- 1College of Veterinary Medicine, Northeast Agricultural University, Harbin, China
- 2Heilongjiang Key Laboratory for Animal Disease Control and Pharmaceutical Development, Harbin, China
- 3College of Science, Northeast Agricultural University, Harbin, China
The imidazole glycerophosphate dehydratase (IGPD) protein is a therapeutic target for herbicide discovery. It is also regarded as a possible target in Staphylococcus xylosus (S. xylosus) for solving mastitis in the dairy cow. The 3D structure of IGPD protein is essential for discovering novel inhibitors during high-throughput virtual screening. However, to date, the 3D structure of IGPD protein of S. xylosus has not been solved. In this study, a series of computational techniques including homology modeling, Ramachandran Plots, and Verify 3D were performed in order to construct an appropriate 3D model of IGPD protein of S. xylosus. Nine hits were identified from 2,500 compounds by docking studies. Then, these nine compounds were first tested in vitro in S. xylosus biofilm formation using crystal violet staining. One of the potential compounds, baicalin was shown to significantly inhibit S. xylosus biofilm formation. Finally, the baicalin was further evaluated, which showed better inhibition of biofilm formation capability in S. xylosus by scanning electron microscopy. Hence, we have predicted the structure of IGPD protein of S. xylosus using computational techniques. We further discovered the IGPD protein was targeted by baicalin compound which inhibited the biofilm formation in S. xylosus. Our findings here would provide implications for the further development of novel IGPD inhibitors for the treatment of dairy mastitis.
Introduction
Bovine mastitis, a multi-factorial disease, causes enormous economic losses in the dairy industry worldwide (Anderson and Azizoglu, 2014). Studies have shown that some bacteria strains, such as Staphylococcus aureus (S. aureus) (Vasudevan et al., 2003), Streptococcus agalactiae (Boonyayatra and Pata, 2016), Escherichia coli (Silva et al., 2014), are the common pathogens that cause mastitis infections in dairy cows. Besides, Tenhagen et al. (2006) (Vasudevan et al., 2003) have reported that Staphylococcus xylosus (S. xylosus) isolated from a bovine mastitis could also cause the mastitis infection in dairy cows. S. xylosus is also one of the coagulase-negative staphylococci (CoNS) (Osman et al., 2016), which has strong ability of biofilm formation (Planchon et al., 2006, 2009). In addition, the L-histidine synthesis pathway is involved in the formation of biofilm in S. xylosus (Xu et al., 2017). The imidazole glycerophosphate dehydratase (IGPD), one of the specific enzymes, catalyzes the dehydration of imidazoleglycerol phosphate (IGP) to imidazoleacetol phosphate (IAP) (Hawkes et al., 1995), which synthesize L-histidine in the synthesis pathway (Dietl et al., 2016).
Currently, the IGPD protein becomes an attractive target for herbicide discoveries due to a necessary role in the histidine biosynthesis (Ahangar et al., 2013). Screening compounds via high-throughput virtual techniques require the 3D structure of a protein. However, the 3D structure of IGPD protein of S. xylosus has not been solved yet. Generally, it has been implemented that a protein sequence with 30% identity to a known structure is considered to be a threshold limit for the accuracy of homology modeling (Xiang, 2006; Henriksen et al., 2010). Recently, the 3D crystal structures of IGPD protein from S. aureus (Henriksen et al., 2010), Arabidopsis thaliana (A. thaliana) (Bisson et al., 2015), Cryptococcus neoformans (C. neoformans) (Chaudhuri et al., 2001) have been solved and are available in the protein databank, which provide us an opportunity to construct the 3D structure of IGPD of S. xylosus.
Computational methods e.g., virtual screening is an attractive and cost-effective way (Sakkiah et al., 2011) to identify potential novel inhibitors against a target protein (Zhu et al., 2007). If the structure of an active compound is available, the computational techniques could help to reduce the number of candidates for experimental validations. Docking-based virtual screening approach is one of the widely used methods to screen active compounds against the IGPD protein.
In this study, after constructing the 3D structure of the IGPD protein, we screened some of the best active compounds and verified their activities against the formation of biofilm in S. xylosus. Nine potential hits were identified using the docking-based virtual screening. The compounds inhibiting biofilm formation of S. xylosus were evaluated by crystal violet staining. One of the compounds, baicalin has shown to significantly inhibit the biofilm formation in S. xylosus in vitro.
