- 1Department of Medical Physics, Faculty of Physics, Ludwig-Maximilians-Universität München, Garching, Germany
- 2Centre Antoine Lacassagne, Nice, France
- 3Department of Medical Physics, Memorial Sloan Kettering Cancer Center, New York, NY, United States
- 4Rinecker Proton Therapy Center, Munich, Germany
- 5University Hospital, Ludwig-Maximilians-Universität München, Munich, Germany
A novel irradiation platform for preclinical proton therapy studies foresees proton imaging for accurate setup and treatment planning. Imaging at modern synchrocyclotron-based proton therapy centers with high instantaneous particle flux is possible with an integration mode setup. The aim of this work is to determine an object’s water-equivalent thickness (WET) with a commercially available large-area CMOS sensor. Image contrast is achieved by recording the proton energy deposition in detector pixels for several incoming beam energies (here, called probing energies) and applying a signal decomposition method that retrieves the water-equivalent thickness. A single planar 114 mm × 65 mm CMOS sensor (49.5 µm pixel pitch) was used for this study, aimed at small-animal imaging. In experimental campaigns, at two isochronous cyclotron-based facilities, probing energies suitable for small-animal-sized objects were produced once with built-in energy layer switching and the other time, using a custom degrader wheel. To assess water-equivalent thickness accuracy, a micro-CT calibration phantom with 10 inserts of tissue-mimicking materials was imaged at three phantom-to-detector distances: 3 mm, 13 mm, and 33 mm. For 3 mm and 13 mm phantom-to-detector distance, the average water-equivalent thickness error compared to the ground truth was about 1% and the spatial resolution was 0.16(3) mm and 0.47(2) mm, respectively. For the largest separation distance of 33 mm air gap, proton scattering had considerable impact and the water-equivalent thickness relative error increased to 30%, and the spatial resolution was larger than 1.75 mm. We conclude that a pixelated CMOS detector with dedicated post-processing methods can enable fast proton radiographic imaging in a simple and compact setup for small-animal-sized objects with high water-equivalent thickness accuracy and spatial resolution for reasonable phantom-to-detector distances.
1 Introduction
To advance preclinical proton therapy research, the SIRMIO project [1] (small-animal proton irradiator for research in molecular image-guided radiation-oncology) aims to build a small-animal proton irradiator equipped with a dedicated beamline to produce a focused, narrow proton beam (σ ≈ 1 mm) with energies from 20 MeV to 50 MeV to irradiate small animals [2]. Imaging for tumor detection, treatment planning, and positioning will be provided by co-registered ultrasound [3] and proton imaging, with the advantage that proton radiography will directly provide the water-equivalent thickness (WET) and proton computed tomography (pCT), the relative (to water) stopping power (RSP) needed for treatment planning [4–6]. Ionoacoustic measurements [3] and an in-beam PET system [7] are used for in vivo range verification.
Three proton imaging modes are foreseen to enable imaging at different treatment centers and applications. A full single-particle tracking tomographic system is developed in-house and will provide tomographic images of the RSP for treatment planning. It consists of floating strip Micromegas front and rear trackers [8] before and after the object to measure the position and direction of individual protons and a time-projection-chamber based on a range telescope to measure the proton’s residual range. Initial simulation studies of the expected performance of the proton tomography prototype suggest improved range predictions in treatment planning compared to conventional (X-ray) planning CT [9].
At synchrocyclotron-based facilities, the proton flux within the microbunches exceeds the particle rate capability of single-particle tracking detectors, limited to 7 MHz cm−2 [8]. As an alternative, imaging behind the object, either based on single-particle dE-measurement with Timepix detectors [10] or integration mode [11–15] using a large-area CMOS sensor, and energy stacking, is developed in parallel to be used in the SIRMIO prototype.
Even though a full tomography in integration mode might be unreasonable due to the acquisition time and dose restrictions, radiographic proton images of the animal can be used for positioning, to do a specific Hounsfield unit (HU) to RSP conversion [16] of the previously recorded tomographic image (typically X-ray cone-beam CT (CBCT)) or the deformation of an X-ray or proton CT with a deformable image registration (DIR) to the daily anatomy as suggested [17]. This work reports on the optimal acquisition settings for the CMOS integration mode that have been determined both experimentally and via Monte Carlo (MC) simulations. Compared to other proton imaging systems, this integration mode setup is portable and compact and is, therefore, especially interesting when the space in the experimental or treatment room is constrained.
In the following, we will present the experimental design of CMOS integration mode proton imaging at two different proton therapy beamlines and the obtained results, which motivate further optimization of the imaging system and post-processing of the acquired data for future applications in SIRMIO.
2 Materials and methods
2.1 Principle of energy stacking
To measure the WET of an object, the energy deposition in the thin sensitive layer of a detector is recorded for the varying incident beam energy, hereafter, also referred to as probing energy. The scanning of the object with several probing energies ensures that the measurement points at high gradients of energy loss per traversed depth in materials are available for the WET determination (see Figure 1A; Supplementary Video S1) for each WET present in the object.
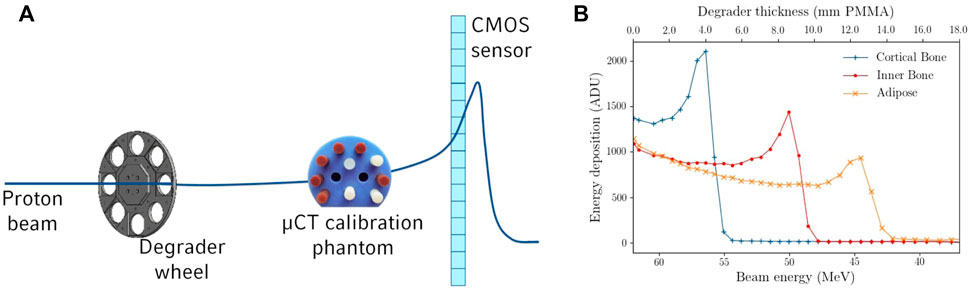
FIGURE 1. (A) Schematic of the experimental setup including a degrader wheel, micro-CT calibration phantom, and CMOS detector. A variable degrader like the depicted wheel is necessary at facilities with fixed proton beam energies. (B) Detector signal corresponding to three different materials of the phantom at varying probing energies or degrader thicknesses (after 62.3 MeV initial energy), respectively.
