- Department of Agricultural and Environmental Sciences (DiSAAT), Research Unit of “Genetics and Plant Biotechnology”, University of Bari Aldo Moro, Bari, Italy
Wheat grain protein content (GPC) and yield components are complex quantitative traits influenced by a multi-factorial system consisting of both genetic and environmental factors. Although seed storage proteins represent less than 15% of mature kernels, they are crucial in determining end-use properties of wheat, as well as the nutritional value of derived products. Yield and GPC are negatively correlated, and this hampers breeding programs of commercially valuable wheat varieties. The goal of this work was the evaluation of genetic variability for quantity and composition of seed storage proteins, together with yield components [grain yield per spike (GYS) and thousand-kernel weight (TKW)] in a durum wheat population obtained by an inter-specific cross between a common wheat accession and the durum cv. Saragolla. Quantitative trait loci (QTL) analysis was conducted and closely associated markers identified on a genetic map composed of 4,366 SNP markers previously obtained in the same durum population genotyped with the 90K iSelect SNP assay. A total of 22 QTL were detected for traits related to durum wheat quality. Six genomic regions responsible for GPC control were mapped on chromosomes 2B, 3A, 4A, 4B, 5B, and 7B, with major QTL on chromosomes 2B, 4A, and 5B. Nine loci were detected for GYS: two on chromosome 5B and 7A and one on chromosomes 2A, 2B, 4A, 4B, 7B, with the strongest QTL on 2B. Eight QTL were identified for TKW, three of which located on chromosome 3A, two on 1B and one on 4B, 5A, and 5B. Only small overlapping was found among QTL for GYS, TKW, and GPC, and increasing alleles coming from both parents on different chromosomes. Good candidate genes were identified in the QTL confidence intervals for GYS and TKW.
Introduction
Mediterranean countries rely heavily on cereal production as main commodity crop of economic importance. Amongst cereals, durum wheat is the leading commercial crop as its consumption is the highest amongst Mediterranean countries. Given the always growing consumers' attention to health, objectives of wheat breeding programs are recently focusing not only on increasing productivity, but also on endowing derived products with a higher nutritional value (Battenfield et al., 2016). Wheat quality is a very complex issue including several components (Marcotuli et al., 2015). Usually, kernel protein quantity and quality are considered key factors of "wheat quality." Wheat quality, that is its ability to be processed into derived foods, is largely determined both by protein quantity—measured as grain protein content (GPC), and by protein quality—given by protein composition. Wheat proteins show high complexity and different interaction with each other, thus making them difficult to characterize. Wheat grain storage proteins are a complex mix of different polypeptide chains traditionally classified according to their solubility (following the sequential Osborne extraction procedure) or composition and structure (Zilic et al., 2011). Grain storage proteins include gliadin, glutenin, albumin, and globulin. In particular, gluten proteins that is gliadins and glutenins, give wheat flour unique extensibility and processing properties and consequently a good quality to derived products. Gluten is made up by proteins able to form a cohesive and visco-elastic dough by mixing flour with water. Differences in wheat processing properties are due to gluten quantity and composition. According to their solubility in aqueous alcoholic solutions, gluten proteins can be divided into soluble gliadins and insoluble glutenins. Gliadins are monomeric proteins responsible for gluten viscosity. Glutenins are polipeptidic aggregates responsible for dough strength and elasticity. After reduction of inter-molecular disulfide bonds, glutenin monomers are classified into high molecular weight (HMW) and low molecular weight (LMW) subunits (Payne et al., 1982; Jackson et al., 1983). Glutenin chains have high molecular weights up to tens of millions (Wrigley, 1996). Differences in glutenin subunits strongly influence end-use quality (Kasarda, 1999), in fact when managing the protein quality, one of the main aim of breeding programs is to select wheat varieties or lines that possess glutenin alleles associated with superior processing characteristics.
Wheat storage proteins are encoded by gene families with highly variable alleles. Gliadins are encoded by complex loci located on the short arms of homoeologous group 1 chromosomes (Gli-A1, Gli-B1, Gli-D1 for omega and gamma) and 6 (Gli-A2, Gli-B2, Gli-D2 for alpha- and beta-) (Shewry et al., 2003). For what concerns gutenins, LMW-GS derive from loci (Glu-3) on the short arms of group 1 chromosome (linked to Gli-1 loci), whereas HMW-GS are coded by complex loci on the group 1 long arms (Glu-1). Each Glu-1 locus contains two tightly linked paralogous genes encoding two different types of HMW-GS, namely the "x-" and a "y-" type, based on their electrophoretic mobility, with a wide combination of different alleles at each locus responsible for high polymorphism.
Hexaploid wheat contains six HMW-GS genes (Glu-1) located on 1A, 1B, and 1D chromosomes, whereas tetraploid wheat accounts for four HMW-GS loci on 1A and 1B. In hexaploid wheat there are five classes of HMW-GS proteins: 1Ax (encoded by Glu-A1),1Bx and 1By (encoded by Glu-B1), 1Dx and 1Dy (encoded by Glu-D1). The y-type genes at the Glu-A1 locus are missing in hexaploid wheat (Galdi and Feldman, 1983). HMW glutenin-subunits from 1D are lacking in durum wheat. In hexaploid wheat, the 1Bx, 1Dx, and 1Dy HMW-GS are constitutively present in all cultivars, whereas 1Ay genes are always silent.
Gliadin and glutenins contribute together to determine wheat dough quality. In particular, the presence of some HMW-GS is crucial for the determination of wheat quality and its attitude to make pasta or bread (Jang et al., 2017). Noteworthy, 80% of the tetraploid genotypes miss the HMW-GS encoded by Glu-A1 locus, with the consequence to be unsuitable for bread making quality (Branlard et al., 1989), in fact durum wheat is usually used to making pasta.
By influencing both the nutritional value and the processing properties of flour/semolina, GPC deeply contributes to determine the economic value of the crop. GPC is a typical quantitative trait controlled by several genes located on almost all chromosomes and influenced by both environment and management practices. As mature wheat grains usually contain 8 to 20% of proteins (Zilic et al., 2011), one of the main objectives of breeders is to identify stable QTL and relative favorable alleles, which can be successfully transferred from high-GPC donor lines to low-GPC varieties.(Kumar et al., 2018). However, reaching of this goal is hampered by the quantitative nature of the trait, which is controlled by multiple genes and strongly influenced by environmental factors and agronomical practices (Blanco et al., 2012). In fact, heritability for GPC has been reported to range from 0.41 (Kramer, 1979) to 0.70 (Suprayogi et al., 2009) depending on genotypes, location of field trials, and computational analysis.
Protein quality and quantity have always been among the crucial topics of wheat breeding. However, the increase of grain protein content is also complicated by its negative association with productivity, the strong environmental influence and the very narrow genetic variation found within the cultivated gene pool (Barneix, 2007; Iqbal et al., 2016). Modern wheat varieties are relatively low in GPC compared to their wild relatives, which could be employed as donors of useful alleles (Avivi, 1978; Nevo et al., 2002; Cakmak et al., 2004; Blanco et al., 2006; Chatzav et al., 2010). For example, wild emmer (Triticum turgidum ssp. dicoccoides) has a wide genotypic variation in several agronomic traits, including grain constituents (Uauy et al., 2006) and abiotic/biotic stress tolerance (Peleg et al., 2005; Ben-David et al., 2010). For this reason, the high genetic variability in the gene pool of wild emmer can be exploited, by crossing with durum wheat, for the improvement of several agronomic and economical important traits. However, its very low yield complicates wheat breeding for yield components.
Improvement of GPC by means of classical breeding methods have always given poor results. Recently, the release of genome sequences and comparative studies with model species (Colasuonno et al., 2013), and the consequent availability of advanced genetic tools such as linkage maps and molecular markers has fasten the improvement of GPC especially thanks to the application of marker-assisted selection (MAS) programs. Genetic factors and candidate genes influencing protein concentration in cultivated and wild wheats are located on all chromosomes. A recent review by Kumar et al. (2018) reported 49 QTL mapping publications with a total of 325 main effect QTL and 42 epistatic QTL for GPC.
Given the complexity of GPC genetic control and the existence of a very narrow genetic variation within cultivated gene pool, the improvement of this trait has always been a challenge for genetic breeders. Biodiversity is becoming an increasingly important issue in agriculture. Significant research of new valuable germplasm, breeding efforts, and subsequent plant multiplication are needed to improve the performance of cereal sector through better-suited varieties. It is fundamental continuing to achieve successful genotypes and to guarantee creation of new genetic diversity to allow a continued genetic gain in a dynamic agricultural environment.
Together with grain protein content, wheat yield is another complex agronomic trait resulting from the interaction of several components which are deeply influenced by environment. Understanding the molecular mechanisms underlying yield-related genes is one of the main objective of breeders. Several QTL have been mapped for some components such as thousand kernel weight, grain weight per spike, tiller number etc. (Huang et al., 2004; Narasimhamoorthy et al., 2006; Zhang et al., 2012), and some functional markers have been developed for thousand-kernel weight (TKW) (Su et al., 2011). However, gene isolation by map-based cloning is hampered by the hugeness and complexity of wheat genome, thus only few genes have been isolated through comparative genetics based on gene synteny (Su et al., 2011; Yang et al., 2012).
In the present work, an inter-specific cross between hexaploid and tetraploid wheat was used to map QTL for GPC and yield-related traits [grain yield per spike (GYS) and TKW], and identify putative candidate genes for the most associated markers. Moreover, the use of an accession of bread wheat as donor of useful genes for durum wheat, allowed to survey new genetic variability for grain quantity and composition, and identify new high quality lines useful in durum breeding programs.
Materials and Methods
Genetic Materials and Field Experiments
A total of 135 recombinant inbred lines (RILs) were obtained by the interspecific cross between the elite durum wheat cultivar Saragolla and the bread wheat accession 02-5B-318 (derived from the Chinese cv. Sumai-3) by advancing single F2 plants to the F7 by single seed descent (SSD) (Giancaspro et al., 2016). The cross produced two RIL populations: a sub-set of RILs were classified as hexaploid as carrying all the D chromosomes, and a sub-set of 135 durum RILs consisted of lines with no D chromosomes. In this work, only the durum RIL was taken into consideration for the study of grain protein content and yield-related traits The tetraploid lines were harvested, and seeds used for replicated field trials and DNA extraction. The parental lines for this interspecific cross were selected because different for several traits including yield components and protein content. The parents and the 135 RILs were evaluated for grain protein content and yield in replicated field trials at the location of Valenzano Bari-Italy for three years (2015–2017). The RILs were evaluated using a randomized complete block design with four replications. Each plots consisted of 1-m rows, 30 cm apart, with 80 seeds sown in each plot and supplemented with nitrogen (10 g/m2). Grain protein content was determined on a 2 gsample of whole meal flour by near-infrared reflectance (NIR) spectroscopy using a SpectraAlyzer device (Basic model, Zeuton). The instrument was calibrated by using a set of 25 whole-meal flour samples belonging to T. turgidum ssp. durum, ssp. dicoccum, and ssp. dicoccoides with known protein concentration and moisture, previously calculated according to official standard methods. Final GPC was expressed as a percentage of proteins on a dry weight basis. On each wheat line, GPC measurement was performed twice then the final value was averaged between the two technical replicates. GYS was measured as the total grain yield per row on the number of spikes per row (about 70–80 spikes). TKW was assessed from a 15 g seed sample per each plot (line). TKW of each wheat line was determined as the average of two technical replicates.
