- UCIBIO-REQUIMTE, Departamento de Química e Bioquímica, Faculdade de Ciências, Universidade do Porto, Porto, Portugal
Iron is a very important transition metal often found in proteins. In enzymes specifically, it is often found at the core of reaction mechanisms, participating in the reaction cycle, more often than not in oxidation/reduction reactions, where it cycles between its most common Fe(III)/Fe(II) oxidation states. QM and QM/MM computational methods that study these catalytic reaction mechanisms mostly use density functional theory (DFT) to describe the chemical transformations. Unfortunately, density functional is known to be plagued by system-specific and property-specific inaccuracies that cast a shadow of uncertainty over the results. Here we have modeled 12 iron coordination complexes, using ligands that represent amino acid sidechains, and calculated the accuracy with which the most common density functionals reproduce the redox properties of the iron complexes (specifically the electronic component of the redox potential at 0 K, ), using the same property calculated with CCSD(T)/CBS as reference for the evaluation. A number of hybrid and hybrid-meta density functionals, generally with a large % of HF exchange (such as BB1K, mPWB1K, and mPW1B95) provided systematically accurate values for , with MUEs of ~2 kcal/mol. The very popular B3LYP density functional was found to be quite precise as well, with a MUE of 2.51 kcal/mol. Overall, the study provides guidelines to estimate the inaccuracies coming from the density functionals in the study of enzyme reaction mechanisms that involve an iron cofactor, and to choose appropriate density functionals for the study of the same reactions.
Introduction
Iron plays an important role in enzyme catalysis. It is present in a wide range of proteins as a cofactor, and intervenes in many biochemical processes, particularly in oxygen transport, and electron transfer. Iron can be often found incorporated in heme prosthetic groups, or it can also occur individually, as an ion cofactor in some metalloproteins, or, more unfrequently, together with sulfide, forming iron-sulfur clusters (Harding et al., 2010; Abbaspour et al., 2014).
One of the most important characteristics of iron is the fact that it can be found in two stable states of oxidation, Fe2+ and Fe3+. It is this characteristic that allows iron to participate in a wide range of redox reactions (Broderick, 2001). In proteins, it is usually found in the active site, coordinated with the side chains of amino acid residues and/or water molecules. Interestingly, the redox potential of the iron ion changes depending on the ligands it is complexed with. In enzymes, through evolution, the ligands to which the iron is coordinated were selected so that its redox potential became the most appropriate for the reaction catalyzed by it (Tamames and Ramos, 2010; Liu et al., 2014).
Iron is of extreme importance to almost all organisms. In adult humans, iron makes up about 0.005% of the total body weight (circa 4 g), most of which is complexed with the heme group in hemoglobin. Iron is also present in cytochromes, heme-containing proteins that are involved in many electron transfer reactions that occur in our body, from oxidative phosphorylation to the synthesis of hormones or the degradation of drugs (Abbaspour et al., 2014).
The reduction potential (or redox potential) of an atom/molecule is, by definition, a measurement of its ability to gain an electron, and therefore be reduced (Equation 1).
The reduction potential is not a fixed value for a given chemical entity, and can change depending on different factors, such as temperature, pH, structure, conformation, and electrostatic environment (Su et al., 2011; Ho et al., 2016). For this reason, the redox potentials of metal cofactors need to be calculated for each enzyme individually. In proteins, iron acts as both an electron donor and acceptor, depending on its oxidation state. When it is in the form of Fe(II), iron will mostly give up one of its electrons, thus being oxidized to Fe(III). On the other hand, when in the form of Fe(III), it can act as a oxidizing agent, gaining one electron, and changing its oxidation state to Fe(II). In some rarer cases, it is possible to find Fe4+ as a cofactor of some enzymes as well, but these cases will not be addressed in this paper.
Since iron is such an important metal in biological processes, with its redox potential changing greatly depending on the ligands coordinated to it, the study of these processes, and the accuracy of the final results, is also determined by how well we understand the redox chemistry of the iron ion. The measurement of reduction potentials in biological systems using experimental methodologies is a very complex and difficult task. The alternative is the use of high-level computational methods, which employ elaborate algorithms, equations, and approximations to simulate the system in question and achieve the most accurate result possible (Riley and Merz, 2007). However, it should be noted that in order to obtain these results one must employ methods that are extremely time consuming and CPU demanding, which is impractical for biological systems such as proteins, where the number of atoms is exceedingly high. It is possible to use less accurate methods when calculating redox potentials in such systems, but the problem in these cases is that, due to their limited accuracy, we might not know which one is the best for each chemical scenario. To solve this problem, we can benchmark the different methods, and compare these results to a reference value, ranking each method as more or less accurate.
The main focus of this work is, therefore, to perform a benchmark study of different computational methods and evaluate their ability to simulate the iron ion in different arrangements, often found in proteins. We selected only density functional theory (DFT) (Thomas, 1927; Hohenberg and Kohn, 1964) methods because of their very good accuracy and applicability on large systems (they have a more favorable scaling with the system size than post-Hartree Fock methods). DFT methods are a good choice when it comes to simulating proteins (Uudsemaa and Tamm, 2003; Riley et al., 2007; Li et al., 2009). The problem comes when selecting which density functional to use for a specific case, since their performance can differ depending on the reaction, model, chemical system, etc. For this reason, benchmarking studies are gaining increasingly more attention, as they give us the ability to make a more informed decision on which DFT methods might be more accurate for the system we want to study.
For this benchmark study, in order to mimic the many complexes that are possible for an iron ion to have in the protein, we constructed 12 models, that span over different coordination numbers and ligands. The models try to mirror the various types of amino acid side chains (and water molecules) that can be most frequently found coordinating iron in proteins. Only one side chain was used in each model, to evaluate the individual effect of each side chain in the redox potential, albeit in proteins the iron coordination shell is made of many (up to six) side chains simultaneously. The coordination shells were completed with water molecules. Water was treated as a coordinating ligand as well.
For each of these models, we calculated the electronic component of the redox potential at 0 K () using the different density functionals. We decided to measure only this component, instead of calculating the redox potential in its entirety for several reasons: this electronic component is the one that contributes the most for the overall potential; it is the only component that can be calculated with CCSD(T), which is the method to be used as a reference; it is the component in which the different density functionals show a larger variation in the final results. Furthermore, calculating only is much easier and faster computationally, and the introduction of the other components in the calculation would render this study much more time consuming with not much improvement in the final results (Su et al., 2011; Ho et al., 2016).
