- 1Department of Applied Mathematics, Hong Kong Polytechnic University, Hong Kong, China
- 2Clinical Research Center, Zhujiang Hospital, Southern Medical University, Guangzhou, China
- 3JC School of Public Health and Primary Care, Chinese University of Hong Kong, Hong Kong, China
- 4Shenzhen Research Institute of Chinese University of Hong Kong, Shenzhen, China
- 5College of Medical Information Engineering, Chengdu University of Traditional Chinese Medicine, Chengdu, China
- 6School of Mathematics and Statistics, Huaiyin Normal University, Huai'an, China
- 7School of Nursing, Hong Kong Polytechnic University, Hong Kong, China
In December 2019, novel coronavirus disease (COVID-19) hit Wuhan, Hubei Province, China and spread to the rest of China and overseas. The emergence of this virus coincided with the Spring Festival Travel Rush in China. It is possible to estimate the total number of COVID-19 cases in Wuhan, by 23 January 2020, given the cases reported in other cities/regions and population flow data between Wuhan and these cities/regions. We built a model to estimate the total number of COVID-19 cases in Wuhan by 23 January 2020, based on the number of cases detected outside Wuhan city in China, with the assumption that cases exported from Wuhan were less likely underreported in other cities/regions. We employed population flow data from different sources between Wuhan and other cities/regions by 23 January 2020. The number of total cases in Wuhan was determined by the maximum log likelihood estimation and Akaike Information Criterion (AIC) weight. We estimated 8 679 (95% CI: 7 701, 9 732) as total COVID-19 cases in Wuhan by 23 January 2020, based on combined source of data from Tencent and Baidu. Sources of population flow data impact the estimates of the total number of COVID-19 cases in Wuhan before city lockdown. We should make a comprehensive analysis based on different sources of data to overcome the bias from different sources.
Introduction
In December 2019, a cluster of patients with pneumonia of unknown causes was reported in Wuhan, Hubei Province, China [1]. On 9 January 2020, a novel coronavirus, named SARS-CoV-2, was identified as the cause of this outbreak [2]. The emergence of this virus coincided with the Spring Festival Travel Rush in China. It was estimated that there would be around 3 billion trips made in China during the period of 10 January to 18 February 2020 [3]. Some researchers have pointed out the risk of the regional and global disease spreading during the Spring Festival Travel Rush [4]. However, due to the small number of severe cases reported by mid-January and most cases were linked to the Huanan Seafood Market of Wuhan city, neither international nor regional traveling restrictions were implemented to Wuhan at the early stage of this outbreak. On 13 January 2020, the first case exported from Wuhan was reported in Thailand and the case numbers dramatically increased after the diagnostic kits became available in mid-January. As of 13 July 2020, there were 85 560 laboratory confirmed cases and 4 648 deaths (58.8 and 83.2% in Wuhan) [5]. In recognition of a wide-spreading outbreak, the government has suspended all public transportations inside Wuhan city since 23 January 2020, and some regional traveling restrictions were also implemented by other cities/regions [6].
Methods
Objective
At the early stage of this outbreak, the cases might have been severely underreported due to the lack of diagnostic kits and insufficient screening for all suspected cases [7, 8]. Several efforts have been made to estimate the COVID-19 case numbers in Wuhan using different modeling approaches, and the estimates range from 4 000 to 75 815 during the period of 18–29 January [8–10].
In this study, we aimed to estimate the number of COVID-19 cases in Wuhan, based on the cases exported from Wuhan to other cities/regions in mainland China and different sources of the population flow data between Wuhan and these cities/regions. We tested the impact of different sources of population flow data on estimating cases in Wuhan before city lockdown and combined different sources of data to overcome the bias from different sources. The estimates were made by 23 January 2020 (before the suspension of public transportations in Wuhan). We assumed that the cases exported from Wuhan were less likely underreported in other cities/regions in mainland China, as stringent temperature screening was implemented at airports and railway stations.
