- Department of Biotechnology, School of Bio Sciences and Technology, Vellore Institute of Technology, Vellore, India
Somatic transformations in the key catalytic residues of the Isocitrate Dehydrogenase (IDH) enzyme assist in the onset of distinct malignancies including glioma. Currently, enasidenib is the FDA-approved drug used to target IDH2 protein. However, the use of enasidenib as a plausible mIDH2 inhibitor is constrained by poor brain penetrating capability and dose-limiting toxicity. Thus, the present study aimed to explore the potential of nutraceuticals to synergistically elevate the efficacy of the existing drugs available for glioma management. The binding affinity and free energy of the nutraceuticals were evaluated using molecular docking and MM-GBSA analysis. The resultant 14 compounds were subjected to machine learning-based rescoring strategies to distinguish binders from nonbinders. The pharmacokinetic and toxicity analysis was also implemented alongside virtual cell line assay. The results of our study identified DB14002 (D-alpha-Tocopherol acetate, analog of Vitamin E) as the potential hit compound with appreciable binding affinity, brain penetrating capability and antineoplastic activity against glioma cell lines. In the end, the conformational stability and dynamic characteristics of DB14002 were examined for a stipulated time frame of 250ns. Indeed, the outcomes of our study culminate the use of DB14002 as a synergistic drug-like candidate which could be translated as a plausible inhibitor of mIDH2 in the forthcoming years.
1 Introduction
Gliomas are the most prevalent adult-onset primary malignant brain tumor, which has an average yearly incidence of 5 cases per 100,000 people [1, 2]. The metabolic reprogramming in glial cells has been perceived as an important hallmark for the onset of glioma progression [3]. Notably, the mutations in the key metabolic pathway enzymes are the primary cause of abnormal metabolism [4]. Further, the accumulation of tumor metabolites imposed by genetic changes in metabolic genes lends more evidence to the significant development and survival of cancer cells [5].
Isocitrate Dehydrogenase (IDH) are the crucial rate-limiting enzymes involved in the tricarboxylic acid cycle which mediate the conversion of isocitrate to α-ketoglutarate (α-KG) through oxidative decarboxylation. It is worth mentioning that IDH also plays a part in a few cellular functions, such as glucose sensing, lipogenesis, glutamine metabolism, mitochondrial oxidative phosphorylation, and control of cellular redox status [6–8]. Recently, mutations in the genes of IDH enzymes were observed in various malignant tumors including gliomas, acute myeloid leukemia (AML), cholangiocarcinoma, chondrosarcoma, pancreatic, colon and prostate cancer to varying extents. The IDH mutations cause the neomorphic enzyme to transform α-KG into the oncometabolite D-2-hydroxyglutarate, which has significant effects on cellular epigenetics and metabolism. Intriguingly, IDH mutations were reported in >80% of WHO grade II and III patients and 73% of grade IV (secondary glioblastoma) cases [9]. Thus, IDH1 and IDH2 gene mutations are important biomarkers with diagnostic, prognostic, and predictive value as they constitute the earliest driver mutations occurring during gliomagenesis and a reliable molecular signature of low-grade gliomas.
The mutations in the IDH2 gene were observed to exhibit few discrete biochemical properties. Firstly, the mutations in the IDH gene are largely somatic and heterozygous. Further, all the mutations occur due to substitution with a single amino acid residue. For instance, the commonly identified mutation in IDH2 is at R140 or R172 residue. These residues are most likely to be substituted with either Q or W for R140 and one of the four amino acids such as K, G, W and M for R172 respectively [10]. Importantly, these point mutations restructure numerous important residues in the active site of IDH. As a result, the affinity for isocitrate and NADP decreases and the affinity for α-KG and NADPH increases, resulting in the formation of the new metabolite D-2HG, which further promotes oncogenesis.
Several investigations have been conducted using in vitro and in silico methods to identify potential inhibitors against the mIDH2 protein. One of the earliest known mIDH2 inhibitors that target acute myeloid leukemia (AML) cells with R140Q mutations is AGI-6780 [11]. However, the in vitro experiments of this study reported a decrease in the activity of both intracellular and extracellular 2-HG levels due to its poor target selectivity. Recently, Enasidenib (AG-221) was also discovered to be a potent and highly targeted inhibitor of IDH2 (R140Q), with an IC50 value of 100 nM. The U.S. Food and Drug Administration (FDA) subsequently approved AG-221 as a potential inhibitor against relapsed or refractory AML with an IDH2 mutation in 2017 [12]. Despite its effective inhibition, some patients in phase trials experienced unfavorable side effects [13]. In addition, evidence from the literature suggests that secondary mutations (Q316E and I319M) in the allosteric site of the mIDH2 protein cause therapeutic resistance against enasidenib in AML patients [14–16]. Despite significant progress in the molecular characterization of gliomas, clinicians still have difficulty due to the limited benefits of standard-of-care options for survival along with the risk of long-term toxicities, including cognitive decline. Recently, to subdue these challenges, the application of nutraceutical interventions was implemented with an aim to develop and design precision medicine strategies that synergistically act with the existing anti-cancer agents and minimize the burden of chemotherapy-related toxicities [17, 18]. For instance, a study on tea polyphenols was reported to modulate various molecular signatures in prostate cancer patients and thereby aid in elevating the effectiveness of the treatment modality [19]. The use of fucoidan (polysaccharide derived from seaweed) in human clinical trials, and animal models, as complementary therapy has been reported to minimize the side effects of anti-cancer chemotherapy and increase the treatment efficacy [20]. Thus, in the present study, an attempt has been made to exploit the nutraceutical subset of the DrugBank database against mIDH2 protein to discover mutation-specific inhibitors to efficiently manage gliomagenesis.
2 Methodology
2.1 Dataset preparation
In the first instance, the crystal coordinates of the mIDH2 protein (PDB code 6VFZ) were extracted from the Protein data bank repository [21]. Further, the small molecule library was created by retrieving the nutraceutical subset (135 compounds) from the DrugBank database. Of note, it is critical to ensure the quality of the structural data to avoid inaccurate predictions. Thus, the “Protein Preparation Wizard”, and “LigPrep” modules of the Schrödinger interface were utilized to perform structural refinement analysis [22]. At the time of preprocessing, the structures of the protein and ligand molecules were verified for chemical correctness by eliminating the bad molecules, unclear bond-order assignments and recognizing atomic clashes, and ambiguous stereo assignments. The pre-processed structures were minimized using the OPLS3e force field [23].
2.2 Molecular docking
On the forehand of molecular docking, the grid parameters were set around the active site of the target protein with the aid of the “Receptor grid generation” wizard of the Schrödinger suite. The catalytic site of the mIDH2 protein comprises the following residues, namely, V161, W164, V294, V297, L298, W306, E316, I319, L320 and G323 [24, 25]. Subsequently, the pre-processed ligand molecules from the nutraceutical subset of the DrugBank database were subjected to an extra precision (XP) docking process. The advantage of XP docking is that it uses a unique and sophisticated scoring function to identify the key structural moiety required for target binding [26]. In order to screen the hit molecules, the compounds with the lowest XP GScore were identified. The score corresponding to enasidenib was set as a threshold throughout our analyses [27].