2.2 Experimental setup
For the experimental implementation of proton imaging in integration mode with a CMOS detector, the energy deposition for all probing energies in the pixelated, integrating detector is recorded (see Figure 2A), resulting in one “raw” image per probing energy. The range of probing energies is adapted to the maximum and minimum WET of the object, which is presumed to range from 0.3 cm to 3.5 cm for a murine specimen or a comparable object. The corresponding highest imaging energy is 65 MeV and the minimum applied in an experiment was about 4 MeV as structures of lower WET, e.g., mouse ears and claws, are of minor interest. The difference between subsequent energy steps can be chosen to find a compromise between the desired WET accuracy and imaging dose.
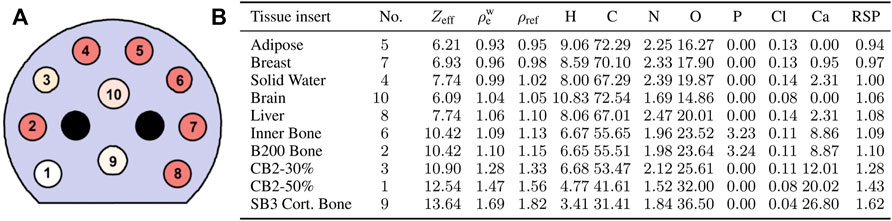
FIGURE 2. (A) Depiction of the micro-CT calibration phantom and (B) tabulation of its related properties, according to the manufacturer (SmART Scientific Solutions, Netherlands): the effective atomic number Zeff, relative-to-water electron density
Experiments were conducted at two different proton therapy centers, employing two different methods to produce the probing energies: the Rinecker Proton Therapy Centre (RPTC) and Centre Antoine Lacassagne (CAL).
2.2.1 Rinecker Proton Therapy Center
The RPTC in Munich, Germany, was equipped with the first cyclotron intended for particle therapy by Varian Medical Systems, Inc. (Palo Alto, California, formerly Accel) in a clinical facility [18]. The superconducting cyclotron and beamline with an automatic energy switching system delivered protons within 75 MeV–245 MeV and switching time of about 4 s to four dosimetrically equivalent treatment rooms with gantries.
2.2.2 Centre Antoine Lacassagne
At CAL in Nice, France, two proton therapy cyclotrons are in clinical operation. One is the prototype Proteus®ONE (IBA, Netherlands) consisting of a superconducting synchrocyclotron and a rotating gantry, which is used to treat deeper tumors such as head and neck or sacrum cancers. The other cyclotron, Medicyc, is used for the treatment of ocular cancers and provides a fixed energy of 62.3 MeV [19–21]. Due to easier accessibility, the presented experiments were carried out at the isochronous Medicyc cyclotron.
2.2.3 CMOS sensor
A commercially available CMOS sensor (Teledyne DALSA, Canada) with a 49.5 µm pixel pitch, a few µm thicknesses of the silicon sensitive layer, and 65 mm × 114 mm sensor area was used1. Through its experimental application at the Centre for Advanced Laser Applications (CALA) in Garching [22], first experience with the detector system was available and the sensor area was deemed sufficient for the coverage of a mouse. Images were acquired with 100 ms exposure time. Depending on the treatment facility where the experiments were performed, the data for all initial beam energies were either taken in continuous readout including empty frames during the automated energy switching of the proton beam delivery system or as separate batches of images. The detector has a 14-bit dynamic range, and the signal intensity in each pixel (proportional to the integrated energy deposition) is given in analog-to-digital units (ADU). The sensor response shows linearity within 3.9% in the range of 0 MeV–21 MeV. Measurements were performed at the Maier-Leibnitz Laboratory tandem accelerator in Garching.
2.2.4 Phantom
For the quantitative evaluation of the acquired proton images, a micro-CT calibration phantom (SmART Scientific Solutions, Netherlands) was imaged. This phantom is made from a 10 mm-thick water-equivalent baseplate with a 30-mm diameter that holds 10 tissue-equivalent inserts (Gammex® materials; 3.5 mm diameter; 16 mm length). The elemental composition by relative weight, effective atomic number Zeff, mass density ρref, and relative-to-water electron density
2.2.5 Radiography experiments
At RPTC, degrader and detector were mounted on the treatment table (see Figure 3). The micro-CT calibration phantom was imaged with a phantom-to-detector distance of 13 mm. The automated energy switching made energy variation possible by executing a treatment plan with a single central spot. In this example, a treatment plan with 2 MeV energy steps from 76 MeV to 96 MeV was irradiated and degraded down to 20 MeV–65 MeV with a 35-mm-thick block of polymethyl methacrylate (PMMA) placed at the isocenter. The RSP of the PMMA block was determined at the Heidelberg Ion Therapy Centre (HIT) to be 1.165 (see section 5). The frames that were recorded during the beam-off time for energy switching were used to subtract the detector’s dark current from the beam-on frames. The drift space between the degrader and the detector was 220 cm for a more homogeneous and reduced proton fluence on the detector (see Figure 3 and section 5).

FIGURE 3. Experimental setup at the RPTC with a PMMA block as the energy degrader on the left and the CMOS detector in its housing on the right.
At CAL, the aforementioned phantom was imaged for three different phantom-to-detector distances: 3 mm, 13 mm, and 33 mm (see Figure 4B) (with 3 mm being the minimal possible distance). The distance between the beam exit and the detector was 125 cm.
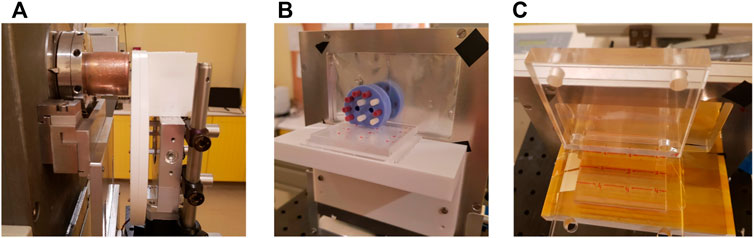
FIGURE 4. Experimental setup at CAL: (A) 3D-printed degrader wheel mounted directly after the collimator at the beam exit. (B) Detector and SmART calibration phantom. The black patches block light from entering through screw holes that lie underneath. (C) Detector and 10 mm-thick PMMA slab for calibration.
