- School of Economics and Management, Beihang University, Beijing, China
Fake news that manipulates political elections, strikes financial systems, and even incites riots is more viral than real news online, resulting in unstable societies and buffeted democracy. While factor that drives the viral spread of fake news is rarely explored. In this study, it is unexpectedly found that the easier contagion of fake news online is positively associated with the greater anger it carries. The same results in Twitter and Weibo indicate that this correlation is independent of the platform. Moreover, mutations in emotions like increasing anger will progressively speed up the information spread. Increasing the occupation of anger by 0.1 and reducing that of joy by 0.1 are associated with the generation of nearly six more retweets in the Weibo dataset. Offline questionnaires reveal that anger leads to more incentivized audiences in terms of anxiety management and information sharing and accordingly makes fake news more contagious than real news online. Cures such as tagging anger in social media could be implemented to slow or prevent the contagion of fake news at the source.
1 Introduction
Fake news refers to information that is fabricated, misleading, and verifiably false [1, 2]. Most people broadly accept information instead of critically questioning its authenticity [1]. In particular, with the boom of social media, on which individuals can be simultaneously producers and consumers of information, ordinary people can easily participate in circulation and gain influence through posting (e.g., tweeting) and reposting (e.g., retweeting). Consequently, the impact of fake news on social media could be disproportionate [3] and profound [4], especially in the political and economic fields [2, 4–6]. Furthermore, fake news is more likely to appear in the highly uncertain conditions of emergencies, such as disease epidemics and outbreaks, accidents, and conflicts [7], which makes the spread of fake news a byproduct of the natural response that people have to disastrous events, and social media can be fertile ground for this spread online [8].
Fake news is more viral than real (true) news online [2]. The mechanism underlying its fast spread, though critical, remains unresolved. Unique structural features in the circulation of fake news, such as long diameters of penetration, have been revealed and have been found to be platform-independent [9–11]. However, fake news is generally verified to be false after explosive circulation [12]; thus, in the early spread, it is essentially not thought to be fake, so the structural uniqueness is the manifestation of its fast spread, rather than a cause that can fundamentally explain its viral proliferation. Individuals, either human or bots, posting and reposting fake news on social media are an alternative cause, in particular, the human that occupies the dominant partition [13]. The spread of news is associated with the friends and followers of the author. Nevertheless, user characteristics fail to sufficiently explain the easy contagion of fake news due to their greater effects on the dissemination of real news [2]. The content of fake news, which was also found to be entangled with spread [2], could offer promising directions for probing the mechanism of its fast spread. More importantly, instead of examining spreading structures [2, 9] and reposter demographics [14] after circulation is ignited, revealing the mechanism at the source independent of user demographics would be powerful for inspiring new cures with a minimum invasion of privacy. Hence, we would rather differentiate fake news from real news at the very beginning of its spread by scrutinizing content to figure out new strategies to mitigate fake news that can be implemented without delay.
Online news content not only delivers factual information but also carries sophisticated emotional signals. The digital contagion of emotions is embedded in information spread, and involves individuals experiencing the same feelings on social media that they feel in face-to-face emotional exchanges that occur offline [6, 15]. Emotions further impact the spread of information, e.g., promoting the sharing of information [16] or shaping the path of the information [17]. When the relevance between content quality and popularity is not strong [18], the emotions involved and their influence on psychological arousal may be the key [2]. Moreover, the spread of different emotions can inherently be distinguished, implying that emotions conveyed by both fake and real news could offer comparative proxy measurements by which to examine the mechanisms underlying their circulation. In fact, fake news is preferentially injected with emotions such as anger for political attacks [19]. However, differentiating fake news from real news is rarely based on emotions delivered in the content and incentives beyond reposting in extant efforts. The discrepancy in users’ perceptions between fake news and real news is unraveled in the emotions of the replies [2], while the emotions that are inherently carried by the news itself are not considered when explaining circulation. In fact, the negative emotions in the content have been shown to cause positive responses (e.g., sympathy) [10], meaning emotions, particularly in the negative parts, should be directly examined when studying the spread of fake news. At the same time, although social media content can be short, simplifying the emotions it carries into a single emotion might cause the emotional richness of the content to be missed [2, 20] and lead to a failure of emotional recognition and inconsistent results [2, 6, 21, 22].
In this paper, we aim to explore why online fake news is more viral than true news from the perspective of emotions, which is still not revealed by current studies. First, we define information dominance to analyze the information flow among different user groups according to the number of followers. Structural virality is used to measure the relationship between diffusion structure and the number of retweets. Employing the basic discrete emotion categories and considering the mixture of them, this paper further examines the emotional differences between fake and true news. Emotions in news texts are detected through emotion lexicon and even machine learning approaches. Besides, factors that may have effects on the spread of information are measured and controlled separately. Furthermore, this paper integrates those factors into logistic regression and linear regression models to quantify the effects of emotions on information spread with the consideration of external shock. Finally, we use offline questionnaires to investigate users’ retweeting motivations which are induced with emotions.
Our results from various datasets consistently demonstrate that fake news contains more anger but less joy than true news does. And anger can make online information more viral by inducing stronger incentives of anxiety management and information sharing. This paper makes some contributions. First, the virality of fake news is validated in the chinese social media platform. Second, the differences of fine-grained emotions between true and fake news are clarified. The effects of emotions on the spread of news are also quantified. Finally, the emotion-induced information spread is constructed to explain why fake news is more viral than true news. This study further proposed an early warning strategy in terms of anger proportion to prevent the spread of fake news from the very beginning.
2 Literature review
Social media acts as a dominant source for people to get information [23]. Unfortunately, it also creates a fertile breeding ground for fake news [24] that could profoundly undermine the stability of modern societies. COVID-19 related fake news hinders the development of health behaviors and encourages wrong practices, thereby increasing the risk of infecting the virus [25]. While fake news in politics can be deliberately biased [5] to manipulate voters’ choices [26] and result in buffeted democracy. In fact, politics and economics are closely linked, and political fake news can accordingly incite shocks in the financial market [27]. Fake news in financial markets also directly induces abnormal trading activities and increases price volatility [28]. Given these adverse effects of fake news in social, political, and economical fields, it is of great importance to explore the diffusion mechanism beyond fake news and develop cures against their spread.