Reference values for , calculated in each system to benchmark the accuracy of the density functionals, were calculated using two post-Hartree-Fock methods: the second order Moller-Plesset perturbation theory (MP2) method, and the single and double coupled cluster theory with perturbative triple correction [CCSD(T)]. The extrapolation energy at the complete basis set (CBS) limit was calculated using two different, widely used extrapolation schemes.
Computational Methods
Model Systems and Structure Optimization
The iron complexes found in proteins are quite diversified. Iron can make complexes with a different number of ligands, and, depending on these, the geometry of the complex will also change. Furthermore, the side chains of the amino acid residues that interact and complex with iron have different functional groups.
In order to take all of these conditions into account in the benchmark, we decided to build 12 model systems, each mimicking a different coordination sphere. These conditions address most of the various coordination states, geometries and ligands that iron complexes can adopt. Our models comprise structures with one, two, four, or six coordinated ligands, which assume geometries of the type linear (for coordination numbers one and two), tetrahedral (for coordination number four), and octahedral (for coordination number six). As for the ligands, we first started with a set of structures in which the iron ion was coordinated exclusively to water molecules, having modeled one structure for each coordination number, with a total of four models. As for the remaining 8, we substituted one of the coordinated waters by one of the following ligands: methoxide (CH3O−), formate (HCOO−), methanethiolate (CH3S−), and methylamine (NH2CH3). This choice of ligands reflects our objective of benchmarking the DFT methods in the context of proteins. Each of these entities represents the side chains of one amino acid that frequently participates in the formation of iron complexes in proteins: CH3O− mimics the side chain of serine, CHOO− that of glutamate and aspartate, CH3S− the side chain of cysteine and NH2CH3 that of lysine. The structures for all the models studied are represented on Figure 1.
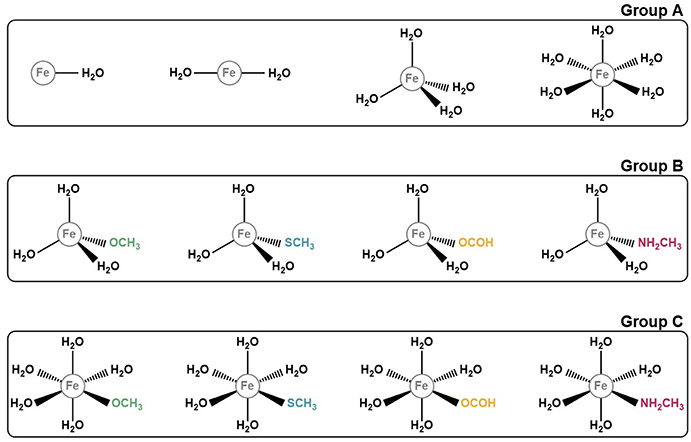
Figure 1. Representation of the 12 iron complexes studied. They were divided in 3 groups according to their ligands for simplicity and for a better discussion. Group A comprises those structures which only have water molecules coordinated to the iron ion. Group B and C include the models with in which one of the ligands mimics an amino acid side chain. The difference between these two groups is their geometry: tetrahedral for group B and octahedral for group C.
After building each of the models, we optimized their geometries using the MP2/6-311+G(d,p) level of theory. One might argue that, for the sake of consistency, it would have been better to calculate for each model using the minimum-energy geometries obtained with each functional we purpose to study. In reality, such study would demand a lot more computational time, for a minimal difference in the final results, as shown in previous studies (Ribeiro et al., 2010; Brás et al., 2011).
Furthermore, MP2 geometries are known to give good results without introducing biases toward any functional. All calculations were performed using the Gaussian 09 package (Frisch et al., 2009).
We optimized the geometries of each complex with both Fe(II) and Fe(III) oxidation states. It is known that, for each oxidation state, the iron ion can have two different spin states. For Fe(II), the high spin multiplicity is five and the low spin multiplicity is one. In the case of Fe(III), the high spin multiplicity is six, and the low one is two. We calculated which of these spin states gave the lowest energy for each oxidation state, and used that spin multiplicity for the remainder of our calculations. In both cases, the high spin multiplicity [five for Fe(II) and six for Fe(III)] was the one that resulted in the lowest energies (Riley and Merz, 2007) (data not shown). As a result, these were the spin states used throughout the following calculations. The <S2> values in each MP2 calculation were checked and compared to the expected values of 6.0 for Fe(II) complexes and 8.75 for Fe(III) complexes. The Gaussian 09 package includes an annihilation step to decrease the amount of spin contamination. For the Fe(II) complexes, we observed an average <S>2 of 6.0079 and 6.0000 before and after annihilation, respectively. Regarding the Fe(III) complexes, the average <S2> before and after the annihilation step were 8.7625 and 8.7501, respectively. Thus, the spin contamination is virtually null.
All calculations were made in gas-phase. As the purpose is to benchmark only and specifically the DFs, we avoided to introduce solvation, so that small differences in solvation would not introduce an unwanted contribution for differences in accuracy of the DFs that are being compared.
Calculation of the Reference Values
In order to correctly benchmark and compare the diverse density functionals, we needed to obtain accurate values for in each of our models.
For this study, we decided to calculate using the very accurate CCSD(T) method (Bartlett and Purvis, 1978; Purvis and Bartlett, 1982; Pople et al., 1987), extrapolated to the CBS limit, and use these values as a reference. To this end, we carried out single point calculations using the MP2/aug-cc-pVXZ (X = 2, 3, 4) and CCSD(T)/aug-cc-pVDZ levels, in vacuum, for each optimized structure, and then we employed two different and well-known extrapolation methods to obtain the final values: one developed by Truhlar (1998) (scheme I) and another by Helgaker et al. (1997), Halkier et al. (1998) (scheme II). Both schemes begin with the extrapolation of the correlation energy to the CBS limit at the MP2 level. The difference between the correlation energies calculated using MP2 and CCSD(T) at the largest basis set possible (for this study, we used aug-cc-pVDZ) is then determined and added to the MP2/CBS value. The extrapolation of the Hartree-Fock (HF) energy is done separately from that of the correlation energy in both schemes as well.
To determine the MP2/CBS energy using scheme I, we employ Equation 2, using the results obtained from the calculations using MP2/aug-cc-pVDZ and MP2/aug-cc-pVTZ. α and β are constants taken from the literature (α = 4.93 and β = 2.13).
As for scheme II, is obtained by exponentially fitting the values obtained for the aug-cc-pVDZ, aug-cc-pVTZ, and aug-cc-pVQZ basis sets. is calculated with Equation 3.
The HF energy is taken from Equation 2, whichever the scheme is used to extrapolate the correlation energy.
