- 1Department of Environmental Sciences, Emory University, Atlanta, GA, United States
- 2Agriculture and Natural Resources, Cooperative Extension at North Carolina Agricultural and Technical State University, Greensboro, NC, United States
- 3Department of Electrical and Computer Engineering, University of Texas at Dallas, Richardson, TX, United States
- 4College of Agriculture, Montana State University, Bozeman, MT, United States
A comprehensive understanding of the long-term data on the crop, soils, environment, climate, and production management would facilitate efficient data-driven decision-making in agriculture production under changing climate. We have employed an explainable machine learning algorithm (random forest model coupled with LIME; Local Interpretable Model-Agnostic Explanations framework) using multi-decadal (1981–2015) data on climate variables, soil properties, and yield of major crops across the Coterminous United States (CONUS). This data-driven approach explained the multi-faceted factors of crop production for corn, soybean, cotton, and wheat under field conditions by leveraging agricultural informatics. We attempted to show how crop yields can better be correlated and explained when production input varies along with changing climatic/environmental and edaphic conditions. Our findings suggest Growing Degree Days (GDDs) as important climatic factors, while water holding capacity is one of the dominant soil properties in interpreting crop yield variability. Our findings will facilitate growers, crop production scientists, land management specialists, stakeholders, and policy makers in their future decision-making processes related to sustainable and long-term soil, water, and crop management practices.
Climate Change—Food Production—Global Food Security Nexus
Climate change is one of the biggest challenges to the world in present times including its threat to global food (and nutritional) security and hunger issues. Globally ~750 million people were undernourished as a direct and indirect effect of climate change in 2019, which is being majorly contributed by a decline in food production, hike in food prices, and increase in social conflicts for land and water availability (Misselhorn et al., 2012; Hobert and Negra, 2020). Thus, it seeks immediate and sustainable adaptation and/or mitigation strategies although a number of them have been already developed to offset the deleterious impact of climate change on food production. The socio-economic development is greatly hindered from local to a global scale with a complex interaction with sustainable food production systems (Burchfield et al., 2022). Increasing temperatures, drought, floods, and increasing carbon dioxide release as a result of climate change in several parts of the world have impacted the production of staple grains and other economically viable crops (Fornara and Tilman, 2009; Aiking, 2011; Herrmann and Bucksch, 2014; Brown et al., 2015; Gitz et al., 2016; Pugh et al., 2016; Mbow et al., 2019). Yet at the same time, global food production would have to be doubled by 2050 to feed the burgeoning population (~9 billion by 2050). Moreover, the crop yield is estimated to decrease by 25% by 2050 due to ongoing climate change (FAO, 2018).
It is critical to understand the impact of climate change on crop yield through the lens of global food security. The future of climate change and its associated impacts is multi-directional and highly unpredictable, which makes planning for mitigation and adaptation a bit complex. Irrespective of other uncertainties in climate change, its potential impacts will likely decrease agricultural productivity in the coming years (Jones and Hassan, 1991; Lobell and Gourdji, 2012). Many climate-smart and climate-resilient agricultural practices and technologies have been evolving as a part of an interdisciplinary approach to manage crop production along with classical agronomic research under the current and future climate change scenario. For example, climate-smart water management (e.g., variable rate irrigation), precision nutrient management (e.g., management zone delineation, use of drones, remote sensing, hyperspectral imaging, GIS mapping, etc.), digital and modern agriculture (e.g., intensive data collection from the individual field), weather-smart agriculture, carbon-smart crop management (e.g., no-tillage, cover crop, sensors-based technology), socio-economic knowledge-based practices, and agricultural extension capacity building are in high demand (Gregory et al., 2005; Nelson et al., 2009; Elliott et al., 2014; Troy et al., 2015; McLennon et al., 2021).
Variability in Crop Yield Due to Climate and Soil Variables
Climate and management practices adopted might have the greatest influence on the agricultural production of a region. Historically, crop genetic development and agronomic management practices are the main drivers for advancement in agricultural production (Messina et al., 2009; Grassini et al., 2013; Bailey-Serres et al., 2019; Van Tassel et al., 2020; Karavolias et al., 2021). Variations in crop yield can be explained by climatic and edaphic (~soil) factors (Leng et al., 2016). The intertwined relationship among these factors and how they impact the management practices can explain the majority of the variation in crop yield across broad temporal- and spatial scales. On the other hand, the impact of climate change and climate variability on the crop yield throughout the USA revolves around the location-soil-crop triangle (Motha and Baier, 2005; Cohn et al., 2016; Kukal and Irmak, 2018a). More specifically, multiple factors could impact crop yields such as genetics (variety selection), climate, soil, management practices, inputs (fertilizer, manure, irrigation, row spacing, planting date and depth, crop density), etc. In addition, there has been technological advancement in crop production which magnificently improved the crop yield and farm production (Raza et al., 2019). However, lately, under the climate change situation, many climate variables could be responsible for substantial modification in crop yield despite advancements in agricultural technologies (Pugh et al., 2016).
There has been an increasing trend in developing data-driven statistical tools such as Machine Learning (ML) algorithms to predict and explain crop yield variability using climate and weather indices (Lobell and Field, 2007; Schlenker and Roberts, 2009; Lobell and Burke, 2010; Lobell and Gourdji, 2012; Urban et al., 2012; Osborne and Wheeler, 2013; Moore and Lobell, 2014; Anderson, 2019). This quantitative data-driven approach warrants a comprehensive understanding of climate and environmental variables with crop yield from long-term data repositories at the county level in the USA. It has been reported that the annual Growing Degree Days (GDD) has been increasing by 50°C every century (Kukal and Irmak, 2018a), thus a strong correlation between crop yield and climate variables is expected. Moreover, the impact is region-specific and crop-specific. For example, the yield of major row and/or crop rotation common in the USA (e.g., corn, soybean, cotton, and wheat) can significantly be impacted by agro-climatic changes under the recent trend of climate change due to its effect on GDD (Kukal and Irmak, 2018b). However, the explanation of temperature (~GDD) effect on crop yield can be contingent on other climate factors (e.g., precipitation) and/or soil properties that as a single factor or in combination can explain the yield variability and might follow a non-linear pattern in their relationship. For example, higher mean yield and its inter-annual variability are often associated with high soil available water holding capacity, while lower inter-annual variability in yield is generally associated with high Soil Organic Matter (SOM) due to its high buffering capacity (Xue et al., 2020).
Climate Variability Across CONUS
The projected increase in surface temperature with an increase in intensity and duration of heat is making the world more food insecure. The possible changes in temperature and precipitation across the USA have the potential to alter the positive impact of carbon dioxide (CO2) emissions. The sixth Intergovernmental Panel on Climate Change (IPCC) reports changing conditions for food production in the mid-to-high latitudes of the CONUS (IPCC, 2021). The continuous warming and spatiotemporal shift in climatic conditions across the CONUS project to the shift in optimum climatic conditions for the production of rain fed crops (e.g., corn, soybean, wheat, etc.) from Iowa and Illinois to Minnesota and Dakotas with modulations in soil productivity (Hoffman et al., 2020).
Broadly, GDD and precipitation are reported to be the two important climatic parameters for yield variability in corn and soybean (Kucharik and Serbin, 2008; Leng et al., 2016; Xu et al., 2016). In general, the increase in the GDD develops more soil moisture stress and decreases the time to crop maturity by impacting the metabolic processes across the corn belt in CONUS (Challinor et al., 2010) eventually leading to a reduction in carbon assimilation (Lobell et al., 2013). Similarly, an increase in GDD decreases the soybean yield up to 16% in southern regions with an increase in temperature up to 2°C while showing no significant changes in yield in northern regions (Jones and Hassan, 1991; Serdeczny et al., 2017). Additionally, a major economic loss (~2.2 billion dollars) has been encountered due to drought in the cotton fields of Texas in 2011 (Acosta-Martinez et al., 2014). Therefore, inter-annual variability of climate factors (e.g., GDD and precipitation) likely influences inter-annual variability in crop yield more than soil properties (Figures 1–3).
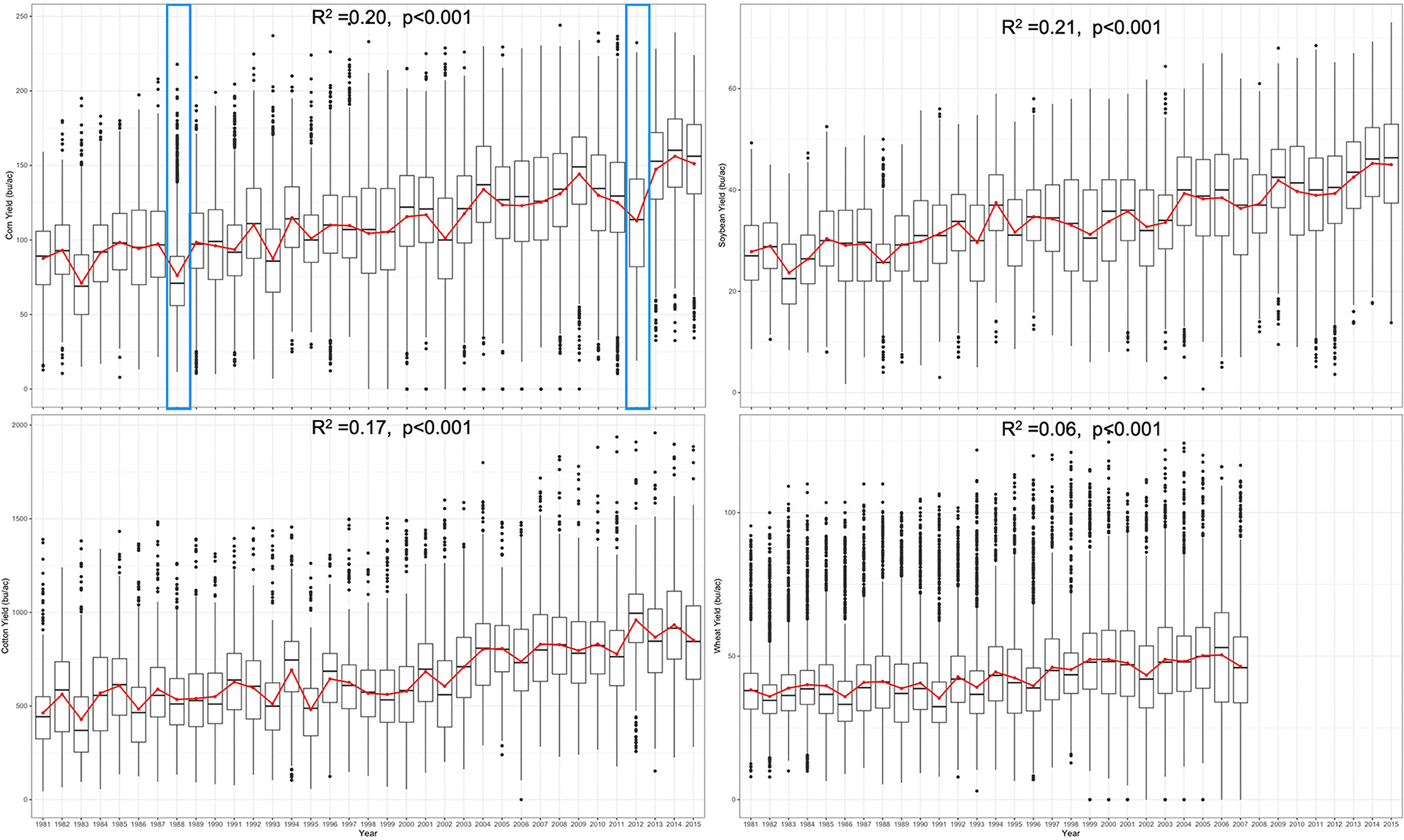
Figure 1. Temporal trends of (a) crop (corn, soybean, cotton, wheat) yields in the ACDC (Agro Climatic Data by County) dataset.