Materials and Methods
Homology Modeling of the IGPD Protein
To date, the 3D structure of the IGPD protein from S. xylosus is not available. In order to construct the 3D structure of the IGPD protein, the protein of homology modeling technique was used for docking and structure-based design. It is pivotal to construct the 3D IGPD structure based on the known structures by homology modeling method. The amino acid sequences of the IGPD protein were isobarically tagged for relative and absolute quantitation (iTRAQ) labeling (Xu et al., 2017; Table 1). Hence, sequence similarity searches were carried out by utilizing BLASTp analysis (Altschul et al., 1990), which revealed suitable templates for the homology modeling. Target sequences of the IGPD protein were constructed based on the build homology models using Discovery Studio (version 3.0), (PS)2-v2: protein structure prediction server (Chen et al., 2006, 2009), as well as MODELLER (Webb and Sali, 2016). The needle pairwise sequence alignment was used to calculate sequence similarity (Li et al., 2015). Sequences identity and similarity from multiple sequence alignments were further confirmed by ClustalW (Larkin et al., 2007). Secondary structure matching (SSM) (Krissinel and Henrick, 2004) was used for superposition of protein structures (Bauer et al., 2008). Finally, the best model with the lowest probability density functions (PDF) total energy and Discrete Optimized Potential Energy (DOPE) score were selected (Shen and Sali, 2006).
Homology Model Validation
The model was evaluated using Ramachandran Plot (Kar et al., 2013), and was verified through the Profile-3D analysis. The qualified models were evaluated using the Qualitative Model Energy Analysis (QMEAN) (Benkert et al., 2008, 2009).
Molecular Docking of Compounds
After the initial model evaluations, molecular docking was carried out using the Discovery Studio (version 3.0). A set of 2,500 compounds from the PubChem Compound Database (https://pubchem.ncbi.nlm.nih.gov/), such as flavonoids, anthraquinones, and benzanthrone were used for the preparation of ligands to generate 3D conformations. The ADMET prediction was used to filter the water-insoluble drugs. The new 3D IGPD protein structure was then used in the docking study. All hydrogen atoms were added to the protein using DS 3.0. The Charmm Force Field was assigned, and the active site was defined. Then, the optimized ligands docked with the homology protein using the DS-CDOCKER protocol. The Pose Cluster Radius was set up to 0.5 and other parameters were used the default settings. Finally, the first hits by virtual screening were further evaluated by biofilm formation assays in vitro.
Biofilm Formation
Bacterial Strains and Culture Conditions
The S. xylosus ATCC700404 strain was used in this study (Yang et al., 2016). S. xylosus was cultured in Trypticase Soy Broth (TSB) (TSB: Summus Ltd, Harbin, Heilongjiang, China) at 37°C for 12 h. Then, all bacterial strains were repeatedly subcultured under the same conditions. The final cultures were used for the minimal inhibitory concentration (MICs) assays and the biofilm formation assays.
Inhibitory Activity of Compounds Against the IGPD
The identified compounds used for MICs assays were determined three times using the protocol described previously (Yang et al., 2016). Briefly, the overnight cultures of S. xylosus were diluted to a density of McFarland 0.5 standard [corresponding to 1 × 108 colony-forming units (CFU)/ml]. Then, the cultures of S. xylosus were diluted 1:100 using sterile TSB. Then 100 μl samples were added to each well of a 96-well plate (Corning Costar® 3599 Corning, NY, USA) containing serial dilutions of compounds in 100 μl culture medium. Control bacteria were cultivated in the absence of compounds. The MICs were determined as the lowest concentration of potential compounds after incubation for 24 h at 37°C.
Biofilm Formation Assay
The biofilm formation assay was carried out to evaluate the ability of S. xylosus biofilm disruption by the screened compounds using 96-well microtiter plates (Corning Costar® 3599 Corning, NY, USA) (Yang et al., 2016). Negative control wells contained broth only. Positive control wells contained culture medium and bacterial suspension. Biofilms were treated as described above (Yang et al., 2016). Briefly, the plates were covered and incubated aerobically for 24 h at 37°C. Then, the content of each well was aspirated and washed three times with 0.25 ml of sterile physiological saline. The remaining attached bacteria were fixed with 0.2 mL of 99% methanol (Guoyao Ltd, China) per well. After 15 min fixation plates were emptied and dried at 37°C. Then, the plates were stained for 5 min with 0.2 mL of 2% crystal violet (Guoyao Ltd., China). The excess stain was rinsed off by placing the plate under running tap water. After the plates were air dried, the dye was resolubilized with 0.16 mL of 33% (v/v) glacial acetic acid (Guoyao Ltd, China) per well. The OD of each well was measured at 570 nm using a microtiter plate reader (DG5033A, Huadong Ltd., Nanjing, Jiangsu, China).
