A Study of Dramatic Action and Emotion Using a Systematic Scan of Stick Figure Configurations
- 1Theater Laboratory, Weizmann Institute of Science, Rehovot, Israel
- 2Department of Molecular Cell Biology, Weizmann Institute of Science, Rehovot, Israel
Comprehending the meaning of body postures is essential for social organisms such as humans. For example, it is important to understand at a glance whether two people seen at a distance are in a friendly or conflictual interaction. However, it is still unclear what fraction of the possible body configurations carry meaning, and what is the best way to characterize such meaning. Here, we address this by using stick figures as a low-dimensional, yet evocative, representation of body postures. We systematically scanned a set of 1,470 upper-body postures of stick figures in a dyad with a second stick figure with a neutral pose. We asked participants to rate the stick figure in terms of 20 emotion adjectives like sad or triumphant and in terms of eight active verbs that connote intent like to threaten and to comfort. The stick figure configuration space was dense with meaning: people strongly agreed on more than half of the configurations. The meaning was generally smooth in the sense that small changes in posture had a small effect on the meaning, but certain small changes had a large effect. Configurations carried meaning in both emotions and intent, but the intent verbs covered more configurations. The effectiveness of the intent verbs in describing body postures aligns with a theory, originating from the theater, called dramatic action theory. This suggests that, in addition to the well-studied role of emotional states in describing body language, much can be gained by using also dramatic action verbs which signal the effort to change the state of others. We provide a dictionary of stick figure configurations and their perceived meaning. This systematic scan of body configurations might be useful to teaching people and machines to decipher body postures in human interactions.
Introduction
Body language pervades our lives. It helps us make sense of the state and intent of others and understand, from a distance, whether people are in a friendly or conflictual interaction. Despite its importance, we lack systematic ways to describe the meaning that body language carries. In other words, we lack ways to map the space of body configurations to fields of perceived meaning. A better understanding of body language is important for understanding basic neuroscience of how meaning is made [1–3], for improved human–machine interaction [4–11], and for training people to better understand body language.
What types of meaning are conveyed by body language is an open question. There are at least two main theories. One theory originates in psychology and considers body language as primarily expressing emotions [1–3, 12]. This emotional body-language theory takes ideas from the facial expression of emotion and applies them to research the perceived emotional meaning of body postures. Indeed, people can quickly understand emotion from a snapshot showing body postures, sometimes as rapidly and accurately as emotions are perceived from faces [13, 14]. Thus, according to the emotional body-language theory, body language primarily speaks in adjectives: happy, sad, triumphant, and sympathetic.
A second theory for body language is that it primarily conveys dramatic actions. A dramatic action is defined as the effort to change the state of another. Dramatic actions can be described by transitive verbs, such as to encourage, to comfort, to threaten, to scold. Unlike emotion adjective which define a state, dramatic action verbs define an effort to change the state of another. Dramatic action theory originates from the practice of theater [15], and was recently operationalized by Liron et al. [16]. Dramatic action theory and emotional body language theory are non exclusive: body language can carry both types of meaning.
Since the concept of dramatic action is not widely known in behavioral research, we provide a brief background. The field of theater often aims to create specific portrayals of human interaction. Accumulated experience shows that instructions for actors based on psychological factors such as emotion, motivation, and narrative are not enough to generate satisfactory performance [15]. Theater directors and actors rely on an additional layer, which is thought to be essential for creating believable interaction. This facet of behavior is dramatic action [17].
Dramatic actions are observable behaviors whose timescale is on the order of seconds. In this way, dramatic actions differ from internal motivations [18, 19], which last the entire play, and goals, which can last an entire scene. A character can change dramatic actions rapidly in an attempt to reach a goal. Dramatic actions are distinct from emotions because they are not states but instead are the efforts made to change the other's state. One can carry out a given dramatic action, such as threaten someone else, while experiencing different emotional states such as happy, angry, or sad.
Dramatic actions are related to a subset of Austin's concept of speech acts [20]. Many dramatic actions, however, are not speech acts, and in fact, do not require speech.
Dramatic actions need not necessarily succeed. An attempt to threaten or to comfort may or may not change the state of the other. Regardless of success or failure, one can still detect the effort made to change the state of the other: the dramatic action. Often, dramatic actions are part of people's habitual behavior and can be performed without conscious deliberation.
Dramatic actions can be conveyed by text: the same text can be said with different dramatic actions. For example, the text “come here” can have a different dramatic action if said by a parent soothing a child, or by a drill sergeant threatening a recruit. Dramatic actions are often conveyed through non-verbal signals including body language and gestures, facial expressions, speech, and physical actions. Even animals and babies can detect, carry out, and respond to dramatic actions [21]. Babies can activate surrounding adults and react to soothing voices; dogs can try to cheer people around them or threaten other dogs.
Currently, most research focuses on emotional body language theory, whereas the dramatic action theory has not been extensively tested. We set out to test both theories on the same stimuli in order to gain a more complete understanding of the range of meanings in body language.
Testing these theories requires a large and unbiased set of body posture stimuli in a social context. Most studies employ pictures of actors or cartoons which cover a small set of the range of possible postures. To obtain a systematic and unbiased set of body posture stimuli, we use stick figures. Stick figures represent the human body with a few angle coordinates [22–24]. By varying these angles, we systematically scanned 1,470 body configurations in a dyad of stick figures. Online participants scored these stick figure images for a set of eight dramatic actions and 20 emotions. Dramatic actions were found somewhat more frequently and strongly than emotions. From an applied point of view, we provide a dictionary of stick figure body postures with defined dramatic action and emotional meanings, which may be useful for research and automated image understanding, and for training people to understand body language.
Methods
Stick Figures
We defined stick figures (SF) made of nine line segments and a circle (Figure 1A), representing two-segment legs (length 0.283 each, in units where SF height is 1), a torso (0.364, including a neck of length 0.055), two-segment arms (0.224 each), and a head (radius 0.07). The segment proportions were taken from average adult anthropometric proportions [25]. There was no representation of ankles, wrists, hands, shoulders, face, etc. The SF configurations were defined by five angles: the angles of the torso, two shoulders, and two elbows (Figure 1A). We discretized these angles in order to sample the SF configuration space: The torso angle θ1 had two values: vertical to the floor and leaning to the left at 30°. The shoulder angles θ2, θ4 each had six options: 90°, 135°, 165°, 195°, 225°, and 270° relative to the neck, clockwise. The elbow angles θ3 and θ5 had seven values: 45°, 90°, 135°, 180°, 225°, 270°, and 315° relative to the arm, clockwise. The number of values for each angle was chosen based on pilot studies to allow for completeness at a reasonable survey size. The legs were not varied in order to allow a feasible survey size, despite the expressiveness of leg posture such as kneeling. Angle combinations in which two segments exactly overlapped were removed. Only unique configurations were included (no two sets of angles yielded the same SFs), resulting in 1,470 unique SFs.