Crop Yield Assessment Using Data-Driven Approach in Climate and Soil Variability
There is a dire need for the development of modeling and simulation strategies for agricultural decision making which often lack an understanding of a comprehensive long-term data-driven approach across CONUS. To that end, it is critical to bridge gaps in global food security, agricultural food production, and climate change nexus (McLennon et al., 2021). Our overall objective was to utilize the digital access of long-term data and interpreting using machine learning (ML) algorithms to understand the impact of climate factors and soil properties on the yield of four major crops across the CONUS for 35 years (1981–2015). The scope of this study considers GDD and precipitation as climatic factors; and soil water holding capacity (WHC), organic matter (OM), texture, pH, slope, erodibility, and soil loss tolerance factor as soil properties. A collective and thorough understanding of the impacts of changing climate and soil properties on crop yield would facilitate building our knowledge and navigating efficient data-driven decision making in agriculture. We interpreted crop yield variation using long-term data-based simulation, and modeling obtained from climate and soil variables is needed.
Our synthesis approach aims to evaluate the impact of climate change drivers and soil properties on the production of major food crops across the CONUS by leveraging agricultural informatics. We have applied an explainable ML algorithm on long-term crop yield data collected by the National Agricultural Statistics Service, United States Department of Agriculture (NAAS-USDA). This data-driven approach explains the multiple climatic and soil factors of crop production under field conditions and how crop yields can better be correlated and explained when production input varies along with changing environmental conditions in the past 35 years. These findings will facilitate growers, crop production scientists, land management specialists, and policymakers in their future decision-making processes related to soil, water, and crop management practices. We have adopted data synthesized from literature (Yun and Gramig, 2019) and quantified to estimate the impacts of climate change and various soil factors on yields of four major crops (corn, soybean, cotton, and wheat) grown in different regions (total 3,070 counties) across CONUS for 35-years (1981–2015).
Data Sources, Statistical Analyses, and Machine (and Explainable) Learning Approaches
Data Source
We adopted a published county-level Agro-Climate Data, which was spatially and temporally consistent for agricultural yields, climate, and soil variables across CONUS (Yun and Gramig, 2019). This publicly-available dataset contains annual crop yield for major crops in the USA (Corn, Soybean, Cotton, and Winter Wheat) synthesized by USDA-NASS (1981–2015). This Agro-Climatic Data by County (ACDC) also includes the major climatic and edaphic variables from publicly available spatial data sources for diverse end-users. We represented temperature effects from PRISM climate data as customizable growing degree days (GDDs). GDD is also known as heat units, and it measures the heat accumulation as crop growth is highly dependent on the ambient temperature in a cumulative stepwise manner except for any extreme events (e.g., drought or disease infestation). It is calculated as a function of the daily maximum temperature (Tmax), daily minimum temperature (Tmin), base temperature (Tbase) as follows (Anandhi, 2016): GDD = (Tmax + Tmin)/2–Tbase.
Daily GDD values are added together from the beginning of the growing season, providing an indication of the energy available for crop growth (Anandhi, 2016). GDD values are accumulated by adding each day's GDD contribution as the season progresses. Cumulative GDD values are used for comparing the progress of a growing season to the long-term average and are useful for estimating crop development stages. Unless stressed by other environmental factors (e.g., soil moisture), development rates from emergence to maturity for many crops depend on air temperature. Because many crop developmental events depend on the accumulation of specific quantities of heat, it is possible to predict when these events should occur during a growing season regardless of differences in air temperature from year to year. GDD units can be used to assess the suitability of a region for the production of a particular crop, estimate the growth stages of crops, heat stress on crops, and predict maturity and yield.
There was a total of 120 GDD intervals in the ACDC dataset (GDD interval of 1°C, ranging between −60 and +60°C). We chose GDD values between −39 and +51°C, which covers the temperature ranges of the crop growing season for the entire period. We further reduced the dimensionality of the GDD data so that it can explain the yields of major crops used in this study more suitably while reducing the chances of over-fitting our Explainable ML model. Therefore, we used cumulative GDDs for 3°C intervals (a total of 24 categories of GDD).
Spatial variations in soil properties across CONUS were adapted from the USDA-NRCS gSSURGO dataset. Only non-forestry agricultural classes were selected following USGS NLCD land cover (~land use) categories. See Yun and Gramig (2019) for more information on numerical, computational, and geo-computation methods used for data generation and processing from original data sources, selection of agricultural masks, and spatial aggregation or disaggregation approaches in the ACDC dataset.
Data Visualizations, Temporal Trend, and Correlation Matrices
All visualizations and temporal trends on yield of major crops, climate factors, and soil properties were evaluated using the ggplot2 (Wickham, 2008) package in R (version 4.1.0) (R Core Team, 2021). Correlation matrices were generated using R-ggstatsplot (Patil, 2018) package. Correlograms were created using adjusted Holm correlation coefficients. All statistical analyses were conducted at the 5% level of significance.
Machine Learning Models
We analyzed four different ML regression models (Random Forest, K-Nearest Neighbor, Decision Tree, and Support Vector Regression) to evaluate non-linear relationships between climate factors, soil properties, and yields of major crops. For evaluating the best regression model, we examined the coefficient of determination (R2) and root mean square error (RMSE) values of each model.
R2, or the coefficient of determination, was determined using the following formula:
where the sum squared regression is the summation of the residuals squared, while the total sum of squares is the addition of data distances from the mean squared.
RMSE, or the Root Mean Square Error, was determined using the following formula:
where Pi is the predicted value, Oi is the observed value, and n is the total number of observations.
The R2 metric is a statistical measure of how close the data is to the fitted regression line. An R2-value closer to one indicates a better fit. The RMSE values explain the measure of the error in the model in predicting crop yield as impacted by climate and soil variables. Higher the RMSE value, farther off the model's predicted value from the expected value. Consequently, a model with a high R2-value and low RMSE value indicates a better fit. After selecting the best regression model, we randomly divided our data between training (80%) and testing (20%) to evaluate the effect of climate and soil variables on the long-term yield of four selected crops. We ranked the features based on the range of variability in crop yields explained by selected climate factors and soil properties.
Explainable Machine Learning Algorithm
To provide explainability within the agricultural yield domain, we used a technique that utilizes yield data on major agricultural crops and features (climate factors and soil properties) in conjunction with the LIME (Local Interpretable Model-Agnostic Explanations) framework for furnishing human-readable results (Figure 4; Ribeiro et al., 2016). The LIME framework provides explainability to any machine learning model. Specifically, it identifies the features most important to the output. Then, it perturbs a sample to generate new ones with corresponding predictions and weights them by proximity to the initial instance. Furthermore, an interpretable model was trained using these newly created samples, and the prediction is explained by interpreting the local model. LIME can be beneficial when the desired human explainability elucidates the impact of the features on the output. In our study, the machine learning model predicted crop yield, in a feature list consisting of climatic and soil factors, and LIME determined the features that are positively or negatively correlated to furnished crop yield predictions. In this scenario, the user can obtain a prediction and a human-readable explanation for the model's decision.
To utilize the LIME framework for elucidating agricultural yield inferences, we utilize a three-step process for obtaining furnished explanations (Figure 4). First, for a given dataset, we employ univariate feature selection for determining the features to be employed in our machine learning models for all classes to be predicted. Univariate selection utilizes statistical tests that assist in computing the features having the best correlation with the output. We used all features here. More specifically, the SelectKBest technique, which uses the chi-squared statistical test, was employed (Pedregosa, 2011). Second, since our data consists of data collected periodically, we utilized machine learning regression models for determining future trends. For each class to be predicted, we trained our regression models on the best features selected in the aforementioned univariate step and evaluated them on novel data to be regressed. Lastly, for each regression model trained, we utilized our LIME framework to explain each outcome of the regressed data.
Splitting the LIME Framework for Different Soil Features and Precipitation Ranges
We further split the ACDC data based on the numerical fluctuations of the desired soil properties and precipitation ranges. This was achieved by utilizing the minimum and maximum values for selected variables to create three intervals of equal distance. The crop yield dataset was split into one of these three groups based on the interval values. Subsequently, we trained Random Forest regression models on these different intervals to evaluate if the effect of temperature (represented by GDD) is contingent on the ranges of soil properties and precipitation values.
