- 1 Department of Cognitive, Linguistic, and Psychological Sciences, Brown University, Providence, RI, USA
- 2 Department of Psychology and Institute for Learning and Brain Sciences, University of Washington, Seattle, WA, USA
Four-year-olds were more accurate at learning causal structures from their own actions when they were allowed to act first and then observe an experimenter act, as opposed to observing first and then acting on the environment. Children who discovered the causal efficacy of events (as opposed to confirming the efficacy of events that they observed another discover) were also more accurate than children who only observed the experimenter act on the environment; accuracy in the confirmation and observation conditions was at similar levels. These data suggest that while children learn from acting on the environment, not all self-generated action produces equivalent causal learning.
Introduction
Before the age of five, children possess sophisticated causal knowledge about the physical (e.g., Bullock et al., 1982), psychological (e.g., Wellman, 1990), and biological (e.g., Inagaki and Hatano, 1993) domains of knowledge. This enables their predictive (e.g., Shultz, 1982), explanatory (e.g., Schult and Wellman, 1997), and counterfactual reasoning (e.g., Harris et al., 1996) abilities. How do children acquire this knowledge and these reasoning abilities?
Both classic theory (e.g., Montessori, 1912/1964; Piaget, 1952) and contemporary research (e.g., Kushnir and Gopnik, 2005; Sommerville et al., 2005; Schulz et al., 2007) suggests that children treat information generated from their own actions as critical for causal learning. Why is this the case? Causal learning requires appreciating particular pieces of conditional probability information – when two events are correlated, it might be because one causes the other or because a third event causes them both (among other possibilities). Conditional probability information can be used to resolve potential confounds among correlated events that might require many observations or observations of events that rarely (or never) occur on their own (e.g., Bacon, 1620/1986). To use a classic philosophical example, I can observe that the rooster crows when the sun rises, but I should not expect the sun to rise at 2:00 a.m. if I make the rooster crow.
Conditional probability information can be learned from observation, but actions typically provide conditional probability information not present in simply observing the environment. Adults learn causal relations better from observing their own actions on a system than from observing the same data without knowing what actions produced the events (Steyvers et al., 2003; Lagnado and Sloman, 2004; Waldmann and Hagmayer, 2005). Four-year-olds also show a similar advantage (Schulz et al., 2007).
But why does acting on the environment produce more accurate causal learning than merely observing data? Generating an action, or more formally, an intervention – an exogenous change to a variable in a causal environment that affects its value, and thus the value of any event directly or indirectly caused by it (see e.g., Pearl, 2000), provides the learner with three pieces of information that have been validated empirically. First, observing the results of actions provides conditional probability data. If a causal relation exists between two events X → Y, then the probability that X occurs given that Y occurs is not necessarily the same as the probability that X occurs given that you make Y occur. Young children can recognize such conditional probability information from observing intervention data (e.g., Gopnik et al., 2001; Schulz and Gopnik, 2004; Sobel and Kirkham, 2006).
Second, actions offer learners anticipatory information in the form of temporal priority: the variable intervened on should be considered a cause of any subsequent or concurrent event. Lagnado and Sloman (2004, 2006) demonstrated that adults are sensitive to this anticipatory information (even over conditional probability information). Infants have little trouble anticipating events (e.g., Haith, 1993) and such anticipation can indicate their understanding of certain causal relations (e.g., Johnson et al., 2003; Sobel and Kirkham, 2006). Further, young children recognize the importance of temporal priority in causal inference (e.g., Bullock et al., 1982; Sophian and Huber, 1984).
Finally, actions allow a learner to be active in his/her learning process – one must decide what action to make. Adults and elementary-school children learn causal structures more accurately from observing the results of their own actions than from observing another learner generate the same data (Kuhn and Ho, 1980; Lagnado and Sloman, 2004; Sobel and Kushnir, 2006). Sobel and Kushnir (2006) also found that adults learn better from their own actions than from being told what to do – the act of generating those actions was, by itself, insufficient to promote accurate learning. Preschoolers’ causal reasoning is influenced more by data from their own actions over another person’s (Fireman et al., 2003; Kushnir and Gopnik, 2005; Kushnir et al., 2009). Even infants, who clearly learn from imitation (e.g., Meltzoff, 1988), register the importance of their own actions when recognizing causal structure from the environment (e.g., Sommerville and Woodward, 2005; Sommerville et al., 2005).
