- 1Department of Propedeutics of Internal Medicine No.1, Fundamentals of Bioethics and Biosafety, Kharkiv National Medical University, Kharkiv, Ukraine
- 2Department of Epidemiology, Kharkiv National Medical University, Kharkiv, Ukraine
- 3Department of Mathematical Modelling and Artificial Intelligence, National Aerospace University "Kharkiv Aviation Institute", Kharkiv, Ukraine
- 4Department of Pulmonology, MNE “Clinical City Hospital No.13” of Kharkiv City Council, Kharkiv, Ukraine
Background: Post-COVID-19 syndrome (PCS) has been increasingly recognized as an emerging problem: 50% of patients report ongoing symptoms 1 year after acute infection, with most typical manifestations (fatigue, dyspnea, psychiatric and neurological symptoms) having potentially debilitating effect. Early identification of high-risk candidates for PCS development would facilitate the optimal use of resources directed to rehabilitation of COVID-19 convalescents.
Objective: To study the in-hospital clinical characteristics of COVID-19 survivors presenting with self-reported PCS at 3 months and to identify the early predictors of its development.
Methods: 221 hospitalized COVID-19 patients underwent symptoms assessment, 6-min walk test, and echocardiography pre-discharge and at 1 month; presence of PCS was assessed 3 months after discharge. Unsupervised machine learning was used to build a SANN-based binary classification model of PCS development.
Results: PCS at 3 months has been detected in 75% patients. Higher symptoms level in the PCS group was not associated with worse physical functional recovery or significant echocardiographic changes. Despite identification of a set of pre-discharge predictors, inclusion of parameters obtained at 1 month proved necessary to obtain a high accuracy model of PCS development, with inputs list including age, sex, in-hospital levels of CRP, eGFR and need for oxygen supplementation, and level of post-exertional symptoms at 1 month after discharge (fatigue and dyspnea in 6MWT and MRC Dyspnea score).
Conclusion: Hospitalized COVID-19 survivors at 3 months were characterized by 75% prevalence of PCS, the development of which could be predicted with an 89% accuracy using the derived neural network-based classification model.
1 Background
Since 2019–2020, the novel SARS-CoV-2 infection has emerged as a global health challenge. During the first year of COVID-19 pandemic, the cumulative effect of overwhelming of healthcare systems, lack of effective etiological treatment, and more severe course of disease that was typical to early SARS-CoV-2 variants had led to high morbidity and significant excess mortality (1), and the cascade of prolonged lockdowns that were introduced in an attempt to slow down the epidemic process imposed an additional strain on economies worldwide (2). Subsequent success of the global vaccination campaign has dramatically reduced the prevalence of severe and life-threatening cases whilst imposing less significant initial effect on the morbidity (3). Moreover, gradual lifting of lockdowns contributed to multifocal re-acceleration of the COVID-19 spread, with the daily new cases count in January 2022 exceeding the parameters during previous peaks more than three-fold (4). As a result, the current phase of lower morbidity that we observe since the beginning of 2023 is being continuously accompanied by an increasing number of patients suffering from long lasting sequelae of SARS-CoV-2 infection.
Unlike in most other respiratory viral infections, persistence of symptoms after the end of the acute phase is highly prevalent in COVID-19 convalescents (5, 6) and is shown to be associated with a decreased quality of life (7). Potential pathogenetic mechanisms are heterogenous and include consequences of direct organs injury during the acute phase, persistent SARS-CoV-2 replication, dysautonomia, altered immune reactivity, coagulopathy, endotheliopathy, and gastrointestinal microbiome disturbances (8, 9). “Long COVID,” the most popular term reserved for this scenario, accounts for all manifestations that persist beyond 4 weeks after disease onset and, therefore, includes the early post-acute period of 4 to 12 weeks (also referred to as “ongoing symptomatic COVID-19”), when one expects to observe good natural dynamics of residual symptoms resolving in a significant proportion of patients (10). At the same time, development of the “post-COVID-19 syndrome,” being defined as persistence of symptoms beyond 12 weeks, presents a more significant socio-economic problem, given the debilitating effect of some of the most prevalent symptoms, such as fatigue, dyspnea, apathy, and cognitive dysfunction (6, 10, 11).
The prevalence of post-COVID-19 syndrome (PCS) ranges from 5 to 37% in the general population of convalescents but may reach as high as 76 to 81% in those who required hospitalization (6, 12). To date, cardiopulmonary rehabilitation remains the mainstay of management of these patients, with locally available resources being at times insufficient to deal with the total flow of new convalescents (13–15). In this setting, creation of tools to assess the risk of subsequent development of PCS basing on pre-discharge data might be used to optimize the selection of candidates for supervised rehabilitation programs following hospitalization.
1.1 Objective
To study the in-hospital clinical characteristics of COVID-19 survivors presenting with self-reported post-COVID-19 syndrome at 3 months and to identify the early predictors of its development.
2 Materials and methods
2.1 Study design and population
By design, this is a cross-sectional prospective observational single-center study that was performed at Kharkiv City Hospital No.13 that at the time served as a specialized tertiary COVID-19 care center. Between January and November 2021, patients that were hospitalized with the diagnosis of pneumonia were evaluated for eligibility criteria that included the age of ≥18 years and positive polymerase chain reaction test for SARS-CoV-2. In total, 265 consecutive eligible patients were identified and invited to participate in the study; 44 of those have declined participation, and 221 were enrolled. The final cohort included 166 patients who have completed the repeated visit at 3 months post-discharge – see Supplementary Figure S1 for the study flowchart.
The study was conducted in compliance with the standards of Helsinki Declaration and was approved by the ethical committee of Kharkiv National Medical University (No. 3/2021). All participants provided written informed consent.
2.2 Clinical data collection
The first visit was performed pre-discharge, in clinically stable patients who were meeting clinical criteria of epidemic safety [resting capillary blood oxygen saturation (SpO2) >93% on room air, absence of acute respiratory symptoms and normal body temperature for ≥3 days starting from the 10th day after the onset of disease (16)] and included the analysis of medical records to collect data on patients’ age, gender, laboratory and instrumental findings, and treatment used; an interview to obtain data on symptoms and medical history; and anthropometry.