Variability in Climate, Soil, and Crop Yield Data Across Conus
While the yield of all four crops, precipitation, and GDD (+5 to +30) generally followed normal distributions, the GDD (+49 to +31) and GDD (+4 to −39) were more skewed toward the left on the x-axis (Supplementary Figures 1, 2). Among different soil properties, WHC, silt content, clay content, erodibility, and soil loss tolerance factors were normally distributed (Supplementary Figure 3). We observed a bimodal distribution of soil pH across 3,070 counties throughout the CONUS. A strongly skewed relationship in soil slope and organic matter (most of the values are concentrated at the lower end of the x-axis) and soil loss tolerance factor (most of the values were concentrated at the higher end of the x-axis) was also noticed. Several studies have reported an average OM in top CONUS soil ranges between 3.0 and 6.0% (Fenton et al., 2008), which closely follows our observation in the ACDC data.
There is a general trend in the increase in all four crop yields over time (Figure 1). We observed a significant increase in crop yields with a 1.72, 1.62, and 1.84-fold increase in yield of corn (R2 = 0.20, p < 0.001), soybean (R2 = 0.21, p < 0.001), and cotton (R2 = 0.17, p < 0.001), respectively, from 1981 to 2015. We also observed inter-annual variability in climate factors, but temporal trends (i.e., regressions between climate data and year in Figure 2) of GDDs (R2 ranged between 1.1e-05 and 0.004, p < 0.001 for all GDD intervals) and precipitation (R2 = 0.01, p < 0.001) were not noticeable. For example, the wheat yield significantly (R2 = 0.06, p < 0.001) increased (~1.21 times) from 1981 to 2007, which can possibly be explained by a combined effect of both climate variables as well as several developmental changes in crop growth and physiology due to modification in management practices. Further, drastic reductions in corn and soybean (and marginally wheat) yields were observed in 1988 and 2012 possibly due to the worst drought in the Midwest with many locations receiving rainfall 40–50% lower than normal (Riebsame et al., 1994). Similarly, a drastic yield reduction of corn was noticed in 2012 (see blue rectangles in Figures 1, 2) as compared to the inter-annual yield variability due to the “Great Drought” of 2012 across the CONUS (Nielsen, 2021). The severe to moderate drought of 2012 impacted 33% of the CONUS during the peak period of the growing season in June (NOAA, 2012). We did not observe any temporal trend in soil properties, except WHC and Soil Loss Tolerance Factor (R2 = 0.002 and p < 0.001 for both).
The inter-annual variability of crop yield was most likely influenced by climatic factors, however, there may have been some critical contribution by management factors that resulted in this yield increase that have varied over these 35 years (Figures 1–3). We noticed that yield modification can possibly be attributed to the adoption of genetically modified and drought tolerant cultivars (Ortiz-Bobea and Tack, 2018), the introduction of precision farm management using modern farm machinery to maximize crop production while minimizing ecological footprint (McLennon et al., 2021), involvement of soil conservation practices e.g., zero or minimum tillage, cover crops, increased planting density and optimizing row spaces have also helped in increasing crop yields (Basche et al., 2016; Assefa et al., 2018; Licht et al., 2019) across the CONUS. Moreover, most of the selected soil properties and climate factors also co-varied and can contribute indirectly to variations in crop yields over space and time (Supplementary Figures 4–7). Consequently, crop yields were significantly correlated with all selected soil properties with the exception of soil OM and WHC for cotton yield (Supplementary Figures 4–7; Johnson and Trout, 2012; Xu J. et al., 2021; Xu T. et al., 2021).
Model Selection and Performance
The best-fitted regression model was chosen based on the coefficient of determination (i.e., R2) and average mean error (i.e., RMSE) values to examine how close the observed data is to fitted regression lines. From our results, the Random Forest model consistently had the highest R2-value, with an average of 0.96 for all four crops (R2-values follow the trend of Random Forest > Decision Tree > K-Nearest Neighbor > Support Vector Regression, where lowest R2-values of 0.56 were obtained for the SVR model, data not shown). Additionally, RMSE values indicated that the Random Forest model consistently had the least amount of error (RMSE) for all four crops, followed by Decision Tree and K-Nearest Neighbor models, and the Support Vector Regression model's predicted value was farthest from the expected values (data not shown). The RMSE value should be minimized as much as possible. Therefore, we elected to employ the Random Forest model in our future experiments on crop yield data.
Model R2-values for corn (Training = 0.96, Testing = 0.73), soybean (Training = 0.97, Testing = 0.75), cotton (Training = 0.96, Testing = 0.71), and wheat (Training = 0.96, Testing = 0.74) further confirmed that the Random Forest model adequately represented the yield of all four crops (Table 1). Among four crops, RMSE values were lowest for soybean (Training = 1.49, Testing = 3.98), followed by wheat (Training = 2.08, Testing = 5.51), corn (Training = 5.50, Testing = 14.70), and highest for cotton (Training = 42.97, Testing = 112.83) (Table 1).
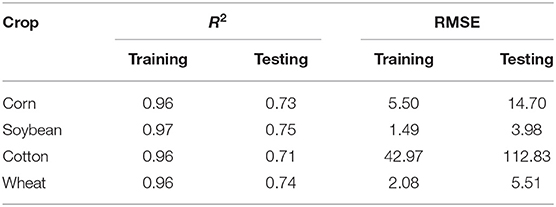
Table 1. Selection of random forest models for training and testing data on yields of corn, soybean, cotton, and wheat from 1981 to 2015 for CONUS.
When analyzing our results for the Random Forest models, we can see that the classifiers had a high R2 score of at least 0.96, thereby corroborating their regression abilities. Furthermore, when examining the RMSE, values for our models, we observe that the scores for testing were diminutive and similar to the training values. A lower RMSE value indicates the competency of the model to furnish output values that are near the actual amount. Since our average error was minimal, the results and derived correlations procured through LIME are valid.
To solve the issue of overfitting and further bolster our models, we can filter the classifier's utilized features to incorporate only the top features. Consequently, we remove previously employed features with low correlation on the output that would reduce the overall robustness of our Random Forest regression models. Additionally, we have tuned the parameters of the Random Forest to incorporate less depth per tree. A higher depth engenders more captured information, thereby resulting in an overfitted model.
Climate and Soil Properties Impact Crop Yield
The crop yield depends on the heterogeneity of climate factors (temperature, here represented as GDD and precipitation; PPT) and soil properties (e.g., WHC, soil organic matter, texture, pH, erodibility, and soil loss tolerance factor, etc.). The exploratory data analysis using the random forest model in our study revealed GDD as the most influential climatic factor for all four crops (corn, soybean, cotton, and wheat) impacting the yield. In contrast, the yield of the crops did not depend or be influenced by any single soil property but was variably affected based on the type of the crop and under the ranges of selected soil properties across CONUS. Figure 5 represents the effect of climatic and soil factors on crop yields defined as feature ranks for the random forest regression model and overall weight of features for the explainable machine learning algorithm (i.e., LIME correlations). The size of the bar for plots representing feature ranks represents the weight of the ranked features, whereas the size and direction of the bar plots representing overall weight in LIME correlations explain the strength of the negative or positive effect of the feature on crop yield. Among the climatic factors, GDDs, in general, indicated the most significant impacts on the yield of the crops in the order of soybean > corn > wheat > cotton. In general, the yield of corn and soybean was positively impacted by the GDD interval of +42 to +45°C. Seasonal precipitation also impacted the yield for soybean, and marginally for corn but not for cotton and wheat (Figures 5A–D). Other studies conducted in semi-arid soils have shown similar results where the yield of wheat was significantly correlated with higher temperature while showing a non-significant relation with precipitation (Sommer et al., 2013). On the other hand, heavy rainfall significantly impacted the boll formation of cotton in vertisols but was non-significantly related to the decreased yield of cotton (Njouenwet et al., 2021).
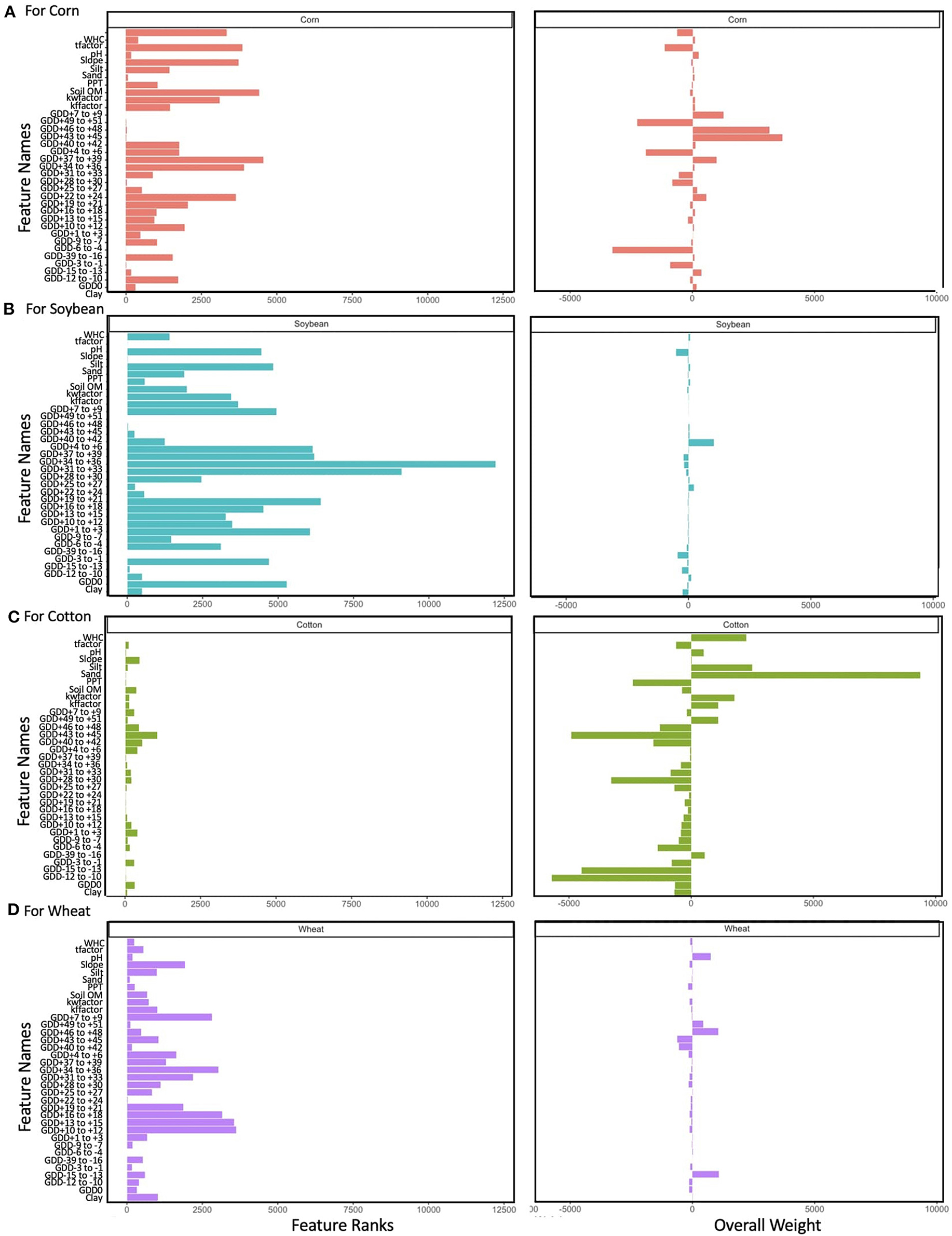
Figure 5. Feature ranks and overall weight of features following explainable machine learning interpretations for (A) corn, (B) soybean, (C) cotton, and (D) wheat.