The present investigation considers a fourth benefit for causal learning from action: discovery, which has been traditionally been considered important in educational environments. Bruner (1961) emphasized that students who discover information for themselves are more motivated to achieve educational goals and more likely to remember learned information. Various research has suggested that students learn better by discovering causal structure through guided activity-based exercises, rather than being directly told what to do or being given unstructured activity (e.g., Kittel, 1957; Shulman and Keisler, 1966; Bredderman, 1983; Mayer, 2004). Our goal is to apply this hypothesis to the process of learning a novel causal structure.
There are some recent studies in cognitive development have emphasized the role of discovery in causal learning. For example, Schulz et al. (2007) suggested that children can discover the causal efficacy of a novel system through free play. Schulz and Bonawitz (2007) demonstrated that preschoolers play more with a toy when its causal efficacy is ambiguous than when they are shown unconfounded evidence about what the toy does. These data suggest that children might learn from discovering the efficacy of the toy on their own through their free play, but they do not manipulate whether the child actually discovers the causal efficacy first or first observes another make that discovery, and then confirm those data. Our hypothesis is that actions that discover novel causal information as opposed to actions that confirm another’s discovery will lead to superior causal learning.
We showed 4-year-olds a novel causal system from which they could learn through their own actions. We wanted to contrast how children learned causal relations when their actions discovered the efficacy of each event in the system with the case in which their actions confirmed the results of another’s actions that had previously demonstrated the efficacy of each event. To do this, one group of children were allowed to play freely with the system, and then they observed the experimenter play with it, whereas the other group was given this procedure in reverse. To ensure that children who watched first were not simply distracted by the free play procedure, we also examined a third group of children who only watched the experimenter act on the causal system. If discovery influences causal learning, then one would expect children to be more accurate when they discover the causal efficacy of events in a system than in either of the other conditions.
Experiment
Methods
Participants
Sixty-one 4-year-olds (30 girls, M = 55.34 months, SD = 3.09, Range = 49–60 months) were recruited from flyers posted in local preschools and list of hospital births. Twenty additional children were tested, but excluded from the study due to experimental or machine error (n = 10), because they failed the control questions (n = 5), due to parental interference (n = 2), refusal to participate (n = 1), because they were colorblind (n = 1) or had a learning disability (n = 1). Fifty-seven children were Caucasian, two children were Hispanic, one child was Asian, and one child was of mixed ethnicity. All children were fluent English speakers. No formal information about SES was collected, but most children appeared to be from upper to middle-class families.
Materials
The lightbox (shown in Figure 1) was constructed from a 20 cm × 15 cm × 8 cm black plastic box. Four colored lights (Red, Yellow, Blue, and Green) were located in the center of the box, each diagonal from an analogous colored button (3 cm in diameter). Pressing the button activated its corresponding light. The box was wired such that any light could cause any other light to activate, and was programmable, such that we could control the causal structure among the lights that children observed at any given time. The programming worked by flipping a series of switched underneath the box that controlled whether each possible causal relation among the lights was present or absent.
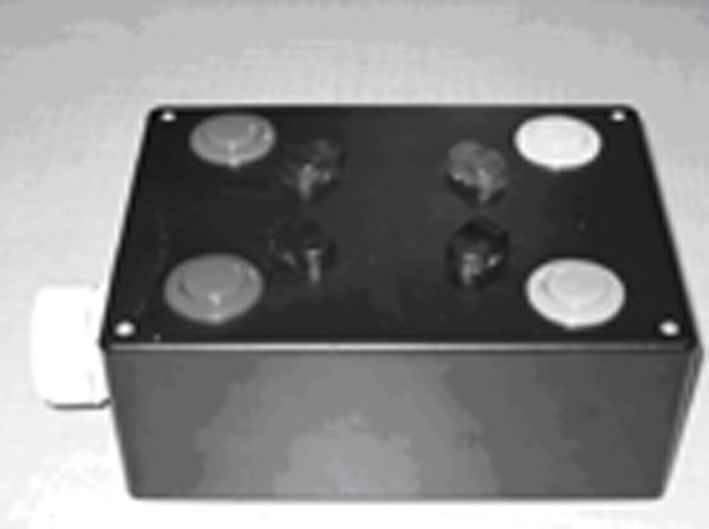
Figure 1 The lightbox used in the procedure. The four buttons, colored red, yellow, green, and blue, activated their corresponding light for as long as the button was depressed.
If a causal link was programmed between two lights (e.g., between light A and light B), pressing the button associated with A would activate both light A and B while pressing the button associated with B would only activate light B. This illumination was immediate, so there was no temporal delay between the activation of light A and B. The lights were illuminated as long as the requisite button was pressed. On one side of the box, there was a white button and speaker. Pressing this button emitted a cartoon-like sound effect.
