- 1Departamento de Matemáticas, Universidad Autónoma Metropolitana-Iztapalapa, Avenida San Rafael Atlixco No.186 Colonia Vicentina, Delegación Iztapalapa, México City, México
- 2Departamento de Física, Universidad Autónoma Metropolitana-Iztapalapa, Avenida San Rafael Atlixco No.186 Colonia Vicentina, Delegación Iztapalapa, México City, México
- 3Unidad Multidiciplinaria de Docencia e Investigación-Juriquilla, Facultad de Ciencias, Universidad Nacional Autónoma de México (UNAM), Boulevard Juriquilla, Querétaro, México
We present a molecular dynamics and theoretical study on the diffusion of interacting particles embedded on the surface of a sphere. By proposing five different interaction potentials among particles, we perform molecular dynamics simulations and calculate the mean square displacement (MSD) of tracer particles under a crowded regime of high surface density. Results for all the potentials show four different behaviors passing from ballistic and transitory at very short times, to sub-diffusive and saturation behaviors at intermediary and long times. Making use of irreversible thermodynamics theory, we also model the last two stages showing that the crowding induces a sub-diffusion process similar to that caused by particles trapped in cages, and that the saturation of the MSD is due to the existence of an entropic potential that limits the number of accessible states to the particles. By discussing the convenience of projecting the motions of the particles over a plane of observation, consistent with experimental capabilities, we compare the predictions of our theoretical model with the simulations showing that these stages are remarkably well described in qualitative and quantitative terms.
1 Introduction
In several physical and biological systems the mass transport phenomena is carried out in surfaces with non vanishing curvatures. The diffusion of bio-molecules and other particles on the surface of liposomes, drops and other curved entities is of high relevance due to its potential applications in biomedicine and technology [1–4]. Characteristic examples of this phenomena can be found in the diffusion of proteins on curved membranes or in the surface diffusion of molecules and chemical solvents in catalytic surfaces [5–8].
In these curved domains, many geometric aspects relating the motion of the particles on surfaces have been studied in recent years [9–14]. These studies are relevant in the understanding of applied problems such as nucleation, spinodal decomposition, adsorption, and phase transitions, since the dynamics of the particles is modified due to the curvature of the surface on which the molecules are embedded [15–18].
In particular, the most relevant quantity to measure in these particle motions is the mean square displacement (MSD). However, most of the previously refereed studies provide geometric information of the MSD and the effective diffusion coefficient only for the case where the interaction of the particles occurs with the surface and not among particles themselves. In this simplification, many expressions can be found in the literature for approximating the short and long time behavior of the MSD, see Ref. [9] and references therein. However, for the case of interacting particles, there are only few numerical works studying the influence of interaction and confinement in the MSD [19–21].
In this work we will consider how the surface diffusion displacements are influenced by the interaction of the particles and the projection of their displacement in a plane of observation. This is because for most experimental cases of interest, the geodesic trajectories of the particles cannot be followed at all times, and only the projection in a plane of observation can be measured [11, 22, 23]. This fact emphasizes that for diffusion over closed surfaces, the observed motility depends on the curvature of the surface and on the plane of observation [24–26].
We present the results of the MSD for interacting particles in a crowded spherical surface by using Molecular Dynamics (MD) simulations and implementing five different pairwise-interaction potentials between the particles in a relatively high surface density medium (
With the aim to provide a physical interpretation of the results of these numerical calculations, we formulate an analytic model based on the generalized Smoluchowski equation with time-dependent coefficients [29–34]. Using this tool, we can identify how the change of perspective, confinement and crowding are incorporated in an effective diffusion coefficient that takes into account the entropic confinement and the anomalous diffusion. The model used here was derived in the context of mesoscopic non-equilibrium thermodynamics (MNET) and has been widely used in order to obtain kinetic equations for transport phenomena, like diffusion-adsorption processes, anomalous diffusion, activated processes, diffusion in pores, and diffusion in the presence of entropic barriers [35–39]. In particular for our purposes, MNET has been successful in describing diffusion on other confined systems [38, 40–42].
The comparison between our numerical simulations and thermodynamic-based model allows us to propose a new interpretation of the observed dynamics of the MSD for diffusion of interacting particles over a sphere, and to compare the different subdiffusive regimes associated with the hardness or softness of the particle collisions. We have chosen a spherical geometry in order to rule out the effects of different local curvatures in the surface. This will also allow us to recover previous theoretical results deduced for the sphere in absence of interaction.
Regarding the organization of work, in Section 2 we present our Molecular Dynamics simulations on the diffusion of finite sized-particles over an spherical surface for different interaction potentials among particles. Then, we study the dynamics of the diffusion and the behavior of the MSD using the results provided by a Smoluchowski description which is presented in Sections 3.1 and 3.2, for free and interacting particles, respectively. Comparison between simulations and model is presented in Section 4. Finally, discussion and concluding remarks are provided in Section 5.
