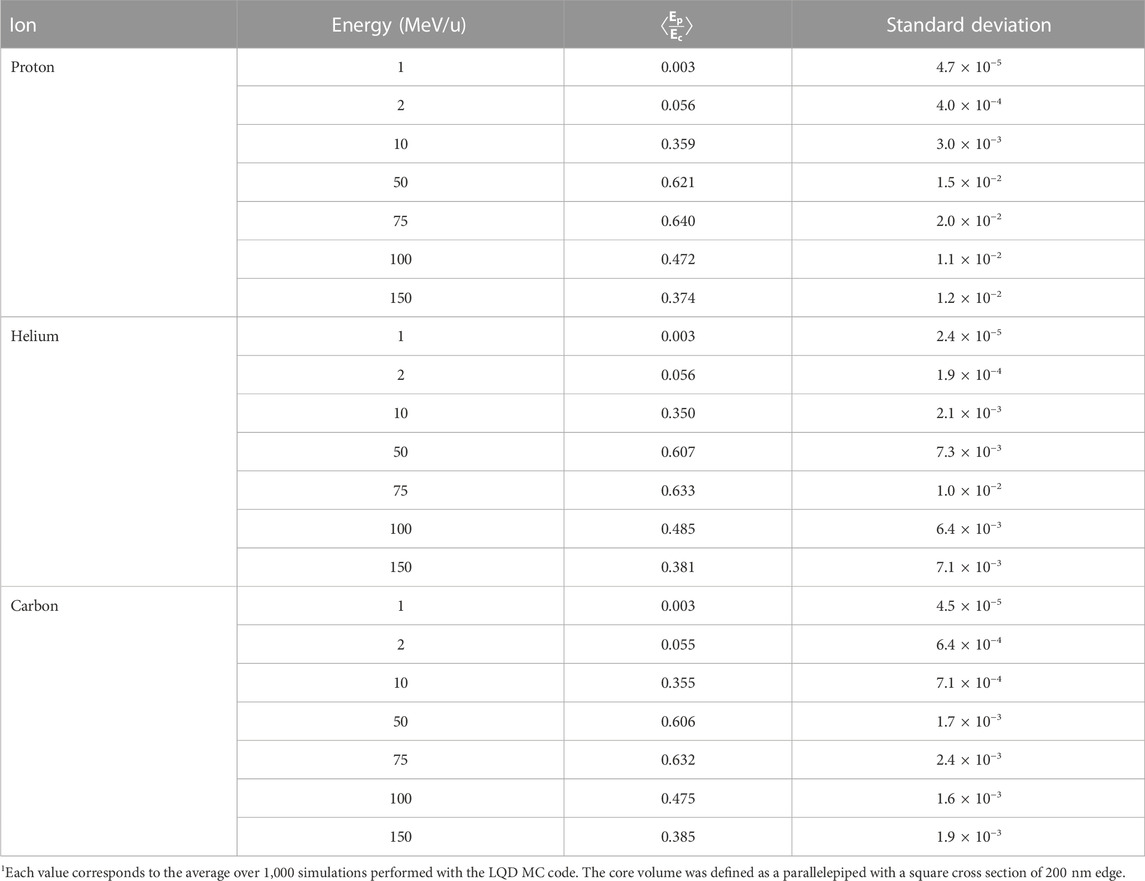
TABLE 5. Average ratio of the energies in the penumbra (Ep) and the core (Ec) for different ions and incident energies1.
3.4 Relative chemical effectiveness and β coefficient
The production and diffusion of OH• radicals has been simulated for different radiation types and energy values. The obtained evolution with time of RCE2 (since this quantity appears squared in the expression of cell survival to global events, Eq. 19) is plotted in Figure 4. The plots show that the hydroxyl radical concentration induced by ions as a function of time decreases faster than the one obtained in response to photons, an effect due to the different spatial distribution of the reactive chemical species produced in the two cases. When they are located along the ion tracks, indeed, they may easily recombine to give water or other (non-radical) molecules, while when they are sparsely distributed the interactions between them are strongly reduced. Even if the OH• recombination is a complex phenomenon, the simulations suggest that the value of RCE increases with decreasing LET, as a general trend. Only one exception is noticed in Figure 4 for 70 MeV protons. The general behavior is illustrated as well in Figure 5, where we have plotted the RCE as a function of LET for protons, helium and carbon ions at a time TRCE = 10–11 s. Let us remind that TRCE is the time interval separating the impact of the incident particles and the moment at which the concentration of OH• radicals is considered. It was shown in a previous work [26] that the influence of TRCE on NanOx predictions is limited and almost independent of the cell line. In particular, it was observed that changing TRCE from 10–11 s to 10–8 s did not affect the linear coefficient α. This is not surprising, as the term
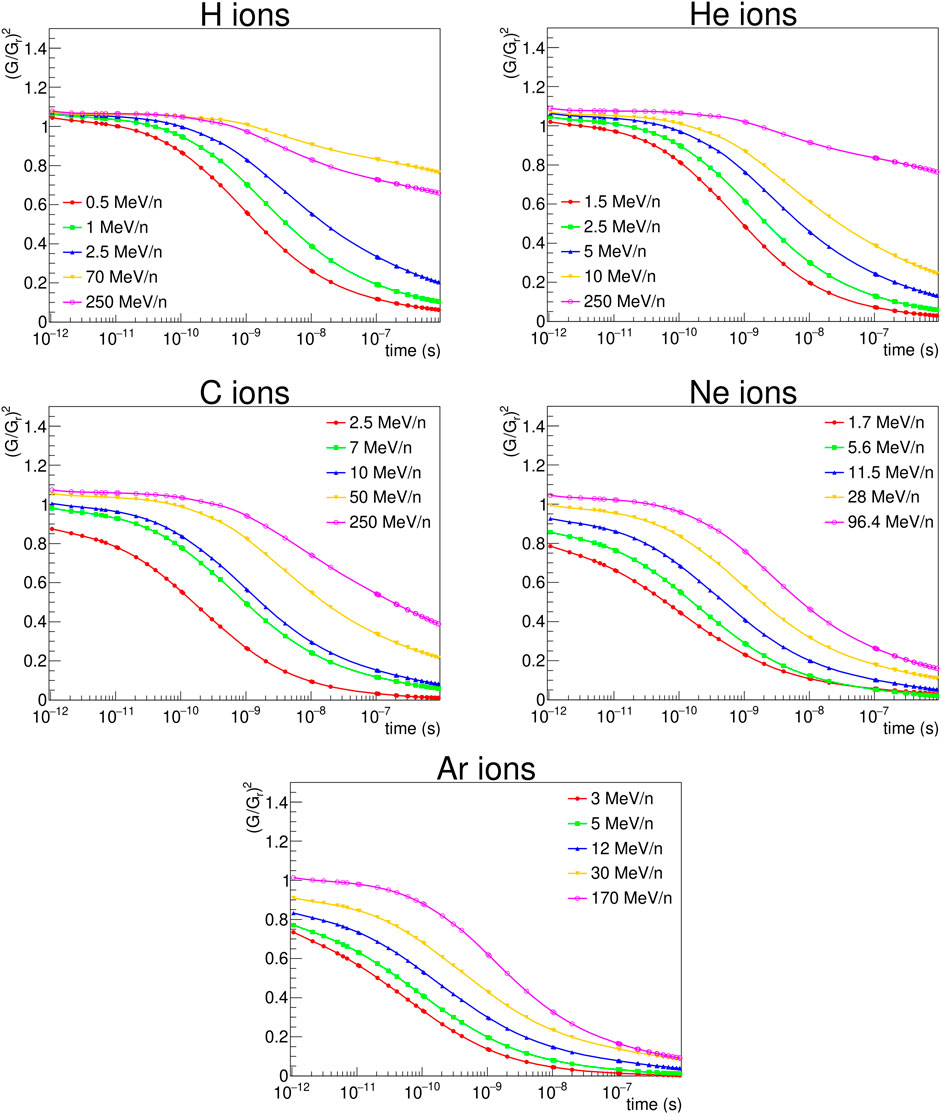
FIGURE 4.
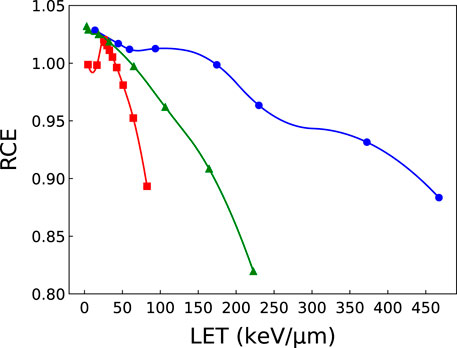
