Research on the generation mechanism and characteristics of an Energy Internet backbone network
- 1North China University of Water Resources and Electric Power Institute of Management and Economics, Zhengzhou, Henan, China
- 2Hefei University of Technology Institute of Management, Hefei, China
- 3State Grid Economic and Technological Research Institute of Henan Province, Zhengzhou, China
- 4North China Electric Power University Institute of Economics and Management, Beijing, China
- 5Beijing Key Laboratory of New Energy and Low-Carbon Development (North China Electric Power University), Beijing, China
The Energy Internet adopts the mechanism of “regional coordination and hierarchical control” to realize the clean power compatibility and reliability in power operation. In the network topology, the traditional tree network is transformed to the hierarchical partition network. First, this paper analyzes the topological features of “hierarchical control, intra-layer partition, interregional interconnection, and regional autonomy” of the Energy Internet. On this basis, the hierarchical ring network autonomy (HRNA) topological generation and evolution mechanism of the Energy Internet is proposed, and the different levels of a Beijing power grid framework are taken as an example to expand and evolve to the Energy Internet. Based on the comparison and analysis of the network characteristics constructed in this paper with relevant literature studies, this mechanism generates a network that is close to the Internet in terms of average degree, network diameter, and aggregation coefficient. However, there is no centrality node with a higher degree of nodes in the Internet topology, which better reflects the equivalence concept of the Energy Internet.
1 Introduction
The Energy Internet is regarded as the future development direction to solve the problems of clean energy compatibility, deep and efficient control, and safe and stable operation of a power system [1–3]. The structural characteristics of “regional autonomy and hierarchical regulation” of the Energy Internet have also been recognized by more and more experts and scholars [4, 5]. Considering the continuity and economy of power system construction, the construction of the Energy Internet does not completely remove the existing power grid but relies on further improvement and adjustment based on the existing power grid structure.
Scholars have studied and expounded the concept, architecture, key technologies, and management schemes of the Energy Internet and constructed its concept and architecture from several levels such as family energy LAN, urban Energy Internet, regional Energy Internet, and global Energy Internet. Home energy LAN is an energy management network at the level of family buildings, including renewable energy power generation equipment, distributed controllable power generation equipment, energy storage system, and various intelligent loads [6]. The urban Energy Internet emphasizes future energy supply and consumption operation patterns and energy management models [7]. The regional Energy Internet focuses on the coupling of network systems such as power networks, electrical transport networks, natural gas networks, and information networks [8]. The global Energy Internet builds a ubiquitous and strong energy network with the UHV power grid as the backbone to promote clean substitution and electric energy substitution [9].
Since the concept of the Energy Internet was put forward, countries worldwide have successively conducted ELAN-level experimental projects. In 2008, Germany selected six pilot regions based on the smart grid for a 4-year “E-Energy” technology innovation promotion plan [10]. “Vision of future energy networks” (VoFEN), a research program executed by the Swiss Federal Institute of Technology, proposes a definition of “energy hub networking” [11, 12]. The National Science Foundation of the US proposed the construction of a future renewable electric energy delivery and management (FREEDM) network [13–15]. In 2011, “Digital Grid Alliance” in Japan advocated the “Digital Grid” and proposed to use “power routers” [16, 17] to coordinate and dispatch power in a certain area. The National Energy Administration and energy companies in China have paid great attention to the development process of the Energy Internet and have initialized multiple experimental projects at microgrid [18], ELAN [19],and urban Energy Internet [20] levels.
In summary, as the evolution direction of the power system in the future, the Energy Internet has been generally recognized. The research on the Energy Internet in various countries is developing from concept proposals and architecture design to plot the implementation stage. At present, the research on the Energy Internet is in the stage of “gradually clarifying the concept, generally agreeing on the framework, and conducting research on key technologies point by point.” The macro topology of “Hierarchical Regulation and Regional Autonomy” of the Energy Internet has been generally agreed. At present, the research results of the network networking mode and network characteristics are mainly “HUHM” and “Complex Hybrid Network.” However, there are some defects, such as the mismatch between the internal structure and energy distribution of the network and the deviation of evolution direction. Therefore, the typical research results in the current literature are compared, and the commonness of advanced grid structures at home and abroad is analyzed in order to provide a research basis for the construction of a macro topology model of the Energy Internet. It is urgent to study the evolution mechanism and network characteristics of the Energy Internet based on the current power system structure.
2 Literature review
2.1 Evolution mechanism and topology of a power network
How to topologicalize a real power system into a simulation network or generate a complex network similar to the characteristics of a power network is the starting point of studying the structure and characteristics of a power system network. At present, relevant research is mainly carried out from the perspectives of the direct topology of a power network, evolution generation based on a complex network, and network reconfiguration considering physical characteristics.
① Direct topology of the power system. Taking the main equipment of the power system as the node and the physical adjacent or influence relationship between the equipment as the connection edge is a common way to solve the topology of a small power grid. Some studies directly topologicalize IEEE39, IEEE118, and IEEEE300 standard networks and conduct simulation analysis based on the obtained networks [21, 22]. Some scholars directly topologicalize the real local power grid to improve the imitative effect of simulation research. For example, [23] directly topologicalized Iran’s 400-kV and 230-kV levels of the power grid structure when studying grid vulnerability. [24] analyzed the centrality of important nodes in the real power grid structure of Guangdong Province in China. ② Evolutionary generation based on the complex network. This method refers to the generation mechanism of complex networks to gradually evolve into a composite grid with similar characteristics to the real grid and studies the properties of a composite grid with different node sizes. The neighbor set information based on preferential attachment (NSIPA) in [25] evolves into the western power grid of the United States, the European power grid, and the Australian power grid. [26] simulated the German, Spanish, and French power grids that are consistent with the actual power grid parameters. [27] chose the network imitating method based on learning (NIMBLE) to generate the interconnected power grid in the western United States. ③ Network reconstruction considering physical characteristics. Some research studies are not satisfied with studying grid performance only from the network structure level and introduce physical characteristic parameters into nodes and edges to generate a weighted graph and directed graph, so that the network can represent the operation mechanism. Related typical studies mainly include the identification of vulnerable nodes by the weighted edge of electricity reactance [28], the study of network vulnerability by weighting power to nodes and edges [29], and the establishment of a weighted network based on power flow to study cascading failure [30].
2.2 Research on power grid characteristics based on the complex network
Power system topology analysis and complex network characteristics have always been the focus of grid vulnerability and cascading fault research. From the end of the 1990s, the study of the network structure of large complex systems [31–33] emerged, and it was soon applied in biology, sociology, transportation, and computer Internet industries, including electricity. Research on complex networks in the power system began in 1998 in the United States Cornell University marked by Watts and Strugatz, who found that the US power grid was a small-world (SW) network [34]. At present, the research on complex networks in the power system analyzes vulnerability and elasticity from small-world and scale-free properties, community structure, and centrality.