Benchmarking of the Density Functionals
We selected a set of 44 density functionals and tested their performance against the values for obtained at the CCSD(T)/CBS level. This selection includes functionals belonging to different classes: 2 local density approximation (LDA) functionals, 7 generalized gradient approximation (GGA), 5 meta-GGA (m-GGA), 12 hybrid-GGA (h-GGA), 10 hybrid-meta-GGA (hm-GGA), 4 double hybrid-GGA (hh-GGA), 1 non-separable gradient approximation (NGA), 1 hybrid-meta-NGA (hm-NGA), 1 meta-NGA (m-NGA), and 1 generalized gradient exchange (GGE) (Table 1). All of the benchmarking calculations were carried out using 6-311+G(2df,2p) basis set, which was selected due to the fact that, for large biological systems, it is the most CBS that can be generally used. Larger basis sets would render the calculations too difficult, making them extremely computationally demanding and time consuming. It is also worth noting that this basis set is frequently close to the DFT CBS limit, and any differences in relative energy (activation energy, reaction energy, redox potentials, etc.) from larger basis sets are usually in the tenths of a kilocalorie per mole. Another reason for the choice of the 6-311+G(2df,2p) basis set was because it is sufficiently large that it minimizes basis set truncation error within DFT, and so we can more accurately evaluate the performance of the functional without the interference from the basis set.
In order to calculate with each of the DFT methods, we used the structures optimized at the MP2/6-311+G(d,p) level of theory, for both Fe(II) and Fe(III) and determined their energies through single point calculations. was then calculated from the difference between each Fe(II)/Fe(III) pair for the corresponding complex. All DFT calculations were carried out using the Gaussian 09 suite (Frisch et al., 2009).
Results and Discussion
Calculation of the Electronic Component of the Reduction Potential at the MP2/CBS Level
In order to obtain an accurate measure of for each iron complex, after the initial geometry optimization of the complexes we used high-level ab initio post-HF methods, with CBS extrapolation to eliminate any basis set truncation error. This was done by calculating these values first with the MP2 method, with aug-cc-pVXZ (X = 2, 3, and 4) with subsequent extrapolation to the MP2/CBS level. Extrapolation to this level was done using two different schemes, as described in the methods section of this paper. These values were used in conjunction with the CCSD(T) results (at the corresponding basis set level) in order to extrapolate the energies to the CCSD(T)/CBS level. In this manner, we ensure that we have reliable values for for each of our 12 models, and that these values can be used as a reference to ascertain the precision of various density functionals in calculating the electronic energy contribution of Fe(II) and Fe(III) in each system. Table 2 summarizes the results obtained for at the MP2 level and the aug-cc-pVDZ, aug-cc-pVTZ, and aug-cc-pVQZ basis sets.
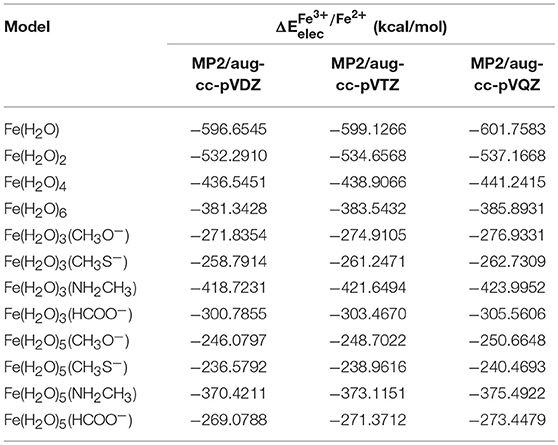
Table 2. Results for calculated at the MP2 level and the aug-cc-pVDZ, aug-cc-pVTZ, and aug-cc-pVQZ basis sets, for each model.
changed by 2–3 kcal/mol when moving from aug-cc-pVDZ to the aug-cc-pVTZ basis set, and by a further ~2–3 kcal/mol when moving from aug-cc-pVTZ to the aug-cc-pVQZ basis set, emphasizing the need for the extrapolation for the CBS limit. As stated previously, we used two different schemes to determine the MP2/CBS energies. The first extrapolation scheme we used was scheme I, developed by D. Truhlar. Table 3 shows the values obtained at the CBS limit using this scheme, as well as the differences between these energies and the corresponding ones at the aug-cc-pVXZ (X = 2, 3, and 4) basis sets.
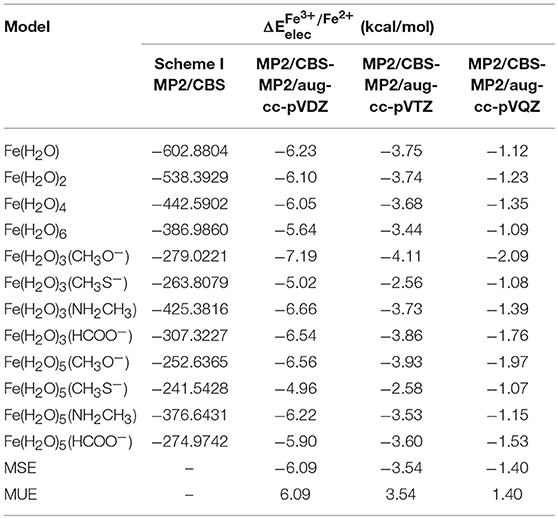
Table 3. obtained at the MP2/CBS level, extrapolated using scheme I, for each model, and the differences between these new energies and the ones obtained in Table 2.
Our results show that MP2/CBS energies obtained with scheme I are closer to those calculated with quadruple-zeta basis set than any of the other basis sets, as expected, and that the difference between the CBS and quadruple-zeta basis set is small, as can be confirmed by comparing the values for the mean signed error (MSE) and mean unsigned error (MUE) for each of them. We can also observe that the convergence of the reduction potential with the basis set is slow, since at quadruple-zeta the values are still 1.40 kcal/mol (in average) away from the CBS limit.
Using scheme II, developed by T. Helgaker and co-workers, to extrapolate to the MP2/CBS level, we obtained the results shown in Table 4. This scheme uses the energies calculated with the aug-cc-pVTZ and aug-cc-pVQZ basis sets for the extrapolation. Since these basis sets are more complete that the ones used in scheme I, it might be expected that the reduction potentials extrapolated using scheme II are more accurate.