HMW-GS and gliadins analyses were performed as described by the method of Payne et al. (1980) and by Bushuk and Zillman (1978), respectively. The cv Chinese Spring of bread wheat and cv Svevo of durum wheat, containing known HMW-GS, were used as standards. HMW-glutenins composition was scored according to Payne's catalogue (Payne and Lawrence, 1983) that named HMW glutenins gene loci as Glu-A1, Glu-1B, and Glu-1D and proteins subunits as 0, 1, 2*, 2 + 12, 5 + 10, 6 + 8, 7 + 9, and 17 + 18.
Genetic Map and QTL Analysis
A durum wheat genetic linkage map obtained by Giancaspro et al. (2016) was used for QTL analysis. The map, covering a total length of 4,227.37 cM, consists of 4,366 SNPs surveyed from the 81,587 sequences of the 90K iSelect array by Illumina CSProR (SanDiego, CA, USA) described by Wang et al. (2014).
ANOVA was conducted for each trait with standard procedures using X-Stat software and genetic variance (σ2G) and broad-sense heritability (h2B) calculated using the variance component estimates. Pearson phenotypic correlation coefficients were calculated by using M-STAT-C software between GPC, GYS, and TKW. The Inclusive Composite Interval Mapping (ICIM) method (Li et al., 2007) was used for QTL mapping with QGene 4.0 software (Joehanes and Nelson, 2008). Scanning interval of 2 cM between markers, and putative QTL with a window size of 10 cM was used for QTL detection. The number of marker cofactors for background control was set by forward regression with a maximum of five controlling markers. Putative QTL were defined as two or more linked markers associated with a trait at LOD ≥3. Positive and negative signs of the estimates QTL effect indicate the contribution of cv Saragolla and the 02-5B-318 accession, respectively. The phenotypic variance explained by each single QTL was quantified by the square of the partial correlation coefficient (R2). Graphical representation of linkage groups and QTL was obtained with MapChart 2.2 Software (Voorrips, 2002).
HMW-GS Analysis
Wheat grains were grinded with porcelain mortar and pestle and boiled for 5' in an extraction buffer - ratio 10:1 (µl/g)—consisting of 0.4 ml β-mercaptoethanol, 4 ml pure water, and 1.7 ml γ-piromin dye (15 mg γ piromin; 2.0 g 100% SDS; 6.25 ml Tris-Hcl, pH 6.8; 10.5 ml water). β-mercaptoethanol served for the reduction of intermolecular disulphide bonds between glutenin subunits, SDS for protein denaturation, and γ-piromin for the visualization of protein bands on electrophoretic gel. Samples were incubated 2 h at room temperature, then centrifuged to recover supernatant. Electrophoresis was performed in SDS-PAGE with 4% stacking gel (pH 6.8) and 10% separating gel (pH8.8) for 24 h at 500 Volts, in a1X running buffer (Tris-SDS-Glycin, pH 8.3). Gels were left 24 h in a dying solution of Comassie Blue R250 composed by 12% tricloroacetic acid and 1%Comassie Brilliant Blue (19:1, v/v), then rinsed overnight with distilled water and visualized on UV. Common wheat cv. Chinese Spring and durum wheat cv. Svevo, with known HMW-GS, were used as standards.
Gliadins Analysis
Gliadin extraction was performed on 30 µg of single-seed flour by following the protocol described in Bushuk and Zillman (1978). 100 µl of 70% ethanol were added to flour and incubated at 24°C for 1 h with brief vortexing at 10 min intervals. Supernatant was collected by centrifuging tubes at 12,000 rpm for 10 min at room temperature. Then, 26 µl of gel buffer was added to 20 µl of supernatant and loaded onto the gel for PAGE separation. Details on gel preparation and run are described in Bushuk and Zillman (1978). The electrophoregrams were evaluated based on gliadin relative mobility.
Candidate Gene Analysis
In order to assign genes to SNPs, sequences corresponding to all the SNP markers mapping in the confidence intervals of QTL for GPC, GYS and TKW were used in a BLAST search using TBLASTX algorithm (http://ncbi.nlm.nih.gov/BLAST), against the wheat draft genome sequence of the tetraploid dicoccoides accession Zavitan (Avni et al., 2017), the durum cv. Svevo (Maccaferri et al., 2019), and the hexaploid Chinese Spring (Appels et al., 2018) in order to assign a putative function to SNPs, and identify candidate genes (CGs) for the traits of interest. Alignments with at least 80% identity and E-value >10-7 were considered, and the corresponding putative genes evaluated for their involvement in metabolic pathways related to protein content and yield components.
Molecular Structure (Exons/Introns) and Function (Corresponding Coding Protein) Was Determined for the Most Associated CG to TWK QTL: Elongation Factor (EF) Gene. Comparison Was Carried on Among Diploid (Triticum Urartu), Tetraploid (T. Durum, Cv. Svevo, T. Dicoccoides), and Hexaploid (T. Aestivum, Cv. Chinese Spring) Wheats. Molecular Characterization Was Obtained by Browsing the Corresponding SNP Sequence (IWB30162, Excalibur_Rep_C106003_475) in the Svevo Genome Browser at: https://Interomics.Eu/Durum-Wheat-Genome and in Plant Ensemble Databases (https://Plants.Ensembl.Org).
Results
Field Traits Analysis
GYS and TKW were evaluated at Valenzano (Bari) in southern Italy for three years (2015, 2016, 2017). Table 1 reports the mean value and the range of GPC and yield components for parents Saragolla and 02-5B-318 and for RI lines, together with variance components and broad-sense heritability estimates, in each of the three environments. GPC values were different between the two parents both in Valenzano 2015, 2016, and 2017, with a mean value of 14.23 for cv Saragolla and 13.56 for 02-5B-318. As expected for a typical quantitative trait, GPC values showed a broad variability across the RI lines in each of the three environments, in fact the phenotypic means of RILs were distributed across a normal curve (Figure 1). The RIL population means (16.4, 16.7, and 15.0 for the three years, respectively), were significantly higher of both mid-parental values and with a minimum value of 12.8 and a maximum value of 22.10 recorded at Valenzano 2015. Differences in mean values and variances of parental lines and RI population observed in the different locations, were very likely due to the different environmental factors. Broad sense heritability (genotype mean basis) of GPC ranged from 0.47 to 0.48 in the three environments.
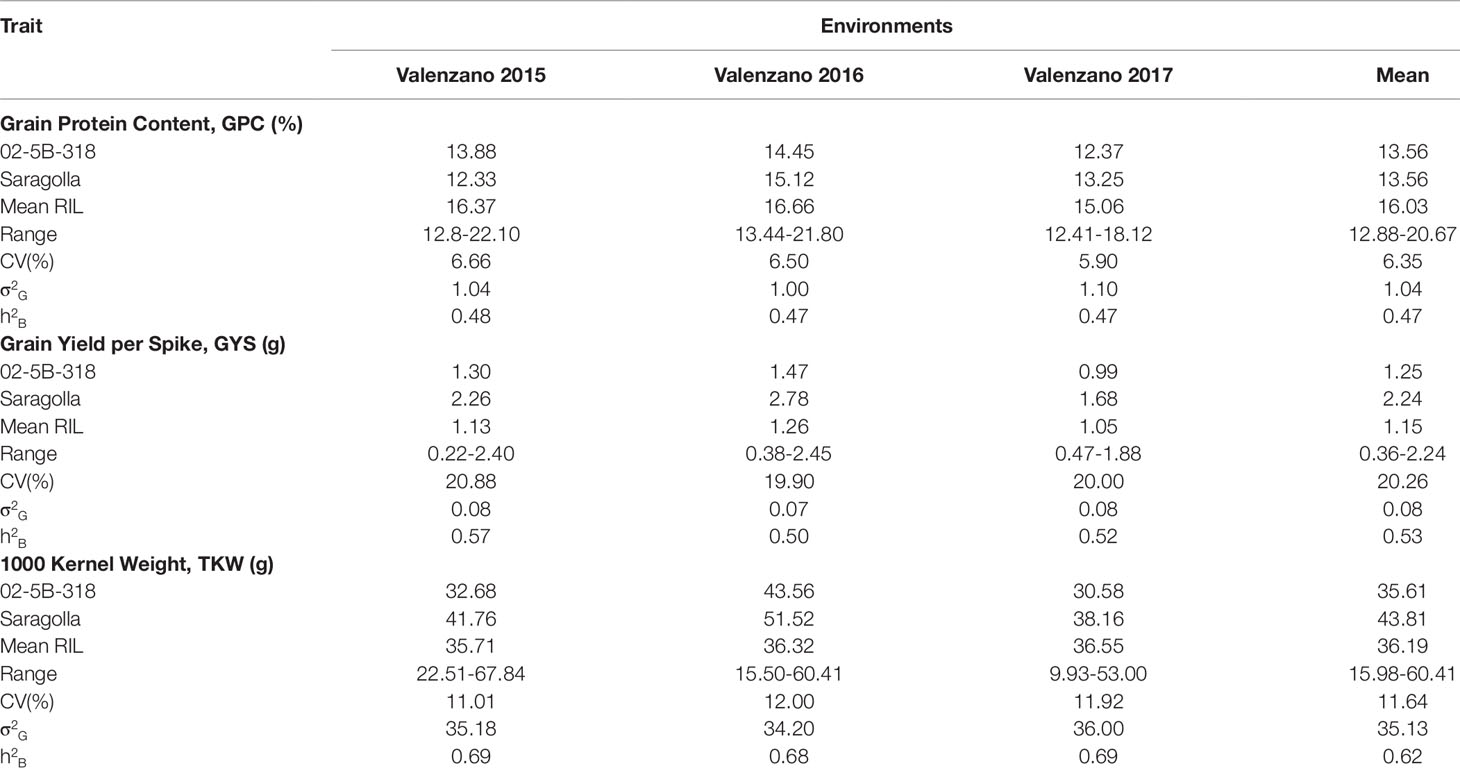
Table 1 Means, ranges, coefficients of variation (CV), genetic variance (σ2G), and heritability (h2B) of grain protein content and grain yield components in the Saragolla x 02-5B-318 RIL population and parental lines, evaluated in three environments.