2.3 Binding energy analysis
The pose with the lowest Glide score for each ligand was rescored using the Prime/MM-GBSA method to predict the binding free energy of a group of ligands to a receptor. The energies of the complex were determined using the OPLS3e force field and generalized-born surface area (GBSA) continuum solvent model, and the docked poses were minimized using Prime’s local optimization feature [28]. In addition to the binding free energy, the interaction energy components of the ligands that contribute to the protein binding were also validated. The following equation is used to calculate the binding free energy (ΔGbind) of the ligand in the active site of the mIDH2 protein.
Where ΔEMM signifies the energy variation in the complex of protein and ligand and the energy summation of ligand-bound and unbounded mIDH2 protein, ΔGsolv denotes the energy variance in the protein-ligand complex’s solvation energy and the energy summation of ligand-bound and unbounded protein, ΔGSA denotes the energy variance and total energy of the surface area of the ligand-bound and unbounded mIDH2 protein.
2.4 Machine learning-based scoring function analysis
Machine learning-based scoring functions (ML-SF) have been extensively employed in recent years to investigate the binding mechanism of small molecules [29]. Indeed, numerous literature evidence has emphasized the extent to which machine-learning SFs have outperformed traditional SFs at predicting binding affinity [30–32]. For instance, a retrospective study by Li et al., demonstrated that ML-based scoring functions outperformed classical SF such as ChemScore, GoldScore and Glide when tested against HIV infection [33]. Another study by Durrant et al., highlighted that neural network-based SF had outperformed AutoDock and Glide docking [34]. Notably, the BINANA algorithm employed in NN delves into the intricate interactions between proteins and ligands, considering 12 distinct characteristics including hydrophobic contacts and active-site flexibility. This comprehensive analysis increases the accuracy of the NNScoring function. Similarly, Gnina, GLM-Score and X-Score showed satisfactory accuracy in recognizing effective antagonists against Nipah virus, Psoriasis, and non-small cell lung cancer [35–37]. Therefore, in the present study, a few ML-based scoring functions such as RF-Score-VS, GNINA, KDEEP, NNScore, SF-CNN and X-Score were adopted to revalidate the mechanism of binding of small molecules.
2.5 In-silico drug-likeliness prediction
The growing body of research shows that deviations from Absorption, Distribution, Metabolism, Excretion (ADME) and toxicity properties were primarily accountable for the rising failure rate of screened molecules in clinical trials [38]. As an effort to assess the drug-likeness of the screened molecules, the QikProp module of the Maestro interface was implemented. Here, descriptors like stars, CNS, Human Oral Absorption (HOA) and blood-brain barrier (logBB) were considered. Further, the Protox-II algorithm was used to evaluate the potential acute toxic endpoints of the screened lead molecules. The server assesses a range of toxicological endpoints, including organ toxicity, oral toxicity, and toxicity targets [39]. In addition, the biological activity of the screened molecules was quantified using Pa and Pi values obtained from the PASS prediction server. This software forecasts the pharmacological effect and biological activity spectrum of the lead compounds based on the chemical structure of the respective compounds [40]. Thus, the employed in silico prediction platform is believed to improve the process of hit selection and optimization and also provide additional insights into the mechanism of toxicity [41].
2.6 Deep learning-based bioactivity prediction
The anticancer drug sensitivity of the reference and the hit molecules was evaluated using the Paccmann tool. It is a multimodal deep learning framework that integrates three primary sources of data such as 1) the structure of the compound in the form of a SMILES sequence, 2) the gene expression profiles (GEP) of tumors, and 3) previous knowledge of intracellular interactions from protein-protein interaction networks to anticipate drug sensitivity determined by IC50 [42]. Shreds of literature evidence highlight that the model performance was found to surpass state-of-the-art results in anticancer drug sensitivity prediction [43]. In addition, the web service can be used to repurpose drugs and offer insightful information about the mechanism of action of the drug molecules.
2.7 Molecular dynamics simulation
The internal motions of proteins hold the key to unlock many of their mysterious biological functions and profound dynamic mechanisms. The molecular dynamics simulation was utilized to understand the flexibility and dynamic characteristics of the macromolecules at the molecular level [44, 45]. Thus, in the present study, MD simulations were performed for the mIDH2-enasidenib and mIDH2-hit complex using the GROMACS version 2020.2. The docked ligands were procured and used for ligand topology generation using CHARMM General Force Field (CGenFF). Similarly, the topology of the protein was generated by employing CHARMM36 forcefield [46]. Subsequently, the systems were enclosed within a dodecahedron box solvated with a Simple Point Charge (SPC) water model [47]. Counter-ions were introduced to ensure an overall neutral charge by replacing the water molecules in the system. The steepest descent algorithm-based energy minimization was first performed for 50,000 cycles to eliminate the steric clash. The system was further minimized and equilibrated into the NVT and NPT steps for 1000 ps. The system was then kept at 300 K and 1 bar using V-rescale, a modified Parrinello-Rahman pressure coupling method and a modified Berendsen thermostat temperature coupling method, respectively [48]. Eventually, the equilibrated systems were subjected to a production run of 250 ns at 300 K and 1 bar pressure.
3 Results
3.1 Molecular docking
Molecular docking studies were performed to inspect the plausible binding modes and the affinity of the ligand molecules within the catalytic cavity of the mIDH2 protein [49]. In the current investigation, a total of 135 nutraceutical compounds retrieved from the DrugBank database were subjected to XP docking by employing the Glide platform of the Schrödinger interface. Initially, the ligand library was prepared, energy minimized and subjected to molecular docking [50]. It is to be noted that only 84 compounds were able to bind with the target protein. The result is shown in Supplementary Table S1. In our analysis, the FDA-approved mIDH2 inhibitor, enasidenib was used as the reference compound throughout the virtual screening process. For instance, the XP GScore of the reference (−6.03 kcal/mol) was set as a cutoff to scrutinize lead molecules. The XP GScore of the docked compounds was observed to be in the range of −7.08 kcal/mol to −1.82 kcal/mol, representing their favorable binding characteristics against the target protein. The remaining compounds in our study did not bind either due to structural defects or optimization issues. Of note, a total of 6 compounds in our docking study exhibit better binding affinity with the lowest XP GScore ≤ −6.03 kcal/mol. Further, all these compounds along with the reference were submitted for binding free energy calculation.
3.2 MM-GBSA binding energy calculation
Molecular docking uncovers the binding interactions and systematic pre-screening of chemical compounds based on shape and energetic compatibility with target proteins. However, docking algorithms cannot reliably predict the binding energy of the ligand molecules, so post-docking analyses are necessary to avoid false positive results. Here, MM-GBSA from the Prime module of the Schrödinger suite is used to accurately predict the binding energy of a protein-ligand complex [51]. The post-docked optimized poses of ligand molecules complexed with mIDH2 protein showed binding energy that varies from −50.88 kcal/mol to −8.28 kcal/mol, as depicted in Table 1. As a result, a total of 14 compounds exhibited better binding free energy than enasidenib with the binding free energy less than −38.54 kcal/mol. Amongst 14 compounds with the lowest free energy, DB14002, DB00118 and DB06750 were stabilized predominantly with the aid of electrostatic energy, lipophilicity, solvation energy and van der Waals energy. In contrast, covalent, lipophilicity and solvation energy were found to be the major stabilizing forces for DB00755, DB01436 and DB06750. A similar pattern of predominance was observed in other hits such as DB14001, DB01892, DB00132, DB00154, DB00163, DB08887, DB00162 and DB00169.