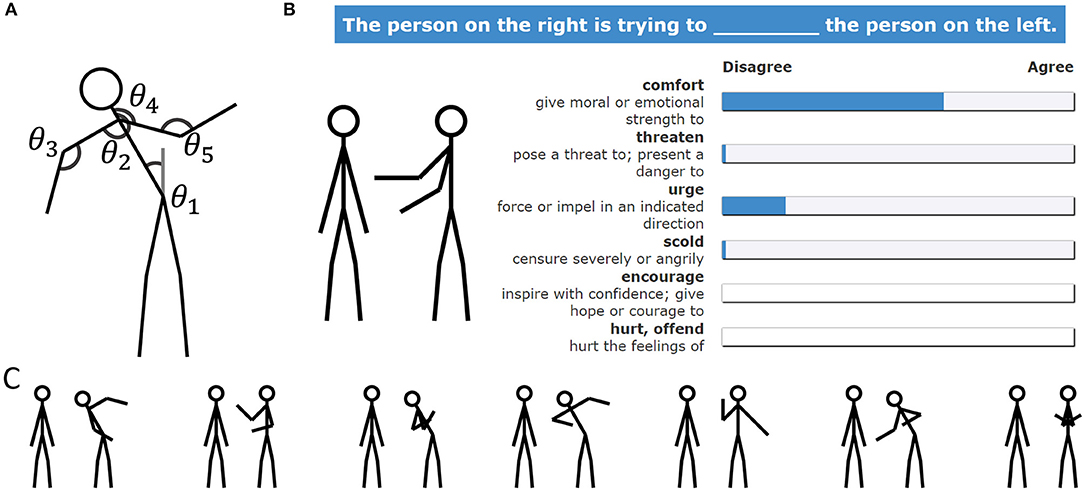
Figure 1. Stimuli and survey designs. (A) Stick figure configurations are defined by five angles. Scanning these angles at a set of values yielded 1,470 unique SF configurations. (B) Schematic of the survey: A stick figure dyad was presented, in which the right SF configuration was one of 1,470 possibilities and the left SF was in a neutral configuration. Online participants marked their agreement level for completing a sentence with each of the eight DA verbs (or, in experiments 2 and 3, with emotion adjectives). (C) Seven typical stimuli from the set of 1,470.
Each stimulus included two SFs (Figures 1B,C). The SF with different configurations was on the right, and the SF on the left had the same configuration in all images with θ1 =0°, θ2 =195°, θ3 =180°, θ4 =165°, θ5 =180°. The distance between the two SFs was 0.55 when the right torso was upright (θ1 =0°) and 0.64 when the right SF torso leaned to the left (θ1 =30°). The latter distance was used to avoid contact between the SFs.
Sampling and Participants
A total of 816 (56% female) participants, recruited on the Amazon Mechanical Turk platform [26], answered the survey (324, 470, and 558 in experiments 1, 2, and 3, respectively). The survey was limited to US residents who passed an English test, with at least 100 previously approved surveys on Mechanical Turk. For every combination of a word and an image, we obtained answers from 20 different participants. The experiment was approved by the institutional review board of the Weizmann Institute of Science, Rehovot, Israel. Informed consent was obtained from all participants before answering the survey.
Survey Design
In order to avoid priming participants with dramatic actions (DA) and emotions in the same experiment, we performed separate experiments: experiment 1 for DAs, experiment 2 for basic emotions [15], and experiment 3 for social emotions. In experiment 2, we asked both for the emotion of the right SF and for the emotion of the left SF. In this analysis, we focus on the right SF. Experiment 2 was completed 4 months after experiment 1. Experiment 3 was completed 12 months after experiment 1.
The participants were shown an image of two SFs and were asked to rate how well a word completes a sentence describing the image (Figure 1B). The rating used a continuous “agree-disagree” slider scale. Instructions for experiment 1 (on DAs) were as follows:
“You are about to see 14 images. After viewing each image, press continue. Then, you will see the same image on the left, together with nine possible suggestions for completing the statement below, and nine bars on the right (see example). Use the bars to indicate your agreement with each statement so that it correctly describes the image, as you see it. The next image will be available only after you marked your answers in the nine bars. “The person on the right is trying to ________ the person on the left”.”
We chose a set of DA verbs based on a previous study of 22 verbs presented with cartoon/clipart stimuli [16]. We chose verbs that were strongly perceived by participants in Liron et al. [16] and omitted verbs that were synonyms, to arrive at a list with eight DA verbs. The eight DA verbs were encourage, support, comfort, urge, hurt, bully, scold, and threaten. These eight verbs and one attention check question were provided in a random order for each image. The attention check question asked the participants to mark one of the ends of the slider scale (“agree” or “disagree”).
The structure of experiment 2 was very similar to that of experiment 1 (Supplementary Figure 1). Instructions for experiments 2 and 3 (on emotions) were identical to experiment 1, except for six emotion adjectives instead of eight DA verbs, and the sentence “The person on the right is feeling ________.” or “The person on the left is feeling ________.” The images were identical to experiment 1, except for a small arrow indicating the SF on the right or left (Supplementary Figure 1). The six emotion adjectives were happy, sad, disgusted, angry, surprised, and afraid. These emotion adjectives are commonly used in emotional body language research [27].
Experiment 3 tested an additional 14 emotions that have been most commonly used in recent studies on emotions [28–31], including social emotions: amusement, awe, confusion, contempt, love, shame, sympathy, compassion, desire, embarrassment, gratitude, guilt, pride, and triumph. The basic emotion angry was used as a control to compare with the results of experiment 2.
The “agree-disagree” slider scale had no initial value. The participants had to click on the slider in order to mark their score. The scale position indicated by the participants was converted to a number between 0 (disagree) and 100 (agree) for analysis.
One unit of the survey was a sequence of 14 images in random order. Experiment 1 had 2,100 units and experiments 2 and 3 had 4,200 units. For each of the 14 visual stimuli, the participants were first presented with a stimulus at the center of the screen, and after clicking on the “next” button, the eight DA verbs or six to eight emotion adjectives and an attention check question were added, arranged in random order (Figure 1B, Supplementary Figure 1).
Data and Statistical Analysis
We removed 36 units in which a respondent failed the English test or answered more than one unit in an hour. The units in which the participants failed two or more attention check questions were removed (47/2,100, 171/4,200, and 189/4,200 of the units in experiments 1, 2, and 3, respectively, which make up 3.9% of the total units). Thus, 2,049, 2,016, and 4,011 units (of 14 images) entered the analysis for experiments 1, 2, and 3, respectively. In total, we analyzed 229,488 word–image responses for experiment 1, 337,764 for experiment 2, and 444,080 for experiment 3. The scores in these experiments showed a distribution with a peak at zero. We counted an identified word as a median score above 50, which is the midpoint between disagree and agree.
To compute the probability that a median score exceeds 50 by chance, we used bootstrapping. For each image and word, we generated 104 bootstrapped datasets as follows: for each participant that answered the question, we chose an answer at random from the set of all answers provided by that participant in the experiment (without attention checks). This preserves the response statistics of each participant. We found that the probability that the median score of at least one word–image pair in an image exceeded 50 in the bootstrapped data is 0.0146 for DA and 1.9·10−4 and 1.1·10−4 for basic and social emotions of the right SF, respectively.
For data clustering, we used the clustergram function in MATLAB R2017b (MathWorks) with correlation distance and clustering along the rows of data only.
Results
Stick Figure Stimuli
In order to generate a systematic and unbiased set of stimuli, we defined stick figures (SFs) with five degrees of freedom (Figure 1A). We kept the legs in a fixed configuration to focus on upper body postures, which can show all emotions [32] and cover the affected space [33]. To focus on body posture and not facial expressions, the SF “head” was a circle.
In order to sample the SF configuration space, we discretized the angles, with two values for the torso angles, six for the shoulder angles, and seven for the elbow angles (Figure 1A). This resulted in 1,470 unique SF configurations (Methods).
To study the body posture of an SF interacting with another SF, we used images that showed two SFs (Figures 1B,C). The right SF had one of the 1,470 configurations, and the other had a constant neutral position.