Transthoracic echocardiography (TTE) was performed by an expert physician who was blinded to patients’ data prior to procedure, using Radmir ULTIMA ultrasound system (Radmir Co., Ukraine). The measurements were performed in strict accordance with the respective guidelines by the American Society of Echocardiography (ASE) and European Association of Cardiovascular Imaging (EACVI) (17–19) and included cardiac chambers morphometry (left atrial and left ventricular [LV] volumes, right atrial area, right ventricular size, relative walls thickness and myocardial mass index of the LV), assessment of the indices of systolic function (LV ejection fraction using Simpson biplane method, mitral and tricuspid annular planes systolic excursion, global longitudinal strain [GLS] of ventricles using the linear method (20–22), and mitral annular s’ velocity in the tissue Doppler mode) and LV diastolic function (mitral e’ velocity and E/e’ ratio).
6-min walk test (6MWT) was performed by a physician using the standard methodology as recommended by the American Thoracic Society guidelines (23), in a self-paced mode, with no use of practice tests, warm-up or non-standardized encouragement. A 20-m hallway was used, determining the selection of models to calculate the individual predicted distance (24). Pulse rate and SpO2 were registered at start and every 30 s thereafter using a Bluetooth-connected pulse oximeter; levels of fatigue and dyspnea were assessed at the baseline and at finish using modified Borg scale. Along with 6-min walk distance (6MWD), reached percent of predicted distance (6MWD%), baseline and minimal capillary oxygen saturation (SpO2base and SpO2min), baseline and maximal heart rate (HRbase and HRmax) were analyzed. Peak oxygen desaturation was calculated as SpO2drop = SpO2min - SpO2base, and reached percent of the predicted maximal heart rate as HRmax% = 100% × HRmax / (208–0.8 × Age) (25).
A follow-up visit at 1 month after discharge followed the same protocol, and the final visit at 3 months was performed distantly (by means of telephone, email, and text messengers) and included re-assessment of symptoms and detection of the PCS which was defined as a self-reported perceived worsening of health status compared to the pre-COVID-19 state or persistence of new symptoms during the last month before the visit.
2.3 Statistical analysis
The data analysis was performed using StatSoft STATISTICA Version 12 software package. Shapiro–Wilk test was used to assess the distribution of data. Continuous variables are reported as mean ± standard deviation (SD) in case of normal distribution and as median [interquartile range] in case of skewed distribution. Categorical variables are reported as counts (percentages). Independent samples t-test was used to compare normally distributed continuous variables, and paired samples t-test was used for longitudinal comparisons. For skewed variables, the comparisons were made using Mann–Whitney U-test or Wilcoxon signed-rank test. Binary and categorical variables were compared using Chi-Square test. The differences were considered significant if p < 0.05. Marginal effects of potential PCS predictors in logistic regression analysis were used as a measure to select inputs for unsupervised machine learning based training of simple artificial neural networks (SANN). Random sampling was used to select training, test and validation subsets of the study cohort in the 70:15:15 proportion. For each set of input variables, 500 binary classification models were trained. This number represented an empirical balance between computational resource allocation and model reproducibility – fluctuating prediction accuracy was observed with fewer models, whereas the consistency in results was achieved at n = 500; further increasing the model count did not yield significant improvements but incurred greater computational time. Automated neural architecture search strategy and Broyden-Fletcher-Goldfarb-Shanno optimization algorithm were used; missing data was imputed by mean values. Predictive performance of the obtained models was assessed as percentage of correctly classified cases from the test and validation subsets. For the final predictive model, 10-fold cross-validation was used to ensure its reproducibility, and ROC analysis performed. A post-hoc approach incorporating assessment of the model accuracy and the dataset effect size using Cohen’s d statistic was used to evaluate the sample size adequacy (26).
3 Results
3.1 Baseline characteristics
The final study cohort included 76 male and 90 female patients at the mean age of 53.7 ± 13.3 years. Visit 1 was performed at the median of 22 days, visit 2 at 53 days, and visit 3 at 116 days after the symptoms onset. Among the observed cohort, 124 (75%) were reporting ongoing new symptoms and/or self-estimated worsening of health status that was classified as post-COVID-19 syndrome. The comparative clinical characteristic of study participants with and without ongoing symptoms at 3 months is presented in Table 1. The patients with PCS were older, more frequently female, had higher BMI and comorbidities burden. In their in-hospital laboratory profile, patients with ongoing symptoms at 3 months had higher values of C-reactive protein, erythrocyte sedimentation rate, and higher proportion of patients with very high interleukin-6 values, which attested to higher inflammatory activity. The observed lower values in estimated glomerular filtration rate were explained by age and gender differences. There were no differences in received treatment, but PCS patients more frequently required oxygen support during hospitalization.
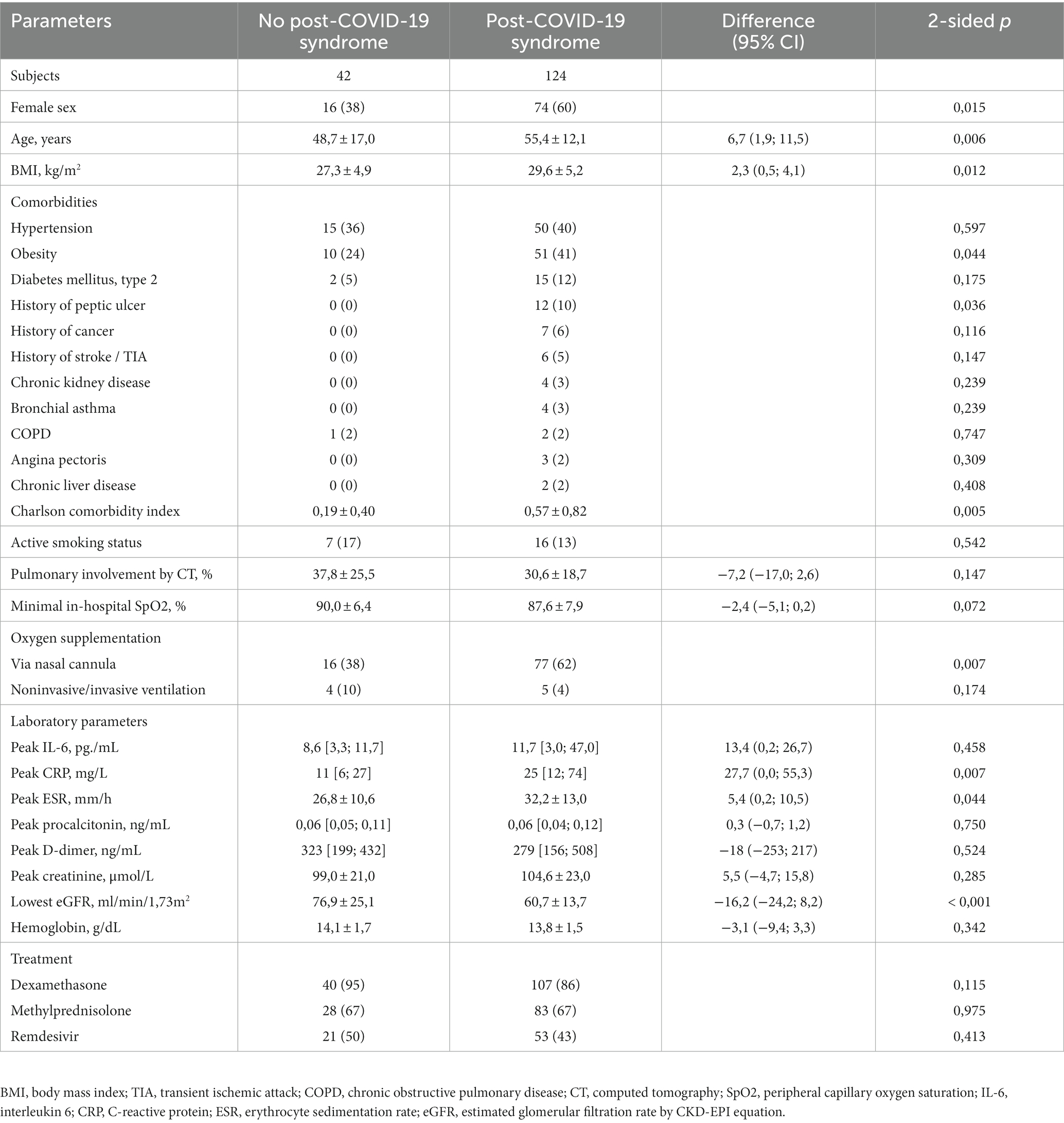
Table 1. Demographics and pre-discharge clinical characteristics of the study participants with and without post-COVID-19 syndrome.
