- 1School of Mechanical Engineering, Hangzhou Dianzi University, Hangzhou, China
- 2College of Environmental Science and Engineering, Tongji University, Shanghai, China
- 3Sanmen Sanyou Technology Co., Ltd., Taizhou, China
- 4Wenzhou Institute of Hangzhou Dianzi University, Wenzhou, China
The development of smart metamaterials has brought changes to human society, and various new products based on smart metamaterials are emerging endlessly. In recent years, smart electromagnetic metamaterials, smart acoustic metamaterials, smart mechanical metamaterials, smart thermal metamaterials and machine learning have attracted much attention in metamaterials. These fields share similar theories, such as multiphysics coupling fields, novel artificial cells and programmability. Through theoretical and technical research, smart metamaterials will show exquisite applications in many fields, such as antenna and optical communication systems, microwave imaging, acoustic stealth, thermal camouflage, etc. In particular, the characteristics of the personalized microstructure design of smart metamaterials perfectly match the characteristics of 3D printing. The combination of them leads the development of metamaterials, which are undoubtedly of great value. In this paper, focusing on the representative key technologies, we review the development history, main research directions and latest applications of smart metamaterials. Finally, the possible development direction of metamaterials is predicted.
Introduction
Changing the properties of materials by changing their composition is the way in which materials science has been developed in the past. The physical properties of natural materials depend on the basic units and structures which make them up, such as atoms, molecules, lattices, etc. These basic units and structures are interrelated, and many complex factors need to be considered when designing materials. Unfortunately, these factors influence and restrict each other, and limit the upper limit of material properties. In 1968, Veselago first proposed the concept of “medium with negative refractive index” [1], which refers to the medium with negative permittivity and permeability. He also theoretically proved some physical phenomena that contradict common sense, such as the inverse propagation of electromagnetic waves, inverse Doppler effect and inverse Cherenkov radiation in negative refractive index media. However, as there is no medium with negative permittivity and permeability in nature, Veselago’s theory cannot be verified experimentally, so people do not pay enough attention to his discovery. It was not until 2001 that Smith et al. [2] from the University of California, San Diego, made media with negative permittivity and permeability in the microwave band with copper composite materials. They used the brilliant design method which id proposed by Professor John Pendry [3–5] from Imperial College London, United Kingdom. Thus, the phenomenon of negative refraction was experimentally confirmed for the first time. The result of this research has stimulated the research enthusiasm for metamaterials.
Smart materials are materials that respond to stimuli in the environment. Differing from traditional functional materials, smart metamaterials can not only possess the above characteristics but also control electromagnetic and elastic waves through special microstructure design, which enables the materials to display novel force, thermal, acoustic and optical properties. In the early days, the design of metamaterials was mainly based on the equivalent medium theory. The core idea is to break through the limitations of natural laws through the well-designed microstructures, so as to obtain extraordinary physical properties that natural materials do not have [6], such as negative refractive index, negative mass density, negative Poisson ratio, negative dielectric constant, negative permeability, negative bulk modulus, anti-sign thermal expansion coefficient, etc. [7, 8]. Compared with the physical properties of the constituent materials, these features are mainly determined by the topology and deformation of the artificial microstructures [9–11]. Although this concept has appeared in the electromagnetic field as early as the last century, it is only in the last decade that the research and development of electromagnetic wave control has been started to achieve novel functions. A Fiber-Metamaterials solution addresses the light in- and out-coupling issues and can provide fundamentally new solutions for photonic-on-a-chip systems for sensing, subwavelength imaging, image processing, and biomedical applications (Figure 1A). As shown in Figure 1B, the structure of a fishnet metamaterial is demonstrated. To be able to precise its effective parameters, an alternative optical vortex based interferometric approach for the characterization of the effective parameters of optical metamaterials (OMMs) is reported by directly measuring the transmission and reflection phase shifts from metamaterials according to the rotation of vortex spiral interference pattern. The results will pave the way for the advancement of new spectroscopic and interferometric techniques to characterize OMMs, metasurfaces, and nanostructured thin films in general. Electromagnetic stealth is also one of the hot research directions in recent years. By adjusting the physical parameters of the stealth material or changing the structure, the resonance can be achieved and the frequency of the signal response can be changed, so as to reduce the signal detection and achieve low observability (Figure 1C).
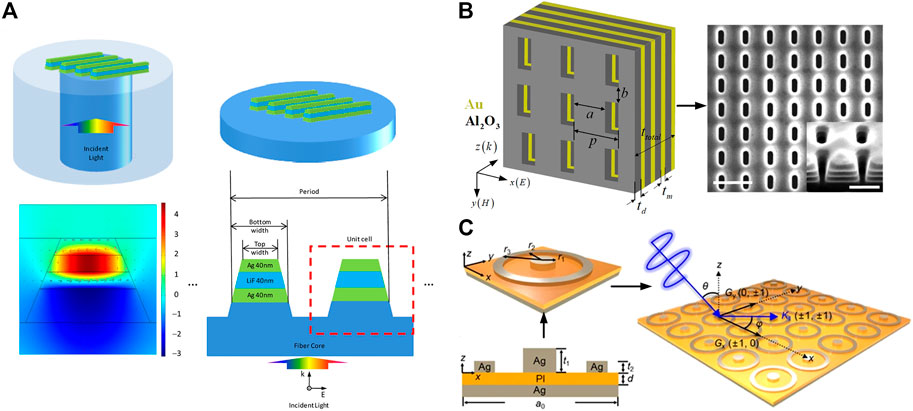
FIGURE 1. (A) A fiber-coupled magnetic metamaterial structure schematically [12]. (B) Schematic diagram of a fishnet metamaterial made of metal-dielectric multilayer stack and top scanning electron microscope image of its structure. The scale bars are 1 µm and 500 nm, respectively [13]. (C) Schematic diagram and surface plasmon polariton excitation of the dual-band perfect absorber. The Metal–insulator–metal structure is composed of Ag and polyimide (PI) layers having thicknesses of t1, t2, and d, and a unit cell period of a0. Unit cell of the circular ring-disk structure, where the disk radius is r1 and the inner and outer radii of the ring are r2 and r3, respectively. Incident light (red line) with polar angle θ encounters a 2D array consisting of circular metal disks and rings. The first Brillouin zone is defined by connecting the perpendicular bisectors of the reciprocal lattice, and a portion of the incident light propagates in the plane containing the periodic pattern with azimuthal angle φ [14].