Scanning Electron Microscopy
The biofilm of S. xylosus was examined by an electron microscopy (FEI Quanta, Netherland), which was described previously (Yang et al., 2016). Briefly, the overnight cultures of S. xylosus were diluted in the sterile THB supplemented with 5 % (v/v) fetal bovine serum (corresponding to 1 × 108 colony-forming units/ mL). Then, the overnight bacterial cultures were diluted with 5 mg/mL baicalin or without baicalin and then were added to each well of a six-well plate containing rough glass slide or normal glass slide. The supernatant was removed after 24 h incubation. The biofilms obtained from bacterial cells were prepared for analysis using a scanning electron microscopy.
Statistical Analysis
All the experiments were performed in triplicates. Data analysis and calculation of standard deviation were performed with SPSS 11.0.0 (IBM, USA).
Results and Discussions
Homology Modeling of the IGPD
The IGPD protein is a potential therapeutic target for herbicide discovery. Here, we used a molecular docking technology to discover the IGPD inhibitors. Up to date, the crystal structure of IGPD protein in S. xylosus has not been obtained. Thus, the homology modeling was used to build the 3D structure of that protein in this study. Generally, a protein sequence with 30% identity to a known structure is considered to be threshold limit for the accuracy of homology modeling (Xiang, 2006; Henriksen et al., 2010). From the Table 2, the 3D structure of IGPD from S. aureus (PDB code: 2AE8F) (Henriksen et al., 2010) was selected as the template (Supplementary Figure 1), which showed 22.8% sequence identity, 46.6% sequence similarity, 74% query cover, and high resolution with 2.01 Å. Further, multiple sequences alignment of IGPD with S. aureus (PDB code: 2AE8F), A. thaliana (PDB code: 2F1DP) (Henriksen et al., 2010) and C. neoformans (PDB code: 1RHYB) (Chaudhuri et al., 2001) were performed using ClustalW (Larkin et al., 2007). The highly conserved region of the structural elements was found, which was shown in Figure 1. Finally, based on the least PDF total energy (4591.1885) and DOPE score (Shen and Sali, 2006) (-19163.410156) the best model (Supplementary Figure 2) was selected and its structural compatibility was verified using several validation servers.
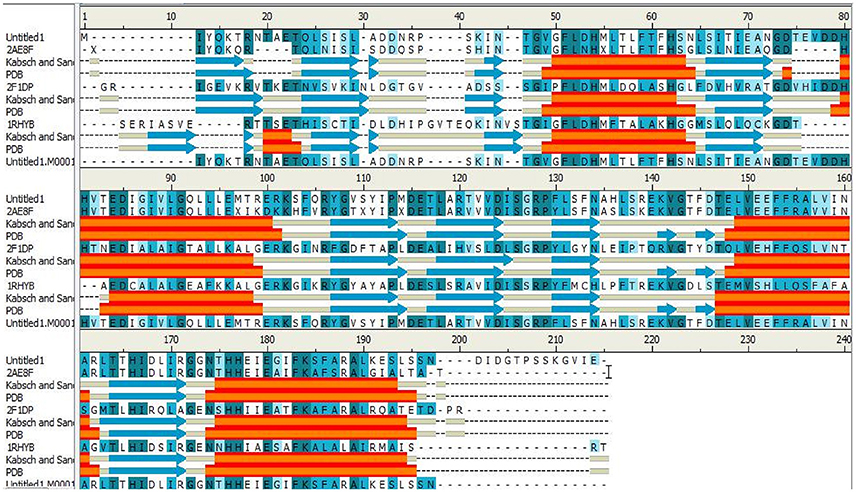
Figure 1. The alignment sequence between IGPD proteins from Staphylococcus xylosus. Staphylococcus aureus (PDB ID: 2AE8F), Arabidopsis thaliana (PDB ID: 2F1DP) and Cryptococcus neoformans (PDB ID: 1RHYB). The α helices are shown as red blocks. The β sheets are shown as blue blocks. Most of the secondary structures are observed to be well conserved.