FIGURE 5. RCE as a function of LET for protons (squares), helium (triangles) and carbon (circles) ions computed at a time of 10–11 s. The solid curves connecting the calculated points are for visualization purposes only. Error bars are not shown given the small value of the standard deviation: σ ≤2×10−4 for protons and helium ions; σ ≤3×10−3 for carbon ions.
It is clear from Figure 5 that while the RCE increases with decreasing LET for all ions, the curves do not exhibit a linear trend. Moreover, the variations in the RCE become more important for low-LET ions.
We have plotted in Figure 6 the ratio β/βr as a function of RCE2 for protons, helium and carbon ions. We remind the reader that β is the macroscopic coefficient of the quadratic term in the LQ model corresponding to ion irradiation, and that βr is the value for reference photon radiation. The coefficient β was obtained by performing a LQ fit on the cell survival fractions computed with NanOx for the human salivary gland (HSG) cell line (βr = 0.0615 Gy−2), taking the cell nucleus as the sole sensitive volume (cylindrical volume with a radius of 7 µm and thickness of 1 µm). As usual, the RCE was computed considering the chemical yields of OH• radicals at a time of 10–11 s for all ions. It can be seen that the ratio β/βr varies with RCE2. Moreover, in the region in which RCE = 1, there is an important variation in β, which indicates that β does not correspond directly to βG⋅RCE2 and that the averaging process over all irradiation configurations plays an important role on the value of β. We obtain different results when we fix RCE = 1 and when we consider the RCE at a time of 10–11 s. Since the latter condition leads to a better agreement with experimental data when comparing cell survival fractions [28], it seems that taking into account the chemical specific energy improves the modeling of biological effects.
