- 1School of Nuclear Science and Technology, Xi’an Jiaotong University, Xi’an, China
- 2State Key Laboratory of Multiphase Flow, Xi’an Jiaotong University, Xi’an, China
Constituent redistribution is a unique phenomenon to metal fuels that threatens the safety of such fuel forms. Therefore, it is imperative to establish models to understand the intrinsic mechanisms and predict the redistribution kinetics. In this work, we derived the conservative field equations of the phase-field model from near-equilibrium thermodynamic theory. A macroscopic constituent redistribution phase-field model was developed by introducing the effect of irradiation on the atom mobility and the effect of temperature on the interface mobility. An expression of phase boundary width, applicable to both microscopic and macroscopic scenarios was proposed. The interfacial parameters of the model and the Zr concentration distribution near the fuel surface were discussed at last. These works may help understand constituent redistribution characteristics and promote the application of the phase-field method in studying constituent redistribution in macroscopic scenarios.
Introduction
Metal fuels are ideal for fast reactors due to their higher fissile and fertile densities, and they possess high thermal conductivity and inherent safety features as demonstrated by the EBR-II (Experimental Breeder Reactor II) ULOF (Unprotected Loss-Of-Flow) and ULOHS (Unprotected Loss-Of-Heat-Sink) experiments (Feldman et al., 1987; Mohr et al., 1987). However, constituent redistribution in metal fuels is a significant threat to fuels’ safety. The initial uniform radial constituent distribution of metal-fuel transforms to an inhomogeneous counterpart due to the redistribution phenomenon driven by thermodynamics. Inhomogeneous distribution of ingredients and fission products significantly impacts fuels’ mechanical and physical properties (Murphy et al., 1969; Kim et al., 2004; Rahn et al., 2021). Therefore, it is crucial to understand and predict the redistribution kinetics.
Murphy et al. first observed the redistribution phenomenon in irradiated U-Pu-Zr fuel rods (Murphy et al., 1969). Thereafter, constituent redistribution was observed in U-Zr alloys at elevated temperatures (Hofman et al., 1996). Researchers have been establishing thermodynamic models to describe the constituent redistribution process. One of the most popular models was a U-10wt%Zr redistribution model (Hofman et al., 1996) based on a thermal diffusion mechanism. In addition, similar models were also established (Nam and Hwang, 1998; Kim et al., 2006) to analyze the constituent redistribution process. More innovatively, a quantitative constituent redistribution model for U-10wt%Zr alloy using the phase-field method was developed recently (Hirschhorn et al., 2019a; Hirschhorn et al., 2021). Phase-field method is good at dealing with thermodynamic problems and interface behaviour issues (Hu et al., 2009; Hu et al., 2010; Millett and Tonks, 2011a; Millett et al., 2011; Ahmed et al., 2014; Tonks et al., 2014; Liang et al., 2016; Mei et al., 2016). This method has been increasingly used for modelling and simulation in the field of nuclear materials in recent years, including U-Zr and U-Pu-Zr (Millett and Tonks, 2011b; Mohanty et al., 2011; Li et al., 2017; Tonks et al., 2018; Hirschhorn et al., 2019b; Hirschhorn et al., 2020). In all the above-described models, chemical diffusion coefficients, which control the diffusion of atoms and determine the final constituent distribution, were artificially enlarged several times by multiple a number, because the original chemical diffusion coefficients were not enough large to satisfy the simulation results. If key parameters in model cannot be theoretically and accurately determined, the subsequent prediction and design of materials in engineering will have a vital threat.
In this work, we have developed a more accurate phase field model than before. To obtain a more accurate diffusion coefficient, we introduced the irradiation-enhanced diffusion theory into the phase-field model of constituent redistribution and discussed the effects of irradiation on both chemical and thermal diffusion in U-10wt%Zr in this work. It has become an accepted fact that the additional vacancies introduced by irradiation will accelerate the diffusion of atoms, and such acceleration is particularly important at lower temperatures where thermal diffusion becomes limited. We also considered the effect of temperature on the interface mobility, which was overlooked by the previous phase-field model. This will also further improve the accuracy of the phase-field model. Besides, element concentration gradient free energy was added into the total free energy expression to make the free energy expression more comprehensive. In addition to making the model more accurate, the interfacial parameters related to phase boundary in our model were redefined and interpreted because the definition of grain boundary under microscopic conditions is unsuitable for phase boundaries under macroscopic conditions (Faulkner et al., 1996; Moelans et al., 2008). Finally, the interfacial parameters of the model and the Zr concentration distribution near the fuel surface were discussed.