3.3 Machine learning-based rescoring analysis
Machine-learning scoring functions trained on protein-ligand complexes have recently shown remarkable potential in virtual screening studies [32, 52, 53]. Therefore, in the current study, six ML-based scoring functions, namely, RF-Score-VS, GNINA, KDEEP, NNScore, SF-CNN and X-Score were used to revalidate the binding potential of the 14 lead compounds. Ligands with lower NNScores compared to the reference are predicted to bind the target protein more tightly. It is evident from Table 2 that most of the compounds, except DB00158 and DB00755, exhibit satisfactory binding efficiency. For instance, DB00158 and DB00755 showed higher pKd values (67.09 µM and 18.27 µM, respectively), indicating weak binding affinities towards the target protein. This higher value is mainly due to the lack of existence of hydrophobic contacts in the complex structure and ultimately leads to poor binding. The number of hydrophobic contacts is mentioned in Supplementary Table S2. It is worth mentioning that the results from the NNScore algorithm correlate well with available literature evidence [54, 55]. Similarly, a lower score of GNINA, KDEEP, SF-CNN and X-Score for ligand compounds when compared to the reference indicates a higher binding affinity for the target protein. In contrast, compounds with greater RF Scores were considered as the lead molecules. Altogether, nine compounds, namely, DB00158, DB00162, DB00163, DB00169, DB01436, DB01892, DB06750, DB14001 and DB14002 outperformed in more than four SFs (Table 2). Therefore, we considered these nine molecules for ADMET analysis to gain insights into their physiochemical and pharmacokinetic behaviour.
3.4 Prediction of ADMET properties
Early prediction of pharmacokinetic (PK) properties was considered advantageous as most of the drugs in clinical trials tend to exhibit poor PK profiles. The PK analysis identified five compounds, namely, DB00162, DB00163, DB00169, DB01892 and DB14002 with appropriate CNS activity. These compounds were also observed to show human oral absorption greater than 80%. The star value of the ligands emphasizes the violation of chemical descriptors from the desirable range. From the results, it is evident that compounds such as DB00162, DB01892 and DB14002 were found to have star values less than 5, highlighting the lower probability of outliers. Table 3 shows the molecular characteristics of the hit molecules, including their molecular weight (MW), skin permeability (QPlogKp), solubility (QPlogS), permeability to the Caco2 cell (QPPCaco), and metabolite (#metab), were found to be within acceptable limits. Altogether, it is evident from the table that the above-mentioned lead molecules showed satisfactory PK properties.
3.5 In-silico drug sensitivity prediction
The primary challenge of lead optimization is to distinguish drug-like compounds from non-drug compounds. The significant toxicological endpoints, namely, immunotoxicity, hepatotoxicity, carcinogenicity, cytotoxicity and mutagenicity were investigated for the reference and the lead molecules. Figure 1 depicts the toxicity profile of the reference and the lead molecules. The reference compound, enasidenib was observed to show immunotoxicity, whereas all the lead molecules except DB00162 pertain to show a nontoxic effect. Due to its mutagenic properties, DB00162 was excluded from antineoplastic activity prediction. Further PASS analysis predicted that compounds such as enasidenib, DB01892 and DB14002 were also found to exhibit antineoplastic activity (Table 4). In essence, the virtual cell line assay analysis portrays that DB14002 showed a lower IC50 of 0.413 µM than enasidenib (4.233 µM) [45]. In line with these results, DB14002 was identified as a hit compound and subjected to binding interaction analysis and scaffold hopping studies.
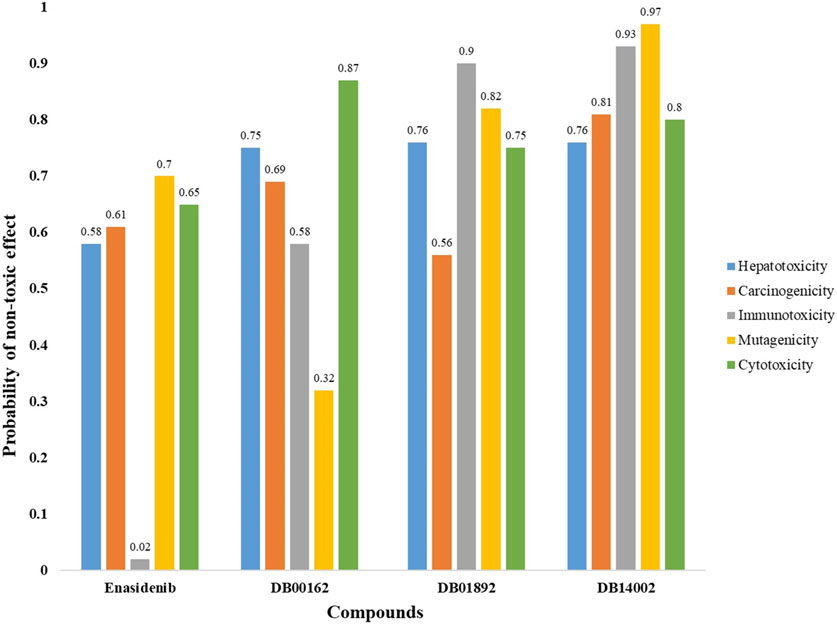
FIGURE 1. Toxicity endpoint analysis for Enasidenib, DB00162, DB01892 and DB14002 using ProTox-II server.
3.6 Interaction profiling
The binding orientation and the key catalytic pocket residues of the target protein were explored to understand the mechanism of action of the reference and hit molecule. The interaction scheme of enasidenib and DB14002 with mIDH2 protein is depicted in Figure 2. The predominant interactions such as hydrogen bonds and hydrophobic interactions were represented with distinct color codes. For instance, blue-colored lines indicate hydrogen bond interactions and grey color line signifies hydrophobic interactions. The result from interaction profiling highlights that the enasidenib extends its binding affinity towards the target protein by establishing two hydrogen bonds with GLN 361 residue at the distance of 1.97 Å and 2.08 Å respectively. In addition, the enasidenib was found to exhibit hydrophobic interactions with ILE 290 and VAL 315 residues of mIDH2 protein. Similarly, DB14002 exhibits two hydrogen bond interactions with TYR 311 and GLN 316 at a distance of 1.91 Å and 2.60 Å respectively. Interestingly, the hit molecule manifests a firm binding by extending hydrophobic interactions with the key scaffolds of the ligand molecule. Of note, the key residues of mIDH2 protein, namely, LEU 160, ILE 319 and GLU 322 were interacting with the tetramethyl scaffold of DB14002 through hydrophobic contacts. The benzopyran group of DB14002 establishes three hydrophobic interactions with residues such as ILE 290, TYR 311 and GLN 316. Another group of residues like TRP 164, VAL 294 and LEU 298 was forming hydrophobic contacts with trimethyl tridecyl moiety of the ligand molecule [56].
The structural backbone of the screened molecule was explored to gain insights into the mechanism of inhibition and efficacy against the mIDH2 protein. The 2D carbon skeleton of DB14002 is commonly known as D-alpha-Tocopherol acetate which is the primary form of vitamin E. Of note, the RRR-alpha-tocopherol stereoisomer is thought to be the natural form of alpha-tocopherol and typically has the highest bioavailability and stability of all the alpha-tocopherol stereoisomers. Due to the biological action of vitamin E, research into its potential to aid in the prevention or treatment of a variety of conditions such as diabetes, ocular conditions, cardiovascular disease and cancer is ongoing. Intriguingly, early studies on vitamin E as a synergistic component with the chemotherapeutic agent were reported to increase the growth inhibitory potential of tumor therapeutic agents on glioma cells and neuroblastoma cells [57]. Given this evidence, we postulate that DB14002 could be a promising drug-like candidate that can be synergistically used with the existing drugs to increase the treatment modality.
