- 1Positron Emission Tomography Research Centre, University of Hull, Hull, United Kingdom
- 2Department of Biomedical Sciences, University of Hull, Hull, United Kingdom
- 3Medical Physics Department, University Hospital ‘S. Orsola-Malpighi’, Bologna, Italy
- 4Medical Image and Signal Processing – MEDISIP, Ghent University, Gent, Belgium
Background: Increased throughput in small animal preclinical studies using positron emission tomography leads to reduced costs and improved efficiency of experimental design, however the presence of multiple off-centre subjects, as opposed to a single centered one, may affect image quality in several ways.
Methods: We evaluated the count rate performance using a NEMA scatter phantom. A Monte Carlo simulation of the system was validated against this dataset and used to simulate the count rate performance for dual scatter phantoms. NEMA NU4 image quality phantoms were then scanned in the central and offset positions, as well as in the offset position next to a uniform activity phantom. Uniformity, recovery coefficients and spillover ratios were then compared, as were two time frames for acquisition.
Results: Count rate performance assessed with a single NEMA scatter phantom was in line with previous literature, with simulated data in good agreement. Simulation of dual scatter phantoms showed an increase in scatter fraction. For the NEMA Image Quality phantom, uniformity and Recovery coefficients were degraded in the offset, and dual phantom cases, while spillover ratios were increased, notably when the chamber was placed nearest the gantry. Image quality metrics were comparable between the 20- and 10 min timeframes.
Conclusion: Dual animal scanning results in some loss of image quality on the Sedecal Argus PET scanner; however, this degradation is within acceptable limits.
1 Introduction
Preclinical Positron Emission Tomography (PET) allows the longitudinal study of a range of biological processes in disease models, as well as being invaluable in the screening of novel diagnostic/theranostic PET probes. It is increasingly common for preclinical PET centers to scan multiple animals simultaneously in order to increase the cost-efficiency and throughput of studies, especially with the use of short-lived PET isotopes such as [
Scanning multiple, radially displaced mice in the Field Of View (FOV) will increase attenuation and scatter, as well as the complexity of scatter correction. Additionally sensitivity and resolution will be reduced, while an increase in the overall activity in the FOV will increase dead time. As such, image quality is likely to degrade, and it is necessary to assess these effects on individual scanner/bed geometries as these vary considerably [3]. Although ultimately the effect on biological quantitation is of greatest importance, use of the NEMA NU4 image quality (IQ) phantom [4] allows direct comparison between systems.
Several groups have reported setups to achieve multiple animal imaging. Aide et al. [5] reported the use of a Siemens Biograph TrueV clinical PET-CT scanner and showed that tracer quantitation in the central and offset positions was highly correlated, albeit with lower system sensitivity and resolution (and higher injected activities) than commonly found in preclinical systems.
Aide et al. [1] went on to characterize a self-manufactured four-animal bed on the Siemens Inveon, demonstrating an increase in spillover ratios and a decrease in resolution for the NEMA IQ phantom; albeit with a high correlation between PET quantitation and biodistribution using this arrangement. Habte et al. [6] characterized a 3- and 4-bed setup for the R4 (Concorde Microsystems/Siemens) and Inveon (Siemens) scanners and demonstrated comparable in vivo quantitation between single and multiple animal scanning as long as attenuation correction was performed. Yagi et al. [7] reported another self-assembled multiple animal bed for the Inveon scanner, evaluating uniformity in mouse phantoms for four animals and demonstrating a comparable decrease to Habte et al. [6]. Seidel et al. [8] reported a dual mouse bed that enabled ECG-gated imaging to be carried out on the Argus PET-CT scanner but did not characterize effects on image quality.
Siepel et al. [9] studied the effects of scanning 2 and 4 animals, axially or radially displaced, in an Inveon scanner using the NEMA NU4 IQ phantom. They reported a decrease in uniformity, a reduction in Recovery Coefficient (RC) and an increase in Spill-Over ratio (SOR) for the 2 and 4 phantom radially-displaced cases, which could be improved by axial displacement. Rominger et al. [10] characterized an 8-mouse bed in the Inveon scanner, demonstrating that scatter correction and reduced overall activity in the field of view was necessary to achieve optimum quantitation in this setting. Using the PET-SORTEO simulation methodology, Reilhac et al. [11] assessed the signal degradation for dual animal scanning on the Inveon and the impact of this on the detection of biological variation. After demonstrating that their simulation gave comparable results to acquired phantom data, they went on to demonstrate that recovery coefficients and uniformity were decreased, while SOR (in this case peak-to-valley measure) were increased.
Most recently, Greenwood et al. [12] characterized a commercial four-chamber bed on a Mediso preclinical PET scanner, using NEMA phantoms and mice. As in previous studies, they demonstrated a decrease in uniformity and recovery coefficients, and an increase in spillover ratios, in the multiple vs. single animal cases, though these increases were within the recently suggested limits for bias [13].
This study seeks to investigate the effect of dual animal scanning on the Sedecal SuperArgus2R preclinical scanner using the NEMA IQ phantom filled with a range of activities; also to assess the effects on IQ for common static scan frame lengths. Initially, we validate a Monte Carlo (MC) simulation of the scanner geometry and use it to compare the effect on count rate performance between single and dual NEMA scatter phantoms. We then go on to assess IQ metrics on phantoms placed either centrally, offset, or offset alongside a 20 mL syringe containing an equal activity, also comparing spillover ratios where air and water chambers are displaced toward the center of the field of view or the bore.
2 Materials and Methods
2.1 SuperArgus2R Scanner
The Sedecal SuperArgus2R preclinical PET scanner (Figure 1) [14–16] features 18 phoswich detector blocks in an arrangement of 2 rings with diameter 118 mm and axial FOV 48.0 mm. In between the two rings there is gap of 8 mm. Each block has a two layers of
The phoswich detector arrangement provides Depth of Interaction (DOI) information, allowing for more uniform images and better radial spatial resolution [17]. As such, this detector configuration should also reduce spatial resolution degradation when scanning dual objects.