Data
We obtained daily number of inbound and outbound domestic passengers traveling by air, train or road to/from Wuhan from two data sources:
(1) Tencent's LBS (location-based services) database (see: https://heat.qq.com/). According to location data of Tencent's mobile software users, population flow number during 10 December 2016 and 24 January 2017 was generated, between Wuhan and 24 cities/regions in China (Anhui, Beijing, Chongqing, Fujian, Gansu, Guangdong, Guangxi, Guizhou, Hainan, Hebei, Heilongjiang, Henan, Hunan, Jiangsu, Jiangxi, Jilin, Liaoning, Ningxia, Shandong, Shanghai, Sichuan, Tianjin, Yunnan, Zhejiang). We assumed that the amount of population flow in 2017 is same as that in 2020.
(2) Baidu map database (see: https://qianxi.baidu.com/). According to location data of Baidu's mobile software users, population flow number from 1 to 20 January 2020 was generated, between Wuhan and 26 cities/regions (Shanxi, Shaanxi, and other cities/regions are the same as Tencent data).
We equally divided the population flow data from different sources separately to get average daily population flow number. Figure 1 shows the geographical location of cities/regions which reported COVID-19 cases and the number of COVID-19 cases in each city/region.
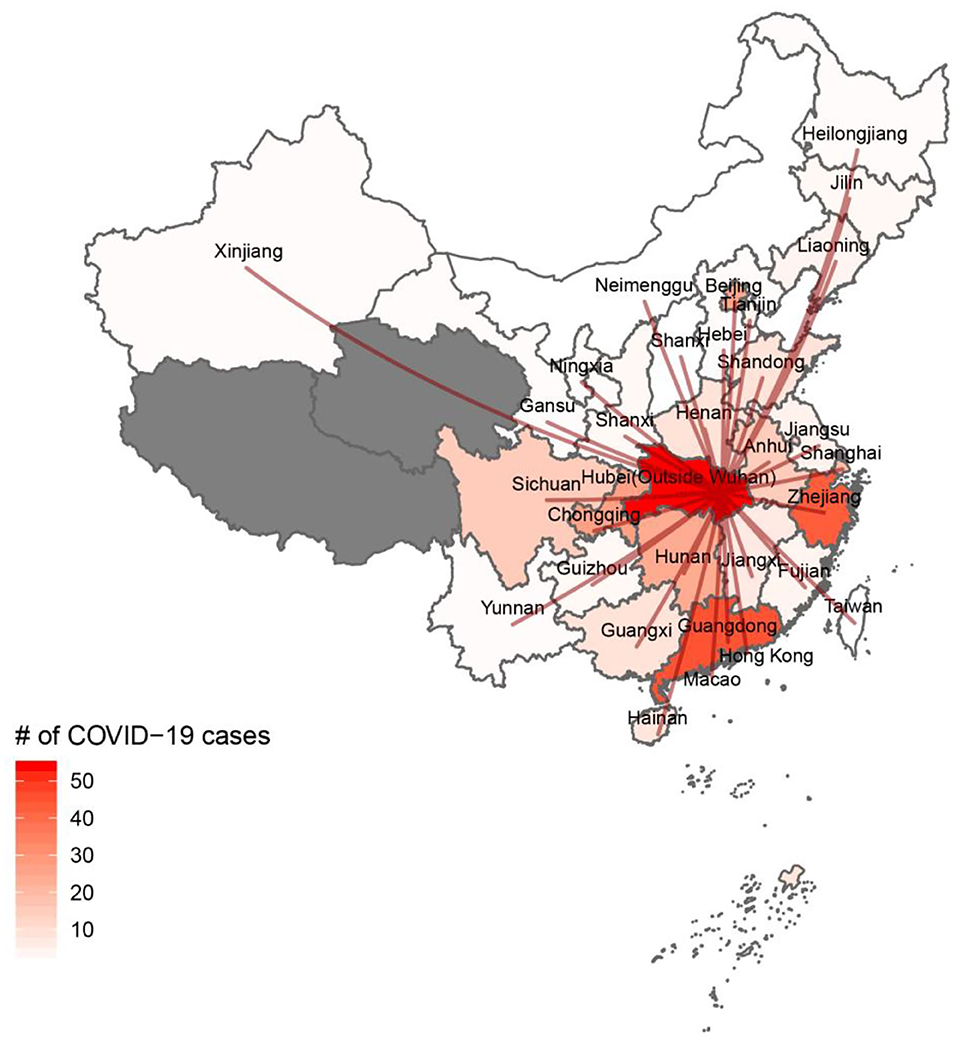
Figure 1. The geographical distribution of exported COVID-19 cases in China. This figure reported number of reported COVID-19 cases in China, the dark gray area indicates the regions with zero COVID-19 cases as of 23 January 2020. Red paths show routes from Wuhan to other cities/regions.
