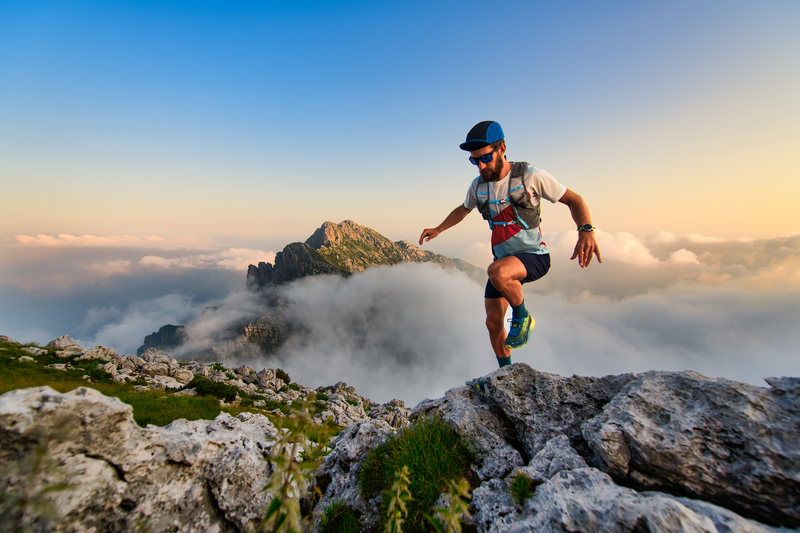
95% of researchers rate our articles as excellent or good
Learn more about the work of our research integrity team to safeguard the quality of each article we publish.
Find out more
ORIGINAL RESEARCH article
Front. Chem. , 12 August 2024
Sec. Medicinal and Pharmaceutical Chemistry
Volume 12 - 2024 | https://doi.org/10.3389/fchem.2024.1425220
This article is part of the Research Topic Exploring Novel Approaches to Small Molecule Kinase Inhibitors in Cancer Treatment View all 5 articles
The Janus kinase 3 (JAK3) family, particularly JAK3, is pivotal in initiating autoimmune diseases such as rheumatoid arthritis. Recent advancements have focused on developing antirheumatic drugs targeting JAK3, leading to the discovery of novel pyrazolopyrimidine-based compounds as potential inhibitors. This research employed covalent docking, ADMET (Absorption, Distribution, Metabolism, Excretion, Toxicity) analysis, molecular dynamics modeling, and MM/GBSA (Molecular Mechanics Generalized Born Surface Area) binding free energy techniques to screen 41 in silico-designed pyrazolopyrimidine derivatives. Initially, 3D structures of the JAK3 enzyme were generated using SWISS-MODEL, followed by virtual screening and covalent docking via AutoDock4 (AD4). The selection process involved the AMES test, binding affinity assessment, and ADMET analysis, narrowing down the candidates to 27 compounds that passed the toxicity test. Further covalent docking identified compounds 21 and 41 as the most promising due to their high affinity and favourable ADMET profiles. Subsequent development led to the creation of nine potent molecules, with derivatives 43 and 46 showing exceptional affinity upon evaluation through molecular dynamics simulation and MM/GBSA calculations over 300 nanoseconds, comparable to tofacitinib, an approved RA drug. However, compounds L21 and L46 demonstrated stable performance, suggesting their effectiveness in treating rheumatoid arthritis and other autoimmune conditions associated with JAK3 inhibition.
Rheumatoid arthritis (RA) is a chronic autoimmune disease that affects a large number of people globally (Hitchon et al., 2023; Klebanoff et al., 2023). With millions of people affected each year, the global incidence of RA emphasizes its tremendous impact on public health. According to recent research, this disabling ailment affects around 1% of the global population (Isaifan, 2020; Alharthi et al., 2022). These statistics highlight the critical need to address the illness burden and provide better solutions for people afflicted. RA requires additional examination for a variety of compelling reasons (ScienceDirect, 2022). Literature data report that JAK (Janus kinase) and other related proteins are involved in the pathophysiology and signalling pathways related to RA. They are essential in controlling immunological responses and inflammation, which are key elements in the onset and development of illness. Even though other proteins are also involved in RA, concentrating on JAK and the JAK-related proteins offers the chance to discover novel molecules that could interfere with the biochemical pathways involved in the onset of RA.
Signalling by JAK3 is involved in many cytokines that promote inflammation. Their suppression thwarts their pro-inflammatory activity. These two kinases contribute to T and B lymphocyte activation and proliferation (Henderson Berg et al., 2022). Their inhibition decreases immune system activation in autoimmune disorders. Inhibiting JAK3 reduces the production of pro-inflammatory cytokines such as interleukin-6 and interleukin-17, which are two important cytokines in rheumatoid arthritis (Henderson Berg et al., 2022; Kotyla et al., 2022). JAK3 inhibitors have shown great success in treating rheumatoid arthritis, decreasing inflammation and symptoms (Boyadzhieva et al., 2022; Chen et al., 2023; Sardana et al., 2023). One immunomodulatory method of action gives them a viable treatment choice for instances that are resistant to traditional treatments such as DMARDs and anti-TNF medicines. Many cytokines, including IL-2, IL-4, IL-7, IL-9, IL-15, and IL-21, contain the gamma subunit (Prasad et al., 2023). The binding of these cytokines to their receptors activates the JAK/STAT signalling pathway by phosphorylation of gamma by receptor-associated JAK kinases (Choy, 2019). The interleukins IL-7 and IL-15 activate JAK3, which is responsible for the phosphorylation of gamma 13. This phosphorylation, in turn, creates binding sites for STAT proteins. Once activated, these proteins boost the transcription of genes involved in lymphocyte proliferation and differentiation (Menet, 2018; Morris et al., 2018). Medication that inhibits JAK3 prevents the phosphorylation of gamma caused by related cytokines. This limits the activation of downstream STAT pathways and, hence, the pro-inflammatory immune response, which is driven mostly by T and B cells. JAK1 and JAK3, through gamma phosphorylation, play a major role in the signal transduction of several pro-inflammatory cytokines, explaining their involvement in autoimmune disorders (Menet, 2018). Regulation of these two kinases modulates immune system activation in autoimmune disorders by exerting similar effects on the JAK/STAT pathway. Preventing these proteins can reduce inflammation and delay disease progression, giving an alternative for individuals who do not respond to traditional treatments. The JAKs have an integrated pseudokinase domain (JH2) that modulates the neighboring kinase domain (JH1). The therapeutic targeting of JH2 domains has been less widely investigated and may represent an avenue to control the JAKs without the deleterious effects associated with targeting the nearby JH1 domain (Henry and Jorgensen, 2023; Rodriguez Moncivais et al., 2023). The FDA recently approved this drug, a TYK2 JH2 ligand, to treat plaque psoriasis, demonstrating the potential of this technique. In this light, the structure and targetability of the JAK pseudokinases are discussed, as well as the status of the development of ligands that bind to these domains (Grant et al., 2023; Henry and Jorgensen, 2023). The known approach to developing small-molecule JAK3 inhibitors is to target the JAK3 kinase domain’s. There’s four JAK protein, JAK1, JAK2, JAK3, and TYK2. JAK consists of a FERM domain, an SH2-linked domain, a kinase domain, and a pseudokinase domain. The kinase domain is essential for JAK function because it allows JAKs to phosphorylate proteins (Christy and Shankari, 2020). Information conveyed by the JAK3 protein influences the formation and maturation of white blood cells known as T lymphocytes, B lymphocytes, and natural killer cells, which are important for immune system regulation. Tofacitinib is a drug used to treat specific autoimmune illnesses, including rheumatoid arthritis (Yang et al., 2021). It works by regulating the immune system to minimize inflammation related to certain illnesses (Hosseini et al., 2020). Tofacitinib has been approved by numerous regulatory bodies, including the Food and Drug Administration (FDA) in the United States, for the treatment of rheumatoid arthritis and other autoimmune illnesses (Mogul et al., 2019; Roskoski JrR., 2023a).
