- 1Potsdam Institute for Climate Impact Research (PIK) – Member of the Leibniz Association, Potsdam, Germany
- 2Department of Physics, Humboldt University, Berlin, Germany
- 3WHU - Otto Beisheim School of Management, Vallendar, Germany
- 4Department of Water, Environment, Construction and Safety, Magdeburg–Stendal University of Applied Sciences, Magdeburg, Germany
Over the last decades, bilateral trade agreements (BTAs) have increased considerably in number and economic relevance. Notably, such agreements substantially affect global trade, since the reorganization of flows of goods and services has prominent impacts on the contracting countries' economies, but also on other parties that are (directly or indirectly) engaged in trade with these countries. Here, we empirically study the effect of BTAs on the input-output linkages between the contractual parties' national economic sectors by defining a new measure of Trade Interconnectedness (TI), which describes the relative importance of direct and indirect production linkages between the two countries in the international trade network. By analyzing its time evolution for each pair of trade agreement partners, we demonstrate that while most BTAs are succeeded by an increase in TI between the contractors, there are some notable exceptions. In particular, comparing the trade profiles of China and the United States (US), we find indications that both countries have been pursuing fundamentally different objectives and strategies related to the negotiation of BTAs.
Introduction
The present US government's announcement to revise the country's trade policy and negotiate "new and better deals" through BTAs [1] has reignited the debate on the effects of such agreements and the underlying interests and strategies. Especially in the last years, BTAs have become an increasingly important and frequently used policy instrument to establish and intensify close trade relationships. In these agreements, countries grant each other trade privileges in terms of concessions on trade barriers, which include reductions of tariffs and quotas as well as easing of market access and of competition provisions. Theory suggests that the dismantling of trade barriers increases trade between the involved economies, which stimulates economic growth in the contracting countries [2]. Previous empirical studies - mainly employing the so-called gravity models - largely confirm a positive effect of BTAs on trade [3–6]. Yet, they also report that this might come at the cost of shifting production away from more efficient suppliers in other countries [3, 6, 7]. Thus, BTAs can enhance some trading relationships and at the same time weaken others that are not directly covered by the agreement. Accordingly, BTAs can change the structure of the international trade network formed by input-output linkages between national economic sectors. Effectiveness of BTAs in enhancing trade among the contracting partners has been shown to depend on the affected countries' specific characteristics. In this context, geographic proximity, common language and/or cultural background, or a similar GDP have been suggested to be beneficial in increasing trade gains [8–11].
Importantly, the effectiveness of BTAs is also determined by the scope and extent of commitments agreed upon [12, 13]. BTAs are very diverse in content and design, reflecting that they might have been negotiated to serve other, strategic (and possibly non-economic) purposes as well [13, 14], e.g., underpinning politically motivated partnerships between countries, increasing bargaining power in trade negotiations with third countries or fostering liberal economic policy reforms at the domestic level [15]. Identifying and assessing the relative importance of the various possible objectives that might drive the negotiations proves difficult and is often a matter of interpretation of the final agreement [14]. In this context, two of the most active players in global trade have been suggested to pursue markedly different interests in establishing BTAs: While past US policy probably had a particular focus on strengthening strategic alliances and rewarding their agreement partners for domestic economic reforms [16], China is often assumed to be particularly interested in gaining economic and, indirectly, political influence in the Asia-Pacific region by tying close economic dependencies [17].
In this study, we investigate the effectiveness of BTAs by assessing their impact on the trade flows between the economic sectors of each pair of contracting countries. To this end, we perceive all trade relationships as an international trade network (ITN), in which the sectors (nodes) are linked by their trade volumes. Network theory applied to trade economics has gained traction in recent years as it allows incorporating topological properties into the analysis [18, 19]. Noteworthy examples include studies on the formation and structure of economic dependencies [20], the resilience of the trade system to an outage of an industry or production facility [21, 22], and the growth relevant dissemination of knowledge and technology [23]. In contrast to gravity models often used for related analyses [3, 5, 6] we can thus take higher orders of mutual economic interdependences into account. In the context of the present work, such higher-order dependencies reflect that BTAs might also affect the demand and supply of sectors indirectly linked with the exporting and importing sectors. The existence of these indirect effects have recently been disclosed by [24], who demonstrates that countries that are more connected to trade agreement blocs benefit by exporting more than those that are more isolated. Taking into account all direct and indirect input-output linkages within and across two respective countries, we introduce a quantitative framework for measuring the trade interconnectedness (TI) between two countries in the ITN. Accounting for all direct and indirect dependencies thus improves on the recently suggested Supply Propagation Connectivity (SPC) measure of Wenz and Levermann [21] which is limited to measure direct dependencies only. Furthermore, we assess the impact of BTAs by evaluating the time evolution of the TI, considering both the trend and changes in the magnitude of the TI after the implementation date of a trade agreement. These methods, along with the description of the utilized data for this study are presented in section 2 of this paper. We analyze the effect of BTAs in general by drawing upon the 107 agreements that took effect between 1995 and 2008 in section 3. Specifically, we compare the results for the BTAs formed by the US and China and thereby provide quantitative empirical evidence for the suggested strategic differences in negotiating BTAs. A sensitivity study of our results with further detailed discussions on the effect of certain parameters of our analysis is presented in section 4, before this paper concludes with a discussion in section 5.
Materials and Methods
Data and Network Construction
The present analysis builds upon the EORA multi-regional input-output (MRIO) database [25, 26], which provides multi-regional input-output tables that depict both national and international intermediate trade flows between 26 industrial sectors of 189 countries. Furthermore, the monetary values of goods that flow into each country's final demand are included. Notably, among the existing MRIO databases, EORA has the broadest (near-global) coverage of national economies and industrial sectors, while other similar data sets may exhibit greater level of detail but cover much fewer countries, rendering them less appropriate for the purpose of the present study. Specifically, as compared with national input-output tables, MRIO tables generally have a rather coarse sectoral detail level but cover many countries, which is essential for studying the impacts of BTAs on the interconnectedness of global trade.
In EORA, input-output tables are available on a yearly resolution. One trade flow in an input-output matrix depicts the sum of the monetary values of all goods and services that have been exchanged between two industrial sectors as intermediate or as final demand in the respective year. The monetary values are provided in nominal US $. The EORA database has been compiled by combining various data sources, including data of national trade statistics, Eurostat, the OECD and the UN Comtrade database (see [26] for details). Our analysis covers the years between 1990 and 2013 as this data was available at the time of performing the analyses presented in this manuscript.
Here, we interpret the input-output tables from the EORA data set as a weighted and directed complex network, which is identified with a time-dependent representation of the ITN for each year [19, 27]. In this network, each node represents an industrial sector of one country that is connected via trade links with a weight proportional to the exchanged trade volume. Moreover, the final demand of each country is included as an absorbing node.