As shown in Table S1, we collected total numbers of reported COVID-19 cases exported from Wuhan to other cities in China by 23 January 2020, and cases which were not exported from Wuhan (e.g., family or hospital clusters) were excluded from the analysis [11]. Thirteen cases were excluded due to the lack of traveling history to Hubei before illness onset. As for 161 cases that not specified the traveling history, we assumed that the probability of a single case being an exported case is θ, and each case is independent of each other. Then all of these unspecified cases follow a binomial distribution. As shown in Equation (1), θ represents the probability that a case is exported from Wuhan and n means the number of COVID-19 cases that not specified the traveling history, which is 161. P represents the probability that k out of n COVID-19 cases came from Wuhan. Since the most cases detected outside Wuhan are exported cases from Wuhan, by 23 January 2020 [12], we assumed that the probability of a case to be an exported case from Wuhan is based on a different level of θ (1, 0.9, 0.8). Then we obtained the expectation number of cases exported from Wuhan in city/region i.
Models
Table S3 presents the general process of the whole method. The total number of COVID-19 cases exported from Wuhan and diagnosed in each city/region outside Wuhan by 23 January 2020 was assumed to follow a Binomial distribution [8], as in Equation (2), where λ is the total number of cases infected in Wuhan by 23 January and pi is the probability of detecting any exported cases from Wuhan in city/region i outside Wuhan in China.
The probability pi can be derived from dividing daily outbound passengers of Wuhan to city/region i by the population size that the Wuhan airport, railway and road serves and multiplying by the mean time for patients from being infected to being detected, see Equation (3).
Then, we used cases exported from Wuhan to estimate the total number of COVID-19 cases infected in Wuhan (λ). Based on the data obtained from each city/region, we obtained the λ by maximum likelihood estimation.
In Equation (4), l(·) and L(·) are the total log-likelihood and the total likelihood, respectively. f(·) is the function for computing the value of the probability density function of the binomial distribution (Equation 2). The k represents the total estimated number of cities/regions. The ni represents the number of cases exported from Wuhan and detected in city/region i, and pi means the probability of finding any exported cases from Wuhan in in city/region i. The 95% confidence intervals (95% CI) of log-likelihood, l, can be calculated after obtaining λ, since residuals of log maximum likelihood estimation follow Chi-square distribution [13]. Then we can extrapolate a 95% CI about the total number of COVID-19 cases infected in Wuhan. As in Equation (5), by deducting the number of exported cases from the total number of cases infected in Wuhan, we got the final estimate of the total number of COVID-19 cases in Wuhan as of 23 January 2020.
We assumed a population of 19 million (catchment population) traveling through the airport, railway stations and highways in Wuhan, and a 10-days delay on average, which accounted for the time interval reported between infection timing and case timing [8]. Since exported cases were much less than those in Wuhan as of 23 January 2020, it was assumed that all cases in other cities/regions outside Wuhan are detected. If cases in other cities/regions are missed, our estimate would underestimate the acute number of cases in Wuhan. In addition, we assumed that all of the passengers outflowed from Wuhan were equally likely to be infected, regardless of transfer passengers or local residents, as passengers may be a high risk of infection while traveling in trains and airplanes cabins.