3.2 Physical performance assessment
Analysis of the 6MWT parameters (see Table 2) has revealed that the apparent decrease of 6-min walk distance (6MWD) that was observed pre-discharge in PCS patients was explained by age and sex differences, evidenced by close values of the reached percent of individually predicted distance (6MWD%). Moreover, the PCS group has paradoxically demonstrated larger between-visits increment of both parameters, resulting in higher 6MWD% values at 1 month. At the same time, the level of subjective symptoms in these patients was significantly higher at visit 2, being explained by much worse improvement from the pre-discharge baseline compared to non-PCS participants. This difference was not explained by the observed values of capillary oxygen saturation throughout the test (there was no difference between groups), nor could it be attributed to worse dysautonomia – despite initially lower heart rate (HR) increment during the test at visit 1, the PCS patients have demonstrated better dynamics of utilization of HR reserve, which resulted in higher reached percent of the individual HR maximum at 1 month. MRC dyspnea scale score at both visits was also higher in PCS participants with the mean values of 2.5 ± 1.1 vs. 2.0 ± 1.0 pre-discharge (p = 0.005) and 1.8 ± 0.8 vs. 1.3 ± 0.6 at visit 2 (p = 0.002).
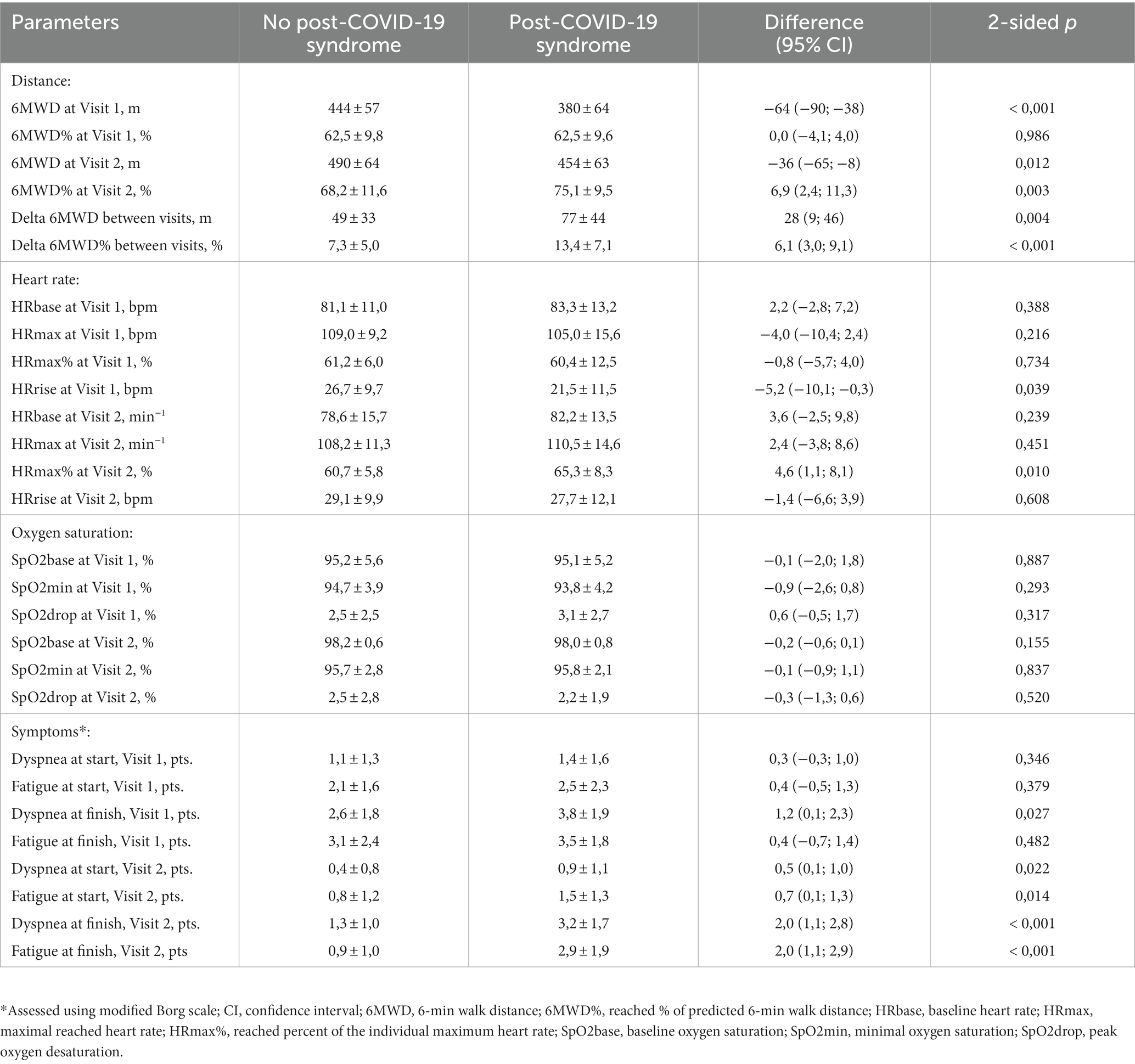
Table 2. One-month post-discharge dynamics of 6-min walk test parameters in patients with and without post-COVID-19 syndrome.