Existing studies have found that the spread of fake news in social media demonstrates some unique characteristics that are different from real news. Even at the early stages of propagation, structural differences between fake and real news have emerged [11] in both Twitter in Japan and Weibo in China, which suggests that those differences are platform-independent. In particular, fake news spreads farther, faster, deeper, and more broadly than true news in the cascade networks [2], that is, fake news is more viral than true news. It indicates that fake news can reach more people and cause greater impacts through social media. Meanwhile, compared with other topics, such as terrorism, natural disasters, science, finance, etc., fake political news are more viral [2]. In the diffusion networks of online news, echo chambers, composed of a group with similar interests or views, play an important role [29]. Core echo chambers of fake news can significantly contribute to their spreads [30]. In fact, differences from the spread of true news can be effectively employed to identify fake news [29]. However, fake news, in general, will be noticed by the official departments only after explosive diffusion and then be proved to be false. And most people don’t question the authenticity of information unless it violates their perception [1]. Therefore, the unique diffusion structures of fake news are the manifestation of its viral spread rather than the fundamental reasons that explain its virality. The more viral spread of fake news also implies that cures against its explosive diffusion should emphasize more on content instead of structure to guarantee the in-time intervention at the very beginning.
The content of fake news, which is the source of later diffusion, should be considered to explore how it stimulates other users to retweet. In fact, the manipulation of content, e.g., emotions in fake news, has been noticed [19], for example, anger is deliberately embedded in fake news to attack politics. [2] compared the emotions of replies in true and fake news and suggested that fake news was more novel and novel tweets were more likely to be retweeted. Nevertheless, they didn’t consider the intrinsic emotions of true and fake news that couldn’t be precisely reflected by the emotions embedded in the replies. Studying the retweeting behavior of users should directly consider the input emotions from the original tweets they received. Hence, the first research question (RQ1) of this paper is:
RQ1: What are the differences in the emotional distributions of real and fake news?
The emotions embedded in the online information can be transmitted to readers accurately without direct interaction or nonverbal hint between users, that is, emotion contagion [15]. Moreover, the viral influence of media in enhancing spreading phenomenon has also been analytically characterized in modelling epidemic spread with awareness and heterogeneous transmission rates in networks [31]. The scale and spread characteristics of social media provide favorable conditions for the spread of emotions [6]. It has been found that emotionally charged tweets tended to be retweeted more often and more quickly than neutral ones [16]. In particular, political tweets, containing moral-emotional words, substantially facilitated their diffusion within ideological group boundaries [17]. The effects of specific emotions on information sharing were also examined in previous efforts. It is found that awe, anger, and anxiety could make online content more viral but the impact of sadness was the opposite [21]. In the contrary, Peters et al. [22] omitted the impact of anger and failed to demonstrate the negative effect of sadness, but found that users are more willing to share the news that provoked interest, surprise, disgust, and happiness. The disputes in these studies may be caused by the neglect of mixed emotions [20, 32]. While it is agreed and acknowledged that emotions carried by content can indeed influence the spread of online information and play key roles in the process from retweeting incentives to behaviors [33]. Motivations behind why users retweet information on social media, such as anxiety management, information sharing, and so forth, have been explored [33–35]. However, few studies examine the relationships between emotions and these motivations beyond the virality of fake news. Hence, we ask the second (RQ2) and third (RQ3) research questions in this study:
RQ2: Can the emotional differences between real and fake news explain why fake news is more infectious than real news?
RQ3: How do the emotions affect the incentives behind news reposting?
3 Materials and methods
3.1 Data collection
We collected eight datasets in total. The main dataset collected on Weibo from 2011 to 2016 includes 10,000 true news items (with 409,865 users) posted by credibly verified users and 22,479 fake news items (with 1,189,186 users) endorsed by an official Weibo committee after wide dissemination (see Supplementary Material S1 for more details). Meanwhile, with the proliferation of fake news during the COVID-19 epidemic and the persistence of political fake news, another dataset related to COVID-19 was collected from Weibo to validate the results in the background of emergency incidents (see Supplementary Material S7.3 for more details). Besides, six more English datasets from Twitter and mainstream news media in the west were also collected for supplementary evidence of culture-independence (Table 1). Specifically, a combined subset from Dataset S1-4, composed of a total of 129,690 news items centered around two topics: COVID-19 and the 2016 United States election, were employed to examine the effects of emotions in information spread; Dataset S5-6, composed of 23,959 fake news items and 21,417 real news items, was employed to reveal the mechanism beyond fake news virality. More details can be found in Supplementary Material S10.
3.2 Methods
3.2.1 Analyzing information dominance and structural virality
The number of followers intuitively represents the influence of users on social media, i.e., more followers means the news, either true or false, will be broadcast to a larger audience and accordingly result in more retweets. Some users in social media, however, will buy a large number of fake followers to improve their influence [36, 37]. It may weaken the rationality of the number of followers as the indicator reflecting the influence of users. To ensure the reliability of this indicator, we define information dominance (ID), which measures to what extent the authors of news items could dominate the spread in other spreader groups. According to the numbers of followers, all users are divided into eight groups, including G0 (users whose follower counts fall in the interval
where Ni,m,j is the number of spreaders who belong to Gj and retweeted m in Gi, and G is the number of groups. Meanwhile, the coverage of m to Gj is defined as
where Nj is the number of users belonging to Gj. According to Ti,m,j and Ci,m,j, the transmission coverage of Gi to Gj is defined as
where Mi is the number of news items in Gi. Then, the information dominance of Gi to Gj is
When the information dominance of Gi (Gout) to Gj (Gin) is positive, i.e.,
Additionally, the number of retweets can represent the spreading capability of a given news item [38]. The diffusion structure can also reflect the very viral nature of the news. Therefore, we further explore the relationship of retweets and structural viraliy. The structural virality is the average distance between all pairs of nodes in a diffusion [39], which can measure the diversity of diffusion structure. It is defined as
where di,j denotes the length of the shortest path between nodes i and j. When v ∼ 2, it can be thought an approximately pure broadcast [39]. The average structural virality of news diffusion with the number of retweets is shown in Supplementary Figure S2. For all true and fake news, approximately 97% of the structural virality is lower than two when the number of retweets is less than 10. Meanwhile, fake news is more viral (longer average path) than true news (K-S test ∼ 0.159, P ∼ 0) in terms of structural virality, which is consistent with previous results on Twitter [2], implying the universality of our dataset from Weibo. Six typical diffusion networks of both fake and real news are also shown in Figure 1 to further illustrate this point.