2 Molecular Dynamics Simulations
We present the details of the simulations as well as a brief description of the interaction potentials used in this work.
2.1 Interaction Potentials
The continuous interaction potentials are defined through:
where
where
where
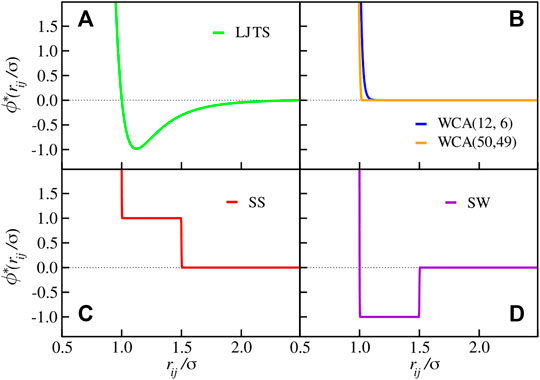
FIGURE 1. Potentials of interacting particles used for the simulations. (A) Potential of Truncated and Shifted Lennard-Jones. (B)
The Square-Well
where ω defines the spatial extent of the particle core in units of σ, q is related to the hardness of the potential, and
2.2 Simulation Method
In this work all quantities are assumed to be expressed in conventional reduced units, with
The simulations were run by
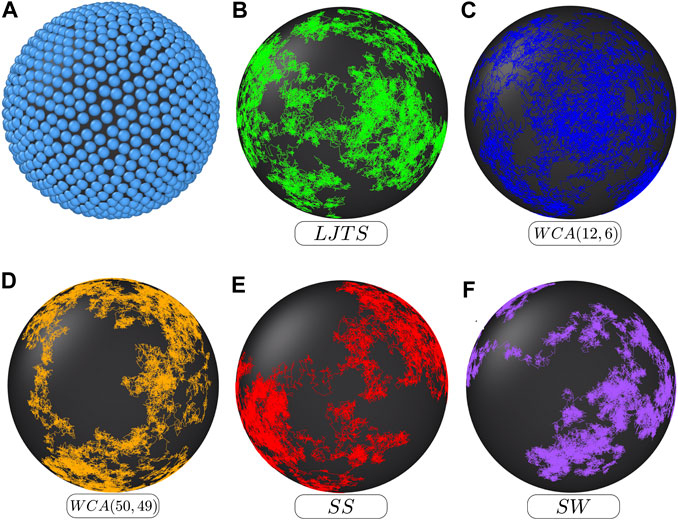
FIGURE 2. (A) Representative instantaneous configuration of the system with N = 1,000 particles on the spherical surface of density
The results of our simulations for the MSD are plotted in Figure 3. At the right side we plot the geodesic MSD over the sphere with the saturation valor of
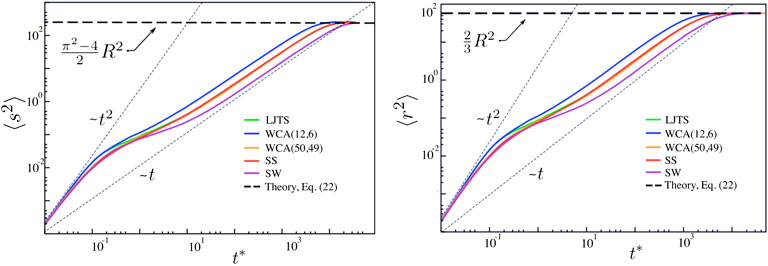
FIGURE 3. MSD of interacting particles over the sphere with the potentials given in Figure 1. The geodesic arc,
The MSD plotted in Figure 3 shows four different stages: 1) the ballistic regime where the MSD increases as
The form of the plots for the MSD in Figure 3 depends on the combined effect of the curvature of the surface, the interaction of the particles and, in the case of the projected displacement, the projection on the observation plane. In the next section we will deal with the connection between these different effects and their effect in the measured effective diffusion coefficient.
3 The MSD of Free and Interacting Particles
Commonly, experimental setups for measuring the diffusion of particles on curved surfaces use confocal microscopy techniques [33, 57, 58]. Due to the focal length inherent to these techniques, the measure of the motions of the particles under study is preferentially performed in terms of their projection to the focal plane [22, 23]. In view of this, in the present section we study the equivalence between the description of the free diffusion over the surface (the geodesic displacement) and compare with the evolution of its projection in the planar disk constrained by the sphere, see Figure 4. First, we will deduce an expression for the MSD of free particles moving at a flat disk by considering how the entropic restriction of the movement (imposed by the fact that the particles cannot travel beyond the radius of the disk) is reflected in the transport properties that are used to describe the motion. Then, we will modify the MNET description to include the interaction and crowding of particles.