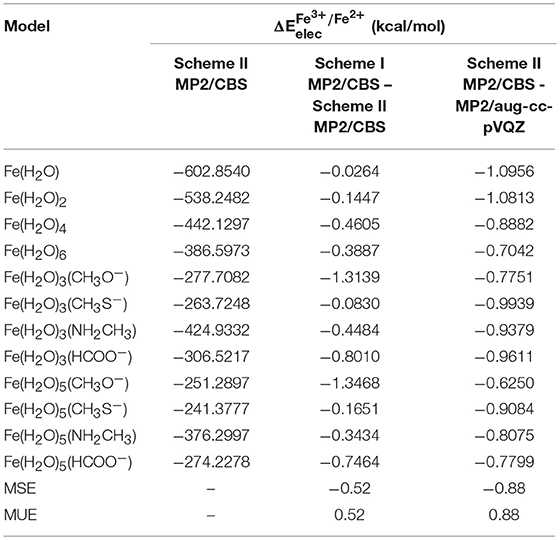
Table 4. obtained at the MP2/CBS level, extrapolated using scheme II for each model, and the differences between these energies and those obtained using scheme I and at the MP2/aug-cc-pVQZ level.
Besides the extrapolated to the MP2/CBS level using scheme II, Table 4 also shows the difference between these values and those extrapolated using scheme I and calculated at the MP2/aug-cc-pVQZ level. From these results, we can observe that there is a slight difference between the scheme II MP2/CBS and MP2/aug-cc-pVQZ energies, with an average of 0.88 kcal/mol. This indicates that, for these systems, the values calculated with quadruple-zeta basis sets are not accurate enough, and extrapolation to the CBS limit is indeed necessary. Furthermore, it is interesting to note that the difference between both extrapolation schemes is small (MUE = 0.52 kcal/mol), but not insignificant, especially considering that both of these methods are expected to yield very accurate results. Although in some cases this difference is small enough that it can be disregarded [0.024 kcal/mol for Fe(H2O) and 0.0830 kcal/mol for Fe(H2O)3(CH3S−)], in cases such as that of Fe(H2O)3(CH3O−) and Fe(H2O)5(CH3O−) it is actually somewhat relevant (~ 1.3 kcal/mol). Since scheme II uses larger basis sets to perform the extrapolation, we chose those energies for the remainder of the work, particularly in the extrapolation to the CCSD(T)/CBS level. It is, however, worth mentioning that, even though the use of large basis sets to get more accurate results is a good practice in systems with a small number of atoms, such methods are impractical for large biological systems, in which case the system would have to be reduced significantly, introducing much larger errors.
Fortunately, DFT methods converge much faster than Post-HF methods in relation to the basis set completeness, and as such they will not be affected by these large basis set truncation errors, as seen here.
Calculation of the Reduction Potential at the CCSD(T)/CBS Level
The last step in the calculation of the reference values for is the extrapolation to the CCSD(T)/CBS level. After obtaining at MP2/CBS, this last step can be easily done by adding to this value the difference between the reduction potentials calculated with MP2 and CCSD(T) (ECCSD(T)−MP2) at the same basis set, aug-cc-pVDZ in our case. This widely used approximation is based on the fact that the difference in correlation energy between CCSD(T) and MP2 does not depend strongly on the basis set, in particular for medium/large basis sets. Due to the high computational cost of CCSD(T) calculations, we obtained with this method with the aug-cc-pVDZ basis set. Previous studies have shown that using a larger basis set in this correction does not significantly alter the extrapolated value, and that the largest difference between using double or triple-zeta when obtaining ECCSD(T)−MP2 was < 0.1 kcal/mol (Jurečka and Hobza, 2002). Jurečka et al. (2006) have also previously stated that double-zeta basis sets are complete enough to obtain a good extrapolation to the CBS limit, and using larger basis sets requires more computational time without much benefit.
Table 5 presents the results at the CCSD(T)/CBS level (as stated previously, CCSD(T)/CBS was extrapolated using the results obtained with extrapolation scheme II). We can observe that the difference between both CCSD(T) and MP2 at the CBS limit is significant, which is a result of the relevant role that correlation energy has in the case of our chemical transformation, which is expectable as an electron is being taken out from the system.
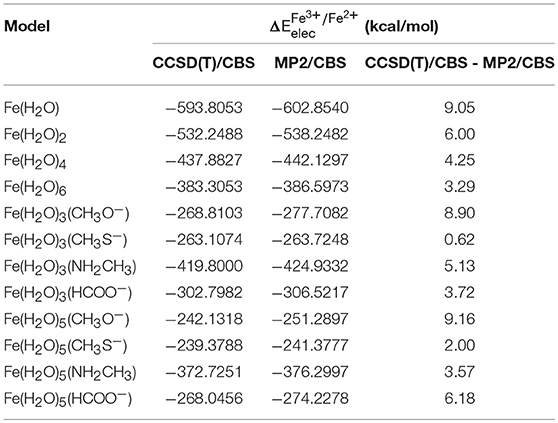
Table 5. obtained at the CCSD(T)/CBS level and at the MP2/CBS level (extrapolated using scheme II), as well as the difference between these two values for each model.
The energies extrapolated to the MP2/CBS level are much closer to the true value than those calculated using a truncated basis set, but these are still, nonetheless, approximations with associated errors that are introduced at different points throughout our calculations. Furthermore, the conversion of the MP2 level into the CCSD(T) level at the CBS limit is also the cause of small error. Overall, it must be taken into account that these results were obtained through several approximations, each of which adding a small error to our final values. In total, we expect our reference values ( calculated at the CCSD(T)/CBS level) to have an associated uncertainty of 1 kcal/mol or less, in most of the cases. As such, a qualitative distinction between density functionals having a difference smaller than 1 kcal/mol between them should not be made.
Benchmarking of the DFT Functionals
After obtaining the reference values for of iron in each of the complexes, we can now use these results to evaluate the performance of the 44 density functionals we have selected. It should be noted that this benchmark study does not represent the overall quality of each of the DFT, but merely indicates, for these systems in specific (which we expect to be representative of aminoacid side-chain coordination shells), which of them are better at estimating the chemical property we wish to study (iron reduction potential, in our case).
From the optimized geometries for all systems, we performed single point calculation with each of the 44 density functionals, and calculated using the basis set 6-311+G(2df,2p). We selected a large basis set in order to minimize truncation errors, which means that the fluctuations on the results arise essentially from the density functional. The values obtained for will be compared to the reference values calculated at the CCSD(T)/CBS level.
In order to make discussing the results easier, and because our study involves several different systems, we decided to group our models in 3 distinct groups (Figure 1). Group A comprises the iron complexes with only water as their ligand, with coordination numbers 1, 2, 4, and 6, whereas groups B and C include complexes which contain one ligand that represents the side chain of an amino acid. What differentiates these 2 groups is the coordination number of the iron ion: 4 for group B and 6 for group C. The iron is complexed with 3 and 5 water molecules, respectively, plus the ligand simulating the amino acid side chain.
For the next part of this paper, we will only analyze the ten functionals that gave the best performance for each case. We decided to present our results in this fashion in order to make a more focused and easy-to-read discussion. A full table displaying the results for all the DFs tested is available in the Supporting Information section of this paper.