The Regional Trade Agreement Information System [28] of the World Trade Organization (WTO) provides details on negotiated regional trade agreements. It contains information on all agreements that have been either notified to the WTO or for which an early announcement has been made from 1948 to today. If a trade agreement is negotiated between exactly two parties, it is referred to as a BTA. Otherwise, a trade agreement with more parties involved is called a multilateral trade agreement. We also speak of a BTA if one contracting party (or both parties) consists of a regional trade bloc itself, e.g., if the European Union negotiates an agreement with Mexico. There exist several types of trade agreements: In a custom union the involved partners agree to pursue only common trade policies with external countries that do not belong to the union. In contrast, free trade agreements allow each partner to pursue their individual trade conditions with any third country. BTAs are often negotiated in the form of free trade agreements, as custom unions include in general more than two partners. The BTAs of the EU with Turkey and Andorra constitue the only exceptions from this among the set of BTAs studied in this work.
For this study, we analyze the impacts of all 107 BTAs with a date of entry into force between 1995 and 2008. To analyze the TI between the two partners in such cases, we first aggregate all trade flows within the respective trade bloc while maintaining the homogeneous sectoral structure. Then, the TI is calculated as described below, while the corresponding set of nodes consists of the 26 industry sectors of the entire trade bloc plus its final demand sector. We attribute the obtained TI to all countries contained in the regional trade bloc.
A complete list of the analyzed trade agreements is provided in Table 1 (Appendix).
Trade Interconnectedness (TI)
In most traditional network representations of the ITN, the trade volume is represented by weighted and directed links that connect two industrial sectors or, at a coarser resolution, two countries [29, 30]. Here, we assess the interconnectedness between two national economies with a newly developed framework that is based upon the interpretation of the ITN as a flow network [31]. Generally, flow networks encode the probabilities of a random walker to move from one node to another. Thus, the ITN as a flow network represents the probabilities that a unit good follows certain paths through different industrial sectors down the supply chain. This probabilistic approach becomes necessary as individual supply chains cannot be traced from existing data.
In this work, we utilize the idea of flow networks to define the trade interconnectedness (TI) between two countries based upon the input and output dependency measures originally used in [27],
Here, wij describes the aggregated monetary value (in nominal US $) of all goods that have been sold in 1 year from sector i to another sector j. The values of () can be interpreted as the empirical probability of a unit good (respectively, of a certain monetary unit) to follow the corresponding edge in the ITN from i to j as a random walker.
With the matrices , the probability that a unit good follows a path of length α from sector i to sector j is given by . Analogously, measures the flow of the associated monetary units. To measure how likely it is for a random walker on the ITN to start from a sector in one country and eventually end in another country, we define the trade interconnectedness (TI) between two countries and as
with denoting the subset of all sectors i that belong to one country c. We refer to as the output TI of to , which can be interpreted as the relative importance of in the role of a consumer for . The relative importance of in the role of a supplier for is analogously quantified by the input TI of to , . In Equation (2), αmax describes the maximal path length (in terms of national economic sectors) of the random walker that is to be considered. We find that a reasonable choice of αmax is twice the average path length between the two subgraphs of the ITN spanned by the national economies of and . A more detailed discussion of this choice and a sensitivity analysis of the results with respect to different values of αmax will be presented in section 4.
As formalized in Equation (2), the dependency measures allow for the definition of the output TI which describes the probability of a unit good that is supplied from to end in . The input TI describes this probability for a flow of successive payments. In Figure 1, we schematically illustrate the paths that contribute to the TI using an exemplary network of trade between China (CHN) and Vietnam (VNM). The output TI of China to Vietnam, TIout(CHN, VNM), accounts for the paths of goods that originate in China and end in Vietnam (see Figure 1A). On the other hand, the input TI of China to Vietnam, TIin(VNM, CHN), takes the paths of the monetary flows into account (see Figure 1B]. Here, the paths are defined in the opposite direction, as the payment flows opposite to the supply of materials, goods or services in the trade network. The definition of the TI is not symmetric: the corresponding paths of this exemplary network that contribute to the TIs of Vietnam to China are illustrated in Figure 1C for TIout(VNM, CHN) and Figure 1D for TIin(CHN, VNM), respectively. Notice that we do not consider paths that traverse a third country in the definition of TI•.
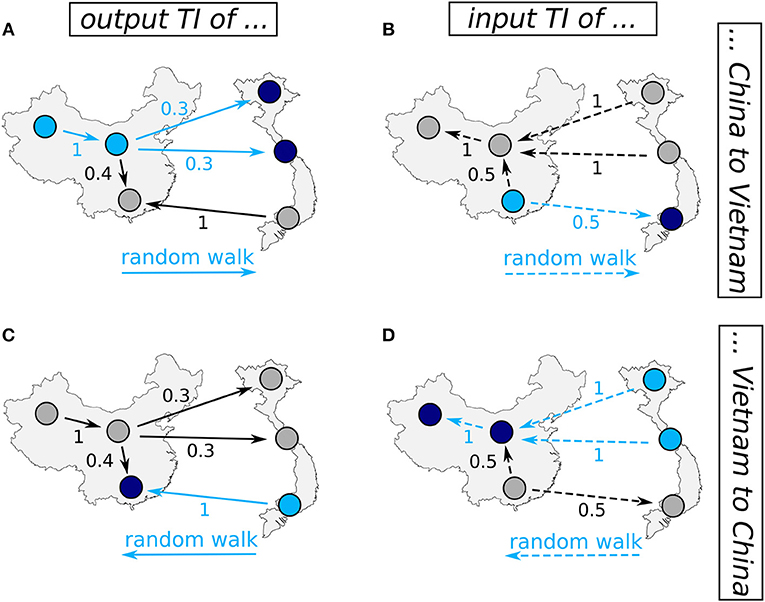
Figure 1. Hypothetical excerpt of the ITN schematically illustrating the contributions to the different directions of TI. Colored circles indicate different industrial sectors, while solid (dashed) arrows indicate the flow of goods (payments). In (A) the paths of goods that contribute to the output TI of China to Vietnam, TIout(CHN, VNM), are highlighted in blue. Here, the unit good starts in China (light blue nodes) and ends in Vietnam (dark blue nodes). The individual path probabilities that are used to compute TIout are the output dependency values which are illustrated by exemplary values at the links. In this example, paths of length one and two exist between the two countries (blue arrows). Supply directions that are not relevant for the supply of China to Vietnam are depicted by gray nodes and arrows. In (B), the paths of payments that contribute to the input TI of China to Vietnam are marked blue, with the payment flow following the opposite direction as compared to the flow of goods in (A). The individual path probabilities used to compute the input TI are the input dependency values . The corresponding paths for the respective TIs of Vietnam to China are depicted in (C,D).
BTA Impact Index
To quantify the impact of a BTA on the TI between the involved countries, we define the BTA impact index Π• that takes both the level and local trend properties of the time series of TI• into account. Thus, the investigation of the BTA impact index allows for a comparison between the impacts of individual BTAs. In contrast, more traditional methods such as as a difference-in-differences approach would only assess the impact of BTAs compared to country pairs without agreement.
Firstly, we investigate if the mean level of TI has changed markedly after the date of entry into force tf of a specific trade agreement. For this purpose, we consider the annual TI values during a 5-year interval before the agreement's implementation and a 5-year interval after the implementation and define a corresponding score as
Here, μ(·) and σ(·) represent the mean value and standard deviation of annual TI values within the respective periods. The score z relates the TI values after tf with the previous levels of the variable. Since the TI• most commonly do not follow a Gaussian distribution, we will utilize a coarse classification of the explicit values of z defined by Equation (3) in the definition of Π•, as it will be described below, instead of considering the precise value of z. In general, a more sophisticated approach to assess potential changes in the level of a random variable would include an analysis of variance (ANOVA), most likely via the Mann-Whitney U test. However, the small sample size of TI• prevents a meaningful interpretation of the test results in this case, which is why we refrain from performing such explicit statistical significance testing at this point.