In this study, JAK inhibitors, especially JAK3 inhibitors, are designed particularly to target JAK3, a protein that is crucial to the development of RA. The danger of interfering with unrelated cellular processes is reduced by carefully controlling JAK3 signalling, potentially lowering side effects and improving treatment specificity. The Cys909 residue of JAK3 is significant because it plays a critical role in the binding affinity and specificity of JAK3 inhibitors. JAK3 inhibitors must bind with Cys909 residue to inhibit JAK3 activity and subsequent signalling processes that contribute to the pathophysiology of RA. Previous studies demonstrated that selective and covalent JAK3 inhibitors target the Cys909 residue through a covalent link via a 1,4-Michael addition process with an acryl group (Figure 1) (Tan et al., 2015; Castelo-Soccio et al., 2023). This covalent alteration improves the inhibitors’ potency and selectivity, resulting in a more effective and targeted suppression of JAK3 activity. Taking into account these data, the acrylaldehyde group was considered a key structural element in the in silico design of our inhibitors for different reasons: a) the link formed between the inhibitor and the Cys909 residue of JAK3 enzyme is covalent and hardly dissociable; b) the covalent interaction interrupt JAK3’s functions and downstream signalling pathways permanently; c) the introduction of the acrylaldehyde group allows the design of inhibitors with tailored properties, enhanced efficiency, and lowered off-target effects. We focused on the in silico design of novel JAK3 inhibitors based on the pyrazolopyrimidine scaffold bearing the acrylaldehyde function (Shankar et al., 2022; Caveney et al., 2023). Pyrazolopyrimidine derivatives are aromatic nitrogen heterocycles with a wide range of biological activities such as antitumor, antimalarial, antiparasitic, insecticidal, antirheumatic, antibacterial, antifungal, cardioprotective, antiviral, anti-inflammatory, and antioxidant (Upadhyay, 2017; Namdeo et al., 2023). Drugs containing the pyrazolopyrimidine scaffold are currently marketed and prescribed to people who have insomnia. However, some new derivatives produce anxiolytic effects with relatively little sedation and are being developed for use as anti-anxiety drugs without sedative effects (these drugs include Zaleplon–hypnotic (Sonata®), Indiplon–hypnotic, Ocinaplon–anxiolytic, and Lorediplon–hypnotic).
Using the Structure-Based Virtual Screening (SBVS) approach, this research aimed to discover JAK3 inhibitors endowed with more potency and selectivity against JAK3 enzyme. The newly developed compounds demonstrated high molecular activity by engaging with their target. These compounds include sulfonamide groups, which are still being researched and used today, despite their difficulties in synthesis. However, with the means available today, we can achieve their synthesis due to their potent effects against autoimmune diseases (Ouyang et al., 2016; Mushtaq and Ahmed, 2023; Lim et al., 2024). In this pilot study, the pkCSM and ADMELab 2.0 tools (Azzam, 2023) were used to perform the ADMET analysis of 41 designed molecules. Compounds with no toxicity (resulted negative to the tests of AMES toxicity) were subjected to a second screening using AutoDock 4.2 software (AutoDock4 and AutoDockTools4, 2022). In addition, molecular dynamics simulations were performed using the GROMACS package (Abraham et al., 2023) for 300 ns to verify the stability of the best complexes. Finally, the binding free energy of two complexes was evaluated using the MM/GBSA method (Valdés-Tresanco et al., 2021). In the realm of Computational-Aided Drug Design (CADD), the objective is to strategically design 41 guides to pinpoint the top two molecules, each with its corresponding affinity. Through meticulous analysis, the development of these two molecules unfolds, revealing novel compounds boasting heightened potency and remarkable affinity. This enhancement is attributed to the essential incorporation of SO2. Consequently, the newly designed molecules exhibit notable stability during molecular dynamics simulations with MM/GBSA, suggesting their potential candidacy for in vivo and in vitro studies. Building upon the success of CADD as a guiding principle for prior in vivo and in vitro investigations, these newly identified molecules emerge as promising subjects for further exploration in the field (Al-Karmalawy et al., 2023).
When experimental structures are lacking, homology modeling emerges as a key tool in the field of protein structure determination (Abrigach et al., 2018). This technique consists of multiple consecutive processes, including template selection, target template alignment, model creation, validation, and assessment. In our study, the model creation phase used a variety of tools, with a focus on the SWISS-MODEL server (Nikolaev et al., 2018), to improve accuracy and dependability.
In this investigation, we used the Uniprot public domain protein database (http://www.uniprot.org/) to identify the protein sequence of Tyrosine-protein kinase JAK3 (P52333 JAK3 HUMAN, FASTA format (Figure 2) (JAK, 2022). P52333 was chosen as the target since it is one of the enzymes involved in the breakdown of host hemoglobin. As a result, significant crystallographic investigations on P52333 with different inhibitors have been done, opening the path for the application of computational approaches to investigate potential anti-rheumatoid arthritis medicines against JAK3 (Simoncic et al., 2002). Using the SWISSMODEL service, the acquired sequence was used to predict a 3D homology model of the Tyrosine-protein kinase JAK3 protein (betacoronavirus, 2019). The Basic Local Alignment Search Tool (BLAST) was used to find homologs that might be used as templates (Homology Modeling and Docking Studies, 2022). The templates were chosen because of their excellent quality, lack of mutations, strong match of ligand structure to experimental data, and resemblance to the target. As a result, the best template receptor (PDB: 4Z16) was chosen as the reference template (Tan et al., 2015). Once the model’s 3D structure was created, it was critical to analyse and certify its correctness.
A crucial stage in forecasting protein structures is evaluating the accuracy of protein structure models. In this work, the Ramachandran plot (Ho and Brasseur, 2005), a useful tool for evaluating protein structures and showing the dihedral angles (Ψ and φ) of amino acid residues, was generated using the SAVES web server. Based on these angles, the plot offers insights into various polypeptide conformations (Homology, 2022). Additionally, to raise the model’s quality significantly, we used the Qualitative Model Energy Analysis (QMEAN) score tool. This parameter enables us to separate superior homology models from worse ones (Benkert et al., 2008). We used the BIOVIASTUDIO program (Systèmes, 2020) to see the protein’s structure. Considering previous research on JAK3 inhibition as a treatment for rheumatoid arthritis, the active site in the protein generated by homology modelling for the covalent docking between ligand and protein, specifically through a covalent link with Cys909, bears important significance. These results emphasize Cys909s crucial function in regulating the activity and specificity of the protein-ligand interaction, particularly when targeting the JAK3 pathway for RA treatment. The discovery and characterization of this active region offer important insights for the design and development of new drugs that target JAK3 more effectively and selectively to cure this crippling condition.
Standard molecular docking and covalent molecular docking are two different techniques used to study the interactions between a ligand and a protein. Standard molecular docking relies on the use of algorithms to predict the most favourable conformation of the ligand-protein complex based on binding energy. Covalent molecular docking, on the other hand, considers covalent interactions between the ligand and the protein. In this case, the ligand is designed to contain a reactive function (warheads) that can form a covalent bond with a specific residue of the protein. This method is beneficial for studying enzymes and proteins involved in diseases, as it allows for specific targeting of the active residues of the protein.
Prior to molecular docking, the ligands were optimized to be docked using Avogadro software. Next, the JAK3 structure was downloaded from the RCSB database (PDB ID: 4Z16). The 4Z16 crystal complex contains the co-crystallized ligand 4LH, the protein 4Z16, and the co-crystal water molecules. The protein was prepared by removing all water molecules followed by protonation (adding hydrogens at pH = 7.00) to the JAK3 protein structure using Discovery Studio software. The active site of 4Z16 is defined by the sphere containing the co-crystallized ligand (4LH). Once the ligand and protein were prepared, we performed molecular docking using AD4 and AutoVina. The three-dimensional grid was defined using the AUTOGRID algorithm, which determines the binding energy of ligands with their receptor (Morris et al., 2008). The default grid size is x = 60, y = 60, and z = 60, with a distance between grid points of 0.375 Å (Morris et al., 2008). The centre of the grid is the active site of the receptor with coordinates (x = −6.68875 Å, y = −14.7757 Å, and z = 1.89597 Å). The docking results obtained by AD4 and Vina were visualized using Discovery Studio software. CovDock (Covalent Docking), the guide for the procedure, explains several actions that may be taken to complete the covalent docking approach utilizing AD4 with flexible side chains (Bianco et al., 2016).
The ligand alignment must first be constructed using the available Covalent.py script, and the ligand structure must be modelled with a piece of the alkylated residue already present. By defining the atom indices in the ligand file or by specifying a SMARTS pattern and the alignment atom indices in the pattern itself, the ligand alignment may be specified. The ligand that has been covalently attached to the residue is the process output. It is significant to remember that before beginning the covalent docking procedure, the ligand structure may need to be decreased in addition to the preceding procedures. Software like Avogadro (Rayan and Rayan, 2017) and the MM2 force field can be used for this. The prepareCovalent.py script may then be run with the reduced structure as an input to create the ligand alignment. Second, PDBQT files for the receptor structure and the covalent ligand must be created. The prepare receptor4.py script may create the default PDBQT files for AutoDock, provided MGLROOT is set up and installed. Thirdly, the stiff and flexible components that will be utilized for docking are generated using PDBQT files. Using the prepare_flexreceptor4.py script, the stiff portion of the receptor is extracted by indicating which residue should be made flexible. The processed ligand is treated in the same manner. Fourth, parameter files are created for the actual computation. The prepare gpf4.py script is used to build the GPF for AutoGrid, while the prepare dpf4.py script is used to generate the DPF for AutoDock. To ensure that the docking score accurately reflects the interaction between the flexible residue (the ligand) and the stiff receptor, the proper energy model must be explicitly defined in the DPF file. The output produced at each stage of the process is available in the output directory, and AutoGrid and AutoDock may be performed using the regular technique. The preparation of the ligand alignment, creation of PDBQT files, creation of stiff and flexible components, preparation of parameter files, and execution of AutoGrid and AutoDock are all included in the covalent docking approach. You will find the exact parameterization necessary for the ligand covalently attached to the protein in our article. Since the Cys909 residue is covalently attached to the ligand, a distinct force field is needed for the computations (Faris et al., 2024a).