①Small-world and scale-free feature analysis. This kind of research studies the possibility and key path of cascading failure of a power grid by analyzing the small-world property and power–law characteristics of degree distribution of a real power grid. Many actual power grids have been confirmed to have small-world characteristics, for example, the Northern China Power Grid, the North China Power Grid, the Western United States Power Grid, and the Brazilian Power Grid [35–38]. Some scholars have pointed out that some power grid structures have certain scale-free characteristics [31, 39]. ② Research on the community structure and centrality. Some studies identify key nodes and links by detecting community structures and centrality nodes in power networks. [40, 41] identified the key nodes of each IEEE standard network and several typical national grids from the aspects of network centrality and electrical centrality, and [24] proposed seven power network centrality metrics. For the grid community structure, [42] proposed the Newman algorithm to detect Italian grid community institutions and network partition, and [43] constructed an intergenerational genetic algorithm GGA + to detect European and North American grid community structures. ③ Network vulnerability and elasticity analysis. The research on the reliability of a power system from the network level is the focus of power network research, and the research hotspots are evolving from robustness and vulnerability to elasticity. Representative studies mainly include the overall robustness analysis of the network [43–45], the vulnerability identification of key nodes and links [46–52], and the dynamic reconfiguration of the elastic grid [53].
2.3 Research on the power network structure for Energy Internet
The Energy Internet, which has the characteristics of deep integration of power information, coordination in “source-grid-load-storage,” and stratified regional autonomy, is the main direction of future power network evolution. Future-oriented research on the power network structure is conducive to the orderly development of a power network. At present, the research of the Energy Internet topological network is mainly carried out from two aspects, interdependent binary network structure and macroscopic unified topology structure.
① Interdependent binary network structure. The research of the interdependent complex network mainly focuses on the characteristics of physical information fusion of the Energy Internet and studies the coupling mechanism and network performance based on the analysis of the power network and information network structure. The concept of an interdependent network and its vulnerability analysis framework are proposed by [54], which provides a theoretical basis for analyzing the interaction between coupling systems. In [55], the interdependence strategy of the power node and information node is elaborated, and the vulnerability point and network edge optimization strategy under different dependency strategies are analyzed. [56] studied the similarity of the topological structure between a power network and information network and proposed a structural stability enhancement strategy based on the vulnerability characteristics of low-degree nodes in a power information fusion system. Regarding the node correspondence scheme of the power information interdependence network, there are many structures such as one-to-one [54], one-to-many [57], many-to-many [58], partial dependence [59], multiple dependence [60], and heterogeneous correspondence [61].② Research on macroscopic unified topological features. In view of the characteristics of “hierarchical control and regional autonomy” of the Energy Internet, some scholars have made exploratory research from the direction of the macro topology of the Energy Internet. On the basis of establishing the backbone network, [62] layered an interconnection of micro-network nodes with different probabilities and constructed a macroscopic unified topology of the Energy Internet. [63] studied the evolution path of the Energy Internet topological nodes and proposed that the Energy Internet has chaotic typing characteristics in the structure. [64] introduced the system network analysis model based on the grid hierarchy and topology structure and put forward the idea of topology analysis of a large power grid under the trend of the Energy Internet based on graph theory. In the study of cascading faults of the power information physical fusion system in the literature [65], the generation mechanism of topological structure evolution of power CPS is constructed based on the seepage theory. [66] constructed an improved biogeography-based optimization (BBO) algorithm to evolve the IEEE-39 and IEEE-118 networks into a hierarchical and partitioned Energy Internet structure.
The above comprehensive analysis of the research on the complex network of the power system and the topology structure of the Energy Internet shows that ① the research studies on the evolution law and characteristics of power grid from the perspective of topological structure analysis and complex network generation have gained a general consensus among scholars and ② topology research of the complex power system under the trend of Energy Internet evolution is still in the exploratory stage. Therefore, starting from the current power grid architecture and considering the characteristics of the Energy Internet, this paper proposes the layered and zoned topology evolution mechanism of the Energy Internet backbone network based on the existing power grid structure and studies the characteristic parameters of the network by taking the Beijing power grid as an example.
3 Characteristics of the hierarchical partitioning structure of the Energy Internet
The realization of energy utilization efficiency optimization and power quality improvement of the Energy Internet relies on its hierarchical distributed control architecture and operation mechanism of “regional autonomy and global coordination” [67]. The hierarchical control of the traditional power system and the hierarchical distributed structure of the Energy Internet are shown in Figure 1.
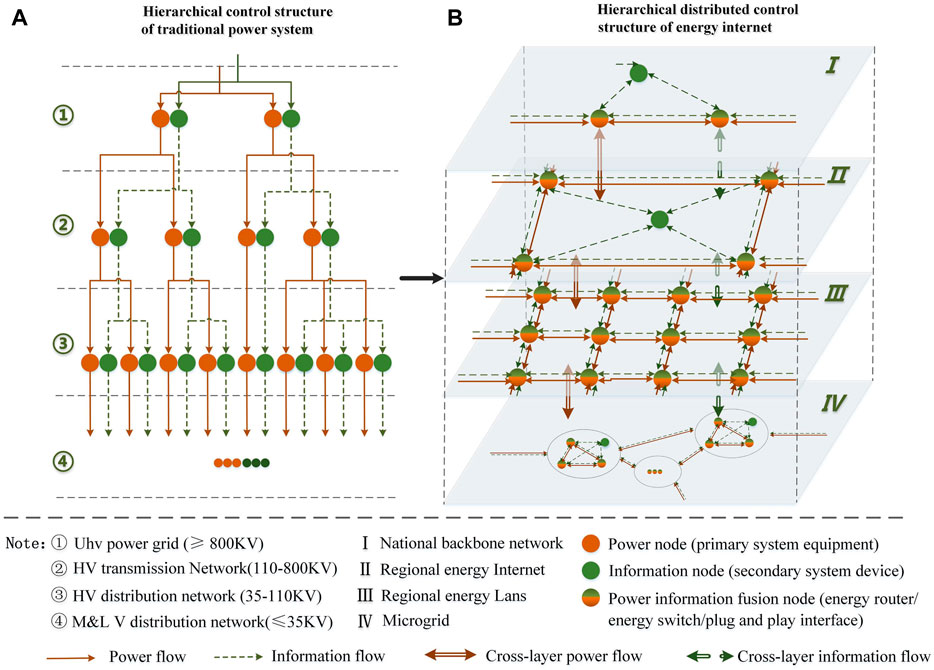
FIGURE 1. Comparison between the traditional power system control structure and Energy Internet autonomy structure. (A): Hierarchical control structure of traditional power system. (B): Hierarchical distributed control structure of energy internet.
The traditional power grid controls the power equipment from top to bottom. Hierarchical management is realized according to the voltage level in different geographical locations, and the power system control is realized through the dependence between the physical and information functions of the equipment. In the Energy Internet, the layering of the power grid is to meet different functional requirements and is no longer limited by the geographical location. The power equipment has realized the information physical fusion, and the same functions are in the same level of management.
3.1 Characteristics of the regional autonomous structure of the Energy Internet
Compared with the top–down tree control structure of the traditional power system, the Energy Internet realizes decentralized and centralized control through a “regional autonomy” mechanism.
On one hand, the Energy Internet requires the two-way flow of information and energy within and between layers. It is difficult to respond to communication delays in a timely manner using the traditional centralized control model, and the use of the traditional centralized control model to process massive, real-time, and nonlinear data requires powerful computing power and high-speed information channels. The “regional autonomy” control mode of distributing the control center in the local area network at all levels is in line with the development direction of information technology such as distributed computing.
