- 1College of Veterinary Medicine, Northeast Agricultural University, Harbin, China
- 2Heilongjiang Key Laboratory for Animal Disease Control and Pharmaceutical Development, Harbin, China
- 3College of Science, Northeast Agricultural University, Harbin, China
Glutamine synthetase (GS), which catalyzes the production of glutamine, plays essential roles in most biological growth and biofilm formation, suggesting that GS may be used as a promising target for antibacterial therapy. We asked whether a GS inhibitor could be found as an anti-infective agent of Staphylococcus xylosus (S. xylosus). Here, computational prediction followed by experimental testing was used to characterize GS. Sorafenib was finally determined through computational prediction. In vitro experiments showed that sorafenib has an inhibitory effect on the growth of S. xylosus by competitively occupying the active site of GS, and the minimum inhibitory concentration was 4 mg/L. In vivo experiments also proved that treatment with sorafenib significantly reduced the levels of TNF-α and IL-6 in breast tissue from mice mastitis, which was further confirmed by histopathology examination. These findings indicated that sorafenib could be utilized as an anti-infective agent for the treatment of infections caused by S. xylosus.
Introduction
Mastitis is the most common and critical disease resulting in huge economic losses through the decreased production and milk quality in the global dairy cattle industry (Refaai et al., 2017; Chen et al., 2018). Furthermore, antimicrobial therapy for this disease has been increasingly challenging due to drug resistance (Virdis et al., 2010). Coagulase-negative staphylococci (CoNS) are mainly involved in the inflammatory processes of bovine udders (Pyörälä and Taponen, 2009; Marsilio et al., 2018). Notably, Staphylococcus xylosus (S. xylosus) accounts for about 40% of clinically isolated CoNS strains that cause cow mastitis (Klibi et al., 2018). Thus, novel antibacterial agents for the treatment of infections, including mastitis, caused by S. xylosus are urgently needed.
Glutamine synthetase (GS) has been described as an unusual multitasking protein that functions as an enzyme, a transcription coregulator, and a chaperone in ammonium assimilation as well as in the modulation and regulation of genes involved in biosynthesis of nitrogen and other important proteins (Schumacher et al., 2015). It is involved in the catalysis of ATP-dependent biosynthesis of glutamine from glutamate and ammonia (Murray et al., 2013). Nitrogen metabolism processes are linked to other metabolic networks via glutamine and glutamate (Liu et al., 2015). Glutamine production is essential for most biological growth and biomass production (Chandra et al., 2010). Remarkably, bacterial biofilm is generally controlled by metabolic processes, and nitrogen formation plays a key role in biofilm formation (Krajewski et al., 2008). Biofilms are a community of microorganisms that attaches to biological and non-biological surfaces. Biofilm-forming bacteria are 10–1,000 times more resistant to antimicrobial agents than planktonic cells (Mah and O'Toole, 2001) and have the ability to avoid phagocytosis by macrophages and neutrophils (Thurlow et al., 2011; Domenech et al., 2013), leading to recurrent infections or chronic inflammation. Therefore, GS inhibitors may represent a novel alternative in the control of bacterial infections.
At present, studying cow mastitis by means of virtual screening of target protein GS has not been reported. We used computational prediction followed by experimental testing (Ung et al., 2016) to characterize GS. This current study is the first to demonstrate which unknown compound in the FDA database has antibacterial activity against S. xylosus infection in vitro and in vivo, opening a new direction for the treatment of dairy cow mastitis. The detailed screening work flow is shown in Figure 1.
Materials and Methods
Computational Prediction
Homology Modeling
The homology modeling technique is widely used to model proteins (Muhammed and Aki-Yalcin, 2019). Since the crystal structure of GS from S. xylosus is not available in the PDB database (https://www.rcsb.org/) at the moment, the construction of the 3D model of GS has become key to subsequent research. The amino acid sequence of GS was retrieved from the UniProt database (http://www.uniprot.org). In order to obtain an optimal template for the homology modeling, we did a sequence similarity search using NCBI-BLAST (http:/blast.ncbi.nlm.nih.gov/) to screen against the PDB database. Subsequently, the model was generated using EasyModeller 4.0 (Kuntal et al., 2010). The steps involved in this process were as previously described (Krieger et al., 2003): (i) The amino acid sequence of the selected template protein was identified by software. (ii) The sequence of GS was aligned to the template sequence(s). (iii) The backbone and loop modeling of the modeling were generated. (iv) The complete protein model of GS was formed and initially optimized.
Model Optimization and Evaluation
A protein model using homology modeling has been reported to produce unfavorable bond lengths, bond angles, torsion angles, and contacts. As a result, it was essential to minimize the energy to regularize local bond and angle geometry and to relax close contacts in the geometric chain (Messaoudi et al., 2013). Thus, the model was optimized and evaluated to verify the accuracy of the predicted GS structure. The model of GS was optimized using the Chiron server. Then, the model of GS was verified using the PROCHECK (Laskowski et al., 1993), ERRAT (Colovos and Yeates, 1993), and Verify 3D (Bowie et al., 1991) programs (http://servicesn.mbi.ucla.edu/SAVES/). The established structure was analyzed and visualized by UCSF Chimera. We aligned the structure of GS and its template to determine the active site of GS.
Virtual Screening
Structure-based virtual screening methods (Siddiquee et al., 2007) are important during the early stages of drug discovery, as they can screen compound databases using the active sites of proteins with known 3D structure (Gogoi et al., 2016). The ZINC15 database (Irwin and Shoichet, 2005) is an open source database and contains available chemical compound database (like the FDA database) prepared for virtual screening. The FDA database of listed compounds including a total of 2,924 compounds was downloaded. OpenBabel software converted the compounds in mol2 format to pdbqt format in batches using the script (Table S1a), ensuring that the virtual screening software AutoDock Vina (Trott and Olson, 2010) runs successfully. The processed compounds were used as the test by the batch processing script (Table S1b).
Molecular Docking
To avoid the drawbacks of rapid virtual screening and to obtain the starting structure of the GS/ligands complexes for molecular dynamics (MD) simulation, a standard docking procedure was carried out to find out whether these compounds have new binding modes with GS, so as to find new and more effective GS inhibitor lead compounds. Based on the results of virtual screening, docking studies were performed using CDOCKER (Wu et al., 2003), which adopts a flexible docking program based on CHARMM. During the docking calculations, all the protein residues were fixed and only the compound atoms were mobile. The module computes scores for each that best fits the potential target, which was termed as CDOCKER ENERGY. The best binding mode obtained was analyzed and visualized by PyMOL v2.0.6 software (Lill and Danielson, 2011).