Surveys of DA and Emotion
The online participants identified DA and emotion words for the SF dyads. We count a word as identified for a given image if its median score between participants exceeds a threshold of 50, which is the midway point between disagree (0) and agree (100). The results are robust to changes of this threshold (Supplementary Figures 2, 3). We find that the participants identified at least one DA or emotion in 54% of the images (798/1,470).
Participants identified at least one DA verb for 40% (591/1,470) of the images (Figure 2A), with an average of 1.7 DA verbs per image. A total of 57% of these images had one reported DA, 20% had two reported DAs, and 23% had three or more reported DAs (Supplementary Figure 4).
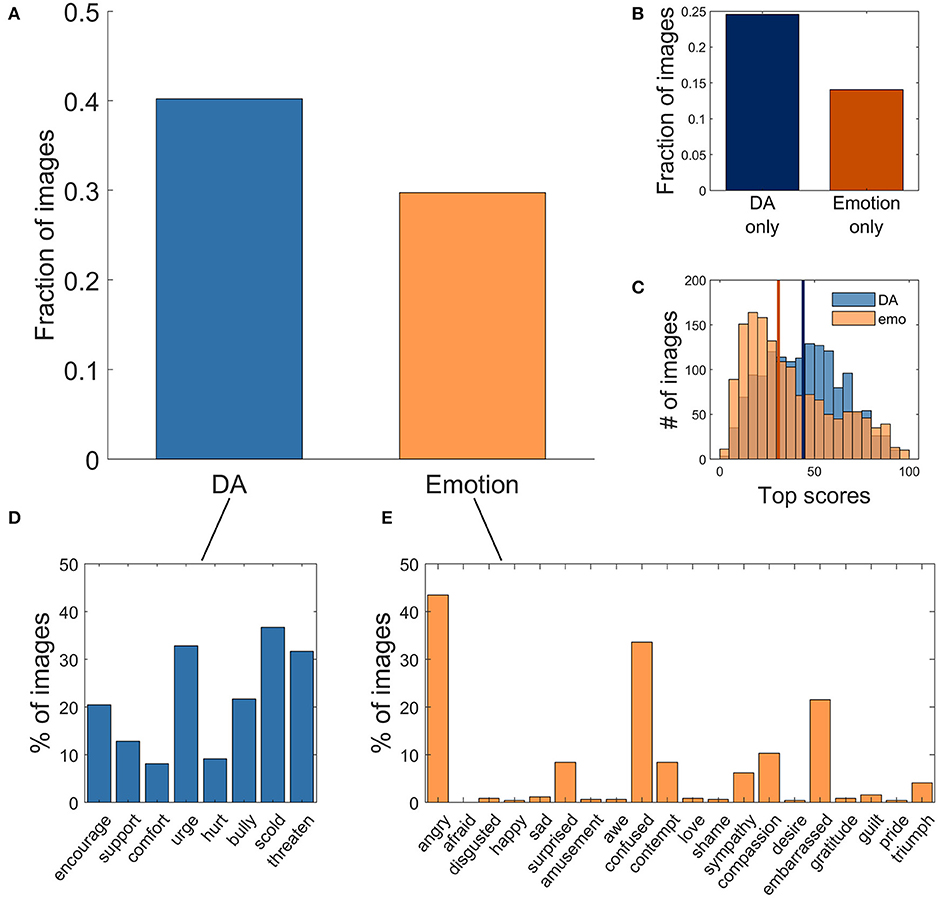
Figure 2. Dramatic actions and/or emotions were found in most images, with the former found somewhat more frequently and strongly. (A) Fraction of the 1,470 images identified with at least one DA (blue) and at least one emotion (orange). (B) Fraction of images identified with at least one DA but no emotion, and at least one emotion but no DA. (C) Distribution of top scores. We compare the eight DA words with the eight emotion words that received the highest scores (angry, confused, surprised, contempt, embarrassed, compassion, triumph, sympathy). The distribution of the highest scores of DA words (median = 44) is different from the distribution of the highest scores of emotion words (median = 31), (Wilcoxon–Mann–Whitney U > 106, p < 10−22 two-tailed) with a large effect size (AUC = 0.61). Vertical lines show the median of each distribution. (D) Percent of images, out of the images identified with at least one DA, that were identified with each of the eight DA verbs. For example, the DA ‘encourage’ was identified in 128 images out of the 591 images where at least one DA was identified. Note that the images were often identified with several DAs so that the histogram sums to more than 100%. (E) Percent of images, out of the images identified with at least one emotion, which were identified with each of the twenty emotion adjectives.
The participants identified at least one emotion word for 29.4% (432/1,470) of the images (Figure 2A), with an average of 1.4 emotion words per image. A total of 63% of these images had one reported emotion, 30% had two reported emotions, and 7% had three or more reported emotions.
The participants identified DAs but not emotions in 361 images and identified emotion but not DA in 207 images (Figure 2B). In general, the scores for DA were higher than the scores for emotions (Wilcoxon–Mann–Whitney test, U > 106, p < 10−22 two-tailed, Figure 2C).
A potential concern is that the comparison was across participants rather than within participants. To address this, we repeated the analysis for a subset of n = 68 participants who participated in both experiments 1 and 2 and answered the surveys for DA and emotion for the same images. We find qualitatively similar conclusions: DAs were identified in 75% of images, emotions in 56% (one-sided paired t-test p < 10−8). The number of cases where DA was identified but not emotion was 3.1-fold higher than the number of cases where emotion was identified but not DA (one-sided paired t-test p < 10−8) (Supplementary Figure 5).
We conclude that the participants identified DAs and emotions in about half of the SF dyads. Both DAs and emotions were found frequently. DAs were perceived somewhat more frequently and strongly. To test how strongly the emotions and DAs are correlated, we performed regression analysis. Emotion scores were found to be able to predict 54 ± 17% of the variance of each DA verb. Conversely, DA scores were found to be able to predict 24 ± 20% of the variance of each emotion (mean ± STD, full results in Supplementary Tables 1, 2). Thus, while emotions and DAs are correlated to a certain extent, the concepts are distinct [16]; both DAs and emotions are required to cover the image dataset.
Prevalence of Different DAs and Emotions
Of the 20 emotions describing the SFs, several emotions were found much more often than others: The top three emotions were angry, confused, and embarrassed, which were found in 21–44% of the images. Other emotion adjectives were rarely found, with 13 of the emotions assigned to <5% of the images (Figure 2E). Note that each image could be described with multiple words so that the fractions of images do not sum up to 100%.
In contrast, different DAs were identified with roughly similar frequencies (Figure 2D) in the range of 8–37%. Thus, the present set of DA verbs seems to allow for a more refined differentiation between these images than the present set of emotion adjectives.
In order to visualize the data, and to further see the relationship between DAs and emotions, we clustered the images and the agreement scores (Figure 3). In the resulting clustergram, images with similar response scores (scores for DAs and emotions) are placed near each other [dendrogram presented in SI (Supplementary Figure 6)]. Similarly, DAs or emotions found in similar images are placed near each other.