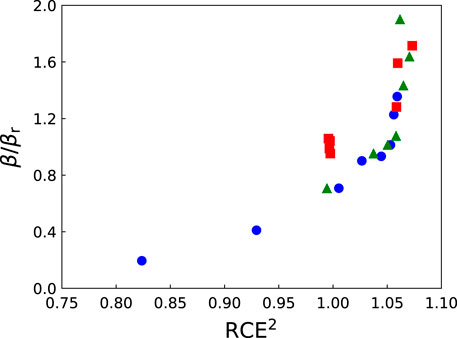
FIGURE 6. The ratio β/βr as a function of RCE2 for protons (squares), helium (triangles) and carbon (circles) ions. The RCE was computed at a time of 10–11 s for all ions. The coefficient β was obtained by performing a linear-quadratic fit on the cell survival fractions computed with NanOx for the HSG cell line (βr =0.0615 Gy−2, cylindrical nucleus with radius of 7 µm and thickness of 1 µm).
3.5 An example of application: Cell survival to local lethal events in the track-segment approximation
In this section we illustrate a simple application example of the NanOx model. Let us consider the irradiation of a single sensitive volume by a monoenergetic, monotype, parallel ion beam. In addition, we will assume that track-segment conditions are fulfilled, that the beam delivers a uniform radiation dose in the surface of influence, and that the particles in the beam are independent. Finally, we will consider that the effect on cell survival of non-local lethal events can be neglected in order to keep only the term
with P(K, D) the probability, modeled with a Poisson distribution, to achieve K impacts in the volume of influence with the dose D;
By defining the mean cell survival to one impact as:
it follows from Eq. 46 that:
By substituting the right side of Eq. 48 into Eq. 45 and writing P(K, D) as a Poisson distribution:
On the other hand:
where
We can observe from Eq. 51 that when only the local lethal events are taken into account, the cell survival probability is given by a pure decreasing exponential, i.e.,:
with:
where
Similarly, for Σ2:
which can be written as well as:
However, by Definition 1, the ions cannot deposit energy outside the surface of influence Σ1, so
4 Discussion
The NanOx model was firstly developed for applications in hadrontherapy [13,50,51]. Its development followed a theoretical study which showed that the local effects (at the nanometric scale) were not enough for describing cell survival, and that it was necessary to include non-local effects [21,22].
NanOx combines some premises from other biophysical models proposed in the literature with some fully innovative aspects. The present section details further the positioning of the NanOx model in the context of the state of the art in particle beam radiation biophysical models.
The extension or adaptation of the existing premises is seen in the following features of the model:
• Usage of the concept of specific energy introduced in microdosimetry; however, the use of this specific energy was extended in NanOx to the nanoscale.
• Introduction of the postulate that the cell survival can be modeled with the product of two components, similar to what was done in the model by Katz et al. [23,24]. However, in the latter model the two components refer to the same mechanism (activation of N targets), while in the NanOx model they are related to two different lethal mechanisms.
• NanOx took the notion of local lethal event introduced in the first version of the LEM [6], but without the assumption that the expression relating the cell survival to the number of local lethal events is a Poisson law.
The fully innovative aspects introduced in the NanOx model were:
• The notion of global events that intends to model the lethal effects of the oxidative stress and of the accumulation of sublethal lesions, induced at the scale of the cell sensitive volumes (the NanOx model in the present version does not consider the interaction by pair of sublethal lesions).
• The notion of chemical specific energy, representing the toxic accumulation of oxidative stress, i.e., the imbalance between the production of reactive oxygen species and antioxidant defenses, or sublethal damage at a cellular level. To the best of our knowledge, for the first time chemical yields were used as an input for a RBE model of cell survival.
• The construction of the ELLF related to the inactivation of nanotargets. With no a priori assumptions on this function except for the monotonic increase, its shape was resolved by means of a linear combination of basis functions and a fit of the weight values of this linear combination to get a good agreement with experimental data. The resulting ELLF presented a threshold and a saturation that were discussed in respect to features of other models [46].