Molecular Dynamics Simulation
The interactions between the GS/sorafenib complex were analyzed using MD simulation in Amber 16 (Case et al., 2005). The general AMBER force field (gaff) and ff14SB force field were used for the ligand and protein, respectively (Wang et al., 2005; Hornak et al., 2006). The energy minimization and equilibration protocol were done using the Sander program (Fu et al., 2018). The bond lengths involving hydrogen atoms were constrained using the SHAKE algorithm (Ryckaert et al., 1977). We determined the preferential binding mode of GS with sorafenib by 60-ns MD simulation based on the docking results. The equilibration of the trajectory was checked by monitoring the equilibration of quantities, such as the root-mean-square deviation (RMSD) with respect to the initial structure. The binding free energy ΔGbind was calculated with the molecular mechanics and Poisson–Boltzmann solvation area (MM/PBSA) methodology for the GS/sorafenib complex based on stable MD trajectory. The free energy decomposition was performed to obtain the key residue contribution to the binding energy of sorafenib in the binding site of GS, analyzed using the MM-PBSA decomposition process. The binding interaction of the ligand–residue pair includes four terms: the Van der Waals contribution (ΔEvdw), the electrostatic contribution (ΔEele), the solvation contribution (ΔEsol), and total contribution (ΔEtotal, ΔEtotal = ΔEvdw + ΔEele + ΔEsol). The energy components are calculated using the same snapshots as the free energy calculation. We choose a total number of 200 snapshots evenly from the last 10 ns on the MD trajectory. The specific details of the complex system have been previously described (Fu et al., 2018).
In vitro Experiments
Bacterial Strains, Growth Conditions, and Reagents
S. xylosus ATCC 700404 was obtained from the American Model Culture Collection Library and grown at 37°C in TSB (Summus Ltd, Harbin, Heilongjiang, China); 98% pure sorafenib was purchased from Beijing Putian Tongchuang Biotech Company and was dissolved in 2.5% DMSO and used at the concentrations indicated.
Minimum Inhibitory Concentration
Minimum inhibitory concentration (MIC) was determined using broth microdilution (Garoff et al., 2018) in TSB medium. The overnight strains were grown in 5 ml TSB medium at 37°C. Then, the cultures of cells were diluted at a concentration of 1 × 105 CFU/ml. Twenty microliters of sorafenib serially diluted in 2.5% DMSO was mixed with 180 μl of TSB containing bacteria in a 96-well microplate (Corning Costar® 3599 Corning, NY, USA). Sorafenib-free 2.5% DMSO mixed with 180 μl of TSB containing bacteria served as the control. The mixtures were incubated for 12 h at 37°C before visual reading. Test procedures and results were determined according to the standard microdilution method recommended by the Clinical and Laboratory Standards Institute (CLSI) (Fothergill, 2012).
Biofilm Formation
S. xylosus was evaluated for its capacity to form a biofilm. Two hundred microliters of cultures was distributed in a 96-well microplate containing 20 μl of sub-MICs of sorafenib solution. The drug concentrations were 1/2, 1/4, 1/8, and 1/16 MIC. The samples without sorafenib served as an untreated control. Similarly, sorafenib-free 2.5% DMSO was used to rule out the effect of solvent on biofilm formation. The specific details have been previously described (Zhou et al., 2018). Eventually, the values of OD at 590 nm were measured for each well using a microtiter plate reader (DG5033A, Huadong Ltd, Nanjing, China).
GS Inhibition Assay
S. xylosus was inoculated into TSB and sorafenib was added at a final concentration of 1/2 MIC (2 mg/L) in a 37°C incubator for 12 h. Control was incubated in the absence of sorafenib. GS test kit (Solarbio Biotechnology Co., Ltd, Beijing, China) was used to determine the activity of GS. The procedure was carried out according to the manufacturer's instructions. Briefly, the sample was centrifuged for 2 min at 12,000 rpm and the clear supernatant was discarded. Then, the bacterial pellet was washed twice with 1 ml of PBS and the supernatant was discarded. Two milliliters of the extract was added to a centrifuge tube containing the bacterial deposits. Bacteria were broken by an ultrasonic cell crusher (Xinzhi Biotechnology Co., Ltd, Ningbo, China). The samples were centrifuged for 10 min at 8,000 g/min at 4°C. The supernatant was collected and set on ice to be tested. Then, 200 μl of supernatant was taken to measure the absorbance at 540 nm in a 96-well microplate using a microtiter plate reader (DG5033A, Huadong Ltd, Nanjing, China).
Determination of Glutamine Content
The content of glutamine was measured using a glutamine test kit (A073, Institute of Biological Engineering, Nanjing, China). According to the manufacturer's instructions, the sample set on the ice from the above procedure was monitored at 630 nm using a UV-7504 ultraviolet spectrophotometer (Jingke Industrial Co., Ltd, Shanghai, China). Furthermore, protein concentrations were calculated by a BCA kit (Solaibao Biotechnology Co., Ltd, Beijing, China).
In vivo Experiments
Animal
Twenty adult (10–12 weeks old) pathogen-free lactating BALB/c female mice that have given birth for 10 days with a mean weight of 30–32 g were purchased from the Experimental Animal Center of the Second Affiliated Hospital of Harbin Medical University. The mice were fed and given water ad libitum, and housed in a controlled environment. The light/dark cycle was 12 h/12 h, the light phase was from 06:00 to 18:00 GMT and the room temperature was 18–22°C. The mice were subjected to the experimental procedure after 3 days of rest. This study was approved and conducted in accordance with the guidelines of the Animal Welfare and Research Ethics Committee of Northeast Agricultural University. Welfare-related assessments and interventions were carried out prior to, during, or after the experiments (Kilkenny et al., 2010).
Treatment of Animals
The mice were randomly divided into four groups with five animals per group (normal group, model group, sorafenib group, and 2.5% DMSO group). The mice were separated from the offspring 12 h before being challenged. Those in the normal group were reared normally. In the model group, 100 μl of S. xylosus with a concentration of 109 CFU/ml was injected into the fourth pair of mouse breasts. In the sorafenib group, the breasts were injected with 100 μl of sorafenib (MIC) after infection for 24 h. The 2.5% DMSO group served as the control.
Enzyme-Linked Immunosorbent Assay
Additional mammary gland tissues from the mice sacrificed at 24 h post-infection were homogenized in normal saline solution. The levels of the inflammatory cytokines TNF-α and IL-6 in the supernatants were measured using an enzyme-linked immunosorbent assay kit (Solaibao Biotechnology Co., Ltd, Beijing, China) according to the manufacturer's instructions. To further investigate the antibacterial activity of sorafenib, the samples were collected and processed as described above to determine the bacterial count by CFU/g.