3.3 Echocardiographic assessment
Retrospective assessment of echocardiographic features of observed patients has only revealed minor differences between PCS and non-PCS study participants (see Table 3). Both groups were showing a strong trend to concentric LV remodeling [refer to (27) for the detailed comparison to matched control], and patients who were subsequently reporting long-lasting symptoms had somewhat smaller ventricular cavities. Despite this fact, systolic atrioventricular annuli excursion was comparable to PCS-free patients, translating into somewhat higher longitudinal strain values (reaching statistical significance in case of RV). The borderline changes in diastolic LV parameters became more apparent at visit 2, with significantly lower e’ velocity (9.4 ± 2.6 vs. 10.7 ± 3.1 cm/s, p = 0,025) and higher E/e’ ratio (7.6 ± 2.4 vs. 6.4 ± 1,6, p = 0,020) being observed in the PCS group; the only other difference that persisted at 1 month was the slightly higher RV free wall strain values in the PCS cohort.
3.4 Prediction of post-COVID-19 syndrome development
To create a tool that would predict the PCS development, unsupervised machine learning was used to train SANN-based binary classification models. In order to avoid overfitting effect in the setting of a relatively small sample size, we applied a two-step approach, where the list of potential inputs was first narrowed down using the assessment of their marginal effects in the logistic regression analysis. In the order of decreasing prognostic value, significant pre-discharge predictors included pre-discharge 6MWD, eGFR, heart rate increment during 6MWT, SBP, RV free wall strain, ESR, LV end-diastolic index, BMI, in-hospital oxygen supplementation, Charlson’s comorbidity index, height, sex, age, and obesity (see Supplementary tables 1–2 and Supplementary figure 2 for exact Somers’ D values and the resulting regression model parameters). As a next step, identified parameters were used as inputs for SANN-based classification model.
The use of the complete set of significant pre-discharge predictors, however, did not result in generation of high-performance models: the best predictive accuracy in the test/validation subset was 83%, which (given the 75% prevalence of PCS) meant that only 1/3 of otherwise false-positive cases could be correctly reclassified compared to the blunt assumption that all participants will develop the PCS. Addition of the parameters obtained during the first follow-up visit that was performed 1 month after discharge (see Supplementary Table 3 for marginal effects in logistic regression analysis) with subsequent stepwise deletion of excessive variables based on the results of global sensitivity analysis of the current version of the model have resulted in creation of the model that was characterized by a 93% predictive performance in the training and 89% in the randomly selected test/validation subsets of the study group (91% for sensitivity, 82% for specificity). The model utilized 13–7-2 SANN architecture and used age, sex, in-hospital levels of CRP, eGFR and need for oxygen supplementation, and level of post-exertional symptoms at 1 month after discharge (fatigue and dyspnea in 6MWT and MRC Dyspnea score) as inputs (Figure 1; see Supplementary Table S4 for network weights and connections; source file available at https://doi.org/10.5281/zenodo.8395451). 10-fold cross-validation that was performed by re-training the neural networks using alternative sampling has allowed to consistently obtain non-inferior accuracy in the test/validation subsets.
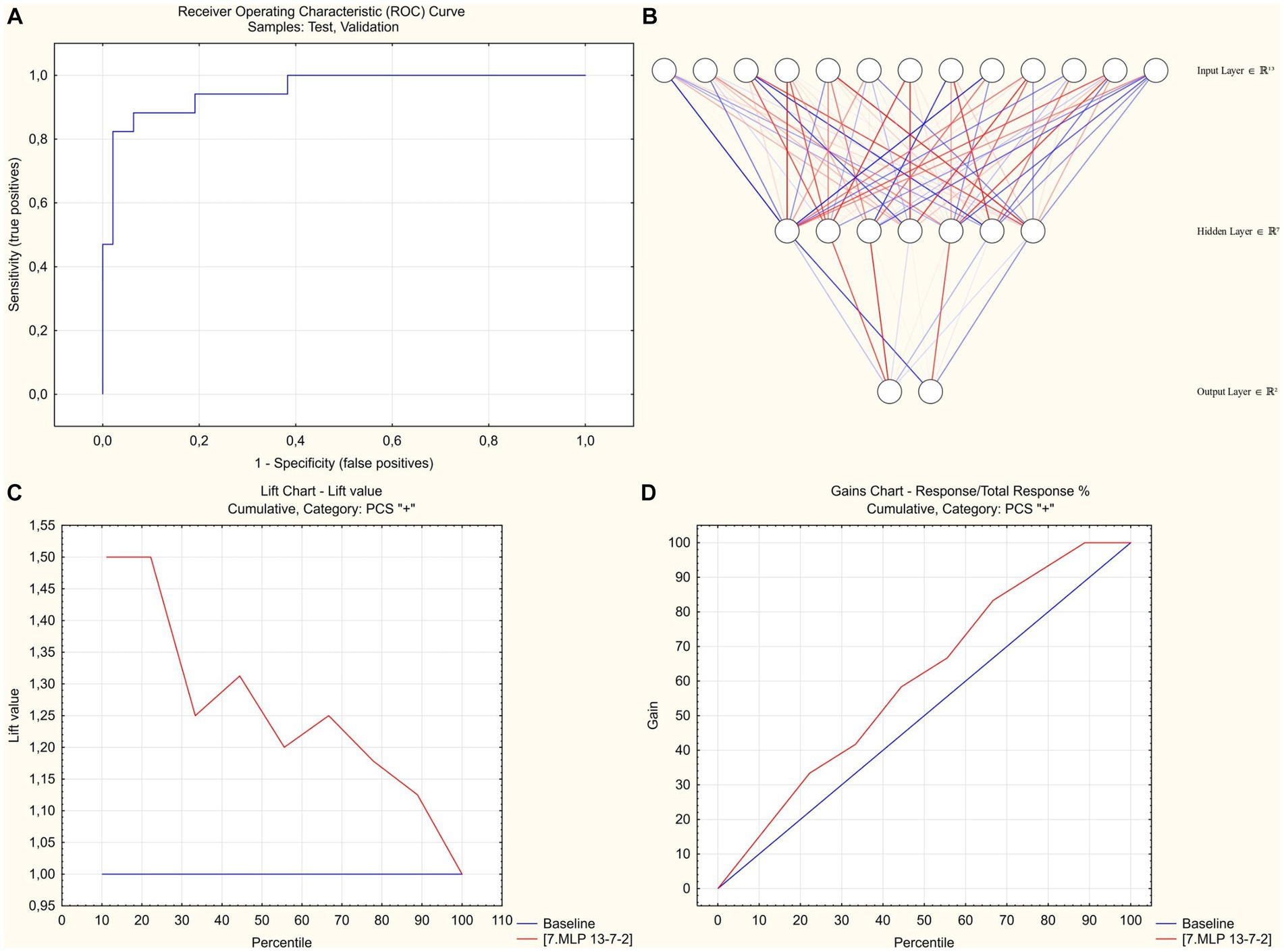
Figure 1. SANN-based classification model to predict post-COVID-19 syndrome at 3 months post-discharge. (A) Receiver operator characteristic analysis. Area under curve = 0,955. (B) 13–7-2 SANN architecture. (C) Lift chart for predicting PCS-positive cases. (D) Gains chart for predicting PCS-positive cases.