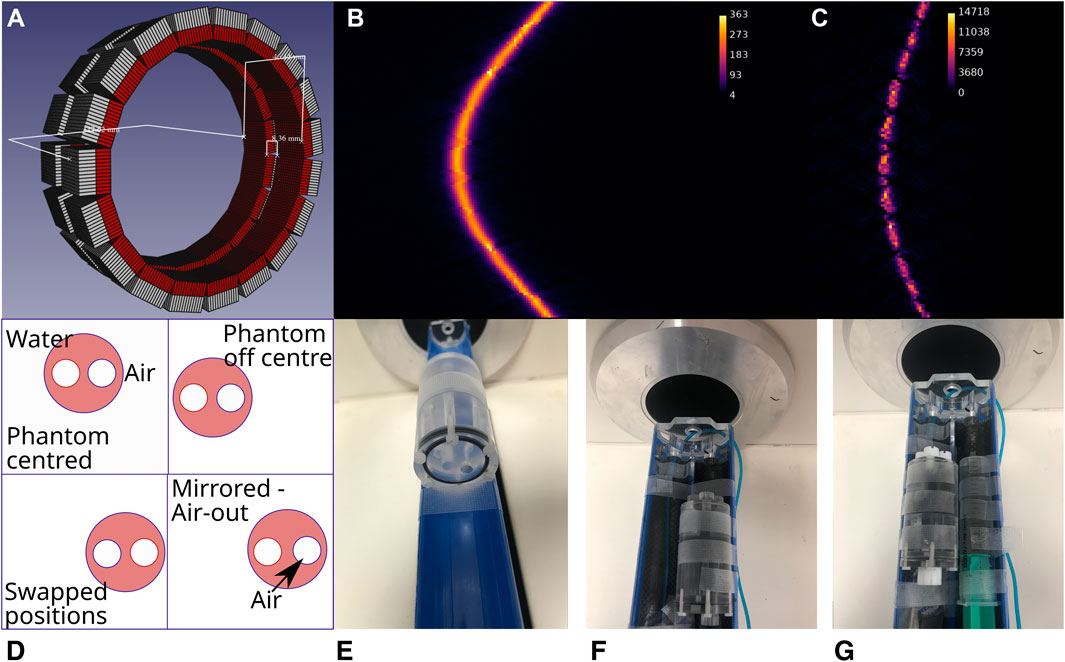
FIGURE 1. (A) Representation of the geometry of the Sedecal SuperArgus 2R detector geometry. The inner layer of crystals (red) are made of LSO:Ce and the outer layer (gray) are GSO:Ce. (B) Sinogram with corrections, generated by Sedecal’s FORE-3D software (size 175 × 128 × 61). (C) Non corrected sinogram created from the .tru file containing only counts (size 117 × 117 × 61), as generated in STIR. The two sinograms are from the axial position 29 of the first acquisition 33 MBq. (D) Illustration of the position of NEMA phantom inside the FOV. The mirror transformation is with respect to the position of the cold containers. (E)–(G) photographs of the phantom in the central, off-centre and dual acquisitions.
2.2 Monte Carlo Simulation Model
Due to the supplied animal bed on the scanner being too small to accommodate two scatter phantoms simultaneously, investigations on a single phantom in an offset bed position and dual phantom scatter fraction were performed in simulation only.
The MC simulation model was developed using the GATE (v.8.1) [18], simulation toolkit. The model was validated over the scanner’s acceptance tests and measurements following the NEMA protocol [19]. The energy window was set to 250–700 keV, the same as the one used in the experiments. The coincidence window was set to 5.0 ns. The emstandard_opt3, physics model, was used without any variance reduction techniques or energy cuts.
2.2.1 Simulations for Count Losses, Scatter Fraction and NECR
A model of the NEMA scatter fraction phantom was simulated in order to compare the counting performance, scatter fraction and Noise Equivalent Count Rate (NECR) for different placements in the FOV, using the validated GATE model. The model phantom matched the manufactured phantom that was used for the validation of the simulation model; a polyethylene cylindrical phantom 70 mm long and 25 mm in diameter (for details see Section 2.3).
The phantom was simulated solo in the center, offset (±19.0 mm on the x axis, as defined in GATE) positions of the FOV [20, 21] as well as in combination with a second phantom. The model of the scanner included all parts of the physical scanner (plastic tubes, shielding etc).
2.3 NEMA Phantom Studies
All acquisitions were made using [18F] produced using an ABT mini-cylotron (ABT, United States).
2.3.1 Assessment of Scatter Fraction, Count Losses, Random Coincidences and NECR
The system was evaluated according to Section 4 of the NEMA NU4-2008 standard [4]. A polyethylene cylindrical phantom 70 mm long and 25 mm in diameter was manufactured and a 3.2 mm hole was drilled 10 mm off center to fit a capillary tube made of Borosilicate glass (World Precision Instruments, 1B200-4). The capillaries had an outer diameter 2.0 mm and inner 1.12 mm.
Phantom data were acquired with a starting activity of approximately 35 MBq of [18F]. A series of 10 min scans followed by a 15 min of rest were acquired, lasting in total for more than 3 half-lives (6 h), using the 250–700 keV energy window.
2.3.2 Raw Data Processing
The NEMA NU4-2008 [4] protocol requires that uncorrected sinograms holding only number of coincidences are processed. However, direct extraction of such data from the scanner was not possible. By default, the scanner saves the recorded coincidences (after application of the energy window) in the proprietary .tru files which essentially are histograms holding counts per detector combination with detector pair coordinates. The scanner’s software has the option to extract pre-corrected (for dead time and decay) FORE-3D sinograms [22] (Figure 1B), typically for Filtered Back-Projection reconstruction, which are not suitable for the execution of the NEMA protocol.
We addressed the issue by appropriately modifying the IO component of the STIR image reconstruction library [23] to read the .tru files and export projection data. Besides the new input function, the issue of the multiple detector layers, which are not currently supported by STIR, was addressed by means of simple geometrical translations in order to assign the events on a single cylindrical model.