With the advancement of advanced manufacturing technology, Metamaterials have also been extended to other control fields. Acoustic metamaterials have the ability to control the acoustic energy flow. With this unique ability, acoustic metamaterials have been used in many applications, such as acoustical absorption [15], acoustical holography [16], acoustical switching [17], energy harvesting [18], and hyperlens [19]. The basic concepts for these applications often rely on subwavelength resonance structures in the form of spatial helices, Helmholtz resonators, membrane resonators, and porous metamaterials. Although these common design methods have great acoustic manipulation capabilities, these methods are mainly passive, resulting in fixed spatial structures that can only yield limited performance and cannot adapt to changing environmental conditions. Therefore, tunable acoustic smart structures are desirable because their acoustic response can be actively controlled according to requirements. The block of mechanical metamaterial is a superatom, which deforms, bends, rotates and breaks under the action of external force, and its design enables the adjacent block to produce the desired collective behavior [7]. In recent years, with the continuous development of advanced manufacturing technologies such as 3D printing and laser selective melting, the research scope of mechanical metamaterials has expanded from dynamic properties to static elastic mechanical properties, such as elastic modulus, Poisson’s ratio, stiffness, strength and other mechanical parameters. It is possible to prepare mechanical metamaterials with more complex microstructures [20]. Based on the microstructure design, mechanical metamaterials can achieve abnormal and singular mechanical properties such as negative Poisson’s ratio [20], lightweight [21], and negative stiffness [22]. The invention of locally resonant acoustic materials has created the field of acoustic metamaterials [23]. Their work has directly inspired metamaterial research beyond electromagnetic wave systems, including acoustic, elastic, or mechanical studies. In 2008, Fan et al. extended the concept of transform optics from wave systems to diffusion systems [24]. In the past decade, thermal metamaterials have been rapidly developed and applied in human daily life, such as thermal stealth [25], thermal camouflage [26] and thermal concentration [27].
This review investigates applications of smart metamaterials in different fields with similar theoretical foundations, such as multiphysics coupling mechanisms, novel artificial cell design, and programmability research. We summarize the fields of technology that have received priority attention from scientists and scholars to achieve smart metamaterials. These fields are smart electromagnetic metamaterials (SEMs), smart acoustic metamaterials (SAMs), smart mechanical metamaterials (SMMs), smart thermal metamaterials (STMs) and smart metamaterials and machine learning (SMML). After summarizing the key technologies and development of these smart metamaterials, we briefly discuss the development trend of smart metamaterials in recent years. Due to limited space, this review cannot cover all aspects, and relevant excellent work can be read from other reviews in recent years.
Development and research of smart metamaterials
Smart electromagnetic metamaterialss
SEMs, also known as information metamaterials, can be classified into digitally programmable metamaterials [28], computing metamaterials [29], and optically switchable metamaterials [30]. Among many research directions, digital coding and programmable metamaterials have become an important research direction. In 2014, Giovampaola and Engheta proposed a method to construct “metamaterial bytes” by spatially mixing “metamaterial bits” [31]. The “metamaterial bits” that they used are made up of particles of materials with different electromagnetic properties, such as silicon with positive permittivity and silver with negative permittivity. However, these metamaterial bytes are still constructed from the equivalent medium. As shown in Figure 2, the concepts of digital metamaterials, programmable metamaterials and metasurfaces were first proposed by Cui et al. in 2014 [28]. The programmable properties of metamaterials mean that they can achieve different functions through digital control and realize real-time regulation of electromagnetic waves. Inspired by the binary representation of digital circuits, digital metamaterials first found a way to design metamaterials from binary. By encoding the phase response of the meta-atom as “0” and “1,” the electromagnetic parameters are simply related to the digital expression [32]. In fact, the method of constructing digital expressions using opposed phase before coding digital metamaterials has been widely used in antenna and optical communication fields. For example, combine a thin artificial magnetic conductor with perfect electric conductor cells into a chessboard like configuration. Around the operational frequency of the artificial magnetic conductor elements, the reflection of the artificial magnetic conductor and perfect electric conductor have opposite phase, so for any normal incident plane wave the reflections cancel out, thus reducing the radar cross-section. The same applies to specular reflections for off-normal incidence angles [33]. Similarly, by designing metamaterial resonant cells, spatial light modulators based on metamaterial absorbers can also be realized in the terahertz-band [34–36].
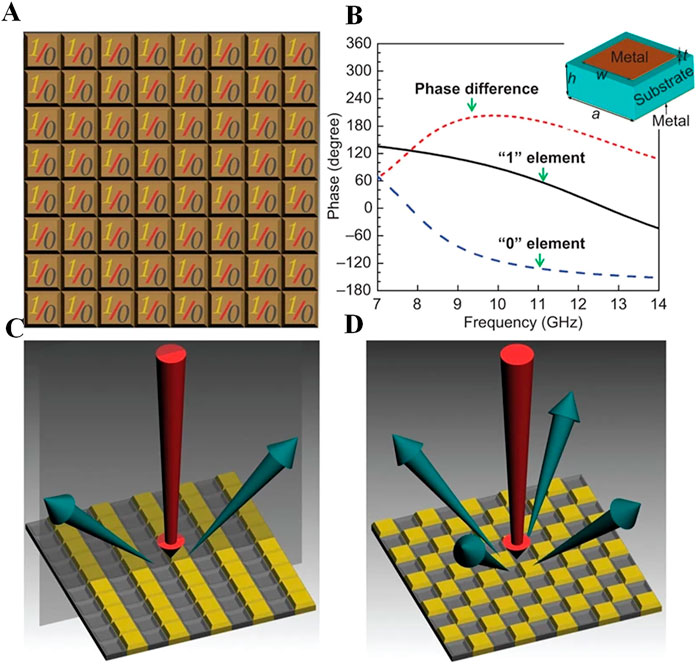
FIGURE 2. The 1-bit digital metasurface and coding metasurface. (A) The 1-bit digital metasurface is composed of only two types of elements: “0”and “1.” (B) A square metallic patch unit structure (inset) to realize the “0” and “1” elements and the corresponding phase responses in a range of frequencies. (C,D) Two 1-bit periodic coding metasurfaces to control the scattering of beams by designing the coding sequences of “0” and “1” elements: (C) the 010101 … /010101... code and (D) 010101 … /101010... code.
Traditional metasurface patterns have been completely replaced by digital coded patterns. Digital metamaterials introduce ideas from information science to simplify the design process and create more functionality. At present, digital metamaterials and programmable metasurfaces have been widely used in microwave imaging [37], information processing [38], and wireless communication [39]. More importantly, programmable metasurfaces build a bridge between the physical and digital world, which enables researchers to explore metasurfaces from the perspective of information science, forming a research system for information metasurfaces [40].
