- Department of Biotechnology, School of Bio Sciences and Technology, Vellore Institute of Technology, Vellore, India
Introduction: Targeting tumor microenvironment is beneficial and present an ideal setting for the development of futuristic immunotherapy. Here, we make use of Nuclear prelamin A recognition factor (NARF), a protein linked to the coactivation of transcriptional regulators in human breast cancer stem cells (CSC) in our investigation.
Methods: In this study, we initially computed the epitope regions possessing the ability to stimulate both T and B cells within the NARF protein. These identified epitope areas were fused with an adjuvant such as RpfB and RpfE as well as linkers like AAY, GPGPG, KK, and EAAAK. The constructed vaccine was further characterized by assessing its physicochemical properties and population coverage. The potential interactions of the designed vaccine with different toll-like receptors were examined by a sequence of computational studies. Of note, docking study were employed to understand its mechanism of action. Molecular dynamics and immune simulation studies were conducted to comprehend more into their structural stability and immune responses. The resultant vaccine was back-translated, codon-optimised and introduced into pET-28 (+) vector.
Results and discussion: We hypothesize from the results that the designed NARF protein-based vaccine in our analysis could effectively provoke the immune responses in the target organism through TLR-7 binding and promotes MHC class-II mediated antigen presentation. Indeed, comprehensive evaluations conducted in both in vitro and in vivo settings are imperative to substantiate the safety and efficacy of the developed vaccine.
1 Introduction
Triple-negative breast cancer (TNBC) is a highly aggressive subtype of breast cancer that progresses more quickly and has a higher chance of metastasis and recurrence [1, 2]. The treatment options are limited to chemotherapy because of the lack of expression of standard molecular targets such as estrogen receptor (ER), progesterone receptor (PR), and human epidermal growth factor receptor 2 (HER2). Apparently, traditional chemotherapeutic agents, such as anthracycline, paclitaxel and alkylating agents, may trigger systemic toxicity and disruptive effects [3]. The challenges related to the inherent heterogeneity and aggressive nature of TNBC are further complicated by the limited availability of prognostic biomarkers for treatment response [4]. Immunotherapy has recently emerged as a game-changing technique in the treatment of a wide range of tumors [5]. It should be noted that the immunogenic landscape observed in TNBC has been correlated with an exciting response to immunotherapy. Clearly, the increased prevalence of tumor-infiltrating lymphocytes (TILs), a substantial tumor mutational load and survival correlations with the extent of T-cell and B-cell infiltration rendered immunotherapy an appealing therapeutic choice for TNBC [6, 7].
One of the most cutting-edge immunotherapeutic technique is the use of cancer vaccines to provoke active immune responses in the breast tumor environment. The key benefit of these subunit or peptide vaccines are their low toxicity and targeted adaptive immune response [8]. Interestingly, the ongoing clinical trials exploring chimeric peptide-based vaccines for TNBC includes MUC1 (NCT00986609), CDX-1401 (NCT02661100) PVX- 10 (NCT03362060), P10s-PADRE (NCT02938442) [9]. The incorporation of novel overexpressed proteins into vaccine design has revolutionized the scientific landscape, paving the way for enhanced treatment strategies. The primary downside of this multi-epitope vaccine is its exclusivity to personalized treatment regimen. Certainly, peptide-based vaccines account for 45.5% of clinical studies for breast cancer [10]. For instance, Rajendran et al. developed a novel transcription factor based MZF-1 and SOX9 vaccines for the management of metastatic TNBC [11, 12]. Tingting et al. developed an octamer-binding transcription factor 4 (OCT4) based anti-cancer vaccine, and it’s in vivo investigations have demonstrated significant tumor growth suppression [13]. Recently, Mahdevar et al. utilized immunoinformatics to identify B and T-cell-inducing epitopes for BORIS cancer-testis antigen against breast cancer. Of note, the developed vaccine considerably triggered immune responses both in vitro and in vivo environment [14]. It is worth mentioning that our team devised a successful multi-epitope vaccine targeting Sema4A, a transmembrane protein that has been recognised as a vital regulator of TNBC [15].
Despite the growing interest on immunotherapy, the prospective use of CSC-associated antigens as vaccines are quite limited. Therefore, in order to effectively battle cancer, it is imperative to identify and eradicate CSC in addition to the elimination of tumor cells [16, 17]. NARF associated with hypoxia-inducible HIF-1 target gene, is currently reported to be overexpressed in human breast cancer patients. It recruits histone lysine demethylase 6A (KDM6A) to OCT4 binding sites to activate the NANOG, SOX2, and KLF4 genes, which specify breast CSC. Note that the HIF-1 pathway is highly activated in TNBC relative to other isotypes and is therefore reported to be an excellent candidate for cancer vaccine development [18, 19]. In light of these references, the current study aimed to establish a subunit vaccine combining immunoinformatics techniques and simulation analysis focusing on NARF, a recently identified oncogenic promoter, to overcome the burden associated with TNBC.
2 Materials and methods
2.1 Protein and adjuvants dataset retrieval
The target protein sequence, namely, NARF (Q9UHQ1), adjuvants such as RpfB (P9WG29) and RpfE (O53177) were retrieved in standard FASTA format from the UniProt database [20]. The three-dimensional structure of immune receptors, namely, TLR-2, TLR-4, TLR-7 and TLR-9 were obtained from the Protein Data bank for our analysis [21].
2.2 Identification and screening of potential immunodominant epitopes
The intricate binding of peptide-MHC molecules represents the most selective phase in the process of antigen presentation. Thus, the NetMHCpan 4.1 and NetMHCIIpan-4.0 tools were used respectively to examine cytotoxic T-Lymphocyte (CTL) and Helper T-Lymphocyte (HTL) epitope region [22]. The identification of linear and discontinuous B-cell epitopes was facilitated by ABCpred and ElliPro tools respectively [23, 24]. The algorithm gives a protrusion index (PI) score to each predicted discontinuous B-cell epitope. Finally, the assessment of antigenicity, allergenicity, immunogenicity, toxicity and interferon inducing properties were predicted respectively using VaxiJen 2.0, AllerTOP 2.0, IEDB and ToxinPred online tools [25–29].
2.3 Designing and assessing the vaccine construct
The determined epitopes were paired with adjuvants and linkers to generate a complete vaccine. The details of linkers for HTL, CTL, B-cell epitopes together with the agonist linker were retrieved from the scientific literature [30–33]. The final construct is subjected to multi-tier analysis to examine the vaccine like characteristics. For instance, The physicochemical properties, including instability index, molecular weight, pI, half-life, and secondary structure distribution, of the vaccine construct were assessed using the Expasy Protparam and PSIPRED online tools [34, 35]. In addition, the immunogenic and allergenic properties of the vaccine construct were examined. Further, the Robetta server was deployed to model the tertiary structure of the vaccine construct [36]. The resultant model was refined by side chain reconstruction and the overall structure was relaxed to enhance the model’s fidelity. The model was then validated to detect any potential errors in the anticipated structure with the aid of overall quality and Z-score [37–41]. The optimised structure was then docked with TLR-2, TLR-4, TLR-7, and TLR-9 using ClusPro 2.0 (PDB IDs: 3A7C, 4G8A, 7CYN, and 3WPF) in order to assess the immunological response potential of the vaccine. The complex that exhibited the lowest energy score and best binding efficiency were selected to gain insights into their binding pattern [42, 43]. The detailed description of the methods was mentioned in our recent publication [15].