On the other hand, through demand-side management, the realization of a high penetration rate of renewable energy generation, optimal utilization of regional energy, and plug-and-play of distributed equipment are the development direction of intelligent distribution grids. In this context, the demand side of the power system is more random in the operation state, and even the change of topology, regional intelligent regulation with short channels, and fast response provide an effective solution. In this regard, the realization of the interconnection of regional grids of the same level and the multi-channel response of control centers at different levels is the physical basis for the implementation of a hierarchical distributed control of the Energy Internet.
3.2 Characteristics of the hierarchical regulation structure of the Energy Internet
Compared with the regional distributed autonomous model, the hierarchical control architecture is widely studied and applied in the power system, different from the traditional hierarchical model of the power system based on geography and grid hierarchy. The Energy Internet also emphasizes the layering of functional requirements.
The traditional hierarchical mode based on geographical planning and voltage level is conducive to the isolation of local fault risk and the upgrading of the power grid. However, in the hierarchical distributed control mode, the inefficiency is caused by the delay of the communication channel. In view of the layering of the Energy Internet, scholars pay more attention to layering from the control function, including the energy router layer, the energy switch layer, the energy interface layer, and other layering methods. Through hierarchical control, the energy efficiency, energy supply quality improvement, and energy balance optimization between each layer of the network are realized.
It can be seen from the above that relying on the physical architecture of the existing power system, on the basis of the existing hierarchical mode, according to the functional requirements of the Energy Internet, the connectivity and intelligent planning and transformation of the power network through energy routers, energy switches, and plug-and-play interfaces are the scientific models for the development of the physical architecture of the power system.
4 Energy Internet backbone network generation mechanism
In this section, according to the characteristics of the hierarchical and partitioned structure of the Energy Internet in the previous section, the layered ring network architecture of the Energy Internet is proposed, and an evolution model is constructed.
4.1 Energy Internet HRNA topological architecture model
Compared with the traditional tree-shaped power supply network, the backbone network under the Energy Internet has certain differences in function and architecture. In terms of function, the backbone network of the Energy Internet is mainly responsible for the access to the trans-regional UHV transmission network, regulation and coordination of local large-scale power generation bases and energy storage equipment, and interaction between different layers of the regional Energy Internet. In terms of architecture, in order to ensure the reliability of power supply and the interaction and coordination between energy routers, the backbone network will develop in the direction of a ring-like network structure with high connectivity and sufficient routing on the basis of a vertical tree.
Analyzing the internationally reliable power grid structures (Singapore and Paris) shows that they all have characteristics such as a “dumbbell” structure, good scalability, high reliability, and sufficient redundancy. Specific designs are made based on the administrative and load distribution characteristics of the region. The comparative analysis of the structural characteristics of international advanced power grid grids is shown in Table 1.
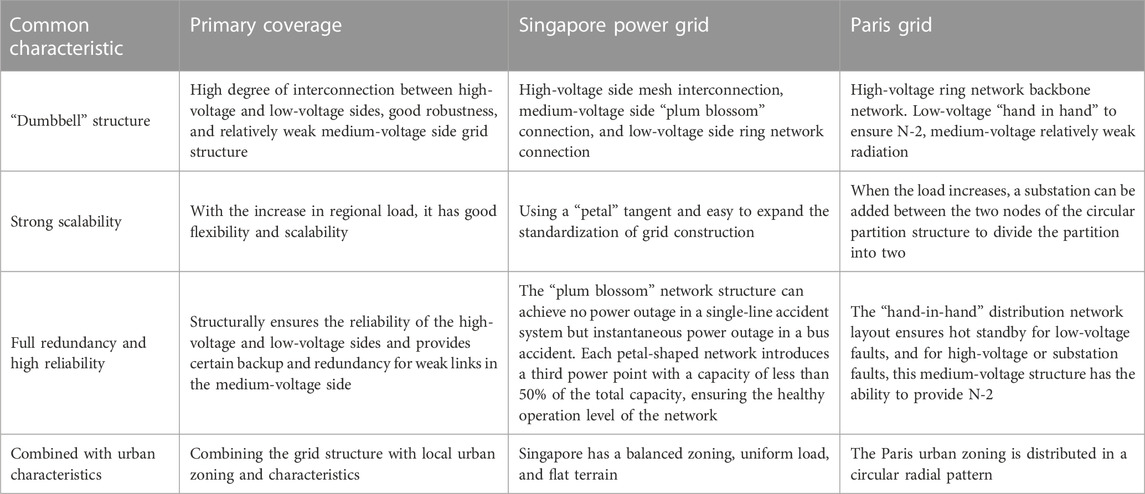
TABLE 1. Comparative analysis of common characteristics of international advanced power grid structures.
Improving power supply reliability while maximizing compatibility with renewable energy access is the main goal of Energy Internet construction. The internationally reliable power grid architecture provides a topology evolution approach for Energy Internet construction. At the same time, an example of topology evolution using the Beijing power grid was presented in Article 5.2 and compared with the Central China 500-kV power grid [57] Energy Internet generated by the HUHM model [64], Internet Network, and US Grid structures.
Considering the reality of the gridded management of the power system and the construction foundation of the Chinese power grid ring network, this paper proposes a layered ring-like network autonomous topology architecture model for the Energy Internet of “hierarchical autonomy, intra-layer partitioning, intra-regional ring formation, and inter-ring interconnection" (Hierarchical Ring Network Autonomy, HRNA), as shown in Figure 2.
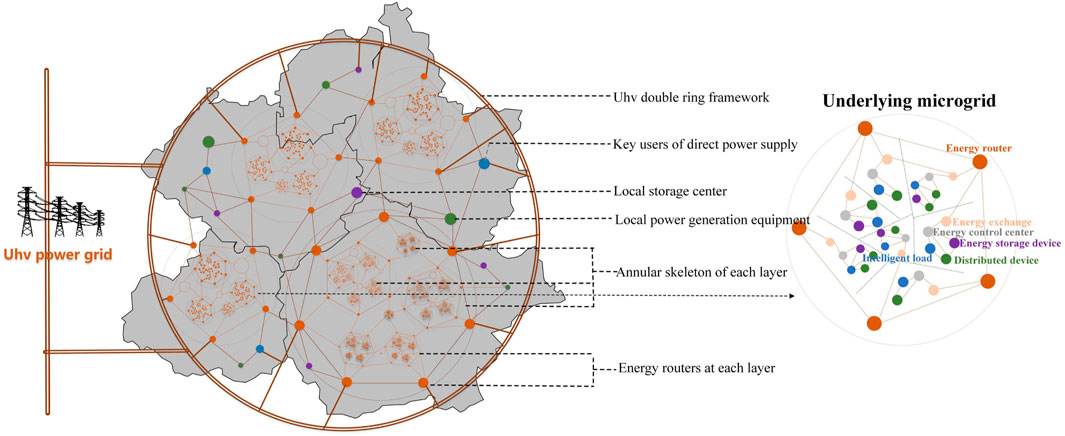
FIGURE 2. Schematic diagram of hierarchical ring network topology of Energy Internet backbone network.