Procedure
The experimenter introduced the lightbox, explained that the lights come on when the buttons are pressed, and demonstrated this to the child. Initially, no light caused any other light to activate. Children were asked to turn on each light to ensure that children knew the color names, and would recognize that pressing the corresponding button would activate the light of the same color. All children in the final analysis answered these questions correctly.
Familiarization phase. The experimenter then surreptitiously reprogrammed the box such that the red light activated the green light, and the yellow light activated blue. Children were shown the side button, which was pressed, emitting a cartoonish sound. The experimenter said, “Now, the box is different – now, it is a puzzle box. In the puzzle, some lights make other lights go. Let me show you. Let’s press the red button.” The experimenter did so, and red and green activated. The experimenter narrated the results and then pressed the green button, which resulted in only green activating. This was also described to the child. Children were told that these data indicated that, “In this puzzle, red makes green go, but green does not make red go.” The experimenter then repeated this procedure for the yellow and blue lights (with yellow making blue go).
The experimenter then asked the child six causal structure questions. Specifically, children were asked whether red caused green and green caused red, whether yellow caused blue and blue caused yellow, and then two other questions about light pairs (determined randomly). If children answered any of these questions incorrectly, corrective feedback was given.
Test phase. Children were told that they would try to learn new puzzles. All children were asked to learn a common cause model (Figure 2A) and a chain model (Figure 2B) involving three lights (the fourth light neither caused nor was caused by any other light). The order in which children learned the two models was counterbalanced. Two particular configurations of colors for each model were used (counterbalanced across children).
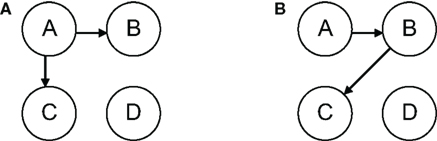
Figure 2 Representations of the causal structures children were asked to learn across the experiments. (A) Shows the common cause model and (B) shows the chain model. In the experiment, all four lights were present, so there was also a fourth light (D) that did not have any causal influence.
One group of children (n = 20) was randomly assigned to the discovery condition. These children were told that it was their turn to play with the box “in order to learn the puzzle.” They were given the box, and allowed to press buttons to activate the lights. Children could intervene on the box for as long as they liked, but they had to press each button at least once, and press all the buttons at least 15 times total (note – they did not have to press each button 15 times; rather, the total number of button-presses had to exceed 15). When children indicated they were finished (and they had made more than 15 button-presses – if not they were encouraged to keep playing), the experimenter brought the lightbox over to his side of the table and pressed each button exactly one time. During this demonstration, he narrated the efficacy of that button press (e.g., “When I press the red button, the red light comes on…”).
A second group of children (n = 20) was randomly assigned to the confirmation condition. These children were given the same procedure as those in the discovery condition, but in reverse order. They first observed the experimenter press each button on the lightbox once (narrating the results), and then could intervene on the box “in order to learn the puzzle” until they indicated they were finished (determined in the same manner as children in the discovery condition).
The final group of children (n = 21) was assigned to the observation condition. These children were given the same demonstration by the experimenter (i.e., one button press on each light, with the experimenter narrating the results), but were never allowed to act on the lightbox themselves. We ran this condition to ensure that any difference between the discovery and confirmation conditions was because of the benefit of discovery, as opposed to children in the confirmation condition learning from the observation, but becoming distracted by the free play or being less motivated to act because they thought they learned from just the demonstration. If either of these were the case, we would expect accuracy in this condition to be superior to that of the confirmation condition. In contrast, if discovery benefits learning, then accuracy in this condition should be similar to accuracy in the confirmation condition, and less accurate than the discovery condition. Critically, in this condition, we used the same language to describe what the experimenter was doing (so that the child would learn the puzzle).
After the free play or demonstration, children in all three groups were then asked a set of causal structure questions to assess how accurately children had learned the relations among the lights. We asked eight questions for each model: concerning the two direct causal relations, those two causal relations in reverse, two questions involving the fourth light, which was not involved in the model, and two questions looking at the indirect causal links between lights in the model. Table 1 shows the exact causal structure questions that were asked. These questions were asked in a random order, different for each child. If children answered every question across both models with “yes” or “no,” they were excluded from the analysis. This ensured that children were not simply parroting back an answer, so that results were more likely representative of the child’s representation of the causal structure. Five children were replaced for this reason.