The 62.3-MeV beam of the Medicyc cyclotron is degraded for proton imaging with a motorized degrader wheel (see Figure 4A), which holds seven PMMA slabs with thickness ranging from 0.5 mm to 3.5 mm in a 3D-printed frame. Each segment has a diameter of 34 mm, adapted to the size of the collimator at beam exit in the treatment room. Up to seven PMMA slabs of 4 mm can be inserted in an additional holder directly behind the wheel at the height of the beam exit, so that the available range in PMMA thickness is from 0 mm to 31.5 mm in steps of 0.5 mm. The RSP of this material was measured to be 1.147 (see section 5). The remaining proton beam energy, therefore, ranges from 5 MeV to 62 MeV according to FLUKA MC simulations (see section 2.3.2).
2.3 Determination of the water-equivalent thickness
For each pixel, the detector signal is recorded as a function of the irradiated probing energies. The signal height displays the energy deposition Edep of the proton beam after having traversed the part of the object that is upstream of the pixel (see Figure 1B). In the case of a homogeneous medium, there is only one Bragg peak position and corresponding WET value of the object to be identified. In more realistic experiments with heterogeneous objects and due to multiple Coulomb scattering, there are different WET components contributing to the integrated signal in each pixel. For accurate WET retrieval, the measured signal must be taken apart into the contributions from several WET values in the object, which is carried out using the Bragg peak decomposition method.
2.3.1 Bragg peak decomposition
To exploit the Bragg peak decomposition for integration mode imaging with a CMOS detector, a reference of this detector’s signal for a series of known WET values is needed.
In this work, this reference is obtained through the measurement of the homogeneous base part of the SmART phantom in the case of the experiments at the RPTC (see section 2.3.2) and through measurements of PMMA plates with known WET for the CAL experiments (see section 2.3.2 and Figure 4C). Due to the limited time at experimental facilities, it is not possible to measure a calibration curve for every WET value needed in the reconstruction. To compensate for this and obtain reference curves at finer WET resolution available for signal decomposition, calibration measurements need to be complemented by FLUKA MC simulations. These simulations are first fine-tuned to reproduce the open-field measurements (see section 5); they are then compared to the calibration measurements and in the last step are used to generate the full lookup table (LUT). The LUT is an m × n matrix of the energy deposition in the detector pixels for each of the m water-equivalent thickness values and n initial beam energies (see Figure 5A).
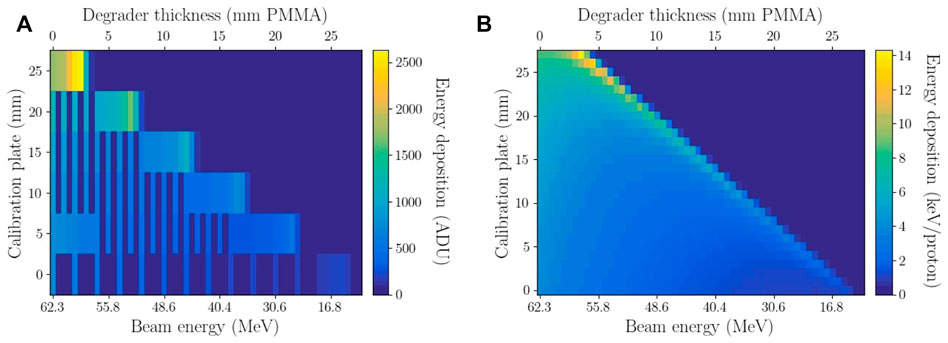
FIGURE 5. Lookup tables from calibration measurements (A) with PMMA plates and (B) complemented by FLUKA MC simulations. Measurements were carried out in steps of 2-mm degrader thickness in the plateau region and with 0.5-mm steps in the Bragg peak region.
The Bragg peak decomposition algorithm retrieves the unknown combination of the WET, given as solution vectors
as described in [25] and [26]. The minimization problem is solved in this work using the function lsq_linear from the scipy. optimize package (scipy version 1.8.0)4 [27].
The signal decomposition method allows for the identification of WET components and their respective weights in the integrated signal. Such an example of the detector signal with range mixing is shown in Figure 6A.
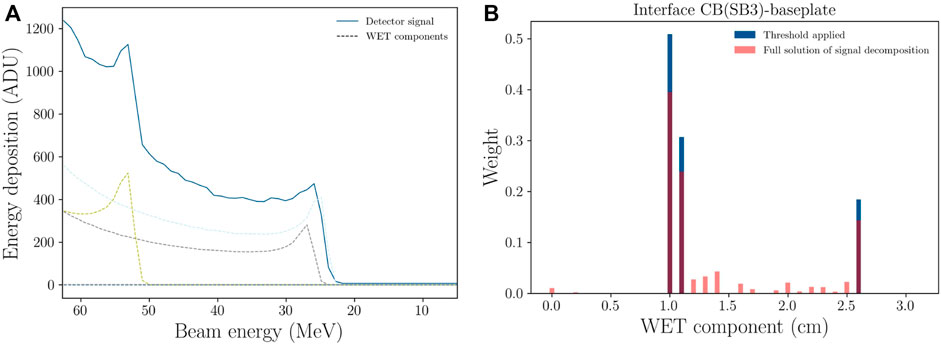
FIGURE 6. Example of the signal decomposition algorithm for a pixel in an interface region between the cortical bone (SB3) insert and the baseplate, leading to range mixing. (A) Measured detector signal in a pixel with a range mixing (solid line). The WET components obtained via Bragg peak decomposition (Figure 6B) are indicated as dashed lines. (B) WET components found as solution corresponding to the signal in (A).
As the solver has no constraints to the maximum of non-zero vector items in
To generate a WET image in 2D, i.e., a radiograph, the WET components in the solution can be combined depending on the application that is foreseen for the image. Conventionally, either the mode of the solution vector WETmax is chosen from the solution vector or the weighted mean of components could be calculated to obtain an image of WETmean [29]. Choosing WETmean for the radiographs presented in this study gives the possibility to reconstruct the radiograph with a smoother WET distribution than tabulated in the LUT, but with a degraded spatial resolution.
2.3.2 Monte Carlo simulations
For the measurements at both proton therapy centers, the experiment was modeled in a FLUKA [30,31] MC simulation (version 2020.0) with the default settings “HADROTHE” (for hadrontherapy). The simulation geometry is set such that the distances between beam exit, degrader, phantom, and detector reflect the experimental setup. The mass density and geometrical dimensions of all present elements were set to the measured values. The ionization potential I was tuned in the simulation so that the range shift measured in the water column (see section 5) was reproduced within 0.022 mm.