Among all the soil properties observed, the WHC affected most of the corn yield followed by soybean, wheat, and cotton. Soil WHC is an important driver for buffering corn yields against climate variability (Williams et al., 2016). Similarly, higher water content (above 75% of field capacity) was reported to decrease the yield of mulched drip-irrigated cotton (Hu et al., 2009). Optimally, corn and soybean growers maintain 50% of the available soil WHC until the corn cob reaches the black layering stage of the crop and most pods in soybean are yellow (Kelley, 2020). Corn and soybean grain yields are optimum at pH range 5.8–6.2 and decrease beyond this range (University of Wisconsin Extension, 2012). Further, rainfall during the pod-filling stage of soybeans can be beneficial for optimized yield as the crop uses more water than the early stages (Pedersen and Lauer, 2004). Among four major crops, soil pH and silt (%) mostly influenced the yield of soybean, followed by corn, wheat, and cotton. In general, the range of optimal soil pH reported was 5.8 to 6.2 for the northern corn-soybean near-neutral to <5.2 for Pacific Northwest wheat (Mahler and McDole, 1987; Ghimire et al., 2017) and >6.3 for soybean (lower critical limit at pH 5.2) (Pothula et al., 2019). Based on the feature ranking of these major four crops, we observed that most of the factors sufficiently explained the yield variability in soybean, followed by corn and wheat, but not for cotton (note the relatively smaller size of bars for feature ranks associated with cotton yield in Figure 5C as compared to other three crops in Figures 5A,B,D). It implies that other factors (e.g., radiation, relative humidity, evaporation, etc.), either as a single variable or co-variable(s), might have impacted cotton yield. For example (Sawan, 2017) demonstrated that flowering and boll formation in cotton can be greatly affected by solar radiation and evapotranspiration.
Splitting Features to Evaluate the Influence of Climatic Factors
Figures 6–9 represent grouped classification of each featured rank of climatic properties (here precipitation) divided into three ranges/categories (low, medium, and high quartiles), respectively, and their impact on crop yields. For example, precipitation (ranged between 0.91 and 1,753 mm) is divided into three quartiles based on its intensity from lowest to highest, where 0.91–468, 469–769, and 770–1,753 mm represent 1st, 2nd, and 3rd quartiles, respectively (Figures 6–9). The highest featured rank for the middle quartile (i.e., 469–613 mm precipitation) showed a positive effect of warming, and corn yield was expressed in the middle quartile range (Figure 6). Thus, a warmer (GDD +40 to +42) and wetter climate favored corn yield but its impact faded in the range of GDD of (+43) to (+45) and (+49) to (+51). The lower and upper base temperature for optimum corn growth and productivity is 50°F (i.e., 10°C) and 86°F (30°C), respectively (Akyuz and Ransom, 2015). A higher yield (7.32 Mg ha−1) of corn occurred at intermediate elevations with mild temperatures and sufficient precipitation (Thomson et al., 2002). Our findings support that overall corn yield response to warming (13% for 1°C) in the mid-latitude CONUS region is much greater than discussed in the IPCC 6th assessment (IPCC, 2021), where corn yields are projected to decrease by 5 to 20% with up to 3–4°C of warming without any climate change adaptation. There were positive impacts of climate factors on crop yield due to higher GDD accumulated during growing seasons for both corn and soybean (Figures 6, 7).
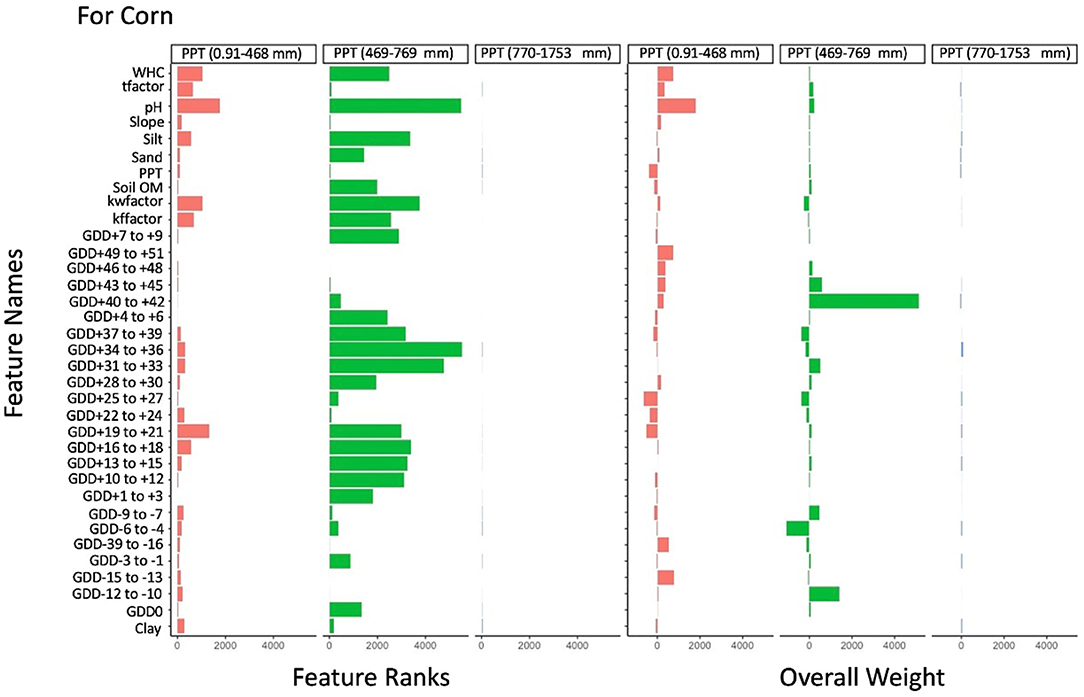
Figure 6. Feature ranks and overall weight of features following explainable machine learning interpretations for corn for low, medium, and high quartiles of precipitation.
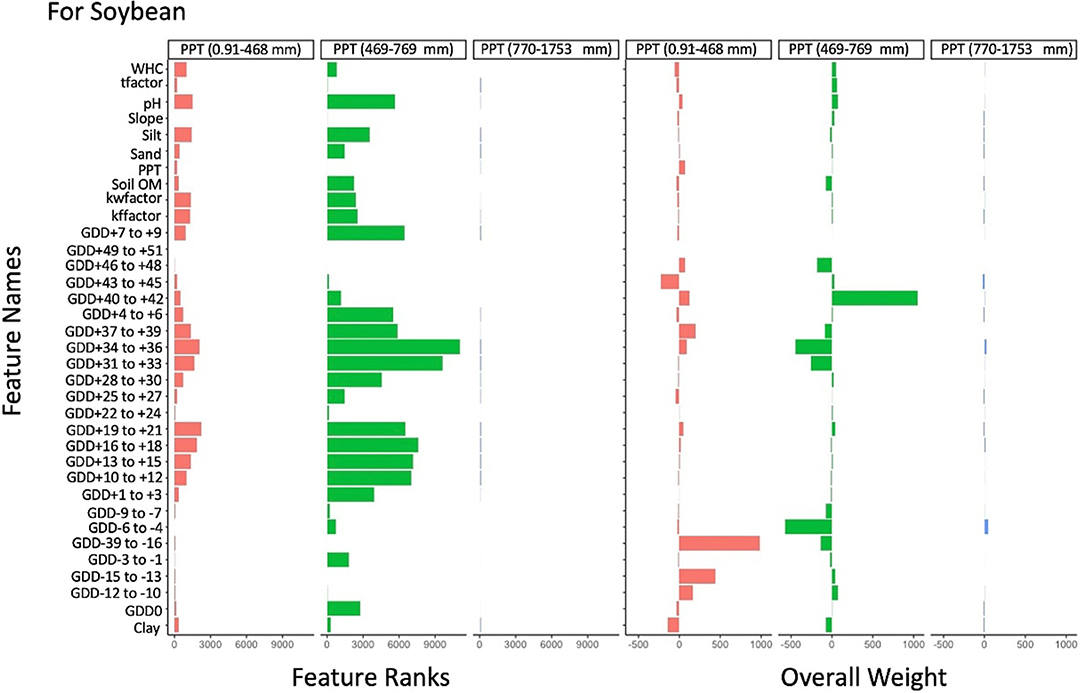
Figure 7. Feature ranks and overall weight of features following explainable machine learning interpretations for soybean for low, medium, and high quartiles of precipitation.
Featured ranking in cotton and wheat showed the highest influence of climatic and soil factors in the middle quartile (i.e., 469–613 mm precipitation, see Figures 8, 9). Positive effects of warming and cotton yield are expressed in the middle quartile range where warmer (GDD +40 to+42) and wetter climates negatively influenced cotton yield. In contrast, cotton yield can possibly be better explained by positive GDD (high values) than for corn and soybean. In years that are extremely hot during the flowering and boll-filling, the crop matures earlier and has smaller bolls than in years when the temperature is cooler (Reddy et al., 1999). Generally, cotton is adapted to high temperature climates of the USA like Texas, Georgia, and Arkansas. However, the elevated temperature declines the boll formation and eventually the harvest index beyond 28°C, reaching zero boll harvest index at 33–34°C (Reddy and Zhao, 2005). A similar observation was reported by Williams et al. (2016) that excessive heat and drought conditions are known to decrease the grain yield of corn. The increase in the wheat yield was observed during drought conditions (i.e., low precipitation quartile) (Figure 9) because it thrives well in low rainfall intensive regions.