Moreover, two important aspects that deserve further discussion are the scales and the level of detail at which biophysical models describe the radiation effects and the way they integrate (or not) non-Poissonian effects. As already mentioned, in the current version of NanOx, the nanometric scale is integrated via the restricted specific energy distributions in 10 nm targets, a size that covers the extension of a DSB, including the diffusion of reactive chemical species. These nanotargets are distributed uniformly in micrometric sensitive volumes (e.g., the cell nucleus or, more generally, any other cell organelle). Moreover, the chemical specific energy is calculated also at the micrometric scale. Therefore, the target geometry covers the microscale and the nanoscale. Besides, stochastic effects inherent to ionizing radiations are fully taken into account. Indeed, each configuration of irradiation is characterized by 1) the distribution of radiation tracks and 2) for each track, by the distribution of energy depositions and radicals with nanoscale resolution.
It is important to point out that the concepts of local lethal and global events in NanOx are intentionally formulated in a sufficiently general way to allow testing distinct mechanisms in the future as the model evolves. For this reason, we do not explicitly deal in NanOx with the different types of DNA damages or their yield.
The question related to using a Poisson or non-Poisson law appears at two levels. At the level of the local events, contrary to the LEM, the NanOx model does not assume a Poisson law. At the level of the radiation track configurations, in the same way as the LEM, the cell survivals are averaged over all the possible values of the number of tracks and their positions. In contrast, the MKM calculates a mean number of lethal events over these irradiation configurations and assumes a Poisson law for calculating the mean cell survival. As this assumption is not valid for high-LET, “non-Poissonian” corrections have been introduced in the extended and derived versions of the model.
It should be noted that the LEM IV [11,19] is very different from the previous versions of the model. The locality is no longer associated to point-like events. The biological effect is determined from the initial spatial distribution of DSB induced by radiation. The calculation of the DSB distribution is based on the local dose (derived from radial dose profiles) deposited in subvolumes of the cell nucleus. MC methods are applied for sampling the DSB location. The LEM IV considers three spatial scales of DNA damage formation assumed to be relevant for cell survival, extending from the nm level to about 10 µm. It does not take into account, however, the degree of complexity of DSB at the nm scale or the formation of chromosome aberrations [20]. The LEM IV assumes a linear-quadratic-linear (LQL) behavior of cell survival, with a threshold dose marking the transition from a LQ to a purely linear behavior.
Similarly to the LEM IV, the MKM [52] divides the sensitive target (cell nucleus) into subunits called domains, having a variety of shapes. Radiation is assumed to create two types of DNA damages: type I lesions are lethal; type II lesions are sublethal, i.e., they can be repaired or converted into lethal lesions (either by spontaneous conversion or binary combination with another sublethal lesion). Cell death occurs if at least one domain contains a lethal lesion. The initial number of lesions in a domain is considered proportional to the specific energy in the domain. Then the evolution of the average number of lesions per domain is computed by solving a set of coupled ordinary differential equations. To connect the number of lesions to the cell survival probability, the original MKM assumed a Poisson distribution of the lethal lesions and a linear-quadratic behavior of cell survival. Later improvements and extensions were made to the MKM, which essentially added ad hoc corrections to account for non-Poissonian effects [7,8,53]. Moreover, other developments have been required to compensate the partial accounting of the stochastic nature of energy deposition in the MKM, which leads to disagreements for high-dose and high-LET irradiations, notably in the prediction of the behavior of the β coefficient [12]. For more details, we refer the reader to the excellent review on the MKM and derived models recently carried out by Bellinzona et al. [54].
The local and global events defined in NanOx are somewhat analogous to the type I and II lesions of the MKM, respectively. However, the notion of global event in NanOx is different at least in two points: 1) it currently does not consider the binary interaction of sublethal lesions, only represents the deleterious effect of their accumulation; 2) NanOx stresses the role of reactive chemical species in radiation damage induction. On the other hand, an interesting aspect of the MKM is the possibility to account for arbitrary dose-rates, something that is currently not considered in the LEM and NanOx.
BIANCA (BIophysical ANalysis of Cell death and chromosome Aberrations) [16,55,56] is a biophysical model allowing to compute cell survival probabilities as well as chromosome aberration dose-response curves for different types of irradiation. The basic idea behind BIANCA is that some radio-induced DNA damages (called cluster lesions) produce two independent chromosome fragments, after which distance-dependent fragment mis-rejoining or un-rejoining give rise to chromosome aberrations. Some of these aberrations (dicentrics, rings and large deletions) lead to cell death. While in the first version of BIANCA the cell survival was computed considering a Poisson distribution of the number of lethal aberrations, the calculation is now performed on a cell-by-cell basis. A cell with at least one lethal aberration is counted as a dead cell, whereas a cell with zero lethal aberrations is counted as a surviving cell. The treatment of lethal aberrations in BIANCA is thus similar to the one of local lethal events in NanOx. Since BIANCA assumes that cell death is only due to chromosome aberrations, it does not consider other origins of cell death. As in NanOx, dose-rate is currently not taken into account in BIANCA.