To overcome the bias from different sources of data, we first evaluated the correlation between two datasets to determine whether there is an apparent inconsistency or discrepancy between different sources of data. We found that the Spearman's rank correlation coefficient of Baidu and Tencent data for the same 24 cities/regions is 0.75, which means that two sources of data are correlated under 99.99% confidential level. We assumed a linear relationship between the Baidu data and Tencent data (see Figure 2). For all observations, we assumed error terms are independent of each other. We also assumed all error terms follow a normal distribution and have the same variance. We then built the linear model (Equation 6) and tested the null hypothesis H0 that α = 0. In Equation (6), NBaidu and NTencent represent the number of population flow data from Baidu and Tencent.
We got the result that estimated coefficient α equals 0.10, β equals 1 272 and P-value for F-test was <0.01. Then we rejected the null hypothesis H0 under 99% CI, which suggests that two sources of data are likely to have a linear relation. Since both sets of data is likely to be reasonable. We then applied Akaike Information Criterion (AIC) [14] to test the fitting result of number of cases exported from Wuhan and detected in city/region i, nest<uscore>i, which follows a binomial distribution (Equation 2), based on Baidu and Tencent data, see Equation (7). To estimate the number of cases exported from Wuhan, the model used estimated the total number of COVID-19 cases infected in Wuhan (λ) from Equation (4). pi is the probability that we will find any exported cases from Wuhan in city/region i outside Wuhan in China, which we have already obtained from Equation (3). Please note that we only included 24 cities/regions of which both Baidu and Tencent have population flow data in AIC weight calculation.
Since Baidu and Tencent data show significant linear relationship, which confirmed with each other that the general pattern of data is rational, we weighted (Equation 8) and combined (Equation 9) the estimated number of cases from Baidu and Tencent based on AIC value to obtain the final estimate.
In Equations (8) and (9), Ws and AICs represents the weight of estimated number of COVID-19 cases infected in Wuhan and AIC value for source s, respectively. λs is the estimate of the total number of cases from Equation (4), based on source s, and λ means the final estimate of total number of cases infected in Wuhan by 23 January 2020.
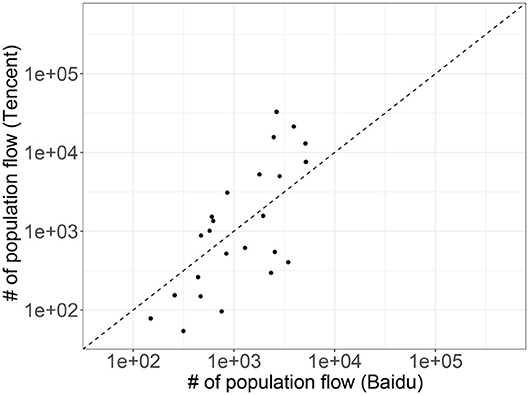
Figure 2. Comparison between the number of population flow data from Baidu and Tencent. Y-axis presents the number of population flow between Wuhan and other cities/regions from Tencent data. X-axis presents the number of population flow between Wuhan and other cities/regions from Baidu data.
Result
Based on the data sourced from Tencent and Baidu, we estimated the total number of cases in Wuhan, λ (Figure 3). Then we estimated the 95% CI of the total number of COVID-19 cases. We estimated 4 672 (4 129, 5 257) and 12 950 (11 510, 14 502) as total cases in Wuhan by 23 January 2020, based on Tencent and Baidu population flow data. In addition, based on the AIC weighting (Table 1), we combined results from Baidu and Tencent and estimated 8 679 (7 701, 9 732) as total cases in Wuhan. Table 2 presents the estimates under different sources and different level of probability of an unspecified case reported in other cities/regions being an exported case from Wuhan. Table S2 present the probability of finding any cases for each city/region outside Wuhan.