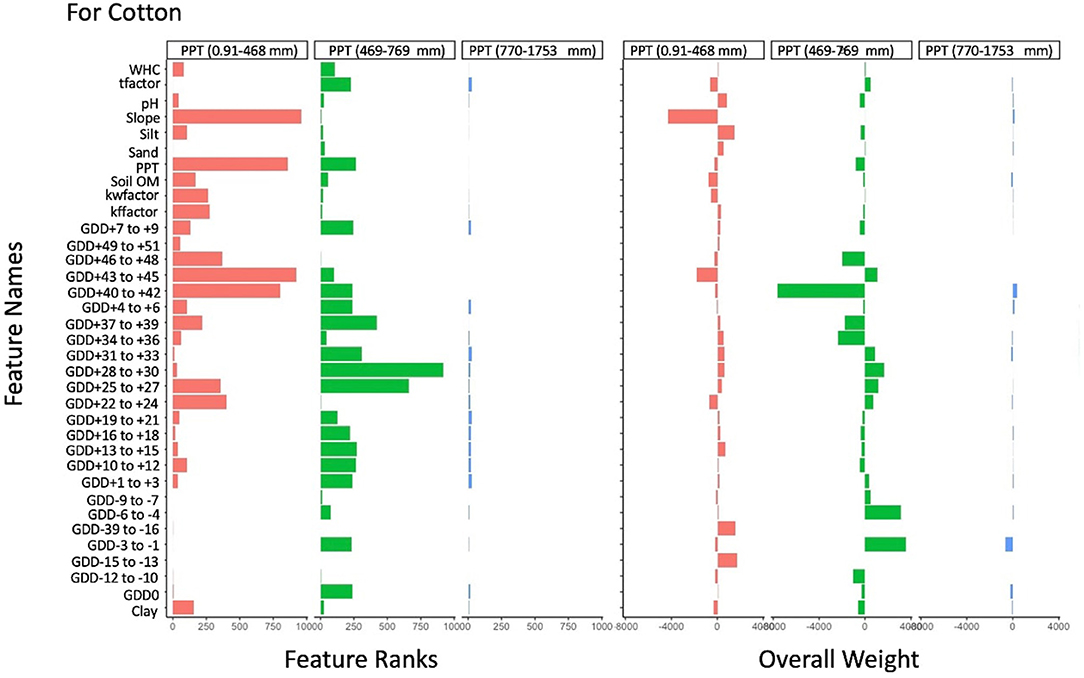
Figure 8. Feature ranks and overall weight of features following explainable machine learning interpretations for cotton for low, medium, and high quartiles of precipitation.
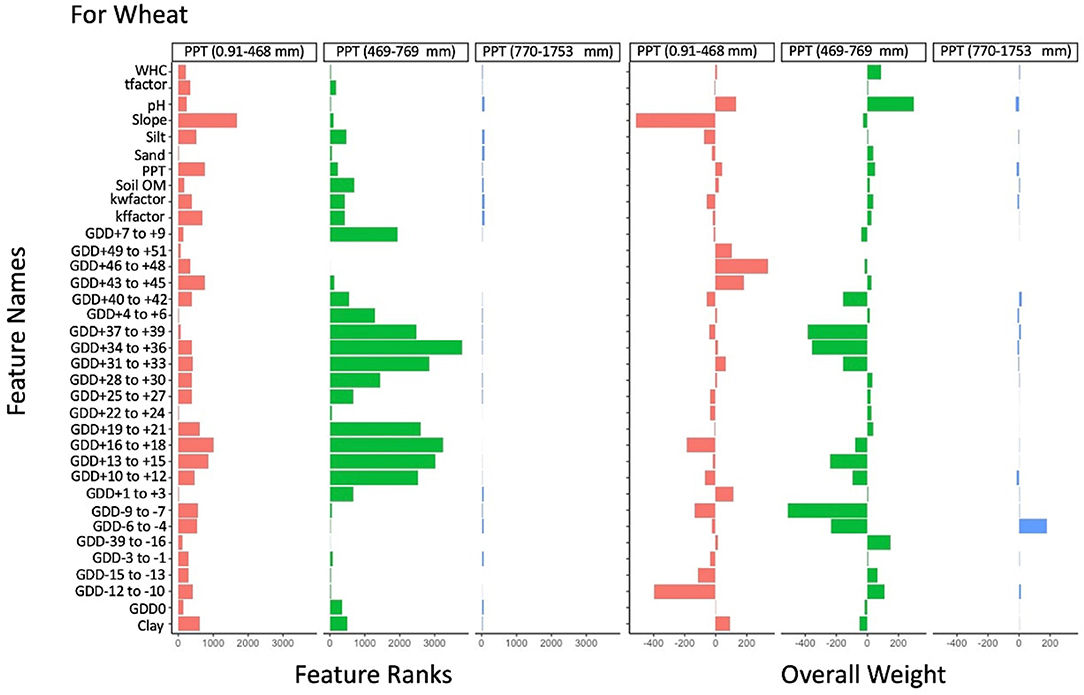
Figure 9. Feature ranks and overall weight of features following explainable machine learning interpretations for wheat for low, medium, and high quartiles of precipitation.
Splitting Features to Evaluate the Influence of Soil Factors
Figures 10–13 and Supplementary Figures 8–15 represent the influence of soil properties divided into three ranges/categories (low, medium, and high quartiles) on crop yields. Soil WHC (ranged between 3.23 and 43.76 cm/cm) is divided into three quartiles: 1st (low: 3.2–19.8 cm/cm); 2nd (medium: 19.8–27.5 cm/cm); and 3rd (high: 27.6–43.8 cm/cm) quartiles, respectively (Figures 10–13). A strong positive influence of GDD (+19 to +21 and +31 to +36°C) on corn and soybean yield was expressed by their feature ranks for the middle quartile of WHC in CONUS (Figure 10). Other studies also reported that optimum precipitation and soil WHC can increase the stability in corn productivity (Williams et al., 2016). For cotton and wheat, GDD (+25 to +27) and GDD (+28 to +30) features ranked high in the medium quartile of soil WHC (Figures 12, 13). GDD (+43 to +45) generally showed a negative impact for all four crops for the low and medium quartiles of soil WHC. However, GDD (+43 to +45) showed either a slightly positive or no impact for the high WHC quartile, which indicates a potential buffering effect of WHC against heat stress (represented by very high GDD) on crop yields (Figures 10–13).
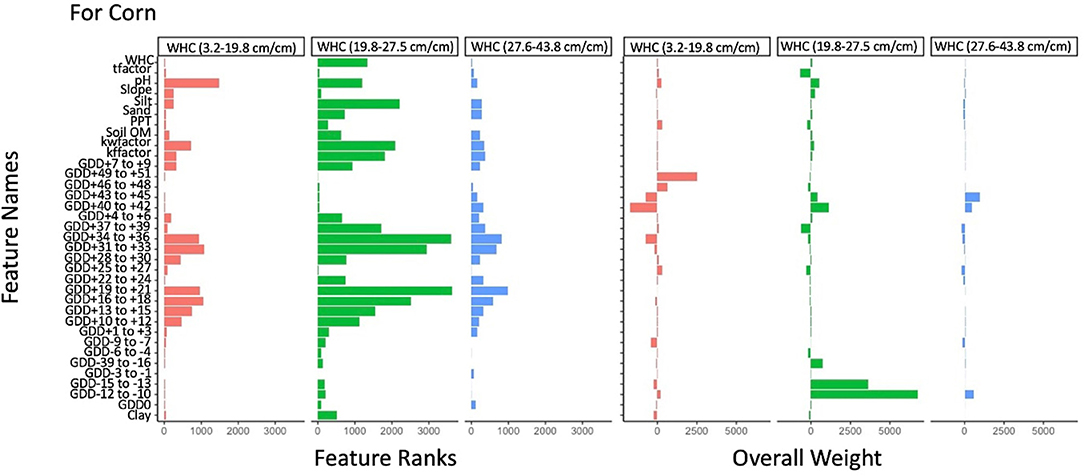
Figure 10. Feature ranks and overall weight of features following explainable machine learning interpretations for corn for low, medium, and high quartiles of water holding capacity.
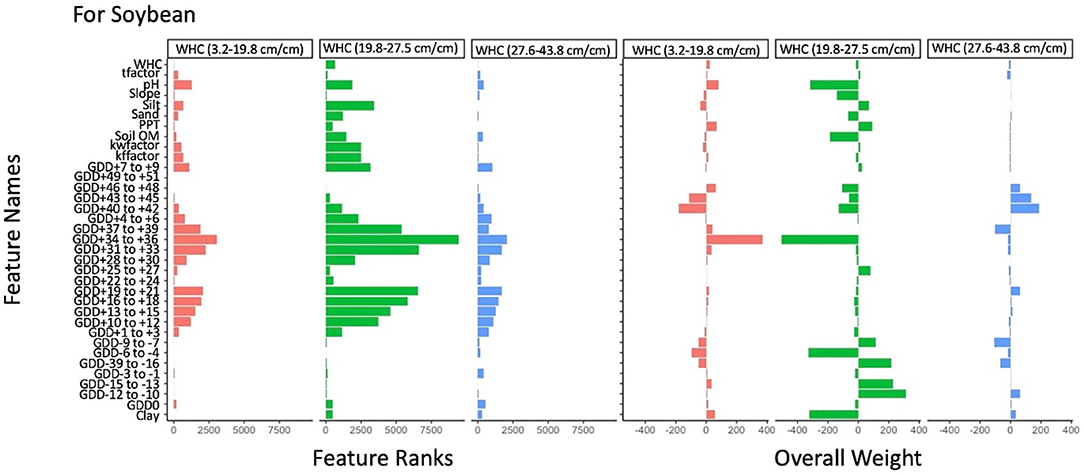
Figure 11. Feature ranks and overall weight of features following explainable machine learning interpretations for soybean for low, medium, and high quartiles of water holding capacity.