3.7 Molecular dynamic simulation studies
3.7.1 RMSD
The simulation studies were implemented to investigate the molecular characteristics and conformational stability of the mIDH2-ligand complex. Throughout the simulation study, the mIDH2-enasidenib complex and mIDH2-DB14002 complex are represented as black and blue trend lines respectively. In the beginning, the enasidenib bound system was found to fluctuate and reach a maximum RMS deviation of 0.45 nm between 0 ns and 40 ns. From 40 ns to 100 ns, the deviation of the trendline was minimized and found to reach a plateau. However, the system attained a state of equilibrium from 100 ns and was observed to maintain the trendline until the end of the 180 ns simulation period. The average RMSD of the enasidenib bound complex was found to be 0.45 ns. In contrast, the DB14002 bound complex was initially found to exhibit a lower RMS deviation of 0.32 nm between 0 ns and 25 ns. Further, the system was found to show fluctuation from 25 ns to 60 ns. Nevertheless, after 60 ns, the DB14002 complex acquired an equilibrium state and prolonged until 150 ns with an RMSD value of 0.35 nm. Further, there was a slight increase in the RMSD trend from 160 ns to 190 ns which might be due to the change in the conformational orientation of the DB14002. Within the 200 ns–250 ns window, both complexes exhibited a recurring deviation from the trendline for every 10 ns. These deviations were characterized by minimal fluctuations, estimated in the order of 0.01 nm–0.02 nm. At the end of 250 ns, DB14002 showed a lesser deviation than the enasidenib (Figure 3). The average RMSD of the reference and hit complex was observed to be 0.45 nm and 0.41 nm respectively. Overall, we observe a similar pattern of the RMSD trend for both the reference and DB14002 highlighting the stability.
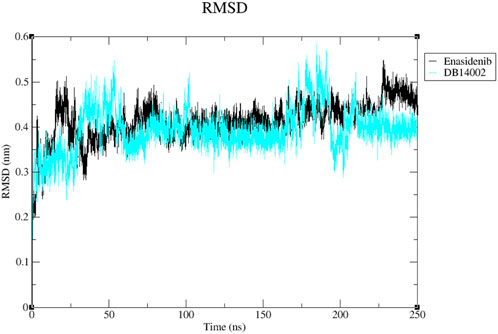
FIGURE 3. The RMSD plot of the Enasidenib (black) and DB14002 (blue) within the time boundary of 250 ns.
3.7.2 RMSF
To understand the overall flexibility of the mIDH2 structure upon ligand binding, the atomistic fluctuations of each residue were evaluated. The residual fluctuations of mIDH2 active site residues are shown in Figure 4. It is noteworthy that all the conserved catalytic residues such as V161, W164, V294, V297, L298, W306, E316, I319, L320 and G323 of both the complex were observed to have lesser residual fluctuation. Thus, it is evident that the DB14002 is unambiguously stable throughout the simulation period of 250 ns.
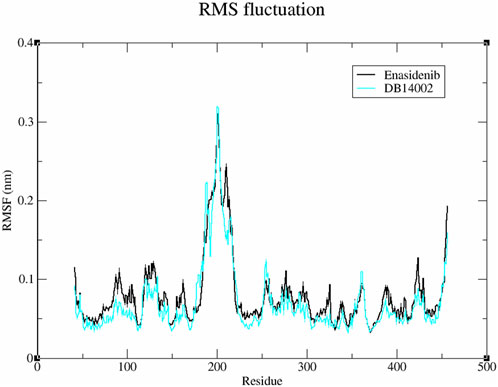
FIGURE 4. The root means square fluctuation (RMSF) plot of the Enasidenib (black) and DB14002 (blue) within the time boundary of 250 ns.
3.7.3 Hydrogen bond interaction
The binding mechanism of the protein-ligand system was evaluated by analyzing the inter-molecular hydrogen bond interactions generated within the stipulated time bound of 250 ns. From Figure 5, it is observed that the enasidenib could interact with mIDH2 protein via 3 hydrogen bonds. In the case of the mIDH2-DB14002 system, the ligand was observed to exhibit 1 hydrogen bond interaction. The result correlates well with the interaction profiling analysis, as it clearly depicts the dominance of hydrophobic interaction in the binding [58].
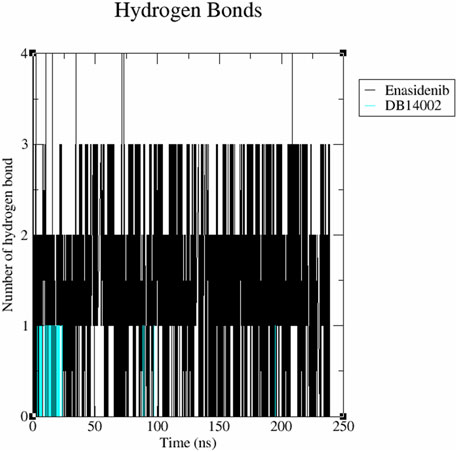
FIGURE 5. The inter-molecular hydrogen bonding scheme of the mIDH2 complexed with Enasidenib and DB14002 within the time frame of 250 ns.
3.7.4 Structural compactness analysis
The structural compactness and stability of the ligand-bound systems were assessed by measuring the radius of gyration (Rg) and Solvent Accessible Surface Area (SASA). The radius of gyration accounts for the mass-weighted root mean square distance of atoms from the centre of mass. Figure 6 displays the variation in gyration radius for both systems as a function of time. The Rg of the docked complexes exhibited a stable trend with very small fluctuations in the order of 0.01 nm. The average Rg values for the docked complex systems of mIDH2-enasidenib and mIDH2-DB14002 were found to be 2.26 nm and 2.25 nm respectively.
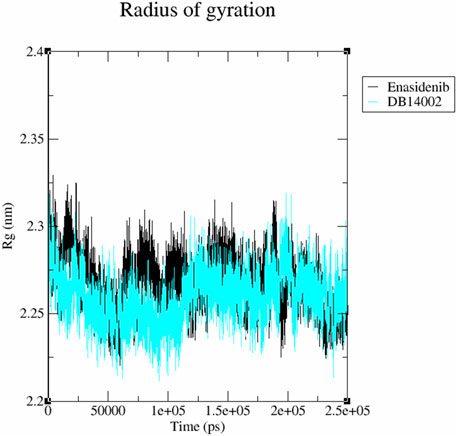
FIGURE 6. Radius of gyration (Rg) plot of the mIDH2 complexed with Enasidenib (black) and DB14002 (blue) within the time frame of 250 ns.
In addition, the accessibility of the mIDH2 surface area for the solvent interaction was inspected using SASA analysis. The average SASA value of mIDH2-enasidenib and mIDH2-DB14002 was observed to be 236.01 nm2 and 235.71 nm2 respectively (Figure 7). The free energy of solvation of mIDH2-enasidenib and mIDH2-DB14002 was found to be 1579.98 kJ/mol/nm2 and 1577.96 kJ/mol/nm2 respectively.