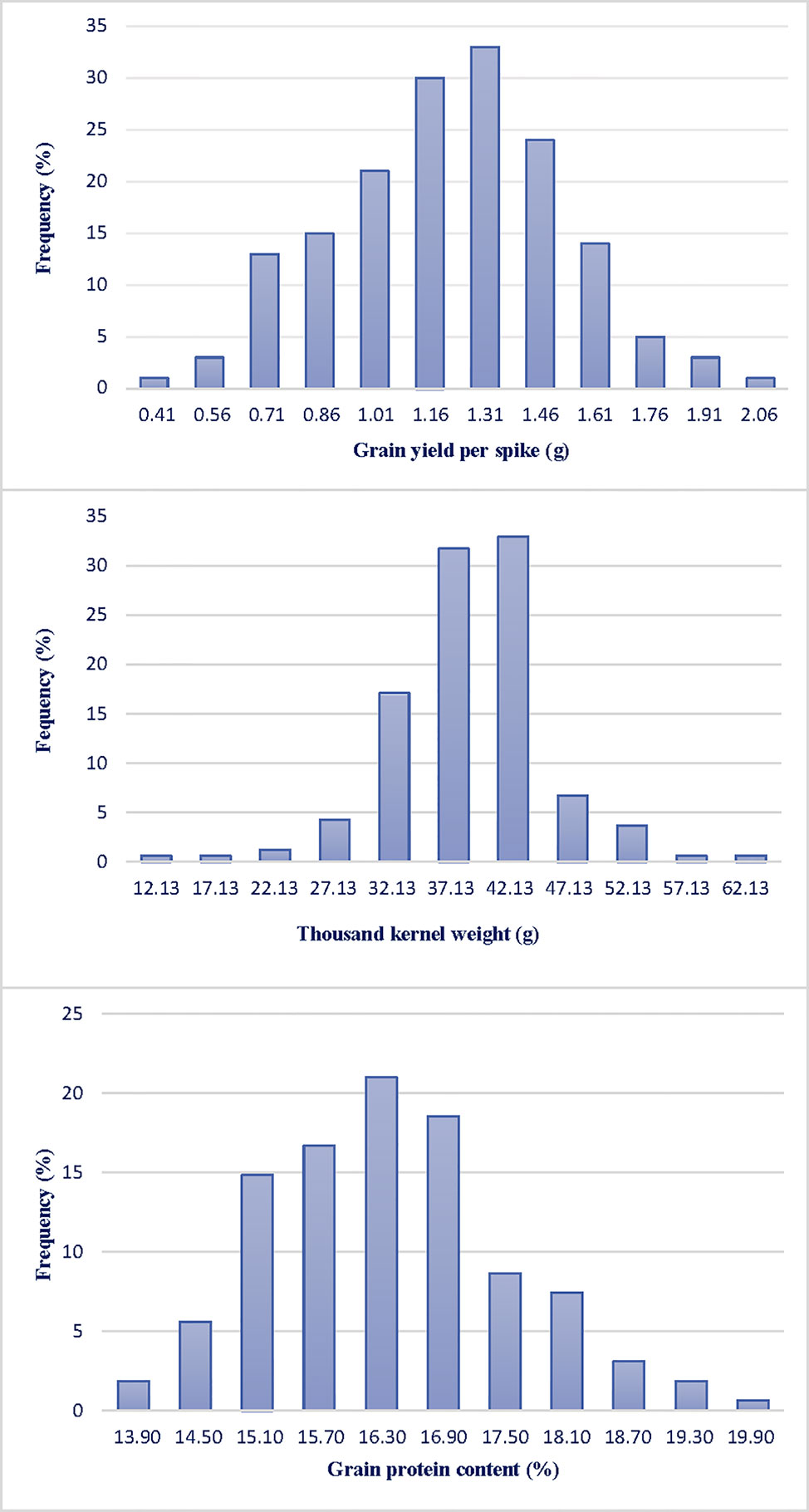
Figure 1 Frequency distribution of grain yield per spike (GYS), thousand kernel weight (TKW) and grain protein content (GPC) in a RIL population derived by the cross between the durum cv Saragolla and the bread wheat accession 02-5B-318. Traits were evaluated at Valenzano (Bari, southern Italy) for three years (2015, 2016, 2017); the mean value of the three years are presented.
Saragolla and 02-5B-318 showed a significant difference also for the yield components GYS and TKW; consequently, a large variation was observed for these two traits in the RIL population in all the three environments. The durum cv. Saragolla showed higher values in each environment for both traits: in particular, the mean values for GYS and TKW were 2.24 and 43.81 g, respect to 1.14 and 35.60 g of the same traits in 02-5B-318 parent. Interestingly, for both GYS and TKW, the trait variation was much larger in the RILs population than between the parental lines, with lowest values of 0.22 and 9.93 g and highest values of 2.45 and 67.84, for GYS and TKW, respectively, which suggested the presence of favorable alleles increasing the trait in both parents. For example, in all the three environments, the difference between the highest and the lowest value of GYS in the RI lines was more than twice the difference between the two parents. Heritability across environments was high for both GYS (mean value of 0.53) and TKW (mean value of 0.69).
As expected, in the present work we found a negative correlation between GPC and yield components (Table 2). In particular, GPC resulted negatively correlated with GYS (r values ranging from -0.09 to -0.16) in all environments, and with 1,000 kernel weight only at Valenzano 2017 (r = -1.00).
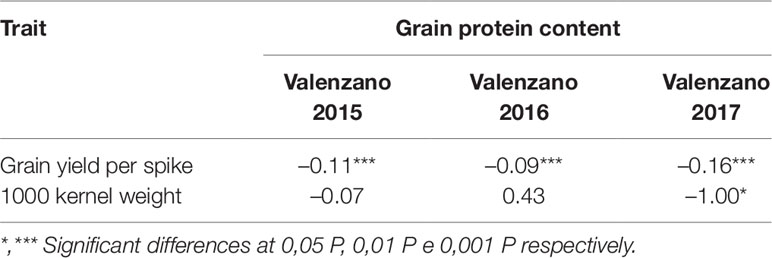
Table 2 Correlation coefficients between grain protein content and grain yield components in the Saragolla x 02-5B-318 RIL mapping population, evaluated in three environments. (Valenzano 2015, Valenzano 2016, Valenzano 2017).
QTL Analysis
QTL analysis was performed according to the Inclusive Composite Interval Mapping method (ICIM, Li et al., 2007). Putative QTL (LOD ≥ 3.0) for GPC, GYS, and TKW in individual environments are listed in Tables 3–5 respectively. QTL positions on the durum wheat linkage map are reported in Figure 2. Suggestive QTL, with a LOD value comprised between 2.0 and 3.0, were also reported.
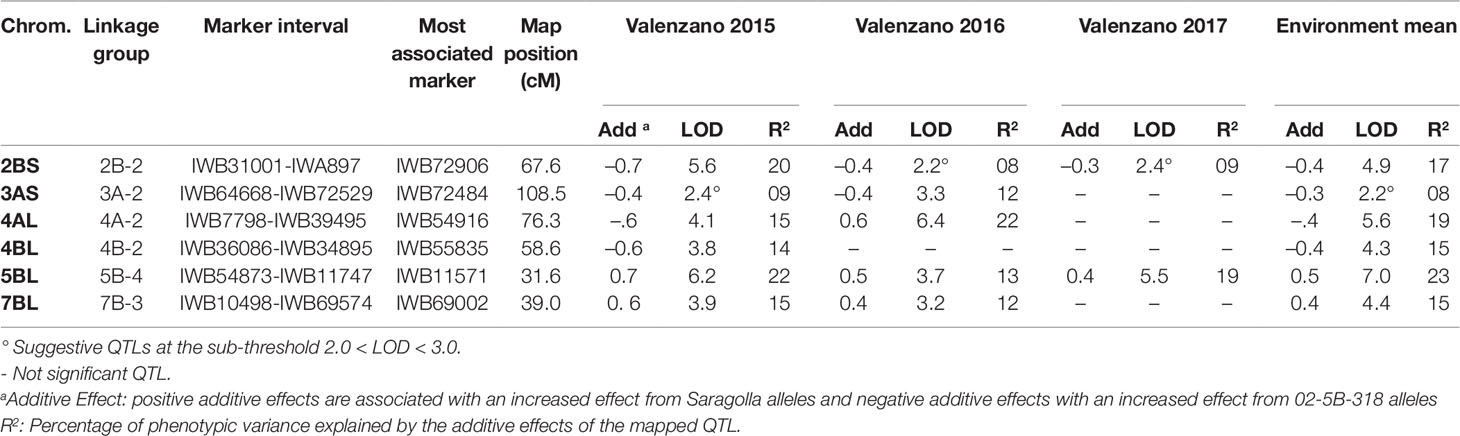
Table 3 QTL for grain protein content (GPC) mapped in the durum wheat RIL population derived from the cross between the bread wheat accession 02-5B-318 and the durum wheat cv. Saragolla, evaluated in three different environments (Valenzano 2015, Valenzano 2016, Valenzano 2017). Analyses were performed by ICIM (Inclusive Composite Interval Mapping).
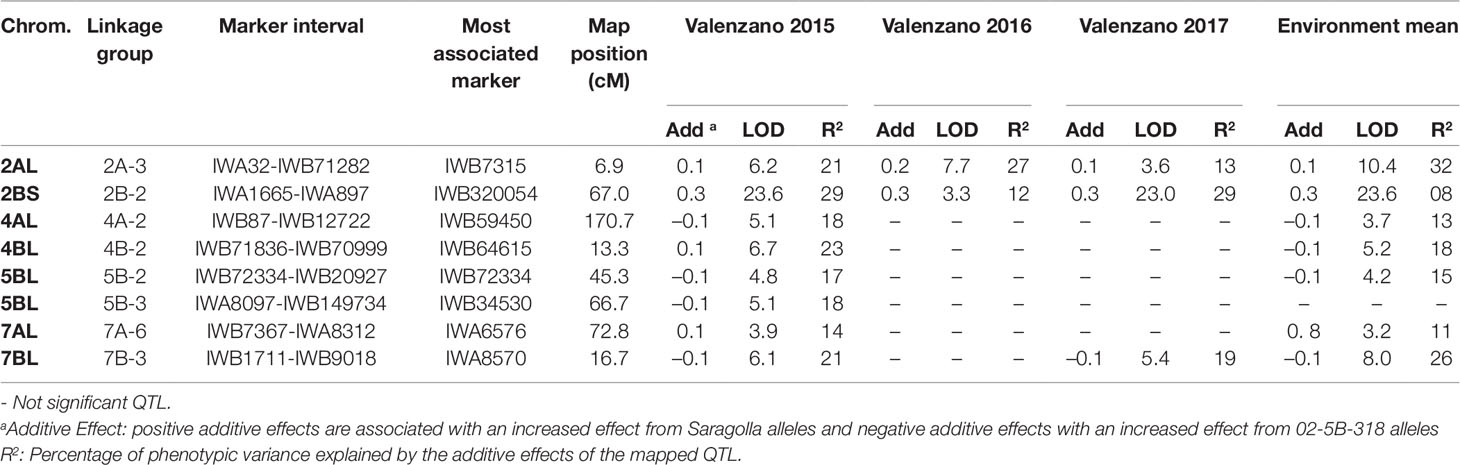
Table 4 QTL for grain yield per spike (GYS) mapped in the durum wheat RIL population derived from the cross between the bread wheat accession 02-5B-318 and the durum wheat cv. Saragolla, evaluated in three different environments (Valenzano 2015, Valenzano 2016, Valenzano 2017). Analyses were performed by ICIM (Inclusive Composite Interval Mapping).