In this study, our primary focus was on predicting new compounds containing an acrylaldehyde moiety that would favour an irreversible covalent bond with the Cys909 residue. For this reason, we employed covalent docking and compared the results with an FDA-approved medication, Tofacitinib, which exhibits potent inhibition of JAK3 but does not form an irreversible covalent bond that dissolves upon binding to JAK3. We chose Tofacitinib for comparison because it provides a suitable reference point due to its known biological activity and lack of irreversible covalent bonding.
To define the physicochemical characteristics and ADME properties of compounds, their SMILE strings were uploaded to ADMETlab 2.0 and pkCSM web programs (Xiong et al., 2021; Azzam, 2023), respectively. The pkCSM web program (Absorption, Distribution, Metabolism, Excretion, and Toxicity) is a computer program that predicts the pharmacokinetic features of small-molecule medicines. The procedure may be found on the webpage online (pkCSM, 2024). ADMET predicts critical factors such as oral bioavailability, plasma protein binding, and tissue distribution using a mix of mathematical models and databases, allowing researchers to swiftly assess a molecule’s potential as a therapeutic candidate (Faris et al., 2023a; Faris et al., 2023b). This strategy can greatly speed up drug development by reducing the need for animal and clinical trials, as well as save money by detecting problematic compounds early in the development process (Shit and Banka, 2019; Lane et al., 2020; Parvathaneni et al., 2023; Shi et al., 2023).
GROMACS is a popular and capable molecular dynamics simulation software suite (Abraham et al., 2023). The CHARMM GUI may be used to conveniently customize the preparation of input files for GROMACS simulations (Graphical User Interface) (CHARMM, 2024). The temperature and solvent conditions in the simulation may be specified using this tool. In our investigation, we simulated a complex in solution at a constant temperature of 310 K. The solvent was neutralized by sodium chloride ions and solvated in a 10 cubic box around the protein with the TIP3P water model. To build the protein-ligand combination for MD simulation, the CHARMM36 force field was used in conjunction with the CHARMM GUI (Brooks et al., 2009; Faris et al., 2024b; Faris et al., 2024c). A typical simulation begins with a minimization stage in which the system is relaxed to a local energy minimum. 200,000 minimization steps are taken in this situation. An equilibration phase in which the system is brought to temperature and mechanical equilibrium is established. This equilibration is done in two stages, with a 2 ns initial equilibration and a 5 ns final equilibration, both at a time step of 0.001 ps After equilibration, the simulation’s manufacturing phase may begin. This phase lasts 300 ns and is used to propagate the system’s molecular dynamics as well as sample and evaluate the characteristics of interest. The findings of a GROMACS simulation give significant insights into the behaviour of biological molecules and their interactions, making it a useful tool in a variety of fields of study.
Dynamics metrics in Supplementary Table S1 include RMSD, measuring the deviation of MD simulated structures from the initial configuration, indicating stability. RMSF highlights residue flexibility, RoG assesses protein compactness, and SASA monitors solvent accessibility. DSSP analyses secondary structure composition, while PCA extracts key components governing molecular dynamics. The average distance between atom locations in the simulated structure and the initial reference structure is measured by RMSD. It is an indicator of how far the molecular dynamics (MD) simulated structure has deviated from its initial configuration. A system with a lower RMSD value has less structural drift and is, therefore, more stable. During the MD simulation, RMSF estimates each residue’s variations around its average location. Higher RMSF values indicate that the residues are more flexible. Finding flexible and stiff protein regions may be done with the help of RMSF. The protein’s mass dispersion around its centre of mass is measured by RoG. It may identify the protein structure’s expansion and compactness. The structure may be folded or compacted if RoG is reduced, whereas compaction or unfolding may be indicated by an increase in RoG. The protein’s surface area that is accessible to the solvent is referred to as its “solvent-accessible surface area (SASA). Changes in SASA may be a sign of protein-ligand interactions, folding, ligand binding, or conformational changes. SASA is frequently used to examine the dynamics and stability of proteins. Each residue is given a secondary structure by the DSSP algorithm by its phi/psi angles and hydrogen bonding patterns. It is employed to examine the secondary structure’s composition and evolution during an MD simulation. For instance, alterations in the amount of beta- or alpha-sheets might shed light on the dynamics, folding, and stability of proteins. The free energy landscape governs the thermodynamics and kinetics of molecular activities within a solution. Principal Component Analysis (PCA) is a linear transformation method that extracts key components from data using a covariance matrix or a correlation matrix (normalized PCA). These matrices are built using atomic coordinates to describe the protein’s available degrees of freedom (DOF), such as Cartesian coordinates that define atomic displacements in each conformation.
The binding free energy (Eq. 1) may be used to calculate the affinity of receptors for small ligands. The gmx_MMPBSA tool was used to determine the binding free energy (Valdés-Tresanco et al., 2021), this corresponds to the most recent version of gmx_PBSA recognized for its performance for all guides, which you may examine (https://valdes-tresanco-ms.github.io/gmx_MMPBSA/v1.5.6). Using the molecular mechanics/generalized Born surface area (MM/GBSA) approach (Kumari et al., 2014; Valdés-Tresanco et al., 2021). The calculation Equations 1–7 that were employed in this investigation are listed below (Valdés-Tresanco et al., 2021): The total energy change represents the overall stability or instability of the protein-ligand complex in its environment. Each term is computed using the relevant equation, which is detailed in the following (Supplementary Table S2).
Recently, as shown in Figure 3, the compound (N-(3-((6-((1-(2-methoxyethyl)-1H-pyrazol-4-yl)amino)-1H-pyrazolo[3,4-d]pyrimidin-1-yl)methyl)phenyl)acrylamide), in a novel series including new derivatives of pyrazolopyrimidine, is a series of isomeric heterocyclic chemical compounds with the molecular formula C6H5N3. They form the central core of a variety of more complex chemical compounds, including some pharmaceuticals and pesticides. It has been characterized as a potent and selective inhibitor of JAK3, with an IC50 value of 0.1 nM, corresponding to a pIC50 value of 10 (Yin et al., 2020; Faris et al., 2023a; Faris et al., 2023b). Using this scaffolding as a basis, a high-speed technique was used to accelerate the creation of 41 new molecules reported in 2.
Through a covalent interaction with the residue Cys909, which is crucial for inhibiting JAK3, this work focused on JAK3. Target P52333 JAK3 HUMAN was used (Bergmann et al., 2014; Bodaar et al., 2022; Bodaar et al., 2022; Roskoski R., 2023b). It is essential to define the protein’s structure using homology modelling before researching ligand-receptor interactions. The UniProtKB database provided the P52333 JAK3 HUMAN target’s main amino acid sequence. The models were chosen based on their high target similarity, high resolution, and well-documented modelling applicability. Due to the PDB structure 4Z16 greater goodness of fit to experimental data and lack of mutations, it was selected as a viable model.
The target sequence was matched with the model (PDB: 4Z16) using the BLAST alignment tool to find the best alignment with a high level of sequence identity. Consequently, we discovered a model with a similarity of 62%, suggesting its robust and dependable character (Figure 4). Following that, we used the Discovery Studio 4.5 program to display the model’s final three-dimensional structure and locate its active site. This model was also validated using the Ramachandran plot and major chain characteristics acquired from PROCHECK (Abrigach et al., 2018).
It is critical to check the final model before beginning any docking analysis to identify any potential flaws or variations from the usual protein structure. To assess the quality of the predicted protein structure, the ProCHECK tool was used. The calculation of phi-psi torsion angles for each residue produced a Ramachandran plot, which revealed that 91.1 percent of amino acid residues were in the core regions (red color), 8.1 percent were in additional allowed regions (yellow color), and 0.8 percent were in generously allowed regions (light yellow color) (Figure 5A). In contrast, only 0% of residues were discovered in banned areas due to their substantial distance from the enzyme’s active site (white color). The measured parameters of our homology model (solid squares) fell within the dark band in each plot, representing well-refined structure results based on the six properties (Figure 5B): (a) Ramachandran plot quality, (b) peptide bond planarity, (c) unfavourable non-bonded interactions, (d) C tetrahedral distortion, (e) main-chain hydrogen bond energy, and (f) overall G-factor versus resolution. Based on these findings, we can certainly infer that our model corresponds to the parameters of a stable design. The validation results from the Ramachandran plot and main-chain parameter plots encourage us to assume that our model for P52333 JAK3 HUMAN was sufficiently dependable to perform ligand-protein docking study. The Ramachandran plot and main-chain parameter plot validation results led us to conclude that the model we generated for P52333 JAK3 HUMAN was sufficiently dependable to perform ligand-protein docking investigation in our study (En-nahli et al., 2022; Mahjoubin-Tehran et al., 2022).