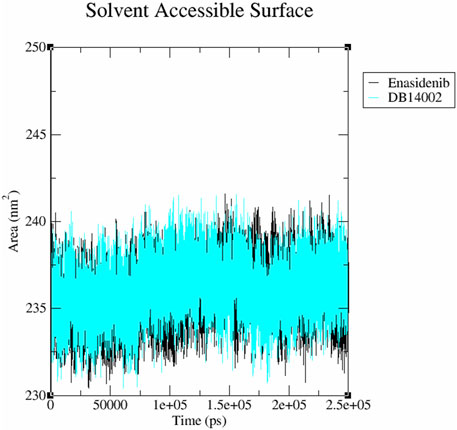
FIGURE 7. The Solvent Accessible Surface Area (SASA) plot of the mIDH2 complexed with Enasidenib (black) and DB14002 (blue) within the stipulated time of 250 ns.
3.7.5 Essential dynamics
Essential dynamics analysis was performed to gain insights into the overall expansion of the protein-ligand complexes for the simulation time frame of 250 ns [59]. In the present study, gmx_covar and gmx_ anaeig modules of gromacs were explored to find the concerted motion of atoms in the protein. Firstly, the atomic fluctuations of the c-alpha atom were recorded to construct the covariance matrix which includes eigenvalues. The sum of eigenvalues represents the overall movement of ligand-bound systems. Then, the eigenvectors were obtained by diagonalizing the covariance matrix. In the end, principal components were constructed and the first two PCs were projected along their phase space to investigate conformational changes. It is evident from Figure 8A that DB14002 was found to enclose smaller conformational space when compared to the enasidenib complex. The trace covariance matrix of the above-mentioned complexes was found to be 9.48 nm2 and 9.84 nm2 respectively. The covariance matrices of the mIDH2-enasidenib and mIDH2-DB14002 were depicted in Figures 8B, C respectively. From the figure, it is clear that the fluctuations between the atoms of enasidenib and DB14002 were between −0.07–0.30 nm2 and -0.20–0.63 nm2 respectively. The larger fluctuation range in the overall movement of the atoms of DB14002 was due to the change in the conformation of the complex structure.
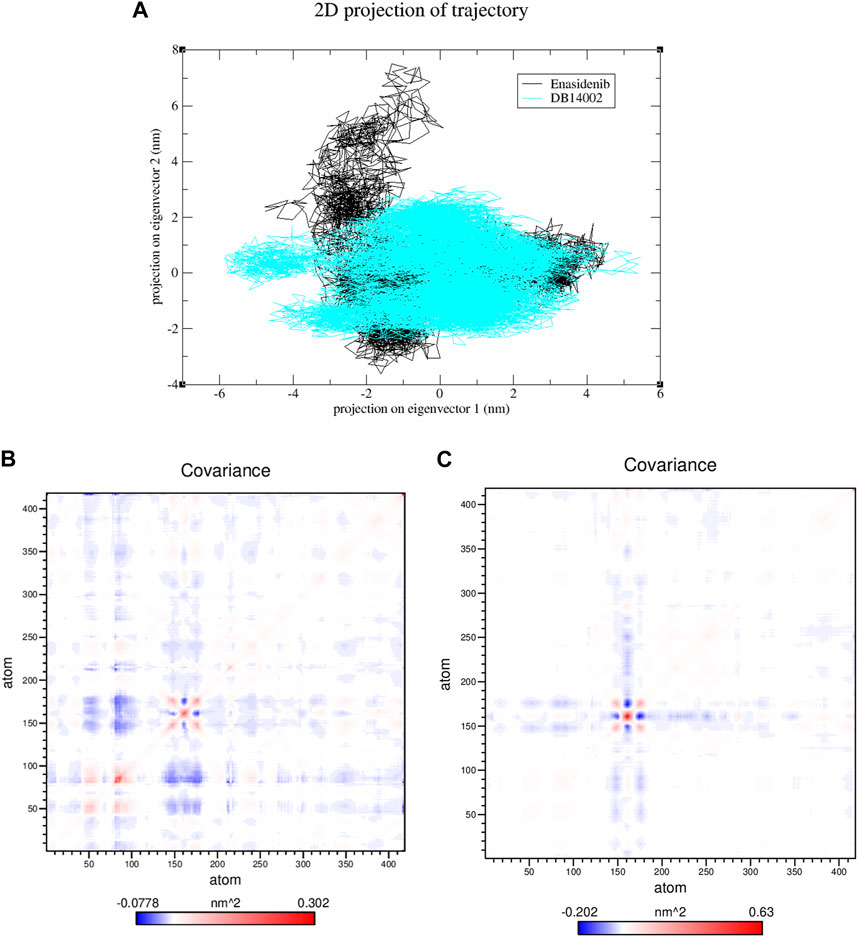
FIGURE 8. (A) The 2-D projection of principal components along its phase space and covariance matrix of the mIDH2 complexed with (B) Enasidenib and (C) DB14002.
3.7.6 Free energy landscape analysis
The interaction energy for the first two principal components was computed to assess the contribution of individual amino acids to the net free energy profile within a simulation time of 250 ns. The red region denotes the minimum in free energy, whereas the cyan and green regions represent metastable conformational states of the complex system. Figure 9 depicts the FELs of enasidenib and DB14002 showing several metastable states linked by energy barriers. The enasidenib and DB14002 systems exhibit two different minimum energy basins corresponding to their conformational states.
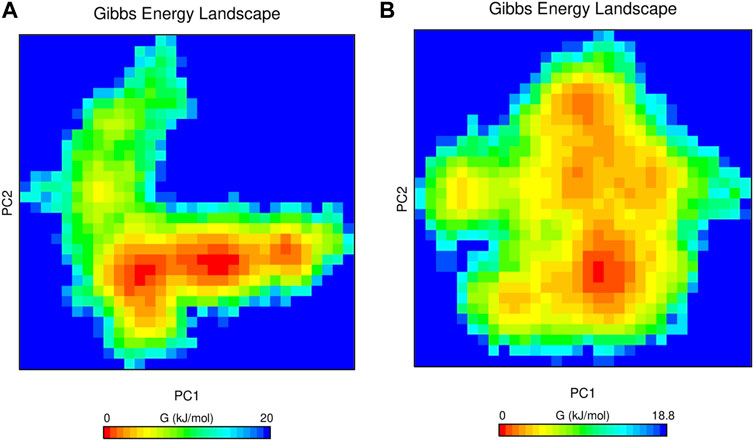
FIGURE 9. The Gibbs free energy landscape of mIDH2 complexed with (A) Enasidenib and (B) DB14002 within the time bound of 250 ns.
4 Discussion
For a long time, cancer researchers aimed to identify novel compounds either from natural sources or other synthetic libraries for the management of disease burden. In contrast, the present study addresses the role of nutraceuticals in disease management particularly against glioma. The results from molecular docking suggest that all the 135 investigated compounds exhibit appreciable binding characteristics toward the mIDH2 protein. In contrast, 14 compounds were found to possess better binding free energy from MM-GBSA analysis. Note that van der Waals interaction and the lipophilicity of the ligands were the major driving forces for the increased overall binding free energy (ΔGbind). Indeed, ML-SF highlights the enhanced binding potential of the nine lead molecules towards the target protein. Subsequent drug likeliness, toxicity endpoint and virtual cell line assay revealed the plausible application of DB14002 (Vitamin E) as an effective drug-like candidate. For instance, deep-learning-based drug sensitivity prediction tools also affirm that DB14002 possesses a lower IC50 of 0.413 µM than enasidenib.