4 Discussion
Despite the end of the COVID-19-related public health emergency that was declared by the World Health organization on May 5, 2023, it remains an ongoing global health issue (28). Moreover, asynchronous trends of its spread and non-uniform levels of vaccination worldwide contribute to the possibility of persistence of epidemic process in separate areas, being associated with a potential for selection of new SARS-CoV-2 variants. Another issue of growing concern is the frequent transition of symptomatic COVID-19 to the chronic phase. Moreover, the recent large meta-analysis shows that the unusually high incidence of post-acute sequelae as assessed at 1 to 3 month after onset of disease (6, 12) is translating into a long-lasting trail of impaired health status: 50% of COVID-19 survivors continue to report at least one new symptom 1 year after acute infection, with the most typical manifestations (such as fatigue, dyspnea, psychiatric symptoms, cognitive deficit, memory impairment) having a potential to impose a long-term debilitating effect (29). Thus, despite having hopefully stabilized the acute COVID-19 problem, we are currently facing a new protracted global health challenge presented by the post-COVID-19 syndrome, and the timely identification of patients at risk of its development might be instrumental for subsequent targeted attempts to improve their expected poor trajectory.
The current study was focused on the assessment of pre- and early post-discharge characteristics of hospitalized COVID-19 survivors who presented with ongoing symptoms or a self-reported persistent decline in the general health status at 3 months after discharge. Compared to the patients who have completely recovered by this term, subjects with PCS were characterized by older age, higher BMI, were more frequently female, had higher burden of comorbidities and more intensive inflammatory response during the acute phase, as evidenced by higher values of CRP, ESR, and larger share of patients with very high IL-6 levels. Despite non-inferior physical functional status as assessed by the 6MWT, PCS patients were reporting higher levels of fatigue and dyspnea, which became more pronounced at 1 month post-discharge due to worse dynamics of improvement compared to symptom-free individuals. Considering an insignificant difference in the minimal SpO2 levels between groups, it was most likely the higher level of subjective symptoms that was driving more frequent in-hospital oxygen supplementation in the PCS cohort. Comprehensive TTE has revealed similar changes in both groups that persisted at 1 month and foremost included a high incidence of concentric LV remodeling and grade I diastolic dysfunction; PCS patients were characterized by smaller ventricular cavities pre-discharge and worse diastolic filling at 1 month but higher RV longitudinal strain throughout the period of follow-up.
Compared to the set of predictors of poor physical functional recovery at 1 month post-discharge in the same cohort of patients (30), age, ESR, eGFR, need for in-hospital oxygen supplementation, and pre-discharge 6MWD have retained their prognostic value for predicting the outcome at 3 months, whereas the extent of radiological pulmonary involvement, pre-discharge SpO2, and history of hypertension became insignificant. At the same time, we revealed a subset of additional predictors of PCS that were either irrelevant (sex, height, BMI, SBP) or had not been analyzed at 1 month (RV free wall strain, LV end-diastolic index, and Charlson’s comorbidity index).
Despite the large number of studies that assessed the epidemiology of PCS, the data on its predictors differ according to different sources, and the strength of observed associations is frequently weak (29). Among the variety of potential predictors, the few that have been identified regularly in different studies include older age (31, 32), history of smoking and/or lung disease (31, 33, 34), disease severity (31, 33, 35), longer hospital stay (35, 36), higher levels of CRP (32, 37), severity of symptoms (36), grade of pulmonary CT changes (35, 38), and requirement of in-hospital and post-discharge oxygen support (34, 35, 39), which puts the results of our study in line with the available body of knowledge.
Despite the poor pre-discharge results of 6MWT and evidence of incomplete early physical functional recovery, we did not find these parameters to be independently predictive of preserved self-reported COVID-19 sequelae at 3 months when accounted for sex, age, and anthropometrical parameters, thus confirming the conclusion of Ladlow et al. (40) about the lack of association between the objective functional limitations and the level of residual symptoms in the setting of PCS. We also have not found significant differences in cardiac structure and function both pre-discharge and after a one-month follow-up: patients with and without the PCS seemed to express similar changes that were in line with those reported before (41, 42).
The difficulties in predicting the development of post-COVID-19 syndrome might be related to the heterogenous underlying mechanisms, differences in vaccination status at the population level, and the constantly changing landscape of temporarily and locally prevailing SARS-CoV-2 variants. The literature search has allowed us to identify several finished studies proposing tools for predicting the outcomes of the acute phase of disease (43), effects of post COVID-19 rehabilitation (44), development of post-acute cardiovascular complications (45), and dynamics of the radiological recovery (46). Additionally, we have identified a published protocol of the study directed at solving the problem of predicting the development of the long COVID syndrome (47). To our knowledge, the current study is the first to date presenting a classification model that allows to predict the development of self-reported post-COVID-19 syndrome based on the acute phase parameters in the cohort of hospitalized patients that were shown to be at higher risk of PCS.
Logistic regression analysis is a traditional first line tool to solve the binary classification tasks; at the same time, it is vulnerable to overfitting when using smaller datasets (n < 500) (48). Machine learning approach in our study has allowed to overcome this obstacle; minimization of the input variables number led the training subset to meet the usual sample size requirements for ML projects with a cases-to-predictors ratio of 15.4:1 (49). Post-hoc analysis involving the input dataset effect size (Cohen’s d statistic = 0,684) and the model’s predictive accuracy of 89% additionally confirmed the appropriateness of the sample size (26).