2.3.2.1 RPTC beam source
In the case of the measurements at RPTC, a FLUKA beam model [32] based on reference measurements in 2012 and 2014 was available. Measurements recorded in 2019 for 75 MeV nominal energy showed that there has been a shift in the mean beam energy from 75.1 MeV to 74.5 MeV at the isocenter [2], but the energy spread remained the same. As a consequence, the mean beam energies in the beam model were adapted to reproduce the reference measurement as described in section 2.3.2.
2.3.2.2 RPTC calibration
The measured energy deposition in the detector as a function of probing energy behind the baseplate of the phantom described in section 2.2.2 was used as reference to validate the energy deposition obtained from the MC simulation. In the simulation, the energy deposition in the detector model after a water column of 9.94 mm, corresponding to the measured WET of the baseplate, was simulated and compared to the measurement for five different circular regions in the baseplate with 3 mm diameter each. The density and ionization potential of the water column used were adapted to reflect the measurement conditions at the RPTC and, therefore, set to ρw = 0.997 g cm−3 and Ie = 73.5 eV [33]. The systematic uncertainty from this calibration approach is the combined uncertainty from the measured RSP (0.2%) [23] of the calibration phantom, the thickness measurement of the phantom baseplate (0.007 mm), and the remaining uncertainty between the MC and measured proton range of 0.67 mm. The combined systematic uncertainty for the WET determination with the MC model of experiments at the RPTC is, therefore, 0.67 mm or 6.7%.
To generate the LUT, the accumulated energy deposition for each pixel in the modeled detector was scored for 2 × 107 particles for each probing energy and WET values from 0 mm to 30 mm in steps of 1 mm with the USRBIN card in FLUKA.
2.3.2.3 CAL beam source
To reproduce the experiments at CAL in a FLUKA simulation, a phase space produced by Ref. [34] served as the beam source. It was scored using the MC code MCNPX and is read in input using a FLUKA user routine.
The ionization potential of the FLUKA material for the PMMA foil used in the degrader wheel (PMMAWheel) was set to I = 71 eV to reproduce the water column measurement described in section 2.2.3.
The size of the treatment room at CAL did not allow for an air gap between the beam exit and detector as large as that at the RPTC, which is why the spatial fluence distribution for different probing energies, due to change in lateral beam size, cannot be neglected in this case, and a separate LUT for each pixel is scored.
For each of the N = 56 measurement points, which correspond to the energy deposition Edep, meas on the detector for all degrader wheel thicknesses, the simulated energy deposition Edep, sim was compared to the measurement with an average point-to-point difference Δpp of the normalized curves:
Similar to the calibration for the RPTC measurements, such minor differences in the signal shape will be suppressed by the threshold in the signal decomposition and not affect WET determination. The remaining difference in the distal 80% from the maximum energy deposition between measurement and simulation is 0.03 mm in PMMA, which leads to an uncertainty in the WET determination of 0.035 mm.
The spot size in terms of the standard deviation σ of a Gaussian fit to the beam profile in the spot center was reproduced with an agreement better than Δσ = 2 mm in both lateral dimensions.
2.3.2.4 CAL calibration
In the first set of simulations, the Bragg peak shift of the calibration pieces in the PeakFinder measurements at HIT was reproduced in a FLUKA simulation by adjusting the ionization potential of a slice of PMMA, set to the determined thickness and mass density in front of a water column (ρ = 0.998 g cm−3, Ie = 77.3 eV) [35]. As a result of this step, the thickness d, mass density ρ, and ionization potential I were known for every piece of PMMA used in the calibration measurements.
In a second set of simulations, the optimized beam source for CAL and the measured WET for each PMMA slab were used to imitate the calibration measurements with the CMOS detector described in Section 5. Those simulations allow us to estimate the uncertainty in the WET determination for the MC obtained LUT. The uncertainty of the calibration is given by the uncertainty of the PeakFinder range shift and its reproduction in the MC simulation, which is combined 0.022 mm. The error in the reproduction of the proton beam range in the open-field measurement is 0.035 mm. Those errors propagate into the simulations that are carried out for comparison to the calibration measurement. The absolute difference in the proton beam range was at maximum 0.11 mm for all calibration measurements, which leads to an error from the calibration smaller than 1%.
This simulation was then used to produce the LUT displayed in Figure 5B that directly provides the expected energy deposition on the detector after having traversed the water-equivalent thicknesses from 0 mm to 32 mm in steps of 1 mm.
2.4 Evaluation
2.4.1 WET accuracy
The measured WET of the Gammex inserts was compared to the experimentally determined RSP values [23] multiplied by the geometrical length in beam direction of the insert, deemed as the ground truth WET. The regions-of-interest (ROIs) in the middle of the inserts with a margin to the edge were chosen to evaluate the WET accuracy independent of the spatial resolution (see Figure 7A). The margin is chosen depending on the blurring that is present in the image. To evaluate the WET accuracy and precision, the average WET and standard deviation within this region is computed. If the margins to the ROI are chosen too small, then pixels with range mixing are included in the evaluation and the WET accuracy cannot be accessed directly.
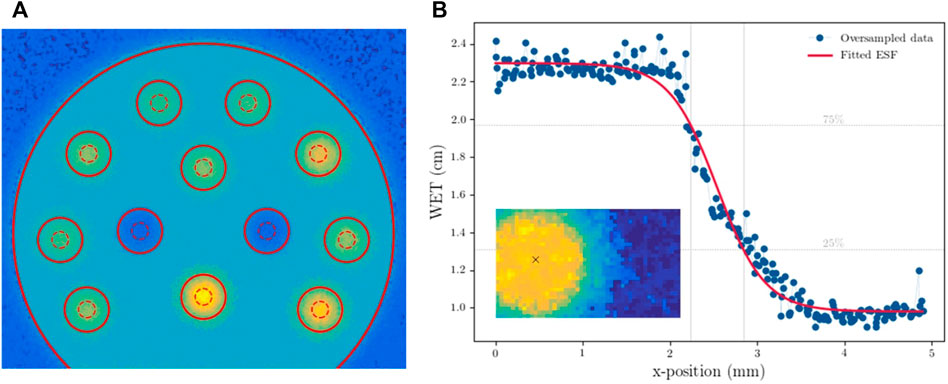
FIGURE 7. (A) Margins from the insert edge (continuous circle) to the evaluated ROI (dotted circle inside) for WET accuracy and precision in a region of the image not blurred by scattering. (B) Combined line profiles at the edge of the SB3 cortical bone insert (depicted on the bottom left) to fit the edge-spread function and calculate the spatial resolution.