Table 1. AIC value and calculated Weight of final estimate for different sources of population data (under different assumptions of, θ, probability of an unspecified case reported in other cities/regions being an exported case from Wuhan).
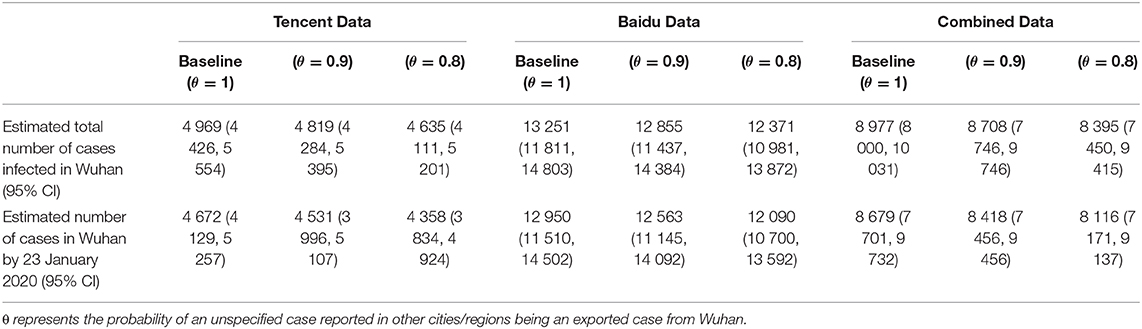
Table 2. Summary table of estimated total number of cases infected in Wuhan (including cases exported from Wuhan to other cities/regions) and number of cases in Wuhan (excluding cases exported from Wuhan to other cities/regions) by 23 January 2020, from different sources of data.
Discussion
A recent study by Imai et al. estimated that a total of 4 000 (95% CI: 1 000–9 700) cases on 18 January 2020 [8]. Compared with the total number of confirmed cases provided by the government as of 23 January 2020, which is 495 [15], Imai et al. obtained around 8-fold of cases before 23 January [8]. This is partly because the screening effort targeting population from Wuhan in other cities is much more effective than the local screening effort in Wuhan due to the worsening situation. Estimates based on combination of Baidu data and Tencent data provided closer result by Imai et al.'s [8] than the official report [15]. Our model is mostly like Imai et al.'s [8]. The difference between the two models is that we estimated the total number of cases in Wuhan based on separated data of each city/region in China. In addition, we applied maximum likelihood estimation by calculating the log-likelihood value for each city/region. Imai et al. [8] obtained the estimate based on overall overseas data, applying maximum likelihood estimation by calculating the simple ratio. In the sensitivity analysis, Table 2 shows that when the probability of an unspecified case reported in other cities/regions being an exported case from Wuhan is close to 1, slight fluctuations of the probability will have little impact on the estimation.
Estimates of the population outflow provided by Baidu and Tencent show substantial fluctuation, leading to results with significant differences. We found that Baidu and Tencent data show significant linear relation, which means that pattern of two sources of data is largely consistent. One possible reason for the phenomenon is that different institutions have a various definition of the number of people flow from one city to another. Methods include people who travel to other cities through Wuhan in the population flow may provide a much more significant figure than those that only calculate people who originally depart from Wuhan. At the same time, multiple round trips may also affect the count. Another possible reason is that Baidu and Tencent would fail to track the whole amount of population flow since not everyone uses mobile phone software from Baidu and Tencent.
Imai et al. suggested that by further improving the definition and testing of COVID-19 cases, and further expanding the scope of epidemic monitoring, the gap between the estimated number and official reported cases would be further narrowed. According to our results, statistics of population flow also play significant roles in estimation. At present, many researches use data from Baidu and Tencent platforms [10, 16–18]. Among them, Tian et al. [18] referred to different data sources to gain a more comprehensive measure of movement volume. According to the data presented in the article, Tian et al. [18] integrated the population flow data first, and then conducted relevant calculation and analysis. In this paper, we calculated estimates from each data source first and then weighted the results. Both of methods provide more reasonable results ranged between conclusions that generated by either data sources, contributing to overcome the bias from different sources.