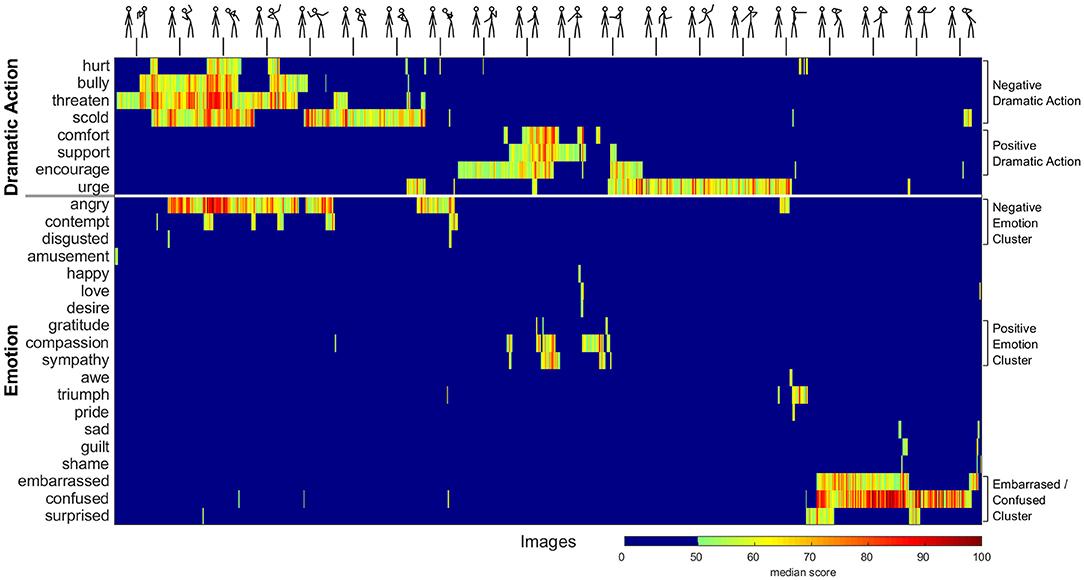
Figure 3. Clustergram of all 798 images identified with at least one DA or emotion. Each column represents a single image, and each row represents a DA or emotion word, arranged by clustering. Hence, images with similar scores are placed adjacently, and DAs found in similar images are placed adjacently. Color represents median score (median scores below 50 are in blue).
We find that DAs cluster into two groups, which can be seen as two large, colored regions in the upper part of the clustergram (Figure 3). These groups are the negative DAs (bully, threaten, scold, and hurt) and the positive DAs (comfort, encourage, and support). The division into positive and negative valence groups is also noticeable when clustering the correlation vectors of the scores of all of the 1,470 images (Supplementary Figure 7). The notion of negative and positive valence for DAs is further supported by principal component analysis of the images based on their DA scores (Supplementary Figure 8), which shows that the first principal axis goes from the most negative to most positive in the following order: threaten, scold, bully, hurt, urge, comfort, support, encourage. The DA “urge” seems to be placed between the negative and positive valence.
Each DA has images in which it is found alone or in combination with other DAs of similar valence. For example, threaten and bully often go together (120 images). Negative DAs were never found together with positive DAs in the same image.
The bottom part of Figure 3 shows that the emotion scores are sparser than DA scores as mentioned above, with 70% of the images showing scores below 50 for all emotions. We find that images with negative DAs are most often identified with the emotion angry (156/295 images).
Images with positive DAs (154 images) are more rarely identified with emotion; only 36 of them are identified with sympathy or compassion. A final cluster of images was scored with the emotions embarrassed and confused, usually without a DA.
We repeated the clustering analysis for all 1,470 images, without zeroing out median scores smaller than 50 (Supplementary Figure 9). The clustergram shows similar results; images identified with DAs are clustered in two groups of positive and negative valence, and DA scores are generally higher than emotion scores.
We conclude that both emotions and DAs are needed to fully describe the images, and that people can differentiate between DAs in the present set of stimuli to a greater extent than they can differentiate between emotions.
Mapping of Body Configuration Space to DA and Emotion
We next asked about the relationship between the SF body coordinates and the emotion and DA responses. For this purpose, we display all of the 1,470 SF configurations in a set of panels (Figures 4A–D).
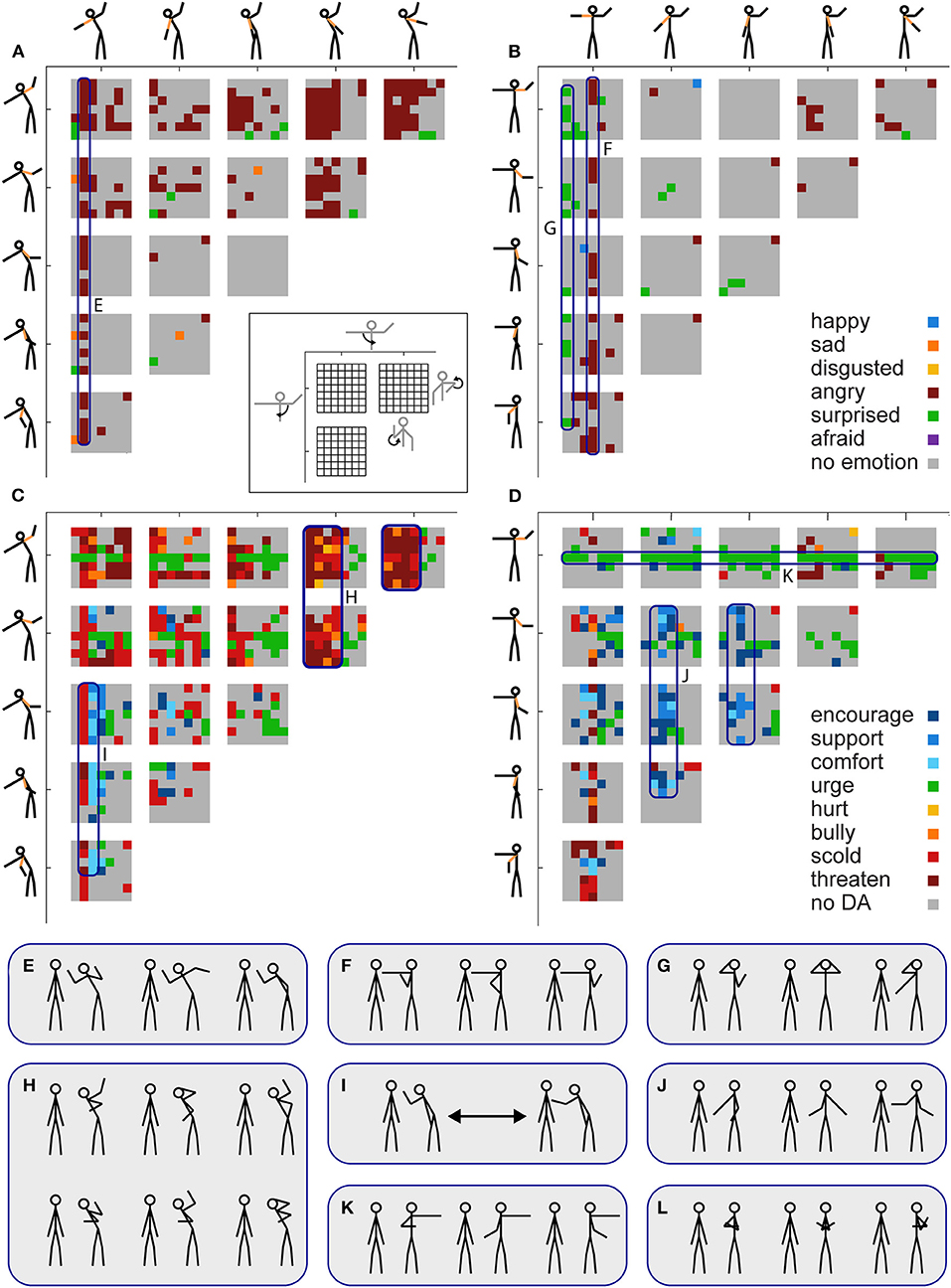
Figure 4. Regions of SF configuration space map to specific emotions and DAs. All 1,470 SF configurations are displayed in a set of panels, arranged as follows. (A,B) indicate tilted and upright torso, respectively. The 5 × 5 combinations of the right and left shoulder angles correspond to 25 matrices, of which 15 are shown due to symmetry. Each matrix corresponds to the 7 × 7 elbow angles (axes in inset). SF configurations are color-coded according to the emotion adjective with the highest score for each image. Images in which no emotion word score exceeded 50 are in gray. (C,D) Same as above except that configurations are color-coded according to the DA with the highest score for each image. Images in which no DA score exceeded 50 are in gray. (E) A group of SFs showing the emotion angry is tilted toward the second SF with an arm at 90° and an elevated forearm (elbow at 90°). (F) Angry is identified in an upright position and a straight horizontal arm toward the second SF. (G) Upright posture together with a hand touching the head is usually identified with the emotion surprise. (H) Many negative DAs are identified in “W-shape” configurations, in which the elbows are high and form sharp angles. (I) A change of one angle (the elbow, from raised hand to low hand) transforms the score from negative valence (left) to positive valence (right). (J) Positive valence DAs are identified with upright configurations with low hands. (K) Urge is identified with a posture of horizontal arm pointing away from the second SF. (L) SFs with the arms crossed are often identified with the emotion angry, and with the DA scold. The figures in (E–L) are examples selected manually.