2.4 Molecular dynamic simulation
The regulation of key cellular functions is governed by macromolecular complexes within living cells. The mechanism of action of these complexes is dynamic and characterised by large, collective conformational changes. Hence, there is a significant need for tools that can efficiently model these motions in an easy and rapid manner. Normal Mode Analysis (NMA) is a very useful technique for determining the collective functional motions of such macromolecules [44–46]. In particular, iMODS a publicly accessible server facilitates the exploration of NMA in internal (dihedral) coordinates. Thus, in the present study the target proteins deformability, B-factor, eigen values, covariance map and elastic network were assessed using the iMODS server [47]. The deformability plot reveals the flexibility of the protein, whereas the B-factor illustrates the atomic distortion from its original equilibrium structure. Similarly, the eigenvalue reflects the molecular motion’s rigidity, which is pivotal in defining the protein’s stability, and a higher score suggests a more substantial resistance to distortion.
2.5 Population coverage, immune simulation and in silico cloning
The Immune Epitope Database (IEDB) was employed to perform a combined population coverage analysis of HLA classes I and II in the global and Indian populations [48]. To simulate the immune response of B and T lymphocytes induced by the prepared vaccine construct, the online simulation server C-ImmSim was utilized [49]. In the present study, the volume of the simulation and the simulation steps were adjusted to 50 and 1,050, respectively. It should be noted that one step of the simulation is equivalent to 8 hours (8 h) of real-time. Hence, 1,050 steps allows the immune response modelling for about 350 days [i.e. (1050 × 8 h)/(24 h)] with about 50 µL of simulation volume. Similarly, the recommended minimum time between two vaccination doses is 4 weeks. Therefore, steps 1, 84, and 168 are the computational approach for administering the vaccine. Precisely, the concept of three doses is to provide an additional strength to our immune system. Further to envision the in silico cloning, the SnapGene software was utilized. With the JCat, back-translation and optimisation were carried out to guarantee the best possible expression of the peptide vaccine in the K12 strain of E. coli. JCat estimates the translation efficiency and calculates organism-specific parameters such as codon adaptation index (CAI) and guanine/cytosine (GC) content. The codon-optimised sequence was then cloned into the pET-28 (+) vector with the aid of SnapGene package [50, 51].
3 Results
3.1 Immunogenic region identification and characterization of the determined epitopes
The initial analysis identified 57 CTL, 101 HTL and 43 B-cell epitope regions in the NARF protein. The epitopes that exhibited the ability to bind to a minimum of two MHC class I and II supertypes were scrutinized. Further data reduction was accomplished through antigenic and toxic characteristics. This process yielded 3CTL, 3HTL and 3 B-cell non-toxic, non-allergenic and antigenic epitopes. The list is shown in Table 1. The resultant HTL, CTL, and B-cell epitopes linked with the aid of GPGPG, AAY, and KK linkers respectively. We have generated multiple vaccine constructs with various TLR-4 agonists as adjuvants to identify the most immunogenic architecture. For instance, EAAAK was used to join the TLR-4 agonists with two ends of the vaccine. It is observed that the construct containing RpfB (N-terminal) and RpfE (C-terminal) adjuvants displayed the maximum immunogenicity of 7.92856, which is considered for further exploration (Table 2). This hierarchical construction process yields the final vaccine design, which has an antigenicity score of 0.63. The designed vaccine is illustrated in Figure 1. Of note, the vaccine construct is found to be non-toxic, non-allergenic and exhibits excellent population coverage. Precisely, the resultant T cell epitopes could cover 96.99% of the global population and 99.38% of the Indian population (Supplementary Figure S1). The construct encompassing RpfB and RpfE adjuvants exhibited substantial physicochemical features such as low molecular weight (71.89 kDa), stability (36.85), thermostability (81.02), hydrophilicity (−0.228), solubility (0.885) and a longer half-life. The computed theoretical isoelectric point (pI) of 5.15 indicated that the designed vaccine was acidic in nature. Table 2 summarises the physicochemical characteristics of vaccine constructs using diverse adjuvants. Four conformational B-cell epitopes were also spotted with scores ranging from 0.683 to 0.963 in the final design (Supplementary Table S1).
3.2 Structural modelling and population coverage
The literature evidence reveals that the α-helix and coiled regions are significant “structural antigen” types that contribute to antibody recognition and greater stability of the vaccine [17, 52]. In the present study, the PSIPRED analysis revealed that the designed vaccine consists of 136 amino acids (19.76%) in α-helix, 398 amino acids (57.93%) in coiled region, and 153 amino acids (22.27%) in β-strand (Supplementary Figure S2A). Based on the findings, it can be concluded that a larger percentage of coiled regions indicates a more stable vaccine construct. The Robetta server was used to create the 3D model of the vaccine, and the model with the highest ERRAT score of 93.83% was selected for examination. Notably, refinement resulted in a significant improvement in Ramachandran plot analysis ranging from 85.80% to 91.40% (Supplementary Figure S2B) and the Z-score of the model was predicted to be −9.88.
3.3 Molecular docking and dynamics of the vaccine construct with potential receptors
The docking results revealed that the designed vaccine binds to the TLR-2 (−1277.5 kcal/mol), TLR-4 (−1120.1 kcal/mol), TLR-7 (−1808.6 kcal/mol), and TLR-9 (−1353.6 kcal/mol) receptors with the lowest binding energy. The docked complexes were explored to gain insight into the binding pattern (Supplementary Figure S3). The number of intermolecular interactions displayed in the complex structures are reported in Supplementary Table S2. Despite the fewer intermolecular interactions in the TLR-7-vaccine complex, the major contribution of the desolvation energy plays a significant role in the successful binding of the partner molecule. Furthermore, the normal mode analysis from the iMODS server modifies the force field at different time intervals and examines the overall stability of the vaccine-TLR complexes. In comparison to the other complexes, the TLR-7 vaccine complex exhibited superior dynamic characteristics such as fewer deformability peaks, minimal atomic displacements, higher eigenvalue (1.2328e−06), lower variance, correlated motions and stiffer complex (Supplementary Figures S2, S3, Supplementary Figures S4 and S5). It is worth mentioning that these results correlate well with the docking studies.