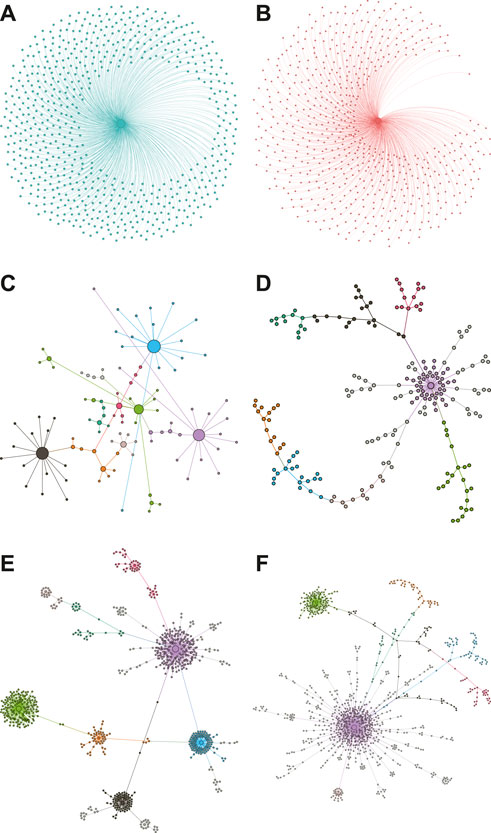
FIGURE 1. Typical examples of diffusion networks for true and fake news items. (A) A true news diffusion network with 630 nodes and v ∼ 2 (pure broadcast). (B) A fake news diffusion network with 600 nodes and v ∼ 2 (pure broadcast). (A,B) are both advertisements. (C) A true news diffusion network with 102 nodes, nine communities, and v ∼ 7.142. The content talks about the descendant of Confucius. (D) A fake news diffusion network with 207 nodes, 17 communities and v ∼ 9.895. The content talks about Red Cross Society of China. (E) A true news diffusion network with 800 nodes, 21 communities, and v ∼ 5.763. The content talks about a North Korean diplomat who joined South Korea. (F) A fake news diffusion network with 997 nodes, 63 communities, and v ∼ 7.748. The content talks about some people using babies to make soup. Different colors represent different communities in the spread.
3.2.2 Constructing treatment and control groups
On the basis of the number of followers on behalf of the broadcasting potential of authors and the number of retweets on behalf of the spreading capability of news [38], we assemble both categories of news into treatment and control groups according to Section 3.2.1 (see Supplementary Material S2). For example, taking fake news with low numbers of followers and high volumes of retweets (LHF news) as the treatment group, the controlled counterparts consist of either fake news with high volumes of followers and low numbers of retweets (HLF news) or true news with high volumes of followers and low numbers of retweets (HLT news). Accordingly, by intentionally selecting news that is weakly retweeted but posted by highly followed authors, the possible effects from users can be controlled to amplify the spread promotion resulting from the particular emotion it carries. Moreover, although fake news is statistically more contagious (longer path, faster speed, lasts longer, and gets more retweets) than real news (see Section 3.2.1 and Supplementary Material S3), not every fake news item is necessarily more viral than any real news item. For instance, the diffusion capability of highly retweeted true news is definitely more powerful than that of lowly circulated fake news. Therefore, we would compare LHF news with HLF news and HLT news first and then extend the comparison to the full spectrum of discrepancies between true (T) news and fake (F) news in terms of emotions.
3.2.3 Analyzing emotion distributions
Starting from the treatment and control groups, we analyzed the differences in emotion distributions between true and fake news. In this paper, we employed five basic cross-cultural emotions, namely, anger, disgust, joy, sadness, and fear [40]. Besides, we used the distribution of five emotions in each tweet to represent the mixture of them. There are three ways to calculate the distribution of emotions, namely, emotion lexicon, classical machine learning models, and deep neural networks. To be more specific, for the emotion lexicon, we segmented all the text into terms and composed a candidate set (see Supplementary Material S4 for more details). Nine well-instructed coders screened 6,155 emotional terms through a WeChat applet, named Word Emotion. Then, the distribution of five emotions is inferred for each news item in our data through the lexicon that covered 87.1% of news items with the remaining considered neutral (see Supplementary Material S4 and Supplementary Material S5). Moreover, we select top 150 keywords which contribute to the separability of the text in different news groups. As shown in Figure 2, the emotion distributions of these keywords have marked differences (see Supplementary Material S6 for more details). Details of emotional approaches ranging from emotion classifiers to alternative distribution measures, which provide consistent evidence in profiling emotion differences later, can be found in Supplementary Material S7. In the meantime, we conducted statistical tests of emotional differences using the Kolmogorov-Smirnov (K-S) test.
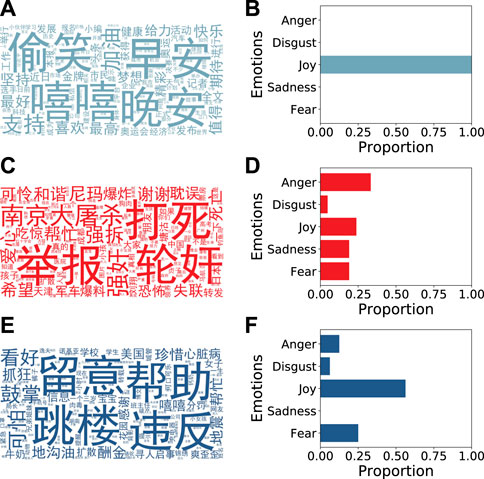
FIGURE 2. Word cloud and emotional distributions of keywords. (A) Word cloud of keywords in HLT news. (B) Emotional distribution of keywords in HLT news. (C) Word cloud of keywords in LHF news. (D) Emotional distribution of keywords. In LHF news. (E) Word cloud of keywords in HLF news. (F) Emotional distribution of keywords in HLF news. All the keywords in the word cloud are translated into English and can be found in the publicly available data at https://doi.org/10.6084/m9.figshare.12163569.v2.
3.2.4 Building logistic and linear regression models
To qualify the impacts of emotions, especially anger (Anger) and joy (Joy), in the spread of fake news, internal factors related to content [41], users [2], and external shocks such as disaster events [7] should be considered and controlled. Specifically, internal factors, including mention (Mention), hashtag (Hashtag), location (Location), date (Date), URL (URL), text length (Length), topic (Topic), Other emotions (Others), number of followers (Follower), number of reciprocal followers (Friend), and external shocks such as emergency (Emergency) constitute control variables. The descriptive statistics and initial analysis of these control variables can be seen in Supplementary Material S8. We first built logit regression models to verify the emotional differences between true and fake news. The logit model for news i is defined as
where pfake,i is the probability that news i is fake, β0 is the intercept, and ɛi is residual. β1, β2, …, β12 and α are the coefficients of the variables. vi,1, vi,2, …, vi,12 represent Angeri, Joyi, Othersi, Followeri, Friendi, Mentioni, Hashtagi, Locationi, Datei, URLi, Lengthi, and Emergencyi respectively. Xi represents topic dummy variables for news i.