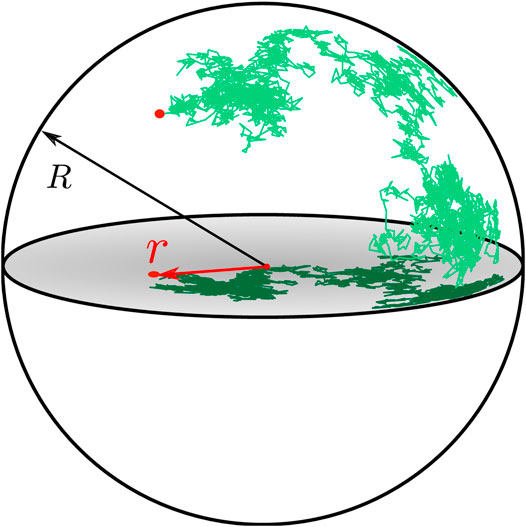
FIGURE 4. Projection on an equatorial plane of the Brownian motion which takes place over the surface of a sphere of radius R. The polar radial coordinate of the particle r is measured on the plane.
3.1 The MSD of Free Punctual Particles
From a thermodynamic point of view, the problem can be tackled by estimating the equivalent force keeping the system confined to diffuse in the planar disk delimited by the surface of the sphere of radius R, see Figure 4. This equivalent force can be written in terms of a Taylor expansion. To first order, this correspond to an harmonic-like potential of the form
The potential here is of an entropic origin, since it is due to the fact that the particles cannot leave the sphere projection or, equivalently, the planar disk. The maximum value of this potential, associated with the equivalent force, can be estimated by using the equipartition theorem [59]. Comparing the mean kinetic energy of the particles,
Hence, the projected motion of the particles constrained to diffuse in a disk under the influence of the equivalent harmonic force can be described by means of the Smoluchowsky equation
where
where the force that constraint the particles motions is
The mean square displacement in the constrained disk system is therefore given by
The time evolution of the MSD is obtained by taking the derivative
And substituting Eq. 8. Assuming zero flux boundary condition:
The comparison of the previous result with the result of diffusion in an infinite planar surface,
where the average here is over the ensemble of non-interacting particles. Eq. 11 is readily solved using that
Substituting the estimated value of
This result has been obtained by using different methods in Ref. [10] and, as expected, only depends upon geometrical considerations resulting from averaging methodologies applied directly to the Laplace-Beltrami diffusion equation.
This result describing the projection of the surface to the disk can be contrasted to the result of particles really moving on a planar disk of radius R, where
Comparison between Eqs 14, 15 shows that the displacement in a sphere respect to a planar projection has an average reduction by a factor of
Where
3.2 The MSD of Interacting Non-punctual Particles
In the previous subsection we have shown that the entropic restrictions, present by the fact that tracer particles move over a surface with a finite number of accessible states, are responsible for the observed saturation value reached by the MSD at long times. However, as we have seen in Figure 3, the simulations show that the time behavior of the MSD at intermediate times scales with a time dependence
This generalization goes along the same lines of Santamaría-Holek et al. [32], where a Smoluchowski description with time-dependent coefficients was used to demonstrate that the exponent characterizing the sub-diffusion is controlled by the nature of the local cages and the free space at disposal for their motion. In our case, since the single particle MSD reported here accounts for the motion of independent particles in a crowded media, the description of the diffusion can be given also in terms of the generalized Smoluchowski equation for the single-particle distribution function [61]. This equation for the probability is the counterpart of the respective generalized Langevin equation with memory effects, commonly used to describe microrheological experiments and has the peculiarity of being equivalent in the long-time regime to the Smoluchowski equation with time dependent coefficients [29, 30]. This fact was generalized in Refs. [31, 33, 62] for the case of power-law dependent memory kernels which are equivalent to time dependent diffusion coefficients of the form
where τ is a characteristic time of the anomalous diffusion process. In this equation, the exponent
Besides the entropic effect introduced through the ratio
This expression for the sub-diffusion exponent shows that it only depends in the radii of the particles and sphere, and on the parameter
Even in this approximation, it results difficult to estimate theoretically the parameters in Eq. 18. However, it is clear that the exponent of the subdiffusive process is linked to the shape of the interacting potential, the curvature of the surface and the projection of the forces to the observation plane. In order to find its value for the different potentials in Figure 1, we will adjust the data of the numerical simulations, using the Smoluchowski equation, but now in terms of this time dependent diffusion coefficient as we detail below.
Therefore, we will consider that the single particle Smoluchowski equation describing the dynamics of a tracer particle in an effective medium of
Where now the time dependent coefficient is given through Eqs. 17, 19. From the last equation, repeating the same procedure of the last section, the temporal evolution of the MSD is obtained to be
Using Eq. 6 we finally have
Which is the general expression for the MSD of the tracer particles valid for the whole time interval. From this equation, it is possible to deduce the temporal behavior of the diffusion coefficient for all the stages of the process in terms of the numerical data provided by the simulations as
Notice that this equation reproduces the expected behavior for short and long times. For short times
At the other hand, when (Eq. 22) is used in combination with the diffusion coefficient in Eq. 17, it accounts only for the process once the sub-diffusion stage has started. At intermediary times, it accounts for the sub-diffusion associated with particle interactions and cage diffusion effects and, for long times, it account for the fact that the particles have a restricted number of accessible states in a finite domain. In the next section we will use the numerical simulation in order to find the general behavior of the temporal diffusion coefficient and the exponents of sub-diffusion for the potentials we have considered in Section 2.