Model development
The phase-field equations
Constituent redistribution is an irreversible process associated with material transport, which is essentially a near-equilibrium thermodynamic phenomenon. During U-Zr constituent redistribution, the Soret phenomenon, also called thermal diffusion, which is driven by the temperature gradient, was observed in the EBR-II X447 irradiation experiment (Hofman et al., 1996). The linear phenomenological law can derive the expression of diffusion flux caused by temperature gradient
where
By substituting Eq. 1 and 2 into Eq. 3, the equation of the evolution of concentration with respect to time can be derived,
That is actually one part of the phase-field model.
In the previous U-Zr component redistribution models, only the pure thermodynamic models were used, and the phase distributions needed to be determined a priori without considering phase evolutions (Hofman et al., 1996; Nam and Hwang, 1998; Kim et al., 2006). Determining the spatial distribution of phases followed by calculating the concentration can cause great inconvenience to deal with phase boundaries. Though phase interfaces are always complicated subjects, they can be effectively dealt with by introducing the phase diffusion equation into the phase-field model. Ever since the beginning of the development of phase-field method, the method has always been proven to have unique advantages in dealing with boundary problems (Millett et al., 2012; Ahmed et al., 2014; Biner, 2017). Another advantage of using phase-field model is that the specific distributions of each phase can be seen intuitively, which is not available in traditional thermodynamic models. Based on these considerations, the Allen-Cahn equation (Allen and Cahn, 1972; Allen and Cahn, 1973) was introduced into the phase-field model to describe the diffusion evolution of phases:
Where,
Where,
The constituent redistribution of U-Zr mainly involves three single phases, γ, a and β. We inherited the idea of Hirschhorn et al. (Hirschhorn et al., 2019a) for the co-evolution of three phases. One order parameter
Where,
Free energy
The selection and discussion of free energy and migration coefficient are manifested below. In the phase-field model, total free energy generally contains volume free energy and gradient free energy (Kim et al., 1999; Chen et al., 2017). The volume free energy is composed of γ phase, a phase, and β phase, and the gradient free energy is composed of the concentration gradient and phase fraction gradient. The expression is as follows:
Where,
Where
It should be noted that it is required that not only the above equations be satisfied near the interface, but also the equilibrium relationship be satisfied at each location point in the system.
Migration coefficient accelerated by radiation
The diffusion coefficient of atoms in a crystal matrix
Where
Where
Where we define
Then the irradiation-enhanced diffusion coefficient can be rewritten as
Thus, the irradiation-enhanced solute diffusion coefficient
where,
and
Where
By introducing the relationship of intrinsic diffusion and irradiation-enhanced diffusion, as shown in Eq. 20, into the phase-field model of constituent redistribution, we can get the diffusion mobility enhanced by irradiation,
Where
Where
The chemical diffusion coefficient in the γ phase (Mohanty et al., 2011) is,
Where,
Where
The thermal migration coefficient in the a-β phase is:
The thermal migration coefficient in the γ phase is:
Where
Simulation methods
The thermodynamic parameters used in our simulation were all inherited from the DP-81 fuel. The DP-81 fuel element was irradiated at the EBR-II reactor by Argonne National Laboratory. The experimental data of the DP-81 pin provided a typical example for verification of this work because there exists not only the temperature profile but also measured data which indicates significant migration of zirconium. The experimental data about Zr concentration we used here were from Figure 7 of Hofman et al.’s article (Hofman et al., 1996). The fuel surface temperature was 900K, while the central temperature was about 988K, and the temperature distribution profile used here was from A. M. Yacout et al. (Yacout et al., 1996). All the simulations were conducted using the explicit finite difference method on the Matlab platform in this work. 1D simulations with 2170 μm long axisymmetric domain discretized into 2,170 elements were used in the current work to represent the DP-81 fuel, as shown in Figure 1. The left side of the 1-D linear segment represents the centerline, and the right side represents the outer surface of the fuel. Zero-flux boundary conditions were applied to all the centerline and the external surface variables. The time step was chosen to be 1s, and a total time length of 1.3 × 105 s was conducted in all simulations.