Then, we built linear regression models to examine the influences of different emotions on the number of retweets. The linear regression model is defined as
where the dependent variable Numretweet,i is the number of retweets within 48 h from the release of news i. Note that over 70% of retweets of fake news and 80% of retweets of real news occurred within 48 h after posting (see Supplementary Material S3). Other settings, e.g., longer than 48 h, do not influence the results. Consistent results based on the other two emotion inference methods can be seen in Supplementary Material S9.
3.2.5 Investigating retweeting motivations of users
Emotions of high arousal, such as anger and joy, are associated with information diffusion, particularly information sharing [16]. To further investigate how anger and joy carried in news influence incentives underlying retweeting, which reignites the circulation of news on social media, offline questionnaires are conducted to bind the emotion divergence between fake news and real news with retweeting incentives. Due to the time consuming and intensive labor costs, it is challenging for questionnaires to cover all the fake news and true news in our data. Therefore, 15 typical news items from groups of HLT news, LHF news, and HLF news are selected to perform the surveys. Similarly, in terms of news in these groups, the possible stimuli from emotions such as anger and joy to the retweeting incentives are hoped to be amplified to ease the following detection.
To guarantee that the selection of news samples from each group is representative, each group of news is clustered before sampling. First, we use the word2vec model [42] to convert the words in each news item into vectors of 200 dimensions and take the mean of these word vectors to represent the news item, i.e., the news item is similarly embedded in a space of 200 dimensions. Then, K-means clustering is employed to cluster each group of news items into five clusters. Next, based on including keywords with high importance in each news item (see Supplementary Material S6) and intrinsic factors such as mentions and hashtags in each group (see Supplementary Material S8), representative texts are sampled from those near the cluster centers. Note that we do not deliberately consider emotion distributions in the selection to avoid the impact of subjective bias on subsequent incentive stimuli and to ensure the objectivity of the results. Finally, we select 15 typical news items (Supplementary Tables S19–S22), and their positions in the group can be found in Figure 3. The sampled texts and the keywords in these texts are distributed evenly in the embedding space of different groups of news, suggesting that they are indeed typical and representative. Notably, the selected keywords that help separate the groups of news in sampling the texts are anticipated to help strengthen the stimuli of reposting incentives, which would further enhance the impact of anger and joy.
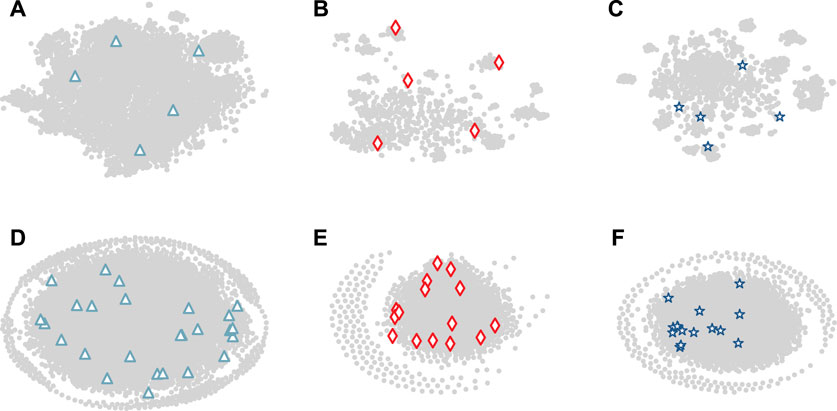
FIGURE 3. Positions of sampled texts and keywords in the embedding space. (A) Text in HLT news. (B) Text in LHF news. (C) Text in HLF news. (D) Keywords in HLT news. (E) Keywords in LHF news. (F) Keywords in HLF news.
Then, we use the 15 typical news items from Weibo dataset to conduct offline questionnaires to profile retweeting incentives towards tweets. The design of the questionnaires ensures that other influencing factors on the interface are consistent, and only representative real or fake news content is used to stimulate users. And keywords that separate fake news from the real in these items were also outlined in questionnaires to strengthen the stimuli of emotions in inducing incentives of retweeting (see Supplementary Material S6). A total of 1,291 valid responses from 1,316 questionnaires were collected from active Weibo users. Finally, for the responses to the questionnaires, we analyzed the differences in motivations among different groups after eliminating subjective bias and then explored the relationships between emotions and motivations. Details in the process of designing, implementing and analyzing questionnaires can be seen in Supplementary Material S12.
4 Results
Emotional signals carried in either fake or real news can be sophisticated, i.e., a combination of elementary compounds rather than a single one [20]. Emotions with a strong presence in the distribution are the feelings that the sender of the news wishes the receivers to experience. The proportion of anger (Figure 4A) in LHF news is expected to be significantly higher than that in both HLF and HLT news, while joy is expected to be lower (Figure 4E). The comparison is then extended to a full spectrum between all fake news and real news, and consistent results, though with shrinking gaps for anger and joy, as expected, are obtained (Figures 4B,F). The results of K-S tests can be seen in Supplementary Material S5. Moreover, the amount of anger in widely circulated news is significantly higher than that in less widely circulated news (see Supplementary Material S5). Furthermore, the dominance of anger in fake news (especially highly retweeted news) and joy in real news (even lowly retweeted news) is further confirmed with better resolution in the distribution of emotional keywords that precisely separate the treatment groups from control groups (see Supplementary Material S6 and Figure 2). These observations persistently suggest that fake news carries more anger yet less joy than real news and imply the possibility that anger might promote the fast spread of fake news online (RQ1). Besides, the divergences in anger and joy between fake news and real news are robust and independent of emotion inference models and emotion distribution measures (see Supplementary Material S7). Even in specific events like COVID-19, the dominance of anger to joy is higher in highly retweeted fake news conformably, which suggests the promotion of anger in the fast spread of fake news (see Supplementary Material S7.3). By contrast, the near overlap in disgust between different types of news (Figures 4C,D), the less occupation of fake news with high sadness (the proportion of sadness is more than 0.5) than real news with high sadness (Figures 4G,H), and the more dominant position of fear in HLF news (Figures 4I,J) indicate their less positive roles in the virality of fake news [16, 21]. Therefore, significant gaps across news groups could also be independent of circulation, and well-controlled inference is accordingly necessary for anger and joy.