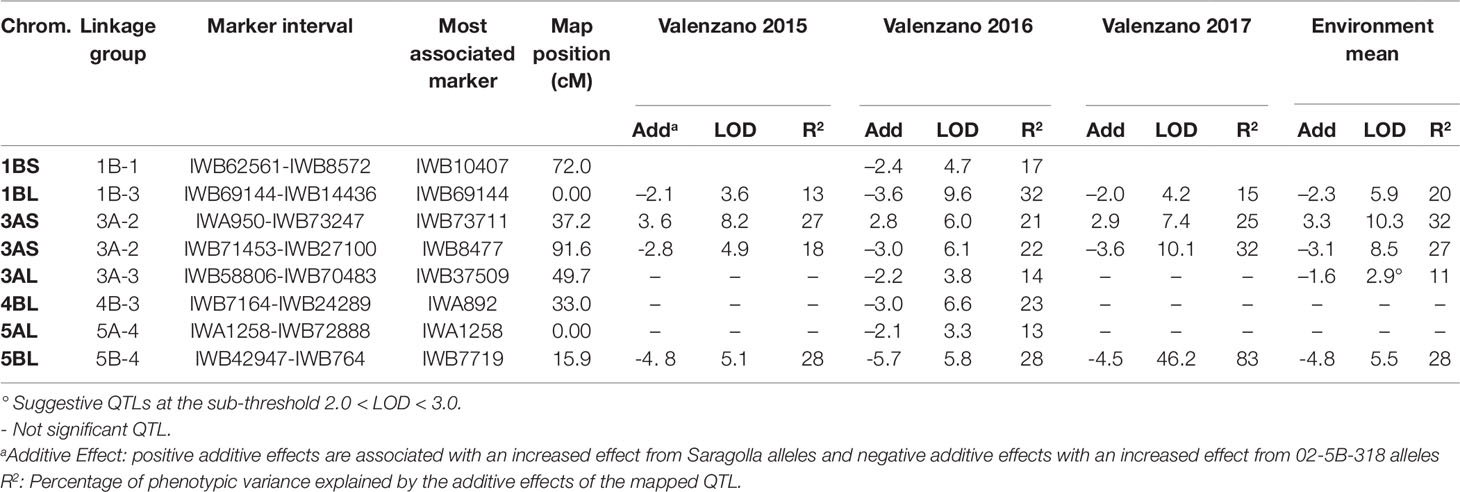
Table 5 QTL for thousand kernel weight (TKW) mapped in the durum wheat RIL population derived from the cross between the bread wheat accession 02-5B-318 and the durum wheat cv. Saragolla, evaluated in three different environments (Valenzano 2015, Valenzano 2016, Valenzano 2017). Analyses were performed by ICIM (Inclusive Composite Interval Mapping).
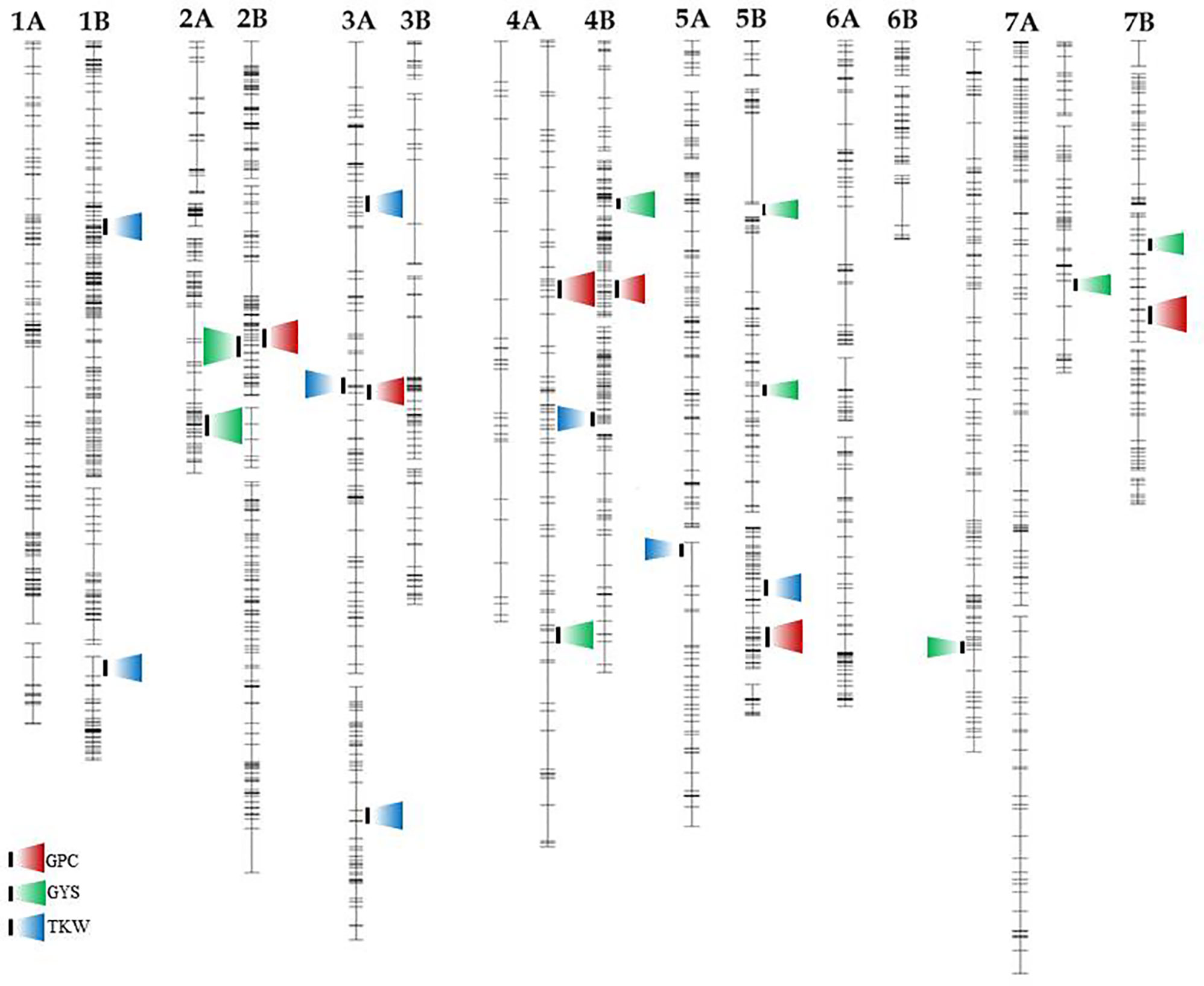
Figure 2 Genetic location of QTL for GYP, TKW, and GPC mapped on the durum wheat linkage map obtained in the RIL population derived by the cross between the durum cv. Saragolla and the bread wheat accession 02-5B-318. Loci for the different traits are reported in different colors (GPC, red; GYS, green; TKW, blue).
In the present work we identified six major QTL for GPC, each on chromosome 2B, 3A, 4A (two loci), 4B, 5B, and 7B, responsible for the highest percentage of the overall phenotypic variation of the trait in the three environments. Interestingly, we found trait-increasing alleles coming from both the high-GPC parent Saragolla and from the low-GPC parent 02-5B-318. Specifically, alleles from Saragolla were those mapped on chromosomes 4A, 5B, and 7B, whereas alleles from 02-5B-318 were those on 2B, 3A, and 4B. The additive effect values ranged from 0.27 to 0.71 of protein content unit. The percentage of phenotypic variation (R2) contributed by each single QTL in each environment was comprised between 8 and 23%. QTL on 2B and 5B were found significant in all the environments, whereas three were significant in two environments, and one was significant in one single location (Table 3).
Eight chromosomal regions were detected for GYS (Table 4), two on chromosome 5B and 7A and one on chromosomes 2A, 2B, 4A, 4B, 7B. Five QTL came from cv Saragolla and four from the 02-5B-318 accession line, three of which were found in all the environments. The strongest QTL was the one detected on 2B chromosome with a LOD score of 23; this stable QTL was responsible of up to 29% of GYS variation, and the positive allele came from Saragolla, the durum parental line with the highest GYS. The GYS QTL on 7B was significant in two environments, and the positive allele came from 02-5B-318. The remaining five QTL mapped for GYS were significant only in one single environment, with a quote of phenotypic variation comprised between 14 and 23%.
In the present work we mapped eight QTL for TKW, three of which were located on chromosome 3A, two on 1B and one on 4B, 5A, and 5B (Table 5). Four QTL were stable in the three environments and significant in the mean of environments, while the others QTL were significant only in one single environment. Each QTL had an additive effect ranging from of 1.67 g (QTL on 3A-3) to 5.67 g (QTL on 5B), and explained 11 to 32% of trait variation. Out of the eight QTL detected, six came from the bread parental line accession 02-5B-318, whereas only two derived from the durum wheat cv Saragolla.
Interestingly, only few overlapping occurred between QTL for grain yield components and QTL for GPC on 2B and 3A chromosomes (Figure 2). In most cases, the QTL was responsible for the genetic control of only one of the traits.
Putative Function and Candidate Gene Detection for GPC and Yield Components
One of the major steps of a gene-based analysis is the assignment of SNPs to genes. In order to identify a putative function for SNP markers and search for CGs for the traits of interest, SNP sequences were BLAST searched against GeneBank non redundant (nr) database. A total of 378 SNP sequences included in QTL regions were analysed, specifically 83 for GPC, 129 for TKW and 166 for GYP (for each QTL, SNPs from co-migrating loci mapped in a confidence interval of 15 cM were considered). Tables 6–8 list SNP loci for which sequence homology was retrieved for GPC, GYS and TKW, respectively. Overall, 22% of the total query sequences matched with known function genes (E value > 10-7), but only 10% corresponded to protein products involved in metabolic pathways related to the traits of interest. Sequences similarity searches revealed storage proteins loci, and genes involved in different cellular processes such as: DNA transcription, chromatin structure determination, response to biotic and abiotic stress, carbon metabolisms, modification of cell wall structure, and intracellular signalling.
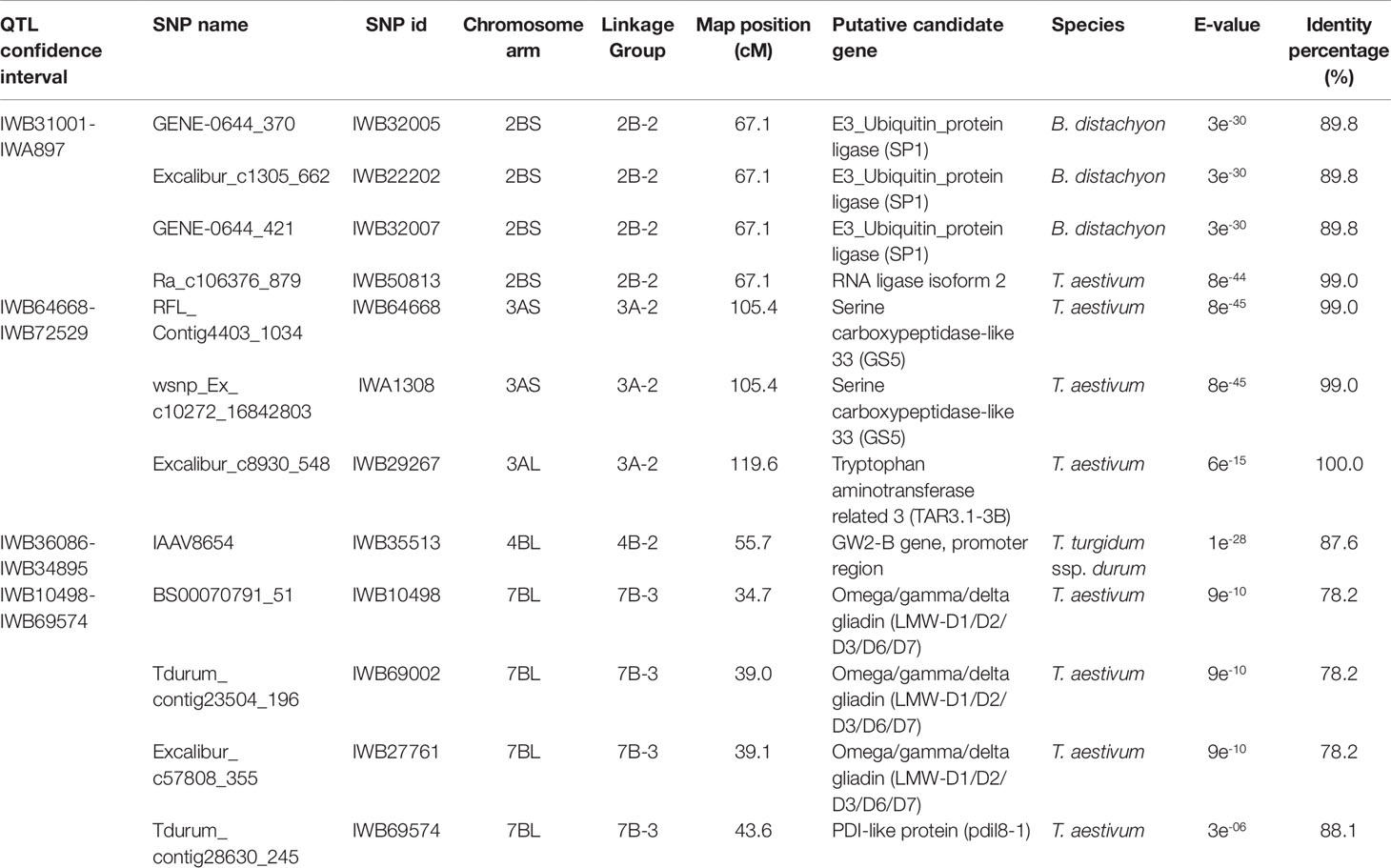
Table 6 Candidate genes identified in the QTL for grain protein content (GPC) mapped in the interspecific RIL population derived by the cross between the hexaploid wheat accession 02-5B-318 and the durum wheat cv. Saragolla (putative function is reported for the SNP markers in the QTL confidence interval showing an identity percentage > 80%).
