- 1School of Economics and Management, Beijing University of Posts and Telecommunications, Beijing, China
- 2School of Arts & Design, Yanshan University, Qinhuangdao, China
- 3Shijiazhuang Posts and Telecommunications Technical College, Shijiazhuang, China
- 4School of Management, Shijiazhuang Tiedao University, Shijiazhuang, China
Information overload and cocoon effect make the phenomenon of emotional polarization easily appear in online knowledge community. The mechanism of emotional polarization of users in knowledge community is analyzed, so as to reveal the formation rule of users’ emotional polarization in knowledge community and summarize the intervention measures, provide a theoretical basis for further effective control of polarized emotions of knowledge community users. Starting from the theory of social network structure, based on the PAD emotional model, starting from the dimensions of Pleasure, Arousal and Dominance, focusing on the degree of user centrality, an index framework of emotional polarization is constructed around three behavioral patterns of information retrieval, information selection and information interaction. SOR model is used to dynamically explore the polarization mechanism under this framework. The results show that the heterogeneity of social network structure has an effect on participants’ emotional perception and information behavior. The polarization of Pleasure has a positive effect on user centrality, and the polarization of Arousal and Dominance have a negative effect. User centrality positively affects their information selection and interaction behavior, but has no significant effect on retrieval behavior. The emotional polarization in the process of community knowledge sharing is decomposed from different perspectives, and the polarization mechanism is shared by combining social network structure and information behavior. From the perspective of application, this is conducive to promoting knowledge sharing, communication learning and information value chain remodeling, and also provides a kind of insightful analysis paradigm for this field.
1 Introduction
With the popularization of online knowledge community, the relationship between different individuals within it has formed a specific social network. From the perspective of social network structure, there are differences in the opportunities and abilities of audiences to obtain information, and information stratification has emerged in the digital society [1], that is, different network structures in the community have different channels and contents to obtain information, and the ability to effectively use information has an impact on the status of the network society. Users have different status in the network society, and their importance and influence are also different, which will lead to corresponding changes in user behavior.
Emotional polarization refers to the extreme situation in which individual emotion deviates from the normal state, and it is an important influencing factor to induce individual extreme behavior. Multiple values and complex interest demands make users often contain emotional views when commenting on information, such as joy, sadness, anger, criticism, praise, etc. These views have a strong personal subjective color. In the network environment, “dematerialization” and “decentralization” coexist at the same time. On the one hand, online people do not have to be forced to submit to elite views, and on the other hand, online people have no real identity background restrictions [2], which makes extreme emotional consequences invisible. In the online knowledge community, the emotional state of users is closely related to the community network structure. The emotional polarization of users will affect the overall public opinion guidance of the community, and even cause the platform to fall into a bad atmosphere in serious cases.
Therefore, this paper takes online knowledge community ‘Zhihu’ as sample data to conduct research, explore the interaction of emotional polarization, network structure and information behavior, dynamically describe the polarization mechanism of users in the community, provide effective suggestions for better dissemination, use and sharing of content in online knowledge community, and promote the further extension and development of content value chain in online knowledge community. At the practical application level, it provides an insightful reference paradigm for expanding the depth and breadth of content services on the Internet platform, effectively controls and guides the emotions of Internet users, promote the sound development of the knowledge ecology of community platform, provide guarantee for the establishment of a more stable user emotional world and knowledge community platform, promote the feasibility construction of emotional polarization mechanism, and realize the sustainable progress and development of cyberspace.
2 Analysis of research status
This paper uses “social network structure”, “emotional polarization”, “user information behavior” as the subject words to conduct literature retrieval and sorting, summarize and analyze the current theoretical research, and quickly and comprehensively understand the research trends and results of scholars in related fields.
2.1 Research status of social network structure
Online community is a virtual organization form that uses digital technology to jointly create data, information and knowledge [3]. In this form of organization, knowledge collaboration takes place in an unprecedented scale and scope, helping those seeking answers to questions to obtain guidance. Participants improve their professional knowledge by learning from others, so that users can transform between content producers and content consumers.
Social network structure refers to the direct or indirect association mode between social members and the association mode existing in the individual set. It is a type of social network based on individual characteristics and group relations [4]. The essence of online community is the collection of community members and their organizational relationships [5], which divides the structure into three aspects: actors, relationships and connections. The social network structure in the online community can be expanded from the overall network measurement and network centrality analysis. The indicators of the overall network measurement include the density of individuals and community centers, the level of network density, clustering coefficient, etc., which are mainly used to measure the degree of tightness between nodes in the community network [6]. Simpson [7] found that there is direct dynamic feedback between social networks and organizational levels. The intimate relationship and reciprocal relationship between members of organizations promote the emergence of the whole network supporting social ties. The types and attributes of organizational levels in social networks, as well as the relative position of individuals in them, will affect and be affected by status differences; Li Zhuoyu [4] analyzed the social network structure based on the knowledge map theory, and the research results revealed the association relationship and path distance of each node in the online community; Deng Jun et al. [8] found the user size of the online knowledge community is positively related to the value users feel from within the community through the structural equation model. A perfect social network structure is conducive to users’ obtaining a higher sense of self-worth; Gao Xiaoyu [9] took the international students as the node to form a new social network relationship through social media, and explored the relationship between the density and type of social network relationship of international students in the host country and cultural identity from the perspective of social network relationship; Wang Feifei et al. [53] believed that the enterprise decision-making under the leadership of the successor is affected by the social network embedded in it, which is reflected in the structural characteristics and relationship characteristics.
Based on previous studies, it can be found that there is a certain correlation between the social network structure of online knowledge community and user behavior, and the social network structure has become an important factor affecting the polarization mechanism of user behavior. At the same time, the social network structure relationship has more far-reaching application value that is worth exploring.
2.2 Research status of emotional polarization
Scholars have various views on the definition of emotion: Shui et al. [10] believed that emotion is an important research topic in psychology, cognitive neuroscience and many other fields; Ye and Ho [11] believed that the actual change of the individual’s environment is an effective reflection of emotion; Cui Xiaomiao and Wang Yi [12] believed that emotion is a state of emotional arousal with a fixed configuration in the human neuroanatomical system, including four basic emotions: anger, fear, sadness and happiness; Wu Weihua et al. [13] defined emotion as people’s general feelings about real life in a specific era, full of shared values and social psychology; Guo Xiaoan et al. [14] believed that there are differences between emotion and sentiment, specifically, emotion emphasized the external performance of the subject’s reaction to things, and sentiment emphasized the endogenous feelings of the subject. In this regard, this paper believes that emotion and sentiment have the same role in controlling behavior, so this paper makes no distinction between emotion and sentiment.