Homology Model Validation
The selected model was then evaluated by Profiles-3D. The calculated verify score for each of residues in the IGPD model was shown in Supplementary Figure 3. The Ramachandran Plot analyzed by RAMPAGE was often used to check the correctness in proteins for the stereochemical feasibility (Gopalakrishnan et al., 2008). The final model was selected (Supplementary Table 1), which had the maximum number of residues in the favored regions (93.26%), minimum disallowed regions (1.69%), and with no significant influence on our modeling and docking studies, since they are far from the active site of the IGPD in Supplementary Figure 4. At the meantime, it was shown to have the best secondary structures in the superposition for IGPD protein (Figure 2). It is also the Superimpose protein that deals with structural superpositions of molecules in a widespread sense (Bauer et al., 2008). Indeed, the Z-score calculated for the template was −3.75, which indicated that the overall geometrical quality of the model was within the acceptable range in comparison with the solved experimental structure using the QMEAN. Overall, the validation results demonstrated that the established homology model of the IGPD should be a reasonable structure, and could be used in the following studies.
Molecular Docking of Compounds
The DS 3.0 was used for the virtual screening of IGPD protein. First of all, 2500 compounds from the PubChem Compound Database were used for the screening, e.g., flavonoids, anthraquinones, and benzanthrone can inhibit the formation of biofilms, especially among the known experiments emodin inhibits Streptococcus suis (S. suis) biofilm formation (Yang et al., 2015), rutin inhibits S. suis biofilm formation (Wang et al., 2017), and caffeic acid derivatives against Candida albicans biofilm (De Vita et al., 2014). In order to discover inhibitors targeting the IGPD faster and more accurate, the ADMET prediction was used to eliminate compounds with unfavorable aqueous solubility compounds. The rest of the compounds were employed in the docking study. This contributes to the better interaction of proteins and compounds (Hiremath, 2009). It is conceived that the 3D structure of A. thaliana of active site is positioned between the two active-site manganese ions (Mn1 and Mn2) (PDB code: 2F1DP) which has shown to play a key role in the process of the IGPD activity in the previous study (Henriksen et al., 2010; Bisson et al., 2015). In this study, the two active-site manganese ions were coped (Figure 3). Then molecular docking studies were performed to find the optimal conformation in the binding site of the IGPD receptor and to identify the interaction between them, as well as to complement the CDOCKER docking studies for the rational design of compounds. According to the calculated score, nine compounds had the best -CDOCKER ENERGY and -CDOCKER INTERACTION ENERGY scores (Table 3). Among them, baicalin was one of the hits. Currently, it has been shown that the interaction mode analysis of nitrogen-containing heterocyclic compounds was found to be some hydrophobic interactions in the IGPD protein of Cryptococcus neoformans (PDB code: 1RHYB) (Shen et al., 2011). However, whether baicalin can inhibit the IGPD protein of S. xylosus has not been reported. By docking analysis, baicalin was one of the strongest in attracting the IGPD protein in S. xylosus when complexes through specific residual interaction with three H-bonds of His 63, Asp 60, and Ala 10 (Figure 3). In addition, there is one hydrophobic interaction with Gly 32. It also plays an important role in the interaction between the IGPD protein and baicalin. The estimated CDOCKER energy of baicalin was found to be −38.9626 kcal/mol. These effects together indicated that baicalin can inhibit IGPD protein of S. xylosus.
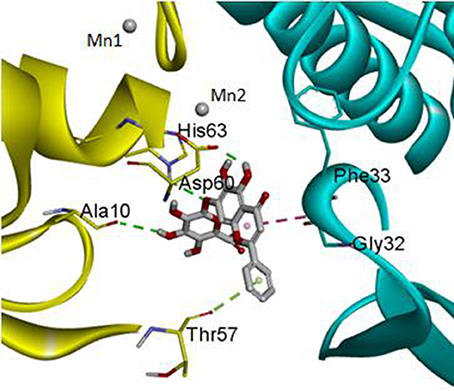
Figure 3. The protein backbone is shown and colored gold, cyan for chains A, A' respectively. The two manganese ions are shown as labeled silvery white spheres, and the atoms of the compound, baicalin, are shown in ball-and-stick format and colored by atom. Polar interactions are shown as dashed colored lines. Among them, the green dotted line represents the hydrogen bond interaction. The pink dotted line represents hydrophobic interaction.