3.4 In silico cloning and immune profile characterization
The codon optimized vaccine sequence displayed an exceptional CAI (0.988) and GC content (56.04%). The sequence is further sandwiched between the pET28a (+) vector’s XhoI and PpuMI restriction sites. The final vaccine clone, with a length of 5,365 base pairs, is depicted in Figure 2. Further, the system response to the designed vaccine was examined by means of simulation study. It is evident from the figure that there was a sharp increase in IgM and IgG antibody levels, followed by a decrease in antigen levels (Figure 3A). Adding together, the elevated levels of cytokines and interleukins were accompanied by a simultaneous reduction in antigen levels (Figure 3B). This trend hypothesizes that the ability of the vaccine’s CTL and HTL epitopes to stimulate immune cells. For instance, the levels of dendritic cells, macrophages, natural killer and cytotoxic T cells generally remained stable with dynamic changes (Figures 3C–F). Collective results from our simulation studies hypothesize that the secondary and tertiary responses were more effective than the primary response.
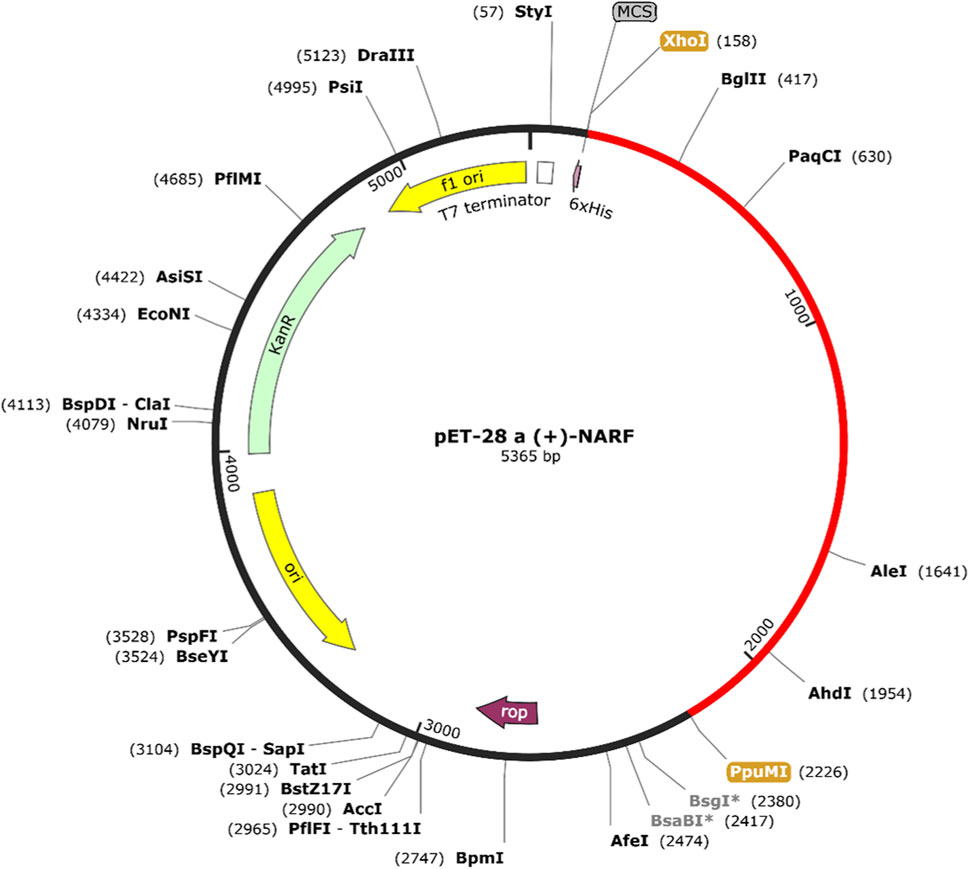
FIGURE 2. Codon optimized vaccine sequence was cloned in silico into the pET28a (+) expression system.
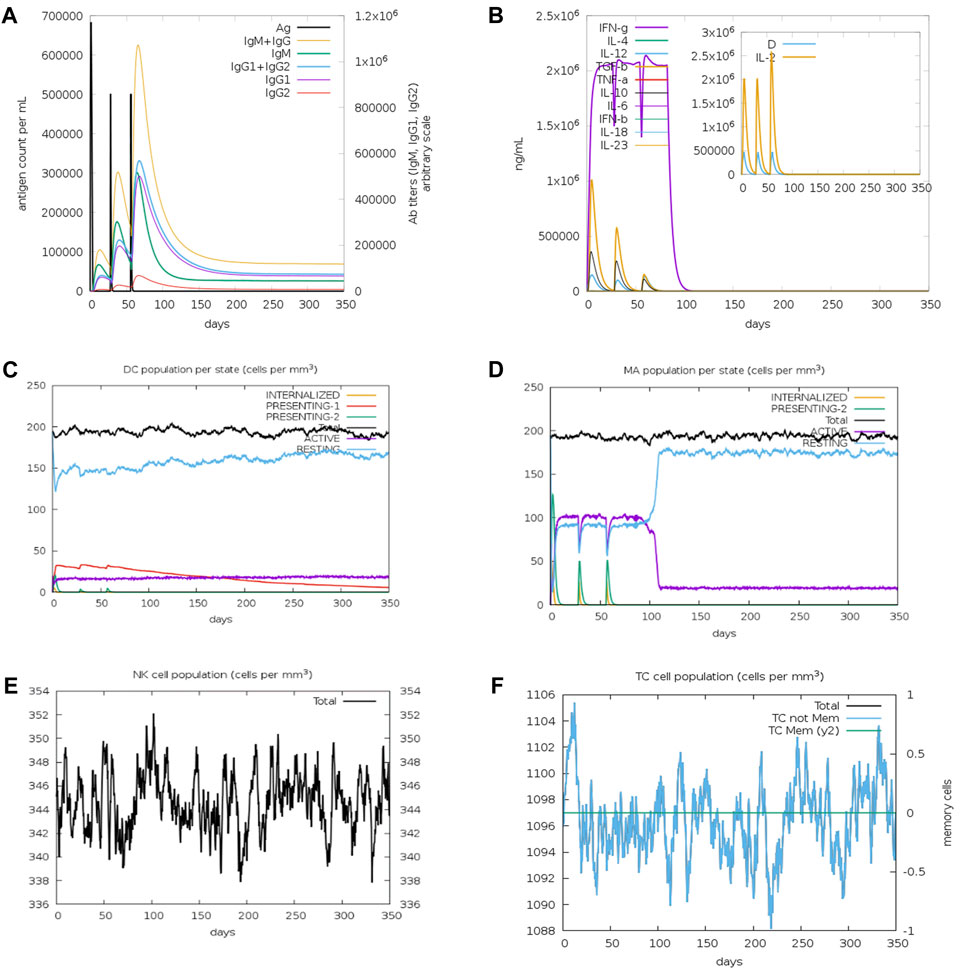
FIGURE 3. Immune simulation profiles of the vaccine construct (A) Immunoglobulin expression profile (B) Cytokines and interleukin expression profiles (C) Dendritic cells (D) Macrophage (E) NK cell and (F) cytotoxic T cell population expression profiles.