Smart acoustic metamaterials
Since both electromagnetic wave and acoustic wave meet the wave equation and have common parameters, such as wave vector, wave impedance and energy flow density, researchers have extended the design idea of electromagnetic metamaterials to the acoustic field and designed acoustic metamaterials that can have various strange effects on acoustic waves [41]. In 2000, Liu first proposed the construction of acoustic metamaterials by using structural cells of the locally resonant type [23], which opened a new way for the research of acoustic metamaterials. Similar to electromagnetic metamaterials, acoustic metamaterials are constructed by artificial design of two or more materials to form periodic/aperiodic geometric structures. The cell size of the structure is much smaller than the wavelength. Corresponding effective elastic parameters of the artificial structural functional materials can be obtained through the long-wave limit. As shown in Figure 3, in addition to local resonance acoustic metamaterials, there are tunable acoustic hypersurfaces [42], labyrinth or space coiled acoustic metamaterials [43] based on the generalized Snell’s law, and porous acoustic metamaterials [44] that use different apertures and subwavelength holes of arbitrary shape to obtain high refractive index.
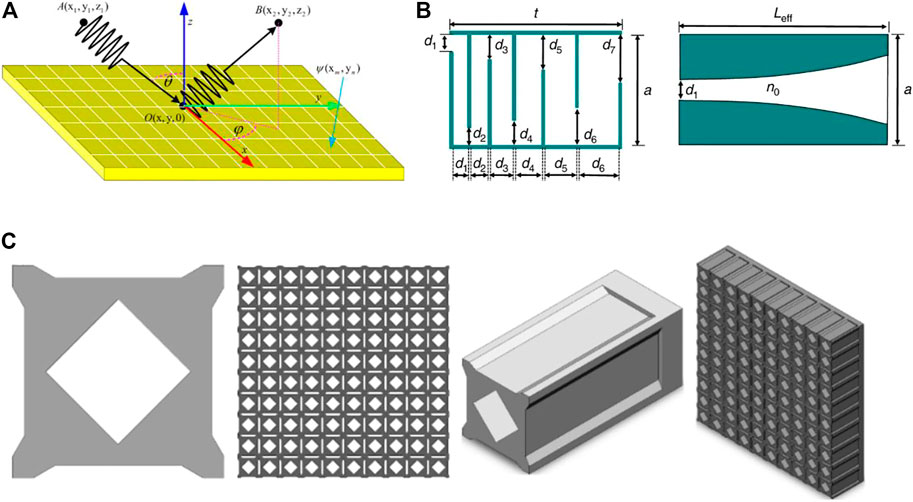
FIGURE 3. (A) Acoustic wave impinges a surface with nonzero phase gradient [42]. (B) Horn-like space-coiling metamaterial unit cell and its equivalent model [43]. (C) Composition of three-dimensional honeycomb porous frequency modulation acoustic metamaterial [44].
Over the past decade, various acoustic structures have been successfully developed to control acoustic waves, bringing new strategies for noise reduction and energy harvesting. Although these common design methods have great acoustic manipulation capability, they are mainly passive. Most acoustic metamaterials with subwavelength scale show good performance around certain frequency bands. Once the structure is established, it cannot adapt to changing circumstances. Using smart materials to design structures has proved to be an effective and promising approach. Smart materials are materials that change shape or properties when exposed to changes in external physical fields, such as light, electric, magnetic, and pressure. Smart materials include electroactive polymers, piezoelectric materials, shape memory materials and magnetic sensitive materials. Due to their inherent advantages such as sensitivity and biocompatibility, smart materials have been widely used in soft robots, actuators, sensors and biomedicine. Modulating external stimuli to manipulate physical properties has attracted increasing interest in the study of smart materials in tunable acoustic structures. Among the currently available works, electrically tuned [45] and magnetically tuned [46] acoustic absorbing smart metamaterials have attractive properties and good design potential.
The fusion of smart materials with acoustic metamaterials offers the opportunity for anomalously effective material properties at tunable frequencies and has great potential. Potential applications that have been identified include acoustic manipulation [47], acoustic absorption [48], acoustic focusing [49], and acoustic stealth [50], which are well beyond traditional materials. A SAM consisting of a mechanical resonator and a piezoelectric element can generate a local resonant band gap near a mechanical or electrical resonance [51]. The main advantage of these electromechanical hybrid resonators over ordinary acoustic metamaterials is that they allow tunable effective mass and stiffness characteristics. Akl has carried out pioneering research on piezoelectric SAMs, especially the analysis and experiments on the composite acoustic resonator with piezoelectric membrane as sensor and actuator [52–55].
Smart mechanical metamaterials
The research of mechanical metamaterials includes design and manufacturing. And structural design is the core of mechanical metamaterials. In recent years, machine learning has developed rapidly. It provides a powerful tool for the automated design of mechanical metamaterials, especially with diverse objective functions [56]. Increasingly complex geometries of mechanical metamaterials pose serious challenges to the material manufacturing technology. And the traditional material reduction manufacturing method is no longer applicable, especially for the structures at the micro or nano scale. So the researchers used additive manufacturing to achieve special complex geometries. Recent developments in additive manufacturing also allow for the manufacture of stimuli-responsive materials, creating structures that can change their geometry, properties, or function. This is often referred to as 4D printing, and the fourth dimension is time.
4D printing is based on 3D printing, but the object is no longer static. Under the external stimulus, the shape, property or function of the object will change with time. Stimulus-responsive shape memory materials based on 4D printing have the advantages of simple manufacturing process, large deformation capacity and various stimulus forms. Common 4D printing stimulus-responsive shape memory materials include shape memory alloys [57], shape memory polymers [58] and shape memory hydrogels [59]. SMMs is an emerging discipline with the continuous development of mechanical design and materials science, and has great application prospects in the fields of engineering and science. 4D printing technology is an optimized rapid prototyping technology. Its integration with SMMs provides new vitality for the development of SMMs. If mechanical metamaterials are reconfigured by replacing traditional materials with stimuli-responsive materials, they will be able to automatically deform, move and change their structural properties or functions in response to external stimulus, such as photothermal effect [60], thermal effect [61], electro mechanical effect [62] and compressive response [63]. To take full advantage of the unique advantages of different material systems, researchers will select appropriate stimuli-responsive materials according to different and application needs. Therefore, SMMs can also be called active mechanical metamaterials. The metamaterial shown in Figure 4 can adaptively switch mechanical properties and shapes without continuous external excitation of the physical field [64].