4 Comparison Between Model and Simulations
We have shown that the projection on the equatorial plane of the geodesic MSD, directly measured in the numerical simulations, is an equivalent form to characterize the diffusive dynamics of the particles. We have explained how the saturation value of the MSD rescales due to the restricted number of accessible states, giving rise to entropic constrictions on the dynamics of the particles.
For short and intermediate times, our methodology allows us to consider how different aspects modify the behavior of the MSD of interacting particles forced to diffuse in the surface of the sphere. All these aspects are clearly represented in Figure 5, where we plot the time-dependent diffusion coefficient,
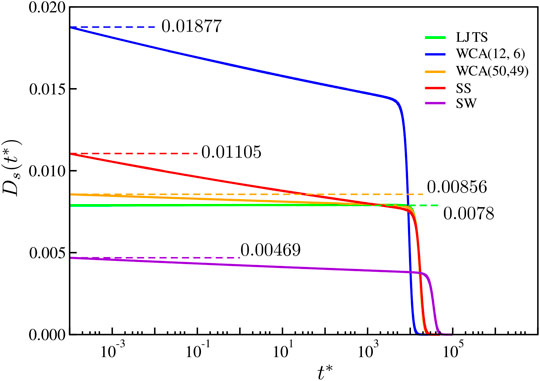
FIGURE 5. Projected surface diffusion coefficient
The first aspect to notice is the reference diffusion coefficient,
The second aspect to notice in the temporal dependence of the diffusion coefficient
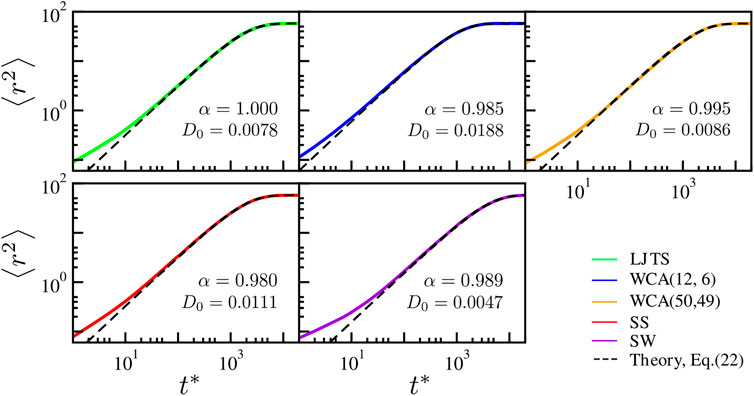
FIGURE 6. Comparison between the projected MSD obtained from simulations (colored solid lines) and theory (black lines) according to Eq. 22. The values of the parameters used are included in each plot. The scaling factor in Eq. 17 is
Figure 5, show that the value of the diffusion constant
Finally, the third aspect to notice is that both the ballistic and long-time behaviors of the MSD appear in all cases since not depend on the interaction potential. Because of this, they do not provide information of the fluid medium where the diffusion occurs. As it is seen in Figure 5, the final drop of the diffusion coefficient to zero occurs earlier as the diffusion coefficient is lower, as expected.
Our work shows that the deviations of the MSD relative to the planar behavior represent the effect that the particle interaction and crowding effects have on the dynamics. This effect is coupled with the presence of an entropic force restricting the number of accessible states. We prove that these two effects are well captured by the projected diffusion coefficient
The excellent agreement in Figure 6 among simulation results and the theory proposed indicates that particle interactions control the cage effect and the sub-diffusion regime. The delay induced by the crowded scenario appears since the transient stage, and its influence is captured by the memory effects that the effective diffusion coefficient
5 Conclusion
We have studied the statistical and dynamical properties of a system of interacting particles constituting a fluid embedded on a sphere’s surface. To understand the link between the microscopic properties of the fluid and the observed MSD, we have proposed a diffusion model incorporating memory and entropic effects that allowed us to derive a simple and powerful expression for the projected time-dependent diffusion coefficient. This expression allows us to characterize the dependence of the diffusive dynamics on the particle interactions and surface curvature. This approach takes into account the change of perspective inherent to the experimental measure of diffusion of particles on closed surfaces. The formalism proposed in this work reproduces also some limiting cases previously studied in the literature and exemplifies, with the use of ideas emerging from irreversible thermodynamics, how we can provide a more flexible description that helps to interpret complex phenomena occurring at the surface.