The emergence of social media and online communities has made people’s communication more convenient. People communicate and express their views through the Internet, which has become an important channel for the public to vent their emotions due to its convenience and inclusiveness. However, due to the anonymity and fragmentation of network information, netizens’ comments are more likely to be distorted or extreme. Users in the network tend to communicate with users with similar views rather than users with opposite positions, so information overload and cocoon room effect make these views extreme [15]. Xing et al. [16] found that social network users with the same opinion have gathered. These users identify with each other and form a group, refusing to accept other different views, which leads to the intensification of group emotional polarization. When the average value of emotions generated by public opinion is greater than the initial value, it indicates that the phenomenon of group emotional polarization has occurred [17]. Yang Guang [19] found that the intensification of emotional polarization is affected by the highly consistent online platform technology with people’s selective contact, motivation reasoning, social identity and social identity mechanism. In addition, Sunstein [20] found that when individuals interact with each other within a group, their emotions and those of the group they belong to will be more extreme, and explained the causes of the irrational network phenomenon; Zhao Wanli et al. [21] believed that emotional polarization is closely related to group polarization, that is, group members have a certain bias at the beginning, after discussion, people continue to move in the direction of bias, and finally form extreme views, so that the groups involved in the discussion fall into an emotional polarization state. At the same time, emotional polarization is also shown in a more macroscopic way: Hobolt et al. [18] carried out an exploration of the western society of emotional polarization. They used surveys and experiments to measure the intensity of the emotional polarization of the parties and the Brexit countries, indicating that emotional polarization can come from identities other than party relations.
As a subjective factor, user emotion affects the direction and atmosphere of community public opinion, and the group emotional polarization has become the focus of scholars’ research.
2.3 Research status of user information behavior
User information behavior includes information retrieval, information selection, information interaction, etc. Information retrieval refers to the process of retrieving effective information according to needs after information is organized, that is, the process of using search engines to find needed information [22]. Generally speaking, users’ demand for information directly leads to their information retrieval behavior. Tan Chunhui et al. [23] built an influencing factor model of information retrieval behavior based on the motivation opportunity capability model, and found that when the motivation, opportunity and capability factors are met at the same time, online knowledge community users are more likely to conduct information retrieval behavior; Deng Shengli et al. [24] concluded that users’ information needs indirectly affect information retrieval behavior by building an information retrieval influencing factor model; Chen Xiaoyu et al. [25] found that information requirements, information satisfaction and affinity will have an impact on information retrieval behavior through regression analysis.
From the perspective of information selection, ensuring the correct adoption of information is critical to successful information seeking, and the benchmark for adoption is whether information is based on needs and can help users make the best decisions [26]. Osatuyi et al. [27] pointed out that the reputation and information quality of contributors are important determinants of the choice of the best answer; Elwalda et al. [28] formed an information adoption model based on information quality and credibility, and proved that social support is the key prerequisite for information quality and credibility by using social support theory and information adoption model; Oliveira et al. [29] studied information adoption characterized by diversification and repeated influence stimuli, and confirmed that labels, forwarding, and influential publishers have significant positive effects on information adoption behavior; Han Zhengbiao et al. [30] revealed the mechanism of emotion in user information behavior model by analyzing literatures related to emotion.
From the perspective of information interaction, Xing Bianbian et al. [31] believed that information interaction includes the acceptance and distribution of information by individuals, which is a two-way information behavior; Tara [32] found that creating personal reputation, establishing or maintaining relationships, and pursuing important commitments in the community will affect users’ observable information interaction behavior.
2.4 Overview of research status
It can be seen from the above domestic and foreign research status that most of the research on online knowledge community users’ emotions and emotional polarization focuses on the overall emotional polarization, that is, emotions are analyzed as a single variable. Few scholars separate emotions into different dimensions, and study the dynamic polarization mechanism of the emotional polarization of each dimension. The existing literature shows that there is a certain correlation between users’ emotions and behavior patterns in the online knowledge community, and the network structure in the community will also affect users’ behavior, but few articles combine the three for research. At the same time, in the research on behavior patterns, domestic and foreign scholars usually study each behavior pattern separately, and few scholars summarize and analyze the behavior pattern as a whole. Based on the above background, this paper explores the emotional polarization indicator framework under the interaction of different dimensions of emotional polarization, network structure and overall users’ behavior mode, dynamically describes the polarization mechanism under this framework, and puts forward relevant suggestions for platform managers and community users.
3 Main concepts and theoretical foundations
This paper analyzes emotional polarization based on the PAD emotional model from Pleasure, Arousal, and Dominance dimensions, taking user centrality as the core and information retrieval, information selection, and information interaction as the three behavioral models to build an indicator framework to describe emotional polarization, so as to determine the background and theoretical basis of the operation mechanism of this paper.
3.1 PAD emotion model theory
Emotion models can be used to describe the types of emotions that arise in humans along the emotional dimension. Good emotion models tend to describe the vast majority of emotional states with a relatively small number of observations. Osgood [33] found that emotional experience can be measured in terms of Evaluation, Potency, and Activity. Mehrabian [34, 35] proposed the PAD emotional model based on this research base for generalization. The PAD three-dimensional emotion space can represent different categories of emotions continuously and smoothly, which in turn can represent the relationship between emotions [36]. It consists of three independent dimensions of Pleasure, Arousal and Dominance. According to the “+” or “-” values of the three descriptive dimensions, 8 kinds of 3D emotion models of 2*2*2 can be generated. In addition, the PAD model can be used in many ways and has the advantages of being fast and intuitive, fault-tolerant, and widely applicable. For example, Guo Yan [37] combined the PAD model to study its role in influencing users’ information behavior; Jiang Ni et al. [38] used the PAD model to assess users’ emotional experience during product use, which can be used to assess task usability, immersiveness, etc., Song Ying [39] constructed a text-oriented 3D emotion computing model for PAD in order to extract the service experience emotion information contained in UGC text. It can be seen that PAD model is more suitable for emotion measurement and can make qualitative judgment on user emotion. Therefore, PAD emotion model is chosen as the theoretical basis in this paper.
3.2 Social network structure
Social network structure can be considered as an organizational structure within a community. It combines micro factors such as individual behavior and individual relationships with the macro world such as social systems and organizational structures through the relationship of networks. Therefore, social network analysis method is widely used in the study of network structure. Social network analysis is a method established for studying the relationships between individuals, informal groups and formal groups. It is often used to reveal the characteristics of interactions between actors [40]. In social network analysis, an individual is regarded as a node in a social network. The connections between different individuals are regarded as the threads connecting the nodes in the social network. The intricate relationships between nodes and threads form the social network.