4 Discussion
The development of a highly effective and therapeutic vaccine targeting TNBC is indispensable due to the scarcity of viable treatment alternatives. Thus, the present study employed a wide range of immunoinformatic methods in a holistic manner to generate a chimeric subunit vaccine against TNBC. Initially, the epitope regions of NARF to stimulate CD4+ or CD8+ T-cell and B-cell immune responses were retrieved [53, 54]. The extracted epitopes were then evaluated for vaccine-like properties using a variety of descriptors including toxicity and antigenicity. Peptide-based vaccines often exhibit low immunogenicity even with appropriate T and B-cell epitopes [55]. Thus, the selected epitopes were combined with appropriate adjuvants and linkers to produce a robust vaccine construct [56]. Note that the AAY linker boosts immunogenicity, and the GPGPG universal spacer is essential for inducing HTL responses. Additionally, B-cell epitopes were connected using KK linkers to enhance the immunogenicity. While the EAAAK was used to fuse the adjuvant for efficient separation of functional domains.
The vaccine design comprising of 687 amino acids possessed a lower molecular weight of 71.89 kDa (<110 kDa) facilitating its easier purification. The vaccine’s preference for acidic surroundings and its suitability for the body’s polar environment are indicated by its pI of 5.15 and GRAVY score of −0.228, respectively. In addition, the instability index of 36.85 (<40) and aliphatic index of 81.02 implies that the vaccine is a stable protein with high thermostability. Furthermore, the higher solubility index of 0.885 suggests that the vaccine is able to dissolve easily in water and other solvents. The extended half-life of 30 h in mammalian reticulocytes (in vitro), >20 h in yeast (in vivo), and >10 h in E. coli (in vivo) ensures prolonged exposure of our vaccine to the immune system [57–59]. Also, the PI score from ElliPro closely correlates with solvent accessibility and has profound effects on the structure and functionality of biological macromolecules [60]. The presence of alpha-helices and coils in our vaccine construct is remarkable, as they are structural antigens recognized by antibodies [61]. Further, the Z-score of −9.88 for the modelled vaccine suggests that it is structurally analogous to experimentally resolved models of similar sizes, validating its near-native conformation. Importantly, the findings of the population coverage suggest that the selected epitopes could be generalized to the vast majority of the global population [62]. TLRs sense unique host molecular patterns and exhibit a crucial role in innate immunity. Notably, the research suggests that lowered expression of TLR-2, 4, 7, and 9 could promote cancer growth and immune evasion in TNBC [63–65]. It is worth noting that the designed vaccine exhibits greater affinity for TLR-4 and TLR-7 than other targets considered in our analysis. It is obvious from the results that the existence of high number of non-bonded interactions together with hydrogen bonds and salt-bridges facilitate the binding of vaccine with TLR protein. This firm binding will promote MHC presentation, which in turn activate subsequent T cell specific responses [66, 67].
The iMODS findings implied that all the complexes, except vaccine-TLR-2, were directed towards each other using affine-model arrows indicating functional roles and strong binding (Figures 4A, 5A, Supplementary Figure S3, Supplementary Figures S4A and S5A). The deformability peaks in Figures 4B, 5B, Supplementary Figures S4B and S5B indicate the regions of flexibility. Notably, a limited number of peaks in the TLR-4 and TLR-7 complexes imply rigid regions of the residues in the complex. The low B-factor values associated with the TLR-4 and TLR-7 complexes in Figures 4C, 5C, Supplementary Figures S4C and S5C highlight the minimal atomic displacements that contribute to the stability of these complexes. Figures 4D, 5D, Supplementary Figures S4D and S5D shows higher eigenvalues of 1.0942e−06, 1.2328e−06 in TLR-4 and TLR-7 complexes, indicating greater stability and antigen presentation. Subsequently, the mode-specific variance, represented in Figures 4E, 5E, Supplementary Figures S4E and S5E, implies their relative contribution to equilibrium motions. It is important to note that the first six normal modes accounted for almost 80% of the variance. Further, the covariance map in Figures 4F, 5F, Supplementary Figures S4F and S5F reveals the coupling between residue pairs in the complex, implying that all complexes are correlated. In addition, Figures 4G, 5G, Supplementary Figures S4G and S5G shows the elastic network analysis of the complexes, with darker grey dots indicating stiffer complexes.
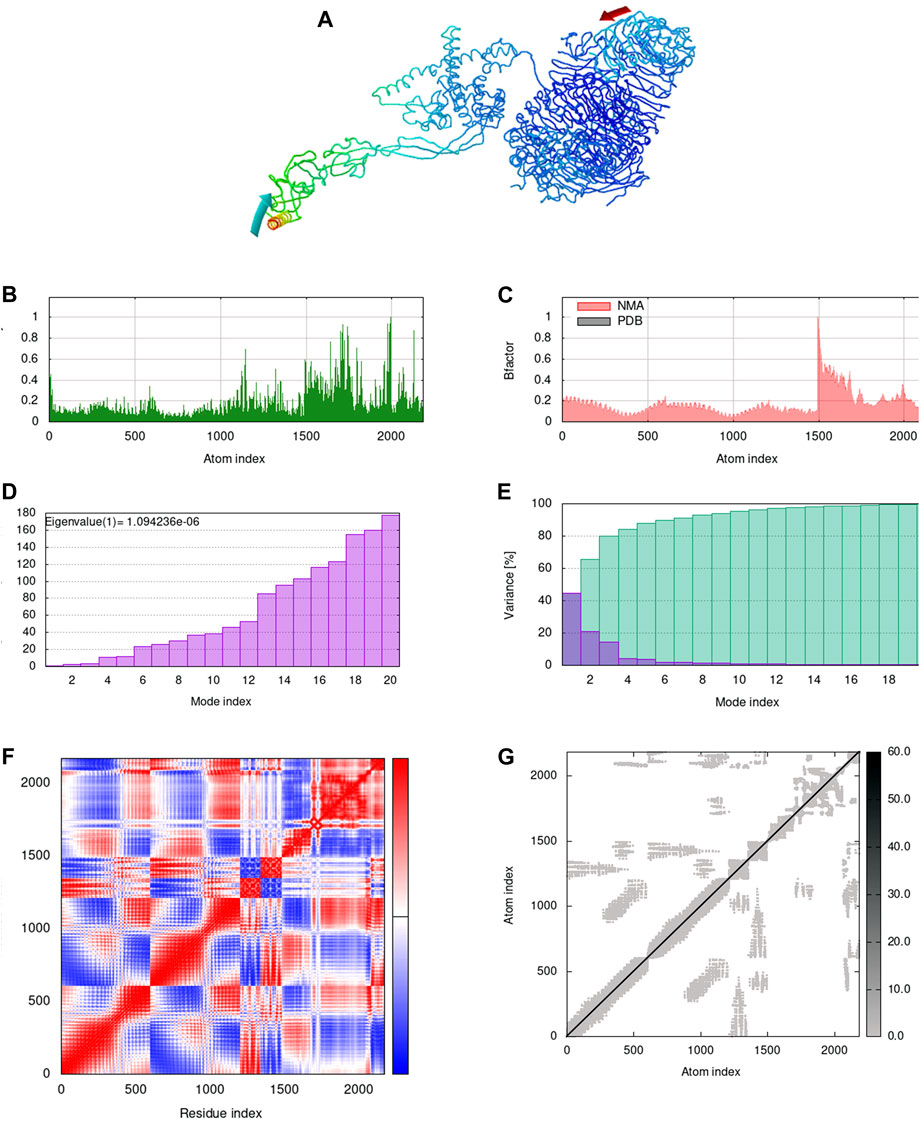
FIGURE 4. Normal mode analysis of docked complex. (A) NMA mobility of the vaccine-TLR-4 complex with affine-model arrows, (B) Deformability plot, (C) B-factor plot, (D) Eigenvalue plot, (E) Normal mode variance plot, (F) Covariance map and (G) Elastic network.