Secondly, we are interested in the evolution of the annual TI values after the date of entry into force of an agreement. and therefore statistically characterize their trend during the interval (including the year of BTA implementation and the five following years). To assess this trend, we consider two possible models: In the first model, we perform a simple linear regression
with the parameters βi (i = 0, 1) and an independent and identically distributed Gaussian error ϵl(t). Alternatively, in order to better recognize oscillating or saturating behavior of the time series during the considered 6-year period, we additionally perform a two-segment piecewise linear regression [32]. The form of this segmented linear model is
with the Heaviside function θ, the (unknown) break-point ψ, trend parameters γi (i = 1, 2) and a Gaussian error term ϵs(t) as in the linear model. In contrast to the linear regression, the model in Equation (5) can also account for one local extreme value during the investigated time period, which would be represented by a change in the signs of the slopes between the two segments. More complex regression models that exhibit multiple break-points cannot be reliably applied due to the coarse (annual) resolution of the considered data. Therefore, we do not consider such more general models, emphasizing that we are only interested in the sign and statistical relevance of short-term (multi-annual) trends after BTA implementation rather than exact functional descriptions of the shape of these trends or explicit quantitative estimates thereof. Since the segmented model contains two additional parameters as compared to the linear regression model, we perform a model selection based upon the Akaike Information Criterion (AIC) [33] to avoid overfitting by the statistical model with a higher number of degrees of freedom.
If the simple linear model is selected by the AIC criterion, we assess the relevance of the trend identified by the linear regression model and categorize it as relevant and positive (+), relative and negative (−) or not relevant (o). Here, we consider the trend of the linear regression model as relevant, if the estimated variance of the error in yl is smaller than the difference Δŷl: = |ŷl(tf)−ŷl(tf+5)| of the values at the margins of the regression period. If , we do not consider the estimated slope of the linear model to be relevant and categorize the trend as (o).
In the case of the segmented model, the considered time series is too short for a similar relevance assessment. Accordingly, if that model is preferred, the additional breakpoint improves the AIC score as compared to the linear regression model. We then consider the slopes of the two segments as relevant. Thus, any pairwise combination between (+) and (−) is possible for the segmented model. Combining both the trend properties and score parameter z of the time series of TI values, we finally define the impact index of a BTA as follows:
As for the TI, we refer to as the output BTA impact index of to ( as the input BTA impact index of to ). The average BTA impact indices Πout and Πin of a country are defined as the average and for the export and import linkages, respectively, taken over all countries that have negotiated a BTA with .
Results
Other than common characteristics like the total trade volume or the absolute values of imports or exports studied in previous works [4, 5], the TI also captures indirect trade effects. Such indirect effects arise, for instance, if a customer increases its output, being likely to demand more input that is required for the production of its goods. This increase in demand, in turn, affects the business of the supplying industry at the input side. Capturing effects at both, demand and supply side individually, TI can be defined in each direction and thus allows distinguishing between the input TI and output TI of one country to another country as trade partner. The input TI (output TI) thereby quantifies the relative importance of one country as a supplier (consumer) for the production of another country . Note that the countries' relative economic relevance for each other is not symmetric. Furthermore, the TI is a relative measure in the sense that it is based on the fraction of a country's total trade activities that is accounted for by a specific partner. For instance, in a global setting in which all countries increase their international exports, the TI between two countries decreases if the growth in bilateral trade volume is smaller than the global average growth (given that the nation's sectoral structure remains the same). Here, we estimate TI for the ITN for each year between 1990 and 2013.
As explained above, theory suggests that BTAs foster trade activities among the partners, which should result in a stronger TI between the involved countries. To assess empirically if this is indeed the case, we analyze the BTA impact indices Π• as defined in Equation (6) for each BTA. A positive value of Π• implies both an increase in the level and a positive trend of the TI and thus reflects that business between the involved countries has gained in relative importance during the first years after BTA implementation. Accordingly, we consider a BTA to be effective if its BTA impact indices are positive.
The resulting probability distributions of BTA impact indices for all partners with a BTA are depicted in dark colors in Figure 2. Note that the underlying distributions of Πout and Πin are based on 214 entries each, since in general, . To put these results into context, we further assess the relevance of the empirically identified impacts of the BTAs by comparing the estimated BTA impact indices with the corresponding values for those pairs of countries that have not entered a trade agreement until 2014. For the latter purpose, we calculate the BTA index for the 15,199 country pairs that have not signed such an agreement within the study period and assume an arbitrary reference year of 2002. As shown in Figure 2, the existence of a BTA commonly coincides with an elevated probability of a positive BTA index. Note that this simple analysis does not allow directly drawing a causal link of the BTA implementation resulting in stronger entangled economic ties, since it would also be compatible with the explanation that countries with generally more positive economic development have a higher tendency toward negotiating trade agreements. Further studies on this aspect would be necessary to further address this point. In general, from the estimated probability distributions, it becomes evident that positive BTA indices are more common than negative values. These results are consistent with the general increase of international trade volumes in the course of the globalization [19] that also affects country pairs without a dedicated trade agreement.
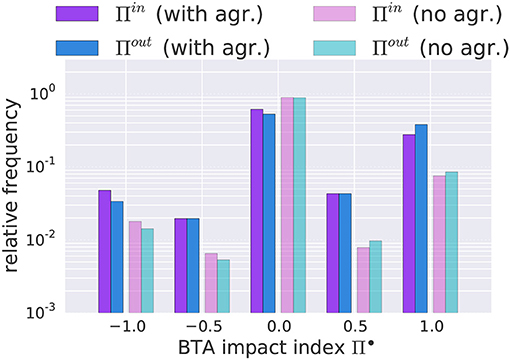
Figure 2. Distribution of the BTA impact indices Πin (purple) and Πout (blue) for all 107 BTAs that have been implemented between 1995 and 2008 (dark colors, see Table 1 in Appendix for a complete list). Light colors show the corresponding results for all 15,199 pairs of countries that have not negotiated bilateral agreements assuming an arbitrary reference year of 2002. Each of the four distributions is normalized to one and thus depicts the relative frequency. Note the logarithmic scaling of the displayed empirical frequencies.
Studying these results in more detail, we find that most countries with BTAs actually exhibit a positive average BTA impact index in both their export (Πout, Figure 3, top panel] and import linkages (Πin, Figure 3, bottom panel] to their partners. For some countries, especially the US, Australia, India and Columbia, the relative importance of the export linkages to their partners has increased more strongly than the relative importance of the import linkages from their partners. On the other hand, for other countries, such as the Philippines, Algeria, the southern African countries and Uruguay, the import linkages from their partners have gained in importance to a larger extent than their corresponding export linkages. Some notable exceptions that exhibit non-positive values of Π for both, exports and imports include the Ukraine, Bahrain, Jordan, and Belarus. The only G20 members with non-positive values are Indonesia and China.