In Vitro Biofilm Formation
The 9 compounds were evaluated against the S. xylosus biofilm formation with different MICs listed in Table 3. The results (Figure 4) showed that baicalin and fisetin had the highest ability to inhibit the formation of S. xylosus biofilm. Then, the ability of inhibition decreases progressively from the emodin and ferulic acid to phycsion, chlorogenic acid, and chrysophanol, whereas, the baicalein and sodium danshensu showed the lowest ability to inhibit the formation of S. xylosus biofilm. Most recently studies have shown that the inhibitory effect of baicalin on biofilm formation in Pseudomonas aeruginosa pathogenicity (Luo et al., 2017) and Cronobacter sakazakii (Jing et al., 2016) were 83.7, 66.6%, respectively. Interestingly, we have shown here baicalin can also inhibit S. xylosus biofilm formation (73.3%). In addition, we further used the scanning electron microscopy method to confirm this finding. As shown in the control (Figure 5B), a thick biofilm made of aggregates and microcolonies of S. xylosus almost completely covered the surface of the rough glass slide. However, when the culture medium was supplemented with 5 mg/mL of baicalin, the individual pairs of S. xylosus and the individual short chains of S. xylosus were attached to the rough glass slide (Figure 5A). This is the first report that baicalin can inhibit the formation of S. xylosus biofilm.
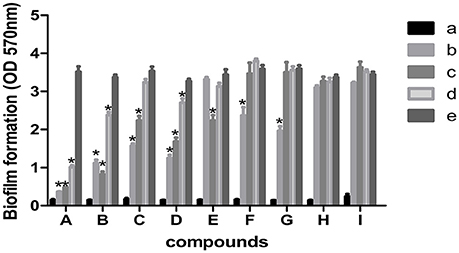
Figure 4. Effect of MICs of compounds at different concentrations on biofilm formation by Staphylococcus xylosus ATCC700494. Data are expressed as means ± standard deviations. Asterisk indicates signifcantly different (*p < 0.05) compared to untreated control bacteria. (A) Baicalin: (a) blank, (b) 5 mg/mL (c) 2.5 mg/mL, (d) 1.25 mg/mL, (e) control. (B) Fisetin: (a) blank, (b) 12 mg/mL (c) 6 mg/mL, (d) 3 mg/mL, (e) control. (C) Emodin: (a) blank, (b) 1/2 MIC (c) 1/4 MIC, (d) 1/8 MIC, (e) control. (D) Ferulic acid: (a) blank, (b) 1/2 MIC (c) 1/4 MIC, (d) 1/8 MIC, (e) control. (E) Physcion: (a) blank, (b) 4.5 mg/mL (c) 2.25 mg/mL, (d) 1.125 mg/mL, (e) control. (F) Chlorogenic: (a) blank, (b) 9 mg/mL (c) 4.5 mg/mL, (d) 2.25 mg/mL, (e) control. (G) Physcion: (a) blank, (b) 1/2 MIC (c) 1/4 MIC, (d) 1/8 MIC, (e) control. (H) Baicalein (a) blank, (b) 1/2 MIC (c) 1/4 MIC, (d) 1/8 MIC, (e) control. (I) Solidum danshensu: (a) blank, (b) 21 mg/mL (c) 10.5 mg/mL, (d) 5.25 mg/mL, (e) control.
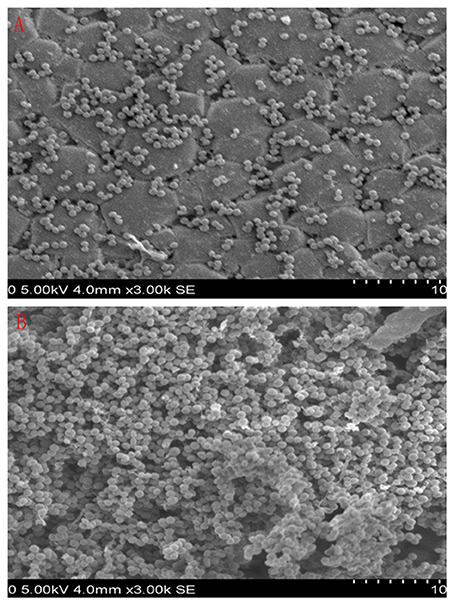
Figure 5. Scanning electron micrographs of S. xylosus ATCC700794 biofilm following growth in TSB supplemented with 5 mg/mL of baicalin (a) or control (b). Controls refer to the absence of baicalin. (A) 5 mg/mL of baicalin. (B) control.
Conclusions
The IGPD inhibitors in S. xylosus are potential new drug targets. We have constructed a 3D structure of IGPD protein. We have discovered 9 compounds that show interaction with IGPD protein. Among them, baicalin showed the highest capacity to inhibit the formation of S. xylosus biofilm in vitro. The novelty of this work lies in the use of the constructed 3D structure of the IGPD protein to screen compounds inhibiting the biofilm formation of S. xylosus, which could accelerate the development of novel compounds for solving the persistent infection of mastitis in dairy cows.