Finally, as suggested in the NEMA protocol, Single Slice Rebinning was performed on the projection data using the tool provided in STIR (Figure 1A). The sum of all bins in the sinograms we generated was equal to the sum of events in the .tru file.
2.3.3 Assessment of Image Quality
A NEMA NU4-2008 image quality phantom [4] containing 2.5 or 10 MBq of 18F (representing low and high activity acquisitions in mice respectively) was scanned at the center and offset positions and alongside a (20 mL) syringe filled with the same activity as illustrated in (Figure 1B). The activity was within
Images were converted into DICOM format and loaded into a Mediso image anaylsis software (Mediso NEMA Tests, NEMA IQ) which automatically recognized the NEMA IQ phantom geometry and generated appropriate ROI’s for uniformity (%Standard Deviation (%STD)), Spill-Over ratio (SOR) and Recovery Coefficient (RC). Generated ROIs were manually positioned over the respective regions of the phantom, according to the protocol’s instructions, to return mean values and the percentage standard deviation.
In addition we sought to investigate whether the above figures of merit were consistent across the FOV. To assess this, we made acquisitions on single NEMA IQ phantoms positioned off-centre on the left- and right-hand side of the bore. For SOR assessments we also performed left- and right-sided offset acquisitions with the air chamber facing either toward or away from the scanner bore, in order to investigate any impact made through the inherent bias of the NEMA IQ phantom geometry. We also investigated the impact on dual phantom scanning when the NEMA IQ phantom is positioned on the left or right imaging bed.
Finally, we performed dynamic acquisitions at 10- and 20 min frames on centrally positioned NEMA IQ phantoms and dual offset positioned phantoms in order to assess the impact of reduced scan time on the same image quality metrics.
2.3.4 Image Reconstruction
PET image reconstruction was performed using Ordered Subsets-Expectation Maximization (OSEM) 2D, with 16 subsets after 2 full iterations. The 2D sinogram was generated using 3D Fourier Rebinning (FORE-3D) on the stored data files provided by the manufacturer. The stored sinograms were corrected for linearity and dead-time . In order to reduce the amount of blurring, the maximum ring difference was set to 16 rings and a span 3; the default settings recommended by the manufacturer. No post-filtering was applied in the reconstructed images.
The reconstruction was performed with all available corrections applied (randoms, scatter and attenuation) and included normalization. The reconstructed images had
3 Results
3.1 Validation of the Simulation Model
The count rates of prompt, true, random, scattered events and the NECR of the measured phantom acquisition are presented in Figure 2A as a function of activity in the FOV, and summarized in Table 1. At a starting activity of 10 MBq, reflecting a suggested maximal injected dose for a mouse, the count loses are approximately
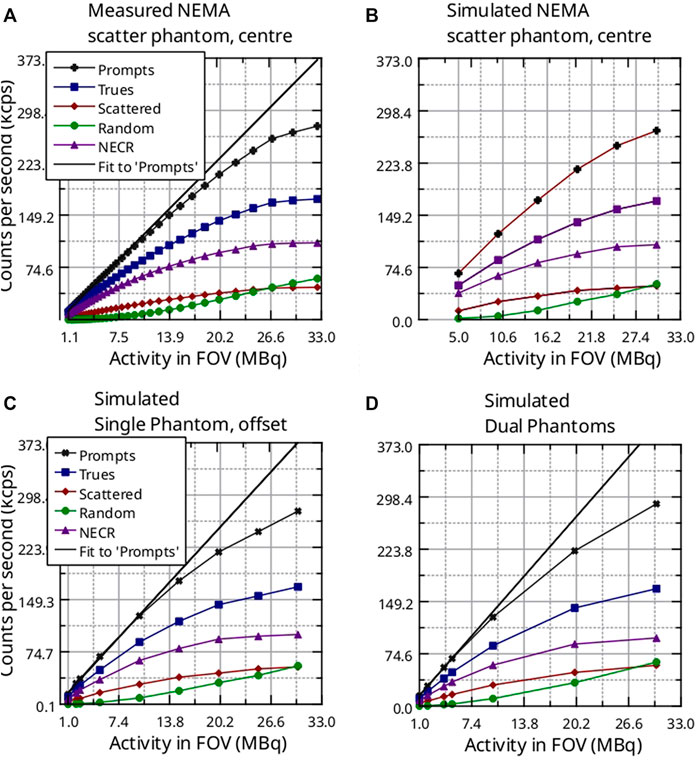
FIGURE 2. Prompt, Trues, Random Scatter rates and NECR for the NEMA scatter phantom for (A) Experimental measurements at the central bed position. (B) Simulated central bed position. (C) Simulated off center, and (D) dual phantom scanning. The linear fit to the Prompts was performed for the first five points.
Furthermore, the scatter rate steadily increases in the measured centrally positioned acquisition until around 25 MBq is reached, peaking at 45.6 kcps (Figure 2A). This closely correlates to the simulated counterpart where the scatter rate peaks at similar activity levels. The simulated data, however, show that
3.2 Simulation of Dual Scatter Phantoms
Simulations of the single NEMA scatter phantom in the offset bed position shows count losses of
3.3 NEMA NU4 Image Quality Phantom
3.3.1 Static Phantom Acquisition
3.3.1.1 Uniformity
The uniformity, (%STD) at the center of the FOV, offset position and dual scanning, for initial activities 2.5- and 10 MBq, is presented in Figure 3A. In Figure 3J, we present the corresponding CT images.