In this work, we have shown in Figure 3 that the dynamics of the MSD reflects four stages. Two, at short times
In the realm of applications, this study allows us to establish a connection with models and experiments studying the diffusion of several tracers in the cytoplasm of living cells which often exhibit heterogeneous distribution of macromolecular crowders [65–67]. This crowding is extremely important in the surface membrane and might affect the properties of anomalous diffusion. It is known, for example, that going from a less to a more crowded region will slow down the dynamics, both in terms of the exponent and diffusivity [68–70]. This results very important since the slow transport in the cell membrane of lipids and proteins is linked with protein cluster formation, phase segregation, lipid droplet formation, signal propagation and other crucial functions occurring on the cell surface which can be enhanced by the presence of sub-diffusion [71, 72].
Data Availability Statement
The original contributions presented in the study are included in the article/Supplementary Material, further inquiries can be directed to the corresponding author.
Author Contributions
IS-H, AL-D and JM-R designed the research. AL-D and JM-V performed the calculations and methology. IS-H, JM-R, AL-D and JM-V performed analysis. IS-H, JM-R, AL-D and SH wrote the original draft. All authors contributed to the analysis and discussion of the data and the writing of the paper.
Funding
SIH is grateful to projects UNAMDGAPA-PAPIIT IN114721 and LANCAD-UNAM-DGTIC-276. ISH acknowledges UNAM-DGAPA for financial support under grant number IN117419.
Conflict of Interest
The authors declare that the research was conducted in the absence of any commercial or financial relationships that could be construed as a potential conflict of interest.
Acknowledgments
Authors appreciate the technical support of Carlos Sair Flores Bautista, Alejandro de León Cuevas, Jair Santiago García Sotelo and Luis Alberto Aguilar Bautista from Laboratorio Nacional de Visualización Científica Avanzada (LAVIS-UNAM)
Footnotes
1Munguía-Valadez J, Chávez-Rojo MA, Moreno-Razo JA, Sambriski EJ (under review). The generalized continuous multiple step potential: model systems and related properties. J Chem Phys.
References
1. Moreno-Razo JA, Sambriski EJ, Abbott NL, Hernández-Ortiz JP, de Pablo JJ. Liquid-crystal-mediated self-assembly at nanodroplet interfaces. Nature (2012) 485(7396):86. doi:10.1038/nature11084
2. Holly C. Gaede and Klaus Gawrisch. Lateral diffusion rates of lipid, water, and a hydrophobic drug in a multilamellar liposome. Biophys J (2003) 85(3):1734–40. doi:10.1016/S0006-3495(03)74603-7
3. Nuytten N, Hakimhashemi M, Ysenbaert T, Defour L, Trekker J, Soenen SJ, et al. Pegylated lipids impede the lateral diffusion of adsorbed proteins at the surface of (magneto)liposomes. Colloids Surf B Biointerfaces (2010) 80(2):227–31. doi:10.1016/j.colsurfb.2010.06.009
4. Danelian E, Karlén A, Karlsson R, Winiwarter S, Hansson A, Löfâs S, et al. Spr biosensor studies of the direct interaction between 27 drugs and a liposome surface: correlation with fraction absorbed in humans. J Med Chem (2000) 43(11):2083–6. doi:10.1021/jm991156g
5. Ledesma-Durán A, Hernández SI, Santamaría-Holek I. Effect of surface diffusion on adsorption-desorption and catalytic kinetics in irregular pores. I. Local kinetics. J Phys Chem C (2017) 121(27):14544. doi:10.1021/acs.jpcc.7b03652
6. Ledesma-Durán A, Hernández SI, Santamaría-Holek I. Effect of surface diffusion on adsorption-desorption and catalytic kinetics in irregular pores. II. Macro-kinetics. J Phys Chem C (2017) 121(27):14557. doi:10.1021/acs.jpcc.7b03653
7. Faraudo J. Diffusion equation on curved surfaces. I. Theory and application to biological membranes. J Chem Phys (2002) 116(13):5831. doi:10.1063/1.1456024
8. Reister-Gottfried E, Leitenberger S, Seifert U. Diffusing proteins on a fluctuating membrane: analytical theory and simulations. Phys Rev E - Stat Nonlinear Soft Matter Phys (2010) 81(3):031903. doi:10.1103/PhysRevE.81.031903