Histopathological Examination
To evaluate the pathological correlates of mastitis, mammary gland tissues isolated from the sacrificed mice at 24 h post-infection were fixed in 4% paraformaldehyde and embedded in paraffin. Five-micrometer sections were prepared and stained with HE for histopathological examination and visualized by light microscopy.
Statistical Analysis
All the assays were performed in triplicate, and the results were expressed as means ± standard deviations. Statistics were determined using SPSS 19.0. Data were analyzed by the Student's t-test. The degree of statistically significance was reported as follows: *p < 0.05, **p < 0.01.
Results and Discussions
Computational Prediction
Homology Modeling and Verification
The template protein used for homology modeling was the crystal structure (PDB code: 4S0R, with an identity similarity 75%) of Bacillus subtilis (B. subtilis) with a resolution of 3.5 Å. Generally, 30% sequence similarity to a known structure is considered to be the threshold limit for accurate homology modeling (Xiang, 2006), showing that it was the expected template of a highly similar sequence and reasonable for modeling and producing efficient structural models. For sequence alignment between GS (UniProt entry: A0A2T4PF96) and its template, see Figure 2A. The 3D structure of GS was carried out using UCSF Chimera (Figure 2B). As shown in Figure 2C, in making comparison between the structures of GS and its template while considering the binding site similarity, the quality of the structure in the binding site region, and the uniqueness of the conformation, the active region of the mimetic enzyme (X: 112.872, Y: 66.117, Z: −19.217, radius: 15 Å) was determined by superimposing proteins. Furthermore, a close comparison of GS and its target revealed that the RMSD differences were not significant (0.732 Å), suggesting that they have similar structure folds. The active site was a “tunnel-like” three-dimensional structure (Figure 2D), which limits the size of ligands and their binding modes.
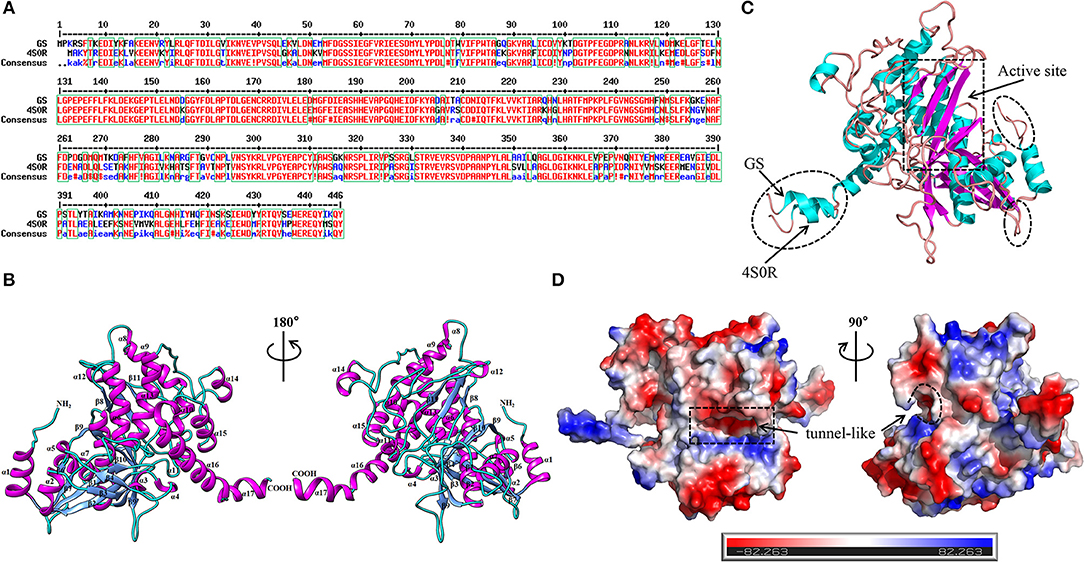
Figure 2. Determination of the 3D structure of the GS. (A) Sequence alignment between the target (GS) and template (PDB code: 4S0R). (B) The 3D structure of the GS. Cartoon diagrams of GS represented in the 180° rotation. The second structures were labeled (α-helices: magenta; β-strands: cornflower blue; random coils: cyan). The N terminus and the C terminus were also labeled. (C) Structural comparison of GS with 4S0R, highlighting their strong structural correspondence in the active site (rectangular dashed lines), and their differences were circled in elliptical dashed lines. (D) Electrostatic potential of the GS observed from different perspectives. The regions with negative or positive charge were colored red or blue, respectively. The catalytic site was marked by the dashed line.
The results of the PROCHECK, ERRAT, and Verify 3D programs are shown in Figures S1–S3. In summary, the verification results proved that the established 3D model of GS was a reasonable structure, which ensured that the subsequent virtual screening was accurate enough.
Virtual Screening
Based on the GS structure, a series of ligands from the FDA database were docked into the protein active site, and their binding affinities were estimated using AutoDock Vina. In Table 1, the optimal conformations with docking energy <-9.0 kcal/mol were selected in the docking search. The binding affinities between the GS and compounds were higher than those of all other compounds tested, implying that these compounds may have stronger inhibitory activity against GS. Hence, these candidate compounds were chosen for further interaction analysis in subsequent experiments.
Molecular Docking
Following the docking simulations, it was observed that only sorafenib in the virtual screening hits (ZINC01493878, Figure 3A) bound with the active site and had the binding free energy (CDOCKER ENERGY: −13.7943 kcal/mol) of GS. The sizes of other small molecules except sorafenib were larger than that of sorafenib to fit into the active cavity, which may be one of the reasons for the unsuccessful docking in this follow-up docking. The potential building mode of the sorafenib with GS at the active cavity was explored in this study. Sorafenib was deeply embedded in the tunnel-like active area (Figure 3B). The molecule is relatively small, leaving room for further optimization toward higher potency (Shen et al., 2014). It was clear that sorafenib bound to GS via hydrogen bonds and electrostatic interactions with the amino acid residues Tyr-158, Glu-186, Asn-212, Arg-333, Arg-318, and Lys-202 (Figure 3C). Since the GS structural level of S. xylosus has not been studied yet, we first mentioned the key amino acids of the active site of the template protein (PDB code: 4S0R). Based on the known studies and analyses, amino acid residues Glu-184, Gly-241, Ser-249, Arg-298, Glu-304, Arg-316, and Arg-335 may have potential links with the catalytic substrates ATP and L-glutamate of the enzyme (Murray et al., 2013; Schumacher et al., 2015). As shown in Figure 1A, the key active amino acids Glu-186, Gly-243, Ser-251, Arg-300, Glu-306, Arg-318, and Arg-337 of the corresponding GS are aligned by sequence similarity. On the one hand, the directly interacting amino acids are Glu-186 and Arg-318, and they may be closely associated with ATP in the process of catalysis. On the other hand, these amino acids are very close in the entire active cavity. Hence, we conclude that the combination of sorafenib with other amino acids may also affect the catalysis of GS on its substrate.