Conclusions
Different sources of population flow data impact the estimates of the total number of COVID-19 cases in Wuhan before city lockdown. We built a model that could be reproduced to employ incompatible sets of population flow data to estimate the number of COVID-19 cases more reasonably. We estimated 8 679 (95% CI: 7 701, 9 732) as total COVID-19 cases in Wuhan by 23 January 2020, based on the combined source of data from Tencent and Baidu. What data source can be used to make the most reliable estimation is not clear yet, though estimates based on a single source of data are likely to be biased. A comprehensive analysis based on different statistics is need before we reach any conclusions.
Data Availability Statement
Publicly available datasets were analyzed in this study. This data can be found here: https://github.com/Larryzza/COVID-19.
Author Contributions
All authors listed have made a substantial, direct and intellectual contribution to the work, and approved it for publication.
Funding
DH was supported by General Research Fund (15205119) of Research Grants Council of Hong Kong and Alibaba (China) Co. Ltd. Collaborative Research grant. WW was supported by National Natural Science Foundation of China (Grant Number 61672013) and Huaian Key Laboratory for Infectious Diseases Control and Prevention (Grant Number HAP201704), Huaian, Jiangsu, China. PC was supported by National Natural Science Foundation of China (Grant Number 81903406).
Disclaimer
Frontiers Media SA remains neutral with regard to jurisdictional claims in published maps and institutional affiliations.
Conflict of Interest
DH received a grant from Alibaba (China) Co. Ltd., Collaborative Research grant.
The remaining authors declare that the research was conducted in the absence of any commercial or financial relationships that could be construed as a potential conflict of interest.
Supplementary Material
The Supplementary Material for this article can be found online at: https://www.frontiersin.org/articles/10.3389/fphy.2020.00336/full#supplementary-material
References
1. Zhu N, Zhang D, Wang W, Li X, Yang B, Song J, et al. A novel coronavirus from patients with pneumonia in China, 2019. N Engl J Med. (2020) 382:727–33. doi: 10.1056/NEJMoa2001017
2. WHO Statement Regarding Cluster of Pneumonia Cases in Wuhan, China. World Health Organization (WHO). Available online at: https://www.who.int/china/news/detail/09-01-2020-who-statement-regarding-cluster-of-pneumonia-cases-in-wuhan-china (accessed January 9, 2020)
3. In 2020, the National Passenger Volume of Spring Festival Transportation Will Reach About 3 Billion Person Times. xinhuanet (in Chinese). Available online at: http://www.xinhuanet.com/2019-12/18/c_1125362460.htm (accessed December 28, 2019).
4. Bogoch II, Watts A, Thomas-Bachli A, Huber C, Kraemer MU, Khan K. Pneumonia of unknown etiology in Wuhan, China: potential for international spread via commercial air travel. J Travel Med. (2020) 27:taaa008. doi: 10.1093/jtm/taaa008
5. Real Time Epidemic Data. Dingxiang doctor (in Chinese). Available online at: https://3g.dxy.cn/newh5/view/pneumonia (accessed July 13, 2020).