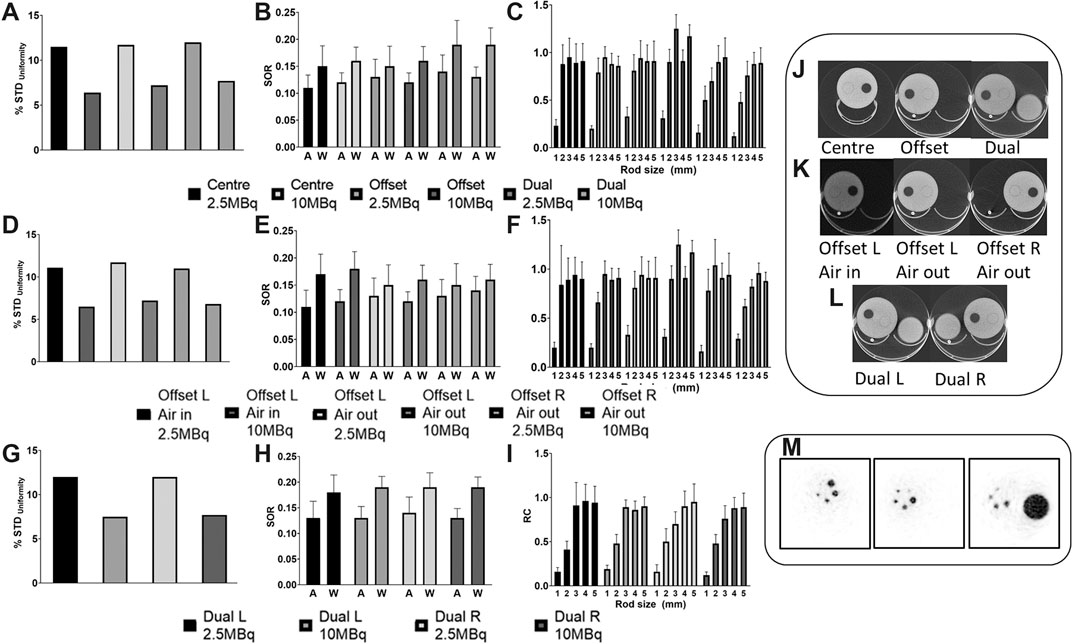
FIGURE 3. Assessment of NEMA NU4 Image Quality for the single centered, single offset and dual phantom cases. (A–C) Uniformity, SOR and Recovery Coefficients for the centered, offset and dual phantom positions indicated in (J). (D–F) Uniformity, SOR and Recovery Coefficients for the single left or right offset position with the air chamber facing the center or edge of the field of view as indicated in (K). (G–I) Uniformity, SOR and Recovery Coefficients for the dual phantom configuration, with the NEMA IQ phantom in the left or right position as indicated in (L). (M) Variable orientation of rods in NEMA NU4 phantom. Error bars: standard deviation.
For both low (2.5 MBq) and high (10 MBq) acquisitions, the %STD increased when the phantom was placed at the offset position or when dual phantoms were scanned, compared to the single centered phantom case. Overall, %STD was higher for the low activity acquisitions (11–12%) vs those of higher activity (6–8%). Dual phantom acquisition returns
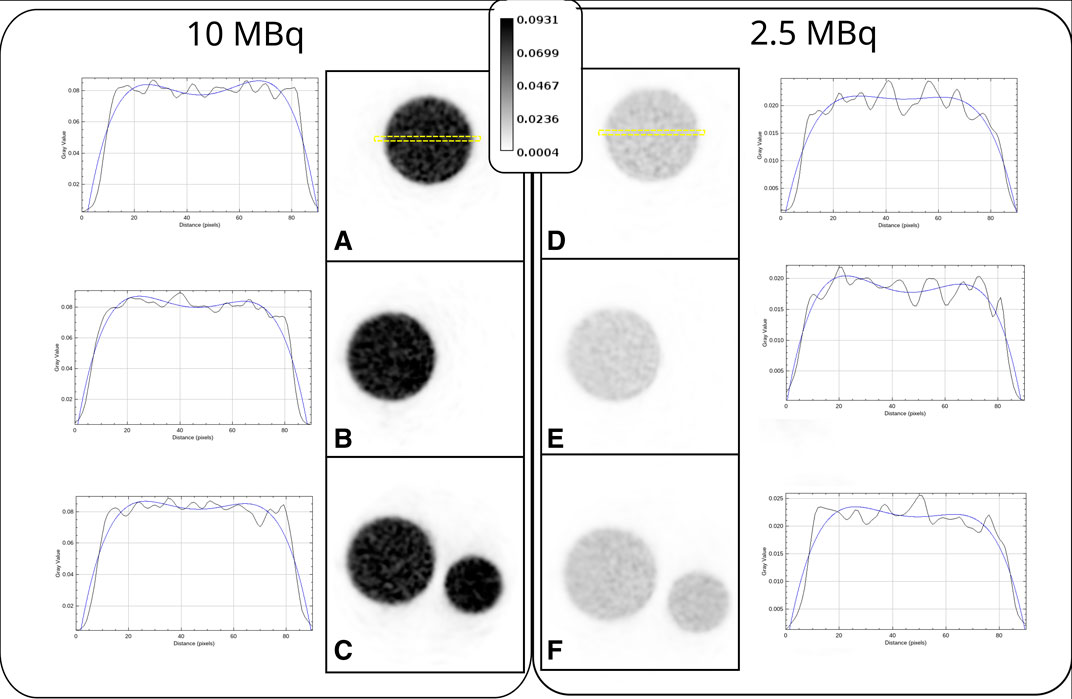
FIGURE 4. Assessment of uniform region asymmetry for the NEMA IQ phantom in the single centered (A, D), offset (B, E) and dual (C, E) cases. Yellow lines indicate selected region. Adjacent graphs indicate line profiles fitted with a polynomial function.
Phantom positioning on the left- or right-hand side of the scanner had little impact on uniformity. Similarly, placement of the air chamber facing inwards or outwards had little effect (Figure 3D, corresponding images Figure 3K).
Likewise, uniformity is comparable between dual phantom acquisitions with the NEMA IQ phantom placed on the left or right side (Figure 3G).
Doubling the scan time from 10 to 20 min reduces %STD by 38.1 and 29.5% in single centered phantom acquisition for 2.5- and 10 MBq, respectively (Figures 5A,D). Increasing the scanning time from 10- to 20 min for dual phantom scanning reduces the %STD of the uniformity by 34.4 and 26.0% for 2.5- and 10 MBq, respectively.