2.4.2 Spatial resolution
To quantify the spatial resolution, an oversampled edge-spread function (ESF) at the interface of each Gammex material insert and the solid water baseplate was obtained from nine line profiles in the image around the center of the insert (see Figure 7B). An error function fit to this oversampled ESF was used to determine the modulation transfer function (MTF). A total of 10% of the MTF was calculated for each insert, and the mean and standard values of all inserts are reported.
2.4.3 Imaging dose
To obtain an estimated imaging dose from FLUKA simulations, the phantom was modeled in the simulation frameworks for the RPTC and CAL with its geometric dimensions and material parameters as stated in Figure 2, and the dose was scored using 100 µm voxel size in the radiography plane and 1 mm slice thickness.
The particle number for the dose estimation was retrieved from the proton fluence at the nozzle exit given by the facility and the irradiation time for all probing energies.
3 Results
3.1 WET accuracy
Margins of 1.1 mm were chosen for the evaluation of the micro-CT phantom radiography at the RPTC and CAL with the phantom-to-detector distance of 13 mm to evaluate a region without blurring from the material interface. The values for all inserts can be found in Table 1. The average of the absolute percentage WET error over all inserts was 0.98% for the RPTC measurements and 1.04% for the measurements at CAL. In the case of 3 mm air gap, the average WET error was 0.87% with a margin to the ROI of 0.3 mm. For the largest air gap of 33 mm, the image quality is drastically reduced through scattering, which leads to an average WET relative error of 30% independent of an ROI margin chosen, as the spatial resolution cannot be evaluated.
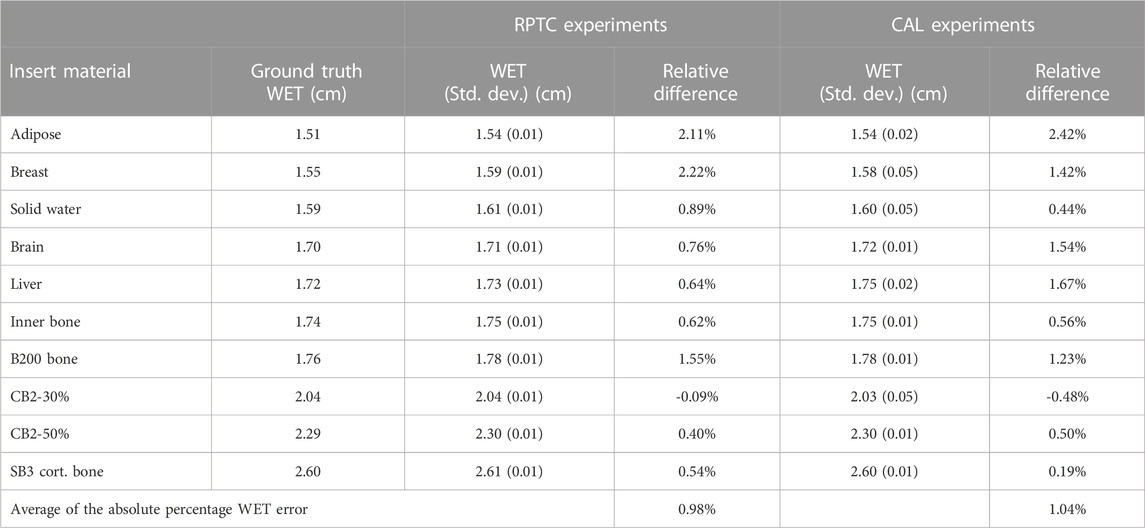
TABLE 1. CAL and RPTC, 13 mm air gap: Comparison of the experimentally determined WET with the ground truth WET (see section 2.4.1).
3.2 Spatial resolution
The spatial resolution of proton radiography from the RPTC with a phantom-to-detector distance of 13 mm was 0.31(3) mm.
The radiographies taken at CAL are shown in Figure 8, and the radiographs for different air gaps between the phantom and detector show the impact of multiple Coulomb scattering on the image quality. The spatial resolution for the radiographs taken at CAL was 0.16(3) mm for the smallest air gap of 3 mm and 0.47(2) mm for 13 mm.
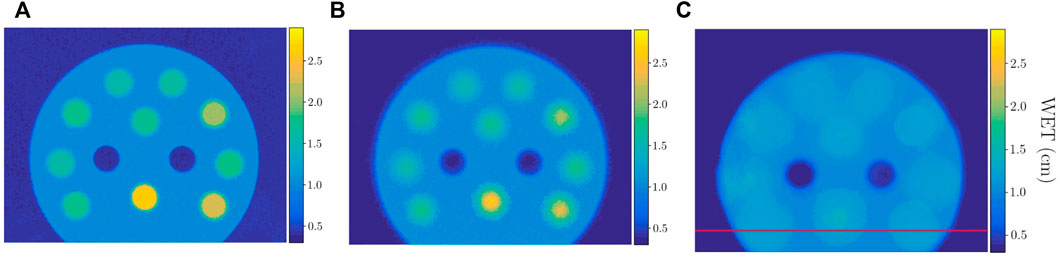
FIGURE 8. WET radiographies at CAL with (A) 3 mm, (B) 13 mm, and (C) 33 mm phantom-to-detector distances (left to right). The red line on the right marks the line profiles in Figure 9.
In the radiography, for the largest air gap, the evaluation of the spatial resolution is not meaningful, as there is not enough WET contrast to fit the edge-spread function.
Figure 9 shows WET profiles for the three different air gaps along a line through the two bone and liver inserts (see line indicated in Figure 8 on the right) compared to the ground truth (see section 2.4.1).
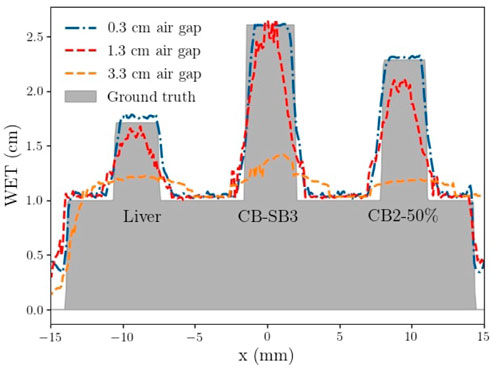
FIGURE 9. Line profiles through three different inserts and ground truth (line indicated in Figure 8) for the radiographic acquisition at CAL.