Finally let us note that similarly to NanOx, other models have been recently proposed to overcome the need of ad hoc non-Poissonian corrections in the MKM and related models. One of them is the generalized stochastic microdosimetric model (GSM2) [14,57], which picks up some of the main assumptions of the MKM to derive a more general and rigorous mathematical formalism. The GSM2 considers both DNA lesion formation and time evolution via a microdosimetric master equation. As other models, it uses microdosimetry spectra (obtained from radiation transport simulations with MC codes such as TOPAS [58]) to include the stochastic nature of energy deposition. Some processes that GSM2 currently does not include are time-delayed repair of DSB and cell apoptosis.
In summary, it is clear that much progress has been achieved in recent years regarding the development of biophysical models able to manage multi-scale approaches and non-Poissonian effects in the description of ionizing radiation effects. We have mentioned here the principles and assumptions on which some of those models are based in order to highlight some similarities and differences with respect to the NanOx model. However, it is out of the scope of this paper to perform a in-depth comparison of NanOx with other models either in terms of predictions or underlying concepts.
Furthermore, it should be mentioned that the implementation in NanOx of radiation stochastic effects at all levels represents a major challenge in terms of computing resources. Relevant approximations were therefore introduced according to the domain of application of the NanOx model. An example of these approximations, quite innovative in RBE modeling, is the separation of the track in core and penumbra, taking advantage of an ion’s track properties for hadrontherapy applications [28]. Finally, very optimized algorithms have been developed in order to make the implementation of the model tractable. They were not mentioned in the paper for the sake of simplicity.
Two aspects that are currently not considered in NanOx are the explicit modeling of radio-induced bystander effects, which are related to the communication between adjacent or nearby cells, and the influence of radiation dose-rate. However, since NanOx predictions are tuned based on experimental data, bystander effects are somehow implicitly included. On the other hand, dose-rate effects may be taken into account in future developments of the model.
5 Conclusion
This work presents a complete description of the formalism of the NanOx model through an exhaustive listing of its main postulates.
The model allows to predict cell survival fractions following irradiations with ions or photons, and consequently to estimate the relative biological effectiveness. NanOx is based on a multiscale description of the interactions between radiation and biological tissues, and accounts for the fluctuations in the energy deposition at several levels: the fluctuations in the number of radiation tracks (or the number of impacts produced by a given macroscopic dose), the stochasticity of the energy pattern along each track, and of the inter-track processes.
The intent of the paper is to stress the fact that the NanOx model offers perspectives of evolution and improvement that are intrinsically related to its formalism. Indeed, by working on NanOx postulates and simplifications, one may explore different scenarios to explain the enhanced radiobiological efficacy of the ions used in innovative radiotherapy techniques such as hadrontherapy, BNCT and TRT with α-particle emitters.
On the other hand, some of the approximations made in the model may be relaxed, and the impact of each one quantitatively evaluated.
Data availability statement
The raw data supporting the conclusion of this article will be made available by the authors, without undue reservation.
Author contributions
MA-A, CM, and MC performed the simulations and wrote the first draft of the paper. MC, ÉT, and MB conceived and developed the NanOx model. ÉT and MB designed and supervised the work and revised the final manuscript. All authors contributed to the article and approved the submitted version.
Funding
This work was performed in the framework of the LabEx PRIMES (ANR-11-LABX-0063) of the Université de Lyon, within the program “Investissements d’ Avenir” (ANR-11-IDEX-0007) operated by the French National Research Agency (ANR). We acknowledge the financial support of the French National Institute of Health and Medical Research (Inserm), through the grant “Apports à l’oncologie de la physique, de la chimie et des sciences de l’ingénieur,” no. 20CP176-00.
Conflict of interest
The authors declare that the research was conducted in the absence of any commercial or financial relationships that could be construed as a potential conflict of interest.
Publisher’s note
All claims expressed in this article are solely those of the authors and do not necessarily represent those of their affiliated organizations, or those of the publisher, the editors and the reviewers. Any product that may be evaluated in this article, or claim that may be made by its manufacturer, is not guaranteed or endorsed by the publisher.