Figure 2 considers the continuity and economy of Energy Internet architecture construction. Referring to the “dumbbell” structure of the foreign advanced power grid, this paper constructs the macro topology of the Energy Internet, and the national backbone node adopts the double-ring radial connection structure with good structural connectivity. The regional energy routing node adopts a hierarchical ring network structure of “intra-layer partitioning, intra-zone ringing, and inter-ring interconnection." It ensures structural reliability and scalability flexibility while maximizing compatibility with existing network structures. The underlying micro-grid adopts the network interconnection with sufficient interconnection and ensures the mutual support of the underlying autonomous units through the structure. Different autonomous units at the same level are connected through electronic devices such as soft switches, and it realizes power transmission or bidirectional power control between different ring networks.
The dual-loop network, backbone node of the country in the Energy Internet, is mainly responsible for the access and scheduling of the trans-regional UHV transmission network. The regional hierarchical ring network mainly coordinates energy routers at all levels, local large-scale power generation bases, and energy storage equipment. The fully interconnected underlying micro-grid ensures the full interaction and efficient coordination of autonomous units through a strong architecture.
4.2 Parameters of the Energy Internet HRNA topology generation mechanism
On the basis of the abovementioned hierarchical, partitioned, and gridded power grid, relevant parameters are set, and the backbone network structure of the Energy Internet is constructed.
The relevant parameters of each level and partition are set as follows:
The partition level of the research area in the grid division process has
The number of partitions of the layer
The number of nodes in the layer
The number of nodes in partition
In the process of generating the backbone network structure of the Energy Internet, the connectivity and average degree of the network are directly related to the reliability requirements of the nodes, and backup requirements vary with the level of the power supply area. In this paper, considering the complexity of power supply in the Energy Internet environment, the current regional grid planning standards for different levels of power distribution are appropriately improved, and energy storage centers are added at important nodes for regulation and backup.
4.3 Evolution mechanism of HRNA backbone network generation in the Energy Internet
On the basis of the above research, the Energy Internet backbone network is networked through the relevant knowledge of graph theory, and the specific steps are as follows.
STEP 1: Generating the UHV double-ring skeleton.
UHV transmission line landing nodes in the outer ring and the inner ring are planned, which radiate from the outer ring to the inner ring. The number of nodes in the outer link is
All edges meet the following criteria.
①when
②when
③
STEP 2: Connecting each layer and each partition into a ring.
According to the principle of “intra-layer partitioning and intra-regional ringing,” the nodes of each layer and each district are connected into a ring. According to the top–down partitioning process, the number of
The following conditions need to be met:
STEP 3: Interconnection of backbone nodes.
According to the power supply level of the node area, the networking mode is selected to connect the nodes in each ring with the upper backbone node or other nodes in the same layer.
For any node
① If
② If
③ If
④ If
⑤ If
STEP 4: Interconnecting with local power generation equipment.
The energy supply side of the Energy Internet adopts the combination of local power supply and UHV supply and makes full use of existing local power generation equipment and energy resources. The local energy supply rate in the study area is
In order to ensure the reasonable layout of district energy equipment, the number of connection routes
Generating new edges meets the following criteria:
STEP 5: Interconnecting with local energy storage centers.
With the development of energy storage technology, it is necessary to plan energy storage nodes of different sizes to stabilize peak and valley loads and improve the level of system backup. If the proportion of regional energy storage capacity to total electricity consumption is
The layout of local energy storage centers should not only consider the spatial distribution balance but also consider the reliability requirements of key power supply nodes. The number of ways
For any node
There are
① If
② If
③ If
④ If
⑤ If
5 Topological characteristics of the Energy Internet backbone network taking the Beijing power grid as an example
The Beijing power grid is the power center of the north China power grid, and the conflict between environmental constraints and reliability requirements is particularly prominent. Adjusting the grid structure actively and constructing the Energy Internet are key paths to improve the reliability of power supply and reducing air pollution. Taking the Beijing power grid as an example, this paper analyzes the evolution mechanism of the backbone grid during the development of the power system to the Energy Internet.
According to the “Beijing Energy Development Plan for the 14th Five-Year Plan” and “The Medium and Long term Development Plan of Beijing Power Grid,” the Beijing power grid will build nine power supply big zones and implement gridded management of a distribution network. In this paper, the power grid architecture of Beijing is evolved in the direction of the Energy Internet by constructing a model, from the three levels of the Energy Internet top network, regional network, and local area network, and the topological characteristics of the generated network are studied. The number of nodes at each voltage level of the Beijing power grid and the number of Energy Internet nodes generated and evolved are shown in Table 2.
5.1 Evolution analysis of the Energy Internet backbone network in Beijing
Considering the sustainability of future power grid construction and the economics of Energy Internet construction, the evolution and construction process of the backbone structure of the Energy Internet in Beijing should fully rely on the existing power supply grid and further evolve on the basis of the existing 500-kV, 220-kV and 110-kV power supply grid structures.
According to the evolution model constructed in this paper, the 500-kV grid structure is evolved into an Energy Internet double-ring skeleton with a certain proportion of “source” and “storage” nodes as well as UHV access nodes. On the basis of the 220-kV grid structure, reasonable power nodes are added, “source” and “storage” nodes are designed according to a certain proportion, and an interconnected ring network is built to obtain a regional-level Energy Internet architecture. On the basis of the 110-kV grid structure, the “Medium and Long term Development Plan of Beijing Power Grid” is referred to add nodes, and the “source” and “storage” node requirements are considered for load balancing at this level, and a LAN-level Energy Internet network is built.
Figure 3 shows the topological structure of all levels of the power grid in Beijing and the Energy Internet evolved. Among them, the 500-kV power supply network refers to the “14th Five-Year Plan for Beijing Energy Development,” and the 220-kV and 110-kV power supply networks are up to 2020.