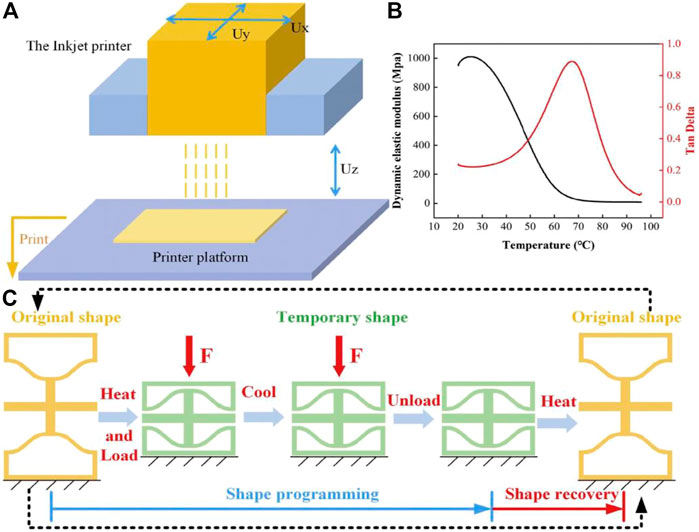
FIGURE 4. (A) 3D printer principle. (B) Dynamic mechanical analysis result of shape memory polymer. (C) Schematic of shape memory effect [64].
SMMs have gradually become a new protagonist in the field of materials and have been endowed with richer functional properties, including reconfigurable [65, 66], programmable [67], elastic wave propagation control [68, 69] and mechanical computing [70, 71]. et al. In the future, the functional properties of natural materials will gradually diminish. In more cases, they will be used as components of functional metamaterials in the form of raw materials. Combined with machine learning and advanced manufacturing processes of 4D printing, SMMs can take full advantage of their flexible design.
Smart thermal metamaterials
Thermal metamaterials are thermal materials with artificial structures, and their novel structures enable them to possess thermal properties or functions that natural materials do not have. It belongs to the emerging Frontier cross-research direction of thermodynamics and statistical physics, thermophysical properties and heat transfer [72]. Since the 21st century, metamaterials have made vigorous progress in the fields of acoustics, optics, elastic wave control and mechanics, which is mainly caused by the huge application demand in these fields. Metamaterials did not make great progress in thermal field until the Fan et al. first introduced the concept of electromagnetic invisibility cloak into thermal field in 2008 [24]. Drawing on the theory of transformation optics, they put forward the theory of transformation heat and predicted the cloak of thermal stealth. At the same time, the phenomenon of heat flow inversion in the system is predicted, which is completely different from the traditional view of “heat flowing to low temperature,” and the concept of negative thermal conductivity is proposed. After more than 10 years of development, thermal metamaterials have achieved various functions, such as thermal stealth [73], thermal transparency [74, 75], thermal camouflage [26], and macroscopic diodes and temperature capture [76], etc.
STMs are artificial composite materials that can sense external heat sources and respond actively, and have the potential to be applied to thermoelectric conversion of micro-nano structures. So far, the basic theories of STMs can be classified into two categories [72]: macroscopic phenomenology theory and microscopic phonon/thermal photon theory. The former controls heat flow and utilizes thermal energy, while the latter utilizes thermal photons for information transmission. Therefore, the application of thermal metamaterials has been developed in a macroscopic and microscopic manner, respectively. The emergence of nonlinear transform thermology makes thermal metamaterials develop towards smart. In addition to linear devices, the effect of this STM is dynamic and changes with the environment. Therefore, following this philosophy, it is crucial to find materials that can change their properties when the external environment changes. Park et al. introduced tunable thermal metamaterials that use the assembly of unit-cell thermal shifters for a remarkable enhancement in multifunctionality as well as manufacturability as show in Figure 5 [77]. In addition, Shen et al. designed and manufactured a thermal cloak concentrator device [78] and a non-energy thermostat [76]. Some of the attractive operations can be achieved by combining nonlinear transformation theory with multi-physical function design. Yang et al. researched the nonlinear responses of thermal metamaterials with 2D or 3D core-shell structures. By calculating the effective thermal conductivity, the nonlinear modulation of the nonlinear magnetic core is derived. Using this theory, a smart thermo-transparent device that can respond to the direction of the thermal field is designed [79]. Wang et al. designed a thermoelectric thermostat cloak with double-layer structure, confirmed the effect predicted by theory with finite element method, and discussed the possibility of experimental demonstration with natural materials [80].
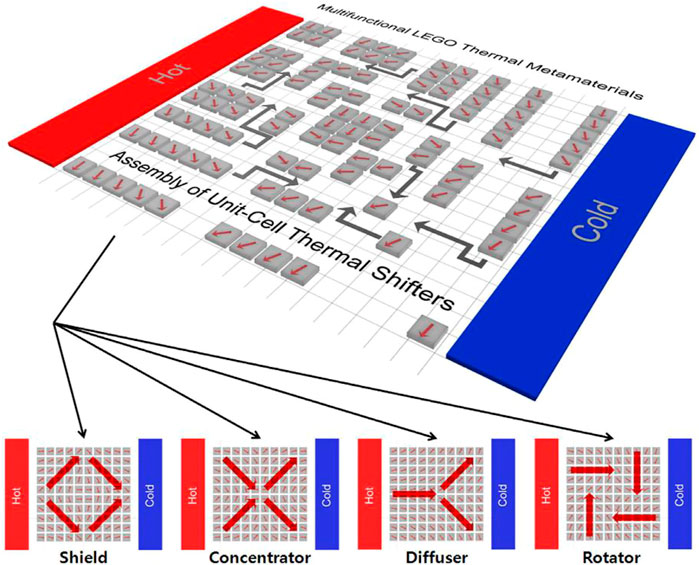
FIGURE 5. Scheme of tunable multifunctional thermal metamaterials [77].
Thermal camouflage or illusion is another important application that has direct military applications. Yang et al proposed simulation and experimental results of thermal invisibility and illusion in single-particle structured materials. By appropriately adjusting the shape factors of individual particles, thermal invisibility and illusion can be achieved simultaneously with a single device. This work not only opens a way for the design of thermal materials based on single-particle structures, but also applies to other physical fields such as electrostatics, magnetostatics and particle dynamics [81]. Compared with static thermal camouflage, dynamic thermal camouflage has greater practicability and prospect in future military applications. For this purpose, the required dynamic thermal camouflage requires an adaptive material that can be initiated in response to external stimuli such as electricity, light, temperature, moisture, machinery, steam, etc. [82].