Author Contributions
Y-HL and J-WB designed the whole experiment; X-RC directed the completion of the experiment; X-TW, M-QH, Y-HZ, W-QC, X-XX, and C-GX provided help during the experiment.
Conflict of Interest Statement
The authors declare that the research was conducted in the absence of any commercial or financial relationships that could be construed as a potential conflict of interest.
Acknowledgments
This work was supported by National Science, Technology support plan of China (grant number 31772787), the earmarked fund for China Agriculture Research System −35, Heilongjiang Postdoctoral Foundation (LBH-Z1), Project supported by the Science and Technology Bureau of Harbin (2017RAQXJ033).
Supplementary Material
The Supplementary Material for this article can be found online at: https://www.frontiersin.org/articles/10.3389/fchem.2017.00098/full#supplementary-material
References
Ahangar, M. S., Vyas, R., Nasir, N., and Biswal, B. K. (2013). Structures of native, substrate-bound and inhibited forms of Mycobacterium tuberculosis imidazoleglycerol-phosphate dehydratase. Acta Crystallogr. 69(Pt 12), 2461–2467. doi: 10.1107/S0907444913022579
Altschul, S. F., Gish, W., Miller, W., Myers, E. W., and Lipman, D. J. (1990). Basic local alignment search tool. J. Mol. Biol. 215, 403–410. doi: 10.1016/S0022-2836(05)80360-2
Anderson, K. L., and Azizoglu, R. O. (2014). Detection and Causes of Bovine Mastitis with Emphasis on Staphylococcus aureus. Raleigh, NC: Encyclopedia of Agriculture & Food Systems, 435–440.
Bauer, R. A., Bourne, P. E., Formella, A., Frömmel, C., Gille, C., Goede, A., et al. (2008). Superimposé: a 3D structural superposition server. Nucleic Acids Res. 36, 47–54. doi: 10.1093/nar/gkn285
Benkert, P., Schwede, T., and Tosatto, S. C. (2009). QMEANclust: estimation of protein model quality by combining a composite scoring function with structural density information. BMC Struct. Biol. 9:35. doi: 10.1186/1472-6807-9-35
Benkert, P., Tosatto, S. C., and Schomburg, D. (2008). QMEAN: a comprehensive scoring function for model quality assessment. Proteins 71, 261. doi: 10.1002/prot.21715
Bisson, C., Britton, K. L., Sedelnikova, S. E., Rodgers, H. F., Eadsforth, T. C., Viner, R. C., et al. (2015). Crystal structures reveal that the reaction mechanism of imidazoleglycerol-phosphate dehydratase is controlled by switching Mn(II) coordination. Structure 23, 1236–1245. doi: 10.1016/j.str.2015.05.012
Boonyayatra, S., and Pata, P. (2016). Antimicrobial resistance of biofilm-forming Streptococcus agalactiae isolated from bovine Mastitis. J. Vet. Sci. Technol. 7:374. doi: 10.4172/2157-7579.1000374
Chaudhuri, B. N., Lange, S. C., Myers, R. S., Chittur, S. V., Davisson, V. J., and Smith, J. L. (2001). Crystal structure of imidazole glycerol phosphate synthase: a tunnel through a (β/α) 8 barrel joins two active sites. J. Biol. Chem. 279, 15491–15498. doi: 10.1016/S0969-2126(01)00661-X
Chen, C. C., Hwang, J. K., and Yang, J. M. (2006). (PS)2: protein structure prediction server. Nucleic Acids Res. 34:W152. doi: 10.1093/nar/gkl187
Chen, C. C., Hwang, J. K., and Yang, J. M. (2009). (PS)2 -v2: template-based protein structure prediction server. BMC Bioinformatics 10:366. doi: 10.1186/1471-2105-10-366
De Vita, D., Friggeri, L., D'Auria, F. D., Pandolfi, F., Piccoli, F., Panella, S., et al. (2014). Activity of caffeic acid derivatives against Candida albicans biofilm. Bioorg. Med. Chem. Lett. 24, 1502. doi: 10.1016/j.bmcl.2014.02.005
Dietl, A. M., Amich, J., Leal, S., Beckmann, N., Binder, U., Beilhack, A., et al. (2016). Histidine biosynthesis plays a crucial role in metal homeostasis and virulence of. Virulence 7, 465–476. doi: 10.1080/21505594.2016.1146848
Gopalakrishnan, K., Saravanan, S., Sarani, R., and Sekar, K. (2008). RPMS: Ramachandran plot for multiple structures. J. Appl. Crystallogr. 41, 219–221. doi: 10.1107/S0021889807053708
Hawkes, T. R., Thomas, P. G., Edwards, L. S., Rayner, S. J., Wilkinson, K. W., and Rice, D. W. (1995). Purification and characterization of the imidazoleglycerol-phosphate dehydratase of Saccharomyces cerevisiae from recombinant Escherichia coli. Biochem. J. 306(Pt 2), 385–397.