We begin our discussion with the systems belonging to group A.
Table 6 represents the results obtained for the ten density functionals that showed the best performance for this group. Performance is measured as the difference between calculated with each DFT and the reference value (MUE). We also show the maximum error (MaxE), which is the model were the difference between the value calculated with the reference value and the energy calculated with the density functional was higher.
Taking these results into account, we can further divide the DFs into 3 separate groups. Group I includes all the density functionals with MUE between 0 and 2.31 kcal/mol (which corresponds to an error below 0.1 V per electron transfer). Group II comprises functionals with MUE between 2.31 and 4.62 kcal/mol (between 0.1 and 0.2 V per electron transfer) and group III encompasses those whose MUE is higher than 4.62 kcal/mol. The density functionals whose performance puts them in group I are BB1K, mPWB1K, and mPW1N. It is interesting to note that these DFs all belong to either the hybrid-meta-GGA or hybrid-GGA class, and that in the whole table only two functionals do not belong to these classes. The first four functionals all have a rather high HF exchange percentage, higher than 40%. The very popular B3LYP functional appears at 9th place in our table as well, and its MUE puts it in group II. It is also worth noting that the Fe(H2O)6 system is the one where the largest error is more frequently found.
We could also measure the performance of the tested functionals in terms of MaxE. However, and taking the results in Table 6 into account, we can observe that both MUE and MaxE show a similar trend, and that those functionals with a smaller MUE also tend to have a smaller MaxE, with a few exceptions. For this reason, we decided to continue to rank the DFs in terms of MUE.
For group B, we performed the same analysis as group A. As stated previously, this group consists of systems with three water molecules and one ligand coordinated to the iron ion in a tetrahedral geometry. Each ligand represents a different amino acid side chain (CH3O− for serine, HCOO− for glutamate and aspartate, CH3S− for cysteine and NH2CH3 for lysine), which correspond to the most common residues that participate in iron complexes of proteins.
The results for group B (Table 7), show that the DFs that display a better accuracy for this group are mostly similar to those that performed better for system A as well. The density functionals that belong to group I in this case are BMK and B3LYP, which are, yet again, either hybrid-meta-GGAs or hybrid-GGAs. The HF correlation percentage for most of the top ten functionals is also higher than 40%, with MN-12L as a remarkable exception. The system that contributes the most to the maximum error in this group is Fe(CH3S−)(H2O)3.
And finally, we reach our last group, group C, composed of iron complexes with coordination number 6, in which one of the ligand is a chemical group representing an amino acid side chain, and the other five are water molecules. The results obtained for the benchmarking performed for group C are present in Table 8. Six functionals have MUE values < 2.31 kcal/mol, placing them in group I. These are mPW1B95, PBE1PBE, B3PW91, wB97X-D, BB1K, and mPWB1K. The percentage of HF exchange is not as high for this group, but still some of the functionals placed in the top ten have more the 40% HF exchange. Again for group C, all of the functionals in group I, and indeed in the whole table, are either hybrid-meta-GGAs or hybrid-GGAs. In accordance with the results for group B, for group C it is also the complex with CH3S− that contributed the most times to the MaxE. B3LYP shows a good performance in this group as well, ranking in the 7th position. We also assessed the contribution of the dispersion effect on the iron complexes of group C, which are those that have a higher number of ligands and, therefore, where the dispersion interactions should be more noticeable. In this sense, we calculated the Grimme's dispersion correction (Grimme et al., 2010; Goerigk and Grimme, 2011) with the top ten functionals (Table 8) and concluded that its inclusion marginally enhances the results, as the average MUE was reduced by ~0.15 kcal/mol. However, considering the precision of the reference CCSD(T)/CBS method (about 0.5–1 kcal/mol), we can assume that the inclusion of the dispersion correction does not significantly influence the results and, consequently, the gathered illations.
Having analyzed the performance of the 44 density functionals in estimating the reduction potentials of iron complexes in the three separate groups, we will now make an overall appreciation of their performance for all the systems studies as a whole.
Table 9 presents the ten functionals that showed the best performance in calculating for the studied iron complexes. Three functionals MUE values that put them in group I: BB1K, mPWB1K, and mPW1B95. All of them belong to the hybrid-meta-GGA class of functionals. The very popular B3LYP functional ranks 5th in this overall analysis. All functionals present in the top ten are either hybrid-meta-GGAs or hybrid-GGAs, and the percentage of HF exchange is higher than 40% in most of the cases. We can therefore conclude that both of these classes are the best at estimating the of the iron complexes studied, and should be used when calculating the reduction potential of iron complexes.
Conclusion
In our study, we analyzed the performance of 44 density functionals in estimating the electronic component of the reduction potential at 0 K of 16 different iron complexes, with a special interest in iron complexes with ligands similar to some amino acid side-chains. We were able to conclude which of these DFs gave results closer to reference values calculated using very high level computational methods, with posterior extrapolation to the very accurate CCSD(T)/CBS level of theory, which was done with two different extrapolation schemes. An initial geometry optimization for each of the 16 systems was carried out with MP2/6-311+G(d,p) level of theory, followed by single-point calculations with each of the 44 selected functional with the 6-311+G(2df,2p) basis set. From the difference between the energies obtained with Fe2+ and Fe3+, we calculated for all complexes, and then compared the results obtained with the reference values, ranking the DFs according to this difference.
Our results show that the difference between the extrapolated values for the level MP2/CBS calculated with both extrapolation schemes is very small (average difference is 0.52 kcal/mol). For the final extrapolation to the CCSD(T)/CBS level of the reduction potentials we selected the values calculated with scheme II, since it uses results obtained with a larger basis set for the extrapolation.
The benchmarking study showed us that the best functionals to calculate the reduction potential of iron complexes, especially those associated to biological systems, belong to the hybrid or hybrid-meta-GGAs classes, and have a high percentage of HF exchange. Overall, BB1K, mPWB1K, and mPW1B95 were the functionals that performed better for all iron complexes studied (average MUE 1.72, 1.93 and 2.28 kcal/mol, respectively). The very popular functional B3LYP gave a rather good performance, ranking 5th in our list of best functionals.
As a final remark it is important to note that there is an estimate of a 1 kcal/mol error in our reference values, which means that it is very difficult to assess the overall performance of functionals that differ by <1 kcal/mol between them. Nevertheless, our results are significant to show which DFs are more likely to perform well with iron complexes that incorporate biological systems, even if we are not able to show which, among all of them, is the single best density functional.
Data Availability
All datasets generated for this study are included in the manuscript and/or the Supplementary Files.