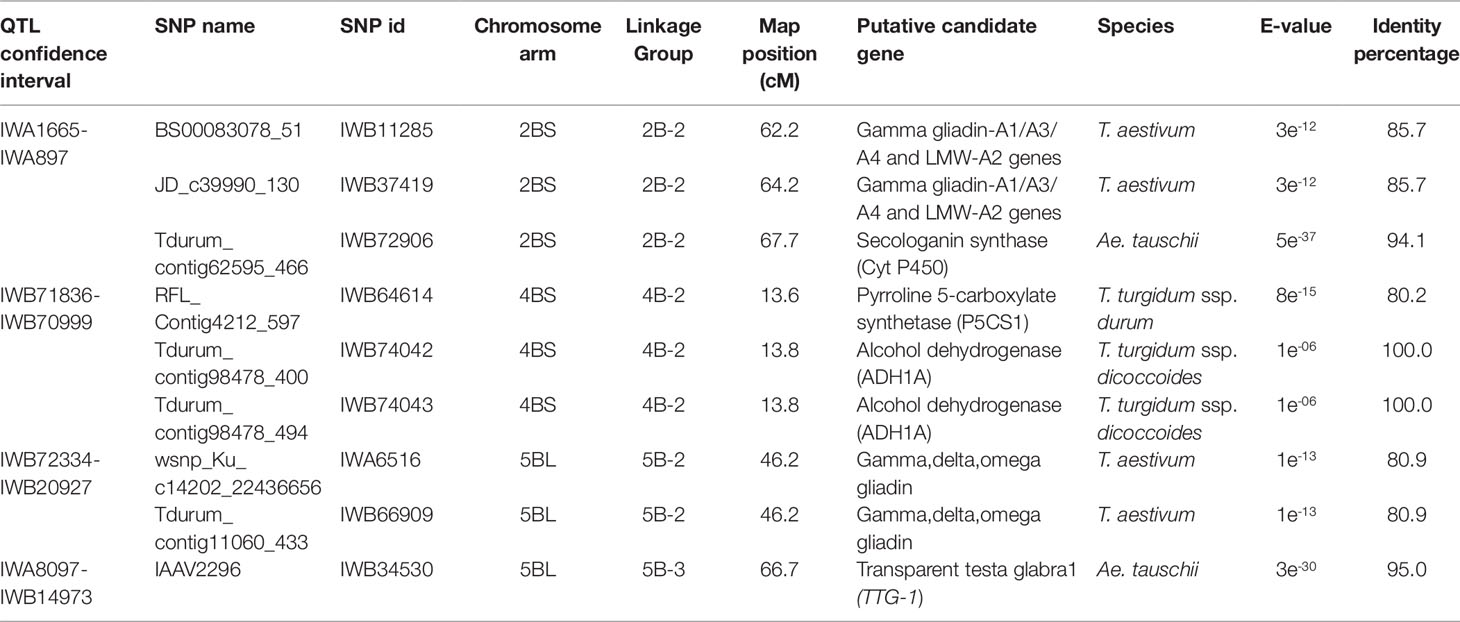
Table 7 Candidate genes identified in the QTL for grain yield per spike (GYS) mapped in the interspecific RIL population derived from the cross between the hexaploid wheat accession 02-5B-318 and the durum wheat cv. Saragolla (putative function is reported for the SNP markers in the QTL confidence interval showing an identity percentage > 80%).
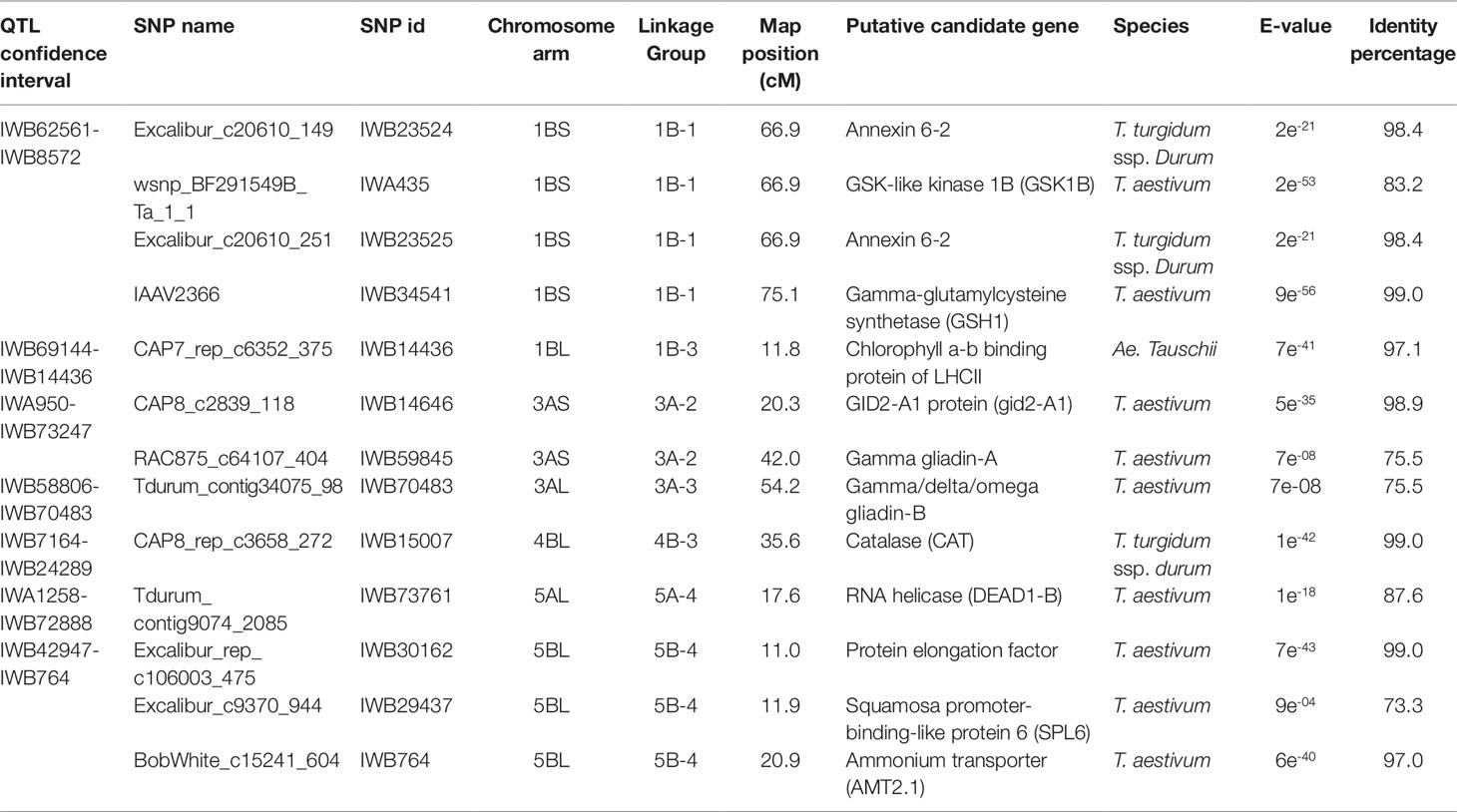
Table 8 Candidate genes identified in the QTL for thousand kernel weight (TKW) mapped in the interspecific RIL population derived by the cross between the hexaploid wheat accession 02-5B-318 and the durum wheat cv. Saragolla (putative function is reported for the SNP markers in the QTL confidence interval showing an identity percentage > 80%).
As reported in Table 6, putative functions retrieved for SNPs included in the GPC-QTL were seed storage proteins (ω/γ/δ gliadins), or enzymes involved in RNA processing (RNA ligase), amino acid modification (serine carboxypeptidase, tryptophan aminotransferase), determination of protein tertiary structure (PDI-like protein) and modification of proteins destined to degradation (Ubiquitin_protein ligase, GW2-B gene promoter).
Table 7 shows the putative function detected for the SNP markers mapped in the GYS-QTL. Some sequences matched with genes for gliadin storage protein; other SNPs were found to fall into genes related to plant response to stress (Secologanin synthase - Cyt P450- and Pyrroline 5-carboxylate synthase) or involved in sugar metabolism (Alcohol dehydrogenase, ADH1A). In this work, a particular attention was paid to the candidate gene matching with the marker IAAV2296 (IWB34530). Bioinformatics research showed that SNP sequence has a high similarity percentage (95%) with the Aegilops tauschii gene for Trasparent-testa-glabra1 (TTG-1). Molecular structure of TTG-1 was compared in diploid (T. urartu, Aegilops tauschii), tetraploid (T. durum, cv. Svevo, T. dicoccoides), and hexaploid (T. aestivum, cv. Chinese Spring) wheats, where genes were located on A, B, and D genome. Details on gene structure of TTG in wheat, together with Arabidopsis, tobacco and rice are reported in Supplementary Table S2.