Henriksen, S. T., Liu, J., Estiu, G., Oltvai, Z. N., and Wiest, O. (2010). Identification of novel bacterial histidine biosynthesis inhibitors using docking, ensemble rescoring, and whole-cell assays. Bioorg. Med. Chem. 18, 5148. doi: 10.1016/j.bmc.2010.05.060
Hiremath, C. N. (2009). Abbreviated Profile of Drugs (A-POD): A Unique Numerical and Graphical Representation for Compound Properties and Its Use in ADMET Prediction. U.S. patent provisional application.
Jing, C., Li, P., Du, X., Wu, X., and Wang, S. (2016). Inhibition of Cronobacter sakazakii biofilms by baicalin. Microbiol. China 43, 1774–1784. doi: 10.13344/j.microbiol.china.150644
Kar, R. K., Ansari, M. Y., Suryadevara, P., Sahoo, B. R., Sahoo, G. C., Dikhit, M. R., et al. (2013). Computational elucidation of structural basis for ligand binding with leishmania donovani adenosine kinase. Biomed Res. Int. 2013:609289. doi: 10.1155/2013/609289
Krissinel, E., and Henrick, K. (2004). Secondary-structure matching (SSM), a new tool for fast protein structure alignment in three dimensions. Acta Crystallogr. 60(Pt 12 Pt 1), 2256. doi: 10.1107/S0907444904026460
Li, W., Cowley, A., Uludag, M., Gur, T., Mcwilliam, H., Squizzato, S., et al. (2015). The EMBL-EBI bioinformatics web and programmatic tools framework. Nucleic Acids Res. 43:W580. doi: 10.1093/nar/gkv279
Luo, J., Dong, B., Wang, K., Cai, S., Liu, T., Cheng, X., et al. (2017). Baicalin inhibits biofilm formation, attenuates the quorum sensing-controlled virulence and enhances Pseudomonas aeruginosa clearance in a mouse peritoneal implant infection model. PLoS ONE 12:e0176883. doi: 10.1371/journal.pone.0176883
Larkin, M. A., Blackshields, G., Brown, N. P., Chenna, R., McGettigan, P. A., McWilliam, H., et al. (2007). Clustal W and Clustal X version 2.0. Bioinformatics 23, 2947–2948. doi: 10.1093/bioinformatics/btm404
Osman, K. M., Abd El-Razik, K. A., Marie, H. S. H., and Arafa, A. (2016). Coagulase-negative staphylococci collected from bovine milk: species and antimicrobial gene diversity. J. Food Saf. 36, 89–99. doi: 10.1111/jfs.12216
Planchon, S., Desvaux, M., Chafsey, I., Chambon, C., Leroy, S., Hébraud, M., et al. (2009). Comparative subproteome analyses of planktonic and sessile Staphylococcus xylosus C2a: new insight in cell physiology of a coagulase-negative Staphylococcus in biofilm. J. Proteome Res. 8, 1797. doi: 10.1021/pr8004056
Planchon, S., Gaillard-Martinie, B., Dordet-Frisoni, E., Bellon-Fontaine, M. N., Leroy, S., Labadie, J., et al. (2006). Formation of biofilm by Staphylococcus xylosus. Int. J. Food Microbiol. 109, 88–96. doi: 10.1016/j.ijfoodmicro.2006.01.016
Sakkiah, S., Thangapandian, S., John, S., and Lee, K. W. (2011). Pharmacophore based virtual screening, molecular docking studies to design potent heat shock protein 90 inhibitors. Eur. J. Med. Chem. 46, 2937–2947. doi: 10.1016/j.ejmech.2011.04.018
Shen, M. Y., and Sali, A. (2006). Statistical potential for assessment and prediction of protein structures. Protein Sci. 15, 2507–2524. doi: 10.1110/ps.062416606
Shen, T., Du, F.-P., Liu, T., Yao, G.-W., Wu, Z., Fang, M.-M., et al. (2011). Molecular simulation of the interaction between imidazole glycerol phosphate dehydrase and nitrogen-containing heterocyclic phosphate inhibitors. Acta Phys. Chim. Sin. 27, 1831–1838. doi: 10.3866/PKU.WHXB20110808