4.1 Limitations of the study
The limitations of our study include possible center-related effects, potential selection bias related to inclusion of less severe cases as a result of inability of persistently O2-dependent patients to participate, negligible level of vaccination in the study population and different prevailing SARS-CoV-2 variants at the time of enrollment compared to today. Hence, the results of the study should be cautiously generalized to the current post-COVID-19 care practice. Given the ever-changing landscape of the acute SARS-CoV-2 infection setting, any types of newly developed predictive tools would require an external validation on the current local cohorts of COVID-19 convalescents as a part of their proper implementation. The proposed model should, therefore, be perceived both as a potential ready-to-use predictive tool and as a proof of concept for the development of similar models based on the more recent data from local populations.
5 Conclusion
Hospitalized patients with SARS-CoV-2 infection were characterized by a 75% prevalence of post-COVID-19 syndrome at 3 months after discharge, with PCS subjects being older, more frequently female, having higher BMI, more intensive acute inflammatory response, and lower eGFR. Higher level of symptoms in the PCS group was not associated with worse physical functional recovery or significant changes on TTE compared to symptoms-free participants. Despite identification of a set of pre-discharge predictors, inclusion of parameters obtained at 1 month proved necessary to obtain a high accuracy ML-based classification model of PCS development; the final list of inputs included age, sex, in-hospital levels of CRP, eGFR and need for oxygen supplementation, and level of post-exertional symptoms at 1 month after discharge (fatigue and dyspnea in 6MWT and MRC dyspnea score).
Data availability statement
The raw data supporting the conclusions of this article will be made available by the authors, without undue reservation.
Ethics statement
The studies involving humans were approved by the Ethical committee of Kharkiv National Medical University. The studies were conducted in accordance with the local legislation and institutional requirements. The participants provided their written informed consent to participate in this study.
Author contributions
OH: Conceptualization, Data curation, Formal analysis, Investigation, Methodology, Software, Writing – original draft, Writing – review & editing. TA: Conceptualization, Supervision, Writing – review & editing. TC: Writing – review & editing. DC: Writing – review & editing. AB: Writing – review & editing. VB: Writing – review & editing. EK: Writing – review & editing. NM: Writing – review & editing. TA: Writing – review & editing. NH: Writing – review & editing. OK: Writing – review & editing. VS: Writing – review & editing.
Funding
The author(s) declare that no financial support was received for the research, authorship, and/or publication of this article.
Acknowledgments
The authors would like to thank M. Yerzina for the technical support in data collection and to acknowledge the work of the hospital staff: O. Morozova, L. Kolisnyk, I. Talalay, V. Kozlov, L. Avdeyeva, who have set conditions for the proper functioning of the clinic.
Conflict of interest
The authors declare that the research was conducted in the absence of any commercial or financial relationships that could be construed as a potential conflict of interest.
The author(s) declared that they were an editorial board member of Frontiers, at the time of submission. This had no impact on the peer review process and the final decision.
Publisher’s note
All claims expressed in this article are solely those of the authors and do not necessarily represent those of their affiliated organizations, or those of the publisher, the editors and the reviewers. Any product that may be evaluated in this article, or claim that may be made by its manufacturer, is not guaranteed or endorsed by the publisher.
Supplementary material
The Supplementary material for this article can be found online at: https://www.frontiersin.org/articles/10.3389/fpubh.2023.1276211/full#supplementary-material
References
1. Covid-19 Excess Mortality Collaborators. Estimating excess mortality due to the COVID-19 pandemic: a systematic analysis of COVID-19-related mortality, 2020-21. Lancet. (2022) 399:1513–36. doi: 10.1016/S0140-6736(21)02796-3
2. Delardas, O, Kechagias, KS, Pontikos, PN, and Giannos, P. Socio-economic impacts and challenges of the coronavirus pandemic (COVID-19): an updated review. Sustainability. (2022) 14:13. doi: 10.3390/su14159699
3. Doroftei, B, Ciobica, A, Ilie, OD, Maftei, R, and Ilea, C. Mini-review discussing the reliability and efficiency of COVID-19 vaccines. Diagnostics (Basel). (2021) 11. doi: 10.3390/diagnostics11040579
4. Worldometers.info. (2023). Delaware, U.S.A.: Dover. Available at: https://www.worldometers.info/coronavirus/worldwide-graphs/ (Accessed March 1, 2023).
5. Astin, R, Banerjee, A, Baker, MR, Dani, M, Ford, E, Hull, JH, et al. Long COVID: mechanisms, risk factors and recovery. Exp Physiol. (2023) 108:12–27. doi: 10.1113/EP090802
6. Gyongyosi, M, Alcaide, P, Asselbergs, FW, Brundel, B, Camici, GG, da Costa, MP, et al. Long COVID and the cardiovascular system - elucidating causes and cellular mechanisms in order to develop targeted diagnostic and therapeutic strategies: a joint scientific statement of the ESC working groups on cellular biology of the heart and Myocardial & Pericardial Diseases. Cardiovasc Res. (2022) 119:336–56. doi: 10.1093/cvr/cvac115
7. Liska, D, Liptakova, E, Babicova, A, Batalik, L, Banarova, PS, and Dobrodenkova, S. What is the quality of life in patients with long COVID compared to a healthy control group? Front Public Health. (2022) 10:975992. doi: 10.3389/fpubh.2022.975992
8. Proal, AD, and VanElzakker, MB. Long COVID or post-acute sequelae of COVID-19 (PASC): an overview of biological factors that May contribute to persistent symptoms. Front Microbiol. (2021) 12:698169. doi: 10.3389/fmicb.2021.698169
9. Kenny, G, Townsend, L, Savinelli, S, and Mallon, PWG. Long COVID: clinical characteristics, proposed pathogenesis and potential therapeutic targets. Front Mol Biosci. (2023) 10:1157651. doi: 10.3389/fmolb.2023.1157651
10. NICE. COVID-19 rapid guideline: managing the long-term effects of COVID-19. COVID-19 rapid guideline: managing the long-term effects of COVID-19. London: National Institute for health and care excellence: Clinical guidelines (2020).