References
1. Schardt D, Elsässer T, Schulz-Ertner D. Heavy-ion tumor therapy: Physical and radiobiological benefits. Rev Mod Phys (2010) 82:383–425. doi:10.1103/RevModPhys.82.383
2. Karger CP, Peschke P. RBE and related modeling in carbon-ion therapy. Phys Med Biol (2018) 63:01TR02. doi:10.1088/1361-6560/aa9102
3. Paganetti H, Niemierko A, Ancukiewicz M, Gerweck LE, Goitein M, Loeffler JS, et al. Relative biological effectiveness (RBE) values for proton beam therapy. Int J Radiat Oncol Biol Phys (2002) 53:407–21. doi:10.1016/S0360-3016(02)02754-2
4. Kanai T, Furusawa Y, Fukutsu K, Itsukaichi H, Eguchi-Kasai K, Ohara H. Irradiation of mixed beam and design of spread-out Bragg peak for heavy-ion radiotherapy. Radiat Res (1997) 147:78–85. doi:10.2307/3579446
5. Furusawa Y, Fukutsu K, Aoki M, Itsukaichi H, Eguchi-Kasai K, Ohara H, et al. Inactivation of aerobic and hypoxic cells from three different cell lines by accelerated 3He-, 12C- and 20Ne-ion beams. Radiat Res (2000) 154:485–96. doi:10.1667/0033-7587(2000)154[0485:IOAAHC]2.0.CO;2
6. Scholz M, Kellerer AM, Kraft-Weyrather W, Kraft G. Computation of cell survival in heavy ion beams for therapy. Radiat Environ Biophys (1997) 36:59–66. doi:10.1007/s004110050055
7. Kase Y, Kanai T, Matsumoto Y, Furusawa Y, Okamoto H, Asaba T, et al. Microdosimetric measurements and estimation of human cell survival for heavy-ion beams. Radiat Res (2006) 166:629–38. doi:10.1667/RR0536.1
8. Inaniwa T, Furukawa T, Kase Y, Matsufuji N, Toshito T, Matsumoto Y, et al. Treatment planning for a scanned carbon beam with a modified microdosimetric kinetic model. Phys Med Biol (2010) 55:6721–37. doi:10.1088/0031-9155/55/22/008
9. Elsässer T, Scholz M. Cluster effects within the local effect model. Radiat Res (2007) 167:319–29. doi:10.1667/RR0467.1
10. Elsässer T, Krämer M, Scholz M. Accuracy of the Local Effect Model for the prediction of biologic effects of carbon ion beams in vitro and in vivo. Int J Radiat Oncol Biol Phys (2008) 71:866–72. doi:10.1016/j.ijrobp.2008.02.037
11. Elsässer T, Weyrather WK, Friedrich T, Durante M, Iancu G, Krämer M, et al. Quantification of the relative biological effectiveness for ion beam radiotherapy: Direct experimental comparison of proton and carbon ion beams and a novel approach for treatment planning. Int J Radiat Oncol Biol Phys (2010) 78:1177–83. doi:10.1016/j.ijrobp.2010.05.014
12. Sato T, Furusawa Y. Cell survival fraction estimation based on the probability densities of domain and cell nucleus specific energies using improved microdosimetric kinetic models. Radiat Res (2012) 178:341–56. doi:10.1667/rr2842.1
13. Cunha M, Monini C, Testa E, Beuve M. NanOx: A new model to predict cell survival in the context of particle therapy. Phys Med Biol (2017) 62:1248–68. doi:10.1088/1361-6560/aa54c9
14. Cordoni F, Missiaggia M, Attili A, Welford SM, Scifoni E, La Tessa C. Generalized stochastic microdosimetric model: The main formulation. Phys Rev E (2021) 103:012412. doi:10.1103/PhysRevE.103.012412
15. Kamp F, Carlson D, Wilkens J. Rapid implementation of the repair-misrepair-fixation (RMF) model facilitating online adaption of radiosensitivity parameters in ion therapy. Phys Med Biol (2017) 62:N285–96. doi:10.1088/1361-6560/aa716b
16. Ballarini F, Carante MP. Chromosome aberrations and cell death by ionizing radiation: Evolution of a biophysical model. Radiat Phys Chem (2016) 128:18–25. doi:10.1016/j.radphyschem.2016.06.009
17. Conte V, Selva A, Colautti G, Hilgers G, Rabus H, Bantsar A, et al. Nanodosimetry: Towards a new concept of radiation quality. Radiat Prot Dosim (2018) 180:150–6. doi:10.1093/rpd/ncx175
18. Cunha M, Testa E, Komova OV, Nasonova EA, Mel’nikova LA, Shmakova NL, et al. Modeling cell response to low doses of photon irradiation—Part 1: On the origin of fluctuations. Radiat Environ Biophys (2016) 55:19–30. doi:10.1007/s00411-015-0621-6
19. Friedrich T, Scholz U, Elsässer T, Durante M, Scholz M. Calculation of the biological effects of ion beams based on the microscopic spatial damage distribution pattern. Int J Radiat Biol (2012) 88:103–7. doi:10.3109/09553002.2011.611213
20. Friedrich T, Ilicic K, Greubel C, Girst S, Reindl J, Sammer M, et al. DNA damage interactions on both nanometer and micrometer scale determine overall cellular damage. Sci Rep (2018) 8:16063. doi:10.1038/s41598-018-34323-9