Figure 5. (A) Ramachandran plot of predicted protein; (B) main-chain parameters obtained by PROCHECK.
QMEAN analysis was used to guarantee the data’s correctness. QMEAN assesses the quality of a protein model by combining three structural descriptions. The QMEAN Z-score assesses the similarity between the structural characteristics of the modeled protein and those seen in experimental structures (Saih et al., 2021).
Table 1 shows the findings for the various scoring function measurements, with related Z-scores: QMEAN: The overall score is 0.67 0.05, suggesting that the structure’s quality is typically rated positively. QMEAN Z-score: 0.49, indicating that the analysed structure deviates somewhat from the predicted distribution. C interaction energy Z-score: The score is −0.63, showing that the C interaction is less common than predicted. The Z-score for every atom pairwise energy is 0.27, indicating that this energy is somewhat greater than predicted. The solvation energy Z-score is −0.07, suggesting that the solvation energy is somewhat lower than predicted. Torsion Z-score: 0.37, indicating that the torsion is slightly beyond the normal range when compared to the reference distribution. Finally, these results reveal that the examined structure is generally of good quality, while elements such as C interaction and torsion deviate from the predicted average. These findings must be considered when interpreting the overall quality of the examined structure.
We conducted an in silico design of 41 molecules, as detailed in Table 2. The initial screening utilized the Ames toxicity index, leading to the identification of 27 non-toxic molecules. The design of novel molecules considered structural elements from known compounds with favourable pIC50 values of 10 (Figure 6). These molecules could form covalent bonds, specifically through acrylaldehyde (depicted in yellow), targeting the Cys909 residue. The blue regions were modified by combining various substituents known for their steric, electrostatic, hydrophobic, and hydrogen bond donor/acceptor properties. These modified structures underwent molecular docking simulations to select molecules with a high affinity (expressed in Kcal/mol), devoid of Ames toxicity and possessing suitable pharmacological characteristics. To validate molecular stability, we employed molecular dynamics simulations, a potent approach demonstrated effectively in previous impactful studies for the synthesis of new molecules.
Thus, a covalent docking technique was used in the following investigation of the 27 non-toxic derivatives. Covalent docking is a molecular modelling technique that predicts probable covalent binding sites between a small chemical and a target protein. This method is very useful in drug design since it allows for the precise targeting of active amino acid residues in the target protein. Covalent docking allows for a more extensive examination of the selected 19 molecules and their potential for interaction with the target protein. This stage will give us a better idea of how these compounds could interact with the target and forecast their efficacy as prospective medications.
As the setup of molecular docking, redocking is the process of repositioning a ligand that has been temporarily withdrawn from a protein binding site. The RMSD is commonly used to determine how closely the redocked pose matches with the crystallographic conformation or a reference conformation. The RMSD of 0.96 Å before redocking (Figure 7) suggests a close match between the redocked position and the reference conformation. In other words, the structure of the relocated ligand is very similar to that obtained experimentally or from a reference posture. Lower RMSD indicates higher structural agreement. This suggests that the redocking approach has been successful and is widely regarded as a good match.
Covalent Docking Screening.Afterward, the molecules selected on 5, representing AMES toxicity, underwent a covalent docking study. Following covalent docking, molecules 41 and 21, with the highest affinity, were selected for analysis and future investigation. To evaluate the pharmacokinetic properties and drug-likeness of the designed derivatives and confirm the respective stabilities, molecular dynamics (MD) simulations and MM/GBSA (Molecular Mechanics-Generalized Born Surface Area) were used. Table 3 provides the results obtained from the covalent docking of the selected molecules.
Molecular docking and covalent docking are two different methods for predicting the interaction between a target molecule and a ligand. Tofacitinib is a reversible inhibitor of JAK kinases. This implies that it does not require the formation of a covalent bond to inhibit its target’s activity. Covalent docking is typically used for molecules that have groups that favour the formation of irreversible bonds with their target. In this study, the target is the ligand-Cys909 (residue). The new compounds designed for covalent docking contain groups that are favourable for this method. Figure 8 shows the different modes and conformations of the ligand with the target during simulation, highlighting the best hot spots for binding to the target.
Figure 8. The new compounds in the active site. (A): L21. (B): L41. (C):43. (D):46. (E): Tofacitinib drug.
Covalent docking, more precisely covalent docking with acryldehyde, produces a Michael addition reaction between the Cys909 residue of JAK3 and a ligand containing acryldehyde. This method permits a covalent link to form between the ligand and the protein, resulting in a stable complex. Researchers may use this approach to investigate the binding affinity and specificity of ligands towards the target protein, JAK3, providing useful information for drug development and design in the context of JAK3 inhibition. The covalent docking with acryldehyde through a Michael addition reaction at position Cys909 offers a viable path for the creation of new JAK3-targeting medicinal drugs (Figures 7–9).
Figure 9. (A) The newly designed ligands on the active site of the JAK3 protein modeled. (B) Tofacitinib on the active site of the JAK3 reference protein (ID: 4Z16). Tofacitinib on the active site of the JAK3 reference protein (ID: 4Z16).
The analysis of covalent docking for new inhibitors allowed us to understand their reactivity in the active site corresponding to their affinity. The new compounds are known for the presence of non-covalent bonds of different types, with the presence of a key covalent bond with residue Cys909, considered the therapeutic target for the discovery of new drugs for rheumatoid arthritis and autoimmune diseases. Compound 21 is known for having three hydrogen bonds, two with Leu905 and one with Leu828 and ARG953, and 10 pi-alkyl bonds, one with Leu905 and ALA966, two with ALA853 and LEU828, and three with LEU956, as well as a carbon-hydrogen bond with GLU903. Compound 41 is known for having three hydrogen bonds, one with Leu905, Leu828, and ARG953, and 11 alkyl and pi-alkyl bonds, one with ARG911, Leu905, and ARG953, Val836, two with Ala853 and Leu828, three with Leu956, and a carbon-hydrogen bond with Tyr904 and Glu903.
Compounds 21 and 41 (Figure 10), following development, provide us with powerful new compounds with high affinity by adding SO2 groups, which show remarkable hydrogen bonds as indicated in the molecular docking analysis. Compound 43 (Figure 11) is known for having four hydrogen bonds, two with Leu905, one with Arg953, Leu905, and Cys909, and eight alkyl and pi-alkyl bonds, one with Arg953, Ala966, Val836, two with Ala853, three with Leu956, and a carbon-hydrogen bond with Gly908. Compound 46 (Figure 11) is known for having five hydrogen bonds, one with Arg953, Asp912, and Cys909, and two with Leu905, and 12 pi-alkyl bonds, one with Ala966, Cys909, Leu905, Val836, two with Leu828, Ala853, Cys909, three with Leu956, a carbon-hydrogen bond with Gly908, and a pi-sigma bond with Gly908.
Figure 10. The interactions between the ligands [(A) 21 and (B) 41] and the JAK3 protein involve 2D and 3D non-covalent bonds, while the interaction between the ligand and Cys909 forms a covalent bond.
Figure 11. The interactions between the ligands [(A) L43 and (B) L46]-JAK3 protein involve 2D and 3D non-covalent bonds, while the interaction between the ligand and Cys909 forms a covalent bond.
In comparison to the approved tofacitinib (Figure 12), observed interactions include five hydrogen bonds, one with Leu828, Arg911, Cys909, two with Leu905, alkyl and pi-alkyl bonds, one with Ala966, Ala853, two with Leu956, Leu828, and a pi-sigma bond with Leu956. The presence of hydrogen bonds plays a crucial role in the reactivity of molecules with proteins, influencing the affinity of binding and the potent biological activity. The newly designed molecules exhibit potent inhibition in the presence of hydrogen bonding, extending to the covalent realm.
Figure 12. The interactions between Tofacitinib and JAK3 protein involve 2D and 3D non-covalent bonds, while the interaction between the ligand and Cys909 forms a covalent bond.
The analysis of covalent docking for the new inhibitors has allowed us to understand their reactivity at the active site corresponding to their affinity. Compounds 21 (−8.85 kcal/mol) and 41 (−8.17 kcal/mol) already show good affinity for the JAK3 target due to their ability to form multiple hydrogen bonds and non-covalent interactions. The development of these compounds by adding SO2 groups has led to molecules 43 (−10.17 kcal/mol) and 46 (−10.28 kcal/mol) with even higher affinity.