11. Soriano, JB, Murthy, S, Marshall, JC, Relan, P, and Diaz, JV. A clinical case definition of post-COVID-19 condition by a Delphi consensus. Lancet Infect Dis. (2022) 22:e102–7. doi: 10.1016/S1473-3099(21)00703-9
12. Carfi, A, Bernabei, R, Landi, F, and Gemelli Against, C-P-ACSG. Persistent symptoms in patients after acute COVID-19. JAMA. (2020) 324:603–5. doi: 10.1001/jama.2020.12603
13. Fugazzaro, S, Contri, A, Esseroukh, O, Kaleci, S, Croci, S, Massari, M, et al. Rehabilitation interventions for post-acute COVID-19 syndrome: a systematic review. Int J Environ Res Public Health. (2022) 19:24. doi: 10.3390/ijerph19095185
14. Reinert, G, Muller, D, Wagner, P, Martinez-Pozas, O, Cuenca-Zaldivar, JN, Fernandez-Carnero, J, et al. Pulmonary rehabilitation in SARS-CoV-2: a systematic review and Meta-analysis of post-acute patients. Diagnostics. (2022) 12:20. doi: 10.3390/diagnostics12123032
15. Chen, H, Shi, H, Liu, X, Sun, T, Wu, J, and Liu, Z. Effect of pulmonary rehabilitation for patients with post-COVID-19: a systematic review and meta-analysis. Front Med. (2022) 9:837420. doi: 10.3389/fmed.2022.837420
16. World Health Organization (WHO). COVID-19 clinical management: living guidance, (2021). WHO. Available at: https://www.who.int/publications/i/item/WHO-2019-nCoV-clinical-2021-1 (Acessed January 25, 2021).
17. Lang, RM, Badano, LP, Mor-Avi, V, Afilalo, J, Armstrong, A, Ernande, L, et al. Recommendations for cardiac chamber quantification by echocardiography in adults: an update from the American society of echocardiography and the European association of cardiovascular imaging. Eur Heart J Cardiovasc Imaging. (2015) 16:233–71. doi: 10.1093/ehjci/jev014
18. Nagueh, SF, Smiseth, OA, Appleton, CP, Byrd, BF 3rd, Dokainish, H, Edvardsen, T, et al. Recommendations for the evaluation of left ventricular diastolic function by echocardiography: an update from the American Society of Echocardiography and the European association of cardiovascular imaging. J Am Soc Echocardiogr. (2016) 29:277–314. doi: 10.1016/j.echo.2016.01.011
19. Rudski, LG, Lai, WW, Afilalo, J, Hua, L, Handschumacher, MD, Chandrasekaran, K, et al. Guidelines for the echocardiographic assessment of the right heart in adults: a report from the American society of echocardiography endorsed by the european association of echocardiography, a registered branch of the European society of cardiology, and the Canadian society of echocardiography. J Am Soc Echocardiogr. (2010) 23:685–713. doi: 10.1016/j.echo.2010.05.010
20. Stoylen, A, Molmen, HE, and Dalen, H. Relation between mitral annular plane systolic excursion and global longitudinal strain in normal subjects: the HUNT study. Echocardiography. (2018) 35:603–10. doi: 10.1111/echo.13825
21. Stoylen, A, Molmen, HE, and Dalen, H. Left ventricular global strains by linear measurements in three dimensions: interrelations and relations to age, gender and body size in the HUNT study. Open Heart. (2019) 6:e001050. doi: 10.1136/openhrt-2019-001050
22. Aurich, M, Fuchs, P, Muller-Hennessen, M, Uhlmann, L, Niemers, M, Greiner, S, et al. Unidimensional longitudinal strain: a simple approach for the assessment of longitudinal myocardial deformation by echocardiography. J Am Soc Echocardiogr. (2018) 31:733–42. doi: 10.1016/j.echo.2017.12.010
23. Laboratories ATSCoPSfCPF. ATS statement: guidelines for the six-minute walk test. Am J Respir Crit Care Med. (2002) 166:111–7. doi: 10.1164/ajrccm.166.1.at1102
24. Duncan, MJ, Mota, J, Carvalho, J, and Nevill, AM. An evaluation of prediction equations for the 6 minute walk test in healthy European adults aged 50-85 years. PLoS One. (2015) 10:e0139629. doi: 10.1371/journal.pone.0139629
25. Tanaka, H, Monahan, KD, and Seals, DR. Age-predicted maximal heart rate revisited. J Am Coll Cardiol. (2001) 37:153–6. doi: 10.1016/S0735-1097(00)01054-8
26. Rajput, D, Wang, WJ, and Chen, CC. Evaluation of a decided sample size in machine learning applications. BMC Bioinform. (2023) 24:48. doi: 10.1186/s12859-023-05156-9
27. Honchar, O, and Ashcheulova, T. Short-Term Echocardiographic Follow-Up After Hospitalization for COVID-19: A Focus on Early Post-Acute Changes. Front Cardiovasc Med. (2023) 10:1250656. doi: 10.3389/fcvm.2023.1250656
28. World Health Organization. Statement on the fifteenth meeting of the IHR (2005) emergency committee on the COVID-19 pandemic. (2023). Available at: https://www.who.int/news/item/05-05-2023-statement-on-the-fifteenth-meeting-of-the-international-health-regulations-(2005)-emergency-committee-regarding-the-coronavirus-disease-(covid-19)-pandemic (Accessed May 20, 2023).