In this paper, we choose the model constructed by Li et al. [41], which divides the social network into two dimensions: relational dimension and structural dimension. Since this paper focuses on the network structure in online communities and explores where users are in the social network of online communities, this paper selects the structural dimension in social networks. User centrality in the structure dimension is chosen as a variable to measure the structure of the network because it is an indicator of the importance of the individual at the center of the network. According to Wang Lu [42], user centrality indicates the user’s ability to engage with others in the network. This indicates that when a user has a high centrality, he or she is in the center of the entire social network and has more connections with the rest of the information nodes and user nodes, i.e., the user has a “star” effect in the online knowledge community. When the centrality of a user is low, the user is at the edge node of the community location, and the user has little or no connection with the rest of the information nodes and user nodes, so the user can be regarded as non-important and an “orphan” in the online knowledge community network structure.
3.3 User information behavior
User information behavior refers to the user’s behavior related to the acquisition, retrieval, utilization, and proliferation of information. However, because there are many factors affecting information behavior, and the definition of information behavior from different perspectives is also different. Therefore, domestic and foreign scholars do not have a unified view on the concept of information behavior. Vanscoy et al. [43] considered information behavior as an important area of knowledge for consulting service providers because it provides structure for understanding user information seeking and use. Zhang Yaxin [44] defined information behavior as the activities of information acquisition, selection, and utilization carried out by users based on the needs of knowledge resources. Qu et al. [45] argued that user information behavior refers to the user’s use of information technology services to efficiently obtain the information they want, as well as their behaviors of selecting and optimizing information. In general, the specific behaviors of users in online knowledge communities include posting for questioning and sharing purposes, information retrieval and browsing, post selection, commenting and liking, and retweeting. In summary, combined with the characteristics of users’ use of online knowledge communities, this paper argues that users’ information behavior is based on users’ need for certain information, spontaneous information search on the platform, and selection based on the validity of the retrieved information, and finally users will interact with other users for information activities. Therefore, information retrieval, information selection, and information interaction are selected as research indicators in this study.
4 Model construction and research hypothesis
4.1 Stimulus-organism-response model
Mehrabian [46] proposed the stimulus-organism-response model (SOR model), which is used to explain the effect of external stimuli on the organism, which will further stimulate individual behavior. Among them, the stimulus factor represents the external factors. From the macroscopic point of view, the external stimulus to the individual can be political, economic, cultural and other factors. From the microscopic point of view, it can be the individual’s current psycho-emotional, physiological state, etc. The organism factor represents the state of the individual in the macro organization, i.e., the user’s position in the macro network. The response factor represents the individual response, including the feedback given by the user psychologically and physiologically. Individuals are stimulated to change their current state, and the difference in state leads to a difference in individual behavior, which is the main generative principle of this model. Since it was proposed, the SOR model has been widely used in many fields such as sociology and management to study user behavior. Pan et al. [47] explored the impact of social support based on emotion-mediated mechanisms on user engagement behavior in online health communities based on the SOR model. Tian et al. [48] used the SOR model to investigate the impact of users’ use of social e-commerce fashion products on their continuous purchase intention.
In this paper, the PAD emotional model theory, network structure, and theories related to user information behavior are incorporated into the SOR model. Among them, the PAD emotional model corresponds to the stimulus factors of the SOR model, and the polarization of pleasure, arousal and dominance in the emotional model all trigger individuals to be stimulated. The network structure corresponds to the organism factors of the SOR model, and the user centrality measures the user’s position in the network structure, while the user network structure position is the external expression of the organism. User information behavior corresponds to the response factor of SOR model, and information retrieval, information selection, and information interaction in user information model as representative behaviors can reflect the response done by users. For users in online knowledge community, their degree of user centrality in the community is affected by their different degrees of pleasure, arousal and dominance. Individuals with different degrees of user centrality will also show different information behaviors in the community. The framework of the final emotional polarization indicator operating mechanism is shown in Figure 1.
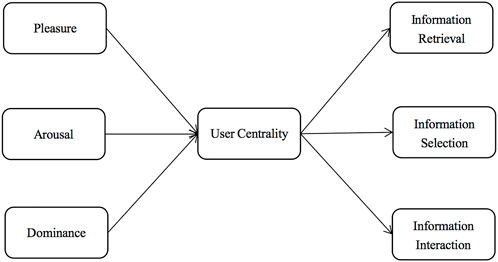
FIGURE 1. Operational mechanism framework of user emotional polarization index in online knowledge community.
4.2 Variable definition and measurement
4.2.1 Pleasure—Arousal—Dominance
Pleasure-P (Pleasure-displeasure) in the PAD three-dimensional emotional model represents the degree to which an individual’s emotional state is positive or negative. Among the many external manifestations of emotional states, “extreme liking” is a state when the degree of pleasure is positively polarized; “extreme disliking” is a state when the degree of pleasure is negatively polarized, both of which can be regarded as having polarized pleasure. The state of “no feeling”, which is neither liked nor disliked, can be regarded as zero pleasure, i.e., the degree of pleasure is not polarized.
Arousal-A (Arousal-nonarousal) in the PAD three-dimensional emotional model indicates the degree of physiological and psychological involvement of the individual. The higher the arousal of the individual, the higher the level of physiological arousal on the nerves and the greater the attentional nature, and vice versa. Among many external manifestations of emotional states, “active participation” is an emotional state in which arousal is positively polarized; “strong resistance” is an emotional state in which arousal is negatively polarized, both of which can be regarded as having polarized arousal. The state of “indifference”, which is neither positive nor negative, is seen as zero arousal, i.e., arousal is not polarized.
Dominance-D (Dominance-submissiveness) in the PAD three-dimensional emotional model indicates the individual’s state of control over situations and others, control and influence over others and the external environment. Among many external manifestations of emotional states, “extreme conceit” is an emotional manifestation with a positive degree of dominance, while “people follow the crowd” is an emotional manifestation with a negative degree of dominance. The emotional state that achieves equality of trust and control between oneself and the surroundings can be considered to have zero dominance.
Based on the research of Mehrabian [46] and Lunardo [49] on emotional states, this paper measured three dimensions by referring to the characteristics of online knowledge communities. As shown in Table 1.
4.2.2 User centrality
User centrality indicates the degree of importance of users in the online knowledge community network structure. When a user is in the central node of the community location, it means that the user has more connections with the rest of the information nodes, user nodes, etc., on the contrary, it means that the user has less connections or even no connections with the rest of the information nodes. Users with high centrality in online knowledge communities often show high extroversion, frequent interaction, high self-monitoring scores, and many direct and indirect relationships. Overall, individuals with higher prestige or social and leadership skills are the ones who have higher user centrality.