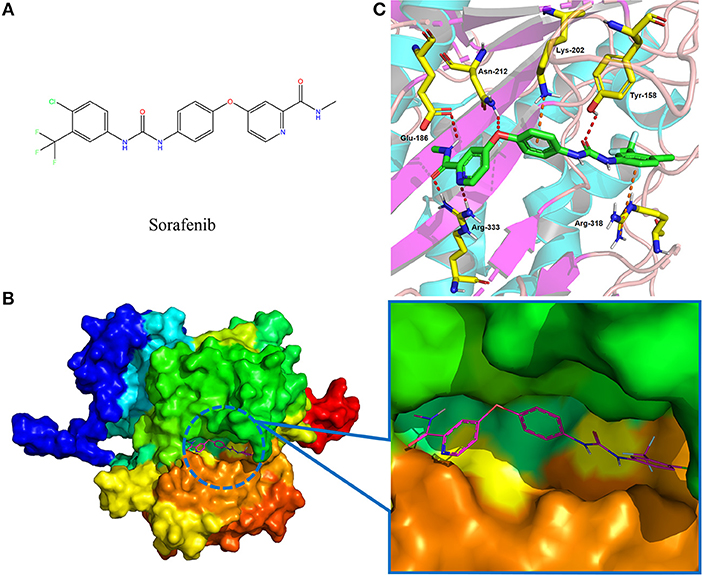
Figure 3. The complex structure model of sorafenib against the binding site of GS. (A) The molecular structure of the sorafenib. (B) Overall view of sorafenib at the tunnel-like active site. Sorafenib was shown in a magenta line model, and GS was shown in a rainbow surface model. (C) Detailed binding mode of sorafenib. Sorafenib was shown in a green stick model, and amino acid residues were shown in yellow sticks. Hydrogen bonds were shown in red dashed lines. Electrostatic interaction bonds were shown in orange dashed lines.
Molecular Dynamics
Initially, the RMSD values of backbone Cα atoms are analyzed to examine whether each system reaches equilibrium, and it can be seen that the complex was found to reach equilibrium at 20 ns (Figure 4A). Figure 4A clearly shows that the RMSD values of the GS/sorafenib complex have a minor change from 20 to 60 ns. The overall results depict the stability of the protein in the simulation time because the RMSD values remain the same with little deviations. The MM/PBSA program was used to calculate the binding free energy from 50 to 60 ns. The ΔEvdw, ΔEele, and ΔGSA energy contributed favorably to ΔGbind, and the positive free energy (ΔGPB/GB) displayed adverse effect for the system. ΔEele made the greatest contribution to the binding free energy of the complex (Fu et al., 2018). Table 2 shows that ΔGbind had a combined free energy value of −25.0237 kcal/mol. As shown in Figure 4B, Tyr-158, Glu-186, Asn-212, and Arg-318 had a strong total binding energy contribution, with ΔEtotal of ≤-1.0 kcal/mol. The residues Tys-202, Glu-306, and Arg-333 also had an appreciable total binding energy contribution, with ΔEtotal of ≤0.5–1.0 kcal/mol. These results suggest that these seven residues are key residues in the GS, except Glu-306, consistent with the CDOCKER docking results. Interestingly, as with the key amino acids mentioned in the molecular docking results, Glu-306 in the GS may be associated with the catalytic substrates L-glutamate of the enzyme. This fully demonstrated the reliability of molecular docking results. The docking displayed the binding orientation in the GS structure, increasing the confidence in our model and approach.
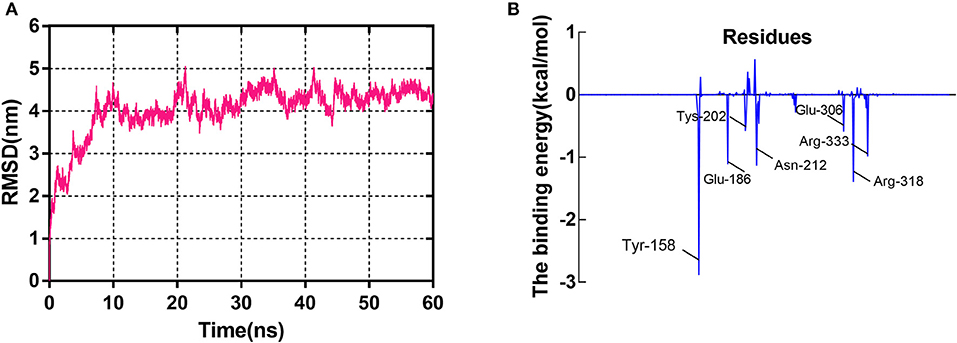
Figure 4. The molecular dynamics (MD) simulation of the GS/sorafenib complex. (A) The root-mean-square deviation (RMSD) calculated for the backbone atoms of the protein during MD simulation. (B) Decomposition of the binding energy on a per-residue basis in the binding sites.
In vitro Experiments
Anti-S. xylosus Activity of Sorafenib
The MIC value for sorafenib against S. xylosus was 4 mg/L, indicating that sorafenib is a relatively highly efficient inhibitor of S. xylosus. In addition, we determined the effect of sub-MICs of sorafenib on biofilm production. As shown in Figure 5A, when compared with the control group, it was observed that the capacity of S. xylosus to form biofilm was significantly inhibited when the sorafenib concentration was 1/2 MIC (2 mg/L), 1/4 MIC (1 mg/L), and 1/8 MIC (0.5 mg/L) (p < 0.05). In contrast, there was no significant effect on the formation of S. xylosus biofilm at 1/16 MIC (0.25 mg/L) (p > 0.05). The effect of the solvent (2.5% DMSO) was also evaluated, and the results indicated that the 2.5% DMSO had no effect on biofilm formation (p > 0.05). These results revealed that sub-inhibitory concentrations of sorafenib could interfere with the biofilm formation of S. xylosus, suggesting that it may be a promising candidate drug in the inhibition of biofilm formation by S. xylosus. However, it is still unclear why sorafenib affected S. xylosus biofilm formation in vitro. Two pertinent questions were considered: (i) How does sorafenib affect S. xylosus biofilm formation at the enzyme level? (ii) Is it really related to the results of our computational prediction? Then, we followed up with a series of experiments below.