21. Beuve M, Colliaux A, Dabli D, Dauvergne D, Gervais B, Montarou G, et al. Statistical effects of dose deposition in track-structure modelling of radiobiology efficiency. Nucl Instrum Methods Phys Res B (2009) 267:983–8. doi:10.1016/j.nimb.2009.02.016
22. Beuve M. Formalization and theoretical analysis of the local effect model. Radiat Res (2009) 172:394–402. doi:10.1667/RR1544.1
23. Katz R, Sharma SC. Response of cells to fast neutrons, stopped pions, and heavy ion beams. Nucl Instrum Meth (1973) 111:93–116. doi:10.1016/0029-554X(73)90101-8
24. Katz R, Zachariah R, Cucinotta FA, Zhang C. Survey of cellular radiosensitivity parameters. Radiat Res (1994) 140:356–65. doi:10.2307/3579113
25. Monini C, Testa E, Beuve M. NanOx predictions of cell survival probabilities for three cell lines. Acta Phys Pol B (2017) 48:1653. doi:10.5506/APhysPolB.48.1653
26. Monini C, Cunha M, Testa E, Beuve M. Study of the influence of NanOx parameters. Cancers (2018) 10:87. doi:10.3390/cancers10040087
27. Monini C, Alphonse G, Rodriguez-Lafrasse C, Testa E, Beuve M. Comparison of biophysical models with experimental data for three cell lines in response to irradiation with monoenergetic ions. Phys Imaging Radiat Oncol (2019) 12:17–21. doi:10.1016/j.phro.2019.10.004
28. Alcocer-Ávila M, Monini C, Cunha M, Testa E, Beuve M. Cell survival prediction in hadrontherapy with the NanOx biophysical model. Front Phys (2022) 10. doi:10.3389/fphy.2022.1011063
29. Gervais B, Beuve M, Olivera G, Galassi M. Numerical simulation of multiple ionization and high LET effects in liquid water radiolysis. Radiat Phys Chem (2006) 75:493–513. doi:10.1016/j.radphyschem.2005.09.015
30. Grosswendt B. Recent advances of nanodosimetry. Radiat Prot Dosim (2004) 110:789–99. doi:10.1093/rpd/nch171
31. Garty G, Schulte R, Shchemelinin S, Leloup C, Assaf G, Breskin A, et al. A nanodosimetric model of radiation-induced clustered DNA damage yields. Phys Med Biol (2010) 55:761–81. doi:10.1088/0031-9155/55/3/015
32. Toulemonde M, Surdutovich E, Solov’yov AV. Temperature and pressure spikes in ion-beam cancer therapy. Phys Rev E (2009) 80:031913. doi:10.1103/PhysRevE.80.031913
33. Surdutovich E, Yakubovich AV, Solov’yov AV. Biodamage via shock waves initiated by irradiation with ions. Sci Rep (2013) 3:1289. doi:10.1038/srep01289
34. Wozny AS, Gauthier A, Alphonse G, Malésys C, Varoclier V, Beuve M, et al. Involvement of HIF-1α in the detection, signaling, and repair of DNA double-strand breaks after photon and carbon-ion irradiation. Cancers (2021) 13:3833. doi:10.3390/cancers13153833
35. Ravanat JL, Douki T, Cadet J. Direct and indirect effects of UV radiation on DNA and its components. J Photoch Photobio B (2001) 63:88–102. doi:10.1016/s1011-1344(01)00206-8
36. Douki T, Ravanat JL, Pouget JP, Testard I, Cadet J. Minor contribution of direct ionization to DNA base damage induced by heavy ions. Int J Radiat Biol (2006) 82:119–27. doi:10.1080/09553000600573788
37. Villagrasa C, Rabus H, Baiocco G, Perrot Y, Parisi A, Struelens L, et al. Intercomparison of micro- and nanodosimetry Monte Carlo simulations: An approach to assess the influence of different cross-sections for low-energy electrons on the dispersion of results. Radiat Meas (2022) 150:106675. doi:10.1016/j.radmeas.2021.106675
38. Gervais B, Beuve M, Olivera G, Galassi M, Rivarola R. Production of HO2 and O2 by multiple ionization in water radiolysis by swift carbon ions. Chem Phys Lett (2005) 410:330–4. doi:10.1016/j.cplett.2005.05.057
39. Colliaux A, Gervais B, Rodriguez-Lafrasse C, Beuve M. Simulation of ion-induced water radiolysis in different conditions of oxygenation. Nucl Instrum Methods Phys Res B (2015) 365:596–605. doi:10.1016/j.nimb.2015.08.057
40. Ali Y, Auzel L, Monini C, Kriachok K, Létang JM, Testa E, et al. Monte Carlo simulations of nanodosimetry and radiolytic species production for monoenergetic proton and electron beams: Benchmarking of GEANT4-DNA and LPCHEM codes. Med Phys (2022) 49:3457–69. doi:10.1002/mp.15609
41. Tessaro V, Poignant F, Gervais B, Beuve M, Galassi M. Theoretical study of W-values for particle impact on water. Nucl Instrum Methods Phys Res B (2019) 460:259–65. doi:10.1016/j.nimb.2018.11.031
42. Tessaro VB, Gervais B, Poignant F, Beuve M, Galassi ME. Monte Carlo transport of swift protons and light ions in water: The influence of excitation cross sections, relativistic effects, and Auger electron emission in w-values. Phys Med (2021) 88:71–85. doi:10.1016/j.ejmp.2021.06.006