Smart metamaterials and machine learning
With the development of computer science, computer-aided design and computer-aided engineering are playing an important role in the development of metamaterials [83]. Machine learning has debuted in material and structure studies as the symbol method since the 1990s, such as high-throughput screening approaches enable both simulation and experimental databases to be compiled, with the data used to build models that enable property prediction, determine feature importance, and guide experimental design between the material and structure parameters and the mechanical performance for the fibre/matrix interfaces in ceramic-matrix composites [84]. In order to capture subtle knowledge among the existing data, machine learning performs superior to the traditional statistical methods in terms of the computational efficiency for a significant class of computationally hard problems in materials science, which, therefore, has been extensively used to address different problems in new materials discovery and material property prediction.
When designing metamaterials, numerical simulation can effectively predict the feasibility of the scheme. The design of materials can use data-driven artificial intelligence and other methods to shorten the development cycle and improve the efficiency by training the computational model through experimental data. Both genetic algorithm and neural networks model are adopted to execute the designing process. The method starts from selection of the structural bases based on the existing studies and then combines performance evaluation together with structural evolution to construct meta-atoms with specified properties [85]. Data-driven design of the metamaterial concept is then summarized in Figure 6. A computational data-driven approach is followed for exploring a new metamaterial concept and adapting it to different target properties, choice of base materials, length scales, and manufacturing processes [86]. Training convolutional neural networks to predict the electromagnetic response of metal-dielectric-metal metamaterials, including complex freeform designs, can be explained to reveal deeper insights into the underlying physics of nanophotonic structures [87].
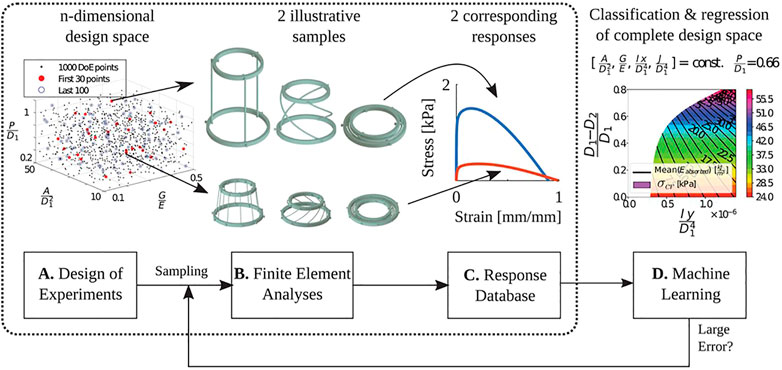
FIGURE 6. Data-driven design of supercompressible metamaterial building block using seven design variables [86].
Machine learning uses computers to mine potential value from data, and learns patterns and characteristics of targets. But it is important to note that whether a machine learned model remains accurate outside of the dataset is always known after validation. Therefore, the independent variables should be kept in the training data space to ensure the correctness of the model.
Summary and prospect
To sum up, this review briefly makes a systematic classification and scientific review of smart metamaterials, but there are many kinds of smart metamaterials and many excellent works that cannot be mentioned. Possible directions for smart metamaterials are listed below. This discussion is intended to be the beginning of a conversation, not the final word.
(1) SEMs can realize real-time regulation of electromagnetic waves, opening up a new way for beam scanning, and can be applied to new field programmable radars in the future. Compared with traditional phased array antennas, it has the advantages of simplicity, efficiency and low cost. We believe that SEMs will have broad development prospects and huge application potential in signal processing, digital communication, information theory, electromagnetic imaging and other fields in the future.
(2) SAMs possess extraordinary physical properties not found in natural materials. Lightweight, low frequency, broadband, small size and strong attenuation are still the goals which are constantly pursued in the research of SAMs.
(3) SMMs regulate elastic waves based on complex topologies. A lot of basic research work remains to be carried out, especially how to transform the traditional theory in the field of condensed matter physical crystallography into the design and characterization of artificial microstructure.
(4) Most of the existing device functions are presented at the macroscopic scale. In order to improve the application value of STMs, nanoscale-based thermal metamaterials need to be designed. Thus, the existing functions of thermal metamaterials will be realized at the micro-nano scale.
(5) One of the most important problems in predicting metamaterial responses. The next step in smart metamaterials and machine learning is to obtain the ideal structures by directly considering the desired response.
Author contributions
Conceptualization, CZ and HD; investigation, HD and JW; data curation, RZ and WL; writing—original draft preparation, YZ and HD; writing—review and editing, YZ, CZ, and ZW; funding acquisition, YZ and RZ.
Funding
This research was funded by the Sichuan Science and Technology Program (No. 2022YFG0274), the Key Research and Development Program of Zhejiang Province (Grant No. 2022C01199), National Natural Science Foundation of China (Grant No. 51875146).
Conflict of interest
JW is employed by the Sanmen Sanyou Technology Co., Ltd.
The remaining authors declare that the research was conducted in the absence of any commercial or financial relationships that could be construed as a potential conflict of interest.
Publisher’s note
All claims expressed in this article are solely those of the authors and do not necessarily represent those of their affiliated organizations, or those of the publisher, the editors and the reviewers. Any product that may be evaluated in this article, or claim that may be made by its manufacturer, is not guaranteed or endorsed by the publisher.