For what concerns TKW, the identified candidate genes in the QTL region were storage ω/γ/δ gliadins, RNA helicase, proteins involved in photosynthesis (Table 8). Attention was focused on candidate genes identified in the confidence interval for the QTL on 5B chromosome, where major QTL for TKW have been cited in literature (Wang et al., 2012; Assanga et al., 2017; Su et al., 2018). In this region, we found very good candidates as some of the SNP markers fell into genes in some way involved in the determination of kernel weight, such as a protein elongation factor (Excalibur_rep_c106003_475), a squamosa promoter-binding-like protein (Excalibur_c9370_944), and an ammonium transporter (BobWhite_c15241_604). Among these, we decided to better characterize the EF gene, whose homologous on 7A chromosome has recently (Zheng et al., 2014) been assessed to be significantly associated with grain number per spike and to potentially increase wheat grain yield and yield-related traits. The molecular structure was compared in diploid (Triticum urartu), tetraploid (T. durum, cv. Svevo, T. dicoccoides), and hexaploid (T. aestivum, cv. Chinese Spring) wheats (Supplementary Table 1). The gene could be identified only on 5B and 5D homoeologous chromosomes, whereas no genes were found on A genome, in both tetraploid and hexaploid genotypes. EF gene is characterized from having several alternative splicing forms, with a variable number of exons/introns.
Grain Protein Composition
In the present work both parents and the complete RIL population were characterized for HMW-GS and for the gliadin Gli-B1 locus (Table 9). For HMW-GS the bread parent has for Glu-A1 gene the 1Ax2* allele encoding for 2* HMW-GS, for Glu-B1 the Bx7 and By9 alleles encoding 7 + 9 HMW-GS and γ42 gliadin subunit for Gli-B1 gene, while the durum wheat cv Saragolla presented the following allele combination: 6 + 8 for HMW-GS and the γ-45 for Gli-B1 gene. A segregation for all genes, reported in Table 9, was observed in the RIL population, and in particular on 135 analyzed RI lines, 72 showed the HMW-GS profile of Saragolla parent (6 + 8) and 63 RI lines the HMW-GS of the bread wheat parent (7 + 9). Moreover, 55 RI lines showed the γ-45 gliadin allele coming from cv Saragolla and 80 the γ-42 allele of the bread wheat parent.
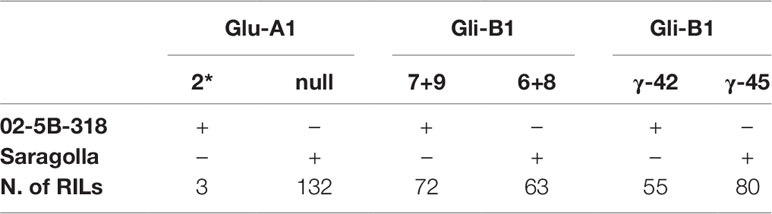
Table 9 HMW-GS and γ-42/γ-45 gliadin segregation in the durum wheat RIL population derived from the cross between the bread wheat accession 02-5B-318 and the durum cv. Saragolla.
Discussion
In the present work, an inter-specific RIL population of 135 tetraploid lines, originally developed by Giancaspro et al. (2016) for investigating the genetic basis of Fusarium head blight (FHB) resistance in durum wheat, was further characterized for GPC, protein composition, and yield components (TKW and GYS). In all the environments, the traits showed a wide difference between the two parents and a big variability in the RIL population. In particular, the range of phenotypic values in the RIL population was larger than between the two parents, clearly indicating the establishment of new genetic variability in the RI lines due to the combination of favorable alleles originating from both parents. This has been confirmed by the fact that the QTL identified for GYS, TKW, and GPC came from both parents (Tables 1–5).
Although wheat is an important source for protein, most of the modern varieties are relatively low in grain protein (10–14%) especially in cultivars containing the Rht1 dwarfing gene (McClung et al., 1986). Breeding efforts to increase GPC have been influenced by the strong impact of environmental conditions and by the negative effect on yield components, especially when the alleles come from wild emmers (Simmonds, 1995; Feil, 1997; Oury et al., 2003; Oury and Godin, 2007).
In this study the evaluation of grain yield components and GPC in three field trials lead us to the identification of 22 QTL distributed among all chromosomes excluding 1A, 3B, and 6A. This confirmed previous studies where QTL for GPC and grain yield were identified on almost all wheat chromosomes (Börner et al., 2002; Marza et al., 2005; McCartney et al., 2005; Quarrie et al., 2005; Huang et al., 2006; Gadaleta et al., 2007; Kuchel et al., 2007; Kumar et al., 2007; Maccaferri et al., 2008; McIntyre et al., 2010; Marcotuli et al., 2017; Mangini et al., 2018). Several QTL were found significant only in a one environment, and several authors reported that when a QTL was detected in more than one environment, a variation in its effects occurred (Huang et al., 2003; Huang et al., 2004; Kumar et al., 2007; Kuchel et al., 2007; Maccaferri et al., 2008; McIntyre et al., 2010).
By comparing the GPC-QTL found in the present study with those reported in the most recent literature, we found that loci on 2BS, 4BL, and 5BL were also reported by Nigro et al. (2019) and they occupy almost the same genomic region. Moreover, QTL on 2B, 4A, and 7B were also found in the work by Marcotuli et al. (2017). On chromosomes 2B and 4A we found QTL on a different chromosome arm (2BS instead of 2BL and 4AL rather than 4AS). For what concerns 7B, different stable QTL for GPC have been reported in different studies (reviewed by Kumar et al., 2018), most of which were located on the short arm of chromosome 7B. However, accordingly to what reported by Blanco et al. (2006) and Marcotuli et al. (2017), we found the QTL on 7B located on the long arm of the chromosome. Also in the work by Roselló et al. (2018) we found GPC loci on 4A, 4B, 5B, and 7B. Differences observed in map position could be due to the different mapping populations used for QTL analyses, the marker coverage of linkage maps, and the high number of genes controlling the trait.
Our work confirmed the negative relationship between GPC and yield component traits (Table 2), although only the QTL for GYS and GPC on 2B chromosome were co-localized. The other QTL on 3A, 4A, 4B, 5B, and 7B showing significant effects on GPC values were interesting because not liked to yield potential, probably because contain genes that influence GPC independently from variation in the grain yield components, and could be used to improve the GPC. The high-density consensus linkage map for durum wheat described by Maccaferri et al. (2019) was used as reference for chromosome localization and SNP markers position to compare our results with those reported in literature.
A total of 16 QTL for GYS and TKW were also detected on almost all chromosomes of durum wheat, of which none coincident. These data are consistent with several works that reported significant QTL for yield components almost on all wheat chromosomes (Araus et al., 2008; Cui et al., 2014; Gupta et al., 2017). QTL for TKW were found on 1B, 3A, 4B, 5A, and 5B chromosomes, while QTL for GYS were localized on homoeologous chromosome group 2, 4, 5, and 7 as also reported by Mangini et al. (2018) and Marcotuli et al. (2017), respectively for loci on 2BS and 4AL, and 2BS. Most of the yield loci mapped in the present work confirm the mapping data reported in recent literature: a very good correspondence was observed for the TKW-QTL detected on 1B (1A), 3A, 4B, 5A, and 5B which were also reported by Wang et al. (2012); Assanga et al. (2017) and Su et al. (2018). In particular, the TKW-QTL identified in the present work on chromosomes 1B, 4B, and 5B localized on the same map confidence interval of those mapped by Wang et al. (2012) and Su et al. (2018).
In the present work, beside surveying new genetic variability for GPC and yield components, some alleles related to grain storage protein composition were transferred from bread wheat to durum wheat. Glutenin subunits or their alleles can be used as indicators of wheat quality and can be used as "markers" for breeding purposes. In particular, we obtained durum lines with 1Ax2* allele encoding for 2* HMW-GS, and lines with alleles encoding 7 + 9 HMW-GS. Branlard and Dardevet (1985) observed that Zeleny sedimentation value had positive correlation with subunits 7 + 9 and 5 + 10, and negative correlation with 2 + 12; moreover, extensibility had relationship with subunits 2* and 17 + 18. γ-gliadins 45 and 42 are valuable markers for good and poor pasta quality, respectively, and this is because the genetic linkage with low molecular weight glutenin subunits (Sapirstein et al., 2007). In the present work, durum lines with γ-42 gliadin subunit have been obtained and the identification of such lines suggests the possibility to improve durum wheat for bread-making processing, which is a common practice in the bakery tradition of certain Italian regions.
In conclusion, in the present work an enlargement of genetic variability has been achieved in a RIL population obtained by the cross of a bread and a durum wheat line with different quality characteristics. Lines with higher protein content and/or composition could be usefully employed directly or as donor lines in future breeding programs. Moreover, for the two yield components, confident CGs were identified associated to the QTL on 5BL: TTG (Transparent testa glabra) for GYS, and EF (Elongation factor) for TKW. In both cases we can assume these genes as good candidates as they have been reported in literature to be correlated to seed storage proteins accumulation or determination of grain number per spike. In particular, TTG proteins have been well characterized in Arabidopsis and Nicotiana: they are involved in the regulation of several processes of plant development and immunity (Wang et al., 2009; Li et al, 2012). In this work, TTG was chosen as putative candidate gene for GYS because other than taking part to anthocyanin biosynthesis (Pang et al., 2009; Zhang and Schrader, 2017), plant thricome formation (Morohashi et al., 2007; Wang et al., 2009), and pathogen resistance (Wang et al., 2009; Truman et al., 2010; Li et al., 2012), it was recently reported to be involved in seed storage proteins accumulation in Arabidopsis (Chen et al., 2015) and Nicotiana (Zhu et al., 2013; Ge et al., 2016).
For what concerns EF, in the work by Zheng et al. (2014)Elongation Factor expression has been reported to increase gradually during common wheat grain filling, expecially in young spikes and developing seedlings. Moreover, Arabidopsis transgenic plants harbouring a TaTEF-7A gene construct, showed a better vegetative growth and an increased silique length, silique number, and grain length. TaTEF-7A was also mapped in wheat using a DH population, resulting in a co-localization with several reported QTL for yield-related traits (spikelet number per spike, flour yield, test weight, and grain yield).
In conclusion, both candidate genes identified in this work well represent a good starting point for dissecting the molecular basis of some yield-related traits, and understanding the genetic mechanism underlying this complex quantitative trait. Moreover, they may also serve as useful tools for developing genetic markers suitable for MAS breeding programs.
Data Availability Statement
All datasets for this study are included in the article/Supplementary Material.
Author Contributions
AgG designed the experiments. SG performed phenotypic characterization of wheat lines for protein content and yield components. SZ performed protein extraction and electrophoretic evaluation. AnG performed QTL analyses and candidate gene identification. AgG and AnG collaborated to data interpretation and writing the manuscript. All authors read and approved the final manuscript.
Conflict of Interest
The authors declare that the research was conducted in the absence of any commercial or financial relationships that could be construed as a potential conflict of interest.
Acknowledgments
This research was funded by Research Grant from ISEA AGROSERVICE SpA (S. Severino Marche, MC, Italy); Italian Ministry of University and Research (MIUR); PRIN Grant n. 2010T7247Z.
Supplementary Material
The Supplementary Material for this article can be found online at: https://www.frontiersin.org/articles/10.3389/fpls.2019.01509/full#supplementary-material
References
Appels, R., Eversole, K., Feuille, C, Keller, B., Rogers, J., Stein, N., et al. (2018). The International Wheat Genome Sequencing Consortium (IWGSC). Shifting the limits in wheat research and breeding using a fully annotated reference genome. Science 361, 6403. doi: 10.1126/science.aar7191
Araus, J. L., Slafer, G. A., Royo, C., Serret, M. D. (2008). Breeding for yield potential and stress adaptation in cereals. Crit. Rev. Plant Sci. 27, 377–412. doi: 10.1080/07352680802467736
Assanga, S., Zhang, G., Tan, C.-T., Rudd, J. C., Ibrahim, A., Xue, Q. (2017). Saturated genetic mapping of wheat streak mosaic virus resistance gene Wsm2 in wheat. Crop Sci. 57 (1), 332–339. doi: 10.2135/cropsci2016.04.0233
Avivi, L. (1978). High grain protein content in wild tetraploid wheat Triticum dicoccoides Korn, in 5th Int. Wheat Genet. Symp. Ed.Ramanujan, S. (India:New Delhi), 372–380.