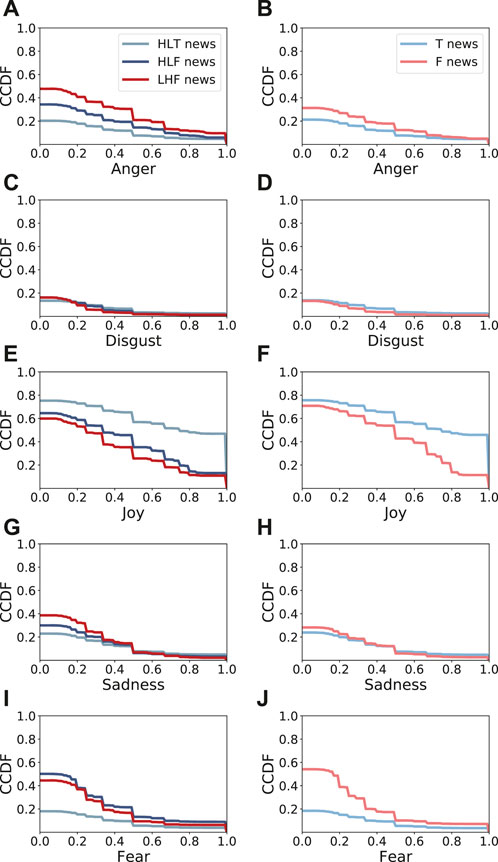
FIGURE 4. Complementary cumulative distribution functions (CCDFs) of emotions. (A,B) The proportion of anger. The proportion of anger greater than 0.5 in LHF news is nearly 3 times as much as that in HLT news (C,D) The proportion of disgust. (E,F) The proportion of joy. The proportion of joy greater than 0.5 in HLT news is more than 2 times as much as that in LHF news (G,H) The proportion of sadness. (I,J) The proportion of fear. The results of K-S tests are shown in Supplementary Material S5, and consistent results from other methods can be seen in Supplementary Material S7.
The results of the logit model for lowly retweeted true (LT) news (control group) and highly retweeted fake (HF) news (treatment group) show that the coefficient of Anger is significantly positive and the coefficient of Joy is negative [Table 2(1)]. Note that other emotions are omitted (Table 2) due to multicollinearity and their trivial impact on circulation. Moreover, for the logit model used to estimate all true and fake news, anger is positively associated with fake news, though with a smaller coefficient and narrower deviation, as anticipated [Table 2(2)]. The results from the logit models further solidify the gaps observed in emotion distributions across groups of news (RQ1).
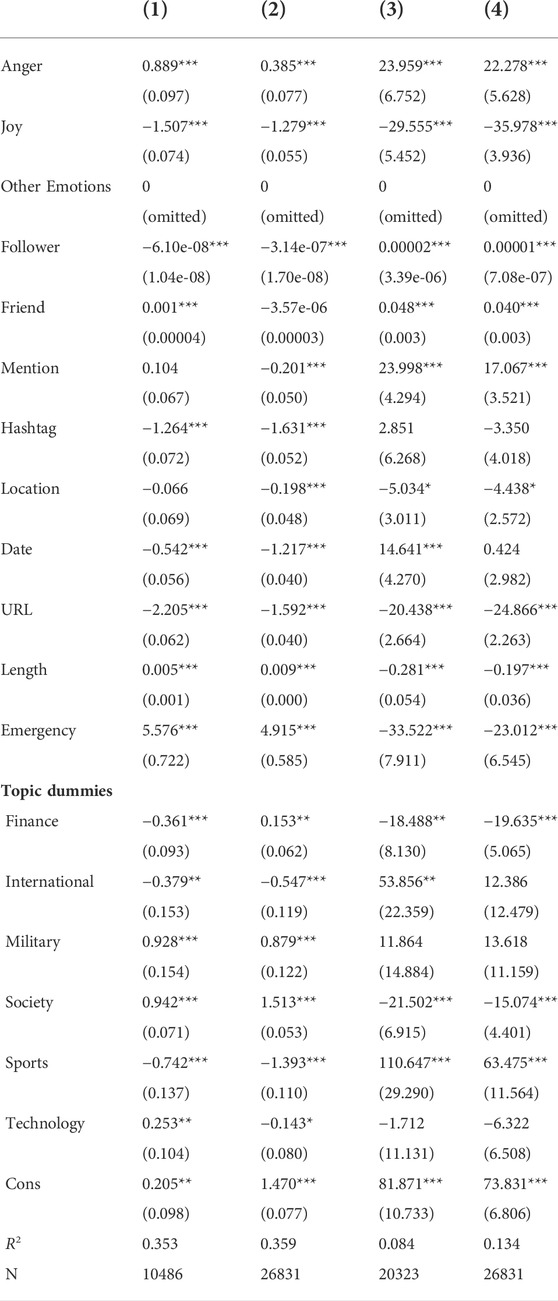
TABLE 2. The results of logit and linear models in different groups. (1) The results of the logit model in LT news and HF news. (2) The results of the logit model in all true news and fake news. (3) The results of the linear model in LF news and HF news. (4) The results of the linear model in lowly retweeted (L) news and highly retweeted (H) news (see Supplementary Material S9 for more details). The values in brackets are the robust standard errors. *P < 0.1,**P < 0.05,***P < 0.01.
To further qualify the influence of both anger and joy in the spread of fake news, a linear regression model with the number of retweets (Retweet) as the dependent variable is established. It is congruously found for fake news and all news that the coefficients of Anger are significantly positive while the coefficients of Joy are negative [Table 2(3) and (4)], suggesting that anger can promote circulation and joy can prevent the spread (RQ2). Specifically, supposing that other factors are fixed, increasing the occupation of anger by 0.1 and reducing that of joy by 0.1 in tweets would lead to 5.8 more retweets, and 2.2 more retweets occur if anger is increased by 0.1 in place of other negative emotions, but joy is fixed [Table 2(4)]. The above relationships between emotions and circulation are robust to alternative emotion detection approaches such as competent machine learning models (see Supplementary Table S17). For other significant factors, although mentions can promote the spread of news [Table 2(3) and (4)], the coefficient of Mention is significantly negative in Table 2(2), which indicates the positive effect of mentions on information spread is more suitable for true news and fake news contains fewer mentions than true news. The coefficient of Emergency is significantly positive in the logit models [Table 2(1) and (2)] but inconsistently negative in the linear models [Table 2(3) and (4)] (see Supplementary Material S8 for more details). Therefore, carrying more anger and less joy is significantly and persistently associated with the fast spread of fake news and this association could make fake news more viral than real news online. More importantly, additional evidence from extensive datasets of English news on both Twitter and mainstream media further confirms that this mechanism is independent of the platform (see Supplementary Material S7).