These compounds form more hydrogen bonds, crucial for reactivity with the protein. Although the approved Tofacitinib also exhibits favourable interactions, the new compounds 43 and 46 appear to be more potent inhibitors based on their lower binding energy.
The newly designed molecules adhere to Lipinski’s rule, but only compounds 21, 41, 43, and 46 were subjected to further study, as they have not exhibited Ames’s toxicity. Considering these preliminary results, compounds 21, 41, 43, and 46, together with Tofacitinib as a reference compound, were further investigated (Table 4) using ADMELab 2.0 platform. The FDA-approved drug Tofacitinib is recommended for the treatment of inflammatory disorders such as rheumatoid arthritis, psoriatic arthritis, and ulcerative colitis. The newly designed molecules, which follow Lipinski’s guidelines, work by suppressing specific immunological signalling pathways, leading to a successful reduction of inflammation and alleviation of associated symptoms.
As reported in Ta, all selected compounds do not violate Lipinski’s rule, suggesting that they could be easily absorbed after oral administration since the Lipinski criteria were respected. The drug-likeness rule proposed by Veber evaluates the number of rotatable bonds (Rb) and the topological polar surface area (TSPA) to predict the ability of drugs to cross the cellular membrane from the gastrointestinal tract. Following Veber’s rule, the number of Rot should be less than 10, and TSPA should be TSPA < 140 and Rb < 10. All molecules reported in Ta respect these parameters, suggesting that they are endowed with good permeable properties.
The evaluation of drug permeability using Caco-2 cell lines serves as a crucial substitute for in vivo conditions. Their similarity to the human intestinal epithelium is indispensable for selecting promising drug candidates in development. Caco-2, with a value > −5.15 log cm/s, suggests proper permeability. A Caco-2 value implies that all molecules are present, and those with absorption greater than 30% suggest good absorption, indicating overall favourable molecule absorption. Distribution parameters suggest that all compounds are poorly distributed to the brain (Log BBB < −1), but compound 21 and tofacitinib can penetrate the CNS having Log PS > −2. The highest VDss value (volume of distribution at steady state in humans. Volume Distribution (VD) is a crucial metric describing in vivo drug distribution by connecting the administered dose to the initial concentration. VD values aid in predicting properties like plasma protein binding, body fluid distribution, and tissue uptake. A VD in the range of 0.04–20 L/kg suggests a correct VD for most compounds, except for compound 21, which exhibits a lower VD of 0.01. In terms of metabolism, as CYP3A4 is a crucial enzyme, the treatment of autoimmune diseases such as RA and cancer consequently involves the analysis of ADMET. Compounds L21 and L41 only inhibit CYP3A2, while compound L43 acts as a substrate and inhibits CYP3A4. On the other hand, compound L46 is a substrate for CYP3A4 and an inhibitor of CY21C19, CY21C9, and CYP3A4. In contrast, Tofacitinib inhibits CYP3A2. None of the substances have kidney OCT-2 substrate activity, and none have demonstrated AMES mutagenic activity. Regarding toxicity, none of the compounds have been linked to skin sensitivity, but all compounds, including tofacitinib, have been associated with hepatotoxicity, except for compound 21, which does not exhibit this toxicity.
Taking into account all the gathered data, the newly designed compounds (Table 5) display favorable drug-like properties, presenting a robust pharmacokinetic profile that ensures efficient oral absorption and suitable distribution in tissues.
A computer technique for simulating the actual physical motions of atoms and molecules is called MD simulation. It offers details on the alterations in protein structure that occur throughout time. Protein dynamics, folding, stability, and interactions may all be studied using MD. MD simulations give a dynamic representation of how proteins behave throughout time. One may gain a thorough knowledge of protein stability, flexibility, compactness, solvent exposure, and secondary structural changes by analysing RMSD, RMSF, RoG, SASA, H-bonds, DSSP, Fel, and PCA from MD trajectories (Figures 13–22).
Figure 13. RMSD, RMSF, SASA, and RoG plots for compounds 41 and 21 interacting with JAK3; Figure is not mentioned in the text.
Figure 18. DSSP analysis was performed during the 300 ns simulation for the Tofacitinib-JAK3 and Apo-protein.
Figure 19. Free energy 2D landscape (FEL) diagram. (A) Composed of 21. (B) Composed of 41. (C) Composed of 43. (D) Composed of 46. (E) Tofacitinib.
Figure 20. Principal component analysis. (A) Composed of 21. (B) Composed of 41. (C) Composed of 43. (D) Composed of 46. (E) Tofacitinib.
Figure 21. Free energy 3D landscape (FEL) diagram. (A) Composed of 21. (B) Composed of 41. (C) Composed of 43. (D) Composed of 46. (E) Tofacitinib.
Figure 22. The conformations that favor minimum energy. (A) Composed of 21. (B) Composed of 41. (C) Composed of 43. (D) Composed of 46. (E) Tofacitinib.
The RMSD analysis suggests a variation between the initial and final positions of the ligand during a 300 ns simulation. The results in Figure 13 show that compounds L21, L41, and L46 A experienced an RMSD ranging between 0.1 nm and 0.2. For compound L43 and Tofacitinib, the RMSD ranged from 0.1 to 0.4. The analysis of the RMSD results suggests stability in the following order: L46, L21, L41, L43, and Tofacitinib. The results of RoG have values ranging between 1.95 and 2 (compounds L46, L21, L41, L43) nm, and for Tofacitinib, they are between 1.95 and 2.05 nm. For SASA, the values are between 1,400 and 1,550 (compounds L46, L21, L41, L43) nm2 and for Tofacitinib between 1,450 and 1,600 nm2. The results of RoG and SASA suggest a compactness of the complexes for the newly designed molecules during the simulation, which is better than the Tofacitinib drug. The analysis of H-bonds shows that the compounds exhibit hydrogen interactions ranging from a minimum of one to a maximum of eight during an H-bond simulation, suggesting their high-affinity stability. The analysis of RMSF presents suitable results for the flexibility of residues for each complex. The RMSF results suggest stable residue movements for compounds L21 and L46 compared to the other complexes, confirming favourable stability for Ligands (L21 and L41) in interaction with JAK3 during a 300 ns simulation.
Contrasted with the apo-protein JAK3, the DSSP analysis (Figures 15–18) of complexes involving Ligands (L21, L41, L43, L46, and Tofacitinib) and JAK3 (Figures 13, 14) revealed that the recently designed compounds exhibited robust structural integrity during the simulation. In comparison to the apo-protein JAK3, the most prominent transitions observed in all three complexes were Coil and Turn, Turn, Extended strand, Coil with 3–10 helix, turn, and Alpha helix. These findings suggest the potential of the newly developed compounds as JAK3 inhibitors, as they consistently maintained stable secondary structures throughout the simulation.
The free energy landscapes are very useful for interpreting and analysing biomolecular processes such as molecular folding, aggregation, and recognition.
The analysis conducted by Fel and PCA (Figures 19–21) suggests that the conformations occurring during the 300 ns energy minima are as follows: For compound 21, a Radius of Gyration (RoG) of 1.90 nm and Root Mean Square Deviation (RMSD) of 0.20 nm were observed, with a PCA distribution of components (PC1 and PC1) ranging between (−2) -(+2) and (−3) -(+4). For compound 41, a RoG of 1.95 nm and RMSD of 0.25 Å were observed, along with a PCA distribution of components (PC1 and PC1) situated between (−2) -(+2) and (−3) -(+3). For compound 43, two minima were identified. The first exhibited a RoG of 1.94 nm and RMSD of 0.15 Å, while the second displayed a RoG of 1.98 nm and RMSD of 0.3 nm. The PCA distribution of components (PC1 and PC1) for the second minimum is situated between (−2) -(+3) and (−2) -(+2). For compound 46, a RoG of 1.91 nm and RMSD of 0.22 Å were observed, along with a PCA distribution of components (PC1 and PC1) ranging between (−10) -(+10) and (0) -(+20). Finally, for Tofacitinib, two minima were identified. The first exhibited a RoG of 1.91 nm and RMSD of 0.20 Å, while the second displayed a RoG of 1.93 nm and RMSD of 0.19 nm. The PCA distribution of components (PC1 and PC1) for the second minimum is situated between (−15) -(+15) and (0) -(+25). The analysis of Fel and PCA allows for defining the confirmations that occur and the minimum energy present in Figure 22.
The MM/GBSA analysis is an important step in the design of new target protein inhibitors, particularly in the field of drug discovery. This technique enables the energetic stability of protein-ligand complexes to be assessed and the binding strength between the two molecules to be predicted. It thus complements molecular docking and molecular dynamics analyses by providing a more accurate estimate of the affinity and stability of the complexes formed. This approach can help researchers select the most promising molecules for further study and guide the design of new compounds with improved affinity and energetic stability.