44. Colliaux A, Gervais B, Rodriguez-Lafrasse C, Beuve M. O2 and glutathione effects on water radiolysis: A simulation study. J Phys Conf Ser (2011) 261:012007. doi:10.1088/1742-6596/261/1/012007
45. Bellinzona EV, Grzanka L, Attili A, Tommasino F, Friedrich T, Krämer M, et al. Biological impact of target fragments on proton treatment plans: An analysis based on the current cross-section data and a full mixed field approach. Cancers (2021) 13:4768. doi:10.3390/cancers13194768
46. Monini C, Cunha M, Chollier L, Testa E, Beuve M. Determination of the effective local lethal function for the NanOx model. Radiat Res (2020) 193:331. doi:10.1667/RR15463.1
47. Schipler A, Iliakis G. DNA double-strand-break complexity levels and their possible contributions to the probability for error-prone processing and repair pathway choice. Nucleic Acids Res (2013) 41:7589–605. doi:10.1093/nar/gkt556
48. Nikjoo H, O’Neill P, Goodhead DT, Terrissol M. Computational modelling of low-energy electron-induced DNA damage by early physical and chemical events. Int J Radiat Biol (1997) 71:467–83. doi:10.1080/095530097143798
49. Pouget JP, Mather SJ. General aspects of the cellular response to low- and high-LET radiation. Eur J Nucl Med (2001) 28:541–61. doi:10.1007/s002590100484
50. Chollier L. Modélisation biophysique de l’interaction des ions à hautes énergies avec de la matière vivante: Application aux traitements de tumeurs par hadronthérapie. Ph.D Thesis. Université Claude Bernard Lyon 1 (2012).
51. Beuve M, Testa E, Dos Santos Cunha M. Procédé d’estimation automatique d’un taux de survie d’une lignée cellulaire irradiée par une dose d d’un rayonnement ionisant (2015). p. FR1554411.
52. Hawkins RB. A statistical theory of cell killing by radiation of varying linear energy transfer. Radiat Res (1994) 140:366–74. doi:10.2307/3579114
53. Hawkins RB. A microdosimetric-kinetic model for the effect of non-Poisson distribution of lethal lesions on the variation of RBE with LET. Radiat Res (2003) 160:61–9. doi:10.1667/RR3010
54. Bellinzona VE, Cordoni F, Missiaggia M, Tommasino F, Scifoni E, La Tessa C, et al. Linking microdosimetric measurements to biological effectiveness in ion beam therapy: A review of theoretical aspects of MKM and other models. Front Phys (2021) 8:578492. doi:10.3389/fphy.2020.578492
55. Carante MP, Aimè C, Cajiao JJT, Ballarini F. BIANCA, a biophysical model of cell survival and chromosome damage by protons, C-ions and He-ions at energies and doses used in hadrontherapy. Phys Med Biol (2018) 63:075007. doi:10.1088/1361-6560/aab45f
56. Carante MP, Embriaco A, Aricò G, Ferrari A, Mairani A, Mein S, et al. Biological effectiveness of He-3 and He-4 ion beams for cancer hadrontherapy: A study based on the BIANCA biophysical model. Phys Med Biol (2021) 66:195009. doi:10.1088/1361-6560/ac25d4
57. Cordoni FG, Missiaggia M, Scifoni E, Tessa CL. Cell survival computation via the generalized stochastic microdosimetric model (GSM2); Part I: The theoretical framework. Radiat Res (2022) 197:218–32. doi:10.1667/RADE-21-00098.1
58. Zhu H, Chen Y, Sung W, McNamara AL, Tran LT, Burigo LN, et al. The microdosimetric extension in TOPAS: Development and comparison with published data. Phys Med Biol (2019) 64:145004. doi:10.1088/1361-6560/ab23a3
Keywords: NanOx, biophysical model, cell survival probability, radiotherapy, oxidative stress, Monte Carlo simulation
Citation: Alcocer-Ávila M, Monini C, Cunha M, Testa É and Beuve M (2023) Formalism of the NanOx biophysical model for radiotherapy applications. Front. Phys. 11:1011062. doi: 10.3389/fphy.2023.1011062
Received: 03 August 2022; Accepted: 20 March 2023;
Published: 04 April 2023.
Edited by:
Julie Constanzo, INSERM U1194 Institut de Recherche en Cancérologie de Montpellier (IRCM), FranceReviewed by:
Rachel Delorme, UMR5821 Laboratoire De Physique Subatomique Et Cosmologie (LPSC), FranceEmanuele Scifoni, Ministry of Education, Universities and Research, Italy
Copyright © 2023 Alcocer-Ávila, Monini, Cunha, Testa and Beuve. This is an open-access article distributed under the terms of the Creative Commons Attribution License (CC BY). The use, distribution or reproduction in other forums is permitted, provided the original author(s) and the copyright owner(s) are credited and that the original publication in this journal is cited, in accordance with accepted academic practice. No use, distribution or reproduction is permitted which does not comply with these terms.
*Correspondence: Michaël Beuve, bWljaGFlbC5iZXV2ZUB1bml2LWx5b24xLmZy