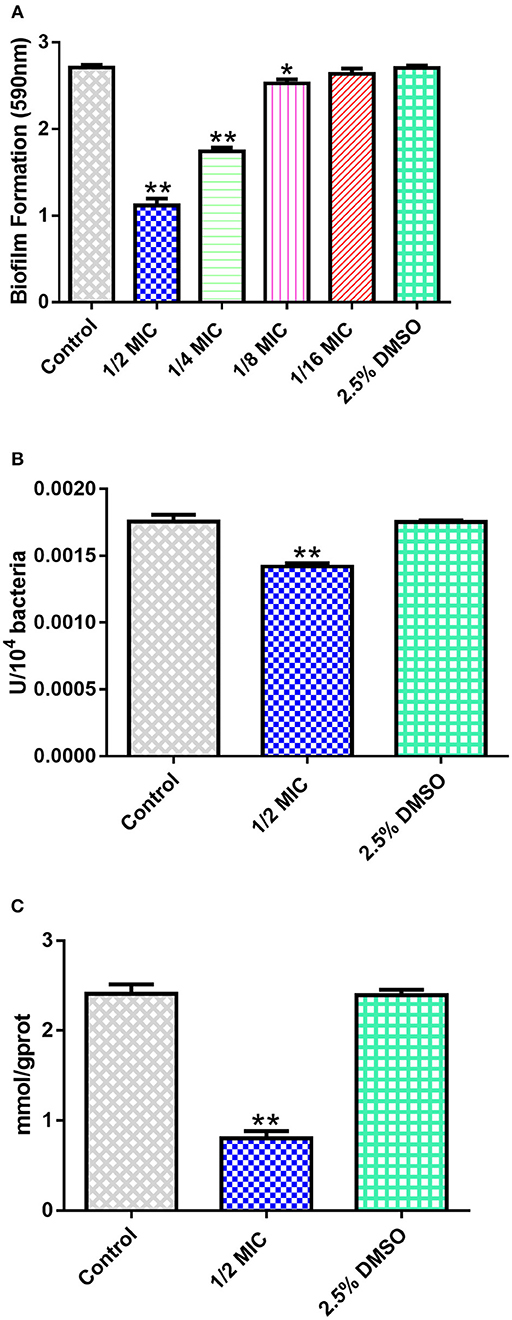
Figure 5. In vitro experiments. (A) Effect of sub-MICs of sorafenib on S. xylosus biofilm formation. (B) The inhibitory effect of 1/2 MIC sorafenib on GS activity. (C) The effect of 1/2 MIC sorafenib on the content of glutamine. The data were expressed as means ± SDs. *p < 0.05, **p < 0.01.
Sorafenib Inhibits GS Activity in S. xylosus
The change in enzyme activity was determined by OD value. As shown in Figure 5B, the activity of GS was significantly lower than that of the control group (p < 0.05). Furthermore, glutamine content was measured to indirectly reflect the activity of GS. It is noteworthy that a similar downward trend was observed when compared with the control group (Figure 5C). Considering the question raised earlier, is the intervention of S. xylosus on biofilm formation achieved by decreasing enzyme activity? In previous studies, GS was encoded by the glnA gene. The loss of the glnA gene results in the inability of B. subtilis to form biofilm on material surfaces, thereby weakening the pathogenicity of pathogens. It also affected cell surface characteristics by changing the chemical composition of the cell wall of B. subtilis (Liu et al., 2015). Interestingly, the structure of GS between S. xylosus and B. subtilis has a high degree of similarity (Figure 2C), so we have reasons to believe that, as we have predicted, sorafenib might be able to bind to the active site of GS, resulting in the reduction of GS activity and glutamine content. Thus, we hypothesized that treatment with sorafenib might inhibit the GS activity and subsequently triggers the reduction of biofilm formation in S. xylosus. It is worth mentioning that sorafenib is already an FDA-approved drug. It has been described as a multi-kinase inhibitor, and it is the first targeted drug that is known to improve the survival rate of patients with advanced hepatocellular carcinoma (Lang, 2008). Hence, the decrease of GS activity or the decrease of biofilm formation may have not only resulted from direct binding of sorafenib to GS. The results may also be related to the known multi-kinase inhibition (Lee et al., 2009).
In vivo Experiments
Determination of TNF-α and IL-6 Inflammatory Factors
TNF-α is an important pro-inflammatory cytokine produced primarily by activated monocyte macrophages (Xu et al., 2006). IL-6 is a pro-inflammatory cytokine that stimulates B cells to produce a variety of inflammatory mediators involved in immune regulation. TNF-α and IL-6 are the most closely associated with inflammation (Winter and Colditz, 2002); they frequently reflect the incidence of mastitis by TNF-α and IL-6 detection methods (Guo et al., 2017). With regard to these observations (Figure 6A), the levels of cytokines, including TNF-α and IL-6, in the infected mice were markedly increased compared with those in the normal group, whereas administration of the sorafenib significantly reduced the S. xylosus-mediated increase in the inflammatory factors levels (p < 0.05). Consistent with these observations (Figure 6B), the bacterial counts in the mammary gland tissues indicated a significant decrease in the sorafenib group compared with the model group (p < 0.05). In addition, the 2.5% DMSO had no effect on the inflammatory cytokine concentration and bacterial counts (p > 0.05). These results showed that the immune cells were stimulated by the pathogenic microorganism to produce TNF-α. The immunoregulation of the sorafenib group inhibited the inflammatory reaction caused by the release of excess TNF-α, which was activated by mononuclear macrophages, and it had control effects on the concentration of IL-6 produced by activated macrophages. In previous studies, sorafenib has been approved by the FDA for the treatment of liver cancer (Lang, 2008). However, its clinical impact on the immune system is unclear. Other studies revealed that sorafenib also has immunomodulatory properties in addition to its anti-cancer effect at a minimal level of applications. It was reported that sorafenib-induced dendritic cells (SIDCs) can inhibit the proliferation of autologous T cells, thereby causing an alteration in their expression of cytokines including IL-1b, IL-2, IL-4, IL-10, IFN-g, IL-6, TNF-α, and CD25 (Zhao et al., 2016). This study was also in line with our findings on the inflammatory factors TNF-α and IL-6. Taken together, these results indicated that not only can sorafenib reduce inflammatory factors by inhibiting the growth of S. xylosus, but it may also act as an immunomodulator in the process.