Based on the research of Hongseok [50] and Tsai [51] on user network structure centrality, this paper measured user centrality by referring to the characteristics of online knowledge communities. As shown in Table 2.
4.2.3 Information retrieval—Information selection—Information interaction
Information retrieval is the most basic behavior of users in online knowledge communities. Users enter the keywords of the topics they want to retrieve through the retrieval portal in the community to get the posts that meet their retrieval expectations. Information retrieval is one of the three user information behaviors that can best reflect current user topic concerns. Online knowledge communities usually provide users with hot lists below the search bar so that users can quickly search and understand recent hot topics.
Information selection is a kind of behavior that users filter and screen all the information they get when using online knowledge communities. Information selection behavior changes based on factors such as user’s current needs or information matching, and selection behavior is the most persistent behavior among user information behavior. As long as users are still using online knowledge communities, information selection will continue to occur. Based on big data, the online knowledge community platform has added the “Guess Your Favorite” function, which will push different content to different users based on their past information selection behavior.
Information interaction is an act of user participation in topic interaction, which is reflected in adding specified people and topics to the following list, and liking, commenting and retweeting posts in the community. Online knowledge communities often use electronic text format to achieve information interaction. This form can maximize the preservation of document records, ensure the security and readability of information, and effectively avoid information distortion during the interaction process.
Based on the research of Shao [52] on user information behavior, this paper measured user information behavior by referring to the characteristics of online knowledge communities. As shown in Table 3.
4.3 Research hypotheses
4.3.1 Pleasure and user centrality
The polarization of pleasure is manifested as a strong liking or dislike for a topic or user. Polarized pleasure is usually accompanied by the publication of some extreme remarks. The participation process has too strong emotional color, and such too strong self-emotional expression will lead to alienation of other users. The importance of users in the community has decreased, that is to say, the polarization of pleasure is related to the centrality of the user’s knowledge network, and this relationship is reverse. Based on such phenomena, this paper proposed the following assumption:
Hypothesis 1. The polarization of pleasure negatively affects user centrality.
4.3.2 Arousal and user centrality
The polarization of arousal is manifested as excessive attention or resistance to some topics and users. Such behavior will lead to overly one-sided information of users, who tend to wander away from the topic of concern at a low level of information acquisition, and thus cannot undertake the task of leading and coordinating other users and sharing information. Therefore, the polarization of arousal degree is related to the centrality of user’s knowledge network, and this relationship is reverse. Based on such phenomena, this paper proposed the following assumption:
Hypothesis 2. The polarization of arousal negatively affects user centrality.
4.3.3 Dominance and user centrality
The polarization of dominance is manifested as extreme self-confidence or inferiority. The overconfident person will not accept the suggestions of others and are relatively strong, which makes most users resist and isolate the overconfident users. The extreme inferiority will reduce the social attributes and values of users in others’ eyes, and will gradually be marginalized in the community. Therefore, the polarization of dominance has a relationship with the centrality of user’s knowledge networks, and this relationship is reverse. Based on such phenomena, this paper proposed the following assumption:
Hypothesis 3. The polarization of dominance negatively affects user centrality.
4.3.4 User centrality and information retrieval
When the user centrality is high, the user is at the central node of the knowledge network structure. In order to consolidate their importance, users will obtain more information through information retrieval. Therefore, there is a positive relationship between user’s knowledge network centrality and their retrieval behavior. Based on such phenomena, this paper proposed the following assumption:
Hypothesis 4. User centrality positively affects user information retrieval.
4.3.5 User centrality and information selection
With the improvement of user centrality, the importance of users has also been increasing, which has prompted users to strengthen their ability to filter and screen information obtained. At the same time, users with high centrality are more inclined to obtain high-quality information to consolidate their central position. Therefore, there is a positive correlation between user’s knowledge network centrality and user’s choice behavior. Based on such phenomena, this paper proposed the following assumption:
Hypothesis 5. User centrality positively affects user information selection.
4.3.6 User centrality and information interaction
When the user centrality is high, users will have more connections with other user nodes. Because the node has a large interpersonal network, it also generates more interactive behaviors. That is, there is a positive correlation between user’s knowledge network centrality and user’s interaction behavior. Based on such phenomena, this paper proposed the following assumption:
Hypothesis 6. User centrality positively affects user information interaction.
5 Research design and empirical analysis
Based on the SOR model with emotional polarization, network structure, and user information behavior as indicators, and under the guidance of the operating mechanism framework of user emotional polarization indicators of online knowledge community constructed above, the questionnaire designed includes seven variables, namely, pleasure, arousal, dominance, user centrality, retrieval behavior, selection behavior, and interaction behavior. The questionnaire design is divided into two parts: the influence of user’s basic information and emotion on user behavior. In the second part, the Likert five-level scale is used to evaluate the emotional state, network structure, location and behavior of the users in the Q&A community. The respondents were required to score 1–5 levels according to their own experience. The corresponding levels are very disapproval, disapproval, meaningless, approval and very approval.
Taking into account the time cost, economic cost, convenience and other factors, the questionnaire was completed through the online questionnaire production platform “Questionstar”. The distribution and recovery of questionnaires to online knowledge community users through the Internet mainly involve the following channels: Zhihu Community Post Bar, Baidu Knows Community, relevant users’ QQ groups and WeChat groups. From 1 March 2022 to 15 April 2022, a total of 462 questionnaires were collected. Based on the comprehensive consideration of filling time, filling profile and filling IP, 398 valid questionnaires were obtained after strict screening, with an effective rate of 86.1%.
5.1 Descriptive statistics, reliability and validity analysis
This study uses R Studio 4.1.3 software to process the sample data obtained from the questionnaire survey, so as to obtain the basic information of the sample and the mean variance of the variables, and complete the reliability and validity analysis of the sample data.
5.1.1 Sample characteristics
Based on the statistics of the above survey results (Table 4), we can conclude that the survey objects have the following characteristics:
In terms of gender, men accounted for 52.26% of the total number, and women accounted for 47.74% of the total number; In terms of age, users aged from 18 to 25 account for the highest proportion. Such users are generally college students. They have more free time and no life pressure, so they will increase the investment in entertainment. Next are users aged from 26 to 35, accounting for about 1/3 of the total, and users aged from 36 to 45 account for 15% of the total. These two types of users are generally young and middle-aged groups who have already worked. Because of various factors such as personal curiosity and social needs, they will choose to use online knowledge communities to enrich their personal experience and solve work problems in addition to daily work. There are few users under the age of 18 and over the age of 46. Users under the age of 18 are minors. The use of the Internet will be controlled by family, society, software and other aspects. The personal ability of users over the age of 46 tends to be saturated, and the demand for using online knowledge communities decreases. This reflects the youth characteristics of the community; In terms of educational background, about 70% of the people have bachelor’s degree or above, indicating that the groups using online knowledge communities are generally highly educated and users have good knowledge reserves; In terms of occupation, students accounted for the highest proportion, followed by enterprise employees. It can be seen from the age structure that the number of users under the age of 18 is small, but the overall proportion of users is high among middle school students, which indicates that most users of online knowledge communities are college students, masters and doctors. The number of employees in the social structure is large, so the proportion is also high.