3.3 Imaging dose
The imaging dose for the proton radiography at the RPTC is estimated to be 8 mGy.
For CAL, the dose for a single radiograph is estimated at 50 mGy. This comparably high value is due to the combination of the minimum beam current from the beamline of 0.1 nA and the maximum frame rate of the detector of 10 fps.
3.4 Imaging time
Imaging time at the RPTC was 94 s for the irradiation of the pre-defined treatment plan.
At CAL, the additional 4-mm slabs of the degrader wheel had to be put in manually, and the beam had to be started and stopped for each degrader step, resulting in an imaging time of around 15 min.
4 Discussion
In terms of the experimental setup, the mechanisms to achieve scattering and energy degradation differed substantially between the two presented experiments. The optimal illumination for imaging would be the homogeneously distributed protons that hit the phantom orthogonal to the detector plane. At the RPTC, a pencil beam is focused to a width of 10 mm at the isocenter, where the PMMA degrader is placed. At CAL, the beam with 34 mm diameter is degraded directly after the nozzle by the variable degrader. After the last element in the beam, there is a 220-cm distance between the PMMA degrader and the detector at the RPTC in comparison to a distance of 125 cm between the degrader wheel and the detector at CAL. Those differences in the setup lead to a greater lateral beam size and a more homogeneous distribution of the protons over the detector surface for RPTC measurements. Monte Carlo simulations showed that the angular distribution of the protons that hit the phantom is narrower for the beam at the RPTC, which is a reason for the better spatial resolution of the proton radiograph taken at the RPTC in comparison to CAL, both at 13 mm air gap.
The calibration and generation of the LUT was more error-prone for the measurements at the RPTC as the baseplate of the phantom itself had to be used for the calibration, and the beam energy variation was carried out with a larger 2 MeV step size. This is reflected in the large systematic uncertainty of 6.7%.
The calibration measurements at CAL using homogeneous slabs of PMMA with the known WET enabled a more thorough fine tuning of the MC simulation to the measurement with a systematic error below 1%.
If the fluence distribution over the field of view (FoV) cannot be considered flat, as it was the case for the measurements at CAL, a specific LUT taking into account the beam shape on the detector is necessary for accurate WET determination, although more computing time is needed to reach sufficient statistics over the 2D plane of the FoV. Radiographies presented in this work were obtained with pixel-specific LUTs, i.e., one value of energy deposition for each probing energy and calibration WET for each pixel in the FoV, as inputs for signal decomposition. As comparison, LUTs with only one value (the accumulated energy deposition in all pixels of the FoV) for each probing energy and calibration WET, independent of the pixel location were used for the WET determination, with the results that the WET accuracy and spatial resolution were similar for experiments at the RPTC, but strongly differed for CAL.
Imaging time at the RPTC was 94 s for the irradiation of the pre-defined treatment plan. This imaging time is largely due to the slow energy switching (4 s for each switch) at the RPTC as this was the first ProBeam® proton therapy system (Varian Medical Systems, Inc., Palo Alto, California) facility and is considerably reduced at newer facilities. The degrader wheel used at CAL was fully automated in the meantime, so that entering the treatment room is not necessary, but irradiation using automatic energy switching stays favorable in terms of the imaging time.
The imaging time and, thus, the dose exposure will also be reduced through a new CMOS detector with a frame rate up to 25 Hz [36, 37].
In addition to the prior dose optimization through the choice of the employed probing energies, dose minimization also depends on the clinical facility to be able to deliver a short burst (
The detrimental effect of proton scattering on image quality can be reduced through data processing with or without the use of prior knowledge about the imaged object [26, 38]. Several methods (Monte Carlo-based and analytical) for the proton scatter correction are currently under investigation. Also, methods employing artificial intelligence are promising candidates to reduce the image quality-diminishing effect of proton scattering.
The feasibility of integration-mode imaging for more heterogeneous objects and small animals was tested in following experimental campaigns. The presented phantom allows for quantitative evaluations but represents a very simplistic case in terms of range mixing. In small animals, a large number of WET contributions can be present in each pixel. If scattering in the object is reduced through the optimal setup (phantom-to-detector distance
5 Summary
Accurate WET retrieval with 1% accuracy and sub-millimeter spatial resolution can be achieved in integration-mode proton imaging using energy stacking and a single CMOS detector behind the object, when limiting the air gap between the detector and the object.
Larger separations between the phantom and the detector remain challenging in integration mode as scattered particles cannot be excluded from the data, although improvements could be enabled through advanced methods in data processing.
This work supports the adoption of integration mode with a CMOS detector for future small animal irradiation platforms, if the setup geometry allows for a minimized
Future studies should aim to confirm the feasibility at synchrocyclotron proton therapy facilities and assess the practical advantages of a next-generation CMOS detector.
Data availability statement
The raw data supporting the conclusions of this article will be made available by the authors, without undue reservation.
Author contributions
KP, MW, and KS designed the study. KS and MW conducted the experiments, with the local support of PH, IY, and JHe at the respective facilities. KS worked on MC simulations and data evaluation, with support from MW and KP. JB, FE, and JS provided support and knowledge on the detector. FE also contributed knowledge on MC simulations. SM and CG supported the proton imaging parts of the work. KN and GL provided the CBCT image of the micro-CT phantom and conversion to the RSP. KS, MW, and KP critically discussed the results and worked on the manuscript. All authors provided feedback and approved the final version of the manuscript.
Funding
The authors acknowledge the financial support from the European Research Council (Grant Agreement ID: 725539), BayFrance (Grant ID: FK312019) and the German Research Foundation (Excellence Cluster “Munich-Centre for Advanced Photonics,” project number: 24819222).
Acknowledgments
The authors acknowledge Maria Carrico, Ines Moskal, and Jannik Esslinger for their early phase studies concerning integration-mode proton imaging with thin pixelated detectors. They also thank Stephan Brons for WET measurements at HIT.
Conflict of interest
The authors declare that the research was conducted in the absence of any commercial or financial relationships that could be construed as a potential conflict of interest.
Publisher’s note
All claims expressed in this article are solely those of the authors and do not necessarily represent those of their affiliated organizations, or those of the publisher, the editors, and the reviewers. Any product that may be evaluated in this article, or claim that may be made by its manufacturer, is not guaranteed or endorsed by the publisher.