Negative stimuli such as anger elicit stronger and quicker emotional reactions and even behavioral responses than positive stimuli such as joy [43]. The odds of being forwarded through e-mails are also impacted by the physiological arousal caused by emotional articles, and those evoking high-arousal positive or negative emotions could be more viral [21]. In the spread of fake news, the incentives behind the action of reposting that reignite circulation are therefore hypothetically associated with the anger and joy the news carries. Taking LHF news as the treatment group and HLF news and HLT news as the control groups, the possible associations between reposting incentives and emotions are examined through offline questionnaires. By selecting 15 typical news items with keywords from these groups, questionnaires are implemented to investigate four motivations for news reposting on social media [35], including anxiety management, information sharing, relationship management, and self-enhancement. The subjects of the surveys are Weibo users, and the overlapping between offline subjects and online users is ensured (see Supplementary Material S12). Preliminary results indicate that the motivation of anxiety management in LHF news is significantly higher than that in the control groups (Figure 5A). Moreover, compared to HLT news, subjects are more intensively incentivized to share information when reposting HLF news and LHF news (Figure 5B). Thus, fake news can stimulate strong motivation for information sharing; in particular, fake news that is widely disseminated can also strengthen the motivation for anxiety management. There is no significant variation in the motivation for relationship management across news groups (Figure 5C), and the motivation for self-enhancement in HLT news is stronger than that in fake news (Figure 5D). What is more interesting is that in questionnaires with keywords highlighted with marks, the unique stimuli of widely circulated fake news for anxiety management is strengthened (see Supplementary Figure S19A). The incentive of information sharing is similarly enhanced for fake news (see Supplementary Figure S19B). All these results imply that the responses to the anger carried by fake news are sharing information and even managing anxiety. To validate this hypothesis, the news in questionnaires is further split into anger-dominated news and joy-dominated news (see Supplementary Material S13) to directly probe the impact of emotions. Compared to the retweeting motivations of joy-dominated news, anger-dominated news stimulates stronger incentives for anxiety management (Figure 5E) and information sharing (Figure 5F). Joy-dominated news ultimately excites stronger self-enhancement than anger-dominated news [Figure 5H]. Meanwhile, no significant difference is observed between anger and joy in terms of relationship management motivation [Figure 5G]. Shuffling emotions randomly further testifies to the significance of these observations (see Supplementary Material S13). Therefore, the greater anger delivered in fake news leads to more incentivized audiences with respect to anxiety management and information sharing, resulting in a greater likelihood of retweets and, thus, more viral contagion (RQ3).
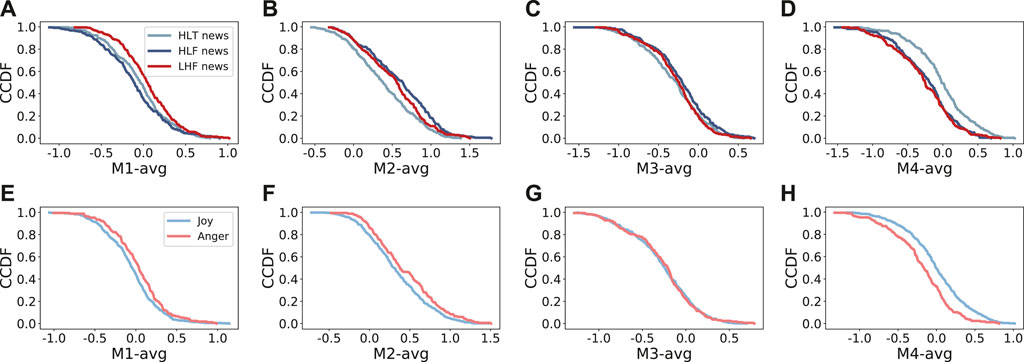
FIGURE 5. The CCDFs of retweeting motivations. (A,E) Anxiety management (M1-avg). (B,F) Information sharing (M2-avg). (C,G) Relationship management (M3-avg). (D,H) Self-enhancement (M4-avg). (A–D) The CCDFs of four motivations in HLT news, HLF news, and LHF news. (E–H) The CCDFs of four motivations in anger-dominated news and joy-dominated news. The results of the K-S tests can be seen in Supplementary Material S13.
5 Discussion
Our findings emphasize the necessity of considering emotions, particularly anger, in understanding the spread of information online. On social media, the associations between information diffusion and embedded emotions have been noted for a long time; however, the profiles of the roles of both positive and negative emotions are inconsistent and even contradictory across diverse contexts [6]. Considering the heterogeneous influence on spreading from negative emotions such as anger and sadness [16, 21], the impact on information diffusion should be examined with respect to well-resolved negative emotions. Instead of simplifying emotions binarily into positive and negative emotions, more elementary emotions are considered in this study, and the distribution of five emotions is inferred to reflect the complete emotional spectrum of news online. As a complex reaction of a person arising from appraisals of self-relevant interactions with the environment, emotion results in direction of attention, action tendencies, and behavior [44]. This more detailed spectrum of emotions identifies an emotion-inducing mechanism, that is, anger can provoke stronger incentives of anxiety management and information sharing, and then contributes to the virality of news online. Moreover, more anger in fake news can explain why it is more viral than real news. From this perspective, emotions could be genes of fake news circulation, and similar to small mutations, they could make the virus go viral. Mutations that increase anger or reduce joy in fake news would enhance its likelihood of being retweeted. Additionally, distinguishing structures in the circulation of fake news could also be deciphered based on the anger such news predominantly carries since anger prefers weak ties in social networks [45] and may inherently forge the diffusion structure of fake news. Meanwhile, the role of joy in preventing spread, especially in fake news, underlines the fundamentality of considering negative emotions of fine granularity to control and deepen future explorations. Therefore, it is anticipated that insights from emotions will improve the extant understanding of online information spread. And these results would also help establish theories regarding emotions to news.