The MM/GBSA findings for the complexes involving ligand-JAK3 reveal significant total binding free energy (ΔTOTAL) values of −24.14 and −58.29 kcal/mol, respectively, in comparison to the tofacitinib-JAK3 complex (Figure 23). Various energy factors were considered when determining these energies. The ΔVDWAALS term signifies van der Waals energy, which is consistently negative across all ligand-protein complexes, suggesting an attractive interaction between the ligand and JAK3 protein atoms. The ΔEEL term, representing electrostatic energy, is also negative, indicating a favourable interaction between the positive and negative charges of the ligand and protein. The ΔEGB term, corresponding to Born solvation energy, is positive, indicating system desolvation upon complex formation. The ΔESURF term, denoting surface energy, is negative, implying a reduction in the surface exposure of molecules upon complex formation. The ΔGGAS term, reflecting the gas-phase energy of the protein-ligand complex, is negative, pointing to a robust ligand-JAK3 affinity. Lastly, the ΔGSOLV term, representing the solvation energy of the protein-ligand complex, is positive, indicating favourable solvation of the systems.
Collectively, these outcomes indicate that newly introduced ligands, in conjunction with the JAK3 protein, exhibit a robust affinity and enhanced energy stability when compared to the tofacitinib-JAK3 complex. This observation suggests their potential as effective JAK3 inhibitors. These findings hold promise and underscore the potential of these recently formulated compounds as inhibitors of JAK3.
To discover new pyrazolopyrimidine derivatives as JAK3 inhibitors based on the most active molecule with a pIC50 = 10 discovered in a previous study, this research used homology modelling, virtual screening, ADMET analysis, covalent docking, molecular dynamics (MD) simulation, and MM/GBSA. Previously, the three-dimensional structure of the JAK3 protein was developed using homology modelling. Then, the MM2 force field was used to extract and reduce the compound set initially. Using AutoDock 4.2, molecules from a set of 41 were selected for further screening. The compounds were initially evaluated by ADMET analysis based on the molecules having no AMES toxicity. The molecular docking results based on affinity and the ADMET results based on no AMES toxicity suggested molecules L21 and L41 for further study. Furthermore, to develop the latest compound based on the addition of SO2, which presents favourable interactions, substituents were added to different electrostatic, steric, hydrophobic, hydrogen bond donor, and acceptor groups to obtain good compounds among the new nine designs by taking the two best with high affinity (kcal/mol), allowing the selection of good molecules L43 and L46. The stability evaluation of the best-ranked compounds L21 and 41 in the first phase and L43 and L46 in the second phase in the JAK3 protein receptor binding pocket was conducted using MD simulation and MMGBSA binding free energy calculations considering that tofacitinib, an FDA-approved drug for the treatment of rheumatoid arthritis, as the reference from the initial docking instant to the instant t. Consequently, this research led to the discovery of new potent inhibitors. In conclusion, using various computational approaches, our work was effective in identifying new pyrazolopyrimidine derivatives as potential JAK3 inhibitors. However, further studies are needed to confirm the effectiveness of these compounds as therapeutic agents, including in vitro and in vivo testing. The computational strategy used in this work can be an efficient tool in the rational development of new drugs for various disorders.
The datasets presented in this study can be found in online repositories. The names of the repository/repositories and accession number(s) can be found in the article/Supplementary Material.
AF: Conceptualization, Data curation, Formal Analysis, Investigation, Methodology, Software, Writing–original draft, Writing–review and editing. IC: Formal Analysis, Investigation, Writing–original draft. RA: Investigation, Software, Writing–review and editing. AA: Investigation, Writing–review and editing. MA: Investigation, Writing–review and editing, Funding acquisition. ME: Supervision, Writing–review and editing.
The author(s) declare that financial support was received for the research, authorship, and/or publication of this article. The authors extend their appreciation to the Deanship of Research and Graduate Studies at King Khalid University for funding this work through Large Research Project under grant number RGP2/376/45.
The authors would like to express their gratitude to King Khalid University, Saudi Arabia, for providing administrative and technical support.
The authors declare that the research was conducted in the absence of any commercial or financial relationships that could be construed as a potential conflict of interest.
All claims expressed in this article are solely those of the authors and do not necessarily represent those of their affiliated organizations, or those of the publisher, the editors and the reviewers. Any product that may be evaluated in this article, or claim that may be made by its manufacturer, is not guaranteed or endorsed by the publisher.
The Supplementary Material for this article can be found online at: https://www.frontiersin.org/articles/10.3389/fchem.2024.1425220/full#supplementary-material
Abrigach, F., Rokni, Y., Takfaoui, A., Khoutoul, M., Doucet, H., Asehraou, A., et al. (2018). In vitro screening, homology modeling and molecular docking studies of some pyrazole and imidazole derivatives. Biomed. Pharmacother. 103, 653–661. doi:10.1016/j.biopha.2018.04.061
Alharthi, M., Hanif, I., and Alamoudi, H. (2022). Impact of environmental pollution on human health and financial status of households in MENA countries: future of using renewable energy to eliminate the environmental pollution. Renew. Energy 190, 338–346. doi:10.1016/j.renene.2022.03.118
Al-Karmalawy, A. A., Mousa, M. H. A., Sharaky, M., Mourad, M. A. E., El-Dessouki, A. M., Hamouda, A. O., et al. (2023). Lead optimization of BIBR1591 to improve its telomerase inhibitory activity: design and synthesis of novel four chemical series with in silico, in vitro, and in vivo preclinical assessments. J. Med. Chem. 67, 492–512. doi:10.1021/acs.jmedchem.3c01708
AutoDock4 and AutoDockTools4 (2022). Automated docking with selective receptor flexibility–Morris–2009–journal of computational chemistry–wiley online library. Available at: https://onlinelibrary.wiley.com/doi/full/10.1002/jcc.21256.
Azzam, K. A. (2023). SwissADME and pkCSM webservers predictors: an integrated online platform for accurate and comprehensive predictions for in silico ADME/T properties of artemisinin and its derivatives. Kompleks. Ispolzovanie Miner. Syra 325, 14–21. doi:10.31643/2023/6445.13
Benkert, P., Tosatto, S. C. E., and Schomburg, D. (2008). QMEAN: a comprehensive scoring function for model quality assessment. Proteins Struct. Funct. Bioinforma. 71, 261–277. doi:10.1002/prot.21715
Bergmann, A. K., Schneppenheim, S., Seifert, M., Betts, M. J., Haake, A., Lopez, C., et al. (2014). Recurrent mutation of JAK3 in T-cell prolymphocytic leukemia. Genes., Chromosomes Cancer 53, 309–316. doi:10.1002/gcc.22141
betacoronavirus (2019). A guideline for homology modeling of the proteins from newly discovered betacoronavirus, 2019 novel coronavirus (2019-nCoV)–dong–2020–journal of Medical Virology–wiley Online Library. Available at: https://onlinelibrary.wiley.com/doi/10.1002/jmv.25768.
Bianco, G., Forli, S., Goodsell, D. S., and Olson, A. J. (2016). Covalent docking using autodock: two-point attractor and flexible side chain methods. Protein Sci. 25, 295–301. doi:10.1002/pro.2733
Bodaar, K., Yamagata, N., Barthe, A., Landrigan, J., Chonghaile, T. N., Burns, M., et al. (2022). JAK3 mutations and mitochondrial apoptosis resistance in T-cell acute lymphoblastic leukemia. Leukemia 36, 1499–1507. doi:10.1038/s41375-022-01558-5
Boyadzhieva, Z., Ruffer, N., Burmester, G., Pankow, A., and Krusche, M. (2022). Effectiveness and safety of JAK inhibitors in autoinflammatory diseases: a systematic review. Front. Med. 9, 930071. doi:10.3389/fmed.2022.930071
Brooks, B. R., Brooks, C. L., Mackerell, A. D., Nilsson, L., Petrella, R. J., Roux, B., et al. (2009). CHARMM: the biomolecular simulation program. J. Comput. Chem. 30, 1545–1614. doi:10.1002/jcc.21287
Castelo-Soccio, L., Kim, H., Gadina, M., Schwartzberg, P. L., Laurence, A., and O’Shea, J. J. (2023). Protein kinases: drug targets for immunological disorders. Nat. Rev. Immunol. 23, 787–806. doi:10.1038/s41577-023-00877-7
Caveney, N. A., Saxton, R. A., Waghray, D., Glassman, C. R., Tsutsumi, N., Hubbard, S. R., et al. (2023). Structural basis of Janus kinase trans-activation. Cell. Rep. 42, 112201. doi:10.1016/j.celrep.2023.112201
CHARMM (2024). CHARMM-GUI. Available at: https://www.charmm-gui.org/.