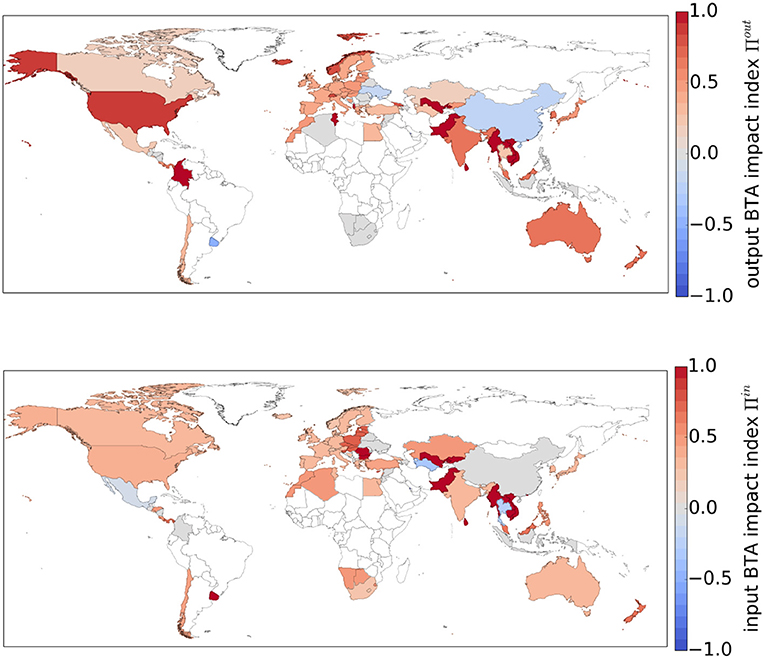
Figure 3. Global maps of average BTA impact indices. Shown are the country's export (Πout, top) and import linkages (Πin, bottom). Red values indicate that on average, the relative importances of the partners have increased for the respective countries. The average is taken over all the trade partners with which a specific country has implemented a BTA between 1995 and 2008.
In this context, it is particularly remarkable that China as one of the world's leading economies did not increase the relative importance of its agreement partners for its domestic production. When examining the composition of the average BTA impact index for China in more detail (Figure 4], the positive values of the score parameter z (see section 2) indicate that for most BTAs, the level of both input and output TI of China to its partners has increased after the date of entry into force. However, there is no continuing positive trend in the TI of China to its partners for any of these agreements (see the red shaded area in the top panels of Figure 4]. This suggests that China's agreement partners did not experience any persistent increase in importance for China's production after the BTAs have been established. In turn, considering the reciprocal TI of China's partners, the relative importance of their export and import linkages to China continuously increased during the first 5 years of the implementation period of the agreement (see bottom panels of Figure 4].
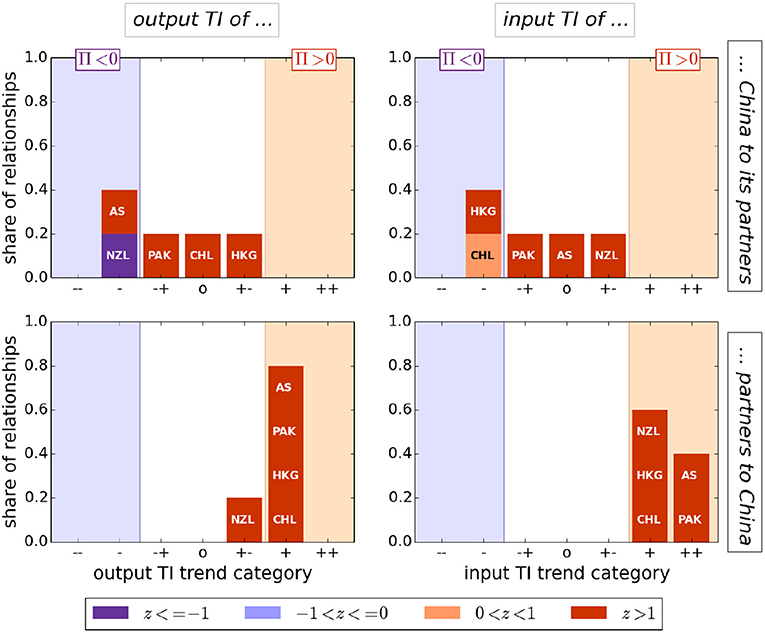
Figure 4. Trade profile for China. The TI of China to its partners (top) and of the partners to China (bottom) in the 5-year period after the date of entry into force tf of the respective BTA. The left and right panels present the results for the export (import) linkages. The partners of China with a BTA include Chile (CHL), Hong Kong (HKG), New Zealand (NZL), Pakistan (PAK) and the Association of Southeast Asian Nations (AS). Values of the score parameter z shown in red (purple) imply a higher (lower) level of TI in the 5-year period after tf. Trade agreements in the red (purple) shaded regions indicate a positive (negative) trend of the TI between the partners after tf. A positive (negative) value of the BTA impact index Π is assigned to a trade agreement if it is succeeded by both a positive trend and a higher level (negative trend and lower level) of the TI.
A distinctively different picture emerges for the BTAs involving the US (Figure 5]. All 8 US partners but Jordan have become more important for US exports. While the importance of the partners' imports for the US reached higher levels after BTA implementation, only Australia, the Central American Common Market and Jordan showed an enduring positive trend in the input TI of the US. In the opposite direction, the US have not become relatively more important for their aforementioned partners. This applies to both, export and import linkages of the partners to the US (except for the import linkages of Morocco). In some cases, we even observe values of Π < 0 with both negative trends and lower levels after the agreement in the TI of the US' partners.
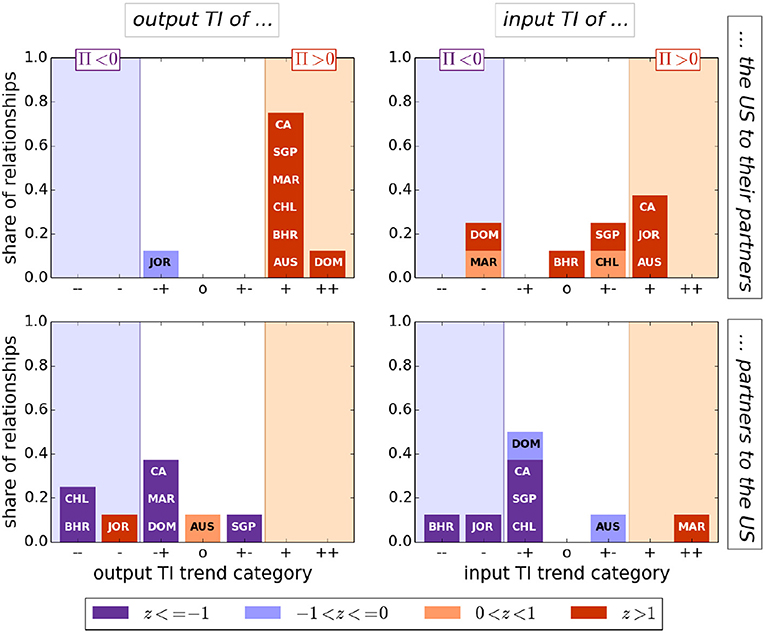
Figure 5. Trade profile for the US, with definitions as in Figure 4. The US have negotiated BTAs with Australia (AUS), Bahrain (BHR), Chile (CHL), the Dominican Republic (DOM), Jordan (JOR), Morocco (MAR), Singapore (SGP) and the Central American Common Market (CA) that became effective between 1995 and 2008.