Among the online knowledge communities that people frequently browse and use, Zhihu, as the first one, accounts for 72%, which can be mainly attributed to the popularity and authority of the community itself. Baidu Knows also uses more than half of them. The main source of traffic is the promotion function of Baidu Browser, which drives the development of Baidu Knows; In terms of registered use time, users from 1–3 years account for the most, and 90% of users have registered for more than half a year, indicating that the vast majority of users have a certain foundation for the use of online knowledge communities; In terms of the frequency of using online knowledge community, 1/3 of the users use it every day, followed by 3–5 times a week, which indicates that the online knowledge community is very sticky, and the number of times per person uses it every week can reach more than 3 days; In terms of the time spent each time using the online knowledge community, the number of people within 30 min is the largest, accounting for 56%; 30 min to 1 h accounts for 26%, 1 h to 2 h accounts for 11%, and more than 2 h accounts for 7%. It indicates that the average usage time is about half an hour, and the actual user activity is not high, which needs to be improved; In terms of interactive form, 34% of the people participated in browsing, collecting and liking, only 23% of the people browse, and 20% of the people frequently ask questions and actively express opinions.
Based on the above analysis, the main characteristics of the online knowledge community user sample are students aged 18–25 years old, and have a bachelor’s degree or higher education background. The online knowledge community they use most often is Zhihu. The duration of use is concentrated in 1–3 years, and the duration of single use is concentrated in 30 min. The main form of user interaction still focus on basic browsing, collection and likes, but the process of uploading and sharing is lacking.
5.1.2 Descriptive statistics
The results of descriptive statistical analysis is based on the scale data are shown in Table 5. The dimensions are the variables and related item factors. The data range of is 1–5. The mean value of the item is the average user recognition of the item, and the standard deviation indicates the dispersion of user recognition.
It can be seen from Table 5 that the difference between the mean values of each item (PD, AN, DS, CE, SE, CH, IN) and the mean values of the corresponding variables (pleasure, arousal, dominance, user-centrality, retrieval behavior, selection behavior, and interaction behavior) remain within 0.5, indicating that the recognition of each observation variable is high. The standard deviation results of variables and items show that there are no abnormal items in the scale data. The volatility of data is large and the data is scattered. This is due to the data difference caused by the difference in the age, occupation, educational background and other foundations of users in the online knowledge community, which also shows that the online knowledge community is highly inclusive of users. To sum up, this survey data is suitable for testing the reliability and validity.
5.1.3 Reliability and discrimination analysis
Different items of the questionnaire in this study are different ways to describe the interaction of emotional polarization, information behavior and network structure, and to reveal the formation rule of emotional polarization of users in knowledge community. Therefore, there should be no significant differences between the different questions under the same variable. In order to test this indicator, reliability testing is often introduced in scientific research to confirm the reliability of the research data and the need for the study. Cronbach coefficient is the most commonly used reliability measurement method in academia, and when Cronbach’s alpha > 0.8, the questionnaire was considered to have good reliability and research value. In this paper, the R language code was used to test the reliability of the questionnaire and the alpha coefficient was calculated as follows:
The final overall Cronbach’s Alpha value for the questionnaire was obtained as 0.952. In addition, a question-total correlation analysis, i.e., the differentiation of the items, is conducted on the questionnaire and the results are shown in Table 6. Generally speaking, if the correlation coefficient between a certain item and the total score is greater than 0.4, it means that the correlation between a certain item and the total score is high, and the differentiation of this question is good. Combined with the overall Alpha value of the questionnaire, this indicates that the overall reliability of the questionnaire is very high, the variables and items of the questionnaire are very reasonably designed, and the questionnaire is highly usable, stable and reliable.
5.1.4 Validity analysis
Validity analysis is used to reflect the corresponding relationship between structure and measurement value. Factor analysis is widely used in academia to measure the structural validity of the scale and questionnaire. The implementation logic of factor analysis is to extract some common factors from all variables and items. Each common factor is highly related to a certain group of specific variables, and these common factors represent the basic structure of the scale. Because the question-item scales used in this thesis are all well-established scales derived from the relevant literature and the reliability-tested data have high reliability, the validated factor analysis method was chosen to test the hypothesis model.
KMO and Buffett sphere tests should be conducted on the survey data before factor analysis. The closer the KMO value is to 1, the stronger the partial correlation between factors, and the better the effect of factor analysis on data. The lower the significance of Buffett’s sphere test results, the stronger the correlation between items. Academics generally believe that when the KMO value is greater than 0.7 and the significance p-value is less than 0.05, it is suitable for factor analysis. In this paper, using the R language code, the measured value of the scale KM test is 0.92, and the significance p is 0.000, as shown in Table 7, indicating that the scale data has passed the test and is suitable for factor analysis.
The mean extracted variance AVE and the combined reliability CR were calculated by RStudio software, where the mean extracted variance AVE was above 0.5 and the combined reliability CR was above 0.8, as shown in Table 8. The results indicate that the factor corresponds to a more representative topic setting and the convergent validity is ideal.
The analysis of the above data shows that the question options designed in the scale have a certain correlation, and the convergent validity is ideal; at the same time, different variables can be differentiated, and the discriminant validity is ideal; the scale meets the requirements of reasonable design and has a good structural effect; the overall validity of the questionnaire meets the requirements, and the measurement results are true and accurate.
5.2 Model empirical analysis
Based on the previous research samples and data analysis, the AMOS software was used to build a relevant model, and the path coefficients and significance coefficients of the variables were analysed according to the relationship between the variables in the model, so that the correctness of the model and the validity of the previous hypothesis could be verified. As shown in Figure 2:
5.2.1 Model fit
The fitting degree of the research model is obtained through R Studio software, as shown in Table 9.
According to the comparison between the coefficients in the table, model requirements and evaluation criteria, the model is ideal, and the fitting degree of sample data and hypothetical model is high.
5.2.2 Model path and research hypothesis analysis
When p-value of significance level< 0.05, this path can be considered as significant; When the S.E. value is > 0, it indicates that all variables under the assumption do not have the same linear relationship. When the variables are significantly correlated, the larger the path coefficient is, the closer the relationship between variables is. The specific values of this research model are shown in Table 10. According to the results, the degree of pleasure polarization has a positive impact on user centrality; dominance polarization adversely affects user centrality; user centrality has a positive impact on selection behavior and interaction behavior; Arousal polarization adversely affects user centrality; User centrality has no significant effect on retrieval behavior.