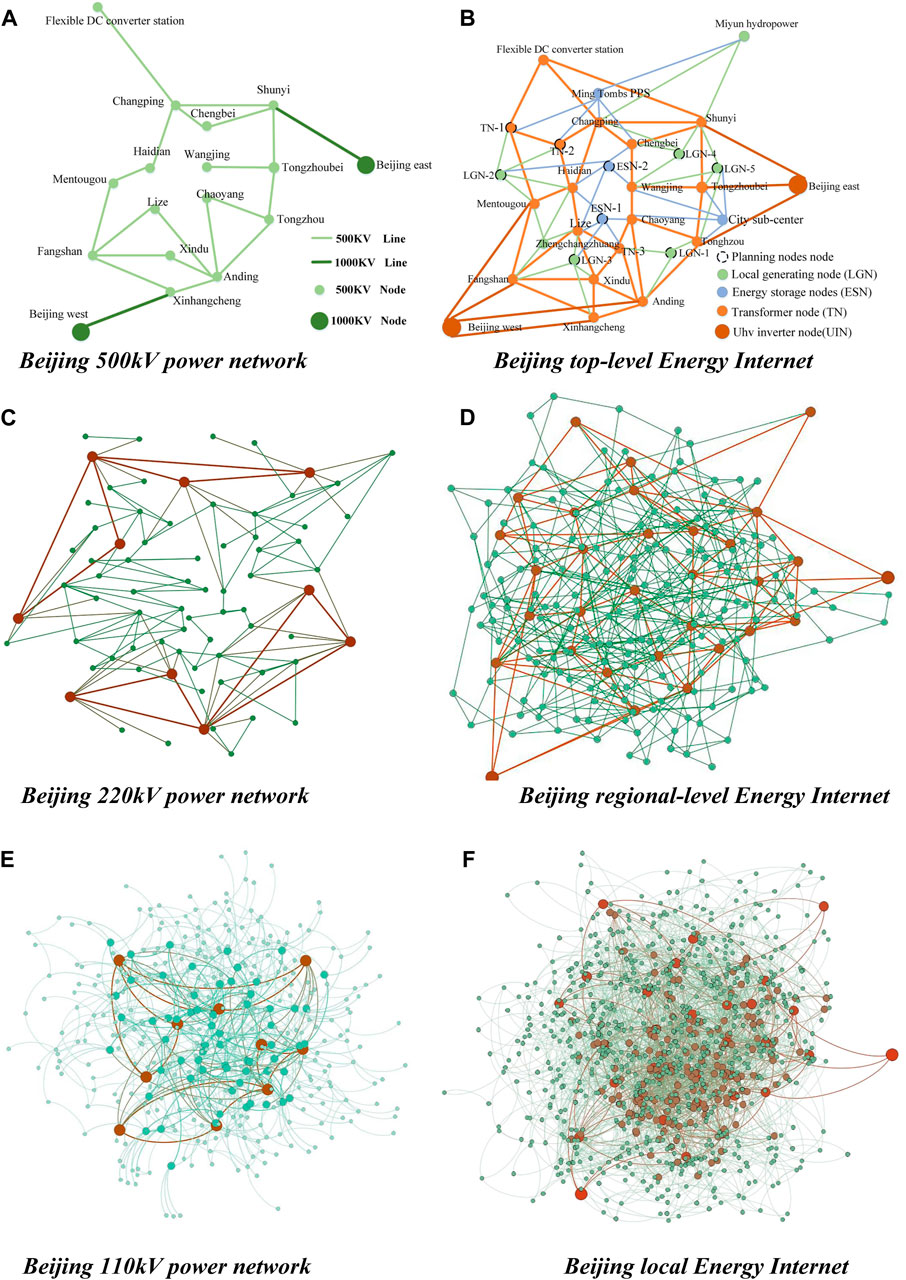
FIGURE 3. Topology of the power grid and Energy Internet at all levels in Beijing. (A): Beijing 500 kV power network. (B): Beijing top-level Energy Internet. (C): Beijing 220 kV power network. (D): Beijing regional-level Energy Internet. (E): Beijing 110 kV power network. (F): Beijing local Energy Internet.
From the above-mentioned evolution of the backbone network of the Beijing power grid to the Energy Internet, the following conclusions can be drawn.
① From the perspective of an energy-using structure, Beijing’s energy-using structure mainly comes from UHV long-distance transmission, local natural gas generator units, and surrounding hydropower. Due to the large number of important and high-grade power users in Beijing, the power grid disaster-bearing capacity and power supply reliability requirements are extremely high, and the deployment of a certain proportion of power generation nodes and energy storage nodes at all levels of the Energy Internet is necessary for peak regulation, emergency response, and improvement in reliability.
② From the perspective of the grid structure, in the future, the construction of the Energy Internet of “partition operation, hierarchical regulation, and source-grid-load-storage coordination” on the basis of the current “double-ring skeleton and nine major partitions” plan of the Beijing power grid is feasible. The current planning and construction direction of the power grid is in line with the concept and structural characteristics of the Energy Internet.
③ From the perspective of the hierarchical structure, the 500-kV-level double-ring skeleton is basically formed, and it is necessary to further rationalize the layout of energy storage and power generation nodes. The 220-kV and 110-kV network structures are still being continuously improved, and the scale of nodes and edges is still far from the proposed Energy Internet structure.
5.2 Characteristic analysis of the backbone network of the Energy Internet in Beijing
The abovementioned Energy Internet topology networks of various voltage levels in Beijing are analyzed in complex network characteristic. Compared with the relevant network characteristics of Ref. [55] and Ref. [62], the characteristics of the Energy Internet backbone network constructed by the HRNA topology generation mechanism and the literature studies are compared and shown in Table 3.
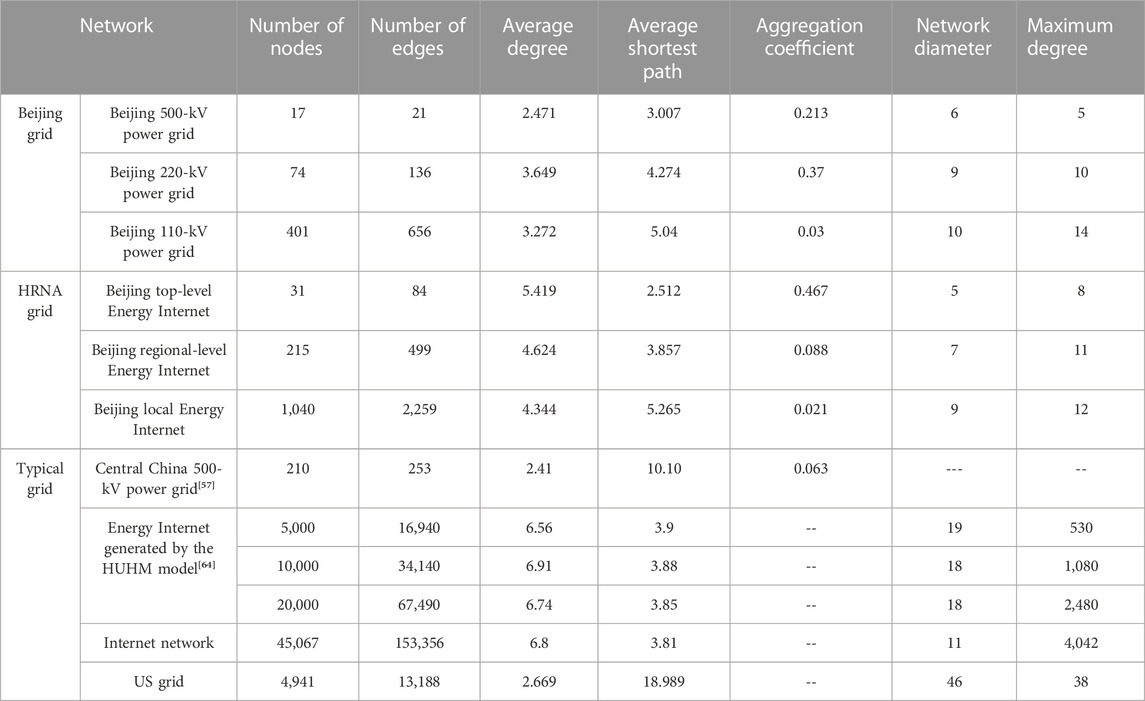
TABLE 3. Comparison of parameter characteristics of the Beijing Energy Internet backbone network with relevant literature.
The comparison leads to the following conclusions.
1) The macroscopic topology characteristics of the Energy Internet generated by the HUHM model in Ref. [62] are relatively close to the Internet network characteristics in terms of average degree, shortest path, and network diameter. However, this paper finds that the topological characteristics of the Beijing Energy Internet backbone network are different from those of both the traditional power grid and the Internet.
① The average degree of the Energy Internet backbone network generated by this mechanism (4.3, 4.6, 5.4) is much greater than that of the traditional power grid (2.7), but there is also a certain gap compared to the Internet (6.8).