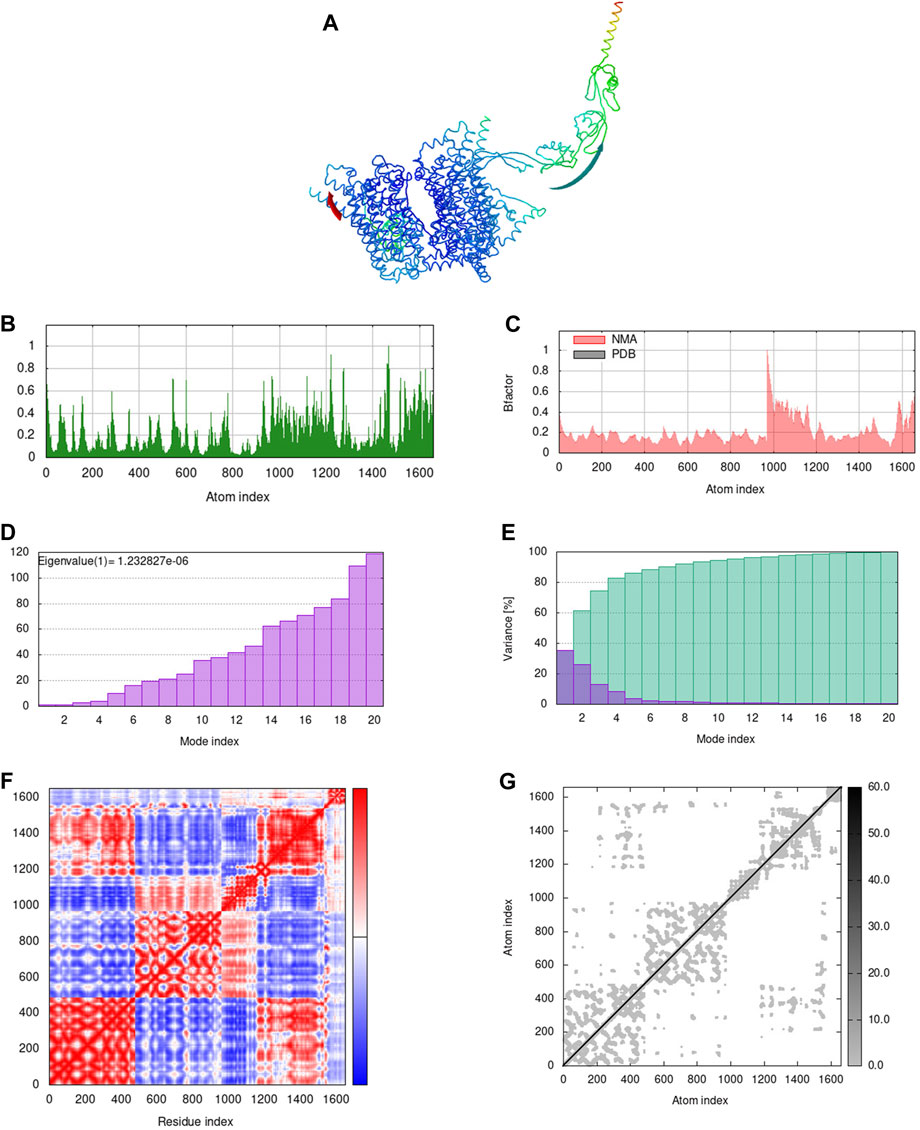
FIGURE 5. Normal mode analysis of docked complex. (A) NMA mobility of the vaccine-TLR-7 complex with affine-model arrows, (B) Deformability plot, (C) B-factor plot, (D) Eigenvalue plot, (E) Normal mode variance plot, (F) Covariance map and (G) Elastic network.
Taken together, TLR-4 and TLR-7 establish stable interactions when compared to other complexes. Evidence from the literature suggests that the firm association of the vaccine with these TLRs signifies a robust immune response, thereby preventing cancer cell evasion [68]. The results are also consistent with our earlier docking analysis. Further, the codon optimized vaccine sequence in E. coli yielded a favourable CAI and GC content values, indicating optimal expression and greater translational efficiency of the engineered vaccine [65, 69]. The overall magnitude of the immune response increased with each booster dose, indicating the strong immune response of our engineered vaccine.
It is worth noting that designing cancer vaccines involves high costs and a time-consuming process. Nevertheless, the benefits of computational (in silico) approaches have significantly reduced the costs and time by using biological modelling techniques. Before delving into laboratory-based experiments for cancer vaccine development, the immunoinformatics approach provides crucial insights into the interaction pattern between the immune system and cancer cells at molecular and cellular levels [70]. For instance, several studies have consistently reported the efficiency of in silico designed vaccine candidates in experimental assessments [13, 17].
Based on evidence from the literature, we hypothesise that our designed vaccine has better antigenic and immunogenic properties, along with better binding affinity for toll like receptors. For instance, the vaccine designed against SOX 9 transcription factor by Rajendran et al. had an antigenicity score of 0.59 and an instability index of 39.84, while our proposed vaccine exhibited an antigenicity score of 0.63 and an instability index of 36.85, demonstrating higher stability and immunogenic properties that evoke the immune response. Similarly, an MZF-1-based vaccine designed for TNBC by Rajendran et al. displayed a lowest immunogenicity score (3.720) and binding affinity score against TLR 2, 4, 7, and 9 (−929.5 kcal/mol, −1060.3 kcal/mol, −975.6 kcal/mol, and −1091.9 kcal/mol), whereas our designed vaccine had an immunogenicity score of 7.92 and binding scores of −1277.5 kcal/mol, −1,120 kcal/mol, −1808 kcal/mol, and −1,353 kcal/mol against TLR 2, 4, 7, and 9. These results imply that the developed vaccine would be efficient in eliciting strong immune responses. Given these considerations, our study on the NARF-based multi-epitope vaccine laid the groundwork for the effective treatment and management of triple-negative breast cancer. In future, the perspectives such as immunosuppressive cell depletion and immunotherapy-based combination therapies could be of important to improve the vaccine’s anti-tumor efficacy [71]. Indeed, in vitro and in vivo experimental validations are crucial to examine the safety and efficacy of the developed vaccine.