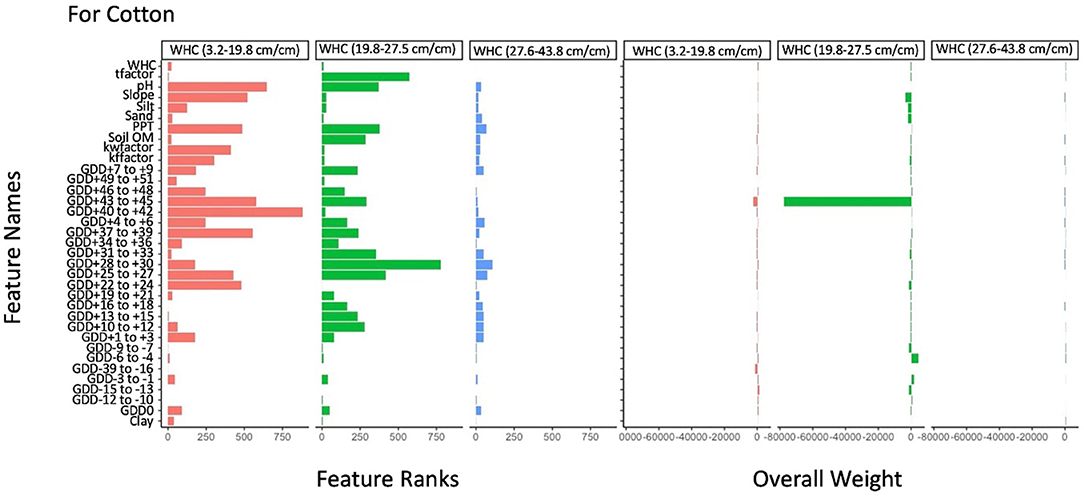
Figure 12. Feature ranks and overall weight of features following explainable machine learning interpretations for cotton for low, medium, and high quartiles of water holding capacity.
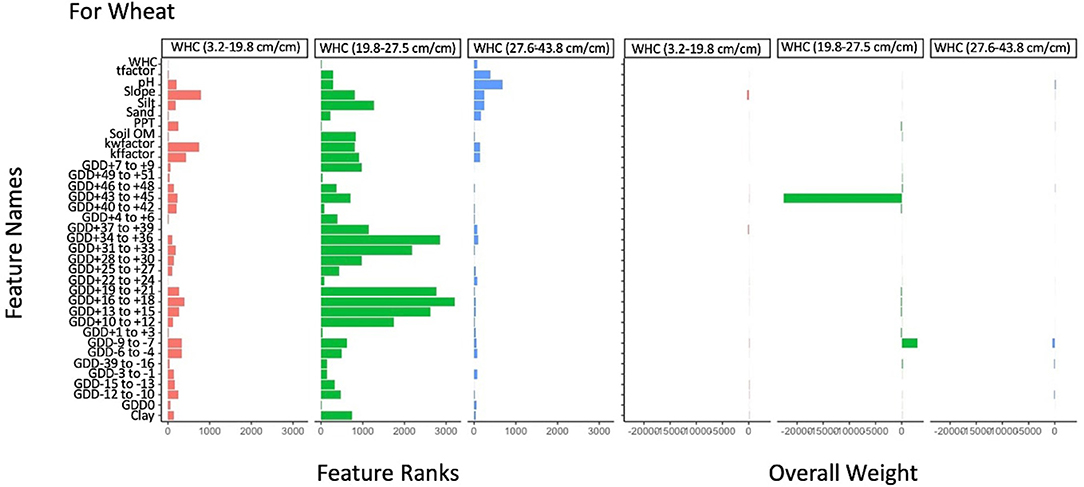
Figure 13. Feature ranks and overall weight of features following explainable machine learning interpretations for wheat for low, medium, and high quartiles of water holding capacity.
Supplementary Figures 8–11 represents the influence of soil OM divided into three categories (low: 0.22–0.93%; medium: 0.94–2.13%; and high: 2.14–90.54%) on crop yield. According to our findings, a strong influence on the yield of corn, soybean, and wheat was expressed by their feature ranks for low to medium quartile ranges of soil OM in CONUS. Low soil organic matter decreases the buffering capacity of soil pH (Jiang et al., 2018). Additionally, the negative correlation of soil pH with crop yield for the low soil OM quartile is possibly due to a combined negative effect of low soil OM and the associated increase in soil pH and likely dispersion of soil particles that makes the conditions unfavorable for crop growth.
Supplementary Figures 12–15 represents the influence of clay content on crop yield which is divided into three ranked categories (low: 0.95–20.5%; medium: 20.6–32.2%; and high: 32.3–65.5%). We observed a strong influence of climatic and soil factors on the yield of corn, soybean, and wheat under the medium clay content category (Supplementary Figures 12, 13, 15). Colder (GDD −39 to −16) climate compromised corn yield and warmer (GDD +40 to +42) climate favored soybean and wheat yield in medium clay soil. On the other hand, the cotton yield was impacted by both low and medium clay content. Colder (GDD −15 to −13) climate compromised the cotton yield under low clay soil, followed by medium clay soil, and showed no effect in high clay soil (Supplementary Figure 14). Higher clay content in soil may have restricted plant growth possibly due to limited nutrient availability, and a cooler temperature might have further reduced the plant physiological metabolism.
Conclusion
Our quantitative synthesis is aimed to provide information on the impact of climate variables and soil properties on the yield of four major crops grown in different regions (total 3,070 counties) across the Conterminous United States (CONUS). We leveraged big data and machine learning algorithms to evaluate the effects of climate factors (temperature; growing degree days; and precipitation) and soil properties on the long-term (1981–2015) yield of corn, soybean, cotton, and wheat. Based on our observation, the most important climatic factor explaining crop yield variability of these major crops is GDD while WHC among many soil properties plays a crucial role. Our empirical approach using the ACDC dataset adequately explained yield variability in corn, soybean, and wheat using climatic and soil factors. However, most of the yield variability in cotton was not sufficiently explained by climatic and soil factors under the current study which warrants further investigation with other climatic variables (e.g., radiation, relative humidity) as well as yet to know soil factors. A proper understanding of these factors and their inclusion in the prediction model would possibly help to improve the performance of predictive models.
Our data-driven synthesis can serve as a guide to growers, crop production scientists, land management specialists, stakeholders, and policy makers for the timely management of sustainable crop production for four major crops in CONUS. It will help to prioritize structural resilience and resource management for food security under climate change scenarios. Although our current analysis is limited to CONUS, we expect that the Explainable ML approach used here can be implied in other agro-climatic regions or eco-regions (e.g., tropical countries) as long as the data related to climate and soil properties are available. Expanding our analysis outside CONUS in the future will also be constrained by the availability of sufficient data for training and testing purpose.
Data Availability Statement
Publicly available datasets were analyzed in this study. This data can be found at: https://www.mdpi.com/2306-5729/4/2/66.
Author Contributions
DS: conceptualization, analysis, interpretation, writing, visualization, and supervising. BD and GJ: interpretation and writing. AK: analysis, writing, and visualization. KB: analysis, visualization, and supervising. All authors contributed to the article and approved the submitted version.
Funding
DS acknowledges funding support from the Emory College of Arts and Sciences, Halle Institute of Global Research, and the University Research Council at Emory University. BD is supported by Agriculture and Natural Resources, Cooperative Extension at North Carolina Agricultural and Technical State University. GJ is supported by the College of Agriculture at Montana State University.
Conflict of Interest
The authors declare that the research was conducted in the absence of any commercial or financial relationships that could be construed as a potential conflict of interest.
Publisher's Note
All claims expressed in this article are solely those of the authors and do not necessarily represent those of their affiliated organizations, or those of the publisher, the editors and the reviewers. Any product that may be evaluated in this article, or claim that may be made by its manufacturer, is not guaranteed or endorsed by the publisher.
Supplementary Material
The Supplementary Material for this article can be found online at: https://www.frontiersin.org/articles/10.3389/fsufs.2022.847892/full#supplementary-material
Supplementary Figure 1. Histograms of crop (corn, soybean, cotton, wheat) yields in the ACDC (Agro Climatic Data by County) dataset.
Supplementary Figure 2. Histograms of climate factors in the ACDC (Agro Climatic Data by County) dataset.
Supplementary Figure 3. Histograms of soil properties in the ACDC (Agro Climatic Data by County) dataset.
Supplementary Figure 4. Correlation matrices between soil properties and climate factors and yields of corn in the ACDC (Agro Climatic Data by County) dataset.
Supplementary Figure 5. Correlation matrices between soil properties and climate factors and yields of soybean factors in the ACDC (Agro Climatic Data by County) dataset.
Supplementary Figure 6. Correlation matrices between soil properties and climate factors and yields of cotton factors in the ACDC (Agro Climatic Data by County) dataset.
Supplementary Figure 7. Correlation matrices between soil properties and climate factors and yields of wheat factors in the ACDC (Agro Climatic Data by County) dataset.
Supplementary Figure 8. Feature ranks and overall weight of features following explainable machine learning interpretations for corn for low, medium, and high quartiles of soil organic matter.
Supplementary Figure 9. Feature ranks and overall weight of features following explainable machine learning interpretations for soybean for low, medium, and high quartiles of soil organic matter.
Supplementary Figure 10. Feature ranks and overall weight of features following explainable machine learning interpretations for cotton for low, medium, and high quartiles of soil organic matter.
Supplementary Figure 11. Feature ranks and overall weight of features following explainable machine learning interpretations for wheat for low, medium, and high quartiles of soil organic matter.
Supplementary Figure 12. Feature ranks and overall weight of features following explainable machine learning interpretations for corn for low, medium, and high quartiles of clay.
Supplementary Figure 13. Feature ranks and overall weight of features following explainable machine learning interpretations for soybean for low, medium, and high quartiles of clay.
Supplementary Figure 14. Feature ranks and overall weight of features following explainable machine learning interpretations for cotton for low, medium, and high quartiles of clay.
Supplementary Figure 15. Feature ranks and overall weight of features following explainable machine learning interpretations for wheat for low, medium, and high quartiles of clay.