29. Zeng, N, Zhao, YM, Yan, W, Li, C, Lu, QD, Liu, L, et al. A systematic review and meta-analysis of long term physical and mental sequelae of COVID-19 pandemic: call for research priority and action. Mol Psychiatry. (2023) 28:423–33. doi: 10.1038/s41380-022-01614-7
30. Honchar, O, and Ashcheulova, T. Spontaneous physical functional recovery after hospitalization for COVID-19: insights from a 1 month follow-up and a model to predict poor trajectory. Front Med. (2023) 10:1212678. doi: 10.3389/fmed.2023.1212678
31. Huang, C, Huang, L, Wang, Y, Li, X, Ren, L, Gu, X, et al. 6-month consequences of COVID-19 in patients discharged from hospital: a cohort study. Lancet. (2021) 397:220–32. doi: 10.1016/S0140-6736(20)32656-8
32. Zhao, YM, Shang, YM, Song, WB, Li, QQ, Xie, H, Xu, QF, et al. Follow-up study of the pulmonary function and related physiological characteristics of COVID-19 survivors three months after recovery. EClinicalMedicine. (2020) 25:100463. doi: 10.1016/j.eclinm.2020.100463
33. Liu, D, Baumeister, RF, Veilleux, JC, Chen, C, Liu, W, Yue, Y, et al. Risk factors associated with mental illness in hospital discharged patients infected with COVID-19 in Wuhan. China Psychiatry Res. (2020) 292:113297. doi: 10.1016/j.psychres.2020.113297
34. Bellan, M, Soddu, D, Balbo, PE, Baricich, A, Zeppegno, P, Avanzi, GC, et al. Respiratory and psychophysical sequelae among patients with COVID-19 four months after hospital discharge. JAMA Netw Open. (2021) 4:e2036142. doi: 10.1001/jamanetworkopen.2020.36142
35. Wu, X, Liu, X, Zhou, Y, Yu, H, Li, R, Zhan, Q, et al. 3-month, 6-month, 9-month, and 12-month respiratory outcomes in patients following COVID-19-related hospitalisation: a prospective study. Lancet Respir Med. (2021) 9:747–54. doi: 10.1016/S2213-2600(21)00174-0
36. Xiong, Q, Xu, M, Li, J, Liu, Y, Zhang, J, Xu, Y, et al. Clinical sequelae of COVID-19 survivors in Wuhan, China: a single-Centre longitudinal study. Clin Microbiol Infect. (2021) 27:89–95. doi: 10.1016/j.cmi.2020.09.023
37. Weng, J, Li, Y, Li, J, Shen, L, Zhu, L, Liang, Y, et al. Gastrointestinal sequelae 90 days after discharge for COVID-19. Lancet Gastroenterol Hepatol. (2021) 6:344–6. doi: 10.1016/S2468-1253(21)00076-5
38. Frija-Masson, J, Debray, MP, Gilbert, M, Lescure, FX, Travert, F, Borie, R, et al. Functional characteristics of patients with SARS-CoV-2 pneumonia at 30 days post-infection. Eur Respir J. (2020) 56:2001754. doi: 10.1183/13993003.01754-2020
39. Mandal, S, Barnett, J, Brill, SE, Brown, JS, Denneny, EK, Hare, SS, et al. 'Long-COVID': a cross-sectional study of persisting symptoms, biomarker and imaging abnormalities following hospitalisation for COVID-19. Thorax. (2021) 76:396–8. doi: 10.1136/thoraxjnl-2020-215818
40. Ladlow, P, O'Sullivan, O, Houston, A, Barker-Davies, R, May, S, Mills, D, et al. Dysautonomia following COVID-19 is not associated with subjective limitations or symptoms but is associated with objective functional limitations. Heart Rhythm. (2022) 19:613–20. doi: 10.1016/j.hrthm.2021.12.005
41. Ingul, CB, Grimsmo, J, Mecinaj, A, Trebinjac, D, Berger Nossen, M, Andrup, S, et al. Cardiac dysfunction and arrhythmias 3 months after hospitalization for COVID-19. J Am Heart Assoc. (2022) 11:e023473. doi: 10.1161/JAHA.121.023473
42. Ovrebotten, T, Myhre, P, Grimsmo, J, Mecinaj, A, Trebinjac, D, Nossen, MB, et al. Changes in cardiac structure and function from 3 to 12 months after hospitalization for COVID-19. Clin Cardiol. (2022) 45:1044–52. doi: 10.1002/clc.23891
43. Rasmy, L, Nigo, M, Kannadath, BS, Xie, Z, Mao, B, Patel, K, et al. Recurrent neural network models (CovRNN) for predicting outcomes of patients with COVID-19 on admission to hospital: model development and validation using electronic health record data. Lancet Digit Health. (2022) 4:e415–25. doi: 10.1016/S2589-7500(22)00049-8
44. Adamo, S, Ambrosino, P, Ricciardi, C, Accardo, M, Mosella, M, Cesarelli, M, et al. A machine learning approach to predict the rehabilitation outcome in convalescent COVID-19 patients. J Pers Med. (2022) 12:12. doi: 10.3390/jpm12030328
45. Gupta, A, Jain, V, and Singh, A. Stacking ensemble-based intelligent machine learning model for predicting post-COVID-19 complications. N Gener Comput. (2022) 40:987–1007. doi: 10.1007/s00354-021-00144-0
46. Sonnweber, T, Tymoszuk, P, Sahanic, S, Boehm, A, Pizzini, A, Luger, A, et al. Investigating phenotypes of pulmonary COVID-19 recovery: a longitudinal observational prospective multicenter trial. elife. (2022) 11:11. doi: 10.7554/eLife.72500
47. Daines, L, Mulholland, RH, Vasileiou, E, Hammersley, V, Weatherill, D, Katikireddi, SV, et al. Deriving and validating a risk prediction model for long COVID-19: protocol for an observational cohort study using linked Scottish data. BMJ Open. (2022) 12:e059385. doi: 10.1136/bmjopen-2021-059385
48. Bujang, MA, Sa'at, N, Sidik, T, and Joo, LC. Sample size guidelines for logistic regression from observational studies with large population: emphasis on the accuracy between statistics and parameters based on real life clinical data. Malays J Med Sci. (2018) 25:122–30. doi: 10.21315/mjms2018.25.4.12
49. Baeza-Delgado, C, Cerda Alberich, L, Carot-Sierra, JM, Veiga-Canuto, D, de Las, M, Heras, B, et al. A practical solution to estimate the sample size required for clinical prediction models generated from observational research on data. Eur Radiol Exp. (2022) 6:10. doi: 10.1186/s41747-022-00276-y
Keywords: COVID-19, hospitalization, convalescence, post-acute COVID-19 syndrome, walk test, echocardiography, clinical decision rules, machine learning
Citation: Honchar O, Ashcheulova T, Chumachenko T, Chumachenko D, Bobeiko A, Blazhko V, Khodosh E, Matiash N, Ambrosova T, Herasymchuk N, Kochubiei O and Smyrnova V (2023) A prognostic model and pre-discharge predictors of post-COVID-19 syndrome after hospitalization for SARS-CoV-2 infection. Front. Public Health. 11:1276211. doi: 10.3389/fpubh.2023.1276211
Edited by:
Natalia Kryvinska, Comenius University, SlovakiaReviewed by:
Ana Afonso, NOVA University of Lisbon, PortugalDávid Líška, Matej Bel University, Slovakia
Copyright © 2023 Honchar, Ashcheulova, Chumachenko, Chumachenko, Bobeiko, Blazhko, Khodosh, Matiash, Ambrosova, Herasymchuk, Kochubiei and Smyrnova. This is an open-access article distributed under the terms of the Creative Commons Attribution License (CC BY). The use, distribution or reproduction in other forums is permitted, provided the original author(s) and the copyright owner(s) are credited and that the original publication in this journal is cited, in accordance with accepted academic practice. No use, distribution or reproduction is permitted which does not comply with these terms.
*Correspondence: Oleksii Honchar, b3YuaG9uY2hhckBrbm11LmVkdS51YQ==