Data availability statement
The original contributions presented in the study are included in the article/Supplementary Material, further inquiries can be directed to the corresponding author.
Author contributions
PP: Writing–original draft. SV: Writing–review and editing.
Funding
The author(s) declare that no financial support was received for the research, authorship, and/or publication of this article.
Acknowledgments
The authors thank the management of Vellore Institute of Technology for providing the facilities to carry out this research work.
Conflict of interest
The authors declare that the research was conducted in the absence of any commercial or financial relationships that could be construed as a potential conflict of interest.
Publisher’s note
All claims expressed in this article are solely those of the authors and do not necessarily represent those of their affiliated organizations, or those of the publisher, the editors and the reviewers. Any product that may be evaluated in this article, or claim that may be made by its manufacturer, is not guaranteed or endorsed by the publisher.
Supplementary material
The Supplementary for this article can be found online at: https://www.frontiersin.org/articles/10.3389/fphy.2024.1342115/full#supplementary-material
References
1. Qiu D, Zhang G, Yan X, Xiao X, Ma X, Lin S, et al. Prospects of immunotherapy for triple-negative breast cancer. Front Oncol (2022) 11:797092. doi:10.3389/fonc.2021.797092
2. Dent R, Trudeau M, Pritchard KI, Hanna WM, Kahn HK, Sawka CA, et al. Triple-negative breast cancer: clinical features and patterns of recurrence. Clin Cancer Res (2007) 13:4429–34. doi:10.1158/1078-0432.CCR-06-3045
3. Liu Z, Li M, Jiang Z, Wang X. A comprehensive immunologic portrait of triple-negative breast cancer. Transl Oncol (2018) 11:311–29. doi:10.1016/j.tranon.2018.01.011
4. Corti C, Giachetti PPMB, Eggermont AMM, Delaloge S, Curigliano G. Therapeutic vaccines for breast cancer: has the time finally come? Eur J Cancer (2022) 160:150–74. doi:10.1016/j.ejca.2021.10.027
5. Yang L, Ning Q, Tang S. Recent advances and next breakthrough in immunotherapy for cancer treatment. J Immunol Res (2022) 2022:1–9. doi:10.1155/2022/8052212
6. Atapour A, Negahdaripour M, Ghasemi Y, Razmjuee D, Savardashtaki A, Mousavi SM, et al. In silico designing a candidate vaccine against breast cancer. Int J Pept Res Ther (2020) 26:369–80. doi:10.1007/s10989-019-09843-1
7. Abdou Y, Goudarzi A, Yu JX, Upadhaya S, Vincent B, Carey LA. Immunotherapy in triple negative breast cancer: beyond checkpoint inhibitors. NPJ Breast Cancer (2022) 8:121. doi:10.1038/s41523-022-00486-y
8. Nicolás-Morales ML, Luisa-Sanjuan A, Gutiérrez-Torres M, Vences-Velázquez A, Ortuño-Pineda C, Espinoza-Rojo M, et al. Peptide-Based vaccines in clinical phases and new potential therapeutic targets as a new approach for breast cancer: a review. Vaccines (2022) 10:1249. doi:10.3390/vaccines10081249
9. Hosseini M, Seyedpour S, Khodaei B, Loghman A-H, Seyedpour N, Yazdi M-H, et al. Cancer vaccines for triple-negative breast cancer: a systematic review. Vaccines (2023) 11:146. doi:10.3390/vaccines11010146
10. Li L, Duns GJ, Dessie W, Cao Z, Ji X, Luo X. Recent advances in peptide-based therapeutic strategies for breast cancer treatment. Front Pharmacol (2023) 14:1052301. doi:10.3389/fphar.2023.1052301
11. Rajendran Krishnamoorthy H, Karuppasamy R. Designing a novel SOX9 based multi-epitope vaccine to combat metastatic triple-negative breast cancer using immunoinformatics approach. Mol Divers (2023) 27:1829–42. doi:10.1007/s11030-022-10539-w
12. Krishnamoorthy HR, Karuppasamy R. Design and in silico validation of a novel MZF-1-based multi-epitope vaccine to combat metastatic triple negative breast cancer. Vaccines (2023) 11:577. doi:10.3390/vaccines11030577
13. Chen T, Liu K, Xu J, Zhan T, Liu M, Li L, et al. Synthetic and immunological studies on the OCT4 immunodominant motif antigen-based anti-cancer vaccine. Cancer Biol Med (2020) 17:132–41. doi:10.20892/j.issn.2095-3941.2019.0224
14. Mahdevar E, Safavi A, Abiri A, Kefayat A, Hejazi SH, Miresmaeili SM, et al. Exploring the cancer-testis antigen BORIS to design a novel multi-epitope vaccine against breast cancer based on immunoinformatics approaches. J Biomol Struct Dyn (2022) 40:6363–80. doi:10.1080/07391102.2021.1883111
15. Paranthaman P, Veerappapillai S. Design of a potential Sema4A-based multi-epitope vaccine to combat triple-negative breast cancer: an immunoinformatic approach. Med Oncol (2023) 40:105. doi:10.1007/s12032-023-01970-6
16. Mahmoodi S, Nezafat N, Negahdaripour M, Ghasemi Y. A new approach for cancer immunotherapy based on the cancer stem cell antigens properties. Curr Mol Med (2019) 19:2–11. doi:10.2174/1566524019666190204114721
17. Cheng P, Jiang F, Wang G, Wang J, Xue Y, Wang L, et al. Bioinformatics analysis and consistency verification of a novel tuberculosis vaccine candidate HP13138PB. Front Immunol (2023) 14:1102578. doi:10.3389/fimmu.2023.1102578
18. Cecil DL, Slota M, O’Meara MM, Curtis BC, Gad E, Dang Y, et al. Immunization against HIF-1α inhibits the growth of basal mammary tumors and targets mammary stem cells in vivo. Clin Cancer Res (2017) 23:3396–404. doi:10.1158/1078-0432.CCR-16-1678
19. Liu Q, Guan C, Liu C, Li H, Wu J, Sun C. Targeting hypoxia-inducible factor-1alpha: a new strategy for triple-negative breast cancer therapy. Biomed Pharmacother (2022) 156:113861. doi:10.1016/j.biopha.2022.113861
20. Bairoch A, Apweiler R, Wu CH, Barker WC, Boeckmann B, Ferro S, et al. The universal protein resource (UniProt). Nucleic Acids Res (2005) 33:D154–9. doi:10.1093/nar/gki070
21. Berman H, Henrick K, Nakamura H. Announcing the worldwide protein data bank. Nat Struct Mol Biol (2003) 10:980. doi:10.1038/nsb1203-980