Author Contributions
RL performed most of the calculations, analyzed the results, and contributed to the writing of the manuscript. DG and PP performed a part of the calculations, analyzed the results, and contributed to the writing of the manuscript. MR and PF planned the research, analyzed the results, and contributed to the writing of the manuscript.
Funding
This work received financial support from the European Union (FEDER funds POCI/01/0145/FEDER/007728) and National Funds (FCT/MEC, Fundação para a Ciência e Tecnologia and Ministério da Educação e Ciência) under the Partnership Agreement PT2020 UID/MULTI/04378/2013 and UID/MULTI/04378/2019, as well as through the project PTDC/QUI-QFI/28714/2017.
Conflict of Interest Statement
The authors declare that the research was conducted in the absence of any commercial or financial relationships that could be construed as a potential conflict of interest.
Acknowledgments
We acknowledge Fundação para a Ciência e Tecnologia through grants IF/00052/2014 and PD/BD/135268/2017.
Supplementary Material
The Supplementary Material for this article can be found online at: https://www.frontiersin.org/articles/10.3389/fchem.2019.00391/full#supplementary-material
References
Abbaspour, N., Hurrell, R., and Kelishadi, R. (2014). Review on iron and its importance for human health. J. Res. Med sci. 19, 164–174.
Adamo, C., and Barone, V. (1997). Toward reliable adiabatic connection models free from adjustable parameters. Chem. Phys. Lett. 274, 242–250. doi: 10.1016/S0009-2614(97)00651-9
Adamo, C., and Barone, V. (1998a). Implementation and validation of the Lacks-Gordon exchange functional in conventional density functional and adiabatic connection methods. J. Comput. Chem. 19, 418–429. doi: 10.1002/(SICI)1096-987X(199803)19:4<418::AID-JCC4>3.0.CO;2-V
Adamo, C., and Barone, V. (1998b). Exchange functionals with improved long-range behavior and adiabatic connection methods without adjustable parameters: the mPW and mPW1PW models. J. Chem. Phys. 108, 664–675. doi: 10.1063/1.475428
Bartlett, R. J., and Purvis, G. D. (1978). Many-body perturbation theory, coupled-pair many-electron theory, and the importance of quadruple excitations for the correlation problem. Int. J. Quant. Chem. 14, 561–581. doi: 10.1002/qua.560140504
Becke, A. D. (1988). Density-functional exchange-energy approximation with correct asymptotic behavior. Phys. Rev. A 38, 3098–3100. doi: 10.1103/PhysRevA.38.3098
Becke, A. D. (1993). A new mixing of hartree–fock and local density-functional theories. J. Chem. Phys. 98, 1372–1377. doi: 10.1063/1.464304
Becke, A. D. (1996). Density-functional thermochemistry. IV. a new dynamical correlation functional and implications for exact-exchange mixing. J. Chem. Phys. 104, 1040–1046. doi: 10.1063/1.470829
Boese, A. D., Martin, J. M., and Handy, N. C. (2003). The role of the basis set: Assessing density functional theory. J. Chem. Phys. 119, 3005–3014. doi: 10.1063/1.1589004
Boese, A. D., and Martin, J. M. L. (2004). Development of density functionals for thermochemical kinetics. J. Chem. Phys. 121, 3405–3416. doi: 10.1063/1.1774975
Brás, N. F., Perez, M. A. S., Fernandes, P. A., Silva, P. J., and Ramos, M. J. (2011). Accuracy of density functionals in the prediction of electronic proton affinities of amino acid side chains. J. Chem. Theory Comput. 7, 3898–3908. doi: 10.1021/ct200309v
Broderick, J. B. (2001). Coenzymes and Cofactors. Encyclopedia of Life Sciences. Chichester, UK: John Wiley and Sons, Ltd.
Burke, K., Perdew, J., Wang, Y., Dobson, J., Vignale, G., and Das, M. (1998). Electronic Density Functional Theory: Recent Progress and New Directions. Springer. 81–111.
Chai, J.-D., and Head-Gordon, M. (2008). Long-range corrected hybrid density functionals with damped atom–atom dispersion corrections. Phys. Chem. Chem. Phys. 10:6615. doi: 10.1039/b810189b
Frisch, M., Trucks, G., Schlegel, H., Scuseria, G., Robb, M., Cheeseman, J., et al. (2009). Gaussian09 Revision D. 01, Gaussian Inc. Wallingford CT. Available online at: http://www.gaussian.com
Gill, P. (1996). A new gradient-corrected exchange functional. Mol. Phys. 89, 433–445. doi: 10.1080/002689796173813
Goerigk, L., and Grimme, S. (2011). A thorough benchmark of density functional methods for general main group thermochemistry, kinetics, and noncovalent interactions. Phys. Chem. Chem. Phys. 13, 6670–6688. doi: 10.1039/c0cp02984j
Grimme, S. (2006). Semiempirical hybrid density functional with perturbative second-order correlation. J. Chem. Phys. 124:034108. doi: 10.1063/1.2148954
Grimme, S., Antony, J., Ehrlich, S., and Krieg, H. (2010). A consistent and accurate ab initio parametrization of density functional dispersion correction (DFT-D) for the 94 elements H-Pu. J. Chem. Phys. 132:154104. doi: 10.1063/1.3382344
Grimme, S., Ehrlich, S., and Goerigk, L. (2011). Effect of the damping function in dispersion corrected density functional theory. J. Comput. Chem. 32, 1456–1465. doi: 10.1002/jcc.21759
Halkier, A., Helgaker, T., Jørgensen, P., Klopper, W., Koch, H., Olsen, J., et al. (1998). Basis-set convergence in correlated calculations on Ne, N2, and H2O. Chem. Phys. Lett. 286, 243–252. doi: 10.1016/S0009-2614(98)00111-0
Hamprecht, F. A., Cohen, A. J., Tozer, D. J., and Handy, N. C. (1998). Development and assessment of new exchange-correlation functionals. J. Chem. Phys. 109, 6264–6271. doi: 10.1063/1.477267
Handy, N. C., and Cohen, A. J. (2001). Left-right correlation energy. Mol. Phys. 99, 403–412. doi: 10.1080/00268970010018431
Harding, M. M., Nowicki, M. W., and Walkinshaw, M. D. (2010). Metals in protein structures: a review of their principal features. Crystallogr. Rev. 16, 247–302. doi: 10.1080/0889311X.2010.485616
Helgaker, T., Klopper, W., Koch, H., and Noga, J. (1997). Basis-set convergence of correlated calculations on water. J. Chem. Phys. 106, 9639–9646. doi: 10.1063/1.473863
Ho, J., Coote, M. L., Cramer, C. J., and Truhlar, D. G. (2016). “Theoretical calculation of reduction potentials,” in Organic Electrochem, 5th Edn, eds O. Hammerich and B. Speiser (CRC Press), 229–259.