9. Castro-Villarreal P, Villada-Balbuena A, Méndez-Alcaraz JM, Castañeda-Priego R, Estrada-Jiménez S. A brownian dynamics algorithm for colloids in curved manifolds. J Chem Phys (2014) 140(21):214115. doi:10.1063/1.4881060
10. Castro-Villarreal P. Intrinsic and extrinsic measurement for brownian motion. J Stat Mech (2014) 2014(5):P05017. doi:10.1088/1742-5468/2014/05/p05017
11. Reister-Gottfried E, Leitenberger SM, Seifert U. Hybrid simulations of lateral diffusion in fluctuating membranes. Phys Rev E - Stat Nonlinear Soft Matter Phys (2007) 75(1):011908. doi:10.1103/PhysRevE.75.011908
12. Castro-Villarreal P. Brownian motion meets riemann curvature. J Stat Mech (2010) 2010(8):P08006. doi:10.1088/1742-5468/2010/08/p08006
13. Apaza L, Sandoval M. Brownian self-driven particles on the surface of a sphere. Phys Rev E (2017) 96(2):022606. doi:10.1103/PhysRevE.96.022606
14. Petersen CF, Schrack L, Franosch T. Static properties of quasi-confined hard-sphere fluids. J Stat Mech (2019) 2019(8):083216. doi:10.1088/1742-5468/ab3342
15. Jinnai H, Koga T, Nishikawa Y, Hashimoto T, Hyde ST. Curvature determination of spinodal interface in a condensed matter system. Phys Rev Lett (1997) 78(11):2248. doi:10.1103/physrevlett.78.2248
16. Saito A, Foley HC. Curvature and parametric sensitivity in models for adsorption in micropores. AIChE J (1991) 37(3):429. doi:10.1002/aic.690370312
17. Tvergaard V. Effect of yield surface curvature and void nucleation on plastic flow localization. J Mech Phys Solid (1987) 35(1):43. doi:10.1016/0022-5096(87)90027-5
18. Roux A, Cuvelier D, Nassoy P, Prost J, Bassereau P, Goud B. Role of curvature and phase transition in lipid sorting and fission of membrane tubules. EMBO J (2005) 24(8):1537. doi:10.1038/sj.emboj.7600631
19. Resende FJ, Costa BV. Molecular-dynamics study of the diffusion coefficient on a crystal surface. Phys Rev B (2000) 61(19):12697. doi:10.1103/physrevb.61.12697
20. Wang D, He C, Stoykovich MP, Schwartz DK. Nanoscale topography influences polymer surface diffusion. ACS Nano (2015) 9(2):1656. doi:10.1021/nn506376n
21. Bulnes FM, Pereyra VD, Riccardo JL. Collective surface diffusion: n-fold way kinetic Monte Carlo simulation. Phys Rev E (1998) 58(1):86. doi:10.1103/physreve.58.86
22. Benavides-Parra JC, Jacinto-Méndez D, Brotons G, Carbajal-Tinoco MD. Brownian motion near a liquid-gas interface. J Chem Phys (2016) 145(11):114902. doi:10.1063/1.4962746
23. Sandoval-Jiménez IM, Jacinto-Méndez D, Toscano-Flores LG, Carbajal-Tinoco MD. Brownian-particle motion used to characterize mechanical properties of lipid vesicles. J Chem Phys (2020) 152(1):014901. doi:10.1063/1.5133092
24. King MR. Apparent 2-d diffusivity in a ruffled cell membrane. J Theor Biol (2004) 227(3):323–6. doi:10.1016/j.jtbi.2003.11.010
25. Naji A, Brown FL. Diffusion on ruffled membrane surfaces. J Chem Phys (2007) 126(23):235103. doi:10.1063/1.2739526
26. Gov NS. Diffusion in curved fluid membranes. Phys Rev E-Stat Nonlinear Soft Matter Phys (2006) 73(4):041918. doi:10.1103/PhysRevE.73.041918
27. Juffer AH, Berendsen HJC. Dynamic surface boundary conditions. Mol Phys (1993) 79(3):623. doi:10.1080/00268979300101501
28. Mie G. Zur kinetischen Theorie der einatomigen Körper. Ann Phys (1903) 316(8):657. doi:10.1002/andp.19033160802
29. Adelman SA. Fokker-Planck equations for simple non‐Markovian systems. J Chem Phys (1976) 64(1):124–30. doi:10.1063/1.431961
30. Tokuyama M, Mori H. Statistical-mechanical theory of random frequency modulations and generalized brownian motions. Prog Theor Phys (1976) 55(2):411–29. doi:10.1143/ptp.55.411
31. Metzler R, Barkai E, Klafter J. Deriving fractional fokker-planck equations from a generalised master equation. Europhys Lett (1999) 46(4):431. doi:10.1209/epl/i1999-00279-7
32. Santamaría-Holek I, Rubi JM. Finite-size effects in microrheology. J Chem Phys (2006) 125(6):64907. doi:10.1063/1.2241190
33. Santamaría-Holek I, Rubí JM, Gadomski A. Thermokinetic approach of single particles and clusters involving anomalous diffusion under viscoelastic response. J Phys Chem B (2007) 111(9):2293. doi:10.1021/jp0675375
34. Sevilla FJ, Rodríguez RF, Gómez-Solano JR. Generalized ornstein-uhlenbeck model for active motion. Phys Rev E (2019) 100:032123. doi:10.1103/PhysRevE.100.032123