22. Reynisson B, Alvarez B, Paul S, Peters B, Nielsen M. NetMHCpan-4.1 and NetMHCIIpan-4.0: improved predictions of MHC antigen presentation by concurrent motif deconvolution and integration of MS MHC eluted ligand data. Nucleic Acids Res (2020) 48:W449–54. doi:10.1093/nar/gkaa379
23. Saha S, Raghava GPS. Prediction methods for B-cell epitopes. Methods Mol Biol (2007) 409:387–94. doi:10.1007/978-1-60327-118-9_29
24. Ponomarenko J, Bui HH, Li W, Fusseder N, Bourne PE, Sette A, et al. ElliPro: a new structure-based tool for the prediction of antibody epitopes. BMC Bioinformatics (2008) 9:514–8. doi:10.1186/1471-2105-9-514
25. Doytchinova I, Flower DR. Bioinformatic approach for identifying parasite and fungal candidate subunit vaccines. Open Vaccin J (2008) 1:22–6. doi:10.2174/1875035400801010022
26. Dimitrov I, Bangov I, Flower DR, Doytchinova I. AllerTOP v.2—a server for in silico prediction of allergens. J Mol Model (2014) 20:2278. doi:10.1007/s00894-014-2278-5
27. Calis JJA, Maybeno M, Greenbaum JA, Weiskopf D, De Silva AD, Sette A, et al. Properties of MHC class I presented peptides that enhance immunogenicity. Plos Comput Biol (2013) 9:e1003266. doi:10.1371/journal.pcbi.1003266
28. Gupta S, Kapoor P, Chaudhary K, Gautam A, Kumar R, Raghava GPS. In silico approach for predicting toxicity of peptides and proteins. PLoS One (2013) 8:e73957. doi:10.1371/journal.pone.0073957
29. Dhanda SK, Vir P, Raghava GPS. Designing of interferon-gamma inducing MHC class-II binders. Biol Direct (2013) 8:30–15. doi:10.1186/1745-6150-8-30
30. Livingston B, Crimi C, Newman M, Higashimoto Y, Appella E, Sidney J, et al. A rational strategy to design multiepitope immunogens based on multiple Th lymphocyte epitopes. J Immunol (2002) 168:5499–506. doi:10.4049/jimmunol.168.11.5499
31. Ayyagari VS, Venkateswarulu TC, Abraham Peele K, Srirama K. Design of a multi-epitope-based vaccine targeting M-protein of SARS-CoV2: an immunoinformatics approach. J Biomol Struct Dyn (2022) 40:2963–77. doi:10.1080/07391102.2020.1850357
32. Li X, Guo L, Kong M, Su X, Yang D, Zou M, et al. Design and evaluation of a multi-epitope peptide of human metapneumovirus. Intervirology (2016) 58:403–12. doi:10.1159/000445059
33. Gasteiger E, Hoogland C, Gattiker A, Duvaud S, Wilkins MR, Appel RD, et al. Protein identification and analysis tools on the ExPASy server. Springer (2005). doi:10.1385/1-59259-890-0:571
34. Magnan CN, Randall A, Baldi P. SOLpro: accurate sequence-based prediction of protein solubility. Bioinformatics (2009) 25:2200–7. doi:10.1093/bioinformatics/btp386
35. Jones DT. Protein secondary structure prediction based on position-specific scoring matrices 1 1Edited by G. Von Heijne. J Mol Biol (1999) 292:195–202. doi:10.1006/jmbi.1999.3091
36. Kim DE, Chivian D, Baker D. Protein structure prediction and analysis using the Robetta server. Nucleic Acids Res (2004) 32:W526–31. doi:10.1093/nar/gkh468
37. Lee GR, Heo L, Seok C. Effective protein model structure refinement by loop modeling and overall relaxation. Proteins Struct Funct Bioinforma (2016) 84:293–301. doi:10.1002/prot.24858
38. Laskowski RA, MacArthur MW, Moss DS, Thornton JM. PROCHECK: a program to check the stereochemical quality of protein structures. J Appl Crystallogr (1993) 26:283–91. doi:10.1107/S0021889892009944
39. Eisenberg D, Lüthy R, Bowie JU. VERIFY3D: assessment of protein models with three-dimensional profiles. Methods Enzymol (1997) 277:396–404. doi:10.1016/S0076-6879(97)77022-8
40. Colovos C, Yeates TO. Verification of protein structures: patterns of nonbonded atomic interactions. Protein Sci (1993) 2:1511–9. doi:10.1002/pro.5560020916
41. Wiederstein M, Sippl MJ. ProSA-web: interactive web service for the recognition of errors in three-dimensional structures of proteins. Nucleic Acids Res (2007) 35:W407–10. doi:10.1093/nar/gkm290
42. Kozakov D, Hall DR, Xia B, Porter KA, Padhorny D, Yueh C, et al. The ClusPro web server for protein–protein docking. Nat Protoc (2017) 12:255–78. doi:10.1038/nprot.2016.169
43. Laskowski RA, Jabłońska J, Pravda L, Vařeková RS, Thornton JM. PDBsum: structural summaries of PDB entries. Protein Sci (2018) 27:129–34. doi:10.1002/pro.3289
44. Kmiecik S, Kouza M, Badaczewska-Dawid AE, Kloczkowski A, Kolinski A. Modeling of protein structural flexibility and large-scale dynamics: coarse-grained simulations and elastic network models. Int J Mol Sci (2018) 19(11):3496. doi:10.3390/ijms19113496
45. Bauer JA, Pavlović J, Bauerová-Hlinková V. Normal mode analysis as a routine part of a structural investigation. Molecules (2019) 24(18):3293. doi:10.3390/molecules24183293
46. Orellana L. Large-scale conformational changes and protein function: breaking the in silico barrier. Front Mol biosciences (2019) 6:117. doi:10.3389/fmolb.2019.00117
47. López-Blanco JR, Aliaga JI, Quintana-Ortí ES, Chacón P. iMODS: internal coordinates normal mode analysis server. Nucleic Acids Res (2014) 42:W271–6. doi:10.1093/nar/gku339
48. Bui H-H, Sidney J, Dinh K, Southwood S, Newman MJ, Sette A. Predicting population coverage of T-cell epitope-based diagnostics and vaccines. BMC Bioinformatics (2006) 7:153–5. doi:10.1186/1471-2105-7-153
49. Rapin N, Lund O, Bernaschi M, Castiglione F. Computational immunology meets bioinformatics: the use of prediction tools for molecular binding in the simulation of the immune system. PLoS One (2010) 5:e9862. doi:10.1371/journal.pone.0009862
50. Grote A, Hiller K, Scheer M, Münch R, Nörtemann B, Hempel DC, et al. JCat: a novel tool to adapt codon usage of a target gene to its potential expression host. Nucleic Acids Res (2005) 33:W526–31. doi:10.1093/nar/gki376
51. Al-Muhanna S, Al-Muhanna A. Construction and transformation of recombinant pet28a expression vector in bl21 (de3) cells with basic bioinformatics analysis. Biochem Cel Arch (2018) 18:147–52.