Figure 4 displays all possible angle configurations in the study; it is a complete display of the five-dimensional stick figure configuration space. There are 5 right shoulder × 5 left shoulder × 7 right elbow × 7 left elbow × 2 torso combinations. These are displayed in a hierarchical manner. There are two columns (each for a torso angle). Each column has two subfigures, one for emotions and the other for DA. Each subfigure shows all the arm configurations; these are ordered into 25 small matrices (5 × 5 shoulder configurations), but only 15 are displayed due to symmetry. Each small matrix shows the 7 × 7 elbow configurations.
We begin with emotions (Figures 4A,B) by coloring each SF configuration according to the strongest emotion identified for the right SF (gray squares have no emotion scores above 50). For simplicity, we consider the six basic emotions (happy, sad, disgusted, angry, surprised, and afraid). We find that the adjective angry is elicited primarily (77%) by the torso tilted toward the other (Figure 4A). It is most prevalent with one arm raised backwards at angle of 90° with respect to the torso (Figure 4A, upper row of panels). Another group of angry SFs with a tilted torso have a raised arm toward the other SF and the elbow is at 90° (Figure 4E). In SFs with an upright torso, angry is found in a stripe in which θ2 = 270° and θ3 = 180°, where a straight horizontal arm points toward the second SF (Figure 4F).
The next most common emotion of the six, surprised, is identified primarily in upright SFs, with a symbolic gesture of hands touching the head (Figure 4G). The three SFs with the highest scores for each emotion are shown in Figure 5A.
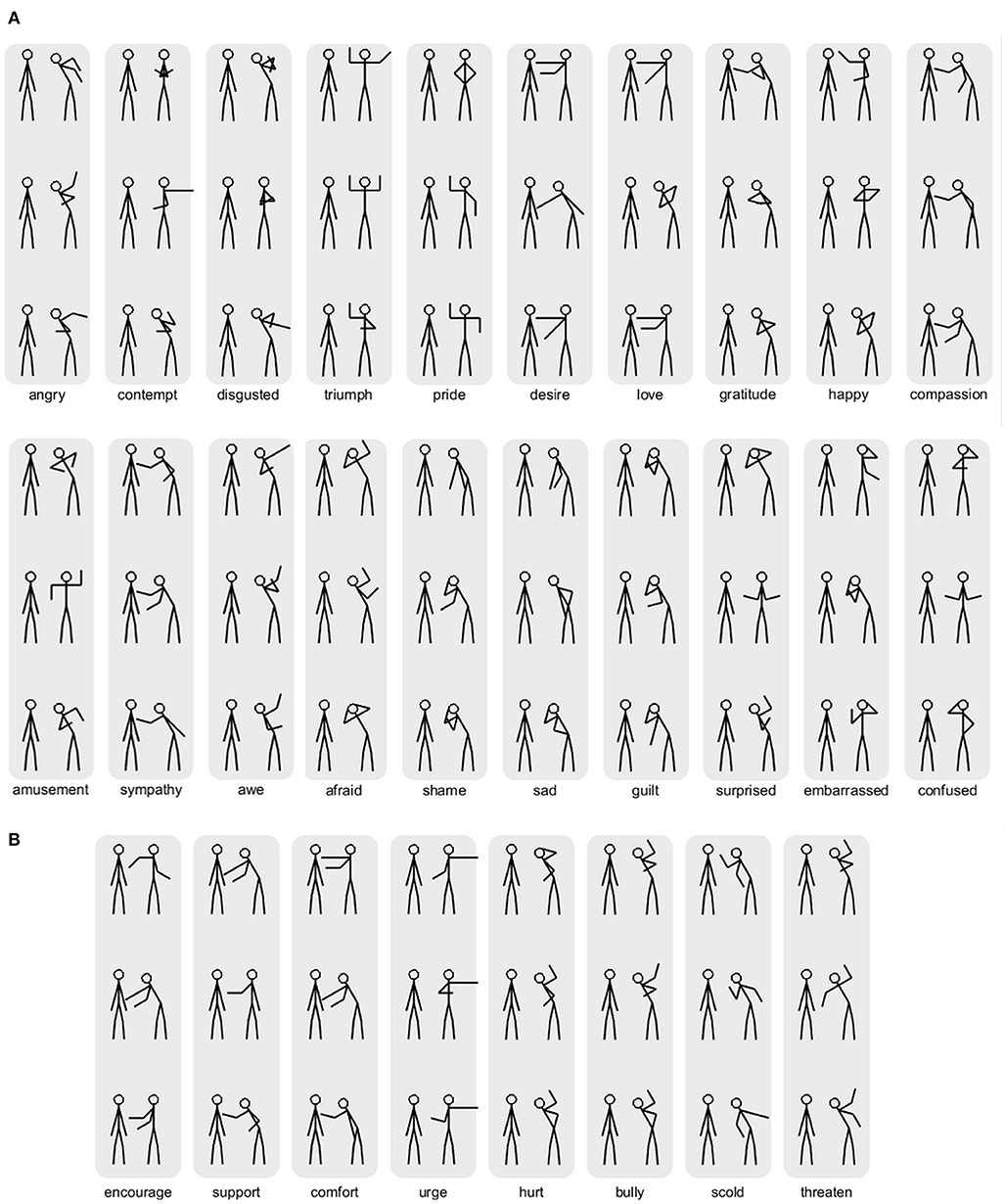
Figure 5. Top images for each emotion and dramatic action. (A) The three top-scoring images for each emotion. For afraid (all top three images) and for the third strongest of happy and pride, the top images did not exceed a median score of 50. (B) The three top-scoring images for each dramatic action.