Results
Children required corrective feedback on 8.5% of the causal structure questions during the familiarization. Children who needed corrective feedback on at least one question (n = 23) did not score differently on the causal structure questions on either model during the test phase than children who needed no corrective feedback (n = 38), both t(59)-values < 0.11, both ns. This suggests that children recognized the basic structure of the task. The proportion of correct responses to the causal structure test questions for each model and condition is shown in Table 2. Preliminary analyses revealed that order, light configuration, and gender had no effect on the total proportion of causal structure questions children answered correctly, all Mann–Whitney tests, all z-values < 1, all ns.
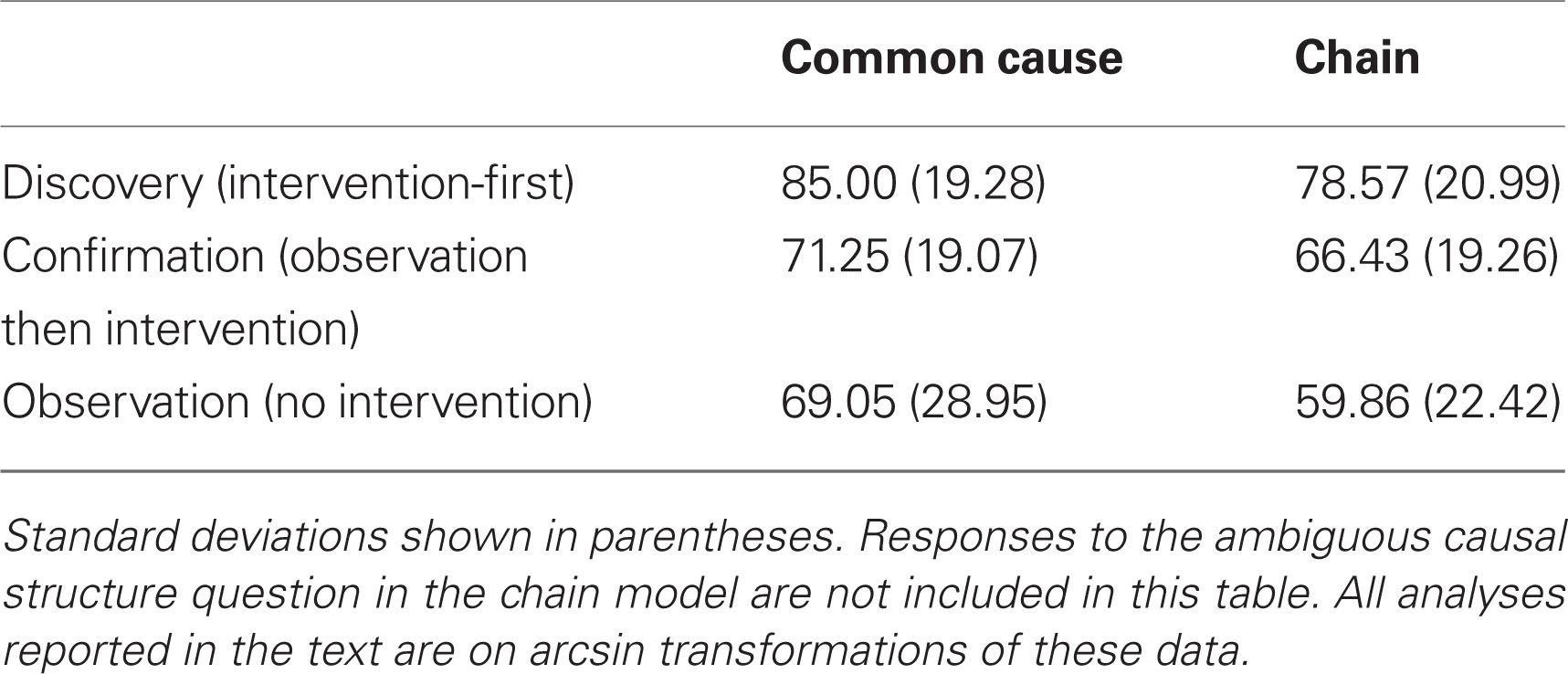
Table 2 Proportion of causal structure questions answered correctly on common cause and chain model across the learning conditions.