Hoe, W.-M., Cohen, A. J., and Handy, N. C. (2001). Assessment of a new local exchange functional OPTX. Chem. Phys. Lett. 341, 319–328. doi: 10.1016/S0009-2614(01)00581-4
Hohenberg, P., and Kohn, W. (1964). Inhomogeneous electron gas. Phys Rev. 136:B864. doi: 10.1103/PhysRev.136.B864
Jurečka, P., and Hobza, P. (2002). On the convergence of the (ΔECCSD(T)–ΔEMP2) term for complexes with multiple H-bonds. Chem. Phys. Lett. 365, 89–94. doi: 10.1016/S0009-2614(02)01423-9
Jurečka, P., Šponer, J., Cern,ý, J., and Hobza, P. (2006). Benchmark database of accurate (MP2 and CCSD(T) complete basis set limit) interaction energies of small model complexes, DNA base pairs, and amino acid pairs. Phys. Chem. Chem. Phys. 8, 1985–1993. doi: 10.1039/B600027D
Karton, A., Tarnopolsky, A., Lamère, J.-F. o, Schatz, G. C., and Martin, J. M. L. (2008). Highly accurate first-principles benchmark data sets for the parametrization and validation of density functional and other approximate methods. derivation of a robust, generally applicable, double-hybrid functional for thermochemistry and thermochemical kinetics†. J. Phys. Chem. A 112, 12868–12886. doi: 10.1021/jp801805p
Kohn, W., and Sham, L. J. (1965). Self-consistent equations including exchange and correlation effects. Phys. Rev. 140, A1133–A1138. doi: 10.1103/PhysRev.140.A1133
Kormos, B. L., and Cramer, C. J. (2002). Adiabatic connection method for X−+RX nucleophilic substitution reactions (X = F, Cl). J. Phys. Organic Chem. 15, 712–720. doi: 10.1002/poc.548
Kozuch, S., Gruzman, D., and Martin, J. M. L. (2010). DSD-BLYP: A general purpose double hybrid density functional including spin component scaling and dispersion correction. J. Phys. Chem. C 114, 20801–20808. doi: 10.1021/jp1070852
Lee, C., Yang, W., and Parr, R. G. (1988). Development of the colle-salvetti correlation-energy formula into a functional of the electron density. Phys. Rev. B 37, 785–789. doi: 10.1103/PhysRevB.37.785
Li, S., Hennigan, J. M., Dixon, D. A., and Peterson, K. A. (2009). Accurate thermochemistry for transition metal oxide clusters. J. Phys. Chem. A 113, 7861–7877. doi: 10.1021/jp810182a
Liu, J., Chakraborty, S., Hosseinzadeh, P., Yu, Y., Tian, S., Petrik, I., et al. (2014). Metalloproteins containing cytochrome, iron–sulfur, or copper redox centers. Chem. Rev. 114, 4366–4469. doi: 10.1021/cr400479b
Lynch, B. J., Fast, P. L., Harris, M., and Truhlar, D. G. (2000). Adiabatic connection for kinetics. J. Phys. Chem. A 104, 4811–4815. doi: 10.1021/jp000497z
Perdew, J. P. (1986a). Erratum: Density-functional approximation for the correlation energy of the inhomogeneous electron gas. Phys. Rev. B 34, 7406–7406. doi: 10.1103/PhysRevB.34.7406
Perdew, J. P. (1986b). Density-functional approximation for the correlation energy of the inhomogeneous electron gas. Phys. Rev. B. 33, 8822–8824. doi: 10.1103/PhysRevB.33.8822
Perdew, J. P., Burke, K., and Ernzerhof, M. (1996b). Generalized gradient approximation made simple. Phys. Rev. Lett. 77, 3865–3868. doi: 10.1103/PhysRevLett.77.3865
Perdew, J. P., Burke, K., and Wang, Y. (1996a). Generalized gradient approximation for the exchange-correlation hole of a many-electron system. Phys. Rev. B 54, 16533–16539. doi: 10.1103/PhysRevB.54.16533
Perdew, J. P., Chevary, J. A., Vosko, S. H., Jackson, K. A., Pederson, M. R., Singh, D. J., et al. (1992). Atoms, molecules, solids, and surfaces: applications of the generalized gradient approximation for exchange and correlation. Phys. Rev. B 46:6671. doi: 10.1103/PhysRevB.46.6671
Perdew, J. P., Chevary, J. A., Vosko, S. H., Jackson, K. A., Pederson, M. R., Singh, D. J., et al. (1993). Erratum: Atoms, molecules, solids, and surfaces: Applications of the generalized gradient approximation for exchange and correlation. Phys. Rev. B 48, 4978–4978. doi: 10.1103/PhysRevB.48.4978.2
Perdew, J. P., Ziesche, P., and Eschrig, H. (1991). Electronic Structure of Solids' 91. Berlin: Akademie Verlag.