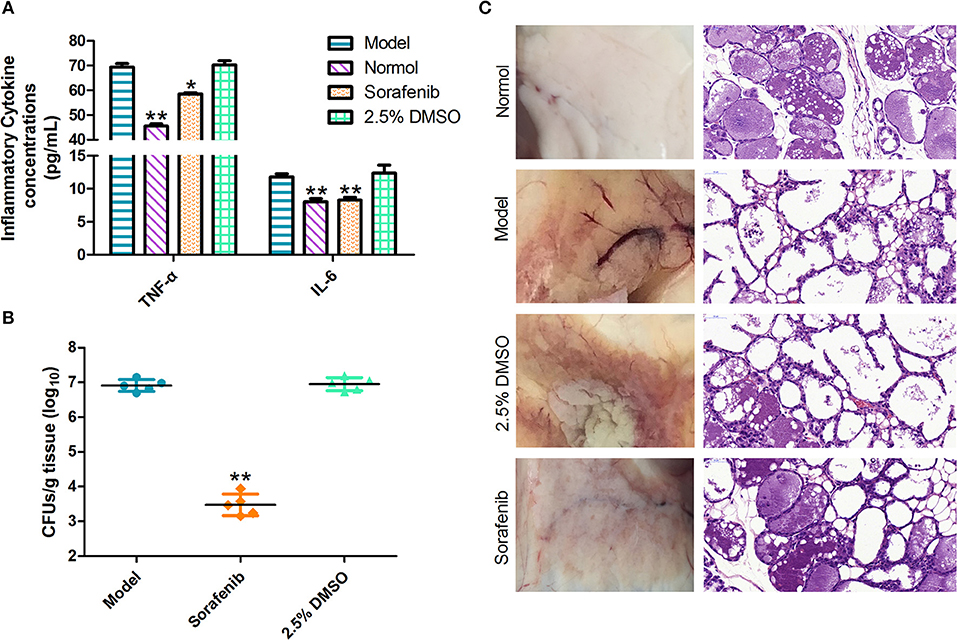
Figure 6. In vivo experiments. (A) Sorafenib reduces the inflammatory response in infected mice. (B) The effect of the sorafenib on the bacterial burden in infected mice. (C) Pathological changes in vision and histopathological analysis. The data were expressed as means ± SDs. *p < 0.05, **p < 0.01.
Histopathological Examination
Following the challenge with S. xylosus, the breasts of lactating mice were injected with sorafenib to evaluate the impact of the treatment. Histopathological analysis of mammary gland tissues at 48 h post-infection was performed. As shown in Figure 6C, results revealed that the structure of the mammary gland was intact, and the epithelial cells were arranged neatly; no pathological change was observed in the normal group. In the model group, the acinar cavity of the mammary gland collapsed and was filled with blood coagulation, and there was a large amount of inflammatory cell infiltration. Besides, the intercellular substance was expanded and the epithelial detachment was seen. Interestingly, in the sorafenib group, the epithelium layer of the mammary gland was intact, and it was columnar or flat. The glandular cavity was highly dilated and filled with secretions. Moreover, apoptotic glandular epithelial cells were observed in the cavity, and inflammatory cell infiltration was intermittently seen in the stroma. There were no abnormalities in the 2.5% DMSO group. The results suggested that there was no pathological change in the mammary gland of mice in the model group before the mouse mastitis model was established. After 24 h of bacterial infection, inflammatory cells infiltrated the mammary gland in a large amount. After treatment with sorafenib, tissue repair, and regeneration were observed in injured tissues, thereby reducing the degree of breast lesions. From the results of our study, it can, therefore, be stated that treatment with sorafenib conferred effective protection against S. xylosus-induced mastitis in the mouse model. As we all know, sorafenib is connected with the known multi-kinase inhibition (Lee et al., 2009). In clinical applications, oral sorafenib was generally well-tolerated in patients with advanced hepatocellular carcinoma, with a manageable adverse event profile. However, it still shows certain side effects, such as diarrhea, hand, and foot skin, and so on (Keating, 2017). Actually, sorafenib shows cytotoxicity that could discourage its administration as an antibacterial agent, although there is a very positive effect on the mouse mastitis model. We consider that some pharmacology studies using a cow model will be used to evaluate efficacy and toxicity in order to explore the clinical application of sorafenib in the next in-depth study. Moreover, the treatment of cow mastitis is usually targeted by breast perfusion. On the one hand, some new pharmaceutical dosage forms can reduce the amount of drug used, like nano-formulations (Huang et al., 2018). We are going to develop sorafenib as a bulk drug into nano-formulations to improve drug targeting and then reduce the dose and the potential side effects of sorafenib. On the other hand, we hope to proceed with the modification of the structure of sorafenib itself, not only enhancing its affinity with GS but also reducing the production of toxicity.
Conclusions
In conclusion, we successfully constructed a rational and hierarchical virtual screening flow to elucidate the potential inhibitor (sorafenib) for GS in S. xylosus. The in vitro study established that we evaluated the anti-S. xylosus activity of sorafenib. In vivo, treatment with the sorafenib remarkably alleviated S. xylosus pathological injury and inflammatory reactions in the mouse model of mastitis infection. Sorafenib has not been previously reported as a GS inhibitor. These results, therefore, provide an interesting template for designing new and more effective GS inhibitors in S. xylosus.
Data Availability
The raw data supporting the conclusions of this manuscript will be made available by the authors, without undue reservation, to any qualified researcher.
Ethics Statement
This study was approved and conducted in accordance with the guidelines of the Animal Welfare and Research Ethics Committee of Northeast Agricultural University. Welfare-related assessments and interventions that were carried out prior to, during, or after the experiment.
Author Contributions
Y-HL designed the whole experiment. W-QC directed the completion of the experiment. Q-WQ, J-PW, J-WB, GB-O, Y-AL, Y-HZ, X-RC, XL, S-DZ, X-XX, and NE were supportive during the experiments.
Funding
This work was supported by the National Key Research and Development Program of China (2018YFD0500300), the National Natural Science Foundation of China (No. 31472231), and the earmarked fund for China Agriculture Research System−35. We thank Shenyang Pharmaceutical University for molecular simulation support.
Conflict of Interest Statement
The authors declare that the research was conducted in the absence of any commercial or financial relationships that could be construed as a potential conflict of interest.