A 2 (Model) × 3 (Condition) mixed Analysis of Variance was performed1. Model was a within-subject factor and condition was a between-subject factors. This analysis revealed a main effect of model: overall, children were better at learning the common cause model than the chain model, F(1, 58) = 6.01, p < 0.05, Partial η2 = 0.094. A main effect of condition was also found, F(2, 58) = 5.20, p < 0.05, Partial η2 = 0.152. No significant interactions were found. Post hoc analyses revealed that children in the discovery condition outperformed children in the confirmation condition (Tukey HSD test, p = 0.05) as well as children in the observation condition (Tukey HSD test, p < 0.05)2. No significant differences were found between children in the confirmation and observation conditions. Simple effect analysis also considered the difference between the models across each condition individually. Only children in the observation condition were more likely to learn the common cause model more accurately than the chain model, t(20) = 2.27, p < 0.05, Cohen’s d = 0.39.
We also analyzed performance compared with chance responding. On both the common cause and chain models, accuracy was greater than chance (50%) in both the discovery, t(19) = 8.11 and 6.09, both p-values < 0.01, and confirmation conditions, t(19) = 4.99 and 3.81, both p-values < 0.01, conditions. Children in the observation condition performed significantly better than chance on the common cause model, t(20) = 3.02, p < 0.05, and marginally better than chance on the chain model, t(20) = 2.02, p = 0.057.
These data suggest that discovering the efficacy of actions influences causal learning beyond confirming the results of another’s actions, but do the actions children generate between these conditions differ? Further, do those action patterns relate to children’s accuracy across the learning conditions? To address these questions, we analyzed whether children’s free play (i.e., their pattern of actions on the lightbox) in the discovery and confirmation conditions differed, and whether meaningful patterns of behavior predicted accuracy. Because free play was unconstrained, children often pressed multiple buttons at the same time, or pressed one button while holding another down, resulting in confounded data. Two research assistants, unaware of the experimental hypotheses, transcribed videotapes of intervention sequences. One research assistant coded the entire data set; the other coded five children selected randomly from each of the two conditions (25% of the data). Agreement was determined in two manners: whether they agreed on the total number of button presses, and whether they agreed on the total number of individual button presses (unconfounded interventions). In both cases, agreement was 90%. The first author resolved all disagreements without knowing whether each child was in the discovery or confirmation condition.
A summary of children’s interventions is shown in Table 3. First, we examined the total number of interventions made by the child (i.e., the number of individual button presses plus the number of cases in which the child pressed at least two buttons at the same time). Although children generated more interventions in the discovery condition for both models, the differences between the conditions was not significant for either model, Mann–Whitney U = 183.50 and 189.00 for the common cause and chain models respectively, z = −0.45 and −0.30, both p-values ns. Across both conditions, there was no relation between the total number of interventions the child generated when learning that model and their accuracy on the test questions, r(40) = −0.05 and 0.18 for the common cause and chain models respectively, both p-values ns.
We next examined whether the number of unconfounded interventions (i.e., individual, single button presses) differed between the conditions and affected causal learning. On both models, children made more unconfounded interventions in the discovery condition, Mann–Whitney U = 125.00 and 110.50 for the common cause and chain models respectively, z = −2.03 and −2.43, both p-values < 0.05. However, accuracy on the test questions did not relate to the total number of unconfounded interventions the children generated, r(40) = −0.06 and 0.14 for the common cause and chain models respectively, both p-values ns. Further, the proportion of unconfounded interventions to confounded interventions each child generated also did not predict learning accuracy, r(40) = −0.06 and 0.09, both p-values ns.
We next considered whether children chose to generate data in a structured manner. Our first measure of structure was whether children simply pressed the same button repeatedly, as opposed to making an intervention on one button and then a different one. We examined the number of times children repeatedly pressed any of the four buttons (a “run” of button presses). As an example, pressing the red button six times in a row, then the yellow button, and then the green button five times would be scored as two runs (one on red, another on green). Children in the discovery condition generated more runs than those in the confirmation condition, Mann–Whitney U = 117.00 and 73.00 for the common cause and chain models respectively, z = −2.32 and −3.52, both p-values < 0.05. The number of runs the child generated was correlated with accurate responses on the chain model, r(40) = 0.40, p < 0.05, but not the common cause model, r(40) = −0.04, ns. The correlation between runs and accuracy on the chain model was marginally significant when condition was factored out, r(37) = 0.279, p = 0.085.
Second, we considered the actual number of times children pressed buttons repeatedly. In the example above, the two runs would equate to nine repeat button presses (i.e., A press of the red button was repeated five times, and the green button was pressed repeatedly four times). Because these data are confounded with the total number of unconfounded interventions children generated, we analyzed the proportion of those interventions that were repeated. Children in the discovery condition had a greater proportion of repeated unconfounded interventions when learning the chain model, Mann–Whitney U = 100.00, z = −2.74, p < 0.01. No difference was found on the common cause model. Across the conditions, the proportion of repeated unconfounded interventions generated by the child was correlated with accurate responses on the chain model, r(40) = 0.37, p < 0.05, but not the common cause model, r(40) = −0.10, ns. As in the above analysis, the correlation between of the proportion of unconfounded interventions that were repeated and learning accuracy on the chain model was marginally significant when intervention condition was factored out, r(37) = 0.29, p = 0.075.