52. Moin AT, Ullah MA, Patil RB, Faruqui NA, Araf Y, Das S, et al. A computational approach to design a polyvalent vaccine against human respiratory syncytial virus. Scientific Rep (2023) 13(1):9702–20. doi:10.1038/s41598-023-35309-y
53. Martinelli DD. In silico vaccine design: a tutorial in immunoinformatics. Healthc Anal (2022) 2:100044. doi:10.1016/j.health.2022.100044
54. Dey J, Mahapatra SR, Singh P, Patro S, Kushwaha GS, Misra N, et al. B and T cell epitope-based peptides predicted from clumping factor protein of Staphylococcus aureus as vaccine targets. Microb Pathog (2021) 160:105171. doi:10.1016/j.micpath.2021.105171
55. Li W, Joshi MD, Singhania S, Ramsey KH, Murthy AK. Peptide vaccine: progress and challenges. Vaccines (2014) 2:515–36. doi:10.3390/vaccines2030515
56. Sanami S, Azadegan-Dehkordi F, Rafieian-Kopaei M, Salehi M, Ghasemi-Dehnoo M, Mahooti M, et al. Design of a multi-epitope vaccine against cervical cancer using immunoinformatics approaches. Sci Rep (2021) 11:12397. doi:10.1038/s41598-021-91997-4
57. Bahrami AA, Payandeh Z, Khalili S, Zakeri A, Bandehpour M. Immunoinformatics: in silico approaches and computational design of a multi-epitope, immunogenic protein. Int Rev Immunol (2019) 38:307–22. doi:10.1080/08830185.2019.1657426
58. Tobias JW, Shrader TE, Rocap G, Varshavsky A. The N-end rule in bacteria. Science (1991) 254:1374–7. doi:10.1126/science.1962196
59. Bachmair A, Finley D, Varshavsky A. In vivo half-life of a protein is a function of its amino-terminal residue. Science (1986) 234:179–86. doi:10.1126/science.3018930
60. Yazdani Z, Rafiei A, Yazdani M, Valadan R. Design an efficient multi-epitope peptide vaccine candidate against SARS-CoV-2: an in silico analysis. Infect Drug Resist (2020) 13:3007–22. doi:10.2147/IDR.S264573
61. Oluwagbemi OO, Oladipo EK, Dairo EO, Ayeni AE, Irewolede BA, Jimah EM, et al. Computational construction of a glycoprotein multi-epitope subunit vaccine candidate for old and new South-African SARS-CoV-2 virus strains. Inform Med Unlocked (2022) 28:100845. doi:10.1016/j.imu.2022.100845
62. Bhattacharya M, Chatterjee S, Nag S, Dhama K, Chakraborty C. Designing, characterization, and immune stimulation of a novel multi-epitopic peptide-based potential vaccine candidate against monkeypox virus through screening its whole genome encoded proteins: an immunoinformatics approach. Trav Med Infect Dis (2022) 50:102481. doi:10.1016/j.tmaid.2022.102481
63. Luchner M, Reinke S, Milicic A. TLR agonists as vaccine adjuvants targeting cancer and infectious diseases. Pharmaceutics (2021) 13:142. doi:10.3390/pharmaceutics13020142
64. Shi S, Xu C, Fang X, Zhang Y, Li H, Wen W, et al. Expression profile of Toll-like receptors in human breast cancer. Mol Med Rep (2020) 21:786–94. doi:10.3892/mmr.2019.10853
65. Kumar S, Shuaib M, Prajapati KS, Singh AK, Choudhary P, Singh S, et al. A candidate triple-negative breast cancer vaccine design by targeting clinically relevant cell surface markers: an integrated immuno and bio-informatics approach. 3 Biotech (2022) 12:72. doi:10.1007/s13205-022-03140-3
66. Jin B, Sun T, Yu X-H, Yang Y-X, Yeo AET. The effects of TLR activation on T-cell development and differentiation. Clin Dev Immunol (2012) 2012:1–32. doi:10.1155/2012/836485
67. Suleman M, Yousafi Q, Ali J, Ali SS, Hussain Z, Ali S, et al. Bioinformatics analysis of the differences in the binding profile of the wild-type and mutants of the SARS-CoV-2 spike protein variants with the ACE2 receptor. Comput Biol Med (2021) 138:104936. doi:10.1016/j.compbiomed.2021.104936
68. Ali M, Pandey RK, Khatoon N, Narula A, Mishra A, Prajapati VK. Exploring dengue genome to construct a multi-epitope based subunit vaccine by utilizing immunoinformatics approach to battle against dengue infection. Sci Rep (2017) 7:9232. doi:10.1038/s41598-017-09199-w
69. Morla S, Makhija A, Kumar S. Synonymous codon usage pattern in glycoprotein gene of rabies virus. Gene (2016) 584:1–6. doi:10.1016/j.gene.2016.02.047
70. Parvizpour S, Razmara J, Pourseif MM, Omidi Y. In silico design of a triple-negative breast cancer vaccine by targeting cancer testis antigens. BioImpacts (2019) 9(1):45–56. doi:10.15171/bi.2019.06
Keywords: nuclear prelamin a recognition factor, cancers, next-generation vaccine, immunotherapy, docking, dynamics simulations, in silico cloning
Citation: Paranthaman P and Veerappapillai S (2024) Tackling suppressive cancer microenvironment by NARF-derived immune modulatory vaccine and its validation using simulation strategies. Front. Phys. 12:1342115. doi: 10.3389/fphy.2024.1342115
Received: 21 November 2023; Accepted: 08 February 2024;
Published: 22 February 2024.
Edited by:
Jayakanthan Mannu, Tamil Nadu Agricultural University, IndiaReviewed by:
Luis Del Pozo-Yauner, University of South Alabama, United StatesJamoliddin Razzokov, Tashkent Institute of Irrigation and Agricultural Mechanization Engineers (TIIAME), Uzbekistan
Copyright © 2024 Paranthaman and Veerappapillai. This is an open-access article distributed under the terms of the Creative Commons Attribution License (CC BY). The use, distribution or reproduction in other forums is permitted, provided the original author(s) and the copyright owner(s) are credited and that the original publication in this journal is cited, in accordance with accepted academic practice. No use, distribution or reproduction is permitted which does not comply with these terms.
*Correspondence: Shanthi Veerappapillai, shanthi.v@vit.ac.in