References
Acosta-Martinez, V., Moore-Kucera, J., Cotton, J., Gardner, T., and Wester, D. (2014). Soil enzyme activities during the 2011 Texas record drought/heat wave and implications to biogeochemical cycling and organic matter dynamics. Appl. Soil Ecol. 75, 43–51. doi: 10.1016/j.apsoil.2013.10.008
Aiking, H. (2011). Future protein supply. Trends Food Sci. Technol. 22, 112–120. doi: 10.1016/j.tifs.2010.04.005
Akyuz, F. A., and Ransom, J. K. (2015). Growing degree day calculation method comparison between two methods in the northern edge of the US Corn Belt. J. Service Climatol. 2015, 1–9. Available online at: https://stateclimate.org/pdfs/journal-articles/2015_Adnan_et_al.pdf
Anandhi, A. (2016). Growing degree days–ecosystem indicator for changing diurnal temperatures and their impact on corn growth stages in Kansas. Ecol. Indicat. 61, 149–158. doi: 10.1016/j.ecolind.2015.08.023
Anderson, R. G. (2019). Irrigation in California: Overview and Relation to Energy. Riverside, CA: Federal Utility Partnership Working Group.
Assefa, Y., Carter, P., Hinds, M., Bhalla, G., Schon, R., Jeschke, M., et al. (2018). Analysis of long term study indicates both agronomic optimal plant density and increase maize yield per plant contributed to yield gain. Sci. Rep. 8, 4937. doi: 10.1038/s41598-018-23362-x
Bailey-Serres, J., Parker, J. E., Ainsworth, E. A., Oldroyd, G. E., and Schroeder, J. I. (2019). Genetic strategies for improving crop yields. Nature 575, 109–118. doi: 10.1038/s41586-019-1679-0
Basche, A. D., Archontoulis, S. V., Kaspar, T. C., Jaynes, D. B., Parkin, T. B., and Miguez, F. E. (2016). Simulating long-term impacts of cover crops and climate change on crop production and environmental outcomes in the Midwestern United States. Agric. Ecosyst. Environ. 218, 95–106. doi: 10.1016/j.agee.2015.11.011
Brown, M., Antle, J., Backlund, P., Carr, E., Easterling, B., Walsh, M., et al. (2015). Climate Change, Global Food Security and the US Food System. Available online at: https://www.usda.gov/oce/energy-and-environment/food-security (accessed December 27, 2021).
Burchfield, E. K., Schumacher, B. L., Spangler, K., and Rissing, A. (2022). The state of US farm operator livelihoods. Front. Sustain. Food Syst. 566, 795901. doi: 10.3389/fsufs.2021.795901
Challinor, A. J., Simelton, E. S., Fraser, E. D. G., Hemming, D., and Collins, M. (2010). Increased crop failure due to climate change: assessing adaptation options using models and socio-economic data for wheat in China. Environ. Res. Lett. 5, 034012. doi: 10.1088/1748-9326/5/3/034012
Cohn, A. S., Vanwey, L. K., Spera, S. A., and Mustard, J. F. (2016). Cropping frequency and area response to climate variability can exceed yield response. Nat. Clim. Chang. 6, 601–604. doi: 10.1038/nclimate2934
Elliott, J., Deryng, D., Müller, C., Frieler, K., Konzmann, M., Gerten, D., et al. (2014). Constraints and potentials of future irrigation water availability on agricultural production under climate change. Proc. Natl. Acad. Sci. U.S.A. 111, 3239–3244. doi: 10.1073/pnas.1222474110
FAO (2018). The Land That Shapes and Sustains Us. Washington, DC: FAO, 46–58. doi: 10.5822/978-1-61091-885-5_4
Fenton, M., Albers, C., and Ketterings, Q. (2008). Soil Organic Matter Agronomy Fact Sheet Series. Cornell, NY: Agronomy Fact Sheet Series University.
Fornara, D. A., and Tilman, D. (2009). Ecological mechanisms associated with the positive diversity–productivity relationship in an N-limited grassland. Ecology 90, 408–418. doi: 10.1890/08-0325.1
Ghimire, R., Machado, S., and Bista, P. (2017). Soil ph, soil organic matter, and crop yields in winter wheat-summer fallow systems. Agron. J. 109, 706–717. doi: 10.2134/agronj2016.08.0462
Gitz, V., Meybeck, A., Lipper, L., Young, C. D., and Braatz, S. (2016). Climate Change and Food Security: Risks and Responses. Food and Agriculture Organization of the United Nations (FAO) Report. Available online at: https://www.fao.org/3/i5188e/I5188E.pdf (accessed August 23, 2021).
Grassini, P., Eskridge, K. M., and Cassman, K. G. (2013). Distinguishing between yield advances and yield plateaus in historical crop production trends. Nat. Commun. 4, 2918. doi: 10.1038/ncomms3918
Gregory, P., Ingram, J. S., and Brklacich, M. (2005). Climate change and food security. Philos. Trans. R. Soc. B Biol. Sci. 360, 2139–2148. doi: 10.1098/rstb.2005.1745
Herrmann, H., and Bucksch, H. (2014). Rainwater. Dict. Geotech. Eng. Geotech. 2:1075. doi: 10.1007/978-3-642-41714-6_180309
Hobert, R., and Negra, C. (2020). Climate Change and the Future of Food. United Nations Found. Available online at: https://unfoundation.org/blog/post/climate-change-and-the-future-of-food/ (accessed December 11, 2021).
Hoffman, A. L., Kemanian, A. R., and Forest, C. E. (2020). The response of maize, sorghum, and soybean yield to growing-phase climate revealed with machine learning. Environ. Res. Lett. 15, 094013. doi: 10.1088/1748-9326/ab7b22
Hu, X., Chen, H., Wang, J., Meng, X., and Chen, F. (2009). Effects of soil water content on cotton root growth and distribution under mulched drip irrigation. Agric. Sci. China 8, 709–716. doi: 10.1016/S1671-2927(08)60269-2
IPCC (2021). Technical Summary. Contribution of Working Group I to the Sixth Assessment Report of the Intergovernmental Panel on Climate Change. IPCC.
Jiang, J., Wang, Y. P., Yu, M., Cao, N., and Yan, J. (2018). Soil organic matter is important for acid buffering and reducing aluminum leaching from acidic forest soils. Chem. Geol. 501, 86–94. doi: 10.1016/j.chemgeo.2018.10.009
Johnson, L. F., and Trout, T. J. (2012). Satellite NDVI assisted monitoring of vegetable crop evapotranspiration in california's san Joaquin Valley. Remote Sens. 4, 439–455. doi: 10.3390/rs4020439
Jones, D. A., and Hassan, O. T. (1991). Climate change and agriculture. Trends Ecol. Evol. 6, 101. doi: 10.1016/0169-5347(91)90186-2
Karavolias, N. G., Horner, W., Abugu, M. N., and Evanega, S. N. (2021). Application of gene editing for climate change in agriculture. Front. Sustain. Food Syst. 296, 685801. doi: 10.3389/fsufs.2021.685801
Kelley, L. (2020). Irrigate all the Way to the End. East Lansing, MI: Michigan State University Extension.
Kucharik, C. J., and Serbin, S. P. (2008). Impacts of recent climate change on Wisconsin corn and soybean yield trends. Environ. Res. Lett. 3, 034003. doi: 10.1088/1748-9326/3/3/034003
Kukal, M. S., and Irmak, S. (2018a). Climate-Driven crop yield and yield variability and climate change impacts on the U.S. great plains agricultural production. Sci. Rep. 8, 3450. doi: 10.1038/s41598-018-21848-2
Kukal, M. S., and Irmak, S. (2018b). US agro-climate in 20th century: growing degree days, first and last frost, growing season length, and impacts on crop yields. Sci. Rep. 8, 6977. doi: 10.1038/s41598-018-25212-2
Leng, G., Zhang, X., Huang, M., Asrar, G. R., and Leung, L. R. (2016). The role of climate covariability on crop yields in the conterminous United States. Sci. Rep. 6, 33160. doi: 10.1038/srep33160
Licht, M. A., Parvej, M. R., and Wright, E. E. (2019). corn yield response to row spacing and plant population in Iowa. Crop. Forage Turfgrass Manag. 5, 190032. doi: 10.2134/cftm2019.05.0032
Lobell, D. B., and Burke, M. B. (2010). On the use of statistical models to predict crop yield responses to climate change. Agric. For. Meteorol. 150, 1443–1452. doi: 10.1016/j.agrformet.2010.07.008
Lobell, D. B., and Field, C. B. (2007). Global scale climate-crop yield relationships and the impacts of recent warming. Environ. Res. Lett. 2, 014002. doi: 10.1088/1748-9326/2/1/014002
Lobell, D. B., and Gourdji, S. M. (2012). The influence of climate change on global crop productivity. Plant Physiol. 160, 1686–1697. doi: 10.1104/pp.112.208298
Lobell, D. B., Hammer, G. L., McLean, G., Messina, C., Roberts, M. J., and Schlenker, W. (2013). The critical role of extreme heat for maize production in the United States. Nat. Clim. Chang. 3, 497–501. doi: 10.1038/nclimate1832
Mahler, R. L., and McDole, R. E. (1987). Effect of soil pH on crop yield in northern idaho 1. Agron. J. 79, 751–755. doi: 10.2134/agronj1987.00021962007900040033x
Mbow, C., Rosenzweig, C., Barioni, L. G., Benton, T. G., Herrero, M., Krishnapillai, M., et al. (2019). “Food security,” in Climate Change and Land: An IPCC Special Report on Climate Change, Desertification, Land Degradation, Sustainable Land Management, Food Security, and Greenhouse Gas Fluxes in Terrestrial Ecosystems, eds P. R. Shukla, J. Skea, E. Calvo Buendia, V. Masson-Delmotte, H.-O. Pörtner, D.C. Roberts, P. Zhai, R. Slade, S. Connors, R. van Diemen, M. Ferrat, E. Haughey, S. Luz, S. Neogi, M. Pathak, J. Petzold, J. Portugal Pereira, P. Vyas, E. Huntley, K. Kissick, M. Belkacemi, J. Malley. Available online at: https://www.ipcc.ch/srccl/chapter/chapter-5/ (accessed January 1, 2022).