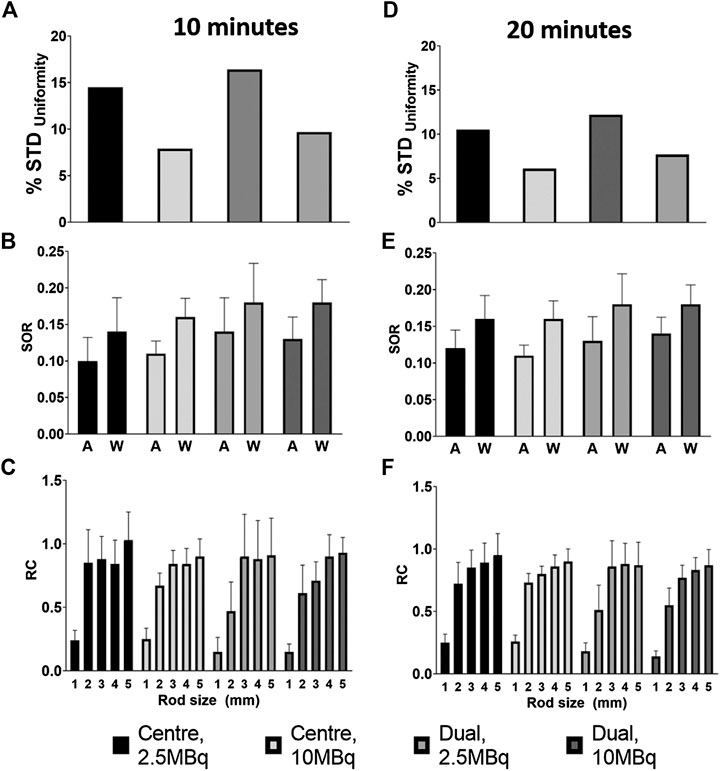
FIGURE 5. Assessment of NEMA NU4 Image Quality for the 10 and 20 min summed frames for single centered and dual phantom cases. (A–C) Uniformity, SOR and Recovery Coefficients for the centered and dual phantom positions, 10 min summed frame. (D–F) Uniformity, SOR and Recovery Coefficients for the single or dual phantom positions, 20 min summed frame. Error bars = standard deviation.
Adding a second activity source, as is the case for dual phantom scanning, increases the %STD 13.1 and 16.2% compared a single centered phantom at 2.5 MBq for both 10- and 20 min acquisition times, respectively. In the case of 10 MBq acquisitions, dual phantom scanning increases the %STD uniformity by 22.8 and 26.2% for 10- and 20 min acquisitions, respectively.
3.3.1.2 Recovery Coefficients
The mean values and %STD for the different phantom positions are presented in Figure 3C. The central slices of the rods taken from the 10 MBq acquisitions are shown in Figure 3M.
With the exception of the 10 MBq offset scan, acquisitions performed on phantoms positioned both centrally and offset return comparable data on recovery coefficients. In the case of the 10 MBq offset data, both the 3- and 5 mm diameter rods return recovery coefficients greater than 1. On the other hand, both offset acquisitions performed at 2.5- and 10 MBq show a more favourable recovery coefficient for the 1 mm compared to the centrally positioned scans. Finally, the dual phantom acquisitions show progressively poorer RCs with reducing rod size, with a reduction of 60 and 24% for the 2- and 1 mm rods, respectively, compared to the single offset acquisitions.
Recovery Coefficients remained generally consistent when the offset phantom was positioned on the left and right side of the scanner FOV (Figure 3F). Left side, air chamber facing outwards continued to show RC greater than 1.0 for 3- and 5 mm rods at 10 MBq, as did the 3 mm rod on the right side phantom, air chamber facing outwards at 2.5 MBq, but to a smaller extent. Interestingly, left side, air chamber facing inwards show a normal expected range of RC’s at 10 MBq, albeit with a comparatively reduced 2 mm RC. No particular observations could be made between 2.5- and 10 MBq acquisitions, except that the 2 mm RC appeared to be poorer at 10 MBq, excluding the aforementioned left side, air out acquisitions. However, these observations could be equally explained by the variability in the exact positioning of the rods.
When the NEMA IQ phantom is positioned at the right-hand side for dual phantom scanning, the 3 mm rod’s RC is poorer at both 2.5- and 10 MBq (Figure 3I). No other noteworthy difference can be seen between the RCs of the dual phantom acquisitions when the NEMA IQ phantom is position at either the left- or right-hand side.
Increasing the scan time from 10- to 20 min makes little difference to the overall mean values of the RC, with none of the mean values changing greater than
3.3.1.3 Spill-Over Ratio
The spill-over ratios for the air and water chambers in the NEMA IQ phantoms positioned centrally, offset and offset in combination with a second phantom are presented in Figure 3B. At central positions, the SOR in the water chamber is
4 Discussion
4.1 Simulation of Multiple Phantoms
Both the measured and simulated prompt counts are in good agreement with Kehl et al. [24] and Zagni et al. [19] however, the latter study reports a marginally higher NECR. We suggest that this difference is due to the data processing method, as our results are produced by processing the raw sinograms, as suggested by the NEMA protocol. Other groups have reported the scanners’ counting performance [14, 25], however those studies used non-standard phantoms, making direct comparison difficult.
The offset and dual phantom simulations do not result in a marked increase in randoms and NECR when compared to the single centred phantom for both 10 to 20 MBq (Figure 2). However, in the simulated studies the scatter fraction increases for both the off-centre and dual phantom compared to the single phantom case at both activity levels (Figure 2; Table 2). Scatter fractions for the single phantom (
It has previously been proposed that the activity in the FOV at the time of acquisition should be approximately
The image quality strongly depends on the many parameters of the reconstruction algorithm [29]. An increase in starting activity would allow for shorter imaging times, however this may result in an increase in absorbed dose to the animal above the threshold for biological effects [30], an issue for longitudinal studies, although our reported modest increase in random events associated with higher activities suggests that imaging with higher activities would have a relatively small impact to image quality.