Supplementary material
The Supplementary Material for this article can be found online at: https://www.frontiersin.org/articles/10.3389/fphy.2022.1044156/full#supplementary-material
Footnotes
1Teledyne Dalsa, CM49 DST CMOS Image Sensor Data Sheet (2014).
2SMART Scientific Solutions B.V., Routine Preclinical CT Calibration Phantom, Promotional Leaflet (2016).
3The RSP of materials is considered independent of the ion species.
4https://docs.scipy.org/doc/scipy/reference/generated/scipy.optimize.lsq_linear.html.
References
1. Parodi K, Assmann W, Belka C, Bortfeldt J, Clevert DA, Dedes G, et al. Towards a novel small animal proton irradiation platform: The SIRMIO project. Acta Oncologica (2019) 58:1470–5. doi:10.1080/0284186X.2019.1630752
2. Gerlach S, Pinto M, Kurichiyanil N, Grau C, Hérault J, Hillbrand M, et al. Beam characterization and feasibility study for a small animal irradiation platform at clinical proton therapy facilities. Phys Med Biol (2020) 65:245045. doi:10.1088/1361-6560/abc832
3. Lascaud J, Dash P, Wieser HP, Kalunga R, Würl M, Assmann W, et al. Investigating the accuracy of co-registered ionoacoustic and ultrasound images in pulsed proton beams. Phys Med Biol (2021) 66:185007. doi:10.1088/1361-6560/ac215e
4. Poludniowski G, Allinson NM, Evans PM. Proton radiography and tomography with application to proton therapy. Br J Radiol (2015) 88:20150134–14. doi:10.1259/bjr.20150134
5. Johnson RP. Review of medical radiography and tomography with proton beams. Rep Prog Phys (2018) 81:016701. doi:10.1088/1361-6633/aa8b1d
6. Krah N, Khellaf F, Létang JM, Rit S, Rinaldi I. A comprehensive theoretical comparison of proton imaging set-ups in terms of spatial resolution. Phys Med Biol (2018) 63:135013. doi:10.1088/1361-6560/aaca1f
7. Lovatti G, Nitta M, Safari M, Gianoli C, Pinto M, Zoglauer A, et al. An advanced simulation and reconstruction framework for a novel in-beam PET scanner for pre-clinical proton irradiation. IEEE, 1 (2021). doi:10.1109/nss/mic42677.2020.9508007
8. Bortfeldt J, Biebel O, Flierl B, Hertenberger R, Klitzner F, Lösel P, et al. Low material budget floating strip Micromegas for ion transmission radiography. Nucl Instr Methods Phys Res Section A: Acc Spectrometers, Detectors Associated Equipment (2017) 845:210–4. doi:10.1016/j.nima.2016.05.003
9. Meyer S, Bortfeldt J, Lämmer P, Englbrecht F, Pinto M, Schnürle K, et al. Optimization and performance study of a proton CT system for pre-clinical small animal imaging. Phys Med Biol (2020) 65:155008. doi:10.1088/1361-6560/ab8afc
10. Würl M, Schnürle K, Bortfeldt J, Oancea C, Granja C, Verroi E, et al. Proton radiography for a small-animal irradiation platform based on a miniaturized Timepix detector. IEEE (2020). doi:10.1109/nss/mic42677.2020.9508073
11. Testa M, Verburg JM, Rose M, Min CH, Tang S, Bentefour EH, et al. Proton radiography and proton computed tomography based on time-resolved dose measurements. Phys Med Biol (2013) 58:8215–33. doi:10.1088/0031-9155/58/22/8215
12. Zygmanski P, Gall KP, Rabin MSZ, Rosenthal SJ. The measurement of proton stopping power using proton-cone-beam computed tomography proton-cone-beam computed tomography. Phys Med Biol (2000).
13. Ryu H, Song E, Lee J, Kim J. Density and spatial resolutions of proton radiography using a range modulation technique. IOP Publishing (2008). doi:10.1088/0031-9155/53/19/012
14. Telsemeyer J, Jäkel O, Martišíková M. Quantitative carbon ion beam radiography and tomography with a flat-panel detector. Phys Med Biol (2012) 57:7957–71. doi:10.1088/0031-9155/57/23/7957
15. Jee KW, Zhang R, Bentefour EH, Doolan PJ, Cascio E, Sharp G, et al. Investigation of time-resolved proton radiography using x-ray flat-panel imaging system. Phys Med Biol (2017) 62:1905–19. doi:10.1088/1361-6560/aa5a43
16. Gianoli C, Göppel M, Meyer S, Palaniappan P, Rädler M, Kamp F, et al. Patient-specific CT calibration based on ion radiography for different detector configurations in 1 H, 4 He and 12 C ion pencil beam scanning. IOP Publishing (2020). doi:10.1088/1361-6560/aba319
17. Palaniappan P, Meyer S, Kamp F, Belka C, Riboldi M, Parodi K, et al. Deformable image registration of the treatment planning CT with proton radiographies in perspective of adaptive proton therapy. Phys Med Biol (2020) 66:045008. in press. doi:10.1088/1361-6560/ab8fc3
18. Krischel D. Advantages and challenges of superconducting accelerator. In: Ion beam therapy: Fundamentals, technology, clinical applications. Springer Berlin Heidelberg (2012). p. 377–96. doi:10.1007/978-3-642-21414-1_23
19. Mandrillon P, Farley N, Brassart N, Hérault J, Susini A, R O. Commissioning and implementation of the Medicyc cyclotron programme. In: Proceedings of the Twelfth International Conference on Cyclotrons and their Applications. Berlin, Germany (1989). p. 613.
20. Hofverberg P, Bergerot JM, Trimaud R, Hérault J. The development of a treatment control system for a passive scattering proton therapy installation. Nucl Instr Methods Phys Res Section A: Acc Spectrometers, Detectors Associated Equipment (2021) 1002:165264. doi:10.1016/j.nima.2021.165264
21. Hérault J, Gérard A, Carnicer A, Aloi D, Peyrichon M-L, Barnel C, et al. 30 years of ocular proton therapy, the Nice view. Cancer/Radiothérapie (2022) 26:1016–26. doi:10.1016/j.canrad.2022.03.004
22. Englbrecht FS. Investigations of radiation shielding, diagnostic methods and imaging for conventional and laser-driven radiation sources. Ph.D. thesis (2022).