Perdew, J. P., and Zunger, A. (1981). Self-interaction correction to density-functional approximations for many-electron systems. Phys. Rev. B 23, 5048–5079. doi: 10.1103/PhysRevB.23.5048
Peverati, R., and Truhlar, D. G. (2011a). Improving the accuracy of hybrid meta-GGA density functionals by range separation. J. Phys. Chem. Lett. 2, 2810–2817. doi: 10.1021/jz201170d
Peverati, R., and Truhlar, D. G. (2011b). M11-L: a local density functional that provides improved accuracy for electronic structure calculations in chemistry and physics. J. Phys. Chem. Lett. 3, 117–124. doi: 10.1021/jz201525m
Peverati, R., and Truhlar, D. G. (2012a). Exchange–correlation functional with good accuracy for both structural and energetic properties while depending only on the density and its gradient. J. Chem. Theory Comput. 8, 2310–2319. doi: 10.1021/ct3002656
Peverati, R., and Truhlar, D. G. (2012b). An improved and broadly accurate local approximation to the exchange–correlation density functional: The MN12-L functional for electronic structure calculations in chemistry and physics. Phys. Chem. Chem. Phys. 14:13171. doi: 10.1039/c2cp42025b
Peverati, R., and Truhlar, D. G. (2012c). Screened-exchange density functionals with broad accuracy for chemistry and solid-state physics. Phys. Chem. Chem. Phys. 14:16187. doi: 10.1039/c2cp42576a
Pople, J. A., Head-Gordon, M., and Raghavachari, K. (1987). Quadratic configuration interaction. a general technique for determining electron correlation energies. J. Chem. Phys. 87, 5968–5975. doi: 10.1063/1.453520
Purvis, I. I. I. G. D., and Bartlett, R. J. (1982). A full coupled-cluster singles and doubles model: the inclusion of disconnected triples. J. Chem. Phys. 76, 1910–1918. doi: 10.1063/1.443164
Ribeiro, A. J., Ramos, M. J., and Fernandes, P. A. (2010). Benchmarking of DFT functionals for the hydrolysis of phosphodiester bonds. J. Chem. Theory comput. 6, 2281–2292. doi: 10.1021/ct900649e
Riley, K. E., and Merz, K. M. (2007). Assessment of density functional theory methods for the computation of heats of formation and ionization potentials of systems containing third row transition metals. J. Phys. Chem. A 111, 6044–6053. doi: 10.1021/jp0705931
Riley, K. E., Op't Holt, B. T., and Merz, K. M. (2007). Critical assessment of the performance of density functional methods for several atomic and molecular properties. J. Chem. Theory Comput. 3, 407–433. doi: 10.1021/ct600185a
Schwabe, T., and Grimme, S. (2006). Towards chemical accuracy for the thermodynamics of large molecules: new hybrid density functionals including non-local correlation effects. Phys. Chem. Chem. Phys. 8:4398. doi: 10.1039/b608478h
Slater, J. C., and Phillips, J. C. (1974). Quantum theory of molecules and solids Vol. 4: the self-consistent field for molecules and solids. Phys Today. 27, 49–50. doi: 10.1063/1.3129035
Su, N. Q., Zhang, I. Y., Wu, J., and Xu, X. (2011). Calculations of ionization energies and electron affinities for atoms and molecules: a comparative study with different methods. Front Chem China. 6, 269–279. doi: 10.1007/s11458-011-0256-3
Tamames, J. A. C., and Ramos, M. J. (2010). Metals in proteins: cluster analysis studies. J Molecul Model. 17, 429–442. doi: 10.1007/s00894-010-0733-5
Tao, J., Perdew, J. P., Staroverov, V. N., and Scuseria, G. E. (2003). Climbing the density functional ladder: nonempirical meta–generalized gradient approximation designed for molecules and solids. Phys Rev Lett. 91:146401. doi: 10.1103/PhysRevLett.91.146401
Thomas, L. H. (1927). “The calculation of atomic fields,” in Mathematical Proceedings of the Cambridge Philosophical Society, Cambridge University Press. 542–548. doi: 10.1017/S0305004100011683
Truhlar, D. G. (1998). Basis-set extrapolation. Chem Phys Lett. 294, 45–48. doi: 10.1016/S0009-2614(98)00866-5
Uudsemaa, M., and Tamm, T. (2003). Density-functional theory calculations of aqueous redox potentials of fourth-period transition metals. J Phys Chem A. 107, 9997–10003. doi: 10.1021/jp0362741
Van Voorhis, T., and Scuseria, G. E. (1998). A novel form for the exchange-correlation energy functional. J Chem Phys. 109, 400–410. doi: 10.1063/1.476577
Vosko, S. H., Wilk, L., and Nusair, M. (1980). Accurate spin-dependent electron liquid correlation energies for local spin density calculations: a critical analysis. Can. J. Phys. 58, 1200–1211. doi: 10.1139/p80-159
Wilson, P. J., Bradley, T. J., and Tozer, D. J. (2001). Hybrid exchange-correlation functional determined from thermochemical data and ab initio potentials. J. Chem. Phys. 115, 9233–9242. doi: 10.1063/1.1412605
Zhao, Y., Lynch, B. J., and Truhlar, D. G. (2004). Development and assessment of a new hybrid density functional model for thermochemical kinetics. J. Phys. Chem. A 108, 2715–2719. doi: 10.1021/jp049908s
Zhao, Y., Schultz, N. E., and Truhlar, D. G. (2005). Exchange-correlation functional with broad accuracy for metallic and nonmetallic compounds, kinetics, and noncovalent interactions. J. Chem. Phys. 123:161103. doi: 10.1063/1.2126975
Zhao, Y., Schultz, N. E., and Truhlar, D. G. (2006). Design of density functionals by combining the method of constraint satisfaction with parametrization for thermochemistry, thermochemical kinetics, and noncovalent interactions. J. Chem. Theory Computat. 2, 364–382. doi: 10.1021/ct0502763
Zhao, Y., and Truhlar, D. G. (2004). Hybrid meta density functional theory methods for thermochemistry, thermochemical kinetics, and noncovalent interactions: the MPW1B95 and MPWB1K models and comparative assessments for hydrogen bonding and van der waals interactions. J Phys Chem A. 108, 6908–6918. doi: 10.1021/jp048147q
Zhao, Y., and Truhlar, D. G. (2007). The M06 suite of density functionals for main group thermochemistry, thermochemical kinetics, noncovalent interactions, excited states, and transition elements: two new functionals and systematic testing of four M06-class functionals and 12 other functionals. Theor. Chem. Accoun. 120, 215–241. doi: 10.1007/s00214-007-0310-x
Keywords: redox potencial, DFT, benchmaking, iron, quantum-chemical calculations
Citation: Listyarini RV, Gesto DS, Paiva P, Ramos MJ and Fernandes PA (2019) Benchmark of Density Functionals for the Calculation of the Redox Potential of Fe3+/Fe2+ Within Protein Coordination Shells. Front. Chem. 7:391. doi: 10.3389/fchem.2019.00391
Received: 22 March 2019; Accepted: 15 May 2019;
Published: 05 June 2019.
Edited by:
Vicent Moliner, University of Jaume I, SpainReviewed by:
Nino Russo, University of Calabria, ItalyJon Mujika, Donostia International Physics Center, Spain
Copyright © 2019 Listyarini, Gesto, Paiva, Ramos and Fernandes. This is an open-access article distributed under the terms of the Creative Commons Attribution License (CC BY). The use, distribution or reproduction in other forums is permitted, provided the original author(s) and the copyright owner(s) are credited and that the original publication in this journal is cited, in accordance with accepted academic practice. No use, distribution or reproduction is permitted which does not comply with these terms.
*Correspondence: Pedro Alexandrino Fernandes, cGFmZXJuYW5AZmMudXAucHQ=
†Present Address: Risnita Vicky Listyarini, Chemistry Education Study Program, Sanata Dharma University, Yogyakarta, Indonesia
Diana Sofia Gesto, UCIBIO, Departamento de Química, Faculdade de Ciências e Tecnologia, Universidade Nova de Lisboa, Caparica, Portugal