Discussion
Four-year-olds whose actions discovered the efficacy of events in a causal system were more accurate at learning causal relations than children whose actions confirmed the efficacy of another’s actions, or children who just observed another person act on the machine. Even though children in the discovery and confirmation conditions were allowed to engage in free play with the lightbox for as long as they wanted, their ability to demonstrate what they had learned from those interventions differed.
The effect of discovery on causal learning does not appear to be an artifact in our comparison between the discovery and confirmation condition. For instance, it could be that children were more interested, and thus more engaged by, the task in the discovery condition than the confirmation condition. We cannot explicitly rule out this possibility, but aspects of the procedure and results speak against this conclusion. First, it is not clear that a single demonstration of each button would result in less engagement overall, particularly because (at least for the causal chain), the exact causal structure was not specified by just observing these data. Second, because children in the discovery condition were required to observe the experimenter activate each button after their free play period, they could also have become unengaged by this observation. Finally, if engagement is driving our results, then one would expect children to be more accurate when they can play with the lightbox than when they just watched. However, there was no difference in performance between the confirmation and observation conditions. This null result (in light of the positive result between the discovery and confirmation conditions) is hard to explain if engagement with the task is solely driving the difference between the discovery and confirmation conditions.
A similar concern with concluding that discovery aids children’s causal learning is that children in the confirmation condition might have believed that they learned the causal structure after the experimenter’s demonstration, and then forget those causal relations during the free play period. Children might have also become distracted by the free play task in the confirmation condition, and forgotten the demonstration they observed. Neither of these concerns appears to be warranted; if they were, then children would not have shown any difference between the discovery and observation conditions. Moreover, few aspects of the nature of children’s own action affected their ability to learn the models, and no aspect of the data that we analyzed affected learning across both of the models.
A point we have not emphasized is that learning across all the conditions, and especially the two in which children were allowed to generate their own interventions, was quite accurate. We draw two conclusions from these results. First, children appeared to understand the novel causal system we presented. This might be unsurprising, given that there are many investigations in which children reason about various novel causal systems (e.g., Siegler, 1975; Bullock et al., 1982; Shultz, 1982; Sophian and Huber, 1984; Gopnik and Sobel, 2000; Schulz et al., 2007). But it is rare that the causal system about which children must reason has conflict with their authentic causal knowledge; there are few instances of lights causing other lights to activate. Some have suggested that children’s inferences are different in this circumstance (e.g., Berzonsky, 1971; Cohen and Oakes, 1993). However, in our procedure, children received much direct instruction about the causal system, in which both buttons caused lights to activate as well as other lights. Perhaps this familiar knowledge helped to bootstrap children’s understanding. Moreover, Buchanan and Sobel (2010) have suggested that preschoolers’ causal inferences are similar when the novel environment in which they must reason is consistent, neutral, or in conflict with their authentic causal knowledge (as least about electronics, the media used here).
Second, and more importantly, children’s overall accuracy suggests that discovering causal structure helps causal learning, but does not solely account for the advantage provided by acting on the environment. We would suggest that in addition to conditional probability, temporal cues, and decision demands, a small but significant advantage to the process of causal learning comes from discovering causal structure. Bruner (1961) suggested several reasons for this benefit. One possibility is that children treat their discoveries as more intrinsically rewarding, and as a result are more motivated to learn when they act first as opposed to when they have been shown the efficacy of all the buttons. A second possibility is that children might be more likely to remember the results of their own discoveries; observing the experimenter press buttons at the end of the discovery condition might consolidate children’s observations into a more easily accessible memory, which is accessed when the child is asked the causal structure questions. Prior work indicates that both children and adults show greater memory for self-generated versus experimenter-performed actions (Engelkamp and Zimmer, 2001; Hornstein and Mulligan, 2004; Yamamoto et al., 2004; Sommerville and Hammond, 2007). A third possibility is that when children act first, they are more inclined to recognize heuristics and strategies that guide their learning – such as connecting what they observed during the familiarization with the task at hand. We suggest that each of these might contribute to preschoolers’ causal learning.