The path analysis and research assumptions of the model are as follows:
1) Pleasure and user centrality of online knowledge community
The relationship between the pleasure polarization of online knowledge community and user centrality is positive correlation, that is, the more extreme the pleasure in the emotional direction, the higher the user centrality. The model data results reject the original Hypothesis 1, and the results are contrary to the hypothesis. This is due to the anonymity and high openness of the online knowledge community. Users can hide their real identity and speak freely during the use process, so it is more likely to occur pleasure polarization. Extreme pleasure state is often accompanied by some extreme words, while other users will prefer these information that can intuitively judge the position rather than some absolutely neutral declarative statements in the process of information selection and use. In addition, users will also choose posts with more intense emotional expression to interact, thus increasing the centrality of the original post owner.
2) Arousal and user centrality of online knowledge community
The relationship between the arousal polarization of online knowledge community and user centrality is negative correlation, that is, the more extreme the arousal in the emotional direction, the lower the user’s centrality. The model data results support the original Hypothesis 2. Users whose activeness is polarized tend to show excessive concern or resistance to some topics. Such users’ knowledge is one-sided and tends to focus on specific topics. It is difficult to receive and disseminate various information at the intermediate nodes of the community, which leads to low centrality of such users.
3) Dominance and user centrality of online knowledge community
The relationship between dominance polarization and user centrality in online knowledge communities is negatively correlated, that is, the more extreme the dominance in the affective direction, the more the user centrality. The results of the model data support the original Hypothesis 3. Dominance indicates the degree of control over community topics and other users, and when dominance is too high, users tend to show arrogance, which is not conducive to maintaining interpersonal relationships; when dominance is too low, users tend to lack independent views, follow the crowd and are not valued, both of which lead to low centrality of the user in the community.
4) User centrality and information retrieval of online knowledge communities
The effect of online knowledge community user centrality on information retrieval is not significant, with little difference in retrieval behaviour when using the community between either star users with higher centrality or marginal users with lower centrality. The model data results reject the original Hypothesis 4. This is because information selection and interaction in online knowledge communities are based on retrieval behaviour, so information retrieval is the most basic information interaction. Users retrieve and browse information according to their own needs and hot tweets, and the results returned by retrieval do not differ according to user centrality, so user centrality does not affect the occurrence of information retrieval.
5) User centrality and information selection of online knowledge communities
User centrality and information selection in online knowledge communities are positively correlated, that is, the higher the centrality of users, the stronger the information selection. The model results support the original Hypothesis 5. Users with higher user centrality are in an important position in the network structure and play an important role in the reception and dissemination of information, and users with higher centrality tend to prefer topics and users with high quality, timeliness or relevance to their interests, so as to obtain higher quality and relevant information and maintain their central position in the community network structure.
6) User centrality and information interaction of online knowledge communities
User centrality and information interaction in online knowledge communities are positively correlated, that is, the higher the centrality of users, the stronger the information interaction. The model data results support the original Hypothesis 6. When users are at the central node of the online knowledge community structure, they will frequently interact with other nodes, including but not limited to answering other users’ questions, reposting posts, following and being followed, etc.
6 Research conclusion and emotional polarization intervention measures
6.1 Research conclusion
This paper takes existing research on online knowledge communities’ emotions, network structure and users’ information behaviour as the theoretical basis, combines the SOR model to establish the operational mechanism framework of online knowledge communities’ users’ emotional polarization index, collects data by means of questionnaires, and uses RStudio and AMOS 26.0 to complete the empirical analysis of the questionnaire data, and draws the following conclusions and recommendations.
(1) The polarization of users’ pleasure has a positive impact on their centrality in the network structure, and when users have their own positions and attitudes in terms of emotions rather than absolute indifference and neutrality, it can strengthen their centrality in the community.
(2) The polarization of users’ arousal and dominance has an inverse effect on their centrality in the network structure, and the polarization of users’ dominance has a greater effect on centrality than the polarization of arousal. An excessive focus on one part of the problem or a strong ego or inferiority complex can reduce the importance of the user in the community as a whole and lead to the user being marginalized.
(3) The effect of user centrality on information retrieval is not significant. In online knowledge communities, information retrieval is the most basic interaction behaviour, and the same retrieval behaviour is generated regardless of the user’s position in the network structure of the online community.
(4) The effect of user centrality on information selection and information interaction is positive, and the effect of user centrality on information interaction is greater than the effect on information selection. When users are at the centre of the online knowledge community network structure, they will enhance their selectivity of information and will also interact more with other nodes and information.
6.2 Emotional polarization intervention measures
According to the results of empirical analysis, the author puts forward suggestions on intervention measures of emotional polarization for users and platform managers in online knowledge communities:
Online knowledge communities are platforms where various users interact freely in the community under the supervision and control of the platform. Based on the above research findings, platform administrators should actively promote the community to connect to relevant software, archive all user information behavior and statistics, push information in the information pool differently for different users, control user speech through system control and correctly guide the community atmosphere; at the same time, introduce function points applicable to the community according to the basic elements of the community such as user network structure to meet the needs of different users. Platform administrator should use big data algorithms to manage and operate corresponding knowledge posts according to different network sizes. They can promote knowledge dissemination by setting the top, strengthening knowledge sharing rewards, and reducing the level required for comments [54]. In addition, the interaction mode of online knowledge communities is basically the same, which can be summarised as users posting questions in the community, answering and forwarding, etc. The singularity of the interaction mode limits the development of online knowledge communities, so new function points can be added or existing functions can be optimised to increase the playability and ease of use of the community. For example, the online knowledge community can be divided into a centralised mode and a distributed mode, and users can switch between the two modes freely. The diffusion mode favours the breadth of topics, mainly focusing on current social hotspots; the centralised mode favours the depth of the user’s area of interest, which may involve some proper nouns or some industry terminology, thus satisfying the user’s interests and personalised resources. In this way, the reliance and loyalty of users are enhanced and the economic value of the online knowledge community as a whole is increased.