The vigorous promotion in circulation from anger implies new weapons against fake news. Although structural signals can be sensed at an early stage to target fake news [11], fake news spreads rapidly and reaches the peak of new retweets in less than 1 hour (see Supplementary Figure S5), so the negative impact has been exposed to a large population of audiences before identification. Moreover, it can take more than 3 days for a post to be rated as false by outside fact-checkers on Facebook [46]. What is worse, like a cat-and-mouse game between manipulation and detection, features derived from content or users that were found to be helpful in machine learning on targeting fake news [47] can be easily converted to inspire future countermeasures for fabricating more sophisticated false news. In particular, fake news related to emergencies is widely disseminated because of its clever combination with anger, which may explain why efforts to counter misperceptions about diseases during epidemics and outbreaks are not always effective [7]. Inefficient or ineffective efforts to detect fake news and debunk misinformation by correcting both calls for new treatments and preventing the spread of anger could be a profound and promising direction. The early deviation in dissemination paths between fake news and real news suggests the rapid effect of anger in shaping retweeting [17]. For example, platforms such as Facebook, Twitter, and Weibo should warn and discourage users as they try to retweet news that delivers too much anger and persuade them to assess the credibility of the information more critically. The trade-off between free speech and fake news prevention is the prime principle; however, a better balance would be achieved by tagging angry news (e.g., with an occupation of anger more than 20%, see Supplementary Material S14 for more details) at the very beginning to make audiences and potential spreaders less emotional and more rational.
There are inevitable limitations that remain to be considered in the future. First, the effects of fake news on offline human behaviors. Online fake news and the emotions it carries can elicit changes in human behaviors in the reality. However, we still don’t know how people change their offline behaviors when facing fake news and how these changes interact with emotions, topics, and so forth, which is worth further exploration. Second, the spillover effects of fake news across different social systems. The social systems are interconnected, and how fake news and its emotions in social media spread to other systems and then lead to greater impacts or even chain reactions needs more in-depth research. Third, the variation of fake news. When spreading in social media, fake news may mutate and produce homologous information of different versions. Analyzing the emotional and propagation differences between different homologous news may bring a more comprehensive understanding and inspire building of theories regarding emotions to information.
Data availability statement
The datasets presented in this study can be found in online repositories. The names of the repository/repositories and accession number(s) can be found below: https://doi.org/10.6084/m9.figshare.12163569.v2.
Author contributions
YC conducted the analysis and wrote the manuscript. JZ conceived of the study, conducted the analysis, wrote the manuscript and oversaw the work.
Funding
This work was supported by NSFC (Grant No. 71871006).
Acknowledgments
A previous preprint version can be found in arXiv [48].
Conflict of interest
The authors declare that the research was conducted in the absence of any commercial or financial relationships that could be construed as a potential conflict of interest.
Publisher’s note
All claims expressed in this article are solely those of the authors and do not necessarily represent those of their affiliated organizations, or those of the publisher, the editors and the reviewers. Any product that may be evaluated in this article, or claim that may be made by its manufacturer, is not guaranteed or endorsed by the publisher.
Supplementary material
The Supplementary Material for this article can be found online at: https://www.frontiersin.org/articles/10.3389/fphy.2022.970174/full#supplementary-material
References
1. Lazer DMJ, Baum MA, Benkler Y, Berinsky AJ, Greenhill KM, Menczer F, et al. The science of fake news. Science (2018) 359:1094–6. doi:10.1126/science.aao2998
2. Vosoughi S, Roy D, Aral S. The spread of true and false news online. Science (2018) 359:1146–51. doi:10.1126/science.aap9559
3. Allen J, Howland B, Mobius M, Rothschild D, Watts DJ. Evaluating the fake news problem at the scale of the information ecosystem. Sci Adv (2020) 6:eaay3539. doi:10.1126/sciadv.aay3539
4. Aral S, Eckles D. Protecting elections from social media manipulation. Science (2019) 365:858–61. doi:10.1126/science.aaw8243
5. Allcott H, Gentzkow M. Social media and fake news in the 2016 election. J Econ Perspect (2017) 31:211–36. doi:10.1257/jep.31.2.211
6. Goldenberg A, Gross JJ. Digital emotion contagion. Trends Cogn Sci (2020) 24:316–28. doi:10.1016/j.tics.2020.01.009
7. Spinney L. In Congo, fighting a virus and a groundswell of fake news. Science (2019) 363:213–4. doi:10.1126/science.363.6424.213
8. Miller G. Researchers are tracking another pandemic, too—Of coronavirus misinformation (2020). Available at: https://www.science.org/content/article/researchers-are-tracking-another-epidemic-too-misinformation (Accessed June 15, 2022).
9. Del Vicario M, Bessi A, Zollo F, Petroni F, Scala A, Caldarelli G, et al. The spreading of misinformation online. Proc Natl Acad Sci U S A (2016) 113:554–9. doi:10.1073/pnas.1517441113
10. Wang Q, Jin Y, Yang T, Cheng S. An emotion-based independent cascade model for sentiment spreading. Knowledge-Based Syst (2017) 116:86–93. doi:10.1016/j.knosys.2016.10.029
11. Zhao Z, Zhao J, Sano Y, Levy O, Takayasu H, Takayasu M, et al. Fake news propagates differently from real news even at early stages of spreading. EPJ Data Sci (2020) 9:7. doi:10.1140/epjds/s13688-020-00224-z
12. Iyengar S, Massey DS. Scientific communication in a post-truth society. Proc Natl Acad Sci U S A (2019) 116:7656–61. doi:10.1073/pnas.1805868115
13. Langin K. Fake news spreads faster than true news on twitter—Thanks to people, not bots (2018). Available at: https://www.science.org/content/article/fake-news-spreads-faster-true-news-twitter-thanks-people-not-bots (Accessed June 15, 2022).