Chen, J.-Y., Feng, Q. L., Pan, H. H., Zhu, D. H., He, R. L., Deng, C. C., et al. (2023). An open-label, uncontrolled, single-arm clinical trial of tofacitinib, an oral JAK1 and JAK3 kinase inhibitor, in Chinese patients with keloid. Dermatol. Basel, Switz. 239, 818–827. doi:10.1159/000532064
Choy, E. H. (2019). Clinical significance of Janus Kinase inhibitor selectivity. Rheumatology 58, 1122–1962. doi:10.1093/rheumatology/kez002
Christy, J., and Shankari, S. (2020). COMPUTATIONAL APPROACH TO STUDY MARINE DERIVED CORTISTATIN A MOLECULAR MECHANISM AS A JANUS KINASE 3 INHIBITOR. Rasayan J. Chem. 13, 1498–1505. doi:10.31788/rjc.2020.1335746
En-nahli, F., Baammi, S., Hajji, H., Alaqarbeh, M., Lakhlifi, T., and Bouachrine, M. (2022). High-throughput virtual screening approach of natural compounds as target inhibitors of plasmepsin-II. J. Biomol. Struct. Dyn. 0, 10070–10080. doi:10.1080/07391102.2022.2152871
Faris, A., Alnajjar, R., Guo, J., AL Mughram, M. H., Aouidate, A., Asmari, M., et al. (2024c). Computational 3D modeling-based identification of inhibitors targeting cysteine covalent bond catalysts for JAK3 and CYP3A4 enzymes in the treatment of rheumatoid arthritis. Molecules 29, 23. doi:10.3390/molecules29010023
Faris, A., Cacciatore, I., Alnajjar, R., Hanine, H., Aouidate, A., Mothana, R. A., et al. (2024b). Revealing innovative JAK1 and JAK3 inhibitors: a comprehensive study utilizing qsar, 3D-pharmacophore screening, molecular docking, molecular dynamics, and MM/GBSA analyses. Front. Mol. Biosci. 11, 1348277. doi:10.3389/fmolb.2024.1348277
Faris, A., Hadni, H., Ibrahim, I. M., and Elhallaoui, M. (2023b). In silico discovery of potent and selective Janus kinase 3 (JAK3) inhibitors through 3D-QSAR, covalent docking, ADMET analysis, molecular dynamics simulations, and binding free energy of pyrazolopyrimidine derivatives. J. Biomol. Struct. Dyn. 0, 4817–4833. doi:10.1080/07391102.2023.2222839
Faris, A., Hadni, H., Saleh, B. A., Khelfaoui, H., Harkati, D., Ait Ahsaine, H., et al. (2023a). In silico screening of a series of 1,6-disubstituted 1H-pyrazolo[3,4-d]pyrimidines as potential selective inhibitors of the Janus kinase 3. J. Biomol. Struct. Dyn. 0, 4456–4474. doi:10.1080/07391102.2023.2220829
Faris, A., Hadni, H., Saleh, B. A., Khelfaoui, H., Harkati, D., Ait Ahsaine, H., et al. (2024a). In silico screening of a series of 1,6-disubstituted 1H-pyrazolo[3,4-d]pyrimidines as potential selective inhibitors of the Janus kinase 3. J. Biomol. Struct. Dyn. 42, 4456–4474. doi:10.1080/07391102.2023.2220829
Grant, A. H., Rodriguez, A. C., Rodriguez Moncivais, O. J., Sun, S., Li, L., Mohl, J. E., et al. (2023). JAK1 pseudokinase V666G mutant dominantly impairs JAK3 phosphorylation and IL-2 signaling. Int. J. Mol. Sci. 24, 6805. doi:10.3390/ijms24076805
Henderson Berg, M.-H., del Rincón, S. V., and Miller, W. H. (2022). Potential therapies for immune-related adverse events associated with immune checkpoint inhibition: from monoclonal antibodies to kinase inhibition. J. Immunother. Cancer 10, e003551. doi:10.1136/jitc-2021-003551
Henry, S. P., and Jorgensen, W. L. (2023). Progress on the pharmacological targeting of janus pseudokinases. J. Med. Chem. 66, 10959–10990. doi:10.1021/acs.jmedchem.3c00926
Hitchon, C. A., Oneil, L., Peschken, C. A., Robinson, D. B., Fowler-Woods, A., and El-Gabalawy, H. S. (2023). Disparities in rheumatoid arthritis outcomes for North American Indigenous populations. Int. J. Circumpolar Health 82, 2166447. doi:10.1080/22423982.2023.2166447
Ho, B. K., and Brasseur, R. (2005). The Ramachandran plots of glycine and pre-proline. BMC Struct. Biol. 5, 14. doi:10.1186/1472-6807-5-14
Homology (2022). Homology modeling of human histone deacetylase 10 and design of potential selective inhibitors. J. Biomol. Struct. Dyn. 37 (14). Available at: https://www.tandfonline.com/doi/full/10.1080/07391102.2018.1521747.
Homology modeling and docking studies (2022). Homology modeling and docking studies of TMPRSS2 with experimentally known inhibitors camostat mesylate, nafamostat and bromhexine hydrochloride to control SARS-coronavirus-2 | biological and medicinal chemistry | ChemRxiv | Camb. Open Engage. Available at: https://chemrxiv.org/engage/chemrxiv/article-details/60c74a2a842e6517e5db2ebe.
Hosseini, A., Gharibi, T., Marofi, F., Javadian, M., Babaloo, Z., and Baradaran, B. (2020). Janus kinase inhibitors: a therapeutic strategy for cancer and autoimmune diseases. J. Cell. Physiology 235, 5903–5924. doi:10.1002/jcp.29593
Isaifan, R. J. (2020). The dramatic impact of the Coronavirus outbreak on air quality: has it saved as much as it has killed so far? Glob. J. Environ. Sci. Manag. 6, 275–288.
JAK (2022). JAK3–tyrosine-protein kinase JAK3–Homo sapiens (human) | UniProtKB | UniProt. Available at: https://www.uniprot.org/uniprotkb/P52333/entry.
Klebanoff, S. D., Rodda, L. B., Morishima, C., Wener, M. H., Yuzefpolskiy, Y., Bettelli, E., et al. (2023). Diminished responses to mRNA-based SARS-CoV-2 vaccines in individuals with rheumatoid arthritis on immune modifying therapies. 2023.01.03.23284167 Prepr. A. T. doi:10.1101/2023.01.03.23284167
Kotyla, P., Gumkowska-Sroka, O., Wnuk, B., and Kotyla, K. (2022). Jak inhibitors for treatment of autoimmune diseases: lessons from systemic sclerosis and systemic lupus erythematosus. Pharm. (Basel) 15, 936. doi:10.3390/ph15080936
Kumari, R., Kumar, R., Consortium, O. S. D. D., and Lynn, A. (2014). g_mmpbsa A GROMACS tool for high-throughput MM-PBSA calculations. J. Chem. Inf. Model. 54, 1951–1962. doi:10.1021/ci500020m
Lane, T. R., Foil, D. H., Minerali, E., Urbina, F., Zorn, K. M., and Ekins, S. (2020). Bioactivity comparison across multiple machine learning algorithms using over 5000 datasets for drug discovery. Mol. Pharm. 18, 403–415. doi:10.1021/acs.molpharmaceut.0c01013
Lim, M., Cong, T. D., Orr, L. M., Toriki, E. S., Kile, A. C., Papatzimas, J. W., et al. (2024). DCAF16-Based covalent handle for the rational design of monovalent degraders. ACS Cent. Sci. doi:10.1021/acscentsci.4c00286
Mahjoubin-Tehran, M., Aghaee-Bakhtiari, S. H., Sahebkar, A., Oskuee, R. K., Kesharwani, P., and Jalili, A. (2022). In silico and experimental validation of a new modified arginine-rich cell penetrating peptide for plasmid DNA delivery. Int. J. Pharm. 624, 122005. doi:10.1016/j.ijpharm.2022.122005
Menet, C. J. (2018). A dual inhibition, a better solution: development of a JAK1/TYK2 inhibitor. J. Med. Chem. 61, 8594–8596. doi:10.1021/acs.jmedchem.8b01397
Mogul, A., Corsi, K., and McAuliffe, L. B. (2019). Baricitinib: the second FDA-approved JAK inhibitor for the treatment of rheumatoid arthritis. Ann. Pharmacother. 53, 947–953. doi:10.1177/1060028019839650
Morris, G. M., Huey, R., and Olson, A. J. (2008). Using AutoDock for ligand-receptor docking. Curr. Protoc. Bioinforma. 24 (8.14.1), Unit 8.14–8.14.40. doi:10.1002/0471250953.bi0814s24
Morris, R., Kershaw, N. J., and Babon, J. J. (2018). The molecular details of cytokine signaling via the JAK/STAT pathway. Protein Sci. 27, 1984–2009. doi:10.1002/pro.3519
Mushtaq, I., and Ahmed, A. (2023). Synthesis of biologically active sulfonamide-based indole analogs: a review. Futur J. Pharm. Sci. 9, 46–13. doi:10.1186/s43094-023-00500-5
Namdeo, P., Gidwani, B., Tiwari, S., Jain, V., Joshi, V., Shukla, S. S., et al. (2023). Therapeutic potential and novel formulations of ursolic acid and its derivatives: an updated review. J. Sci. Food Agric. 103, 4275–4292. doi:10.1002/jsfa.12423
Nikolaev, D. M., Shtyrov, A. A., Panov, M. S., Jamal, A., Chakchir, O. B., Kochemirovsky, V. A., et al. (2018). A comparative study of modern homology modeling algorithms for rhodopsin structure prediction. ACS Omega 3, 7555–7566. doi:10.1021/acsomega.8b00721
Ouyang, G., Tong, R., Li, J., Bai, L., Ouyang, L., Duan, X., et al. (2016). Synthesis and antitumor evaluation of novel 5-hydrosulfonyl-1H-benzo[d]imidazole-2(3H)-one derivatives. Molecules 21, 516. doi:10.3390/molecules21040516
Parvathaneni, M., Awol, A. K., Kumari, M., Lan, K., and Lingam, M. (2023). Application of artificial intelligence and machine learning in drug discovery and development. J. Drug Deliv. Ther. 13, 151–158. doi:10.22270/jddt.v13i1.5867
pkCSM (2024). pkCSM. Available at: https://biosig.lab.uq.edu.au/pkcsm/.