References
1. Vesselago V THE ELECTRODYNAMICS OF SUBSTANCES WITH SIMULTANEOUSLY NEGATIVE VALUES OF $\epsilon$ AND μ. Sov Phys Usp (1968) 10(4):509–14. doi:10.1070/pu1968v010n04abeh003699
2. Pendry H., Stewart Y. I., Stewart W. J. Extremely low frequency plasmons in metallic mesostructures. Phys Rev Lett (1996) 76(25):4773–6. doi:10.1103/PhysRevLett.76.4773
3. Pendry J. B., Holden A. J., Robbins D. J., Stewart W. Magnetism from conductors and enhanced nonlinear phenomena. IEEE Trans Microw Theor Tech (1999) 47(11):2075–84. doi:10.1109/22.798002
4. Pendry J. B. Negative refraction makes a perfect lens. Phys Rev Lett (2000) 85(18):3966–9. doi:10.1103/PhysRevLett.85.3966
5. Shelby R. A, Smith D. R, Schultz S, 292. New York NY (2001). American Association for the Advancement of Sciencep. 77–9. doi:10.1126/science.1058847Experimental verification of a negative index of refractionScience5514
6. Srivastava A Elastic metamaterials and dynamic homogenization: A review. Int J Smart Nano Mater (2015) 6(1):41–60. doi:10.1080/19475411.2015.1017779
7. Bertoldi K., Vitelli V., Christensen J., van Hecke M. Flexible mechanical metamaterials. Nat Rev Mater (2017) 2(11):17066. doi:10.1038/natrevmats.2017.66
8. Wu W., Hu W., Qian G., Liao H., Xu X., Berto F. Mechanical design and multifunctional applications of chiral mechanical metamaterials: A review. Mater Des (2019) 180:107950. doi:10.1016/j.matdes.2019.107950
9. Chaturvedi P., Hsu K., Zhang S., Fang N. New frontiers of metamaterials: Design and fabrication. MRS Bull (2008) 33(10):915–20. doi:10.1557/mrs2008.199
10. Kadic M., Milton G. W., van Hecke M., Wegener M. 3d metamaterials. Nat Rev Phys (2019) 1(3):198–210. doi:10.1038/s42254-018-0018-y
11. Zheludev N. I The road ahead for metamaterials. Science (2010) 328(5978):582–3. doi:10.1126/science.1186756
12. Wang X., Venugopal G., Zeng J., Chen Y., Lee D. H., Litchinitser N. M., et al. Optical fiber metamagnetics. Opt Express (2011) 19(21):19813–21. doi:10.1364/oe.19.019813
13. Cao W., Gao J., Yang X. Determination of effective parameters of fishnet metamaterials with vortex based interferometry. Opt Express (2020) 28(14):20051–61. doi:10.1364/oe.391873
14. Kim J., Han K., Hahn J. W. Selective dual-band metamaterial perfect absorber for infrared stealth technology. Sci Rep (2017) 7(1):6740. doi:10.1038/s41598-017-06749-0
15. Yang M., Sheng P. An integration strategy for acoustic metamaterials to achieve absorption by design. Appl Sci (2018) 8(8):1247. doi:10.3390/app8081247
16. Fan S. W., Zhu Y., Cao L., Wang Y. F., Li Chen A., Merkel A., et al. Broadband tunable lossy metasurface with independent amplitude and phase modulations for acoustic holography. Smart Mater Struct (2020) 29(10):105038. doi:10.1088/1361-665X/abaa98
17. Lee K. H., Yu K., Al Ba’ba’a H., Xin A., Feng Z., Wang Q. Sharkskin-inspired magnetoactive reconfigurable acoustic metamaterials. Research (2020) 2020:1–13. doi:10.34133/2020/4825185
18. Ma K. J., Tan T., Liu F. R., Zhao L. C., Liao W. H., Zhang W. M. Acoustic energy harvesting enhanced by locally resonant metamaterials. Smart Mater Struct (2020) 29(7):075025. doi:10.1088/1361-665X/ab8fcc
19. Chiang T. Y., Wu L. Y., Tsai C. N., Chen L. W. A multilayered acoustic hyperlens with acoustic metamaterials. Appl Phys A (2011) 103(2):355–9. doi:10.1007/s00339-011-6306-3
20. Mercer C., Speck T., Lee J., Balint D. S., Thielen M. Effects of geometry and boundary constraint on the stiffness and negative Poisson's ratio behaviour of auxetic metamaterials under quasi-static and impact loading. Int J Impact Eng (2022) 169:104315. doi:10.1016/j.ijimpeng.2022.104315
21. Chen J., Xu W., Wei Z., Wei K., Yang X. Stiffness characteristics for a series of lightweight mechanical metamaterials with programmable thermal expansion. Int J Mech Sci (2021) 202-203:106527. doi:10.1016/j.ijmecsci.2021.106527
22. Zhu S., Tan X., Chen S., Wang B., Ma L., Wu L. Quasi-all-directional negative stiffness metamaterials based on negative rotation stiffness elements. Phys Status Solidi B (2020) 257(6):1900538. doi:10.1002/pssb.201900538
23. Liu Z., Zhang X., Mao Y., Zhu Y. Y., Yang Z., Chan C. T., et al. Locally resonant sonic materials. Science (2000) 289(5485):1734–6. doi:10.1126/science.289.5485.1734
24. Fan C. Z., Gao Y., Huang J. P. Shaped graded materials with an apparent negative thermal conductivity. Appl Phys Lett (2008) 92(25):251907. doi:10.1063/1.2951600
25. Duan J, Liu R, Xu H, Zhang R, Zhang B, Kim B. S Emt conversion of composite broadband Absorbent metamaterials for stealth application over X-bands. IEEE Access (2020) 8:153787–98. doi:10.1109/ACCESS.2020.3018018
26. Zhang J., Huang S., Hu R. Adaptive radiative thermal camouflage via synchronous heat conduction. Chin Phys Lett (2021) 38(1):010502. doi:10.1088/0256-307x/38/1/010502
27. Zhang Q., Zhu W., Feng J., Zhou J., Deng Y. High-efficient heat flux manipulation of micro-scale thermal metamaterials with facile functional unit design. Mater Des (2021) 204:109657. doi:10.1016/j.matdes.2021.109657
28. Cui T. J., Qi M. Q., Wan X., Zhao J., Cheng Q. Coding metamaterials, digital metamaterials and programmable metamaterials. Light Sci Appl (2014) 3(10):e218. doi:10.1038/lsa.2014.99
29. Zangeneh-Nejad F., Sounas D. L., Alù A., Fleury R. Analogue computing with metamaterials. Nat Rev Mater (2021) 6(3):207–25. doi:10.1038/s41578-020-00243-2
30. Kafesaki M., Shen N. H., Tzortzakis S., Soukoulis C. M. Optically switchable and tunable terahertz metamaterials through photoconductivity. J Opt (2012) 14(11):114008. doi:10.1088/2040-8978/14/11/114008
31. Della Giovampaola C., Engheta N. Digital metamaterials. Nat Mater (2014) 13(12):1115–21. doi:10.1038/nmat4082
32. Ma Q., Cui T. J. Information metamaterials: Bridging the physical world and digital world. PhotoniX (2020) 1(1):1. doi:10.1186/s43074-020-00006-w
33. Paquay M., Iriarte J. C., Ederra I., Gonzalo R., de Maagt P. Thin AMC structure for radar cross-section reduction. IEEE Trans Antennas Propag (2007) 55(12):3630–8. doi:10.1109/TAP.2007.910306