14. Guess A, Nagler J, Tucker J. Less than you think: Prevalence and predictors of fake news dissemination on Facebook. Sci Adv (2019) 5:eaau4586. doi:10.1126/sciadv.aau4586
15. Kramer AD, Guillory JE, Hancock JT. Experimental evidence of massive-scale emotional contagion through social networks. Proc Natl Acad Sci U S A (2014) 111:8788–90. doi:10.1073/pnas.1320040111
16. Stieglitz S, Dang-Xuan L. Emotions and information diffusion in social media—Sentiment of microblogs and sharing behavior. J Management Inf Syst (2013) 29:217–48. doi:10.2753/mis0742-1222290408
17. Brady WJ, Wills JA, Jost JT, Tucker JA, Van Bavel JJ. Emotion shapes the diffusion of moralized content in social networks. Proc Natl Acad Sci U S A (2017) 114:7313–8. doi:10.1073/pnas.1618923114
18. Acerbi A. Cognitive attraction and online misinformation. Palgrave Commun (2019) 5:15. doi:10.1057/s41599-019-0224-y
19. Higgins M. Mediated populism, culture and media form. Palgrave Commun (2017) 3:3. doi:10.1057/s41599-017-0005-4
20. Penz E, Hogg MK. The role of mixed emotions in consumer behaviour. Eur J Marketing (2011) 45:104–32. doi:10.1108/03090561111095612
21. Berger J, Milkman KL. What makes online content viral? J Marketing Res (2012) 49:192–205. doi:10.1509/jmr.10.0353
22. Peters K, Kashima Y, Clark A. Talking about others: Emotionality and the dissemination of social information. Eur J Soc Psychol (2009) 39:207–22. doi:10.1002/ejsp.523
23. Westerman D, Spence PR, Van Der Heide B. Social media as information source: Recency of updates and credibility of information. J Comput Mediat Commun (2014) 19:171–83. doi:10.1111/jcc4.12041
24. Hameleers M, Powell TE, Van Der Meer TG, Bos L. A picture paints a thousand lies? The effects and mechanisms of multimodal disinformation and rebuttals disseminated via social media. Polit Commun (2020) 37:281–301. doi:10.1080/10584609.2019.1674979
25. Tasnim S, Hossain MM, Mazumder H. Impact of rumors and misinformation on Covid-19 in social media. J Prev Med Public Health (2020) 53:171–4. doi:10.3961/jpmph.20.094
26. Zimmermann F, Kohring M. Mistrust, disinforming news, and vote choice: A panel survey on the origins and consequences of believing disinformation in the 2017 German parliamentary election. Polit Commun (2020) 37:215–37. doi:10.1080/10584609.2019.1686095
27. Rapoza K. Can ’fake news’ impact the stock market? (2017). Available at: https://www.forbes.com/sites/kenrapoza/2017/02/26/can-fake-news-impact-the-stock-market/(Accessed June 15, 2022).
28. Clarke J, Chen H, Du D, Hu YJ. Fake news, investor attention, and market reaction. Inf Syst Res (2020) 32(1):35–52. doi:10.1287/isre.2019.0910
29. Pierri F, Piccardi C, Ceri S. Topology comparison of twitter diffusion networks effectively reveals misleading information. Sci Rep (2020) 10:1372. doi:10.1038/s41598-020-58166-5
30. Choi D, Chun S, Oh H, Han J, Kwon TT. Rumor propagation is amplified by echo chambers in social media. Sci Rep (2020) 10:310. doi:10.1038/s41598-019-57272-3
31. Shang Y. Modeling epidemic spread with awareness and heterogeneous transmission rates in networks. J Biol Phys (2013) 39:489–500. doi:10.1007/s10867-013-9318-8
32. Williams P, Aaker JL. Can mixed emotions peacefully coexist? J Consumer Res (2002) 28:636–49. doi:10.1086/338206
33. Dafonte-Gómez A. News media and the emotional public sphere| audiences as medium: Motivations and emotions in news sharing. Int J Commun (2018) 12:20.
34. Metzger MJ, Flanagin AJ, Mena P, Jiang S, Wilson C. From dark to light: The many shades of sharing misinformation online. Media Commun (2021) 9:134–43. doi:10.17645/mac.v9i1.3409
35. Sudhir S, Unnithan AB. Measuring consumer motivations to share rumors: Scale development. Int J Online Marketing (2014) 4:51–67. doi:10.4018/ijom.2014070104
36. Jang B, Jeong S, Kim C. Distance-based customer detection in fake follower markets. Inf Syst (2019) 81:104–16. doi:10.1016/j.is.2018.12.001
37. Zhang Y, Lu J. Discover millions of fake followers in weibo. Soc Netw Anal Min (2016) 6:16. doi:10.1007/s13278-016-0324-2
38. Wang X, Lan Y, Xiao J. Anomalous structure and dynamics in news diffusion among heterogeneous individuals. Nat Hum Behav (2019) 3:709–18. doi:10.1038/s41562-019-0605-7
39. Goel S, Anderson A, Hofman J, Watts DJ. The structural virality of online diffusion. Management Sci (2016) 62:180–96. doi:10.1287/mnsc.2015.2158
40. Sauter DA, Eisner F, Ekman P, Scott SK. Cross-cultural recognition of basic emotions through nonverbal emotional vocalizations. Proc Natl Acad Sci U S A (2010) 107:2408–12. doi:10.1073/pnas.0908239106
41. Suh B, Hong L, Pirolli P, Chi EH. Want to be retweeted? Large scale analytics on factors impacting retweet in twitter network. In: 2010 IEEE Second International Conference on Social Computing (IEEE) (2010). p. 177–84.
42. Shi B, Zhao J, Xu K. A word2vec model for sentiment analysis of weibo. In: 2019 16th International Conference on Service Systems and Service Management (IEEE) (2019). p. 1–6.
43. Baumeister RF, Bratslavsky E, Finkenauer C, Vohs KD. Bad is stronger than good. Rev Gen Psychol (2001) 5:323–70. doi:10.1037/1089-2680.5.4.323
44. Lazarus RS. Progress on a cognitive-motivational-relational theory of emotion. Am Psychol (1991) 46:819–34. doi:10.1037/0003-066x.46.8.819
45. Fan R, Xu K, Zhao J. Weak ties strengthen anger contagion in social media. arXiv [Preprint] (2020). Available from: https://arxiv.org/abs/2005.01924 (Accessed June 15, 2022).
46. Ortutay B. Fact-checking fake news on Facebook works - just too slowly (2017). Available at: https://apnews.com/e03283b4169f4d8c8a7e51042d61bcb5 (Accessed June 15, 2022).
47. Shu K, Sliva A, Wang S, Tang J, Liu H. Fake news detection on social media: A data mining perspective. SIGKDD Explor Newsl (2017) 19:22–36. doi:10.1145/3137597.3137600
48. Chuai Y, Zhao J. Anger makes fake news viral online. arXiv [Preprint] (2020). Available from: https://arxiv.org/abs/2004.10399.
Keywords: fake news, information spread, emotion contagion, computational social science, social physics, social media
Citation: Chuai Y and Zhao J (2022) Anger can make fake news viral online. Front. Phys. 10:970174. doi: 10.3389/fphy.2022.970174
Received: 15 June 2022; Accepted: 30 June 2022;
Published: 22 August 2022.
Edited by:
Matjaž Perc, University of Maribor, SloveniaCopyright © 2022 Chuai and Zhao. This is an open-access article distributed under the terms of the Creative Commons Attribution License (CC BY). The use, distribution or reproduction in other forums is permitted, provided the original author(s) and the copyright owner(s) are credited and that the original publication in this journal is cited, in accordance with accepted academic practice. No use, distribution or reproduction is permitted which does not comply with these terms.
*Correspondence: Jichang Zhao, amljaGFuZ0BidWFhLmVkdS5jbg==