We next consider the DAs assigned to the SF configurations (Figures 4C,D). We find that negative DAs are found primarily with the torso tilted toward the other (Figure 4C), especially with arms held high and away from the other in a W-like shape (Figure 4H). Another region with negative DAs has an arm directed toward the other with the elbow at 90° (Figure 4I, left). Interestingly, a move of a single angle (elbow) in this region changes the DA to a positive one (Figure 4I, right). This is an example of a small angle change that generates the opposite meaning. With an upright torso, negative DAs are found, for example, in a stripe with one arm pointing directly at the other, perhaps in a symbolic gesture (Figure 4F).
Positive DAs are elicited primarily by upright SFs with at least one arm toward the other, with an elbow angle of more than 90° (Figure 4J).
The DA urge, which has a neutral valence, is primarily elicited by SFs with one arm pointing horizontally away from the other SF (Figure 4K). This may correspond to a symbolic representation. The three SFs with the highest scores for each DA are shown in Figure 5B.
Although DAs were identified in more images than emotions, there are cases in which emotions were identified but not DAs. These include 33 images identified with the emotion surprised. Many of these images show SFs with at least one hand touching the head (Figures 4B,G). This may correspond to a symbolic representation of surprise. Another class of SFs was identified with the emotions angry and disgusted and with no DA or the DA scold. These configurations include SFs with arms crossed (Figures 4L, 5). Thus, emotion and DA can serve as complementary approaches to understanding dyadic images.
For completeness, we show in the SI two additional versions of this figure. The first version (Supplementary Figure 10) shows the strongest emotions and DAs for all SFs, not just those with a score higher than 50. The second version (Supplementary Figure 11) shows the second strongest word for each stick figure.
Discussion
To study the meaning of body language in terms of emotion and dramatic action, we developed a method to generate a large and unbiased dataset of body postures: a systematic scan of stick figure configurations, in a dyad where one stick figure had varying upper body configurations and the other had a neutral stance. We asked the online participants to score the images in terms of 20 emotions and eight dramatic action verbs. We find that 40% of the images were scored with dramatic actions and 29% were scored with emotions. All eight dramatic actions were found in many different images, but among the emotions, angry, confused and embarrassed were much more common than the others. We conclude that both emotions and dramatic actions are needed to understand body language. Thus, dramatic actions, which are verbs that describe the effort to change the state of another, can add to our set of concepts for the meanings of body language and complement the more widely tested emotions.
This unbiased scan of stick figure configurations confirms that stick figures are evocative stimuli: a dramatic action or emotion was identified in 54% of the images. This agrees with previous research that shows that people are able to make sense of stick figures or other schematic representations of agents [6, 34–36]. The full dictionary of the 1,470 stick figure images and their dramatic action and the scores of the six basic emotions is provided in the SI (Supplementary Figure 12).
Dramatic actions elicited somewhat stronger and more expressive signal than emotions in the present dataset. This may be due to the focus on body configuration rather than faces. Faces are the stimuli most commonly used to study the expression of emotion [2, 37–42]. The present list of eight dramatic action verbs can be extended to include additional dramatic actions, for example, from the list of 150 dramatic actions complied in Liron et al. [16].
The systematic scan of body configurations allowed us to ask about the continuity of the relationship between body coordinates and emotions/dramatic actions. We find that similar stick figure configurations often have similar dramatic actions (Figures 4C,D). A wide class of configurations with the arms raised, especially with elbows at sharp angles (W-configurations, Figure 4H), are identified with negative dramatic actions such as bully and threaten [43]. These configurations hint at tensed muscles. Positive dramatic actions are identified most often for configurations in which there is an arm toward the other stick figure with an elbow angle above 90° (Figure 4J).
There are interesting boundaries in the stick figure configuration space in which changing a single angle can change the dramatic action from positive to negative valence. For example, on the arm directed toward the other, changing the elbow angle [44] from 135° (low hand) to 90° (raised hand) can change the dramatic action valence from positive to negative (Figure 4I). In other cases, the configurations seem to resemble symbols, where there are one or two angles whose precise value is essential for the meaning (changing these angles changes the scoring), while other angles can be changed widely without a change in the scoring. For example, configurations in which one arm touches the head are identified with surprise, regardless of choices of the other angles (Figure 4G). One arm directed horizontally toward the other stick figure or hands crossed away from the other stick figure are often identified with anger (Figure 4F), whereas an arm directed horizontally away from the other stick figure is identified with the dramatic actions urge, despite changes in the other angles (Figure 4K).
The stick figures with negative dramatic actions most often (83%) had the torso leaning toward the other stick figure. Previous studies have shown contrasting findings regarding the emotional valence of leaning forward, which was identified with positive [45] or negative [34, 46, 47] valence. In comparing these results, it is important to note that the present study uses an unbiased scan of stick figure configurations, which may include configurations not sampled in other studies.
How do dramatic actions work? How does body language act to change the state of the other? One might speculate about at least two possible mechanisms, one for situations of cooperation and the other for those of competition. In a cooperative mode, dramatic actions may rely in part on the human tendency to mirror the other [48–50]. Dramatic actions can work by assuming a body configuration similar to that desired in the other, hoping to entrain the muscular system of the other [51–53]. For example, the dramatic action comfort aims to reduce the arousal and negative emotion of the other. The stick figures with this dramatic action have arms at a low position, which indicates relaxed muscles. If the entrainment is successful, the other will also relax their muscles, hopefully inducing a relaxed emotional state. In real life, to perform this dramatic action, one tends to relax the chest muscles by breathing deeply and lowering the pitch of voice, and so on.
In a competitive mode, negative dramatic actions may work by enlarging the body, a common strategy used by animals to appear more formidable [54]. An example is the W-configuration stick figures with the arms raised above the shoulder and away from the other (Figure 4H). Negative dramatic actions also involve configurations with activated muscles in the arms and torso [Figures 4H,I (left)], which may indicate readiness to attack.
In this study, the dataset of stick figures is limited in order to maintain a feasible number of configurations. The study focuses only on variations in the upper body, with a constant distance between the figures, and in each dyad the receiving stick figure is kept neutral. The study can be extended to a wider range of stick figure space, including changes in the configuration of the second stick figure in the dyad, changes in distance between the stick figures, changes in leg coordinates, and scanning more values for each angle. This can impact the range of dramatic actions and emotions found. For example, adding changes in the leg coordinates can allow kneeling figures, which can access dramatic actions with aspects of dominance such as “to beg” and “to flatter.” Examples of such stick figures are provided in the SI (Supplementary Figure 13). Adding features such as facial expression, wrists, fingers, or indicators of gaze direction is also needed for a more complete study. Using realistic silhouettes instead of stick figures can help viewers better understand 3D postures. The study can be extended to include motion, which adds important information for the perception of action [55–61]. This study used respondents from one country (United States), and testing in other countries can help resolve how culture can make a difference [62, 63], for example, in symbols.
The present methodology allows further research to address several questions. How would the results change if the neutral stick figure were not present? What happens if participants are able to manipulate the pose directly, perhaps with a prompt to produce a particular emotion or dramatic actions? Could one direct human actors to create particular emotion/action states and then capture these states using machine vision tools? If so, would these states overlap with the states identified in the current manuscript? Another interesting question is whether the semantic relationship in the space of words corresponds to relationships in the space of stick figures. One such analysis could compute the distance between the words using WordNet or other word embeddings and check if this distance is related to distances in the space of stick figures.
We hope that the present dataset serves as a resource for further studies, for example, as stimuli for neuroscience experiments on the perception of dramatic actions or for training human recognition of dramatic actions. The top-scoring images for each dramatic action and emotion can be used as potentially strong stimuli, and the stick figures with weaker scores can be used for tests of individual differences in perception. The dictionary can also be used to label images or videos with dramatic actions and emotions by extracting stick-figure skeletons from the images using pose estimation algorithms and matching them to the present stick figure collection. It would be fascinating to learn which brain circuits perceive dramatic actions, and what cultural, situational, and individual factors contribute to how people make sense of body language.