Prasad, P., Verma, S., Ganguly, N. K., Chaturvedi, V., and Mittal, S. A. (2023). Rheumatoid arthritis: advances in treatment strategies. Mol. Cell. Biochem. 478, 69–88. doi:10.1007/s11010-022-04492-3
Rayan, B., and Rayan, A. (2017). Avogadro program for chemistry education: to what extent can molecular visualization and three-dimensional simulations enhance meaningful chemistry learning? WJCE 5, 136–141. doi:10.12691/wjce-5-4-4
Rodriguez Moncivais, O. J., Chavez, S. A., Estrada Jimenez, V. H., Sun, S., Kirken, R. A., et al. (2023). Structural analysis of janus tyrosine kinase variants in hematological malignancies: implications for drug development and opportunities for novel therapeutic strategies. Int. J. Mol. Sci. 24, 14573. doi:10.3390/ijms241914573
Roskoski, Jr R. (2023a). Deucravacitinib is an allosteric TYK2 protein kinase inhibitor FDA-approved for the treatment of psoriasis. Pharmacol. Res. 189, 106642. doi:10.1016/j.phrs.2022.106642
Roskoski, R. (2023b). Deucravacitinib is an allosteric TYK2 protein kinase inhibitor FDA-approved for the treatment of psoriasis. Pharmacol. Res. 189, 106642. doi:10.1016/j.phrs.2022.106642
Saih, A., Baba, H., Bouqdayr, M., Ghazal, H., Hamdi, S., Kettani, A., et al. (2021). In silico analysis of high-risk missense variants in human ACE2 gene and susceptibility to SARS-CoV-2 infection. BioMed Res. Int. 2021, 1–10. doi:10.1155/2021/6685840
Sardana, K., Bathula, S., and Khurana, A. (2023). Which is the ideal JAK inhibitor for alopecia areata – baricitinib, tofacitinib, ritlecitinib or ifidancitinib–revisiting the immunomechanisms of the JAK pathway. Indian Dermatology Online J. 14, 465. doi:10.4103/idoj.idoj_452_22
ScienceDirect (2022). Rheumatoid arthritis microenvironment insights into treatment effect of nanomaterials–ScienceDirect. Available at: https://www.sciencedirect.com/science/article/abs/pii/S1748013221002838.
Shankar, A., McAlees, J. W., and Lewkowich, I. P. (2022). Modulation of IL-4/IL-13 cytokine signaling in the context of allergic disease. J. Allergy Clin. Immunol. 150, 266–276. doi:10.1016/j.jaci.2022.06.012
Shi, Y., Wang, J., Ren, P., ValizadehAslani, T., Zhang, Y., Hu, M., et al. (2023). Fine-tuning BERT for automatic ADME semantic labeling in FDA drug labeling to enhance product-specific guidance assessment. J. Biomed. Inf. 138, 104285. doi:10.1016/j.jbi.2023.104285
Shit, P., and Banka, H. (2019). A feature-reduced discretized random forest model for oral bioavailability data classification. Comput. Intell. Theor. Appl. Future Directions-Volume II ICCI- 2017, 27–37. doi:10.1007/978-981-13-1135-2_3
Simoncic, P. D., Lee-Loy, A., Barber, D. L., Tremblay, M. L., and McGlade, C. J. (2002). The T cell protein tyrosine phosphatase is a negative regulator of janus family kinases 1 and 3. Curr. Biol. 12, 446–453. doi:10.1016/s0960-9822(02)00697-8
Systèmes, D. (2020). Free download: BIOVIA discovery Studio visualizer. Dassault systèmes. Available at: https://discover.3ds.com/discovery-studio-visualizer-download.
Tan, L., Akahane, K., McNally, R., Reyskens, K. M. S. E., Ficarro, S. B., Liu, S., et al. (2015). Development of selective covalent Janus kinase 3 inhibitors. J. Med. Chem. 58, 6589–6606. doi:10.1021/acs.jmedchem.5b00710
Upadhyay, R. (2017). Therapeutic and pharmaceutical potential of cinnamomum tamala. Pharm. Pharm. Sci. 6, 18–28.
Valdés-Tresanco, M. S., Valdés-Tresanco, M. E., Valiente, P. A., and Moreno, E. (2021). gmx_MMPBSA: a new tool to perform end-state free energy calculations with GROMACS. J. Chem. theory Comput. 17, 6281–6291. doi:10.1021/acs.jctc.1c00645
Xiong, G., Wu, Z., Yi, J., Fu, L., Yang, Z., Hsieh, C., et al. (2021). ADMETlab 2.0: an integrated online platform for accurate and comprehensive predictions of ADMET properties. Nucleic Acids Res. 49, W5–W14. doi:10.1093/nar/gkab255
Yang, X., Zhan, N., Jin, Y., Ling, H., Xiao, C., Xie, Z., et al. (2021). Tofacitinib restores the balance of γδTreg/γδT17 cells in rheumatoid arthritis by inhibiting the NLRP3 inflammasome. Theranostics 11, 1446–1457. doi:10.7150/thno.47860
Yin, Y., Chen, C. J., Yu, R. N., Shu, L., Wang, Z. J., Zhang, T. T., et al. (2020). Novel 1H-pyrazolo[3,4-d]pyrimidin-6-amino derivatives as potent selective Janus kinase 3 (JAK3) inhibitors. Evaluation of their improved effect for the treatment of rheumatoid arthritis. Bioorg. Chem. 98, 103720. doi:10.1016/j.bioorg.2020.103720
Keywords: tyrosine kinase, autoimmune diseases, rheumatoid arthritis, cancer, JAK3
Citation: Faris A, Cacciatore I, Alnajjar R, Aouidate A, AL Mughram MH and Elhallaoui M (2024) Computational insights into rational design and virtual screening of pyrazolopyrimidine derivatives targeting Janus kinase 3 (JAK3). Front. Chem. 12:1425220. doi: 10.3389/fchem.2024.1425220
Received: 29 April 2024; Accepted: 12 June 2024;
Published: 12 August 2024.
Edited by:
Cristian O. Salas, Pontificia Universidad Católica de Chile, ChileReviewed by:
Khaled Mohamed Darwish, Suez Canal University, EgyptCopyright © 2024 Faris, Cacciatore, Alnajjar, Aouidate, AL Mughram and Elhallaoui. This is an open-access article distributed under the terms of the Creative Commons Attribution License (CC BY). The use, distribution or reproduction in other forums is permitted, provided the original author(s) and the copyright owner(s) are credited and that the original publication in this journal is cited, in accordance with accepted academic practice. No use, distribution or reproduction is permitted which does not comply with these terms.
*Correspondence: Abdelmoujoud Faris, YWJkZWxtb3Vqb3VkLmZhcmlzQHVzbWJhLmFjLm1h
†ORCID: Abdelmoujoud Faris, orcid.org/0000-0003-0781-6523; Radwan Alnajjar, orcid.org/0000-0003-1869-9596; Ivana Cacciatore, orcid.org/0000-0001-6253-0443; Mohammed H. AL Mughram, orcid.org/0000-0002-7343-4083
Disclaimer: All claims expressed in this article are solely those of the authors and do not necessarily represent those of their affiliated organizations, or those of the publisher, the editors and the reviewers. Any product that may be evaluated in this article or claim that may be made by its manufacturer is not guaranteed or endorsed by the publisher.
Research integrity at Frontiers
Learn more about the work of our research integrity team to safeguard the quality of each article we publish.