Simulation results
As described before, the driving forces acting on the Zr migration can be divided into two parts, i.e., heat transport term due to the temperature gradient and chemical potential gradient term which tends to equalize the concentration distribution. The diffusion coefficient controls the amount of Zr relocated, and heat transport and chemical potential gradient control the direction and the trend of the redistribution. To highlight the importance of radiation-accelerated diffusion in constituent redistribution models, we compared the simulation results between redistribution without the effect of irradiation and redistribution with the effect of irradiation, as shown inFigure 2, 3. Figure 2 shows the simulation results without considering the accelerated diffusion of irradiation. It can be seen that although Zr still tends to move to the high-temperature region due to the existence of the temperature gradient, there is almost no constituent redistribution. While the simulation results of Figure 3 are much in good agreement with the experimental data.
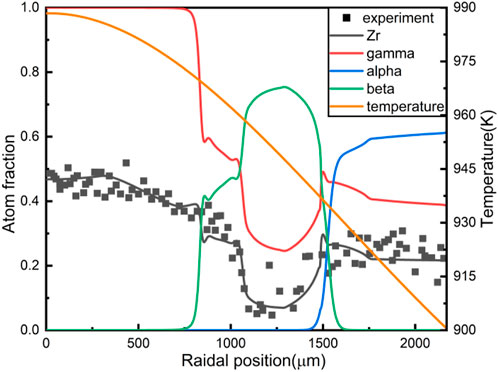
FIGURE 3. The results of U-10wt%Zr constituent redistribution with introducing the radiation-enhanced diffusion.
A noticeable feature of the Zr redistribution in Figure 3 is that a “Zr well” formed in the middle radial region of the fuel slug. Zr atoms migrate towards the fuel centre at high temperatures, causing the Zr concentration in the fuel centre to increase and U concentration to decline correspondingly. Consequently, the concentration of Zr in the middle radial region is relatively low. Phase distribution is also in line with the experimental results. The red line is the γ phase fraction, and the γ phase is mainly located in the central region of the fuel, coexisting with the β phase in the middle radial region, and coexisting with the a phase near the outer fuel surface.
The comparison of the Zr concentration redistribution simulated by different models, including the model in this work, are shown in Figure 4. It is found that the introduction of the radiation-enhanced diffusion coefficient into the existing phase-field model seems to be able to replace reasonably the artificial increase of the diffusion coefficient. And by improving the rationality of some parameters of the phase-field model, such as the influence of temperature on the interface mobility, the accuracy of the simulation is significantly improved.
Discussion
Discussion of interfacial parameters
The interface energy and the interface width occupy extraordinary significance in the phase-field model. The model parameters are often phenomenological and don’t possess the essence of natural physical properties (Moelans et al., 2008). Therefore, it is necessary to analyze the parameters and their effects on the simulation results. In this section, we first discussed the interface energy and then discussed the interface width by defining the macro phase boundary width.
For the KKS model, the chemical energy is decoupled from the interfacial energy, and the interfacial energy is only provided by the potential well term and the gradient term (Aagesen et al., 2018). It should be noted that the interface energy is not a specific phase boundary energy but rather a homogenized parameter describing all the phase boundaries in the two-phase region. The interface energy of phase boundaries in the two-phase areas in the discrete system model can thus be written as:
Where
It is well known that the interfacial width is chosen mainly based on computational consideration in interface growth models. The phase boundary, which refers to the boundary between different phases, will be discussed in the following, referencing the definition of the grain boundary. Strictly speaking, there are many possibilities to define a measure for the boundary once the diffusion surface thickness reaches infinity. For example, Moelans et al. (Faulkner et al., 1996; Moelans et al., 2008) described the grain boundary based on the absolute value of the gradient.
Where
Since the profiles are steepest at the middle of the diffuse boundary region, a grain boundary width defined in this way can be used as numerical criteria for stability and accuracy. However, the description of the boundary in the microscopic situation does not apply to the situation of the macroscopic edge. Here, we proposed a new definition of boundary width based on Moelans et al., as shown in Eq. 35.