Molecular dynamics simulations were carried out to ascertain the sturdiness of DB14002. It is evident from the RMSD plot that the observed unstable fluctuations in the MD trajectories of both systems might be due to the orientation change of respective ligands in the active pocket of mIDH2. Overall RMSD analysis showed that no significant fluctuations were observed for any of the systems, indicating that there are minimal structural deviations or conformational changes upon compound binding. In addition, the RMSF plot demonstrates that the active site residues primarily contributed to the binding of DB14002 to the target protein and thus exhibited fewer fluctuations than the reference compound. The hydrogen bond analysis was found to be consistent with MM-GBSA and interaction analysis, as the hydrophobic contacts were the primary contributors to the lower binding free energy of the hit complex. The results from structural compactness analysis demonstrated that the orientation of the protein structure does not alter after ligand binding. Additionally, the lower free energy of solvation for mIDH2-DB14002 also suggests that the system is more compact than the enasidenib-bound complex. Collectively, from essential dynamics, we culminate that the DB14002-bound complex was occupying smaller conformational phase space along with equivalent trace covariance value highlighting its stability. The energy distribution from the FEL of enasidenib and DB14002 bound systems was explored to acquire information about the overall conformational variability of the system. It is worth mentioning that the DB14002 system was found to possess a wider energy basin signifying greater thermodynamic stability than the enasidenib bound system. Collective simulation results highlight that Alpha-tocopherol (DB14002), a Vitamin E-based analog could be utilized as a potential agent for the management of glioma.
In light of vitamin E’s biological action, research is ongoing to unveil its potential to treat a wide range of disease conditions, including cancer. For instance, preclinical studies in animal models have shown that tocopherol isomers inhibit the progression of cancer growth in breast, lung and liver carcinogenesis by inducing cell cycle arrest [60–62]. A study by Lawson et al in 2004 reported that α-TEA (Alpha-tocopherol ether-linked acetic acid analog) was effective in decreasing the metastasis and tumor burden in many mice models as the attached acetic acid moiety to the phenolic ring is more stable than other isomers [63]. Additionally, in a xenograft model of cisplatin-resistant human ovarian cancer cells, combinations of α-TEA and cisplatin also markedly decreased tumor burden and metastasis. The shreds of literature evidence illustrate great promise for the use of α-TEA in combination with specific chemotherapeutic drugs in the treatment of ovarian and breast cancer [64]. Recently, a study by Mazzini et al in 2016 emphasized the anticancer activity of vitamin E analogs in glioma cells [65]. In light of this evidence together with our findings, we hypothesize that the commonly available dairy supplement Vitamin E and its structural analog may be effective against mIDH2 as a supportive agent that synergistically combines with existing drugs for the implicit management of glioma in the near future.
Data availability statement
The original contributions presented in the study are included in the article/Supplementary Material, further inquiries can be directed to the corresponding author.
Author contributions
PM: Writing–original draft. RK: Writing–review and editing.
Funding
The author(s) declare no financial support was received for the research, authorship, and/or publication of this article.
Acknowledgments
The authors thank the management of the Vellore Institute of Technology for providing the facilities to carry out this research work. The authors also acknowledge support from the Bioinformatics Resources and Applications Facility (BRAF), C-DAC, Pune.
Conflict of interest
The authors declare that the research was conducted in the absence of any commercial or financial relationships that could be construed as a potential conflict of interest.
Publisher’s note
All claims expressed in this article are solely those of the authors and do not necessarily represent those of their affiliated organizations, or those of the publisher, the editors and the reviewers. Any product that may be evaluated in this article, or claim that may be made by its manufacturer, is not guaranteed or endorsed by the publisher.
Supplementary material
The Supplementary Material for this article can be found online at: https://www.frontiersin.org/articles/10.3389/fphy.2024.1345834/full#supplementary-material
References
1. Weller M, van den Bent M, Preusser M, Le Rhun E, Tonn JC, Minniti G, et al. EANO guidelines on the diagnosis and treatment of diffuse gliomas of adulthood. Nat Rev Clin Oncol (2021) 18(3):170–86. doi:10.1038/s41571-020-00447-z
2. Cairns RA, Harris IS, Mak TW. Regulation of cancer cell metabolism. Nat Rev Cancer (2011) 11(2):85–95. doi:10.1038/nrc2981
3. Hanahan D, Weinberg RA. Hallmarks of cancer: the next generation. Cell (2011) 144(5):646–74. doi:10.1016/j.cell.2011.02.013
4. Nagarajan A, Malvi P, Wajapeyee N. Oncogene-directed alterations in cancer cell metabolism. Trends Cancer (2016) 2(7):365–77. doi:10.1016/j.trecan.2016.06.002
5. Nowicki S, Gottlieb E. Oncometabolites: tailoring our genes. FEBS J (2015) 282(15):2796–805. doi:10.1111/febs.13295
6. Reitman ZJ, Yan H. Isocitrate dehydrogenase 1 and 2 mutations in cancer: alterations at a crossroads of cellular metabolism. J Natl Cancer Inst (2010) 102(13):932–41. doi:10.1093/jnci/djq187
7. Koh HJ, Lee SM, Son BG, Lee SH, Ryoo ZY, Chang KT, et al. Cytosolic NADP+-dependent isocitrate dehydrogenase plays a key role in lipid metabolism. J Biol Chem (2004) 279(38):39968–74. doi:10.1074/jbc.m402260200
8. Badur MG, Muthusamy T, Parker SJ, Ma S, McBrayer SK, Cordes T, et al. Oncogenic R132 IDH1 mutations limit NADPH for de novo lipogenesis through (D) 2-hydroxyglutarate production in fibrosarcoma cells. Cell Rep (2018) 25(4):1680–026. doi:10.1016/j.celrep.2018.10.099