McLennon, E., Dari, B., Jha, G., Sihi, D., and Kankarla, V. (2021). Regenerative agriculture and integrative permaculture for sustainable and technology driven global food production and security. Agron. J. 113, 4541–4559. doi: 10.1002/agj2.20814
Messina, C., Hammer, G., Dong, Z., Podlich, D., and Cooper, M. (2009). Modelling Crop Improvement in a G×E×M Framework via Gene-Trait-Phenotype Relationships. Netherlands: Academic Press. doi: 10.1016/B978-0-12-374431-9.00010-4
Misselhorn, A., Aggarwal, P., Ericksen, P., Gregory, P., Horn-Phathanothai, L., Ingram, J., et al. (2012). A vision for attaining food security. Curr. Opin. Environ. Sustain. 4, 7–17. doi: 10.1016/j.cosust.2012.01.008
Moore, F. C., and Lobell, D. B. (2014). Adaptation potential of European agriculture in response to climate change. Nat. Clim. Chang. 4, 610–614. doi: 10.1038/nclimate2228
Motha, R. P., and Baier, W. (2005). Impacts of present and future climate change and climate variability on agriculture in the temperate regions: North America. Increas. Clim. Var. Chang. Reduc. Vulnerabil. Agric. For. 137–164. doi: 10.1007/1-4020-4166-7_7
Nelson, G. C., Rosegrant, M. W., Koo, J., Robertson, R., Sulser, T., Zhu, T., et al. (2009). Climate Change: Impact on Agriculture and Costs of Adaptation. Washington, DC: IFPRI.
Nielsen, R. L. (2021). Historical Corn Grain Yields in the U.S. Purdue University. Available online at: https://www.agry.purdue.edu/ext/corn/news/timeless/yieldtrends.html (accessed January 1, 2022).
Njouenwet, I., Vondou, D. A., Ashu, S. V. N., and Nouayou, R. (2021). Contributions of seasonal rainfall to recent trends in cameroon's cotton yields. Sustainability 13, 12086. doi: 10.3390/su132112086
NOAA (2012). National Drought Overview. Retrieved from https://www.ncdc.noaa.gov/sotc/drought/20120 (accessed January 1, 2022).
Ortiz-Bobea, A., and Tack, J. (2018). Is another genetic revolution needed to offset climate change impacts for US maize yields? Environ. Res. Lett. 13, 124009. doi: 10.1088/1748-9326/aae9b8
Osborne, T. M., and Wheeler, T. R. (2013). Evidence for a climate signal in trends of global crop yield variability over the past 50 years. Environ. Res. Lett. 8, 024001. doi: 10.1088/1748-9326/8/2/024001
Patil, I. (2018). ggstatsplot: “ggplot2” Based Plots With Statistical Details. Zenodo. doi: 10.5281/zenodo.2074621,2018
Pedersen, P., and Lauer, J. G. (2004). Soybean growth and development in various management systems and planting dates. Crop Sci. 44, 508–515. doi: 10.2135/cropsci2004.5080
Pedregosa, F. (2011). Scikit-learn: machine learning in python, Pedregosa et al. J. Mach. Learn. Res. 12, 2825–2830. Available online at: https://scikit-learn.org/stable/modules/generated/sklearn.feature_selection.SelectKBest.html (accessed August 23, 2021).
Pothula, S. K., Grewal, P. S., Auge, R. M., Saxton, A. M., and Bernard, E. C. (2019). Agricultural intensification and urbanization negatively impact soil nematode richness and abundance: a meta-analysis. J. Nematol. 51, 1–17. doi: 10.21307/jofnem-2019-011
Pugh, T. A. M., Müller, C., Elliott, J., Deryng, D., Folberth, C., Olin, S., et al. (2016). Climate analogues suggest limited potential for intensification of production on current croplands under climate change. Nat. Commun. 7, 12608. doi: 10.1038/ncomms12608
R Core Team (2021). R: A Language and Environment for Statistical Computing. Vienna: R Foundation for Statistical Computing. Available online at: https://www.R-project.org/ (accessed August 23, 2021).
Raza, A., Razzaq, A., Mehmood, S. S., Zou, X., Zhang, X., Lv, Y., et al. (2019). Impact of climate change on crops adaptation and strategies to tackle its outcome: a review. Plants 8, 34. doi: 10.3390/plants8020034
Reddy, K. R., Davidonis, G. H., Johnson, A. S., and Vinyard, B. T. (1999). Temperature regime and carbon dioxide enrichment alter cotton boll development and fiber properties. Agron. J. 91, 851–858. doi: 10.2134/agronj1999.915851x
Reddy, K. R., and Zhao, D. (2005). Interactive effects of elevated CO2 and potassium deficiency on photosynthesis, growth, and biomass partitioning of cotton. Field Crop. Res. 94, 201–213. doi: 10.1016/j.fcr.2005.01.004
Ribeiro, M. T., Singh, S., and Guestrin, C. (2016). Local Interpretable Model Agnostic Explanations (LIME): An Introduction. O'Reilly Media. Available online at: https://www.oreilly.com/content/introduction-to-local-interpretable-model-agnostic~explanations-lime/ (accessed December 27, 2021).
Riebsame, W. E., Parton, W. J., Galvin, K. A., Burke, I. C., Bohren, L., Young, R., et al. (1994). Integrated modeling of land use and cover change. Bioscience 44, 350–356. doi: 10.2307/1312385
Sawan, Z. M. (2017). Cotton production and climatic factors: studying the nature of its relationship by different statistical methods. Cogent Biol. 3, 1292882. doi: 10.1080/23312025.2017.1292882
Schlenker, W., and Roberts, M. J. (2009). Nonlinear temperature effects indicate severe damages to U.S. crop yields under climate change. Proc. Natl. Acad. Sci U.S.A. 106, 15594–15598. doi: 10.1073/pnas.0906865106
Serdeczny, O., Adams, S., Baarsch, F., Coumou, D., Robinson, A., Hare, W., et al. (2017). Climate change impacts in Sub-Saharan Africa: from physical changes to their social repercussions. Reg. Environ. Chang. 17, 1585–1600. doi: 10.1007/s10113-015-0910-2
Sommer, R., Glazirina, M., Yuldashev, T., Otarov, A., Ibraeva, M., Martynova, L., et al. (2013). Impact of climate change on wheat productivity in Central Asia. Agric. Ecosyst. Environ. 178, 78–99. doi: 10.1016/j.agee.2013.06.011
Thomson, A. M., Brown, R. A., Ghan, S. J., Izaurralde, R. C., Rosenberg, N. J., and Leung, L. R. (2002). Elevation dependence of winter wheat production in eastern Washington state with climate change: a methodological study. Clim. Change 54, 141–164. doi: 10.1023/A:1015743411557
Troy, T. J., Kipgen, C., and Pal, I. (2015). The impact of climate extremes and irrigation on US crop yields. Environ. Res. Lett. 10, 054013. doi: 10.1088/1748-9326/10/5/054013
University of Wisconsin Extension (2012). Got Low pH? Adjust Soil for Corn Production. Retrieved from: https://www.goldenharvestseeds.com/agronomy/articles/got-low-ph-adjust-soil-for-corn-production#:~:text=If%20pH%20drops%2C%20applying%20lime,delays%20and%20compacting%20the%20soil. (accessed December 27, 2021).
Urban, D., Roberts, M. J., Schlenker, W., and Lobell, D. B. (2012). Projected temperature changes indicate significant increase in interannual variability of U.S. maize yields: a letter. Clim. Change 112, 525–533. doi: 10.1007/s10584-012-0428-2
Van Tassel, D. L., Tesdell, O., Schlautman, B., Rubin, M. J., DeHaan, L. R., Crews, T. E., et al. (2020). New food crop domestication in the age of gene editing: genetic, agronomic and cultural change remain co-evolutionarily entangled. Front. Plant Sci. 11, 789. doi: 10.3389/fpls.2020.00789
Williams, A., Hunter, M. C., Kammerer, M., Kane, D. A., Jordan, N. R., Mortensen, D. A., et al. (2016). Soil water holding capacity mitigates downside risk and volatility in US rainfed maize: time to invest in soil organic matter? PLoS ONE 11, e0160974. doi: 10.1371/journal.pone.0160974
Xu, H., Twine, T. E., and Girvetz, E. (2016). Climate change and maize yield in Iowa. PLoS ONE 11, e0156083. doi: 10.1371/journal.pone.0156083
Xu, J., Mohamed, E., Li, Q., Lu, T., Yu, H., and Jiang, W. (2021). Effect of humic acid addition on buffering capacity and nutrient storage capacity of soilless substrates. Front. Plant Sci. 12, 644229. doi: 10.3389/fpls.2021.644229
Xu, T., Guan, K., Peng, B., Wei, S., and Zhao, L. (2021). Machine learning-based modeling of spatio-temporally varying responses of rainfed corn yield to climate, soil, and management in the U.S. corn belt. Front. Artif. Intell. 4, 647999. doi: 10.3389/frai.2021.647999
Xue, J., Bali, K. M., Light, S., Hessels, T., and Kisekka, I. (2020). Evaluation of remote sensing-based evapotranspiration models against surface renewal in almonds, tomatoes and maize. Agric. Water Manag. 238, 106228. doi: 10.1016/j.agwat.2020.106228
Keywords: climate change, crop production, environment, soils, explainable machine learning, local interpretable model-agnostic explanations
Citation: Sihi D, Dari B, Kuruvila AP, Jha G and Basu K (2022) Explainable Machine Learning Approach Quantified the Long-Term (1981–2015) Impact of Climate and Soil Properties on Yields of Major Agricultural Crops Across CONUS. Front. Sustain. Food Syst. 6:847892. doi: 10.3389/fsufs.2022.847892
Received: 03 January 2022; Accepted: 18 March 2022;
Published: 13 April 2022.
Edited by:
Amos P. K. Tai, The Chinese University of Hong Kong, ChinaReviewed by:
Roberto Quiroz, Centro Agronomico Tropical De Investigacion Y Ensenanza Catie, Costa RicaMuhammad Ashfaq, University of Agriculture, Faisalabad, Pakistan
Jia Mao, The Chinese University of Hong Kong, China
Copyright © 2022 Sihi, Dari, Kuruvila, Jha and Basu. This is an open-access article distributed under the terms of the Creative Commons Attribution License (CC BY). The use, distribution or reproduction in other forums is permitted, provided the original author(s) and the copyright owner(s) are credited and that the original publication in this journal is cited, in accordance with accepted academic practice. No use, distribution or reproduction is permitted which does not comply with these terms.
*Correspondence: Debjani Sihi, ZGViamFuaS5zaWhpQGVtb3J5LmVkdQ==