Supplementary Material
The Supplementary Material for this article can be found online at: https://www.frontiersin.org/articles/10.3389/fchem.2019.00381/full#supplementary-material
References
Bowie, J. U., Lüthy, R., and Eisenberg, D. (1991). A method to identify protein sequences that fold into a known three-dimensional structure. Science 253, 164–170. doi: 10.1126/science.1853201
Case, D. A., Cheatham, T. E., Darden, T., Gohlke, H., Luo, R., Merz, K. M., et al. (2005). The Amber biomolecular simulation programs. J. Comput. Chem. 26, 1668–1688. doi: 10.1002/jcc.20290
Chandra, H., Basir, S. F., Gupta, M., and Banerjee, N. (2010). Glutamine synthetase encoded by glnA-1 is necessary for cell wall resistance and pathogenicity of Mycobacterium bovis. Microbiology 156, 3669–3677. doi: 10.1099/mic.0.043828-0
Chen, Z., Xia, H., Shen, H., Xu, X., Arbab, A. A. I., Li, M., et al. (2018). Pathological features of Staphylococcus aureus induced mastitis in dairy cows and isobaric-tags-for-relative-and-absolute-quantitation proteomic analyses. J. Agric. Food Chem. 66, 3880–3890. doi: 10.1021/acs.jafc.7b05461
Colovos, C., and Yeates, T. O. (1993). Verification of protein structures: patterns of nonbonded atomic interactions. Protein Sci. 2, 1511–1519. doi: 10.1002/pro.5560020916
Domenech, M., Ramos-Sevillano, E., Garíca, E., Moscoso, M., and Yuste, J. (2013). Biofilm formation avoids complement immunity and phagocytosis of Streptococcus pneumoniae. Infect. Immun. 81, 2606–2615. doi: 10.1128/IAI.00491-13
Fothergill, A. W. (2012). “Antifungal susceptibility testing: Clinical Laboratory and Standards Institute (CLSI) methods,” in Interactions of Yeasts, Moulds, and Antifungal Agents, ed G. Hall (Humana Press), 65–74. doi: 10.1007/978-1-59745-134-5_2
Fu, Y., Sun, Y. N., Yi, K. H., Li, M. Q., Cao, H. F., Li, J. Z., et al. (2018). Combination of virtual screening protocol by in silico toward the discovery of novel 4-hydroxyphenylpyruvate dioxygenase inhibitors. Front. Chem. 6:15. doi: 10.3389/fchem.2018.00014
Garoff, L., Huseby, D. L., Alzrigat, L. P., and Hughes, D. (2018). Effect of aminoacyl-tRNA synthetase mutations on susceptibility to ciprofloxacin in Escherichia coli. J. Antimicrob. Chemother. 73, 3285–3292. doi: 10.1093/jac/dky356
Gogoi, D., Baruah, V. J., Chaliha, A. K., Kakoti, B. B., Sarma, D., and Buragohain, A. K. (2016). 3D pharmacophore-based virtual screening, docking and density functional theory approach towards the discovery of novel human epidermal growth factor receptor-2 (HER2) inhibitors. J. Theor. Biol. 411, 68–80. doi: 10.1016/j.jtbi.2016.09.016
Guo, Y. F., Xu, N. N., Sun, W. J., Zhao, Y. F., Li, C. Y., and Guo, M. Y. (2017). Luteolin reduces inflammation in Staphylococcus aureus-induced mastitis by inhibiting NF-kB activation and MMPs expression. Oncotarget 8, 28481–28493. doi: 10.18632/oncotarget.16092
Hornak, V., Abel, R., Okur, A., Strockbine, B., Roitberg, A., and Simmerling, C. (2006). Comparison of multiple amber force fields and development of improved protein backbone parameters. Proteins 65, 712–725. doi: 10.1002/prot.21123
Huang, Y. F., Xie, P., Yang, S. T., Zhang, X., Zeng, G., Xin, Q., et al. (2018). Carbon nanoparticles suspension injection for the delivery of doxorubicin: comparable efficacy and reduced toxicity. Mater. Sci. Eng. C-Mater. Biol. Appl. 92, 416–423. doi: 10.1016/j.msec.2018.07.012
Irwin, J. J., and Shoichet, B. K. (2005). ZINC - A free database of commercially available compounds for virtual screening. J. Chem. Inf. Model. 45, 177–182. doi: 10.1021/ci049714+
Keating, G. M. (2017). Sorafenib: a review in hepatocellular carcinoma. Targeted Oncol. 12, 243–253. doi: 10.1007/s11523-017-0484-7
Kilkenny, C., Browne, W., Cuthill, I. C., Emerson, M., and Altman, D. G. (2010). Animal research: reporting in vivo experiments: the ARRIVE guidelines. Br. J. Pharmacol. 160, 1577–1579. doi: 10.1111/j.1476-5381.2010.00872.x
Klibi, A., Maaroufi, A., Torres, C., and Jouini, A. (2018). Detection and characterization of methicillin-resistant and susceptible coagulase-negative staphylococci in milk from cows with clinical mastitis in Tunisia. Int. J. Antimicrob. Agents 52, 930–935. doi: 10.1016/j.ijantimicag.2018.07.026
Krajewski, W. W., Collins, R., Holmberg-Schiavone, L., Jones, T. A., Karlberg, T., and Mowbray, S. L. (2008). Crystal structures of mammalian glutamine synthetases illustrate substrate-induced conformational changes and provide opportunities for drug and herbicide design. J. Mol.Biol. 375, 217–228. doi: 10.1016/j.jmb.2007.10.029
Krieger, E., Nabuurs, S. B., and Vriend, G. (2003). Homology modeling. Methods Biochem. Anal. 44, 509–523. doi: 10.1002/0471721204.ch25
Kuntal, B. K., Aparoy, P., and Reddanna, P. (2010). EasyModeller: a graphical interface to MODELLER. BMC Res. Notes 3:226. doi: 10.1186/1756-0500-3-226
Lang, L. (2008). FDA approves sorafenib for patients with inoperable liver cancer. Gastroenterology 134:379. doi: 10.1053/j.gastro.2007.12.037
Laskowski, R. A., Macarthur, M. W., Moss, D. S., and Thornton, J. M. (1993). PROCHECK: a program to check the stereochemical quality of protein structures. J. Appl. Cryst. 26, 283–291. doi: 10.1107/S0021889892009944
Lee, W. J., Lee, J. L., Chang, S. E., Lee, M. W., Kang, Y. K., Choi, J. H., et al. (2009). Cutaneous adverse effects in patients treated with the multitargeted kinase inhibitors sorafenib and sunitinib. Br. J. Dermatol. 161, 1045–1051. doi: 10.1111/j.1365-2133.2009.09290.x
Lill, M. A., and Danielson, M. L. (2011). Computer-aided drug design platform using PyMOL. J. Comput. Aided Mol. Des. 25, 13–19. doi: 10.1007/s10822-010-9395-8
Liu, J., Prindle, A., Humphries, J., Gabalda-Sagarra, M., Asally, M., Lee, D. Y., et al. (2015). Metabolic co-dependence gives rise to collective oscillations within biofilms. Nature 523, 550–554. doi: 10.1038/nature14660
Mah, T. F., and O'Toole, G. A. (2001). Mechanisms of biofilm resistance to antimicrobial agents. Trends Microbiol. 9, 34–39. doi: 10.1016/S0966-842X(00)01913-2