Sensitivity Analysis: Influence of the Maximal Path Length
The consideration of higher-order (indirect) effects of trade flows is crucial to account for the potential existence of strong mutual production dependencies between industries that are not direct suppliers or consumers of each other but still members of the same supply chain. These dependencies arise, for example, if two industries have the same trade partner j, i.e., if the industries i and k are connected by a path of length 2. Specifically, in a scenario in which j buys commodities (inputs) from k and sells goods to i, the node i might be affected by a supply shortage of k which is further mediated via j [34–36]. To further assess the impact of these higher-order dependencies, we investigate the role of the maximal path length αmax in the definition of the TI.
The nodes representing the final demand take the role of sinks in the economic flow network of goods, causing a fast saturation in TIout with increasing αmax. In contrast, these nodes become sources of payment flows for which a converging behavior of TIin is not observed. This is illustrated in Figure 6 showing the distributions of TIout and TIin, respectively, for different values of αmax. Here, all pairs of countries are accounted for that have negotiated a BTA in the investigated time period. It can be seen that the values of TIin do not converge for economically reasonable path lengths. Furthermore, we observe in Figure 7 that the BTA impact indices Πin of the countries' inputs show a tendency toward smaller values with increasing αmax. In Figures 7A,B, the input BTA impact indices Πin of all countries are shown for αmax = 1 (αmax = 10). A trend toward smaller values can be observed, for example, in Europe, Australia, Algeria and Central America. This general trend occurs because loops within one country of the trade network gain importance for the TIin for higher values αmax. The probabilities of these national loops decrease with time, as international trade has increased in the investigated time period [19]. An example of the time series of TIin of Algeria to the European Union is displayed in Figure 8. With increasing maximal path length, the BTA impact index decreases, as national loops become less probable in the more recent years.
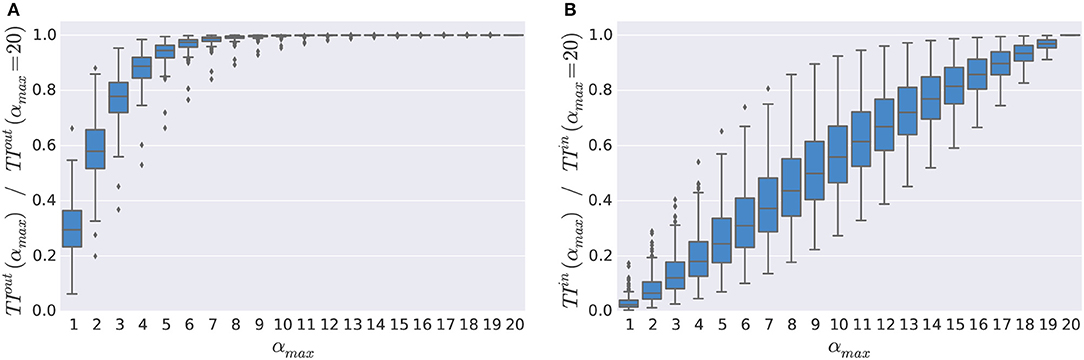
Figure 6. Box plot of the distributions of the trade interconnectedness (A) TIout and (B) TIin taken over all country pairs with a BTA (see Table 1 in the Appendix for different choices of the maximal path length αmax). The distributions depict the TI• values in the ITN of 2002. In both panels, the ratio of TI• with respect to its value at a reference maximal path length of αmax = 20 is shown. The box depicts the quartiles of the distributions with the median indicated within the box. Outliers are displayed if they exceed 1.5 times the inter-quartile range.
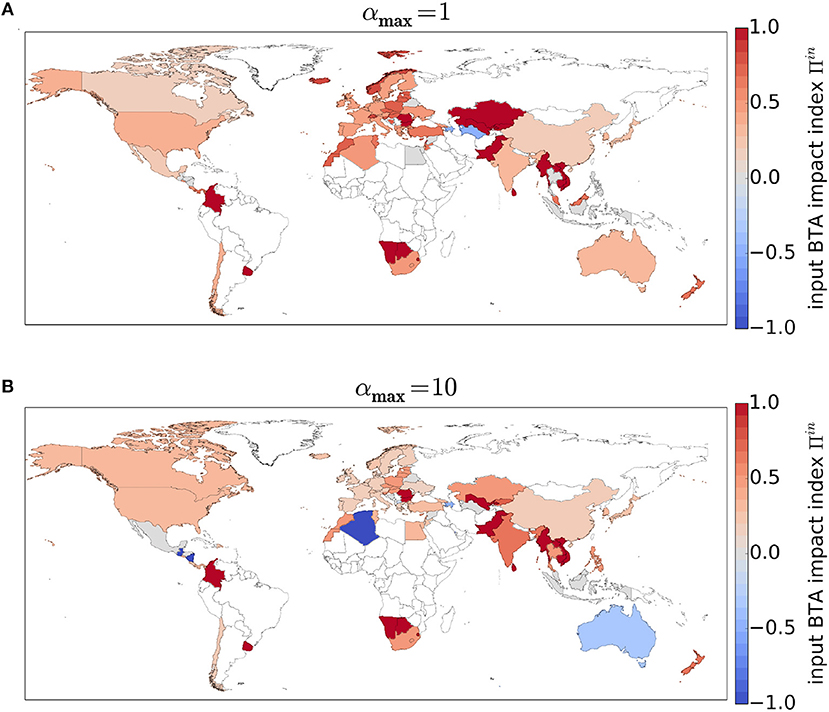
Figure 7. Global maps of the average input BTA impact indices Πin for different choices of the maximal length (A) αmax = 1 and (B) αmax = 10. The colors and averages can be interpreted as described in Figure 3.
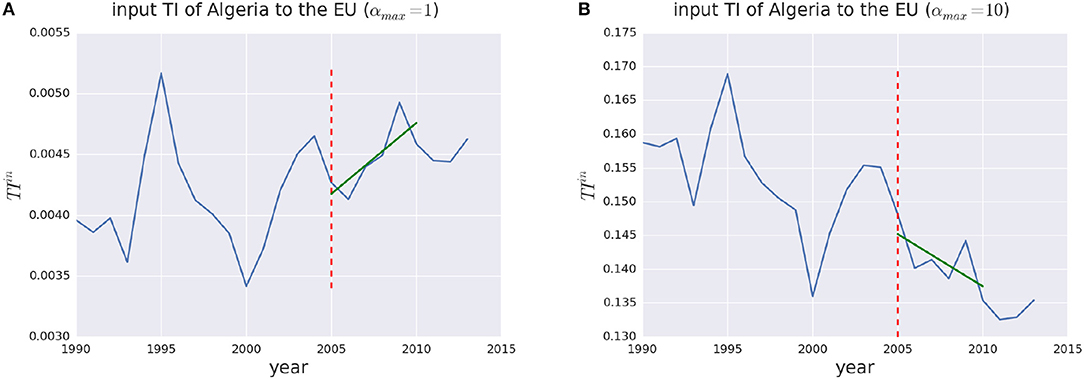
Figure 8. The input trade interconnectedness TIin of Algeria to the European Union for differenct choices of the maximal path length: (A) αmax = 1 and (B) αmax = 10. The year of 2005 in which the BTA came into effect is indicated by the red vertical line. The regression model selected by the AIC criterion is displayed by the green line indicating the corresponding maximum likelihood fit.