② The average shortest path of the network obtained by the HRNA mechanism (2.5, 3.8, 5.2) in this paper is also between the traditional power grid (18.9) and the Internet (3.81) without considering the special needs of the top-level Energy Internet network (2.5), but it is especially close to the Internet.
③ The network diameter (5, 7, 9) constructed in this paper is much smaller than the traditional grid (46), and it is also smaller than the Internet diameter (11).
④ In terms of the maximum degree, the generated network in this paper (8, 11, 12) is closer to the traditional power grid (38), but it is quite different from the Internet (4042) and the results of the construction network (530, 1090, 2480) in Ref. [62].
Based on the above analysis, the characteristics of the Energy Internet network generated in this paper mainly have two points. First, compared with the traditional power grid, although the proportion of nodes and edges is not much different, the node degree is larger, the network diameter is smaller, and the average shortest path is smaller than those of the traditional tree structure with more leaf nodes to a more closely interacted network structure. Second, the gap between the Energy Internet and the Internet structure is large in terms of the maximum degree; it is shown that in the Energy Internet, there is no central node with star-shaped topological characteristics and more of a ring-like and network-like topology.
2) Longitudinal comparison is made between different levels of networks generated by the HRNA mechanism in this paper. The characteristics of the Energy Internet at different levels are different from those of the current power grid.
It can be seen from the table that ① the characteristics of the 500-kV grid of the Central China power grid and the 500-kV grid of the Beijing power grid in 2020 are similar. After evolving into the top-level Energy Internet with a “double-ring skeleton” structure, the average degree (5.4) and aggregation coefficient (0.47) are larger, the network aggregation is higher, nodes support each other better, and power supply reliability is higher. ② Compared with the characteristics of the 220-kV network in Beijing, the average degree increase in the regional Energy Internet is not particularly large (3.6→4.6), and the average shortest path (4.2→3.8) and the maximum degree (10→11) are also small. It shows that the 220-kV structural connectivity of the Beijing power grid is good, and the development rhythm is good and takes into account the reliability requirements. ③ The 110-kV power grid and the local Energy Internet are very similar in their characteristic structures (average degree: 3.272→4.344; average shortest path: 5.04→5.265; aggregation coefficient: 0.03→0.021; network diameter: 10→9; and maximum degree: 14→12), and the foundation for the construction of the Energy Internet is very good through improvement and adjustment. ④ It is not difficult to see from the comparison of the characteristics of the top-level Energy Internet to the regional and local level networks that with the reduction in the level and the increase in nodes, the average degree and aggregation coefficient of the network continue to decrease and the tightness of the network tends to be stable.
5.3 Analysis of network characteristics and differentiation reasons
The reasons for the differences in topological characteristics between the energy interconnection network generated in this paper and related literature studies (literature [62], US power grid and internet) are analyzed as follows:
① The average degree of the energy interconnection network generated in this paper is between the power grid and the Internet. This shows that compared with the traditional power grid, the connectivity of the Energy Internet is better, and the grid structure is stronger, but it does not reach the level of Internet connectivity.
② The diameter and average path of the energy interconnection network generated in this paper are close to the Internet. This shows that the Energy Internet is close to the Internet in the degree of aggregation and has the characteristics of short connection path and rapid diffusion;
③ The maximum degree of nodes in the network generated in this paper is different from that in the internet, and it does not exist as the central node connecting most nodes in the Internet. However, it is similar to the traditional power grid. On one hand, it can well reflect the characteristics of distribution, shared peer-to-peer and regional autonomy. On the other hand, it shows that the high connectivity level of the network depends on the regular and orderly interconnection, rather than the relay and aggregation of the central node.
6 Conclusion
In this paper, the evolution path and network characteristics of the traditional tree-shaped power grid under the trend of “hierarchical control and zoning coordination” architecture of the Energy Internet are studied. The network characteristics are analyzed by taking the 500-kV, 220-kV, and 110-kV grid structures of the Beijing power grid as an example, and the conclusions are as follows.
① Considering the continuity and economy of power grid construction, it is valuable and feasible to evolve the traditional tree-shaped power grid structure into a multi-layer network structure of the Energy Internet with hierarchical partitions.
② Using the Energy Internet HRNA evolution mechanism proposed in this paper, the structure of different levels of the grid structure in the Beijing power grid evolves in the direction of the Energy Internet, and it is found that the current direction of power grid planning and construction is in line with the direction of the Energy Internet structure.
③ The network generated by the mechanism proposed is close to the Internet in terms of average degree, network diameter, and aggregation coefficient, but there is no central node with a very high maximum degree in the Internet topology, which, to a certain extent, reflects the concept of Energy Internet equivalence.
Data availability statement
The original contributions presented in the study are included in the article/Supplementary material; further inquiries can be directed to the corresponding author.
Author contributions
XL: writing–original draft and writing–review and editing. ZZ: writing–original draft and writing–review and editing. YZ: writing–original draft and writing–review and editing. PL: writing–review and editing. LJ: writing–review and editing.
Funding
The author(s) declare financial support was received for the research, authorship, and/or publication of this article. This study is supported by the Natural Science Foundation of China “Research on a disaster chain model of ‘formation-transmission-warning-prevention’ for a power system based on the Energy Internet” (72001080), Henan Province Philosophy and Social Science Planning project (2020CJJ090), and Henan University Philosophy and Social Science Innovation Team Funding Project (2019-CXTD-12; 2024-CXTD-10).
Acknowledgments
The authors thank the participants who helped improve the paper by many pertinent comments and suggestions.
Conflict of interest
The authors declare that the research was conducted in the absence of any commercial or financial relationships that could be construed as a potential conflict of interest.
Publisher’s note
All claims expressed in this article are solely those of the authors and do not necessarily represent those of their affiliated organizations, or those of the publisher, the editors, and the reviewers. Any product that may be evaluated in this article, or claim that may be made by its manufacturer, is not guaranteed or endorsed by the publisher.