34. Chan W. L., Chen H. T., Taylor A. J., Brener I., Cich M. J., Mittleman D. M. A spatial light modulator for terahertz beams. Appl Phys Lett (2009) 94(21):213511. doi:10.1063/1.3147221
35. Savo S., Shrekenhamer D., Padilla W. J. Liquid crystal metamaterial absorber spatial light modulator for thz applications. Adv Opt Mater (2014) 2(3):275–9. doi:10.1002/adom.201300384
36. Watts C. M., Shrekenhamer D., Montoya J., Lipworth G., Hunt J., Sleasman T., et al. Terahertz compressive imaging with metamaterial spatial light modulators. Nat Photon (2014) 8(8):605–9. doi:10.1038/nphoton.2014.139
37. Lv Z., Li Z., Xu W., Pei Y. Implementing fractional order fourier transformation and confocal imaging with microwave computational metamaterials. EPL (Europhysics Letters) (2020) 131(2):24001. doi:10.1209/0295-5075/131/24001
38. Li L., Cui T. J. Information metamaterials – from effective media to real-time information processing systems. Nanophotonics (2019) 8(5):703–24. doi:10.1515/nanoph-2019-0006
39. Tian S., Zhang X., Wang X., Han J., Li L. Recent advances in metamaterials for simultaneous wireless information and power transmission. Nanophotonics (2022) 11(9):1697–723. doi:10.1515/nanoph-2021-0657
40. Cui TJ, Li L, Liu S, Ma Q, Zhang L, Wan X, et al. Information metamaterial systems. iScience (2020) 23(8):101403. doi:10.1016/j.isci.2020.101403
41. Song H., Ding X., Cui Z., Hu H. Research progress and development trends of acoustic metamaterials. Molecules (2021) 26(13):4018. doi:10.3390/molecules26134018
42. Esfahlani H., Karkar S., Lissek H., Mosig J. R. Acoustic carpet cloak based on an ultrathin metasurface. Phys Rev B (2016) 94(1):014302. doi:10.1103/PhysRevB.94.014302
43. Ghaffarivardavagh R., Nikolajczyk J., Glynn Holt R., Anderson S., Zhang X. Horn-like space-coiling metamaterials toward simultaneous phase and amplitude modulation. Nat Commun (2018) 9(1):1349. doi:10.1038/s41467-018-03839-z
44. Zhou Y., Li H., Ye M., Shi Y., Gao L. Novel design scheme for structural fundamental frequency of porous acoustic metamaterials. Materials (2022) 15(19):6569. doi:10.3390/ma15196569
45. Bacigalupo A., De Bellis M. L., Misseroni D. Design of tunable acoustic metamaterials with periodic piezoelectric microstructure. Extreme Mech Lett (2020) 40:100977. doi:10.1016/j.eml.2020.100977
46. Zhao J., Li X., Wang W., Wang Y., Zhu L., Liu Y. Membrane-type Acoustic metamaterials with tunable frequency by a compact magnet. The J Acoust Soc America (2019) 145(5):EL400–EL4. doi:10.1121/1.5107431
47. Tian Z., Shen C., Li J., Reit E., Gu Y., Fu H., et al. Programmable acoustic metasurfaces. Adv Funct Mater (2019) 29(13):1808489. doi:10.1002/adfm.201808489
48. Koutserimpas T. T., Rivet E., Lissek H., Fleury R. Active acoustic resonators with reconfigurable resonance frequency, absorption, and bandwidth. Phys Rev Appl (2019) 12(5):054064. doi:10.1103/PhysRevApplied.12.054064
49. Liao G., Wang Z., Luan C., Liu J., Yao X., Fu J. Broadband controllable acoustic focusing and asymmetric focusing by acoustic metamaterials. Smart Mater Struct (2021) 30(4):045021. doi:10.1088/1361-665X/abeb2e
50. Yu G., Qiu Y., Li Y., Wang X., Wang N. Underwater acoustic stealth by a broadband 2-bit coding metasurface. Phys Rev Appl (2021) 15(6):064064. doi:10.1103/PhysRevApplied.15.064064
51. Xiao H., Li T., Zhang L., Liao W. H., Tan T., Yan Z. Metamaterial based piezoelectric acoustic energy harvesting: Electromechanical coupled modeling and experimental validation. Mech Syst Signal Process (2023) 185:109808. doi:10.1016/j.ymssp.2022.109808
52. Akl W., Baz A. Multi-cell active acoustic metamaterial with programmable bulk modulus. J Intell Mater Syst Structures (2010) 21(5):541–56. doi:10.1177/1045389X09359434
53. Akl W., Baz A. Analysis and experimental demonstration of an active acoustic metamaterial cell. J Appl Phys (2012) 111(4):044505. doi:10.1063/1.3686210
54. Allam A., Elsabbagh A., Akl W. Experimental demonstration of one-dimensional active plate-type Acoustic metamaterial with adaptive programmable density. J Appl Phys (2017) 121(12):125106. doi:10.1063/1.4979020
55. Allam A., Elsabbagh A., Akl W. Modeling and design of two-dimensional membrane-type Active acoustic metamaterials with tunable anisotropic density. J Acoust Soc America (2016) 140(5):3607–18. doi:10.1121/1.4966627
56. Wu L., Liu L., Wang Y., Zhai Z., Zhuang H., Krishnaraju D., et al. A machine learning -based method to design modular metamaterials. Extreme Mech Lett (2020) 36:100657. doi:10.1016/j.eml.2020.100657
57. Milleret A. 4d printing of Ni–Mn–Ga magnetic shape memory alloys: A review. Mater Sci Tech (2022) 38(10):593–606. doi:10.1080/02670836.2022.2062655
58. Chan B. Q. Y., Low Z. W. K., Heng S. J. W., Chan S. Y., Owh C., Loh X. J. Recent advances in shape memory soft materials for biomedical applications. ACS Appl Mater Inter (2016) 8(16):10070–87. doi:10.1021/acsami.6b01295
59. Chen Z., Li Y., Li Q. M. Hydrogel-driven origami metamaterials for tunable swelling behavior. Mater Des (2021) 207:109819. doi:10.1016/j.matdes.2021.109819
60. Wang L. C., Song W. L., Fang D. Twistable origami and kirigami: From structure-guided smartness to mechanical energy storage. ACS Appl Mater Inter (2019) 11(3):3450–8. doi:10.1021/acsami.8b17776
61. Zhang Y. J., Wang L. C., Song W. L., Chen M., Fang D. Hexagon-twist frequency reconfigurable antennas via multi-material printed thermo-responsive origami structures. Front Mater (2020) 7:7. doi:10.3389/fmats.2020.600863
62. Wang Y., Cheng X. D., Song W. L., Ma C. J., Bian X. M., Chen M. Hydro-sensitive sandwich structures for self-tunable smart electromagnetic shielding. Chem Eng J (2018) 344:342–52. doi:10.1016/j.cej.2018.03.097
63. Korpas L. M., Yin R., Yasuda H., Raney J. R. Temperature-responsive multistable metamaterials. ACS Appl Mater Inter (2021) 13(26):31163–70. doi:10.1021/acsami.1c07327