In order to provide a more detailed view on the trade profile of China, we illustrate China's input TI to its partners for the choices of αmax = 1 in Figure 9A and for αmax = 10 in Figure 9B. We observe that the trade agreements of China with New Zealand and Hong Kong follow the general tendency toward a lower BTA impact index with increasing maximal path length. However, in the input TI of China to Pakistan, a higher maximal path length increases the BTA impact index. The corresponding time series of the TI are shown in Figure 10. It can be seen that the higher BTA impact index can be attributed to a changing behavior of the TIin in 2009 with increasing αmax. In this year, the Great Recession triggered by the global financial crisis caused a decline in international trade, interrupting the general globalization trend in this year [19]. Thus, in this exceptional year, higher probabilities for national loops were likely to be observed as compared to the previous and following years. This exception is responsible for the increase of the input BTA impact index of China to Pakistan for increasing αmax.
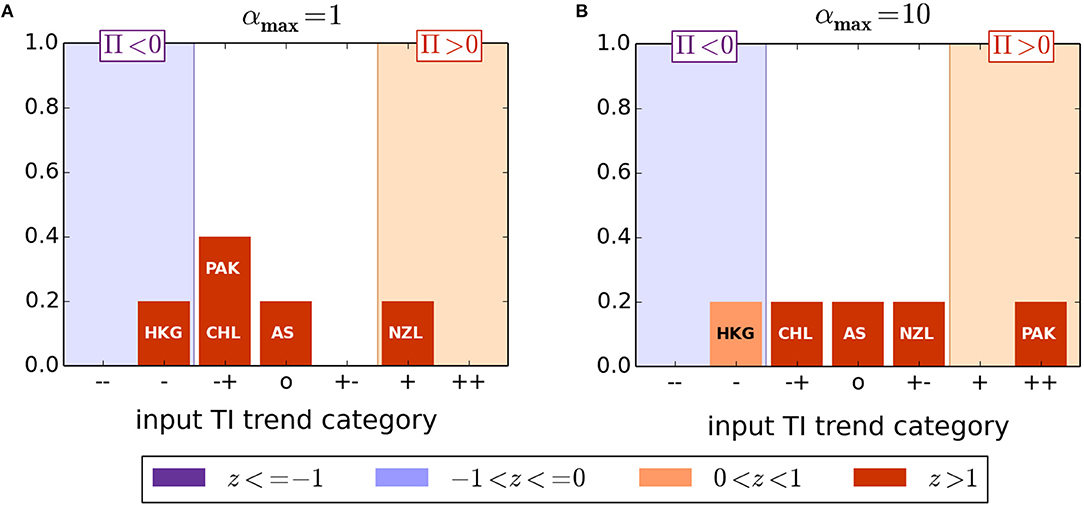
Figure 9. Trade profile for the input TI of China to its partners for a maximal path length of (A) αmax = 1 and (B) αmax = 10. The definitions and labels correspond to Figure 4.
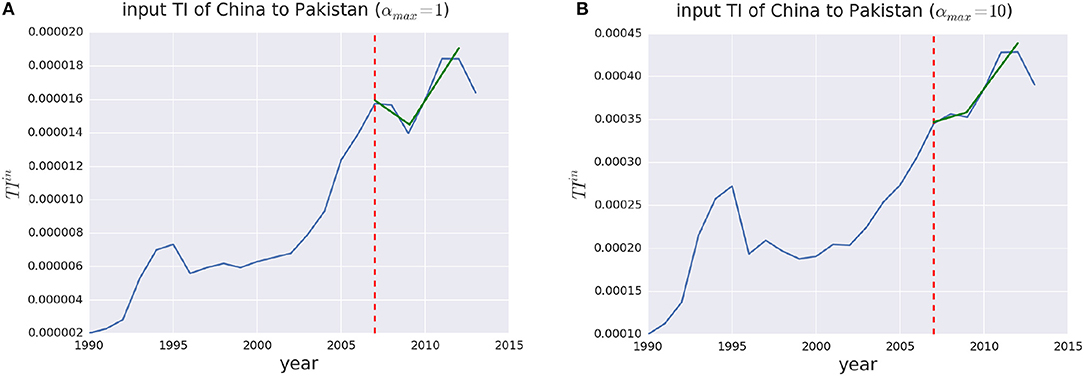
Figure 10. Input TI of China to Pakistan for (A) αmax = 1 and (B) αmax = 10 with definitions as in Figure 8.
The above discussion illustrates that the maximal path length αmax should not be chosen arbitrarily large, since otherwise longer paths within one country would be increasingly overrepresented in TIin. Studying this effect in more detail by means of probabilistic methods based upon the flow network representation used in this study might present an interesting research avenue for further methodological work, but may have limited economic value since such longer paths may crucially depend on the individual supply chains calling for case-specific interpretations. On the other hand, our analysis also demonstrates that higher-order effects, that are mediated through supply chains, affect the TI.
In view of this trade-off, we have set αmax to twice the average path length between the two subgraphs and of the ITN in all calculations presented in section 3. This choice has been motivated by the probability distribution of the average path lengths 〈d〉 for all country pairs with a trade agreement (Figure 11]. It thus allows the consideration of sufficiently high-order paths between these subgraphs while at the same time avoiding too large contributions from loops within any of them.
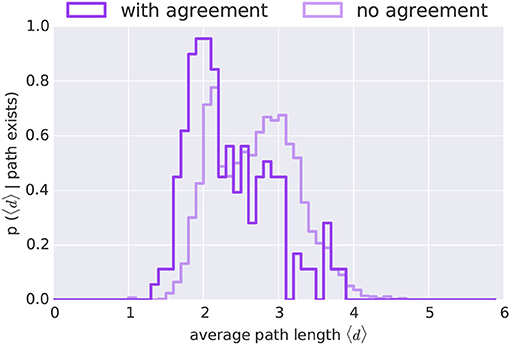
Figure 11. Probability distribution of the average path length of subnetworks of the ITN spanned by all pairs of countries with a bilateral trade agreement (solid purple) and without any agreement (light purple), given that a direct path between the countries exists. The values are obtained from the ITN for the year 2002.
We finally emphasize that the methodological framework used in this work can potentially provide a basis for addressing further more specific research questions in the context of BTAs, including the dependency of the efficiency of such agreements on their overall number and/or affected trade volume, respectively. Another interesting issue would be the existence of interferences between different BTAs affecting the same national economic sectors directly or indirectly via BTAs affecting some relevant trade partner. We outline further in-depth investigations along these lines as a relevant topic of future research.