We considered three conditions in the experiment – in which children intervene first then observe, observe first then intervene, or only observe. Because children did not differ in their learning from observation then intervention and only observation, one might also consider how children respond to a condition in which they only intervene. We believe there are two possible patterns of response. One would be there would be no difference between the present discovery condition. This pattern of response might support an advantage of pure discovery learning as opposed to learning from more guided discovery (Mayer, 2004). We suspect that this would not be the case. In the present experiment, children were given explicit instructions and guidance that potentially constrained the nature of their free play (for example, no child played with the lightbox in any manner other than pressing the buttons). As a result, we would suspect that such a condition would result in learning on par with the observation then intervention or observation alone conditions. Guided discovery involves providing children with constraints upon their interventions, but also consolidating and reviewing the results of those actions. Future research should investigate how each of these aspects of our procedure independently influences children’s causal learning.
What we have not considered is whether similar effects of discovery in a novel causal learning environment are in place in older children. Data from adults (e.g., Lagnado and Sloman, 2004; Sobel and Kushnir, 2006) and older children (e.g., Kuhn and Ho, 1980) suggests that discovery plays some role in causal learning, but its effects have not been isolated. Investigating the role of discovery in older children’s learning might also shed light on the relation between the role of discovery (or learning from one’s own actions in general) in causal learning and the role of discovery in educational settings. For instance, in many middle school science classrooms, teachers present a laboratory demonstration, and then ask students to generate the same set of actions. We suggest that there is line of research that could consider the benefits of reversing those actions – using direct instruction to guide children through a topic, question, method, and possible solution, but allowing them to discover data first as opposed to confirming their teacher’s actions. Future research could consider this question.
Conflict of Interest Statement
The authors declare that the research was conducted in the absence of any commercial or financial relationships that could be construed as a potential conflict of interest.
Acknowledgments
This work was supported by NSF (DLS-0518161). We would like to thank all of the parents and children who participated in this research. We would also like to thank Esra Aksu, Emily Blumenthal, Claire Cook, Emily Hopkins, and Kristen Sylvester who helped with data collection and coding, and Philip Parker who helped with stimulus design.
Footnotes
- ^Because the lightbox worked in a deterministic manner, the indirect causal question (Does A→C) on the chain model was ambiguous (the data were equally consistent with it being true or false). Thus, it was not included in the analyses. As such, scores on the common cause model were a proportion out of eight questions, and scores on the chain model were a proportion out of seven questions. The analyses presented here were performed on an arcsin transformation of these proportions. The same is true for the correlations reported in the next section.
- ^If we were to treat the comparisons with the observation condition as a control comparison and use a Dunnett correction instead of the Tukey, we obtain the same significance levels.
References
Berzonsky, M. D. (1971). The role of familiarity in children’s explanations of physical causality. Child Dev. 42, 705–715.
Bredderman, T. (1983). Effects of activity-based elementary science on student outcomes: a quantitative synthesis. Rev. Educ. Res. 53, 499–518.
Buchanan, D. W., and Sobel, D. M. (2010). “Causal stream location effects in preschoolers,” in Proceedings of the 32nd Annual Meeting of the Cognitive Science Society, Portland, OR.
Bullock, M., Gelman, R., and Baillargeon, R. (1982). “The development of causal reasoning,” in The Developmental Psychology of Time, eds W. J. Friedman (New York: Academic Press), 209–254.
Cohen, L. B., and Oakes, L. M. (1993). How infants perceive simple causality. Dev. Psychol. 29, 421–433.
Engelkamp, J., and Zimmer, H. (2001). Categorical and order information in free recall of action phrases. Psicologica 22, 71–96.
Fireman, G., Kose, G., and Solomon, M. J. (2003). Self-observation and learning: the effect of watching oneself on problem solving performance. Cogn. Dev. 18, 339–354.
Gopnik, A., and Sobel, D. M. (2000). Detecting blickets: how young children use information about novel causal powers in categorization and induction. Child Dev. 71, 1205–1222.
Gopnik, A., Sobel, D. M., Schulz, L., and Glymour, C. (2001). Causal learning mechanisms in very young children: two, three, and four-year-olds infer causal relations from patterns of variation and co-variation. Dev. Psychol. 37, 620–629.
Haith, M. M. (1993). “Future-oriented processes in infancy: the case of visual expectations,” in Visual Perception and Cognition in Infancy, ed. C. Granrud (Hillsdale, NJ: Erlbaum), 235–264.
Harris, P. L., German, T., and Mills, P. (1996). Children’s use of counterfactual thinking in causal reasoning. Cognition 61, 233–259.