Data Availability Statement
The datasets generated for this study can be found in online repositories. The names of the repository/repositories and accession number(s) can be found in the article/Supplementary Material.
Ethics Statement
The studies involving human participants were reviewed and approved by the institutional review board of the Weizmann Institute of Science, Rehovot, Israel. The participants provided their written informed consent to participate in this study.
Author Contributions
NR, YL, and UA designed the study, wrote and revised the manuscript. NR carried out online surveys. NR and YL wrote the software and analyzed the data. All authors contributed to the article and approved the submitted version.
Funding
Braginsky Center for the Interface between Science and the Humanities, at the Weizmann Institute of Science. The funder had no role in the design or conduct of this research.
Conflict of Interest
The authors declare that the research was conducted in the absence of any commercial or financial relationships that could be construed as a potential conflict of interest.
Acknowledgments
The authors thank the Braginsky Center for the Interface between Science and the Humanities, at the Weizmann Institute of Science, for support. The authors thank Yuval Hart, Yael Korem, Avi Mayo, Ruth Mayo, Michal Neeman, Lior Noy, Tzachi Pilpel, Itamar Procaccia, Hila Sheftel, Michal Shreberk, Daniel Zajfman, and the members of the Alon lab for fruitful discussions and their comments on the manuscript.
Supplementary Material
The Supplementary Material for this article can be found online at: https://www.frontiersin.org/articles/10.3389/fphy.2021.664948/full#supplementary-material
References
1. de Gelder B. Towards the neurobiology of emotional body language. Nat Rev Neurosci. (2006) 7:242–9. doi: 10.1038/nrn1872
2. de Gelder B. Why bodies? Twelve reasons for including bodily expressions in affective neuroscience. Philos Trans R Soc B Biol Sci. (2009) 364:3475–84. doi: 10.1098/rstb.2009.0190
3. Kret ME, Pichon S, Grèzes J, de Gelder B. Similarities and differences in perceiving threat from dynamic faces and bodies. An fMRI study. Neuroimage. (2011) 54:1755–62. doi: 10.1016/j.neuroimage.2010.08.012
4. Metallinou A, Lee CC, Busso C, Carnicke S, Narayanan, et al. The USC CreativeIT database: a multimodal database of theatrical improvisation. In: Multimodal Corpora: Advances in Capturing, Coding and Analyzing Multimodality. Valletta: Springer (2010). p. 55.
5. Beck A, Cañamero L, Hiolle A, Damiano L, Cosi P, Tesser F, et al. Interpretation of emotional body language displayed by a humanoid robot: a case study with children. Int J Soc Robot. (2013) 5:325–34. doi: 10.1007/s12369-013-0193-z
6. Beck A, Stevens B, Bard KA, Cañamero L. Emotional body language displayed by artificial agents. ACM Trans Interact Intell Syst. (2012) 2:1–29. doi: 10.1145/2133366.2133368
7. Poria S, Cambria E, Bajpai R, Hussain A. A review of affective computing: from unimodal analysis to multimodal fusion. Inf Fusion. (2017) 37:98–125. doi: 10.1016/j.inffus.2017.02.003
8. Zeng Z, Pantic M, Roisman GI, Huang TS. A survey of affect recognition methods: audio, visual, and spontaneous expressions. IEEE Trans Pattern Anal Mach Intell. (2009) 31:39–58. doi: 10.1109/TPAMI.2008.52
9. Calvo RA, D'Mello S. Affect detection: an interdisciplinary review of models, methods, and their applications. IEEE Trans Affect Comput. (2010) 1:18–37. doi: 10.1109/T-AFFC.2010.1
10. Karg M, Samadani AA, Gorbet R, Kühnlenz K, Hoey J, Kulić D, et al. Body movements for affective expression: a survey of automatic recognition and generation. IEEE Trans Affect Comput. (2013) 4:341–59. doi: 10.1109/T-AFFC.2013.29
11. Kleinsmith A, Bianchi-Berthouze N. Affective body expression perception and recognition: a survey. IEEE Trans Affect Comput. (2013) 4:15–33. doi: 10.1109/T-AFFC.2012.16
12. Darwin C. The Expression of the Emotions in Man and Animals. London: John Murray (1872). doi: 10.1037/10001-000
13. Meeren HKM, van Heijnsbergen CCRJ, de Gelder B. Rapid perceptual integration of facial expression and emotional body language. Proc Natl Acad Sci USA. (2005) 102:16518–23. doi: 10.1073/pnas.0507650102
14. Gliga T, Dehaene-Lambertz G. Structural encoding of body and face in human infants and adults. J Cogn Neurosci. (2005) 17:1328–40. doi: 10.1162/0898929055002481
16. Liron Y, Raindel N, Alon U. Dramatic action: a theater-based paradigm for analyzing human interactions. PLoS ONE. (2018) 13:e0193404. doi: 10.1371/journal.pone.0193404
19. Ryan RM, Deci EL. Intrinsic and extrinsic motivations: classic definitions and new directions. Contemp Educ Psychol. (2000) 25:54–67. doi: 10.1006/ceps.1999.1020
22. Aggarwal JK, Cai Q. human motion analysis: a review. Comput Vis Image Underst. (1999) 73:428–40.
23. Downing PE. A cortical area selective for visual processing of the human body. Science (80-.). (2001) 293:2470–3. doi: 10.1126/science.1063414
24. Herman M. Understanding Body Postures of Human Stick Figures. College Park, MD: University of Maryland at College Park (1979).
25. Roebuck JA, Kroemer KHE, Thomson WG. Engineering anthropometry methods. Aeronaut J. (1975) 79:555.
26. Buhrmester M, Kwang T, Gosling SD. Amazon's mechanical turk: a new source of inexpensive, yet high-quality, data? Perspect Psychol Sci. (2011) 6:3–5. doi: 10.1177/1745691610393980
27. Schindler K, Van Gool L, de Gelder B. Recognizing emotions expressed by body pose: a biologically inspired neural model. Neural Networks. (2008) 21:1238–46. doi: 10.1016/j.neunet.2008.05.003
28. Keltner D, Cordaro DT. Understanding multimodal emotional expressions: recent advances in basic emotion theory. In: The Science of Facial Expression. New York, NY: Oxford University Press (2017). p. 57–75.
29. Cowen AS, Keltner D. Self-report captures 27 distinct categories of emotion bridged by continuous gradients. Proc Natl Acad Sci USA. (2017) 114:E7900–9. doi: 10.1073/pnas.1702247114
30. Haidt J. The moral emotions. In: Davidson RJ, Scherer KR, Goldsmith HH, editors. Handbook of Affective Sciences. Oxford: Oxford University Press (2003). p. 852–70.