Avni, R., Nave, M., Barad, O., Baruch, K., Twardziok, S. O., Gundlach, H. (2017). Wild emmer genome architecture and diversity elucidate wheat evolution and domestication. Science 357 (346), 93–97. doi: 10.1126/science.aan0032
Börner, A., Schumann, E., Fürste, A., Cöster, H., Leithold, B., Röder, M. (2002). Mapping of quantitative trait loci determining agronomic important characters in hexaploid wheat (Triticum aestivum L.). Theor. Appl. Genet. 105 (6-7), 921–936. doi: 10.1007/s00122-002-0994-1
Barneix, A. J. (2007). Physiology and biochemistry of source-regulated protein accumulation in the wheat grain. J. Plant Physiol. 164 (5), 581–590. doi: 10.1016/j.jplph.2006.03.009
Battenfield, S. D., Guzmán, C., Gaynor, R. C., Singh, R. P., Dreisigacker, S., et al. (2016). Genomic selection for processing and end-use quality traits in the CIMMYT spring bread wheat breeding program. Plant Genome 9, 2. doi: 10.3835/plantgenome2016.01.0005
Ben-David, R., Xie, W. L., Peleg, Z., Saranga, Y., Dinoor, A., Fahima, T. (2010). Identification and mapping of PmG16, a powdery mildew resistance gene derived from wild emmer wheat. Theor. Appl. Genet. 121, 499–510. doi: 10.1007/s00122-010-1326-5
Blanco, A., Simeone, R., Gadaleta, A. (2006). Detection of QTLs for grain protein content in durum wheat. Theor. Appl. Genet. 112, 1195–1204. doi: 10.1007/s00122-006-0221-6
Blanco, A., Mangini, G., Giancaspro, A., Giove, S., Colasuonno, P., Simeone, R., et al. (2012) Relationships between grain protein content and grain yield components through QTL analyses in a RIL population derived from two elite durum wheat cultivars. Mol. Breeding 30 (1), 79–92.
Branlard, G., Dardevet, M. (1985). Diversity of grain protein and bread quality. II. Correlation between high molecular weight subunits of glutenin and flour characteristics. J. Cereal Sci. 3, 345–354. doi: 10.1016/S0733-5210(85)80007-2
Branlard, G., Autran, J. C., Monneveux, P. (1989). High molecular weight glutenin subunits in durum wheat (T. durum). Theor. Appl. Genet. 78, 353–358. doi: 10.1007/BF00265296
Bushuk, W., Zillman, R. R. (1978). Wheat cultivar identification by gliadin electrophoregrams. i. apparatus, method and nomenclature. Can. J. Plant Sci. 58 (2), 505–515. doi: 10.4141/cjps78-076
Cakmak, I., Torun, A., Millet, E., Feldman, M., Fahima, T., Korol, A. B. (2004). Triticum dicoccoides: an important genetic resource for increasing zinc and iron concentration in modern cultivated wheat. Soil. Sci. Plant Nutr. 50, 1047–1054. doi: 10.1080/00380768.2004.10408573
Chatzav, M., Peleg, Z., Ozturk, L., Yazici, A., Fahima, T. I. (2010). Genetic diversity for grain nutrients in wild emmer wheat: potential for wheat improvement. Ann. Bot. 105 (7), 1211–1220. doi: 10.1093/aob/mcq024
Chen, M., Zhang, B., Li, C., Kulaveerasingam, H., Chew, F. T., Yu, H. (2015). TRANSPARENT TESTA GLABRA1 Regulates the Accumulation of Seed Storage Reserves in Arabidopsis. Plant Physiol. 169, 391–402. doi: 10.1104/pp.15.00943
Colasuonno, P., Maria, M. A., Blanco, A., Gadaleta, A. (2013). Description of durum wheat linkage map and comparative sequence analysis of wheat mapped DArT markers with rice and Brachypodium genomes. BMC Genet. 14, 114. doi: 10.1186/1471-2156-14-114
Cui, F., Zhao, C., Ding, A., Li, J., Wang, L., Li, X. (2014). Construction of an integrative linkare map and QTL mapping of grain yield-related traits using three related wheat RIL populations. Theor. Appl. Genet. 127, 659–675. doi: 10.1007/s00122-013-2249-8
Feil, B. (1997). The inverse yield-protein relationship in cereals: possibilities and limitations for genetically improving the grain protein yield. Trends In Agron. 1, 103–119.
Gadaleta, A., Mangini, G., Mulè, G., Blanco, A. (2007) Characterization of dinucleotide and trinucleotide EST-derived microsatellites in the wheat genome. Euphytica 153 (1-2), 73–85.
Galdi, G., Feldman, M. (1983). Diploidization of endosperm protein genes in polyploid wheats. Proc. 6th Int. Wheat Genetics Symp. (Kyoto, Japan), 1119–1123.
Ge, J., Li, B., Shen, D., Xie, J., Long, J., Dong, H. (2016).Tobacco TTG2 regulates vegetative growth and seed production via the predominant role of ARF8 in cooperation with ARF17 and ARF19. BMC Plant Biol. 16, 126. doi: 10.1186/s12870-016-0815-3
Giancaspro, A., Giove, S. L., Zito, D., Blanco, A. (2016). Mapping QTLs for Fusarium head blight resistance in an interspecific wheat population. Front. Plant Sci. 7, 1381. doi: 10.3389/fpls.2016.0138124
Gupta, P. K., Balyan, H. S., Gahlaut, V. (2017). QTL analysis for drought tolerance in wheat: present status and future possibilities. Agronomy 7, 5. doi: 10.3390/agronomy7010005
Huang, X. Q., Cöster, H., Ganal, M. W., Röder, M. S. (2003). Advanced backcross QTL analysis for the identification of quantitative trait loci alleles from wild relatives of wheat (Triticum aestivumL.). Theor. Appl. Genet. 106, 1379–1389. doi: 10.1007/s00122-002-1179-7
Huang, X. Q., Kempf, H., Ganal, M. W., Röder, M. S. (2004). Advanced backcross QTL analysis in progenies derived from a cross between a German elite winter wheat variety and a synthetic wheat (Triticum aestivum L.). Theor. Appl. Genet. 109, 933–943. doi: 10.1007/s00122-004-1708-7
Huang, X. Q., Cloutier, S., Lycar, L., Radovanovic, N., Humphreys, D. G., Noll, J. S., et al. (2006). Molecular dissection of QTLs for agronomic and quality traits in a doubled haploid population derived from two Canadian wheats (Triticum aestivum L.). Theor. Appl. Genet. 113, 753–766.29. doi: 10.1007/s00122-006-0346-7
Iqbal, M., Moakhar, N. P., Strenzke, K., Haile, T., Pozniak, C., Hucl, P., et al. (2016). Genetic improvement in grain yield and other traits of wheat grown in Western Canada. Crop Sci. 56, 613–624. doi: 10.2135/cropsci2015.06.0348
Jackson, E. A., Holt, L. M., Payne, P. I. (1983). Characterization of high-molecular weight gliadin and low-molecular-weight glutenin subunits of wheat endosperm by two dimensional electrophoresis and the chromosomal localization of their controlling genes. Theor. Appl. Genet. 66, 29–37. doi: 10.1007/BF00281844
Jang, Y. R., Beom, H. R., Altenbach, S. B., Lee, M. K., Lim, S. H., Lee, J. Y. (2017). Improved method for reliable HMW-GS identification by RP-HPLC and SDS-PAGE in common wheat cultivars. Molecules 22 (7), 1055. doi: 10.3390/molecules22071055
Joehanes, R., Nelson, J. C. (2008).QGene 4.0, an extensible Java QTL-analysis platform. Bioinformatics 24, 2788–2789. doi: 10.1093/bioinformatics/btn523
Kasarda, D. D. (1999). Glutenin polymers: The in vitro to in vivo transition. Cereal Foods World 44, 566–571.