Hornstein, S. L., and Mulligan, N. W. (2004). Memory for actions: enactment and source memory. Psychon. Bull. Rev. 11, 367–372.
Inagaki, K., and Hatano, G. (1993). Young children’s understanding of the mind-body distinction. Child Dev. 64, 1534–1549.
Johnson, S. P., Amso, D., and Slemmer, J. A. (2003). Development of object concepts in infancy: evidence for early learning in an eye-tracking paradigm. Proc. Natl. Acad. Sci. USA 100, 10568–10573.
Kittel, J. E. (1957). An experimental study of the effect of external direction during learning on transfer and retention of principles. J. Educ. Psychol. 48, 391–405.
Kuhn, D., and Ho, V. (1980). Self-directed activity and cognitive development. J. Appl. Dev. Psychol. 1, 119–133.
Kushnir, T., and Gopnik, A. (2005). Young children infer causal strength from probabilities and interventions. Psychol. Sci. 16, 678–683.
Kushnir, T., Wellman, H. M., and Gelman, S. A. (2009). A self-agency bias in children’s causal inferences. Dev. Psychol. 45, 597–603.
Lagnado, D. A., and Sloman, S. (2004). The advantage of timely intervention. J. Exp. Psychol. Learn. Mem. Cogn. 30, 856–876.
Lagnado, D. A., and Sloman, S. (2006). Time as a guide to cause. J. Exp. Psychol. Learn. Mem. Cogn. 32, 451–460.
Mayer, R. E. (2004). Should there be a three-strikes rule against pure discovery learning? The case for guided methods of instruction. Am. Psychol. 59, 14–19.
Meltzoff, A. N. (1988). Infant imitation and memory: nine-month-olds in immediate and delayed tests. Child Dev. 59, 217–225.
Piaget, J. (1952). The Origins of Intelligence in Children. Madison, WI: International Universities Press.
Schult, C. A., and Wellman, H. M. (1997). Explaining human movements and actions: children’s understanding of the limits of psychological explanation. Cognition 62, 291–324.
Schulz, L., and Bonawitz, E. B. (2007). Serious fun: preschoolers play more when evidence is confounded. Dev. Psychol. 43, 1045–1050.
Schulz, L. E., Gopnik, A., and Glymour, C. (2007). Preschool children learn causal structure from conditional independence. Dev. Sci. 10, 322–332.
Siegler, R. S. (1975). Defining the locus of developmental differences in children’s causal reasoning. J. Exp. Psychol. 20, 512–525.
Sobel, D. M., and Kirkham, N. Z. (2006). Blickets and babies: the development of causal reasoning in toddlers and infants. Dev. Psychol. 42, 1103–1115.
Sobel, D. M., and Kushnir, T. (2006). The importance of decision demands in causal learning from interventions. Mem. Cognit. 34, 411–419.
Sommerville, J. A., and Hammond, A. J. (2007). Treating another’s actions as one’s own: children’s memory of and learning from joint activity. Dev. Psychol. 43, 1003–1018.
Sommerville, J. A., and Woodward, A. L. (2005). Pulling out the intentional structure of action: the relation between action processing and action production in infancy. Cognition 95, 1–30.
Sommerville, J. A., Woodward, A. L., and Needham, A. (2005). Action experience alters 3-month-old infants’ perception of others’ actions. Cognition 96, B1–B11.
Sophian, C., and Huber, A. (1984). Early developments in children’s causal judgments. Child Dev. 55, 512–526.
Steyvers, M., Tenenbaum, J. B., Wagenmakers, E. J., and Blum, B. (2003). Inferring causal networks from observations and interventions. Cogn. Sci. 27, 453–489.
Keywords: causal learning, interventions, self-generated action
Citation: Sobel DM and Sommerville JA (2010) The importance of discovery in children’s causal learning from interventions. Front. Psychology 1:176. doi: 10.3389/fpsyg.2010.00176
Received: 04 June 2010;
Paper pending published: 07 June 2010;
Accepted: 01 October 2010;
Published online: 02 November 2010.
Edited by:
Susan C. Johnson, Ohio State University, USACopyright: © 2010 Sobel and Sommerville. This is an open-access article subject to an exclusive license agreement between the authors and the Frontiers Research Foundation, which permits unrestricted use, distribution, and reproduction in any medium, provided the original authors and source are credited.
*Correspondence: David M. Sobel, Department of Cognitive, Linguistic and Psychological Sciences, Brown University, Box 1821, Providence, RI 02912, USA. e-mail:ZGF2ZV9zb2JlbEBicm93bi5lZHU=