31. Plant EA, Hyde JS, Keltner D, Devine PG. The Gender Stereotyping of Emotions. Psychol Women Q. (2000) 24:81–92. doi: 10.1111/j.1471-6402.2000.tb01024.x
32. Gunes H, Piccardi M. Bi-modal emotion recognition from expressive face and body gestures. J Netw Comput Appl. (2007) 30:1334–45. doi: 10.1016/j.jnca.2006.09.007
33. Pollick FE, Paterson HM, Bruderlin A, Sanford AJ. Perceiving affect from arm movement. Cognition. (2001) 82:B51–61. doi: 10.1016/S0010-0277(01)00147-0
34. Coulson M. Attributing emotion to static body postures: recognition accuracy, confusions, and viewpoint dependence. Nonverbal Behav J. (2004) 28:117–39. doi: 10.1023/B:JONB.0000023655.25550.be
35. Heider F, Simmel M. An experimental study of apparent behavior. Am J Psychol. (1944) 57:243. doi: 10.2307/1416950
36. Kleinsmith A, Bianchi-Berthouze N. Recognizing affective dimensions from body posture. In: Paiva ACR, Prada R, Picard RW, editors. Affective Computing Intelligent Interaction: Second International Conference, ACII 2007 Lisbon, Portugal, September 12-14, 2007 Proceedings, Berlin: Springer (2007). p. 48–58.
37. Izard CE. Facial expressions the regulation of emotions. J Pers Soc Psychol. (1990) 58:487. doi: 10.1037/0022-3514.58.3.487
38. Rosenberg EL, Ekman P. Coherence between expressive and experiential systems in emotion. Cogn Emot. (1994) 8:201–29. doi: 10.1080/02699939408408938
39. Matsumoto D, Keltner D, N.Shiota M, O'Sullivan M, Frank M. Facial expressions of emotion. In: Handbook of Emotions. New York, NY: The Guilford Press (2008). p. 211–34.
40. Du S, Tao Y, Martinez AM. Compound facial expressions of emotion. Proc Natl Acad Sci USA. (2014) 111:E1454–62. doi: 10.1073/pnas.1322355111
41. Durán JI, Reisenzein R, Fernández-Dols J-M. Coherence between emotions and facial expressions: a research synthesis. In: The Science of Facial Expression. New York, NY: Oxford University Press (2017). p. 107–29.
42. Rosenberg EL, Ekman P. What the Face Reveals: Basic and Applied Studies of Spontaneous Expression Using the Facial Action Coding System (FACS). New York, NY: Oxford University Press (2020).
43. Aronoff J, Woike BA, Hyman LM. Which are the stimuli in facial displays of anger happiness? Configurational bases of emotion recognition. J Pers Soc Psychol. (1992) 62:1050–66. doi: 10.1037/0022-3514.62.6.1050
44. Ben-Yosef G, Assif L, Ullman S. Full interpretation of minimal images. Cognition. (2018). 171:65–84. doi: 10.1016/j.cognition.2017.10.006
45. Schouwstra SJ, Hoogstraten J. Head position and spinal position as determinants of perceived emotional state. Percept Mot Skills. (1995) 81:673–4. doi: 10.1177/003151259508100262
46. Harrigan JA, Rosenthal R. Physicians' head and body positions as determinants of perceived rapport. J Appl Soc Psychol. (1983) 13:496–509. doi: 10.1111/j.1559-1816.1983.tb02332.x
47. Mehrabian A, Friar JT. Encoding of attitude by a seated communicator via posture position cues. J Consult Clin Psychol. (1969) 33:330–6. doi: 10.1037/h0027576
48. Rizzolatti G, Fadiga L, Gallese V, Fogassi L. Premotor cortex and the recognition of motor actions. Cogn Brain Res. (1996) 3:131–41. doi: 10.1016/0926-6410(95)00038-0
49. Hasson U, Frith CD. Mirroring beyond: coupled dynamics as a generalized framework for modelling social interactions. Philos Trans R Soc B Biol Sci. (2016) 371:20150366. doi: 10.1098/rstb.2015.0366
50. Noy L, Dekel E, Alon U. The mirror game as a paradigm for studying the dynamics of two people improvising motion together. Proc Natl Acad Sci USA. (2011) 108:20947–52. doi: 10.1073/pnas.1108155108
51. Huis in ‘t Veld EMJ, van Boxtel GJM, de Gelder B. The Body Action Coding System II: muscle activations during the perception and expression of emotion. Front Behav Neurosci. (2014) 8:330. doi: 10.3389/fnbeh.2014.00330
52. Hess U, Fischer A. Emotional mimicry as social regulation. Personal Soc Psychol Rev. (2013) 17:142–57. doi: 10.1177/1088868312472607
53. Dimberg U, Thunberg M, Elmehed K. Unconscious facial reactions to emotional facial expressions. Psychol Sci. (2000) 11:86–9. doi: 10.1111/1467-9280.00221
55. Atkinson AP, Dittrich WH, Gemmell AJ, Young AW. Emotion perception from dynamic and static body expressions in point-light and full-light displays. Perception. (2004) 33:717–46. doi: 10.1068/p5096
56. Giese MA, Poggio T. Cognitive neuroscience: neural mechanisms for the recognition of biological movements. Nat Rev Neurosci. (2003) 4:179–92. doi: 10.1038/nrn1057
57. Barraclough NE, Xiao D, Oram MW, Perrett DI. The sensitivity of primate STS neurons to walking sequences and to the degree of articulation in static images. Prog Brain Res. (2006) 154:135–48. doi: 10.1016/S0079-6123(06)54007-5
58. Bruce C, Desimone R, Gross CG. Visual properties of neurons in a polysensory area in superior temporal sulcus of the macaque. Neurophysiol J. (1981) 46:369–84. doi: 10.1152/jn.1981.46.2.369
59. Jellema T, Perrett DI. Neural representations of perceived bodily actions using a categorical frame of reference. Neuropsychologia. (2006) 44:1535–46. doi: 10.1016/j.neuropsychologia.2006.01.020
60. Oram MW, Perrett DI. Responses of Anterior Superior Temporal Polysensory (STPa) Neurons to “Biological Motion” Stimuli. J Cogn Neurosci. (1994) 6:99–116. doi: 10.1162/jocn.1994.6.2.99
61. Goldberg H, Christensen A, Flash T, Giese MA, Malach R. Brain activity correlates with emotional perception induced by dynamic avatars. Neuroimage. (2015) 122:306–17. doi: 10.1016/j.neuroimage.2015.07.056
62. de Gelder B, In 'T Veld EMJ. Cultural differences in emotional expressions and body language. In: Chiao JY, Li SC, Seligman R, Turner R, editors, The Oxford Handbook of Cultural Neuroscience. Oxford: Oxford University Press (2015) doi: 10.1093/oxfordhb/9780199357376.013.16
Keywords: social neuroscience, emotional body language, social psychology, human-computer interaction, emotion elicitation, physics of behavior, psychophysics
Citation: Raindel N, Liron Y and Alon U (2021) A Study of Dramatic Action and Emotion Using a Systematic Scan of Stick Figure Configurations. Front. Phys. 9:664948. doi: 10.3389/fphy.2021.664948
Received: 06 February 2021; Accepted: 23 April 2021;
Published: 07 June 2021.
Edited by:
Orit Peleg, University of Colorado Boulder, United StatesReviewed by:
Greg Stephens, Vrije Universiteit Amsterdam, NetherlandsYen-Ling Kuo, Massachusetts Institute of Technology, United States
Moti Fridman, Bar Ilan University, Israel
Copyright © 2021 Raindel, Liron and Alon. This is an open-access article distributed under the terms of the Creative Commons Attribution License (CC BY). The use, distribution or reproduction in other forums is permitted, provided the original author(s) and the copyright owner(s) are credited and that the original publication in this journal is cited, in accordance with accepted academic practice. No use, distribution or reproduction is permitted which does not comply with these terms.
*Correspondence: Uri Alon, uri.alon@weizmann.ac.il