This equation is an extension of Eq. 34. In phase-field method, it can be used to express the phase boundaries and make the boundaries describable and discussible in macroscopic scenarios. For example, in a microscopic system with grain boundaries, two crystal grains can be distinguished by
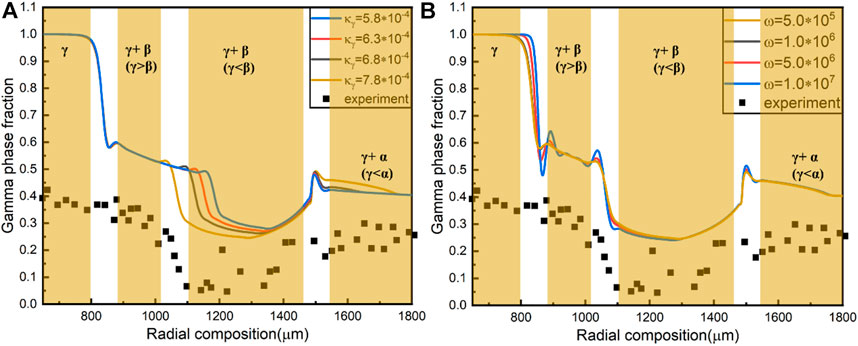
FIGURE 5. (A) γ Phase fraction as a function of
To get the values of interface energy
The interfacial energy
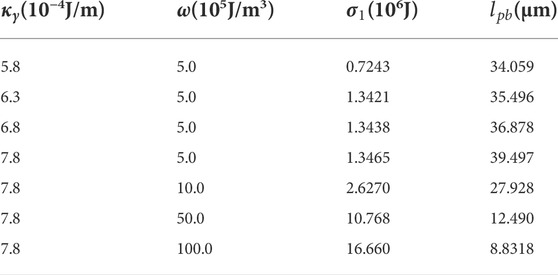
TABLE 2. Different
Discussion of the results near the fuel surface
Obviously, there is a distinct difference between the simulation results and the experimental data near the fuel surface among all constituent redistribution models, shown as Figure 4. Some Zr atoms migrate to the region between 1700 and 2000μm, making the Zr concentration higher than the initial concentration (0.225). Under normal circumstances, there is hardly Zr migration due to the small atom diffusion coefficient in the a-U phase. The specific reason is unknown, but it shall not be interpreted to be caused by temperature because the heat of transport parameter
Conclusion
Combined with traditional thermodynamics, the phase-field model coupling the radiation-enhanced diffusion model was constructed in this work. Firstly, the results of Zr redistribution simulated by the phase-field model were given and compared with the results of the model without the effect of radiation, which demonstrated the importance and necessity of introducing the radiation-enhanced diffusion model. Secondly, the influence of temperature on the kinetic coefficients was also imported to improve the rigour of the model. The computational results of the improved model appear to be in better agreement with the experimental data, compared to the previous models. Thirdly, an expression of boundary width applied to the macroscopic scenario was proposed to analyse the phase distribution. Through the analysis of phase interfacial parameters, the magnitude of the potential barrier played an essential role in forming a sharp interface and the energy coefficients had few effects on the redistribution results. This model may further help extend the application of phase-field in the constituent redistribution.
Data availability statement
The original contributions presented in the study are included in the article/supplementary material, further inquiries can be directed to the corresponding authors.
Author contributions
CW: The realization of the phase-field model; Manuscript writing WL: Study conception and design; Model modification idea DY: Study conception and design; The manuscript revised ZQ: Calculation of rate theory.
Funding
This work was supported by the NSAF Joint Fund (No. U2130105), the National Natural Science Foundation of China (No. 11675126) and the Innovative Scientific Program of CNNC.
Conflict of interest
The authors declare that the research was conducted in the absence of any commercial or financial relationships that could be construed as a potential conflict of interest.
Publisher’s note
All claims expressed in this article are solely those of the authors and do not necessarily represent those of their affiliated organizations, or those of the publisher, the editors and the reviewers. Any product that may be evaluated in this article, or claim that may be made by its manufacturer, is not guaranteed or endorsed by the publisher.