35. Reguera D, Rubí JM, Vilar JM. The mesoscopic dynamics of thermodynamic systems. J Phys Chem B (2005) 109(46):21502. doi:10.1021/jp052904i
36. Pan W, Vekilov PG, Lubchenko V. Origin of anomalous mesoscopic phases in protein solutions. J Phys Chem B (2010) 114(22):7620. doi:10.1021/jp100617w
37. Dekker H. Nonisothermal activation: nonequilibrium thermodynamics of metastable mesoscopic systems. Phys Rev, A (1991) 43(8):4224. doi:10.1103/physreva.43.4224
38. Santamaría-Holek I, Hernández S, García-Alcántara C, Ledesma-Durán A. Review on the macro-transport processes theory for irregular pores able to perform catalytic reactions. Catalysts (2019) 9(3):281. doi:10.3390/catal9030281
39. Santamaría-Holek I, Grzywna ZJ, Rubi JM. Entropic effects in diffusion-adsorption processes in micropores. Eur Phys J Spec Top (2013) 222(1):129. doi:10.1140/epjst/e2013-01831-2
40. Reguera D, Rubí JM. Kinetic equations for diffusion in the presence of entropic barriers. Phys Rev E-Stat Nonlinear Soft Matter Phys (2001) 64(6):061106. doi:10.1103/PhysRevE.64.061106
41. Chacón-Acosta G, Pineda I, Dagdug L. Diffusion in narrow channels on curved manifolds. J Chem Phys (2013) 139(21):214115. doi:10.1063/1.4836617
42. Santamaría-Holek I, Ledesma-Durán A, Hernández SI, García-Alcántara C, Andrio A, Compañ V. Entropic restrictions control the electric conductance of superprotonic ionic solids. Phys Chem Chem Phys (2020) 22(2):437. doi:10.1039/c9cp05486c
43. Andersen HC, Weeks JD, Chandler D. Relationship between the hard-sphere fluid and fluids with realistic repulsive forces. Phys Rev A (1971) 4(4):1597. doi:10.1103/physreva.4.1597
44. Jover J, Haslam AJ, Galindo A, Jackson G, Müller EA. Pseudo hard-sphere potential for use in continuous molecular-dynamics simulation of spherical and chain molecules. J Chem Phys (2012) 137(14):144505. doi:10.1063/1.4754275
45. Julien-Piera V, Gilles T, Pascal V. Dynamics of a monodisperse Lennard-Jones system on a sphere. Mol Phys (2014) 112(9–10):1330. doi:10.1080/00268976.2014.901568
46. Julien-Piera V, Gilles T, Pascal V. Mode-coupling approach for the slow dynamics of a liquid on a spherical substrate. J Chem Phys (2015) 143(8):084505. doi:10.1063/1.4928513
47. Julien-Piera V, Gilles T, Pascal V. Glassy dynamics of dense particle assemblies on a spherical substrate. J Chem Phys (2018) 148(16):164501. doi:10.1063/1.5027389
48. Swope WC, Andersen HC, Berens PH, Wilson KR. A computer simulation method for the calculation of equilibrium constants for the formation of physical clusters of molecules: application to small water clusters. J Chem Phys (1982) 76(1):637. doi:10.1063/1.442716
49. Yamamoto E, Akimoto T, Yasui M, Yasuoka K. Origin of subdiffusion of water molecules on cell membrane surfaces. Sci Rep (2014) 4:4720. doi:10.1038/srep04720
50. Chipot C, Comer J. Subdiffusion in membrane permeation of small molecules. Sci Rep (2016) 6:35913. doi:10.1038/srep35913
51. Tan P, Liang Y, Xu Q, Mamontov E, Li J, Xing X, et al. Gradual crossover from subdiffusion to normal diffusion: a many-body effect in protein surface water. Phys Rev Lett (2018) 120(24):248101. doi:10.1103/PhysRevLett.120.248101
52. Ghosh SK, Cherstvy AG, Grebenkov DS, Metzler R. Anomalous, non-Gaussian tracer diffusion in crowded two-dimensional environments. New J Phys (2016) 18(1):013027. doi:10.1088/1367-2630/18/1/013027
53. Schwille P, Korlach J, Webb WW. Fluorescence correlation spectroscopy with single-molecule sensitivity on cell and model membranes. Cytometry (1999) 36(3):176–82. doi:10.1002/(sici)1097-0320(19990701)36:3<176::aid-cyto5>3.0.co;2-f
54. Golan Y, Sherman E. Resolving mixed mechanisms of protein subdiffusion at the t cell plasma membrane. Nat Commun (2017) 8(1):15851–15. doi:10.1038/ncomms15851
55. Ehrig J, Petrov EP, Schwille P. Near-critical fluctuations and cytoskeleton-assisted phase separation lead to subdiffusion in cell membranes. Biophys J (2011) 100(1):80–9. doi:10.1016/j.bpj.2010.11.002
56. Weiss M, Elsner M, Kartberg F, Nilsson T. Anomalous subdiffusion is a measure for cytoplasmic crowding in living cells. Biophys J (2004) 87(5):3518–24. doi:10.1529/biophysj.104.044263