23. Hudobivnik N, Schwarz F, Johnson T, Agolli L, Dedes G, Tessonnier T, et al. Comparison of proton therapy treatment planning for head tumors with a pencil beam algorithm on dual and single energy CT images. Med Phys (2016) 43:495–504. doi:10.1118/1.4939106
24. Niepel K, Stanislaski M, Würl M, Doerringer F, Pinto M, Dietrich O, et al. Animal tissue-based quantitative comparison of dual-energy CT to SPR conversion methods using high-resolution gel dosimetry. Phys Med Biol (2020), 0. doi:10.1088/1361-6560/abbd14
25. Meyer S, Magallanes L, Kopp B, Tessonnier T, Landry G, Dedes G, et al. Tomographic imaging with Carbon ion beams. In: 2016 IEEE Nuclear Science Symposium, Medical Imaging Conference and Room-Temperature Semiconductor Detector Workshop, Strasbourg, France, October 29-November 06, 2016 (IEEE) (2017). doi:10.1109/NSSMIC.2016.8069538
26. Krah N, Testa M, Brons S, Jäkel O, Parodi K, Voss B, et al. An advanced image processing method to improve the spatial resolution of ion radiographies. Phys Med Biol (2015) 60:8525–47. doi:10.1088/0031-9155/60/21/8525
27. Stark PB, Parker RL. Bounded-variable least-squares: An algorithm and applications. Comput Stat (1995) 10.
28. West D, Sherwood A. Proton-scattering radiography. Non-Destructive Test (1973) 6:249–57. doi:10.1016/0029-1021(73)90072-8
29. Gianoli C, Meyer S, Magallanes L, Paganelli C, Baroni G, Parodi K. Analytical simulator of proton radiography and tomography for different detector configurations. Physica Med (2019) 59:92–9. doi:10.1016/j.ejmp.2019.03.002
30. Ferrari A, Sala PR, Fasso A, Ranft J. Fluka: A multi-particle transport code. CERN Yellow Reports: Monographs (2005). Cern-2005-010, Infn/Tc 05/11, Slac-R-773. doi:10.2172/877507
31. Böhlen TT, Cerutti F, Chin MPW, Fassò A, Ferrari A, Ortega PG, et al. The FLUKA code: Developments and challenges for high energy and medical applications. Nucl Data Sheets (2014) 120:211–4. doi:10.1016/j.nds.2014.07.049
32. Würl M, Englbrecht F, Parodi K, Hillbrand M. Dosimetric impact of the low-dose envelope of scanned proton beams at a ProBeam facility: Comparison of measurements with TPS and MC calculations. Phys Med Biol (2016) 61:958–73. doi:10.1088/0031-9155/61/2/958
33. Englbrecht FS. Experimental based Monte Carlo modeling of a clinical proton beam. [Master's thesis]. Germany: Ludwig-Maximilians Universität München (LMU) (2014).
34. Carnicer A, Angellier G, Gérard A, Garnier N, Dubois C, Amblard R, et al. Development and validation of radiochromic film dosimetry and Monte Carlo simulation tools for acquisition of absolute, high-spatial resolution longitudinal dose distributions in ocular proton therapy. Radiat Measurements (2013) 59:225–32. doi:10.1016/j.radmeas.2013.06.011
35. Tessonnier T, Böhlen TT, Ceruti F, Ferrari A, Sala P, Brons S, et al. Dosimetric verification in water of a Monte Carlo treatment planning tool for proton, helium, carbon and oxygen ion beams at the Heidelberg Ion Beam Therapy Center. Phys Med Biol (2017) 62(16):6579–659. doi:10.1088/1361-6560/aa7be4
36. Schnürle K, Bortfeldt J, Englbrecht FS, Gianoli C, Hofverberg P, Meyer S, et al. CMOS integration mode proton imaging for a small animal irradiator. In: K Parodi, K Lauber, G Landry, F Verhaegen, and P Bartelds, editors. 5th Conference on Small Animal Precision Image-Guided Radiotherapy, (Program booklet including abstracts), Munich, Germany, March 21-23, 2022, 114 (2022). doi:10.5282/ubm/epub.92720
37. Schnürle K, Bortfeldt J, Englbrecht FS, Gianoli C, Hofverberg P, Meyer S, et al. CMOS integration mode proton imaging. In: 23rd international workshop on radiation imaging detectors (iWoRiD2022), Riva del Garda, Italy, June 26-30, 2022 (2022). doi:10.5282/ubm/epub.92775
38. Schnürle K, Bortfeldt J, Englbrecht FS, Gianoli C, Hartmann J, Hofverberg P, et al. Development of scatter correction for integration mode proton imaging for a small animal irradiation platform. In: Poster at the Joint Conference of the ÖGMP, DGMP and SGSMP, September 19-22, 2021. Dreiländertagung der Medizinischen Physik (2021). doi:10.5282/ubm/epub.92774
Keywords: proton imaging, small animal, particle therapy, integration mode, CMOS
Citation: Schnürle K, Bortfeldt J, Englbrecht FS, Gianoli C, Hartmann J, Hofverberg P, Meyer S, Niepel K, Yohannes I, Vidal M, Landry G, Hérault J, Schreiber J, Parodi K and Würl M (2023) Development of integration mode proton imaging with a single CMOS detector for a small animal irradiation platform. Front. Phys. 10:1044156. doi: 10.3389/fphy.2022.1044156
Received: 14 September 2022; Accepted: 21 December 2022;
Published: 12 January 2023.
Edited by:
Marie Vanstalle, Université de Strasbourg, FranceReviewed by:
Massimo Minuti, Universities and Research, ItalyChristian Finck, UMR7178 Institut Pluridisciplinaire Hubert Curien (IPHC), France
Copyright © 2023 Schnürle, Bortfeldt, Englbrecht, Gianoli, Hartmann, Hofverberg, Meyer, Niepel, Yohannes, Vidal, Landry, Hérault, Schreiber, Parodi and Würl. This is an open-access article distributed under the terms of the Creative Commons Attribution License (CC BY). The use, distribution or reproduction in other forums is permitted, provided the original author(s) and the copyright owner(s) are credited and that the original publication in this journal is cited, in accordance with accepted academic practice. No use, distribution or reproduction is permitted which does not comply with these terms.
*Correspondence: Katia Parodi, Katia.Parodi@physik.uni-muenchen.de Katrin Schnürle, Katrin.Schnuerle@physik.uni-muenchen.de
†Present address: Klinik für Strahlentherapie, Krankenhaus Barmherzige Brüder, Regensburg, Germany
‡These authors have contributed equally to this work and share senior authorship