Silva, V. O., Soares, L. O., Silva Júnior, A., Mantovani, H. C., Chang, Y. F., and Moreira, M. A. S. (2014). Biofilm formation on biotic and abiotic surfaces in the presence of antimicrobials by Escherichia coli isolates from cases of bovine mastitis. Appl. Environ. Microbiol. 80, 6136. doi: 10.1128/AEM.01953-14
Tenhagen, B.-A., Köster, G., Wallmann, J., and Heuwieser, W. (2006). Prevalence of mastitis pathogens and their resistance against antimicrobial agents in dairy cows in Brandenburg, Germany. J. Dairy Sci. 89, 2542–2551. doi: 10.3168/jds.S0022-0302(06)72330-X
Vasudevan, P., Nair, M. K., Annamalai, T., and Venkitanarayanan, K. S. (2003). Phenotypic and genotypic characterization of bovine mastitis isolates of Staphylococcus aureus for biofilm formation. Vet. Microbiol. 92, 179. doi: 10.1016/S0378-1135(02)00360-7
Wang, S., Wang, C., Gao, L., Cai, H., Zhou, Y., Yang, Y., et al. (2017). Rutin inhibits Streptococcus suis biofilm formation by affecting CPS biosynthesis. Front. Pharmacol. 8:379. doi: 10.3389/fphar.2017.00379
Webb, B., and Sali, A. (2016). Comparative Protein Structure Modeling Using MODELLER. Raleigh, NC: John Wiley & Sons, Inc.
Xiang, Z. (2006). Advances in homology protein structure modeling. Curr. Protein Pept. Sci. 7, 217–227. doi: 10.2174/138920306777452312
Xu, C. G., Yang, Y. B., Zhou, Y. H., Hao, M. Q., Ren, Y. Z., Wang, X. T., et al. (2017). Comparative proteomic analysis provides insight into the key proteins as possible targets involved in aspirin inhibiting biofilm formation of Staphylococcus xylosus. Front. Pharmacol. 8:543. doi: 10.3389/fphar.2017.00543
Yang, Y. B., Chen, J. Q., Zhao, Y. L., Bai, J. W., Ding, W. Y., Zhou, Y. H., et al. (2016). Sub-MICs of azithromycin decrease biofilm formation of Streptococcus suis and increase capsular polysaccharide content of S. suis. Front. Microbiol. 7:1659. doi: 10.3389/fmicb.2016.01659
Yang, Y. B., Wang, S., Wang, C., Huang, Q. Y., Bai, J. W., Chen, J. Q., et al. (2015). Emodin affects biofilm formation and expression of virulence factors in Streptococcus suis ATCC700794. Arch. Microbiol. 197, 1173. doi: 10.1007/s00203-015-1158-4
Keywords: imidazole glycerophosphate dehydratase (IGPD), Staphylococcus xylosus, homology model, virtual screening, biofilm formation
Citation: Chen X-R, Wang X-T, Hao M-Q, Zhou Y-H, Cui W-Q, Xing X-X, Xu C-G, Bai J-W and Li Y-H (2017) Homology Modeling and Virtual Screening to Discover Potent Inhibitors Targeting the Imidazole Glycerophosphate Dehydratase Protein in Staphylococcus xylosus. Front. Chem. 5:98. doi: 10.3389/fchem.2017.00098
Received: 25 August 2017; Accepted: 30 October 2017;
Published: 10 November 2017.
Edited by:
Nino Russo, Università della Calabria, ItalyReviewed by:
Ana Carolina Rennó Sodero, Universidade Federal do Rio de Janeiro, BrazilRobert Vianello, Rudjer Boskovic Institute, Croatia
Copyright © 2017 Chen, Wang, Hao, Zhou, Cui, Xing, Xu, Bai and Li. This is an open-access article distributed under the terms of the Creative Commons Attribution License (CC BY). The use, distribution or reproduction in other forums is permitted, provided the original author(s) or licensor are credited and that the original publication in this journal is cited, in accordance with accepted academic practice. No use, distribution or reproduction is permitted which does not comply with these terms.
*Correspondence: Jing-Wen Bai, YmFpamluZ3dlbkBuZWF1LmVkdS5jbg==
Yan-Hua Li, bGl5YW5odWExOTcwQDE2My5jb20=