For users of online knowledge communities, as arousal and dominance can have a significant impact on user centrality, it is necessary to strengthen the acquisition of all aspects of information in online knowledge community, do not pay too much attention to some topics and refuse to accept knowledge in other directions, and maintain a stable attitude. Online knowledge community is a highly inclusive community that everyone can participate in. We should accept all users and information in the community equally. In healthy communities with low level of community rewards and innovation knowledge, it is particularly important to improve emotional support. For example, maintain a civilized and friendly network order and guide users to respect the knowledge achievements of others; establish and improve the content review and user reporting mechanism to automatically delete or independently screen uncivilized content [55]. Reasonable control of their own emotional expression, under the premise of appropriate enhancement of emotional expression, friendly interaction with other users. In addition, the difference in centrality does not affect the user’s information retrieval behaviour, so users should accurately control their own needs in the process of use, and improve their ability to express themselves when retrieving information, their ability to filter information and their own cultural literacy, so as to stop spreading bad information, thus enhancing the individual’s fame and prestige in the online knowledge community, and attracting capital and traffic while enriching themselves.
6.3 Limitations of the study
The model was designed by integrating the existing research base, although some of the findings were achieved, there is still much room for improvement in the article as a whole. For example, the model uses the PAD 3D model of emotion, which is based on static emotions at specific points in time when users participate in filling out the questionnaire, but users’ emotional states may change dynamically at different points in time when using online knowledge communities, so using static nodes to categorize them uniformly is obviously not comprehensive and accurate. In addition, there are many factors that influence the organizational structure of users other than centrality, and only the most obvious and easily statistically measurable centrality has been chosen as the indicator for consideration in this paper. In terms of theory, we can also start with the classical group polarization theory, summarize the connotation, dynamic mechanism and key influencing factors of the emotional polarization in the perspective of social network structure, and propose corresponding intervention measures of emotional polarization according to its generative mechanism and dynamic mechanism. In summary, this study will continue to improve and enhance the data optimization, model construction and theory in the future.
Data availability statement
The raw data supporting the conclusion of this article will be made available by the authors, without undue reservation.
Author contributions
XF and HM designed the study, WL collected the data, and JC performed calculations. WL and JC analyzed and interpreted the data and wrote the manuscript.
Acknowledgments
The authors acknowledge the financial support from the National Natural Science Foundation of China (No. 11905042), Natural Science Foundation of Hebei Province (No. G2021203011), Project of Social Science Development of Hebei Province (No. 20210501003).
Conflict of interest
The authors declare that the research was conducted in the absence of any commercial or financial relationships that could be construed as a potential conflict of interest.
Publisher’s note
All claims expressed in this article are solely those of the authors and do not necessarily represent those of their affiliated organizations, or those of the publisher, the editors and the reviewers. Any product that may be evaluated in this article, or claim that may be made by its manufacturer, is not guaranteed or endorsed by the publisher.
References
1. Shen Y. Research on the emotional polarization of online public opinion in the "Cotton Age" from the perspective of Information Stratification. Future Develop (2022) 46(2):50–3.
2. Gao JF, Huang W. Estimation of emotional polarization of opinion clusters in network public opinion field. Libr Inf Serv (2019) 63(10):106–14.
3. Godinho MA, Borda A, Kariotis T, Molnar A, Kostkova P, Liaw ST. Knowledge co-creation in participatory policy and practice: Building community through data-driven direct democracy. Big Data Soc (2021) 8(1):205395172110194. doi:10.1177/20539517211019430
4. Li ZY. Social network structure of knowledge transmission: A case study of MOOC. Inf Sci (2022) 40(05):180–93.
5. Zhao D, Zhang Q, Ma F. Communication that changes lives: An exploratory research on a Chinese online hypertension community. Libr Hi Tech (2020) 38(4):883–96. doi:10.1108/lht-08-2019-0172
6. Chen HL, Wei RB, Men XP. The Correlation analysis of Social network analysis methods and Research Topics. Inf Sci (2022) 40(09):38–46.
7. Simpson CR. Social support and network formation in a small-scale horticulturalist population. Scientific Data (2022) 9:570. doi:10.1038/s41597-022-01516-x
8. Deng J, Wei Y, Li J, Zhang ZS, Wang R. Intend to research the continuous use of online knowledge community of users, zhihu, for example. J intelligence Sci (2021) 33(05):138–55.
9. Gao XY. Connecting to other countries: The social network structure of students studying in China and the cultural identity of the host country. J Xi'an Int Stud Univ (2022) 30(02):119–23.
10. Shui X, Zhang M, Li Z. A dataset of daily ambulatory psychological and physiological recording for emotion research. Scientifici Data (2021) 8:161. doi:10.1038/s41597-021-00945-4
11. Ye S, Ho KKW. College students' twitter usage and psychological well-being from the perspective of generalised trust: Comparing changes before and during the COVID-19 pandemic. Libr Hi Tech (2022). ahead-of-print. doi:10.1108/LHT-06-2021-0178
12. Cui XM, Wang Y. Red files show the emotional dimensions of communication. Shanxi: Shanxi Files (2022).
13. Wu WH, Miao KP. Self-expression, collective memory and emotional linkage: A collage of short videos. China Journalism Commun Res (2021) 2021(03):187–99.
14. Guo XA, Li H. Emotional labor and emotional labor: Misuse, discrimination and its interpretation of the concept of. Press (2021) 2021:56–68. doi:10.15897/j.carolcarrollnki/g2.20211015.002cn51-1046
15. Jiang S, Wang H. Group polarization based on agent emotional characteristics and credibility. Complexity (2021). doi:10.1155/2021/9983541
16. Xing Y, Wang X, Qiu C, Li Y, He W. Research on opinion polarization by big data analytics capabilities in online social networks. Technol Soc (2022) 68:101902. doi:10.1016/j.techsoc.2022.101902
17. Carvalho VDH, Costa APCS. Towards corpora creation from social web in Brazilian Portuguese to support public security analyses and decisions. Libr Hi Tech (2022). ahead-of-print. doi:10.1108/LHT-08-2022-0401
18. Hobolt SB, Thomas J, Tilley J. Divided by the vote: Affective polarization in the wake of the Brexit referendum. Br J Polit Sci (2021) 51:1476–93. doi:10.1017/s0007123420000125
19. Yang G. Do intelligent media exacerbate the polarization of public opinion? - based on media technology, information characteristics and individual psychological analysis. J youth press (2022) 18:15–9. doi:10.15997/j.carolcarrollnkiQNJZ.2022.18.011
20. Sunstein CR. The law of group polarization. J Polit Philos (2002) 10(2):175–95. doi:10.1111/1467-9760.00148
21. Zhao WL, Xie R. Digital inequality and social stratification: The effect of information communication technology on social inequality. Sci Soc (2020) 10(01):32–45.
22. Wu J, Hong R, Tian Q. Special issue on cross-modal retrieval and analysis. Int J Multimedia Inf Retrieval (2022) 11:523. doi:10.1007/s13735-022-00265-2
23. Tan CH, Ren JH. Research on the combination of factors influencing users' information searching behavior in virtual academic community: A qualitative comparative analysis method based on Fuzzy Sets. Mod Inf (2022) 42(04):39–51.