Kramer, T. (1979). Environmental and genetic variation for protein content in winter wheat (Triticum aestivum L.). Euphytica 28, 209–218. doi: 10.1007/BF00056577
Kuchel, H., Williams, K. J., Langridge, P., Eagles, H. A., Jefferies, S. P. (2007). Genetic dissection of grain yield in bread wheat: I. QTL Anal. Theor. Appl. Genet. 115, 1029–1041. doi: 10.1007/s00122-007-0629-7
Kumar, N., Kulwal, P. L., Balyan, H. S., Gupta, P. K. (2007). QTL mapping for yield and yield contributing traits in two mapping populations of bread wheat. Mol. Breed. 19, 163–177. doi: 10.1007/s11032-006-9056-8
Kumar, A., Jain, S., Elias, E. M., Ibrahim, M., Sharma, L. K. (2018). an overview of QTL identification and marker-assisted selection for grain protein content in wheat, in Eco-friendly Agro-biological Techniques for Enhancing Crop Productivity. Eds.Sengar, A., Singh, R. (Singapore: Springer). doi: 10.1007/978-981-10-6934-5_11
Li, H., Ye, G., Wang, J. (2007). A modified algorithm for the improvement of composite interval mapping. Genetics 175, 361–374. doi: 10.1534/genetics.106.066811.40
Li, B., Gao, R., Cui, R., Lü, B., Li, X., Zhao, Y., et al. (2012). Tobacco TTG2 suppresses resistance to pathogens by sequestering NPR1 from the nucleus. J. Cell Sci. 125, 4913–4922. doi: 10.1242/jcs.111922
Maccaferri, M., Sanguineti, M. C., Corneti, S., Ortega, J. L., Salem, M. B., Bort, J. (2008). Quantitative trait loci for grain yield and adaptation of durum wheat (Triticum durum Desf.) across a wide range of water availability. Genetics 178, 489–511. doi: 10.1534/genetics.107.077297
Maccaferri, M., Harris, N. S., Twardziok, S. O., Pasam, R. K., Gundlach, H., Spannagl, M., et al. (2019). Durum wheat genome highlights past domestication signatures and future improvement targets. Nat. Genet. 51, 885–895. doi: 10.1038/s41588-019-0381-3
Mangini, G., Gadaleta, A., Colasuonno, P., Marcotuli, I., Signorile, A. M., Simeone, R. (2018). Genetic dissection of the relationships between grain yield components by genome-wide association mapping in a collection of tetraploid wheats. PloS One 13 (1), e0190162. doi: 10.1371/journal.pone.0190162
Marcotuli, I., Houston, K., Waugh, R., Fincher, G. B., Burton, R. A., Blanco, A., Gadaleta, A. (2015) Genome wide association mapping for arabinoxylan content in a collection of tetraploid wheats. PloS One: 10 (7): e0132787
Marcotuli, I., Gadaleta, A., Mangini, G., Signorile, A. M., Zacheo, S. A., Blanco, A., et al. (2017). Development of a high-density snp-based linkage map and detection of QTL for ß-glucans, protein content, grain yield per spike and heading time in durum wheat. Int. J. Mol. Sci. 18, 1329. doi: 10.3390/ijms18061329
Marza, F., Bai, G. H., Carver, B. F., Zhou, W. C. (2005). Quantitative trait loci for yield and related traits in the wheat population Ning7840 Clark. Theor. Appl. Genet. 112, 688–698. doi: 10.1007/s00122-005-0172-3
McCartney, C. A., Somers, D. J., Humphreys, D. G., Lukow, O., Ames, N., Noll, J. (2005). Mapping quantitative trait loci controlling agronomic traits in spring wheat cross RL4452× 'AC Domain'. Genome 48 (5), 870–883. doi: 10.1139/g05-055
McClung, A. M., Cantrell, R. G., Quick, J. S., Gregory, R. S. (1986). Influence of the Rht1 semidwarf gene on yield, yield components, and grain protein in durum wheat1. Crop Sci. 26, 1095–1099. doi: 10.2135/cropsci1986.0011183X002600060001x
McIntyre, C. L., Mathews, K. L., Rattey, A., Chapman, S. C., Drenth, J., Ghaderi, M. (2010). Molecular detection of genomic regions associated with grain yield and yield-related components in an elite bread wheat cross evaluated under irrigated and rainfed conditions. Theor. Appl. Genet. 120, 527–541. doi: 10.1007/s00122-009-1173-4
Morohashi, K., Zhao, M., Yang, M., Read, B., Lloyd, A., Lamb, R., et al. (2007). Participation of the Arabidopsis bHLH factor GL3 in trichome initiation regulatory events. Plant Physiol. 145, 736–746. doi: 10.1104/pp.107.104521
Narasimhamoorthy, B., Gill, B. S., Fritz, A. K., Nelson, J. C., Brown-Guedira, G. L. (2006). Advanced backcross QTL analysis of a hard winter wheat×synthetic wheat population. Theor. Appl. Genet. 112, 787–796. doi: 10.1007/s00122-005-0159-0
Nevo, E., Korol, A. B., Beiles, A., Fahima, T. (2002). Evolution of wild emmer and wheat improvement: population genetics, genetic resources, and genome organization of wheat's progenitor, Triticum dicoccoides. New York:Springer, Berlin Heidelberg. doi: 10.1007/978-3-662-07140-3
Nigro, D., Gadaleta, A., Mangini, G., Colasuonno, P., Marcotuli, I., Giancaspro, A., et al. (2019). Candidate genes and genome-wide association study of grain protein content and protein deviation in durum wheat. Planta. doi: 10.1007/s00425-018-03075-1
Oury, F. X., Godin, C. (2007). Yield and grain protein concentration in bread wheat: how to use the negative relationship between the two characters to identify favourable genotypes?. Euphytica 157 (1-2), 45–57. doi: 10.1007/s10681-007-9395-5
Oury, F. X., Berard, P., Brancourt-Hulmel, M., Depatureaux, C., Doussignault, G., Galic, N. (2003). Yield and grain protein concentration in bread wheat: a review and a study of multi-annual data from a French breeding program. J. Genet. Breed. 57, 59–68.
Pang, Y., Wenger, J. P., Saathoff, K., Peel, G. J., Wen, J., Huhman, D., et al. (2009). A WD40 repeat protein from Medicago truncatula is necessary for tissue specific anthocyanin and proanthocyanidin biosynthesis but not for trichome development. Plant Physiol. 151, 1114–1129. doi: 10.1104/pp.109.144022
Payne, P. I., Lawrence, G. J. (1983). Catalogue of alleles for the complex gene loci, Glu-A1, Glu-B1, and Glu-D1which code for HMW-GS of glutenin in hexaploid wheat. Cereal Res. Commun. 11, 29–35.
Payne, P. I., Lawn, C. N., Mudd, E. E. (1980). Control by homoeologous group 1 chromosomes of the high-molecular-weight subunits of glutenin, a major protein of wheat endosperm. Theor. Appl. Genet. 58 (3-4), 113–120.59. doi: 10.1007/BF00263101
Payne, P. I., Holt, L. M., Worland, A. J., Law, C. N. (1982). Structural and genetical studies on the high-molecular-weight subunits of wheat. Part 3. Telocentric mapping of the subunit genes on the long arms of homoeologous group 1 chromosomes. Theor. Appl. Genet. 63, 129–128. doi: 10.1007/BF00303695
Peleg, Z., Fahima, T., Abbo, S., Krugman, T., Nevo, E., Yakir, D. (2005). Genetic diversity for drought resistance in wild emmer wheat and its ecogeographical associations. Plant Cell Environ. 28, 176–191. doi: 10.1111/j.1365-3040.2005.01259.x
Quarrie, S. A., Steed, A., Calestani, C., Semikbodskii, A., Lebreton, C., Chinoy, -VC. (2005). A high-density genetic map of hexaploid wheat (Triticum aestivum L.) from the cross Chinese Spring x SQ1 and its use to compare QTLs for grain yield across a range of environments. Theor. Appl. Genet. 110, 865–880. doi: 10.1007/s00122-004-1902-7
Roselló, M., Royo, C., Álvaro, F., Villegas, D., Nazco, R., Soriano, J. M. (2018). Pasta-making quality qtlome from mediterranean durum wheat landraces. Front. Plant Sci. 9, 1512. doi: 10.3389/fpls.2018.01512
Sapirstein, H. D., David, P., Preston, K. R., Dexter, J. E. (2007). Durum wheat breadmaking quality: Effects of gluten strength, protein composition, semolina particle size and fermentation time. J. Cereal Sci. 45, 150–161. doi: 10.1016/j.jcs.2006.08.006
Shewry, P. R., Halford, N. G., Lafiandra, D. (2003). Genetics of wheat gluten proteins. Adv. Genet. 49, 111–184. doi: 10.1016/S0065-2660(03)01003-4
Simmonds, N. W. (1995). The relation between yield and protein in cereal grain. J. Sci. Food Agric. 67, 309–315. doi: 10.1002/jsfa.2740670306
Su, Z. Q., Hao, C. Y., Wang, L. F., Dong, Y. C., Zhang, X. Y. (2011). Identification and development of a functional marker of TaGW2 associated with grain weight in bread wheat (Triticum aestivum L.). Theor. Appl. Genet. 122, 211–223. doi: 10.1007/s00122-010-1437-z
Su, Q., Zhang, X., Zhang, W., Zhang, N., Song, L., Liu, L. (2018). QTL detection for Kernel size and weight in bread wheat (Triticum aestivum L.) using a high-density SNP and SSR-based linkage map. Front. Plant Sci. 9, 1484. doi: 10.3389/fpls.2018.0148467
Suprayogi, Y., Pozniak, C. J., Clarke, F. R., Clarke, J. M., Knox, R. E. (2009). Identification and validation of quantitative trait loci for grain protein concentration in adapted Canadiam durum wheat populations. Theor. Appl. Genet. 119, 437–448. doi: 10.1007/s00122-009-1050-1
Truman, W. M., Bennett, M. H., Turnbull, C. G., Grant, M. R. (2010). Arabidopsis auxin mutants are compromised in systemic acquired resistance and exhibit aberrant accumulation of various indolic compounds. Plant Physiol. 152, 1562–1573. doi: 10.1104/pp.109.152173
Uauy, C., Brevis, J. C., Dubcovsky, J. (2006). The high grain protein content gene Gpc-B1 accelerates senescence and has pleiotropic effects on protein content in wheat. J. Exp. Bot. 57, 2785–2794. doi: 10.1093/jxb/erl047
Voorrips, R. E. (2002).MapChart: Software for the graphical presentation of linkage maps and QTLs. J. Hered. 93, 77–78. doi: 10.1093/jhered/93.1.77
Wang, Y., Liu, R., Chen, L., Liang, Y., Wu, X., Li, B., et al. (2009). Nicotiana tabacum TTG1 contributes to ParA1-induced signalling and cell death in leaf trichomes. J. Cell Sci. 122, 2673–2685. doi: 10.1242/jcs.049023
Wang, L., Ge, H., Hao, C., Dong, Y., Zhang, X. (2012). Identifying loci influencing 1,000-Kernel weight in wheat by microsatellite screening for evidence of selection during breeding. PloS One 7, e29432. doi: 10.1371/journal.pone.0029432
Wang, S. W., Forrest, D., Allen, K., Chao, A., Huang, S., Huang, B. E., et al. (2014). Characterization of polyploid wheat genomic diversity using a high-density 90,000 single nucleotide polymorphismarray. Plant Biotechnol. J. 12, 787–796.
Yang, Z. B., Bai, Z. Y., Li, X. L., Wan, P., Wu, Q. X., Yang, L., et al. (2012). SNP identification and allelic-specific PCR markers development for TaGW2, a gene linked to wheat kernel weight. Theor. Appl. Genet. 125, 1057–1068. doi: 10.1007/s00122-012-1895-6
Zhang, B., Schrader, A. (2017). Transparent testa glabra 1-dependent regulation of flavonoid biosynthesis. Plants 6, 65. doi: 10.3390/plants6040065
Zhang, D. L., Hao, C. Y., Wang, L. F., Zhang, X. Y. (2012). Identifying loci influencing grain number by microsatellite screening in bread wheat (Triticum aestivum L.). Planta 236, 1507–1517. doi: 10.1007/s00425-012-1708-9
Zheng, J., Liu, H., Wang, Y., Wang, L., Chang, X., Jing, R. (2014). TEF-7A, a transcript elongation factor gene, influence yeld-related traits in bread wheat (Triticum aestivum L.). J. Exp. Bot. 65 (18), 5351–5365. doi: 10.1093/jxb/eru306
Zhu, Q., Li, B. Y., Mu, S. Y., Han, B., Cui, R. Z., Xu, M. Y., et al. (2013). TTG2- regulated development is related to expression of putative AUXIN RESPONSE FACTOR genes in tobacco. BMC Genomics 14, 806. doi: 10.1186/1471-2164-14-806
Keywords: grain protein content (GPC), protein quality, quantitative trait loci (QTL), wheat, genetic map
Citation: Giancaspro A, Giove SL, Zacheo SA, Blanco A and Gadaleta A (2019) Genetic Variation for Protein Content and Yield-Related Traits in a Durum Population Derived From an Inter-Specific Cross Between Hexaploid and Tetraploid Wheat Cultivars. Front. Plant Sci. 10:1509. doi: 10.3389/fpls.2019.01509
Received: 11 June 2019; Accepted: 30 October 2019;
Published: 22 November 2019.
Edited by:
Jose Luis Gonzalez Hernandez, South Dakota State University, United StatesReviewed by:
Maria Itria Ibba, International Maize and Wheat Improvement Center (Mexico), MexicoQijun Zhang, North Dakota State University, United States
Karl D. Glover, South Dakota State University, United States
Copyright © 2019 Giancaspro, Giove, Zacheo, Blanco and Gadaleta. This is an open-access article distributed under the terms of the Creative Commons Attribution License (CC BY). The use, distribution or reproduction in other forums is permitted, provided the original author(s) and the copyright owner(s) are credited and that the original publication in this journal is cited, in accordance with accepted academic practice. No use, distribution or reproduction is permitted which does not comply with these terms.
*Correspondence: Agata Gadaleta, agata.gadaleta@uniba.it