4.2 Uniformity
Our results indicate a higher level of noise for the single phantom case than previously reported [3]. However, this data was acquired using a single bed position and with a different activity (3.7 vs. 2.5 MBq). It has been previously reported that multiple bed positions increase variability [31].
When more than one or off-centre sources of activity are present in the scanner, our findings suggest that the Sedecal Super Argus 2R scanner performs favourably compared to similar studies used other scanners. Siepel et al. [9] reported an
4.3 Recovery Coefficients
Recovery coefficients generally decreased for the dual case, as reported previously. The variation that is sometimes seen in RCs (e.g., the 10 MBq offset case) may be explained by the placement of the 1 mm rod being geometrically closer to the center of the FOV in case of the offset acquisitions as the exact position of rod geometry was not factored in our acquisition protocol (see Figure 3M).
Similar behavior has been observed and reported on by Aide et al. [32] and Siepel et al. [9], who attributed this to the combined effect of ‘over-estimation of scatter correction plus the non-negativity constraint in the image reconstruction’. Furthermore, Greenwood et al. [12] showed that the smaller, 1 mm diameter rod was not discernible when scanning four phantoms simultaneously, which stands by our observations that RC diminishes as more sources of activity are introduced into the FOV.
It should also be noted that the NEMA protocol derives the
The phoswich detector arrangement inherently provides DOI information, allowing for an improved and more uniform spatial resolution in the images [17]. This detector configuration should naturally reduce the spatial resolution degradation when scanning dual objects, allowing for reconstruction methods without Point Spread Function (PSF) correction to perform well under these conditions. This is in contrast to the reported performance of OSEM 2D reconstruction for the Inveon [9, 32].
Our data shows that RC values do not significantly change between 10- and 20 min imaging frames. However, as expected, the %STD of the RCs is markedly higher on the shorter imaging time frames regardless of activity or scan orientation.
4.4 Spillover Ratios
Our data indicated a dependence of SOR on gantry proximity. The apparent increase on the water container in the offset case could be a result either of limitations of the scatter correction algorithm, or by photons scattered by the Delrin tube, in close proximity at the edge of the gantry.
When scanning two subjects, the SOR increases by 17 and 12% for water and air, respectively. Siepel et al. [9] reported an approx.
No notable difference was observed in SOR between 10 and 20 min frames at both high and low activities, and between single centered and dual phantom scanning.
As Greenwood et al. [12] demonstrated, increasing the number of iterations during reconstruction may improve the SOR values. However, this may come at the expense of noise amplification, as shown by Gaitanis et al. [34]. The above, has not been assessed here.
When comparing simulated phantom data to NEMA IQ acquisition, it is worth noting that the SuperArgus2R has an axial FOV smaller than that of the NEMA phantom (47 vs. 50 mm), and thus NEMA IQ acquisitions used multiple bed positions. However, data on scatter fraction and NECR were acquired using effectively a single bed position as the NEMA protocol does not specify a similar setting for this case.
Image quality is also dependent on energy discrimination, and our studies used the 250–700 keV setting as used in other studies [3] and suggested to provide a good balance between counting performance and scatter rejection [35].
The primary application of preclinical PET at the University of Hull PET Research center is to assess the pharmacokinetics and biodistribution of novel imaging probes [36–39], for this application the degradation in image quality predicted for the two animal case is acceptable. For the application of existing tracers to provide physiological readouts in disease models, our data suggest that higher injected doses provide better image quality overall if dual animal scanning is warranted for logistical reasons [40].
5 Conclusion
In this study, we present the impact of dual subject scanning on the PET signal and image quality using the Sedecal SuperArgus 2R preclinical scanner. In brief, our experiments demonstrate a decrease in image quality between the single and dual phantom cases, however of less magnitude than similar decreases reported for other scanners.
We conclude that the Sedecal AuperArgus 2R preclinical scanner is, therefore, suitable for dual animal scanning, particularly in biodistribution studies of novel radiotracers - as is a key operational objective of our facility. Regarding investigations looking into more subtle biological changes or smaller regions of interest, the scanning protocol and injected dose should be carefully considered to ensure that image quality is optimized throughout the field of view.
Data Availability Statement
The datasets generated for this study are available upon reasonable request to the corresponding authors.
Author Contributions
NE and JW contributed equally to this work and share first authorship. LC performed the scans of the NEMA scatter phantom for the characterisation of the scanner. NE and LC processed the raw data acquired from the scanner, and did the image analysis for the image quality phantom. NE and PC performed the GATE simulations, validated the simulations to the measured data, and performed the Monte Carlo investigation. JW and IR performed the acquisition and analysis of the NEMA IQ phantom. FZ developed the original GATE Monte Carlo simulation model for the Argus scanner and advised in the processing of the data acquired from the scanner. CC contributed to the conception and design of the study. CC and SA are the grant holders and were involved in the organization and planning of the work and discussions on the results. All authors contributed in the drafting of the paper.
Conflict of Interest
The authors declare that the research was conducted in the absence of any commercial or financial relationships that could be construed as a potential conflict of interest.
Acknowledgments
We thank Assem Allam and his family for the generous donation to help found the PET Research Center at the University of Hull and for their continued support. We acknowledge the Viper High Performance Computing facility of the University of Hull and its support team. The authors would like to thank D. P. Roberts (PET Research Center, Univ. of Hull) for his valuable support and training.
Supplementary Material
The Supplementary Material for this article can be found online at: https://www.frontiersin.org/articles/10.3389/fphy.2020.531662/full#supplementary-material.