Discussion and Conclusions
This study has provided quantitative evidence that BTAs in most cases result in a stronger TI between the involved partners during the first years after the respective agreement came into force. This finding further strengthens the corresponding conclusions from previous studies on direct trade flows in the gravity model. However, our analysis goes beyond these investigations by accounting for indirect effects that are mediated by cross-sectoral input-output linkages as well. A positive BTA impact index can potentially indicate that one main objective in the negotiation process for the respective country has been the interest in obtaining easier access to the partner's domestic market. The origins of such a positive index can then be twofold: On the one hand, with decreasing trade barriers new market opportunities are unlocked and result in an above average increase in trade volume between the agreement partners. On the other hand, a positive BTA impact index is also achieved if existing trade relations are substituted in favor of the agreement partner and at the expense of a third party. To quantify and identify the respective effects of trade generation vs. trade diversificaton, further comprehensive investigations on the trade relationships of each individual country are required, which however go beyond the scope of the present work. However, we have also identified BTAs with a non-positive BTA impact index. In these cases, the hypothesis that a BTA negatively affects such third parties is less likely. In turn, countries with a negative BTA impact index may have either pursued different strategic objectives than to simply boost the bilateral trade with the partners, or just did not achieve their original goals in the context of the BTA's implementation.
We have demonstrated that most western economies, as well as Japan and South Korea have increased the TI to their BTA partners for their export linkages to a larger extent than for their import linkages. This could indicate that these economies mainly focus in the negotiations on developing new sales markets for their respective domestic economies. On the other hand, countries in southern Africa, Uruguay, and the Philippines have increased the TI to their partners in import linkages to a larger extent than in their export linkages, suggesting a primary focus on fostering their own economic development on the long run by increasing the flow of goods and knowledge into the respective country.
China is the only member of the G20 exhibiting a negative BTA impact index regarding the TI for the export linkages to its partners. This observation would be compatible with the hypothesis that China pursues a different objective in its trade agreement negotiations than most other countries. The lack of a persistent increase in the TI of China to its partners can be explained by two predominant facts: On the one hand, China had already enjoyed easy access to the more open markets of its partners, such as in the case of Hong Kong, before the corresponding BTAs were implemented [17]. On the other hand, China has continuously increased both the number of trading partners and its international trade volume since the 1990s. In the course of these developments, the BTAs of China did not trigger a dis-proportionally large and persistent increase in bilateral trade with its agreement partners as compared to China's other trade activities. In contrast, as China has gradually become economically more important for its partners, its motivation for negotiating BTAs might have explicitly included the strategic goal to increase its economic and political influence among its trading partners.
The BTA impact profile of the US is distinctively different from that of China. The consistently positive BTA impact indices for the US' export linkages to their partners emphasize a possible focus on the stimulation of their own exports during the negotiations. Although the importance of import linkages from the agreement partners has also increased for the US, a persistent positive trend could only be observed for Australia, Central America, and Jordan. However, these increases in trade volume are less important for the US' partners as compared to their market expansions toward any third countries. The fact that both input and output TI of most partners to the US have reached significantly lower levels after the implementations of their respective BTAs indicates that even with increasing bilateral trade volumes, the economies of the partners have become less dependent on the US as trade partner. One possible reason for this observation could simply be a higher attractivity of other national markets for the affected trade partners in serving as alternative partners, e.g., due to generally lower labor costs in such third countries. Since corresponding strategic decisions are usually based on company-specific individual considerations, national political measures can only provide general economic boundary conditions, but will hardly be able to fully resolve the challenge of international economic competition.
The methodology employed in this study can be utilized for follow-up studies on specific BTAs or particular sectorial impacts of such agreements. For example, for a specific agreement of interest, the method unveils if any third countries have been potentially discriminated as a result of that agreement. More detailed case studies are rendered possible for input-output data with a higher sectoral detail. Moreover, we emphasize that our approach can be further adapted and extended to also allow for an assessment of the impact on TI among the partners of multilateral trade agreements, such as the North American Free Trade Agreement or the European Union. Furthermore, impact analyses may also clarify the relationship of the measures proposed in this work with other economic factors. Importantly the interrelationship with GDP growth is of great interest. Investigating the behavior of our measures within the framework of recently developed state-of-the-art network theoretical growth models (e.g., [37–40]) certainly provides a promising avenue for further research. Note that the methods presented in this work do not allow making forecasts about future BTA implementations or terminations, as would be particularly relevant in the context of ongoing discussions on drastically changed national economic and trade policies in various countries. Besides the already mentioned reconsiderations of the current US government, the possible impact of the planned withdrawal of the United Kingdom from the European Union (known as the Brexit) on the British national economy as well as the inner-European trade networks would be of great interest. However, as long as being based on empirical data instead of comparable economic model outputs, with the framework used in this work such impact studies can only be performed in retrospective, thus exceeding our current scope. However, corresponding investigations would provide an interesting subject of future work.
Data Availability Statement
The EORA multi-regional input-output database utilized for this study is available online at www.worldmrio.com.
Author Contributions
All authors listed have made a substantial, direct and intellectual contribution to the work, and approved it for publication.
Funding
This work has been financially supported by the German Federal Ministry of Education and Research (BMBF) via the Young Investigators Group CoSy-CC2 (grant no. 01LN1306A). We acknowledge the European Regional Development Fund (ERDF), the German Federal Ministry of Education and Research and the Land Brandenburg for supporting this project by providing resources on the high performance computer system at the Potsdam Institute for Climate Impact Research.
Conflict of Interest Statement
The authors declare that the research was conducted in the absence of any commercial or financial relationships that could be construed as a potential conflict of interest.
Supplementary Material
The Supplementary Material for this article can be found online at: https://www.frontiersin.org/articles/10.3389/fphy.2018.00134/full#supplementary-material
References
1. Office of the US Trade Representative (USTR). The President's 2017 Trade Policy Agenda (2017). Available online at: https://ustr.gov/sites/default/files/files/reports/2017/AnnualReport/AnnualReport2017.pdf (Accessed October 30, 2017)
3. Carràre C. Revisiting the effects of regional trade agreements on trade flows with proper specification of the gravity model. Eur Econ Rev. (2006) 50:223–47. doi: 10.1016/j.euroecorev.2004.06.001
4. Baier SL, Bergstrand JH. Do free trade agreements actually increase members' international trade? J Int Econ. (2007) 71:72–95. doi: 10.1016/j.jinteco.2006.02.005
5. Cipollina M, Salvatici L. Reciprocal trade agreements in gravity models: a meta-analysis. Rev Int Econ. (2010) 18:63–80. doi: 10.1111/j.1467-9396.2009.00877.x
6. Anderson JE, Yotov YV. Terms of trade and global efficiency effects of free trade agreements, 19902002. J Int Econ. (2016) 99:279–98. doi: 10.1016/j.jinteco.2015.10.006
7. Dai M, Yotov YV, Zylkin T. On the trade-diversion effects of free trade agreements. Econ Lett. (2014) 122:321–5. doi: 10.1016/j.econlet.2013.12.024
8. Krugman P. Is Bilateralism Bad? Cambridge, MA: National Bureau of Economic Research (1989). p. 2972. Available online at: https://ideas.repec.org/p/nbr/nberwo/2972.html
9. Krugman PR. The move toward free trade zones. In: Proceedings - Economic Policy Symposium. Jackson Hole (1991). p.7–58. Available online at: https://ideas.repec.org/a/fip/fedkpr/y1991p7-58.html
10. Frankel JA, Stein E, Wei SJ. Regional trading arrangements: natural or supernatural. Am Econ Rev. (1996) 86:52–6.
11. Vicard V. Determinants of successful regional trade agreements. Econ Lett. (2011) 111:188–90. doi: 10.1016/j.econlet.2011.02.010
12. Ghosh S, Yamarik S. Does trade creation measure up? A reexamination of the effects of regional trading arrangements. Econ Lett. (2004) 82:213–9. doi: 10.1016/j.econlet.2003.06.001
13. Dür A, Baccini L, Elsig M. The design of international trade agreements: Introducing a new dataset. Rev Int Organ. (2014) 9:353–75. doi: 10.1007/s11558-013-9179-8
14. Whalley J. Why do countries seek regional trade agreements? In: Frankel JA, editor. The Regionalization of the World Economy. Cambridge, MA: National Bureau of Economic Research; University of Chicago Press (1998). p. 63–90.