References
1. Zhang X, Shahidehpour M, Alabdulwahab A, Abusorrah A. Optimal expansion planning of energy hub with multiple energy infrastructures. IEEE Trans Smart Grid (2015) 6(5):2302–11. doi:10.1109/tsg.2015.2390640
2. Sheikhi A, Rayati M, Bahrami S, Mohammad Ranjbar A. Integrated demand side management game in smart energy hubs. IEEE Trans Smart Grid (2015) 6(5):675–83. doi:10.1109/tsg.2014.2377020
3. Paudyal S, Canizares C, Bhattacharya K. Optimal operation of industrial energy hubs in smart grids. IEEE Trans Smart Grid (2015) 6(2):684–94. doi:10.1109/tsg.2014.2373271
4. Sun Q, Fei T, Zhang H, Ma D. Construction of dynamic coordinated optimization control system for energy internet. Proc IEEE (2015) 35(14):3667–77. doi:10.13334/j.0258-8013.pcsee.2015.14.022
5. Zeng M, Yang Y, Liu D, Zeng B, Ouyang S, Lin H, et al. Generation-grid -Load-Storage coordinative optimal operation mode of energy internet and key technologies. Power Syst Tech (2016) 40(1):114–24. doi:10.13335/j.1000-3673.pst.2016.01.016
6. Zhang D, Shah N, Papageorgiou LG. Efficient energy consumption and operation management in a smart building with microgrid. Energ Convers Manag (2013) 74:209–22. doi:10.1016/j.enconman.2013.04.038
7. Wang X, Kong XY, Sun FY, Zhang CZ, Zhang . Research on multi-energy coordinated intelligent management technology of urban power grid under the environment of energy internet. Appl Sciences-Basel (2019) 9(13):2608. (in English). doi:10.3390/app9132608
8. Yang SX, Nie TQ, Li CC Research on the contribution of regional Energy Internet emission reduction considering time-of-use tariff. Energy (2022) 239:122170. (in English). doi:10.1016/j.energy.2021.122170
9. Aleksic S Energy-efficient communication networks for improved global energy productivity. Telecommunication Syst (2013) 54(2):183–99. (in English). doi:10.1007/s11235-013-9726-x
10. Cao Y, Li Q, Tan Y. A comprehensive review of Energy Internet: basic concept, operation and planning methods, and research prospects. J. Mod. Power Syst. Clean Energy (2018) 6, 399–411. doi:10.1007/s40565-017-0350-8
11. Shams MH, Shahabi M, Kia M, Heidari A, Lotfi M, Shafie-Khah M, et al. Optimal operation of electrical and thermal resources in microgrids with energy hubs considering uncertainties. Energy (2019) 187:115949. doi:10.1016/j.energy.2019.115949
12. Mansour-Saatloo A, Agabalaye-Rahvar M, Mirzaei MA, Mohammadi-Ivatloo B, Abapour M, Zare K Robust scheduling of hydrogen based smart micro energy hub with integrated demand response. J Clean Prod (2020) 267:122041. doi:10.1016/j.jclepro.2020.122041
13. Huang AQ, Crow ML, Heydt GT, Zheng JP, Dale SJ The future renewable electric energy delivery and management (freedm) system: the energy internet. Proc Ieee (2011) 99(1):133–48. doi:10.1109/Jproc.2010.2081330
14. Liang ZG, Guo R, Li J, Huang AQ A high-efficiency pv module-integrated dc/dc converter for pv energy harvest in freedm systems. Ieee Trans Power Elect (2011) 26(3):897–909. doi:10.1109/Tpel.2011.2107581
15. Kotb MF, El-Saadawi M, El-Desouky EH Overcurrent protection relay-based arduino uno for freedm system. Int Trans Electr Energ Syst (2019) 29–6. doi:10.1002/2050-7038.12017
16. Takahashi R, Kitamori Y, Hikihara T Ac power local network with multiple power routers. Energies (2013) 6(12):6293–303. doi:10.3390/en6126293
17. Hua HC, Qin YC, Xu HX, Hao CT, Cao JW Robust control method for dc microgrids and energy routers to improve voltage stability in energy internet. Energies (2019) 12:1622–9. doi:10.3390/en12091622
18. Lin S, Liu C, Li D Bi-Level multiple scenarios collaborative optimization configuration of CCHP regional multi-microgrid system considering power interaction among microgrids. Proc CSEE (2019) 27:1–14. doi:10.13334/j.0258-8013.pcsee.190275
19. Yang MI, Hongye LIU, Genxin S Two-layer power optimization allocation of multi-energy local networks oriented to energy internet. Electric Power Automation Equipment (2018) 38:1–10.
20. Wan C, Jia Y, Li B Research status and prospect of energy trading mode and user demand response in urban energy internet. Automation Electric Power Syst (2019) 43:29–40. doi:10.16081/j.issn.1006-6047.2018.07.001
21. Chen ZH, Wu JJ, Xia YX, Zhang X Robustness of interdependent power grids and communication networks: a complex network perspective. Ieee Trans Circuits Syst Ii-Express Briefs (2018) 65(1):115–9. (in English). doi:10.1109/tcsii.2017.2705758
22. Xie BH, Tian XG, Kong LL, Chen WM The vulnerability of the power grid structure: a system analysis based on complex network theory. Sensors (2021) 21(21):7097. (in English). doi:10.3390/s21217097
23. Shahpari A, Khansari M, Moeini A Vulnerability analysis of power grid with the network science approach based on actual grid characteristics: a case study in Iran. Physica a-Statistical Mech Its Appl (2019) 513:14–21. doi:10.1016/j.physa.2018.08.059
24. Lin ZZ, Wen FS, Wang HF, Lin GQ, Mo TW, Ye XJ CRITIC-based node importance evaluation in skeleton-network reconfiguration of power grids. Ieee Trans Circuits Syst Ii-Express Briefs (2018) 65(2):206–10. (in English). doi:10.1109/tcsii.2017.2703989
25. Mei L, Tang DS, Wang TY, Du WB, Xia YX, Cao XB Predicting the evolution process of infrastructure networks with an NSIPA link prediction method. Ieee Trans Circuits Syst Ii-Express Briefs (2019) 66(11):1895–9. (in English). doi:10.1109/tcsii.2019.2903558
26. Espejo R, Lumbreras S, Ramos A A complex-network approach to the generation of synthetic power transmission networks. Ieee Syst J (2019) 13(3):3050–8. doi:10.1109/jsyst.2018.2865104
27. Soltan S, Loh A, Zussman G A learning-based method for generating synthetic power grids. Ieee Syst J (2019) 13(1):625–34. doi:10.1109/jsyst.2018.2825785
28. Xie BH, Li CF, Wu ZL, Chen WM Topological modeling research on the functional vulnerability of power grid under extreme weather. Energies (2021) 14(16):5183. (in English). doi:10.3390/en14165183
29. Panigrahi P, Maity S Structural vulnerability analysis in small-world power grid networks based on weighted topological model. Int Trans Electr Energ Syst (2020) 30(7). doi:10.1002/2050-7038.12401
30. Chen C, Zhou X, Li Z, He ZH, Li ZT, Lin XN Novel complex network model and its application in identifying critical components of power grid. Physica a-Statistical Mech Its Appl (2018) 512:316–29. (in English). doi:10.1016/j.physa.2018.08.095
33. Albert R, Jeong H, Barabasi AL Error and attack tolerance of complex networks. Nature (2000) 406, 378–82. doi:10.1038/35019019
34. Rosato V, Bologna S, Tiriticco F. Topological properties of high-voltage electrical transmission networks. Electric Power Syst Res (2005) 77:99–105. doi:10.1016/j.epsr.2005.05.013
35. Shengwei M, Yingying W, Laijun C. Overviews and prospects of the cyber security of smart grid from the view of conplex network theory (2023).
36. Meng Z, Lu Z, Song J Comparison analysis of the small-world topological model of Chinese and American power grids (2004).
37. Chao-yang C, Yong Z, Ming C, Bing-hong W. Review of large power grid vulnerability based on complex network theory. Control and Decision (2022) 37 (04), 782–798. doi:10.13195/j.kzyjc.2021.0126
38. Cao Y, Chen X, Sun K Identincation of vulnerable lines in Power grid based on complex network theory. In: Power Engineering Society General Meeting. IEEE (2007).
