- Laboratoire de Psychologie Cognitive, Department of Psychology, National Center for Scientific Research, Provence University, Marseille, France
A commentary on
Practice effects in large-scale visual word recognition studies: a lexical decision study on 14,000 Dutch mono- and disyllabic words and nonwords.
by Keuleers, E., Diependaele, K., and Brysbaert, M. (2010). Front. Psychol. 1:174. doi: 10.3389/fpsyg.2010.00174.
The Dutch Lexicon Project (DLP, Keuleers et al., 2010) is the third published database providing lexical decision times for a large number of items (after the ELP, Balota et al., 2007, and the FLP, Ferrand et al., 2010). In this commentary, we address the issue of the amount of item variance that models should really try to account for in the DLP (Spieler and Balota, 1997).
As noted by Seidenberg and Plaut (1998), to test the descriptive adequacy of simulation models with item-level databases, one needs to estimate the amount of error variance (i.e., sources of variance that are unspecific to item processing and that models cannot, in principle, capture) and, conversely, the amount of item variance that models should try to account for. One way to address this issue is to create independent groups of participants from a single database, and to compute the correlation between the item performances averaged over participants in each group (Courrieu et al., in press; Rey et al., 2009). One can show that the expected value of such correlations has the form of an intraclass correlation coefficient (ICC):

where ρ is the ICC, n is the number of participants per group, and q is the ratio of the item related variance on the noise variance for the considered database (for more details, see Courrieu et al., in press or Rey et al., 2009).
As discussed in Courrieu et al. (in press), there are basically two methods for estimating ρ and q. The first one is based on a standard analysis of variance (ANOVA) of the database. This method is fast, accurate, and it provides suitable confidence limits for the ICC estimate. The other method is of Monte Carlo type. It is based on a permutation resampling procedure, which is computationally more demanding and more sensitive to missing data than the ANOVA method. However, this approach is distribution free and much more flexible than the ANOVA.
In order to apply these methods, the database needs to be available in the form of a m × n table, where m is the number of items, and n is the number of participants. The DLP database clearly fulfils this requirement, with m = 14089, and n = 39. The ELP and FLP databases are more problematic from this point of view because each participant provided data only for a subset of the whole set of items. A possible solution is to create “virtual” participants by mixing the data of various participants, previously transformed to z-scores (Faust et al., 1999), but this needs further investigations.
Fortunately, no such a problem occurs with the DLP database, however, the important proportion of missing data in this database (16%) prevents from applying the permutation resampling method. Nevertheless, an ANOVA based analysis provided an overall ICC equal to 0.8448, with a 99% confidence interval of (0.8386, 0.8510), indicating that this database contains about 84.5% of reproducible item variance1. A model that accounts for less than 83.86% of the empirical item variance probably under-fits the data, while a model that accounts for more than 85.10% of the empirical item variance probably over-fits the data (in general because it uses too many free parameters). Of course, this estimation is task-dependent and language dependent. Using a different task, a different language, a different set of items (e.g., monosyllabic or disyllabic words), or a different population sample (e.g., older adults) might generate different outcomes.