References
1. Aide N, Visser EP, Lheureux S, Heutte N, Szanda I, Hicks RJ. The motivations and methodology for high-throughput pet imaging of small animals in cancer research. Eur J Nucl Med Mol Imag (2012) 39:1497–509. doi:10.1007/s00259-012-2177-x
2. Eckelman WC. Further discussions on choosing the number of animals for an experiment. Nucl Med Biol (2008) 35:1–2. doi:10.1016/j.nucmedbio.2007.10.002
3. Goertzen AL, Bao QN, Bergeron M, Blankemeyer E, Blinder S, Canadas M, et al. Nema nu 4-2008 comparison of preclinical pet imaging systems. J Nucl Med (2012) 53:1300–9. doi:10.2967/jnumed.111.099382
4. Weber S, Bruyndonckx P, Chatziioannou AF, Clark JC, Daube-Witherspoon ME, Di Domenico G, et al. Performance measurements of small animal positron emission tomographs. J Nucl Med (2002) 43:59p–59p.
5. Aide N, Desmonts C, Beauregard JM, Beyer T, Kinross K, Roselt P, et al. High throughput static and dynamic small animal imaging using clinical pet/ct: potential preclinical applications. Eur J Nucl Med Mol Imag (2010a) 37:991–1001. doi:10.1007/s00259-009-1352-1
6. Habte F, Ren G, Doyle TC, Liu HG, Cheng Z, Paik DS. Impact of a multiple mice holder on quantitation of high-throughput micropet imaging with and without ct attenuation correction. Mol Imag Biol (2013) 15:569–75. doi:10.1007/s11307-012-0602-y
7. Yagi M, Arentsen L, Shanley RM, Hui SK. High-throughput multiple-mouse imaging with micro-pet/ct for whole-skeleton assessment. Phys Med-Eur J Med Phys (2014) 30:849–53. doi:10.1016/j.ejmp.2014.06.003
8. Seidel J, Bernardo ML, Wong KJ, Xu BY, Williams MR, Kuo F, et al. Simultaneous ecg-gated pet imaging of multiple mice. Nucl Med Biol (2014) 41:582–6. doi:10.1016/j.nucmedbio.2014.03.015
9. Siepel FJ, van Lier Mgjtb , Chen M, Disselhorst JA, Meeuwis APW, Oyen WJG, et al. Scanning multiple mice in a small-animal pet scanner: influence on image quality. Nucl Instrum I& Methods Phys Res Sect A Accel Spectrom Detect Assoc Equip (2010) 621:605–10. doi:10.1016/j.nima.2010.05.057
10. Rominger A, Mille E, Zhang S, Boning G, Forster S, Nowak S, et al. Validation of the octamouse for simultaneous 18f-fallypride small-animal pet recordings from 8 mice. J Nucl Med (2010) 51:1576–83. doi:10.2967/jnumed.110.078451
11. Reilhac A, Boisson F, Wimberley C, Parmar A, Zahra D, Hamze H, et al. Simultaneous scanning of two mice in a small-animal pet scanner: a simulation-based assessment of the signal degradation. Phys Med Biol (2016) 61:1371–88. doi:10.1088/0031-9155/61/3/1371
12. Greenwood HE, Nyitrai Z, Mocsai G, Hobor S, Witney TH. High-throughput pet/ct imaging using a multiple-mouse imaging system. J Nucl Med (2020) 61:292–7. doi:10.2967/jnumed.119.228692
13. McDougald W, Vanhove C, Lehnert A, Lewellen B, Wright J, Mingarelli M, et al. Standardization of preclinical pet/ct imaging to improve quantitative accuracy, precision, and reproducibility: a multicenter study. J Nucl Med (2020) 61:461–8. doi:10.2967/jnumed.119.231308
14. Wang YC, Seidel J, Tsui BMW, Vaquero JJ, Pomper MG. Performance evaluation of the ge healthcare explore vista dual-ring small-animal pet scanner. J Nucl Med (2006) 47:1891–900.
15. Green MV, Ostrow HG, Seidel J, Pomper MG. Experimental evaluation of depth-of-interaction correction in a small-animal positron emission tomography scanner. Mol Imag (2010) 9:311–8. doi:10.2310/7290.2010.00038
16. Kastis GA, Gaitanis A, Fernandez Y, Kontaxakis G, Fokas AS. Evaluation of a spline reconstruction technique: comparison with FBP, MLEM and OSEM. Hawaii: IEEE Nucl Sci Symp Conf Rec (2007) 3187:3282–7. doi:10.1109/nssmic.2010.5874412
17. Seidel J, Vaquero JJ, Siegel S, Gandler WR, Green MV. Depth identification accuracy of a three layer phoswich pet detector module. IEEE Trans Nucl Sci 46 (1999) 485–90. doi:10.1109/23.775567
18. Jan S, Comtat C, Strul D, Santin G, Trebossen R. Monte Carlo simulation for the ecat exact hr+ system using gate. IEEE Nucl Sci Symp Conf Rec (2003) 1–5: 2545–8. doi:10.1109/Nssmic.2003.1352409
19. Zagni F, D’Ambrosio D, Spinelli AE, Cicoria G, Fanti S, Marengo M. Accurate modeling of a doi capable small animal pet scanner using gate. Appl Radiat Isot (2013) 75:105–14. doi:10.1016/j.apradiso.2013.02.003
20. Efthimiou N, Panayiotakis GS, Varvarigou A, Bouziotis P, Loudos G, Kandarakis I, et al. Small animal positron emission tomography using a dual head prototype based on pspmts and fpga readout. IEEE Int Worksh Imaging Syst Techniq (2008) 12:29–33. doi:10.1109/IST.2008.4659935
21. Efthimiou N, Maistros S, Tripolitis X, Samartzis A, Loudos G, Panayiotakis G. Tomographic evaluation of a dual-head positron emission tomography system. Meas Sci Technol (2011) 22:19. doi:10.1088/0957-0233/22/11/114010
22. Daube-Witherspoon ME, Muehllehner G. Treatment of axial data in three-dimensional PET. J Nucl Med: Off Pub Soc Nucl Med (1987) 28:1717–24.