9. Cohen AL, Holmen SL, Colman H. IDH1 and IDH2 mutations in gliomas. Curr Neurol Neurosci Rep (2013) 13:345–7. doi:10.1007/s11910-013-0345-4
10. Yan H, Parsons DW, Jin G, McLendon R, Rasheed BA, Yuan W, et al. IDH1 and IDH2 mutations in gliomas. N Engl J Med (2009) 360(8):765–73. doi:10.1056/nejmoa0808710
11. Wang F, Travins J, DeLaBarre B, Penard-Lacronique V, Schalm S, Hansen E, et al. Targeted inhibition of mutant IDH2 in leukemia cells induces cellular differentiation. Science (2013) 340(6132):622–6. doi:10.1126/science.1234769
12. Yen K, Travins J, Wang F, David MD, Artin E, Straley K, et al. AG-221, a first-in-class therapy targeting acute myeloid leukemia harboring oncogenic IDH2 mutations. Cancer Discov (2017) 7(5):478–93. doi:10.1158/2159-8290.CD-16-1034
13. Stein EM, DiNardo CD, Pollyea DA, Fathi AT, Roboz GJ, Altman JK, et al. Enasidenib in mutant IDH2 relapsed or refractory acute myeloid leukemia. Blood (2017) 130(6):722–31. doi:10.1182/blood-2017-04-779405
14. Intlekofer AM, Shih AH, Wang B, Nazir A, Rustenburg AS, Albanese SK, et al. Acquired resistance to IDH inhibition through trans or cis dimer-interface mutations. Nature (2018) 559(7712):125–9. doi:10.1038/s41586-018-0251-7
15. Gao M, Zhu H, Fu L, Li Y, Bao X, Fu H, et al. Pharmacological characterization of TQ 05310, a potent inhibitor of isocitrate dehydrogenase 2 R140Q and R172K mutants. Cancer Sci (2019) 110(10):3306–14. doi:10.1111/cas.14152
16. Yao K, Liu H, Liu P, Liu W, Yang J, Wei Q, et al. Molecular modeling studies to discover novel mIDH2 inhibitors with high selectivity for the primary and secondary mutants. Comput Biol Chem (2020) 86:107261. doi:10.1016/j.compbiolchem.2020.107261
17. Reed D, Raina K, Agarwal R. Nutraceuticals in prostate cancer therapeutic strategies and their neo-adjuvant use in diverse populations. NPJ Precis Oncol (2018) 2(1):15. doi:10.1038/s41698-018-0058-x
18. Mondul AM, Weinstein SJ, Layne TM, Albanes D. Vitamin D and cancer risk and mortality: state of the science, gaps, and challenges. Epidemiologic Rev (2017) 39(1):28–48. doi:10.1093/epirev/mxx005
19. Gontero P, Marra G, Soria F, Oderda M, Zitella A, Baratta F, et al. A randomized double-blind placebo-controlled phase I–II study on clinical and molecular effects of dietary supplements in men with precancerous prostatic lesions. Chemoprevention or “chemopromotion”. The Prostate (2015) 75(11):1177–86. doi:10.1002/pros.22999
20. Hsu HY, Hwang PA. Clinical applications of fucoidan in translational medicine for adjuvant cancer therapy. Clin Transl Med (2019) 8(1):15–8. doi:10.1186/s40169-019-0234-9
21. Konteatis Z, Artin E, Nicolay B, Straley K, Padyana AK, Jin L, et al. Vorasidenib (AG-881): a first-in-class, brain-penetrant dual inhibitor of mutant IDH1 and 2 for treatment of glioma. ACS Med Chem Lett (2020) 11(2):101–7. doi:10.1021/acsmedchemlett.9b00509
22. Madhavi Sastry G, Adzhigirey M, Day T, Annabhimoju R, Sherman W. Protein and ligand preparation: parameters, protocols, and influence on virtual screening enrichments. J Comput Aid Mol Des (2013) 27:221–34. doi:10.1007/s10822-013-9644-8
23. Roos K, Wu C, Damm W, Reboul M, Stevenson JM, Lu C, et al. OPLS3e: extending force field coverage for drug-like small molecules. J Chem Theor Comput (2019) 15(3):1863–74. doi:10.1021/acs.jctc.8b01026
24. Poonan P, Agoni C, Soliman ME. Dual-knockout of mutant isocitrate dehydrogenase 1 and 2 subtypes towards glioma therapy: structural mechanistic insights on the role of vorasidenib. Chem Biodivers (2021) 18(7):e2100110. doi:10.1002/cbdv.202100110
25. Salifu EY, Agoni C, Soliman ME. Highlighting the mechanistic role of Olutasidenib (FT-2102) in the selective inhibition of mutated isocitrate dehydrogenase 1 (mIDH1) in cancer therapy. Inform Med Unlocked (2022) 28:100829. doi:10.1016/j.imu.2021.100829
26. Friesner RA, Murphy RB, Repasky MP, Frye LL, Greenwood JR, Halgren TA, et al. Extra precision glide: docking and scoring incorporating a model of hydrophobic enclosure for protein-ligand complexes. J Med Chem (2006) 49(21):6177–96. doi:10.1021/jm051256o
27. Mullard A. FDA approves first-in-class cancer metabolism drug. Nat Rev Drug Discov (2017) 16(9):593–4. doi:10.1038/nrd.2017.174
28. Lyne PD, Lamb ML, Saeh JC. Accurate prediction of the relative potencies of members of a series of kinase inhibitors using molecular docking and MM-GBSA scoring. J Med Chem (2006) 49(16):4805–8. doi:10.1021/jm060522a
29. Maia EHB, Assis LC, De Oliveira TA, Da Silva AM, Taranto AG. Structure-based virtual screening: from classical to artificial intelligence. Front Chem (2020) 8:343. doi:10.3389/fchem.2020.00343
30. Li H, Sze KH, Lu G, Ballester PJ. Machine-learning scoring functions for structure-based virtual screening. Wiley Interdiscip Rev Comput Mol Sci (2021) 11(1):e1478. doi:10.1002/wcms.1478
31. Li H, Leung KS, Wong MH, Ballester PJ. Low-quality structural and interaction data improves binding affinity prediction via random forest. Molecules (2015) 20(6):10947–62. doi:10.3390/molecules200610947
32. Wójcikowski M, Ballester PJ, Siedlecki P. Performance of machine-learning scoring functions in structure-based virtual screening. Sci Rep (2017) 7(1):46710. doi:10.1038/srep46710
33. Li L, Wang B, Meroueh SO. Support vector regression scoring of receptor–ligand complexes for rank-ordering and virtual screening of chemical libraries. J Chem Inf Model (2011) 51(9):2132–8. doi:10.1021/ci200078f
34. Durrant JD, McCammon JA. NNScore 2.0: a neural network receptor-ligand scoring function. J Chem Inf Model (2011) 51:2897–903. doi:10.1021/ci2003889
35. Abduljalil JM, Elfiky AA, Sayed ESTA, AlKhazindar MM. Computational identification of drug-like marine natural products as potential RNA polymerase inhibitors against Nipah virus. Comput Biol Chem (2023) 104:107850. doi:10.1016/j.compbiolchem.2023.107850
36. Sahib SA, Al-Salami AM, Al-Tajer NF, Al-Mashhadani SA. Role of the accuracy of machine learning in predicting the outcome of methotrexate treatment in plaque Psoriasis. Iraq Med J (2022) 68(2):73–82.