Because this analysis has already been applied to different large-scale databases using different experimental paradigms and different languages (i.e., a naming task with English and French disyllabic words, Courrieu et al., in press, and a perceptual identification task with English monosyllables, Rey et al., 2009), it is now possible to directly compare these results. Indeed, for each database, a different q ratio has been estimated and one can now plot the resulting evolution of the ICC as a function of the number of participants for each database (see Figure 1). This figure clearly shows that there are important variations across experimental paradigms and languages (or population samples, which is still a confounded factor in the present situation) and that these variations can be explicitly quantified. For example, to reach the same amount of reproducible variance obtained in the DLP database (i.e., 84.5% with 39 participants), one would need to have 90 participants in the English perceptual identification task from Rey et al. (2009).
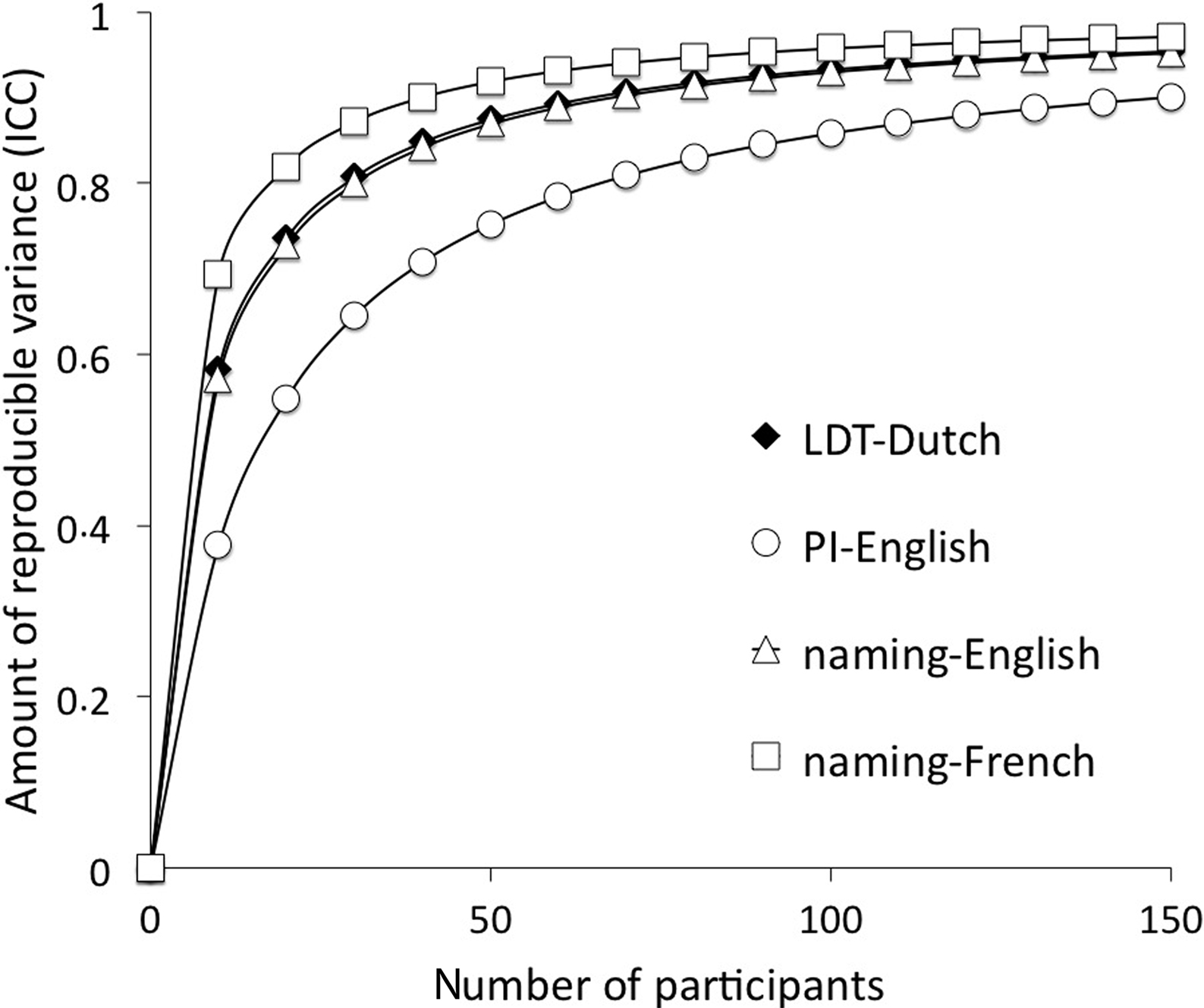
Figure 1. Evolution of the amount of reproducible variance ICC as a function of the number of participants in four databases: the DLP (Lexical decision task in Dutch, LDT-Dutch), Rey et al. (2009; Perceptual identification task with English monosyllables, PI-English), Courrieu et al. (in press; Naming disyllables in English and French: naming-English + naming-French). For each of these databases, the estimated q parameter was respectively: 0.1396, 0.0607, 0.1333, 0.2269.
To conclude, the purpose of the present commentary was to provide a precise estimate of the amount of reproducible variance that is present in the DLP database and to compare the evolution of the reproducible variance across tasks or languages. By providing this information, it is now possible to precisely test the descriptive adequacy of any model that could generate item-level predictions trying to account for item variance in the DLP database.
Acknowledgment
Part of this study was funded by ERC Research Grant 230313.
Footnote
- ^Note that the ICC is in the order of a squared correlation, therefore providing a direct estimate of the amount of reproducible variance (for a justification, see Courrieu et al., in press).
References
Balota, D. A., Yap, M. J., Cortese, M. J., Hutchison, K. A., Kessler, B., Loftis, B., Neely, J. H., Nelson, D. L., Simpson, G. B., and Treiman, R. (2007). The English Lexicon Project. Behav. Res. Methods 39, 445–459.
Courrieu, P., Brand-D’Abrescia, M., Peereman, R., Spieler, D., and Rey, A. (in press). Validated intraclass correlation statistics to test item performance models. Behav. Res. Methods doi: 10.1007/s13428-010-0002–7. [Epub ahead of print].
Faust, M. E., Balota, D. A., Spieler, D. H., and Ferraro, F. R. (1999). Individual differences in information- processing rate and amount: implications for group differences in response latency. Psychol. Bull. 125, 777–799.
Ferrand, L., New, B., Brysbaert, M., Keuleers, E., Bonin, P., Méot, A., Augustinova, M., and Pallier, C. (2010). The French Lexicon Project: lexical decision data for 38,840 French words and 38,840 pseudowords. Behav. Res. Methods 42, 488–496.
Keuleers, E., Diependaele, K., and Brysbaert, M. (2010). Practice effects in large-scale visual word recognition studies: a lexical decision study on 14,000 Dutch mono- and disyllabic words and nonwords. Front. Psychol. 1:174. doi: 10.3389/fpsyg.2010.00174.
Rey, A., Courrieu, P., Schmidt-Weigand, F., and Jacobs, A. M. (2009). Item performance in visual word recognition. Psychon. Bull. Rev. 16, 600–608.
Seidenberg, M., and Plaut, D. C. (1998). Evaluating word reading models at the item level: matching the grain of theory and data. Psychol. Sci. 9, 234–237.
Citation: Rey A and Courrieu P (2010) Accounting for item variance in large-scale databases. Front. Psychology 1:200. doi: 10.3389/fpsyg.2010.00200
Received: 24 October 2010;
Accepted: 25 October 2010;
Published online: 24 November 2010.
Copyright: © 2010 Rey and Courrieu. This is an open-access article subject to an exclusive license agreement between the authors and the Frontiers Research Foundation, which permits unrestricted use, distribution, and reproduction in any medium, provided the original authors and source are credited.
*Correspondence:YXJuYXVkLnJleUB1bml2LXByb3ZlbmNlLmZy