References
Aagesen, L. K., Gao, Y., Schwen, D., and Ahmed, K. (2018). Grand-potential-based phase-field model for multiple phases, grains, and chemical components. Phys. Rev. E 98 (2), 023309. doi:10.1103/physreve.98.023309 | |
Ahmed, K., Pakarinen, J., Allen, T., and El-Azab, A. (2014). Phase field simulation of grain growth in porous uranium dioxide. J. Nucl. Mater. 446 (1-3), 90–99. doi:10.1016/j.jnucmat.2013.11.036 |
Allen, S. M., and Cahn, J. W. (1973). A correction to the ground state of FCC binary ordered alloys with first and second neighbor pairwise interactions. Scr. Metall. 7 (12), 1261–1264. doi:10.1016/0036-9748(73)90073-2 |
Allen, S. M., and Cahn, J. W. (1972). Ground state structures in ordered binary alloys with second neighbor interactions. Acta Metall. 20 (3), 423–433. doi:10.1016/0001-6160(72)90037-5 |
Aziz, M. J. (1996). Interface attachment kinetics in alloy solidification. Metallurgical Mater. Trans. A 27 (3), 671–686. doi:10.1007/bf02648954 |
Aziz, M. J., and Kaplan, T. (1988). Continuous growth model for interface motion during alloy solidification. Acta Metall. 36 (8), 2335–2347. doi:10.1016/0001-6160(88)90333-1 |
Aziz, M. J. (1982). Model for solute redistribution during rapid solidification. J. Appl. Phys. 53 (2), 1158–1168. doi:10.1063/1.329867 |
Biner, S. B. (2017). An overview of the phase-field method and its formalisms. Cham, Switzerland: Springer International Publishing, 1–7. Programming Phase-Field Modeling. |
Chen, W., Peng, Y., Li, X. A., Chen, K., Ma, J., Wei, L., et al. (2017). Phase-field study on geometry-dependent migration behavior of voids under temperature gradient in UO2 crystal matrix. J. Appl. Phys. 122 (15), 154102. doi:10.1063/1.4996692 |
Dinsdale, A. (1991). SGTE data for pure elements. Calphad 15 (4), 317–425. doi:10.1016/0364-5916(91)90030-n |
Faulkner, R. G., Song, S., and Flewitt, P. E. J. (1996). A model describing neutron irradiation-induced segregation to grain boundaries in dilute alloys. Metallurgical Mater. Trans. A 27 (11), 3381–3390. doi:10.1007/bf02595431 |
Faulkner, R. G., Song, S., Flewitt, P. E. J., and Fisher, S. B. (1997). Irradiation-enhanced solute diffusion in alloys. J. Mat. Sci. Lett. 16 (14), 1191–1194. doi:10.1007/bf02765407 |
Feldman, E. E., Mohr, D., Chang, L. K., Planchon, H., Dean, E., and Betten, P. (1987). EBR-II unprotected loss-of-heat-sink predictions and preliminary test results. Nucl. Eng. Des. 101 (1), 57–66. doi:10.1016/0029-5493(87)90150-6 |
Galloway, J., Unal, C., Carlson, N., Porter, D., and Hayes, S. (2015). Modeling constituent redistribution in U-Pu-Zr metallic fuel using the advanced fuel performance code BISON. Nucl. Eng. Des. 286, 1–17. doi:10.1016/j.nucengdes.2015.01.014 |
Hirschhorn, J., Aitkaliyeva, A., Adkins, C., and Tonks, M. (2019). The microstructure and thermodynamic behavior of as-cast U-24Pu-15Zr: Unexpected results and recommendations for U-Pu-Zr fuel research methodology. J. Nucl. Mater. 518, 80–94. doi:10.1016/j.jnucmat.2019.02.039 |
Hirschhorn, J., Tonks, M., Aitkaliyeva, A., and Adkins, C. (2020). Reexamination of a U-Zr diffusion couple experiment using quantitative phase-field modeling and sensitivity analysis. J. Nucl. Mater. 529, 151929. doi:10.1016/j.jnucmat.2019.151929 |
Hirschhorn, J., Tonks, M., and Matthews, C. (2021). A CALPHAD-informed approach to modeling constituent redistribution in Zr-based metallic fuels using BISON. J. Nucl. Mater. 544, 152657. doi:10.1016/j.jnucmat.2020.152657 |
Hirschhorn, J., Tonks, M. R., Aitkaliyeva, A., and Adkins, C. (2019). A study of constituent redistribution in U-Zr fuels using quantitative phase-field modeling and sensitivity analysis. J. Nucl. Mater. 523, 143–156. doi:10.1016/j.jnucmat.2019.05.053 |
Hofman, G. L., Hayes, S. L., and Petri, M. C. (1996). Temperature gradient driven constituent redistribution in U- Zr alloys. J. Nucl. Mater. 227 (3), 277–286. doi:10.1016/0022-3115(95)00129-8 |