24. Deng SL, Chen XY, Fu SX. Research on the influence of users' information demand on information search in social question-and-answer community -- based on the mediating role of question-and-answer community involvement. Inf Sci (2017) 35(07):3–15.
25. Chen XY, Fu SX, Deng SL. Research on the influencing factors of social question and answer users' information search: A mixed approach perspective. Libr Inf Serv (2018) 62(20):102–11.
26. Sarthak C, Sabuj KC. Unfolding social learning parameters behind the selection of information sources against cyclones: A perspective of information seeking behavior research. Libr Inf Sci Res (2022) 44(4):101194. doi:10.1016/j.lisr.2022.101194
27. Osatuyi B, Passerini K, Turel O. Diminishing returns of information quality: Untangling the determinants of best answer selection. Comput Hum Behav (2022) 126:107009. doi:10.1016/j.chb.2021.107009
28. Elwalda A, Erkan İ, Rahman M, Zeren D. Understanding mobile users' information adoption behaviour: An extension of the information adoption model. J Enterprise Inf Manage (2022) 35(6):1789–811. doi:10.1108/jeim-04-2020-0129
29. Oliveira JF, Marques-Neto HT, Karsai M. Measuring the effects of repeated and diversified influence mechanism for information adoption on Twitter. Soc Netw Anal Min (2022) 12:16. doi:10.1007/s13278-021-00844-x
30. Han ZB, Zhai RR. Research on the mechanism of emotion in user information behavior model. Inf Theor Pract (2022) 45(12):103–10.
31. Xing DB, Zhang WN. Research on the emotional experience of archival we-media users based on information interaction behavior. Arch Construction (2019) 2019:35.
32. Zimmerman T. Social noise: The influence of observers on social media information behavior. J Documentation (2022) 78:1228–48. doi:10.1108/jd-08-2021-0165
33. Osgood CE. Dimensionality of the Semantic space for communication via Facial expressions. Scand J Psychol (1966) 7(1):1–30. doi:10.1111/j.1467-9450.1966.tb01334.x
34. Mehrabian A. Pleasure-arousal-dominance: A general framework for describing and measuring individual differences in temperament. Curr Psychol (1996) 14(4):261–92. doi:10.1007/bf02686918
35. Mehrabian A, LjunggrEn E. Emotional correlates of preferences for situation-activity combinations in everyday life. Genet Soc Gen Psychol Monogr (1997) 123(4):461–77.
36. Sun Y, Ma HJ, Zhang XY. PAD under the dimension of depth emotion associated modeling study. J Electron Des Eng (2022) 30(07):47–52. doi:10.14022/j.issn1674-6236.2022.07.010
37. Guo Y. A study on the information behavior of users in social question-and-answer community based on PAD emotion model. Mianyang, China: Southwest university of science and technology (2020).
38. Jiang N, Li RN, Liu CY, Fang H. PAD emotion model application in the user's emotional experience evaluation. J packaging Eng (2021) 22:413–20.
39. Song Y. Research on multidimensional emotion computing model for user experience quality. Shanghai, China: Donghua University (2022).
40. Zhang SH, Wu YT, Yu HS. Urban innovation correlation network in Shandong Province based on improved gravity model and social network analysis. J Ludong Univ (2022) 38(04):342–9.
41. Li YQ, Huang Y. Relationship between individual characteristics and social network: Its localization development. Adv Psychol Sci (2014) 22(11):1801–13. doi:10.3724/sp.j.1042.2014.01801
43. VanScoy A, Julien H, Harding A. Integration of information behavior into reference and information services education: A syllabus study. J Educ Libr Inf Sci (2022) 2022:20220.
44. Zhang YX. Research on personal information network infringement. Shenyang, China: Shenyang University of Technology (2016).
45. Qu NW, Xia ZJ, Wang YM. Based on users' information behavior of social media rumours effect research. J intelligence Sci (2021) 33(01):111–9. doi:10.13833/j.iSSN.1007-7634.2021.01.015
47. Pan TT, Lv YJ. Study on the influence factors of user participation behavior in online health community based on SOR model. Inf Serv (2002) 43(02):76–83.
48. Hewei T, Youngsook L. Factors affecting continuous purchase intention of fashion products on social E-commerce: SOR model and the mediating effect. Entertainment Comput (2022) 41:100474. doi:10.1016/j.entcom.2021.100474
49. Lunardo R, Mbengue A. Perceived control and shopping behavior: The moderating role of the level of utilitarian motivational orientation. J Retailing Consumer Serv (2009) 16(6):434–41. doi:10.1016/j.jretconser.2009.06.004
50. Oh H, Kilduff M. The ripple effect of personality on social structure: Self-monitoring origins of network brokerage. J Appl Psychol (2008) 93(5):1155–64. doi:10.1037/0021-9010.93.5.1155
51. Tsai W, Ghohal S. Social capital and value creation: The role of intrafirm networks. Acad Manage J (1998) 41(4):464–76. doi:10.5465/257085
52. Shao G. Understanding the appeal of user-generated media: A uses and gratification perspective. Internet Res (2009) 19(1):7–25. doi:10.1108/10662240910927795
53. Wang FF, Wu J, Lu YZ, Zhu Y. Research on the impact of the characteristics of social network embedded by successors on inefficient investment -- an empirical analysis based on structural and relational embeddedness. Investment Res (2022) 41(11):4–24.
54. Chu JW, Li JX. Research on the evolution of user's knowledge sharing strategy in virtual knowledge community -- based on scale-free network theory. Inf Sci (2022) 2022:1–9.
Keywords: knowledge community, social network structure, emotional polarization, mechanism, Zhihu
Citation: Li W, Chen J, Ma H and Feng X (2023) Research on emotional polarization mechanism of knowledge community from the perspective of social network structure —An empirical study on ‘Zhihu’ question and answer learning community. Front. Phys. 11:1139475. doi: 10.3389/fphy.2023.1139475
Received: 07 January 2023; Accepted: 28 February 2023;
Published: 10 March 2023.
Edited by:
Ye Wu, Beijing Normal University, ChinaReviewed by:
Fatao Wang, Hebei University of Economics and Business, ChinaLili Feng, Hebei GEO University, China
Copyright © 2023 Li, Chen, Ma and Feng. This is an open-access article distributed under the terms of the Creative Commons Attribution License (CC BY). The use, distribution or reproduction in other forums is permitted, provided the original author(s) and the copyright owner(s) are credited and that the original publication in this journal is cited, in accordance with accepted academic practice. No use, distribution or reproduction is permitted which does not comply with these terms.
*Correspondence: Hongjing Ma, MjUxMjcxNDM3QHFxLmNvbQ==