64. Hu W., Ren Z., Wan Z., Qi D., Cao X., Li Z., et al. Deformation behavior and band gap switching function of 4d printed multi-stable metamaterials. Mater Des (2021) 200:109481. doi:10.1016/j.matdes.2021.109481
65. Wu Y., Guo G., Wei Z., Qian J. Programming soft shape-morphing systems by harnessing strain mismatch and snap-through bistability: A review. Materials (2022) 15(7):2397. doi:10.3390/ma15072397
66. Xin X., Liu L., Liu Y., Leng J. 4d printing auxetic metamaterials with tunable, programmable, and reconfigurable mechanical properties. Adv Funct Mater (2020) 30(43):2004226. doi:10.1002/adfm.202004226
67. Bai Y., Liu C., Li Y., Li J., Qiao L., Zhou J., et al. Programmable mechanical metamaterials with tailorable negative Poisson’s ratio and arbitrary thermal expansion in multiple thermal deformation modes. ACS Appl Mater Inter (2022) 14(31):35905–16. doi:10.1021/acsami.2c08270
68. Chen Y., Hu J., Huang G. A design of active elastic metamaterials for control of flexural waves using the transformation method. J Intell Mater Syst Structures (2016) 27(10):1337–47. doi:10.1177/1045389X15590273
69. Chen Y. Y., Huang G. L. Active elastic metamaterials for subwavelength wave propagation control. Acta Mech Sin (2015) 31(3):349–63. doi:10.1007/s10409-015-0402-0
70. Zheng B., Xu J. Mechanical logic switches based on DNA-inspired acoustic metamaterials with ultrabroad low-frequency band gaps. J Phys D Appl Phys (2017) 50(46):465601. doi:10.1088/1361-6463/aa8b08
71. Zhang H., Wu J., Fang D., Zhang Y. Hierarchical mechanical metamaterials built with scalable tristable elements for ternary logic operation and amplitude modulation. Sci Adv (2021) 7(9):eabf1966. doi:10.1126/sciadv.abf1966
72. Wang J., Dai G., Huang J. Thermal metamaterial: Fundamental, application, and outlook. iScience (2020) 23(10):101637. doi:10.1016/j.isci.2020.101637
73. Yang C., Niu S., Chang H., Wang Y., Feng Y., Zhang Y., et al. Thermal infrared and broadband microwave stealth glass windows based on multi-band optimization. Opt Express (2021) 29(9):13610–23. doi:10.1364/oe.424226
74. He X., Wu L. Thermal transparency with the concept of neutral inclusion. Phys Rev E (2013) 88(3):033201. doi:10.1103/PhysRevE.88.033201
75. Zeng L., Song R. Experimental observation of heat transparency. Appl Phys Lett (2014) 104(20):201905. doi:10.1063/1.4878738
76. Shen X., Li Y., Jiang C., Huang J. Temperature trapping: Energy-free maintenance of constant temperatures as ambient temperature gradients change. Phys Rev Lett (2016) 117(5):055501. doi:10.1103/PhysRevLett.117.055501
77. Park G., Kang S., Lee H., Choi W. Tunable multifunctional thermal metamaterials: Manipulation of local heat flux via assembly of unit-cell thermal shifters. Sci Rep (2017) 7(1):41000. doi:10.1038/srep41000
78. Shen X., Li Y., Jiang C., Ni Y., Huang J. Thermal cloak-concentrator. Appl Phys Lett (2016) 109(3):031907. doi:10.1063/1.4959251
79. Yang S., Xu L., Huang J. Metathermotics: Nonlinear thermal responses of core-shell metamaterials. Phys Rev E (2019) 99(4):042144. doi:10.1103/PhysRevE.99.042144
80. Wang J., Shang J., Huang J. P. Negative energy consumption of thermostats at ambient temperature: Electricity generation with zero energy maintenance. Phys Rev Appl (2019) 11(2):024053. doi:10.1103/PhysRevApplied.11.024053
81. Yang S., Xu L. J., Wang R. Z., Huang J. P. Full control of heat transfer in single-particle structural materials. Appl Phys Lett (2017) 111(12):121908. doi:10.1063/1.4994729
82. Mandal J., Jia M., Overvig A., Fu Y., Che E., Yu N., et al. Porous polymers with switchable optical transmittance for optical and thermal regulation. Joule (2019) 3(12):3088–99. doi:10.1016/j.joule.2019.09.016
83. Bishara D., Xie Y., Liu W. K., Li S. A state-of-the-art review on machine learning-based multiscale modeling, simulation, homogenization and design of materials.Netherlands Archives of Computational Methods in Engineering (2022). doi:10.1007/s11831-022-09795-8
84. Vasudevan R. K., Choudhary K., Mehta A., Smith R., Kusne G., Tavazza F., et al. Materials science in the artificial intelligence age: High-throughput library generation, machine learning, and a pathway from correlations to the underpinning physics. Mrs Commun (2019) 9(3):821–38. doi:10.1557/mrc.2019.95
85. Wu L., Liu L., Wang Y., Zhai Z., Zhuang H., Krishnaraju D., et al. A machine learning -based method to design modular metamaterials. Extreme Mech Lett (2020) 36:100657. doi:10.1016/j.eml.2020.100657
86. Bessa M. A, Glowacki P., Houlder M. Bayesian machine learning in metamaterial design: Fragile becomes supercompressible. Adv Mater (2019) 31(48):1904845. doi:10.1002/adma.201904845
Keywords: smart metamaterials, electromagnetic metamaterials, acoustic metamaterials, mechanical metamaterials, thermal metamaterials, machine learning
Citation: Zheng Y, Dai H, Wu J, Zhou C, Wang Z, Zhou R and Li W (2022) Research progress and development trend of smart metamaterials. Front. Phys. 10:1069722. doi: 10.3389/fphy.2022.1069722
Received: 14 October 2022; Accepted: 07 November 2022;
Published: 22 November 2022.
Edited by:
Hui Chen, Ningbo University, ChinaReviewed by:
Qiujiao Du, China University of Geosciences Wuhan, ChinaXiaodong Zhang, Huaqiao University, China
Copyright © 2022 Zheng, Dai, Wu, Zhou, Wang, Zhou and Li. This is an open-access article distributed under the terms of the Creative Commons Attribution License (CC BY). The use, distribution or reproduction in other forums is permitted, provided the original author(s) and the copyright owner(s) are credited and that the original publication in this journal is cited, in accordance with accepted academic practice. No use, distribution or reproduction is permitted which does not comply with these terms.
*Correspondence: Huajie Dai, cWlubG9uZ2NyY2NAZm94bWFpbC5jb20=; Chuanping Zhou, emhvdWNwQGhkdS5lZHUuY24=; Zhiwen Wang, d3p3MzA0OTk3MjQ2MEBoZHUuZWR1LmNu; Rougang Zhou, NDIxNDFAaGR1LmVkdS5jbg==