Hu, S., Henager, C. H., Heinisch, H. L., Stan, M., Baskes, M. I., and Valone, S. M. (2009). Phase-field modeling of gas bubbles and thermal conductivity evolution in nuclear fuels. J. Nucl. Mater. 392, 292–300. doi:10.1016/j.jnucmat.2009.03.017 |
Hu, S., Li, Y., Sun, X., Gao, F., Devanathan, R., Henager, C. H., et al. (2010). Application of the phase-field method in predicting gas bubble microstructure evolution in nuclear fuels. Int. J. Mater. Res. 101, 515–522. doi:10.3139/146.110304 |
Kim, S. G., Kim, W. T., and Suzuki, T. (1999). Phase-field model for binary alloys. Phys. Rev. E 60 (6), 7186–7197. doi:10.1103/physreve.60.7186 | |
Kim, Y. S., Hayes, S. L., Hofman, G. L., and Yacout, A. (2006). Modeling of constituent redistribution in U-Pu-Zr metallic fuel. J. Nucl. Mater. 359 (1-2), 17–28. doi:10.1016/j.jnucmat.2006.07.013 |
Kim, Y. S., Hofman, G. L., Hayes, S. L., and Sohn, Y. (2004). Constituent redistribution in U-Pu-Zr fuel during irradiation. J. Nucl. Mater. 327 (1), 27–36. doi:10.1016/j.jnucmat.2004.01.012 |
Kreuzer, H. J. (1981). Nonequilibrium thermodynamics and its statistical foundations. London, United Kingdom: Clarendon Press, 438.
Li, Y., Hu, S., Sun, X., and Stan, M. (2017). A review: Applications of the phase field method in predicting microstructure and property evolution of irradiated nuclear materials. NPJ Comput. Mat. 3 (1), 16–17. doi:10.1038/s41524-017-0018-y |
Liang, L., Mei, Z. G., Kim, Y. S., Ye, B., Hofman, G., Anitescu, M., et al. (2016). Mesoscale model for fission-induced recrystallization in U-7Mo alloy. Comput. Mater. Sci. 124, 228–237. doi:10.1016/j.commatsci.2016.07.033 |
Mei, Z. G., Liang, L., Kim, Y. S., Wiencek, T., O'Hare, E., Yacout, A. M., et al. (2016). Grain growth in U-7Mo alloy: A combined first-principles and phase field study. J. Nucl. Mater. 473, 300–308. doi:10.1016/j.jnucmat.2016.01.027 |
Millett, P. C., El-Azab, A., and Wolf, D. (2011). Phase-field simulation of irradiated metals Part II: Gas bubble kinetics. Comput. Mater. Sci. 50, 960–970. doi:10.1016/j.commatsci.2010.10.032 |
Millett, P. C., and Tonks, M. (2011). Application of phase-field modeling to irradiation effects in materials. Curr. Opin. Solid State Mater. Sci. 15 (3), 125–133. doi:10.1016/j.cossms.2010.10.002 |
Millett, P. C., and Tonks, M. (2011). Phase-field simulations of gas density within bubbles in metals under irradiation. Comput. Mater. Sci. 50, 2044–2050. doi:10.1016/j.commatsci.2011.02.006 |
Millett, P. C., Tonks, M. R., Biner, S. B., Zhang, L., Chockalingam, K., and Zhang, Y. (2012). Phase-field simulation of intergranular bubble growth and percolation in bicrystals. J. Nucl. Mater. 425 (1-3), 130–135. doi:10.1016/j.jnucmat.2011.07.034 |
Moelans, N., Blanpain, B., and Wollants, P. (2008). Quantitative analysis of grain boundary properties in a generalized phase field model for grain growth in anisotropic systems. Phys. Rev. B 78 (2), 024113. doi:10.1103/physrevb.78.024113 |
Mohanty, R. R., Bush, J., Okuniewski, M. A., and Sohn, Y. (2011). Thermotransport in γ (bcc) U-Zr alloys: A phase-field model study. J. Nucl. Mater. 414 (2), 211–216. doi:10.1016/j.jnucmat.2011.03.028 |
Mohanty, R. R., Guyer, J. E., and Sohn, Y. H. (2009). Diffusion under temperature gradient: A phase-field model study. J. Appl. Phys. 106 (3), 034912. doi:10.1063/1.3190607 |
Mohr, D., Chang, L. K., Feldman, E. E., Betten, P., and Planchon, H. (1987). Loss-of-primary-flow-without-scram tests: Pretest predictions and preliminary results. Nucl. Eng. Des. 101 (1), 45–56. doi:10.1016/0029-5493(87)90149-x |
Murphy, W. F., Beck, W. N., Brown, F. L., Koprowski, B. J., and Neimark, L. A. (1969). Postirradiation examination of U-Pu-Zr fuel elements irradiated in EBR-II to 4.5 atomic percent Burnup. Illinois, United States: Argonne National Laboratory.