6. Wang C, Horby PW, Hayden FG, Gao GF. A novel coronavirus outbreak of global health concern. Lancet. (2020) 395:470-473. doi: 10.1016/S0140-6736(20)30185-9
7. Zhao S, Musa SS, Lin Q, Ran J, Yang G, Wang W, et al. Estimating the unreported number of novel coronavirus (2019-nCoV) cases in china in the first half of january 2020: a data-driven modelling analysis of the early outbreak. J Clin Med. (2020) 9:388. doi: 10.3390/jcm9020388
8. Imai N, Dorigatti I, Cori A, Riley S, Ferguson NM. Report 2: Estimating the Potential Total Number of Novel Coronavirus (2019-nCoV) Cases in Wuhan City, China. Imperial College London. (2020). Available online at: https://spiral.imperial.ac.uk/bitstream/10044/1/77150/12/2020-01-22-COVID19-Report-2.pdf
9. Nishiura H, Kobayashi T, Yang Y, Hayashi K, Miyama T, Kinoshita R, et al. The rate of underascertainment of novel coronavirus (2019-nCoV) infection: estimation using japanese passengers data on evacuation flights. J Clin Med. (2020) 9:419. doi: 10.3390/jcm9020419
10. Wu JT, Leung K, Leung GM. Nowcasting and forecasting the potential domestic and international spread of the 2019-nCoV outbreak originating in Wuhan, China: a modelling study. Lancet. (2020) 395:689–97. doi: 10.1016/S0140-6736(20)30260-9
11. Situation report of the Pneumonia Cases Caused by the Novel Coronavirus. National Health Commission of Each Province of People's Republic of China (in Chinese). Available online at: http://www.nhc.gov.cn/ (accessed 23 January 2020).
12. Du Z, Wang L, Cauchemez S, Xu X, Wang X, Cowling BJ, et al. Risk for transportation of coronavirus disease from wuhan to other cities in China. Emerg Infect Dis. (2020) 26:1049–52. doi: 10.3201/eid2605.200146
13. Wilks SS. The large-sample distribution of the likelihood ratio for testing composite hypotheses. Ann Math Stat. (1938) 9:60–2. doi: 10.1214/aoms/1177732360
14. Akaike H. Information theory and an extension of the maximum likelihood principle. In: Parzen E, Tanabe K, Kitagawa G, editors. Selected Papers of Hirotugu Akaike. New York, NY: Springer New York. (1998). p. 199–213. doi: 10.1007/978-1-4612-1694-0_15
15. Report of Hubei Provincial Health Committee on Pneumonia Caused by New Coronavirus. Health Commission of Hubei Province (in Chinese). Available online at: http://wjw.hubei.gov.cn/fbjd/dtyw/202001/t20200124_2014626.shtml] (accessed January 24, 2020).
16. Ai S, Zhu G, Tian F, Li H, Gao Y, Wu Y, et al. Population movement, city closure and spatial transmission of the 2019-nCoV infection in China. medRxiv. (2020). doi: 10.1101/2020.02.04.20020339. [Epub ahead of print].
17. Jin G, Yu J, Han L, Duan S. The impact of traffic isolation in Wuhan on the spread of 2019-nCov. medRxiv. (2020). doi: 10.1101/2020.02.04.20020438. [Epub ahead of print].
Keywords: COVID-19, mobility, pneumonia, transportation, outbreaks
Citation: Zhuang Z, Cao P, Zhao S, Lou Y, Yang S, Wang W, Yang L and He D (2020) Estimation of Local Novel Coronavirus (COVID-19) Cases in Wuhan, China from Off-Site Reported Cases and Population Flow Data from Different Sources. Front. Phys. 8:336. doi: 10.3389/fphy.2020.00336
Received: 16 May 2020; Accepted: 20 July 2020;
Published: 01 September 2020.
Edited by:
Aristides (Aris) Moustakas, Natural History Museum of Crete, University of Crete, GreeceCopyright © 2020 Zhuang, Cao, Zhao, Lou, Yang, Wang, Yang and He. This is an open-access article distributed under the terms of the Creative Commons Attribution License (CC BY). The use, distribution or reproduction in other forums is permitted, provided the original author(s) and the copyright owner(s) are credited and that the original publication in this journal is cited, in accordance with accepted academic practice. No use, distribution or reproduction is permitted which does not comply with these terms.
*Correspondence: Weiming Wang, d2VpbWluZ3dhbmcyMDAzQDE2My5jb20=; Lin Yang, bC55YW5nQHBvbHl1LmVkdS5oaw==; Daihai He, ZGFpaGFpLmhlQHBvbHl1LmVkdS5oaw==