15. Baccini L, Urpelainen J. International institutions and domestic politics: can preferential trading agreements help leaders promote economic reform? J Polit. (2014) 76:195–214. doi: 10.1017/S0022381613001278
16. Feinberg RE. The political economy of United States free trade arrangements. World Econ. (2003) 26:1019–40. doi: 10.1111/1467-9701.00561
17. Antkiewicz A, Whalley J. China's new regional trade agreements. World Econ. (2005) 28:1539–57. doi: 10.1111/j.1467-9701.2005.00746.x
18. Serrano A, Boguna M. Topology of the world trade web. Phys Rev E. (2003) 68:015101. doi: 10.1103/PhysRevE.68.015101
19. Maluck J, Donner RV. A network of networks perspective on global trade. PLOS ONE (2015) 10:e0133310. doi: 10.1371/journal.pone.0133310
20. Fagiolo G, Squartini T, Garlaschelli D. Null models of economic networks: the case of the world trade web. J Econ Int Coordinat. (2013) 8:75–107. doi: 10.1007/s11403-012-0104-7
21. Wenz L, Levermann A. Enhanced economic connectivity to foster heat stress related losses. Sci Adv. (2016) 2:e1501026. doi: 10.1126/sciadv.1501026
22. Li W, Kenett DY, Yamasaki K, Stanley HE, Havlin S. Ranking the economic importance of countries and industries. J Netw Theory Finan. (2017) 3:1–17. doi: 10.21314/JNTF.2017.031
23. Gould D, Kenett DY, Panterov G. Multidimensional Connectivity: Benefits, Risks, and Policy Implications for Europe and Central Asia. Policy Research Working Paper. (2017) p. 8438.
24. Sopranzetti S. Overlapping free trade agreements and international trade: a network approach. World Econ. (2018) 41:1549–66. doi: 10.1111/twec.12599
25. Lenzen M, Kanemoto K, Moran D, Geschke A. Mapping the structure of the world economy. Environ Sci Techn. (2012) 46:8364–81. doi: 10.1021/es300171x
26. Lenzen M, Moran D, Kanemoto K, Geschke A. Building eora: a global multi-regional input-Output database at high country and sector resolution. Econ Syst Res. (2013) 25:20–49. doi: 10.1080/09535314.2013.769938
27. Maluck J, Donner RV. Distributions of positive correlations in sectoral value added growth in the global economic network. Eur Phys J B. (2017) 90:26. doi: 10.1140/epjb/e2016-70485-7
28. World Trade Organization. Regional Trade Agreements Information System (2017).
Available online at: http://rtais.wto.org/UI/PublicMaintainRTAHome.aspx (Accessed November 03, 2017).
29. Garlaschelli D, Loffredo MI. Structure and evolution of the world trade network. Physica A. (2005) 255:138–44. doi: 10.1016/j.physa.2005.02.075
30. Fagiolo G, Reyes J, Schiavo S. World-trade web: Topological properties, dynamics, and evolution. Phys Rev E. (2009) 79:036115. doi: 10.1103/PhysRevE.79.036115
31. Donner RV, Hernández-García E, Ser-Giacomi E. Introduction to Focus Issue: Complex network perspectives on flow systems. Chaos (2017) 27:035601. doi: 10.1063/1.4979129
32. Muggeo VMR. Estimating regression models with unknown break-points. Statis Med. (2003) 22:3055–71. doi: 10.1002/sim.1545
33. Akaike H. A new look at the statistical model identification. IEEE Trans Autom Control. (1974) 19:716–23.
34. Otto C, Willner SN, Wenz L, Frieler K, Levermann A. Modeling loss-propagation in the global supply network: The dynamic agent-based model acclimate. J Econ Dynam Control. (2017) 83:232–69. doi: 10.1016/j.jedc.2017.08.001
35. Bierkandt R, Wenz L, Willner S, Levermann A. Acclimate: a model for economic damage propagation. Part I: basic formulation of damage transfer within a global supply network and damage conserving dynamics. Environ Syst Decis. (2014) 43:507–24. doi: 10.1007/s10669-014-9523-4
36. Wenz L, Willner S, Bierkandt R, Levermann A. Acclimate - a model for economic damage propagation. Part II: a dynamic formulation of the backward effects of disaster-induced production failures in the global supply network. Environ Syst Decis. (2014) 34:525–39. doi: 10.1007/s10669-014-9521-6
37. Hausmann R, Hidalgo CA. The network structure of economic output. J Econ Growth. (2011) 16:309–42. doi: 10.1007/s10887-011-9071-4
38. Acemoglu D, Carvalho VM, Ozdaglar A, Tahbaz-Salehi A. The network origins of aggregate fluctuations. Econometrica. (2012) 80:1977–2016. doi: 10.3982/ECTA9623
39. Cristelli M, Tacchella A, Pietronero L. The heterogeneous dynamics of economic complexity. PLOS ONE (2015) 10:e0117174. doi: 10.1371/journal.pone.0117174
Keywords: trade agreements, international trade, complex networks, network of networks, random walk
Citation: Maluck J, Glanemann N and Donner RV (2018) Bilateral Trade Agreements and the Interconnectedness of Global Trade. Front. Phys. 6:134. doi: 10.3389/fphy.2018.00134
Received: 01 August 2018; Accepted: 07 November 2018;
Published: 27 November 2018.
Edited by:
Thiago Christiano Silva, Universidade Católica de Brasília, BrazilReviewed by:
Dror Y. Kenett, Johns Hopkins University, United StatesBenjamin Miranda Tabak, Fundação Getúlio Vargas, Brazil
Francesco Caravelli, Los Alamos National Laboratory (DOE), United States
Copyright © 2018 Maluck, Glanemann and Donner. This is an open-access article distributed under the terms of the Creative Commons Attribution License (CC BY). The use, distribution or reproduction in other forums is permitted, provided the original author(s) and the copyright owner(s) are credited and that the original publication in this journal is cited, in accordance with accepted academic practice. No use, distribution or reproduction is permitted which does not comply with these terms.
*Correspondence: Reik V. Donner, cmVpay5kb25uZXJAcGlrLXBvdHNkYW0uZGU=