57. Zhong Y, Zhao L, Tyrlik PM, Wang G. Investigating diffusing on highly curved water-oil interface using three-dimensional single particle tracking. J Phys Chem C (2017) 121(14):8023–32. doi:10.1021/acs.jpcc.7b01721
58. Sarmiento-Gomez E, Santamaría-Holek I, Castillo R. Mean-square displacement of particles in slightly interconnected polymer networks. J Phys Chem B (2014) 118(4):1146. doi:10.1021/jp4105344
59. Mendoza CI, Santamaría-Holek I, Pérez-Madrid A. Effective temperatures and the breakdown of the Stokes-einstein relation for particle suspensions. J Chem Phys (2015) 143(10):104506. doi:10.1063/1.4930550
60. Gustafsson S, Halle B. Diffusion on a flexible surface. J Chem Phys (1997) 106(5):1880–7. doi:10.1063/1.473326
61. Mason TG, Ganesan K, van Zanten JH, Wirtz D, Kuo SC. Particle tracking microrheology of complex fluids. Phys Rev Lett (1997) 79(17):3282. doi:10.1103/physrevlett.79.3282
62. Rodríguez RF, Salinas-Rodríguez E, W Dufty J. Fokker-Planck and Langevin descriptions of fluctuations in uniform shear flow. J Stat Phys (1983) 32(2):279–98. doi:10.1007/BF01012711
63. Happel J, Brenner H. Low Reynolds number hydrodynamics: with special applications to particulate media, Vol. 1. Berlin, Germany: Springer Science & Business Media (2012).
64. Saxton MJ. A biological interpretation of transient anomalous subdiffusion. i. qualitative model. Biophys J (2007) 92(4):1178–91. doi:10.1529/biophysj.106.092619
65. Szymanski J, Weiss M. Elucidating the origin of anomalous diffusion in crowded fluids. Phys Rev Lett (2009) 103(3):038102. doi:10.1103/PhysRevLett.103.038102
66. Sharonov A, Bandichhor R, Burgess K, Petrescu AD, Schroeder F, Kier AB, et al. Lipid diffusion from single molecules of a labeled protein undergoing dynamic association with giant unilamellar vesicles and supported bilayers. Langmuir (2008) 24(3):844–50. doi:10.1021/la702600w
67. Javanainen M, Hammaren H, Monticelli L, Jeon JH, Miettinen MS, Martinez-Seara H, et al. Anomalous and normal diffusion of proteins and lipids in crowded lipid membranes. Faraday Discuss (2013) 161:397–59. doi:10.1039/c2fd20085f
68. Guigas G, Weiss M. Sampling the cell with anomalous diffusion - the discovery of slowness. Biophys J (2008) 94(1):90–4. doi:10.1529/biophysj.107.117044
69. Jeon JH, Monne HM, Javanainen M, Metzler R. Anomalous diffusion of phospholipids and cholesterols in a lipid bilayer and its origins. Phys Rev Lett (2012) 109(18):188103. doi:10.1103/PhysRevLett.109.188103
70. Goose JE, Sansom MS. Reduced lateral mobility of lipids and proteins in crowded membranes. PLoS Comput Biol (2013) 9(4):e1003033. doi:10.1371/journal.pcbi.1003033
71. Metzler R, Jeon JH, Cherstvy AG. Non-brownian diffusion in lipid membranes: experiments and simulations. Biochim Biophys Acta (2016) 1858(10):2451–67. doi:10.1016/j.bbamem.2016.01.022
Keywords: molecular simulations, mean square displacement, surface sphere, diffusion coefficient, curved surfaces
Citation: Ledesma-Durán A, Munguía-Valadez J, Moreno-Razo JA, Hernández SI and Santamaría-Holek I (2021) Entropic Effects of Interacting Particles Diffusing on Spherical Surfaces. Front. Phys. 9:634792. doi: 10.3389/fphy.2021.634792
Received: 28 November 2020; Accepted: 08 January 2021;
Published: 25 March 2021.
Edited by:
Ramon Castañeda-Priego, University of Guanajuato, MexicoReviewed by:
Leonardo Dagdug, Metropolitan Autonomous University, MexicoPavel Castro-Villarreal, Autonomous University of Chiapas, Mexico
Andrey Cherstvy, University of Potsdam, Germany
Copyright © 2021 Ledesma-Durán, Munguía-Valadez, Moreno-Razo, Hernández and Santamaría-Holek. This is an open-access article distributed under the terms of the Creative Commons Attribution License (CC BY). The use, distribution or reproduction in other forums is permitted, provided the original author(s) and the copyright owner(s) are credited and that the original publication in this journal is cited, in accordance with accepted academic practice. No use, distribution or reproduction is permitted which does not comply with these terms.
*Correspondence: I. Santamaria Holek, isholek.fc@gmail.com