39. Albert R, Albert I, Nakarado GL. Structural vulnerability of the North American power grid. Phys Rev (2004) 69:025103. doi:10.1103/PhysRevE.69.025103
40. Amani AM, Jalili M Power grids as complex networks: resilience and reliability analysis. Ieee Access (2021) 9:119010–31. doi:10.1109/access.2021.3107492
41. Liu B, Li Z, Chen X, Huang YH, Liu XD Recognition and vulnerability analysis of key nodes in power grid based on complex network centrality. Ieee Trans Circuits Syst Ii-Express Briefs (2018) 65(3):346–50. (in English). doi:10.1109/tcsii.2017.2705482
42. Zhao CZ, Zhao JT, Wu CC, Wang XL, Xue F, Lu SF Power grid partitioning based on functional community structure. Ieee Access (2019) 7:152624–34. (in English). doi:10.1109/access.2019.2948606
43. Guerrero M, Banos R, Gil C, Montoya FG, Alcayde A Evolutionary algorithms for community detection in continental-scale high-voltage transmission grids. Symmetry-Basel (2019) 11(12):1472. doi:10.3390/sym11121472
44. Jiang J, Xia YX, Xu S, Shen HL, Wu JJ An asymmetric interdependent networks model for cyber-physical systems. Chaos (2020) 30(5):053135. doi:10.1063/1.5139254
45. Guo WZ, Wang H, Wu ZP Robustness analysis of complex networks with power decentralization strategy via flow-sensitive centrality against cascading failures. Physica a-Statistical Mech Its Appl (2018) 494:186–99. doi:10.1016/j.physa.2017.12.002
46. Alonso M, Turanzas J, Amaris H, Ledo AT Cyber-physical vulnerability assessment in smart grids based on multilayer complex networks. Sensors (2021) 21(17):5826. doi:10.3390/s21175826
47. Fang JK, Su C, Chen Z, Sun HS, Lund P Power system structural vulnerability assessment based on an improved maximum flow approach. Ieee Trans Smart Grid (2018) 9(2):777–85. doi:10.1109/tsg.2016.2565619
48. Zang TL, Lei J, Wei X, Huang T, Wang T, Perez-Jimenez MJ, et al. Adjacent graph based vulnerability assessment for electrical networks considering fault adjacent relationships among branches. Ieee Access (2019) 7:88927–36. doi:10.1109/access.2019.2926148
49. Galindo-Gonzalez CC, Angulo-Garcia D, Osorio G Decreased resilience in power grids under dynamically induced vulnerabilities. New J Phys (2020) 22(10):103033. doi:10.1088/1367-2630/abb962
50. Fan WL, Zhang XM, Mei SW, Huang SW, Wei W, Ding LJ Vulnerable transmission line identification using ISH theory in power grids. Iet Generation Transm Distribution (2018) 12(4):1014–20. doi:10.1049/iet-gtd.2017.0571
51. Fan WL, Zhang XM, Mei SW, Huang SW Vulnerable transmission line identification considering depth of K-shell decomposition in complex grids. Iet Generation Transm Distribution (2018) 12(5):1137–44. doi:10.1049/iet-gtd.2017.0767
52. Liu FZ, Xiao B, Li H Finding key node sets in complex networks based on improved discrete fireworks algorithm. J Syst Sci Complexity (2021) 34(3):1014–27. doi:10.1007/s11424-020-9023-1
53. Aziz T, Lin ZZ, Waseem M, Liu SY Review on optimization methodologies in transmission network reconfiguration of power systems for grid resilience. Int Trans Electr Energ Syst (2021) 31(3). doi:10.1002/2050-7038.12704
54. Buldyrev SV, Parshani R, Paul G, Stanley HE, Havlin S. Catastrophic cascade of failures in interdependent networks, 464, 1025–8. doi:10.1038/nature089322010)
55. Ji X, Wang B, Liu D, Zhao T. Review on interdependent networks theory and its applications in the structural vulnerability analysis of electrical cyber-physical system (2023).
56. Xingpei J, Bo W, Zhaoyang D, Guo C, Dichen L, Daqian W, et al. Vulnerability evaluation and link addition protection strategy research of electrical cyber-physical interdependent networks. Power Syst Tech. (2016) 1872–8. doi:10.13335/j.1000-3673.pst.2016.06.038
57. Huang Z, Wang C, Ruj S, Stojmenovic M, Nayak A. Modeling cascading failures in smart power grid using interdependent complex networks and percolation theory. In: 2013 IEEE 8th Conference on Industrial Electronics and Applications (ICIEA); 19-21 June 2013; Melbourne, VIC, Australia. IEEE (2013). doi:10.1109/ICIEA.2013.6566517
58. HuangWangStojmenovic ZCM, Nayak A. Balancing system survivability and cost of smart grid via modeling cascading failures. IEEE Trans Emerging Top Comput (2013) 1:45–56. doi:10.1109/TETC.2013.2273079
59. Zhou D, Gao J, Stanley HE, Havlin S. Percolation of partially interdependent scale-free networks. Phys Rev E Stat Nonlin Soft Matter Phys (2013) 87(5):052812. doi:10.1103/PhysRevE.87.052812
60. Shao J, Buldyrev SV, Havlin S, Stanley HE (2011). Cascade of failures in coupled network systems with multiple support-dependence relations. Phys Rev E Stat Nonlin Soft Matter Phys. 83 (3), 036116. doi:10.1103/PhysRevE.83.036116
61. Parandehgheibi M, Modiano E 2013. Robustness of interdependent networks:The case of communication networks and the power grid. ArXiv.doi:10.1109/GLOCOM.2013.6831395
62. Cai W, Zhao H, Wang J, Lin C. A unifying network topological model of the energy internet macro-scope structure (2023).
63. Li C, Li X, Tian S, Yuan J. Challenges and prospects of risk transmission in electric power and information depth fusion for energy internet. Dianli Xitong Zidonghua/Automation Electric Power Syst (2021) 41:17–25. doi:10.7500/AEPS20160927014
64. Lang Y, Jing LI, Luo Y Large power grid topology analysis based on graph partitioning. Dianli Xitong Baohu Yu Kongzhi/Power Syst Prot Control (2017) 45(23):108–15. doi:10.7667/PSPC171060
65. Han Y, Guo C, Zhu B. Modeling cascading failures in cyber physical power system based on improved percolation theory (2023).
66. Liu FY, Gu B, Qin SW, Zhang KY, Cui L, Xie G Power grid partition with improved biogeography-based optimization algorithm. Sustainable Energ Tech Assessments (2021) 46:101267. doi:10.1016/j.seta.2021.101267
Keywords: energy internet, topological structure, evolutionary mechanism, network characteristics, backbone network
Citation: Li X, Zhang Z, Zhou Y, Li P and Ji L (2023) Research on the generation mechanism and characteristics of an Energy Internet backbone network. Front. Phys. 11:1287889. doi: 10.3389/fphy.2023.1287889
Received: 03 September 2023; Accepted: 13 November 2023;
Published: 08 December 2023.
Edited by:
Yongxiang Xia, Hangzhou Dianzi University, ChinaReviewed by:
Haicheng Tu, Hangzhou Dianzi University, ChinaXi Zhang, Beijing Institute of Technology, China
Copyright © 2023 Li, Zhang, Zhou, Li and Ji. This is an open-access article distributed under the terms of the Creative Commons Attribution License (CC BY). The use, distribution or reproduction in other forums is permitted, provided the original author(s) and the copyright owner(s) are credited and that the original publication in this journal is cited, in accordance with accepted academic practice. No use, distribution or reproduction is permitted which does not comply with these terms.
*Correspondence: Yucheng Zhou, zhouyuchengwork@163.com