37. Joshi T, Joshi T, Pundir H, Sharma P, Mathpal S, Chandra S. Predictive modeling by deep learning, virtual screening and molecular dynamics study of natural compounds against SARS-CoV-2 main protease. J Biomol Struct Dyn (2021) 39(17):6728–46. doi:10.1080/07391102.2020.1802341
38. Egan WJ, Merz KM, Baldwin JJ. Prediction of drug absorption using multivariate statistics. J Med Chem (2000) 43(21):3867–77. doi:10.1021/jm000292e
39. Banerjee P, Eckert AO, Schrey AK, Preissner R. ProTox-II: a webserver for the prediction of toxicity of chemicals. Nucleic Acids Res (2018) 46(W1):W257–63. doi:10.1093/nar/gky318
40. Filimonov DA, Lagunin AA, Gloriozova TA, Rudik AV, Druzhilovskii DS, Pogodin PV, et al. Prediction of the biological activity spectra of organic compounds using the PASS online web resource. Chem Heterocycl Compd (2014) 50:444–57. doi:10.1007/s10593-014-1496-1
41. Banerjee P, Dehnbostel FO, Preissner R. Prediction is a balancing act: importance of sampling methods to balance sensitivity and specificity of predictive models based on imbalanced chemical data sets. Front Chem (2018) 6:362. doi:10.3389/fchem.2018.00362
42. Manica M, Oskooei A, Born J, Subramanian V, Sáez-Rodríguez J, Rodriguez Martinez M. Toward explainable anticancer compound sensitivity prediction via multimodal attention-based convolutional encoders. Mol Pharmaceutics (2019) 16(12):4797–806. doi:10.1021/acs.molpharmaceut.9b00520
43. Cadow J, Born J, Manica M, Oskooei A, Rodríguez Martínez M. PaccMann: a web service for interpretable anticancer compound sensitivity prediction. Nucleic Acids Res (2020) 48(W1):W502–8. doi:10.1093/nar/gkaa327
44. Khan FI, Aamir M, Wei DQ, Ahmad F, Hassan MI. Molecular mechanism of Ras-related protein Rab-5A and effect of mutations in the catalytically active phosphate-binding loop. J Biomol Struct Dyn (2017) 35(1):105–18. doi:10.1080/07391102.2015.1134346
45. Syed SB, Khan FI, Khan SH, Srivastava S, Hasan GM, Lobb KA, et al. Mechanistic insights into the urea-induced denaturation of kinase domain of human integrin-linked kinase. Int J Biol Macromol (2018) 111:208–18. doi:10.1016/j.ijbiomac.2017.12.164
46. Huang J, MacKerell AD. CHARMM36 all-atom additive protein force field: validation based on comparison to NMR data. J Comput Chem (2013) 34(25):2135–45. doi:10.1002/jcc.23354
47. Wu Y, Tepper HL, Voth GA. Flexible simple point-charge water model with improved liquid-state properties. J Chem Phy (2006) 124(2):024503. doi:10.1063/1.2136877
48. Zhang Y, Wang L. The WRKY transcription factor superfamily: its origin in eukaryotes and expansion in plants. BMC Evol Biol (2005) 5:1–12. doi:10.1186/1471-2148-5-1
49. Vilar S, Karpiak J, Costanzi S. Ligand and structure-based models for the prediction of ligand-receptor affinities and virtual screenings: development and application to the β2-adrenergic receptor. J Comput Chem (2010) 31(4):707–20. doi:10.1002/jcc.21346
50. Dolghih E, Bryant C, Renslo AR, Jacobson MP. Predicting binding to p-glycoprotein by flexible receptor docking. Plos Comput Biol (2011) 7(6):e1002083. doi:10.1371/journal.pcbi.1002083
51. Sgobba M, Caporuscio F, Anighoro A, Portioli C, Rastelli G. Application of a post-docking procedure based on MM-PBSA and MM-GBSA on single and multiple protein conformations. Eur J Med Chem (2012) 58:431–40. doi:10.1016/j.ejmech.2012.10.024
52. Ashtawy HM, Mahapatra NR. Task-specific scoring functions for predicting ligand binding poses and affinity and for screening enrichment. J Chem Inf Model (2018) 58(1):119–33. doi:10.1021/acs.jcim.7b00309
53. Yang C, Chen EA, Zhang Y. Protein–ligand docking in the machine-learning era. Molecules (2022) 27(14):4568. doi:10.3390/molecules27144568
54. Durrant JD, McCammon JA. BINANA: a novel algorithm for ligand-binding characterization. J Mol Graph Model (2011) 29(6):888–93. doi:10.1016/j.jmgm.2011.01.004
55. Patil R, Das S, Stanley A, Yadav L, Sudhakar A, Varma AK. Optimized hydrophobic interactions and hydrogen bonding at the target-ligand interface leads the pathways of drug-designing. PloS one (2010) 5(8):e12029. doi:10.1371/journal.pone.0012029
56. Ma R, Yun CH. Crystal structures of pan-IDH inhibitor AG-881 in complex with mutant human IDH1 and IDH2. Biochem Biophys Res Commun (2018) 503(4):2912–7. doi:10.1016/j.bbrc.2018.08.068
57. Prasad KN, Edwards-Prasad J, Ramanujam S, Sakamoto A. Vitamin E increases the growth inhibitory and differentiating effects of tumor therapeutic agents on neuroblastoma and glioma cells in culture. Proc Soc Exp Biol Med (1980) 164(2):158–63. doi:10.3181/00379727-164-40840
58. Verma S, Grover S, Tyagi C, Goyal S, Jamal S, Singh A, et al. Hydrophobic interactions are a key to MDM2 inhibition by polyphenols as revealed by molecular dynamics simulations and MM/PBSA free energy calculations. PloS one (2016) 11(2):e0149014. doi:10.1371/journal.pone.0149014
59. Maisuradze GG, Liwo A, Scheraga HA. Principal component analysis for protein folding dynamics. J Mol Biol (2009) 385(1):312–29. doi:10.1016/j.jmb.2008.10.018
60. Komiyama K, Iizuka K, Yamaoka M, Wantanabe H, Tsuchiya N, Umezawa I. Studies on the biological activity of tocotrienols. Chem Pharm Bull (1989) 37(5):1369–71. doi:10.1248/cpb.37.1369
61. Gould MN, Haag JD, Kennan WS, Tanner MA, Elson CE. A comparison of tocopherol and tocotrienol for the chemoprevention of chemically induced rat mammary tumors. Am J Clin Nutr (1991) 53(4):1068S–70S. doi:10.1093/ajcn/53.4.1068s
62. Guthrie N, Gapor A, Chambers AF, Carroll KK. Inhibition of proliferation of estrogen receptor–negative MDA-MB-435 and–positive MCF-7 human breast cancer cells by palm oil tocotrienols and tamoxifen, alone and in combination. J Nutr (1997) 127(3):544S–8S. doi:10.1093/jn/127.3.544s
63. Lawson KA, Anderson K, Simmons-Menchaca M, Atkinson J, Sun L, Sanders BG, et al. Comparison of vitamin E derivatives α-TEA and VES in reduction of mouse mammary tumor burden and metastasis. Exp Biol Med (2004) 229(9):954–63. doi:10.1177/153537020422900913
64. Anderson K, Simmons-Menchaca M, Lawson KA, Atkinson J, Sanders BG, Kline K. Differential response of human ovarian cancer cells to induction of apoptosis by vitamin E succinate and vitamin E analogue, α-TEA. Cancer Res (2004) 64(12):4263–9. doi:10.1158/0008-5472.can-03-2327
Keywords: mutated isocitrate dehydrogenase 2 (mIDH2), molecular docking, MM-GBSA calculations, machine learning, molecular dynamic simulation
Citation: Murali P and Karuppasamy R (2024) Exploring the potential of nutraceutical to combat gliomas: focus on mIDH2 protein. Front. Phys. 12:1345834. doi: 10.3389/fphy.2024.1345834
Received: 28 November 2023; Accepted: 15 February 2024;
Published: 29 February 2024.
Edited by:
Umashankar Vetrivel, Indian Council of Medical Research (ICMR), IndiaReviewed by:
Dhamodharan Prabhu, Karpagam Academy of Higher Education, IndiaXingcheng Lin, North Carolina State University, United States
Copyright © 2024 Murali and Karuppasamy. This is an open-access article distributed under the terms of the Creative Commons Attribution License (CC BY). The use, distribution or reproduction in other forums is permitted, provided the original author(s) and the copyright owner(s) are credited and that the original publication in this journal is cited, in accordance with accepted academic practice. No use, distribution or reproduction is permitted which does not comply with these terms.
*Correspondence: Ramanathan Karuppasamy, kramanathan@vit.ac.in