Marsilio, F., Francesco, C. E. D., and Martino, B.D. (2018). Chapter 4—Coagulase-positive and coagulase-negative staphylococci animal diseases. 43–50. doi: 10.1016/B978-0-12-813547-1.00004-2
Messaoudi, A., Belguith, H., and Ben Hamida, J. (2013). Homology modeling and virtual screening approaches to identify potent inhibitors of VEB-1 beta-lactamase. Theor. Biol. Med. Model. 10:10. doi: 10.1186/1742-4682-10-22
Muhammed, M. T., and Aki-Yalcin, E. (2019). Homology modeling in drug discovery: overview, current applications, and future perspectives. Chem. Biol. Drug Des. 93, 12–20. doi: 10.1111/cbdd.13388
Murray, D. S., Chinnam, N., Tonthat, N. K., Whitfill, T., Wray, L. V., Fisher, S. H., et al. (2013). Structures of the Bacillus subtilis glutamine synthetase dodecamer reveal large intersubunit catalytic conformational changes linked to a unique feedback inhibition mechanism. J. Biol. Chem. 288, 35801–35811. doi: 10.1074/jbc.M113.519496
Pyörälä, S., and Taponen, S. (2009). Coagulase-negative staphylococci—Emerging mastitis pathogens. Vet. Microbiol. 134, 3–8. doi: 10.1016/j.vetmic.2008.09.015
Refaai, W., Gad, M., and Mahmmod, Y. (2017). Association of claw disorders with subclinical intramammary infections in Egyptian dairy cows. Vet. World 10, 358–362. doi: 10.14202/vetworld.2017.358-362
Ryckaert, J. P., Ciccotti, G., and Berendsen, H. J. C. (1977). Numerical integration of the cartesian equations of motion of a system with constraints: molecular dynamics of n-alkanes. J. Comput. Phys. 23, 327–341. doi: 10.1016/0021-9991(77)90098-5
Schumacher, M. A., Chinnam, N. B., Cuthbert, B., Tonthat, N. K., and Whitfill, T. (2015). Structures of regulatory machinery reveal novel molecular mechanisms controlling B. subtilis nitrogen homeostasis. Genes Dev. 29, 451–464. doi: 10.1101/gad.254714.114
Shen, Q., Chen, J., Wang, Q., Deng, X. B., Liu, Y., and Lai, L. H. (2014). Discovery of highly potent TNF alpha inhibitors using virtual screen. Eur. J. Med. Chem. 85, 119–126. doi: 10.1016/j.ejmech.2014.07.091
Siddiquee, K., Zhang, S., Guida, W. C., Blaskovich, M. A., Greedy, B., Lawrence, H. R., et al. (2007). Selective chemical probe inhibitor of Stat3, identified through structure-based virtual screening, induces antitumor activity. Proc. Natl. Acad. Sci. U.S.A. 104, 7391–7396. doi: 10.1073/pnas.0609757104
Thurlow, L. R., Hanke, M. L., Fritz, T., Angle, A., Aldrich, A., Williams, S. H., et al. (2011). Staphylococcus aureus biofilms prevent macrophage phagocytosis and attenuate inflammation in vivo. J. Immunol. 186, 6585–6596. doi: 10.4049/jimmunol.1002794
Trott, O., and Olson, A. J. (2010). Software news and update AutoDock Vina: improving the speed and accuracy of docking with a new scoring function, efficient optimization, and multithreading. J. Comput. Chem. 31, 455–461. doi: 10.1002/jcc.21334
Ung, P. M. U., Song, W. X., Cheng, L. L., Zhao, X. B., Hu, H. L., Chen, L. G., et al. (2016). Inhibitor discovery for the human GLUT1 from homology modeling and virtual screening. ACS Chem. Biol. 11, 1908–1916. doi: 10.1021/acschembio.6b00304
Virdis, S., Scarano, C., Cossu, F., Spanu, V., Spanu, C., and De Santis, E. P. (2010). Antibiotic resistance in Staphylococcus aureus and coagulase negative staphylococci isolated from goats with subclinical mastitis. Vet. Med. Int. 2010:517060. doi: 10.4061/2010/517060
Wang, J. M., Wolf, R. M., Caldwell, J. W., Kollman, P. A., and Case, D. A. (2005). Development and testing of a general amber force field (vol 25, pg 1157, 2004). J. Comput. Chem. 26:114. doi: 10.1002/jcc.20145
Winter, P., and Colditz, I. G. (2002). Immunological responses of the lactating ovine udder following experimental challenge with Staphylococcus epidermidis. Vet. Immunol. Immunopathol. 89, 57–65. doi: 10.1016/S0165-2427(02)00184-8
Wu, G., Robertson, D. H., Brooks, C. L., and Vieth, M. (2003). Detailed analysis of grid-based molecular docking: a case study of CDOCKER—A CHARMm-based MD docking algorithm. J. Comput. Chem. 24, 1549–1562. doi: 10.1002/jcc.10306
Xiang, Z. (2006). Advances in homology protein structure modeling. Curr. Protein Pept. Sci. 7, 217–227. doi: 10.2174/138920306777452312
Xu, J. T., Xin, W. J., Zang, Y., Wu, C. Y., and Liu, X. G. (2006). The role of tumor necrosis factor-alpha in the neuropathic pain induced by Lumbar 5 ventral root transection in rat. Pain 123, 306–321. doi: 10.1016/j.pain.2006.03.011
Zhao, X. X., Cao, M., Lu, Z., Wang, T., Ren, Y., Liu, C., et al. (2016). Small-molecule inhibitor sorafenib regulates immunoreactions by inducing survival and differentiation of bone marrow cells. Innate Immun. 22, 493–502. doi: 10.1177/1753425916659702
Keywords: glutamine synthetase, molecular docking, sorafenib, anti-infective, mastitis
Citation: Cui W-Q, Qu Q-W, Wang J-P, Bai J-W, Bello-Onaghise G, Li Y-A, Zhou Y-H, Chen X-R, Liu X, Zheng S-D, Xing X-X, Eliphaz N and Li Y-H (2019) Discovery of Potential Anti-infective Therapy Targeting Glutamine Synthetase in Staphylococcus xylosus. Front. Chem. 7:381. doi: 10.3389/fchem.2019.00381
Received: 04 March 2019; Accepted: 10 May 2019;
Published: 04 June 2019.
Edited by:
Nino Russo, University of Calabria, ItalyReviewed by:
Sixue Zhang, Southern Research Institute, United StatesFrancesco Ortuso, Università degli Studi Magna Græcia di Catanzaro, Italy
Copyright © 2019 Cui, Qu, Wang, Bai, Bello-Onaghise, Li, Zhou, Chen, Liu, Zheng, Xing, Eliphaz and Li. This is an open-access article distributed under the terms of the Creative Commons Attribution License (CC BY). The use, distribution or reproduction in other forums is permitted, provided the original author(s) and the copyright owner(s) are credited and that the original publication in this journal is cited, in accordance with accepted academic practice. No use, distribution or reproduction is permitted which does not comply with these terms.
*Correspondence: Yan-Hua Li, liyanhua1970@163.com