23. Thielemans K, Tsoumpas C, Mustafovic S, Beisel T, Aguiar P, Dikaios N, et al. STIR: Software for tomographic image reconstruction release 2. Phys Med Biol (2012) 57:867–83. doi:10.1088/0031-9155/57/4/867
24. Kehl MI, Honer M, Schubiger PA, Ametamey SM. Small animal pet imaging: is strict standardization the key to more robust data? Eur J Nucl Med Mol Imag (2010) 37:S388.
25. Yang CC, Seidel J, Wang Y, Lee JS, Pomper MG, Tsui BMW. Validation of gate Monte Carlo simulation of the performance characteristics of a ge explore vista small animal pet system. IEEE Nucl Sci Symp Conf Rec (2007) 3187:1–11. doi:10.1109/Nssmic.2007.4436803
26. Goertzen AL. Coincidences originating from a single photon: an unrecognized and potentially significant source of scatter in small animal pet? 2009 IEEE Nucl Sci Symp Conf Rec 1–5 (2009) 2888–91. doi:10.1109/Nssmic.2009.5401622
27. Watson CC, Casey ME, Bendriem B, Carney JP, Townsend DW, Eberl S, et al. Optimizing injected dose in clinical pet by accurately modeling the counting-rate response functions specific to individual patient scans. J Nucl Med (2005) 46:1825–34.
28. Chang T, Chang G, Clark JJ. Reliability of predicting image signal-to-noise ratio using noise equivalent count rate in pet imaging. Med Phys (2012) 39:5891–900. doi:10.1118/1.4750053
29. Karakatsanis NA, Fokou E, Tsoumpas C. Dosage optimization in positron emission tomography: state-of-the-art methods and future prospects. American journal of nuclear medicine and molecular imaging (2015) 5:527–47.
30. Taschereau R, Chatziioannou AF. Monte Carlo simulations of absorbed dose in a mouse phantom from 18-fluorine compounds. Med Phys (2007) 34:1026–36. doi:10.1118/1.2558115
31. Rahmim A, Lodge M, Karakatsanis N, Vladimir Y, Panin Y, Zhou Y, et al. Dynamic whole-body pet imaging: principles, potentials and applications. Eur J Nucl Med Mol Imag (2019) 46:501–18. doi:10.1007/s00259-018-4153-6
32. Aide N, Desmonts C, Briand M, Meryet-Figuiere M, Poulain L. High-throughput small animal pet imaging in cancer research: evaluation of the capability of the inveon scanner to image four mice simultaneously. Nucl Med Commun (2010b) 31:851–8. doi:10.1097/MNM.0b013e32833dc61d
33. Hallen P, Schug D, Schulz V. Comments on the nema nu 4-2008 standard on performance measurement of small animal positron emission tomographs. EJNMMI Phys (2020) 7:27–35. doi:10.1186/s40658-020-0279-2
34. Gaitanis A, Kontaxakis G, Spyrou G, Panayiotakis G, Tzanakos G. Pet image reconstruction: a stopping rule for the mlem algorithm based on properties of the updating coefficients. Comput Med Imag Graph (2010) 34:131–41. doi:10.1016/j.compmedimag.2009.07.006
35. Kuntner C, Stout D. Quantitative preclinical pet imaging: opportunities and challenges. Front Phys (2014) 2:13–9. doi:10.3389/fphy.2014.00012
36. Burke BP, Baghdadi N, Kownacka AE, Nigam S, Clemente GS, Al-Yassiry MM, et al. Chelator free gallium-68 radiolabelling of silica coated iron oxide nanorods via surface interactions. Nanoscale (2015) 7:14889–96. doi:10.1039/c5nr02753e
37. Entract GM, Bryden F, Domarkas J, Savoie H, Allott L, Archibald SJ, et al. Development of pdt/pet theranostics: synthesis and biological evaluation of an (18)f-radiolabeled water-soluble porphyrin. Mol Pharm (2015) 12:4414–23. doi:10.1021/acs.molpharmaceut.5b00606
38. Burke BP, Miranda CS, Lee RE, Renard I, Nigam S, Clemente GS, et al. (64)cu pet imaging of the cxcr4 chemokine receptor using a cross-bridged cyclam bis-tetraazamacrocyclic antagonist. J Nucl Med (2020) 61:123–8. doi:10.2967/jnumed.118.218008
39. Allott L, Miranda C, Hayes A, Raynaud F, Cawthorne C, Smith G. Synthesis of a benzoxazinthione derivative of tanaproget and pharmacological evaluation for pet imaging of pr expression. EJNMMI Radiopharm Chem (2019) 4:1. doi:10.1186/s41181-018-0054-z
Keywords: preclinical PET CT, dual phantom scanning, multiple animal scanning, Monte Carlo simulation, image quality, NEMA NU4, Sedecal Argus 2R
Citation: Efthimiou N, Wright JD, Clayton L, Renard I, Zagni F, Caribé PR, Archibald SJ and Cawthorne CJ (2021) Influence of Multiple Animal Scanning on Image Quality for the Sedecal SuperArgus2R Preclinical PET Scanner. Front. Phys. 8:531662. doi: 10.3389/fphy.2020.531662
Received: 31 January 2020; Accepted: 26 November 2020;
Published: 12 January 2021.
Edited by:
Ivo Rausch, Medical University of Vienna, AustriaReviewed by:
Daniele De Paula Faria, University of São Paulo, BrazilPeter Homolka, Medical University of Vienna, Austria
Copyright © 2021 Efthimiou, Wright, Clayton, Renard, Zagni, Caribé, Archibald and Cawthorne. This is an open-access article distributed under the terms of the Creative Commons Attribution License (CC BY). The use, distribution or reproduction in other forums is permitted, provided the original author(s) and the copyright owner(s) are credited and that the original publication in this journal is cited, in accordance with accepted academic practice. No use, distribution or reproduction is permitted which does not comply with these terms.
*Correspondence: Christopher J. Cawthorne, Y2hyaXN0b3BoZXIuY2F3dGhvcm5lQGt1bGV1dmVuLmJl; Nikos Efthimiou, bmlrb3MuZWZ0aGltaW91QGllZWUub3Jn