Nam, C., and Hwang, W. (1998). A calculation model for fuel constituent redistribution and temperature distribution on metallic U-10Zr fuel slug of liquid metal reactors. Nucl. Eng. Technol. 30 (6), 507–517.
Olander, D. R. (1976). Fundamental aspects of nuclear reactor fuel elements. TID-26711-P1. Berkeley (USA): California University.
Quaini, A., Guéneau, C., Gossé, S., Dupin, N., Sundman, B., Brackx, E., et al. (2018). Contribution to the thermodynamic description of the corium-the U-Zr-O system. J. Nucl. Mater. 501, 104–131. doi:10.1016/j.jnucmat.2018.01.023 |
Rahn, T., Capriotti, L., Di Lemma, F., Trowbridge, T. L., Harp, J. M., and Aitkaliyeva, A. (2021). Investigation of constituent redistribution in U-Pu-Zr fuels and its dependence on varying Zr content. J. Nucl. Mater. 557, 153301. doi:10.1016/j.jnucmat.2021.153301 |
Rest, J. (1993). Kinetics of fission-gas-bubble-nucleated void swelling of the alpha-uranium phase of irradiated U-Zr and U-Pu-Zr fuel. J. Nucl. Mater. 207, 192–204. doi:10.1016/0022-3115(93)90261-v |
Shewmon, P. (1989). Diffusion in solids. The minerals, Metals & Materials Series (Cham, Switzerland: Springer), 53–130.
Tonks, M. R., Cheniour, A., and Aagesen, L. (2018). How to apply the phase field method to model radiation damage. Comput. Mater. Sci. 147, 353–362. doi:10.1016/j.commatsci.2018.02.007 |
Tonks, M. R., Zhang, Y., Bai, X., and Millett, P. C. (2014). Demonstrating the temperature gradient impact on grain growth in UO2 using the phase field method. Mater. Res. Lett. 2, 23–28. doi:10.1080/21663831.2013.849300 |
Vizoso, D., and Deo, C. (2021). Determination of vacancy formation energies in binary UZr alloys using special quasirandom structure methods. Front. Mat. 8, 243. doi:10.3389/fmats.2021.692660 |
Wang, M., Zong, B., and Wang, G. (2009). Grain growth in AZ31 Mg alloy during recrystallization at different temperatures by phase field simulation. Comput. Mater. Sci. 45, 217–222. doi:10.1016/j.commatsci.2008.09.010 |
Wen, Y. H., Wang, B., Simmons, J. P., and Wang, Y. (2006). A phase-field model for heat treatment applications in ni-based alloys. Acta Mater. 54, 2087–2099. doi:10.1016/j.actamat.2006.01.001 |
Keywords: U-Zr, constituent redistribution, phase-field method, irradiation-induced diffusion, interface
Citation: Wen C, Liu W, Yun D and Qian Z (2022) A phase-field model with irradiation-enhanced diffusion for constituent redistribution in U-10wt%Zr metallic fuels. Front. Mater. 9:950785. doi: 10.3389/fmats.2022.950785
Received: 23 May 2022; Accepted: 22 August 2022;
Published: 15 September 2022.
Edited by:
Yu-Hong Zhao, North University of China, ChinaReviewed by:
Hock Jin Quah, University of Science Malaysia, MalaysiaHoubing Huang, Beijing Institute of Technology, China
Copyright © 2022 Wen, Liu, Yun and Qian. This is an open-access article distributed under the terms of the Creative Commons Attribution License (CC BY). The use, distribution or reproduction in other forums is permitted, provided the original author(s) and the copyright owner(s) are credited and that the original publication in this journal is cited, in accordance with accepted academic practice. No use, distribution or reproduction is permitted which does not comply with these terms.
*Correspondence: Wenbo Liu, bGl1d2VuYm9AeGp0dS5lZHUuY24=; Di Yun, ZGl5dW4xOTc5QHhqdHUuZWR1LmNuJiN4MDIwMGE7