- 1 Laboratory of Integrative Neuroscience and Cognition, Department of Physiology and Biophysics, Georgetown University Medical Center, Washington, DC, USA
- 2 Department of Neuropsychology, Max Planck Institute for Human Cognitive and Brain Sciences, Leipzig, Germany
- 3 Center for Functional and Molecular Imaging, Georgetown University Medical Center, Washington, DC, USA
The speech signal consists of a continuous stream of consonants and vowels, which must be de- and encoded in human auditory cortex to ensure the robust recognition and categorization of speech sounds. We used small-voxel functional magnetic resonance imaging to study information encoded in local brain activation patterns elicited by consonant-vowel syllables, and by a control set of noise bursts. First, activation of anterior–lateral superior temporal cortex was seen when controlling for unspecific acoustic processing (syllables versus band-passed noises, in a “classic” subtraction-based design). Second, a classifier algorithm, which was trained and tested iteratively on data from all subjects to discriminate local brain activation patterns, yielded separations of cortical patches discriminative of vowel category versus patches discriminative of stop-consonant category across the entire superior temporal cortex, yet with regional differences in average classification accuracy. Overlap (voxels correctly classifying both speech sound categories) was surprisingly sparse. Third, lending further plausibility to the results, classification of speech–noise differences was generally superior to speech–speech classifications, with the no\ exception of a left anterior region, where speech–speech classification accuracies were significantly better. These data demonstrate that acoustic–phonetic features are encoded in complex yet sparsely overlapping local patterns of neural activity distributed hierarchically across different regions of the auditory cortex. The redundancy apparent in these multiple patterns may partly explain the robustness of phonemic representations.
Introduction
Speech perception requires a cascade of processing steps that lead to a surprisingly robust mapping of the acoustic speech stream onto phonological representations and, ultimately, meaning. This is only possible due to highly efficient acoustic decoding and neural encoding of the speech signal throughout various levels of the auditory pathway. Imagine listening to a stream of words beginning with dee…, goo…, or dow…, uttered by different talkers: One usually does not experience any difficulty in perceiving, categorizing, and further processing these speech sounds, although they may be produced, for example, by a male, female, or child, whose voices differ vastly in fundamental frequency. The mechanisms with which the brain accomplishes the invariant categorization and identification of speech sounds and which subareas of auditory cortex are crucially involved remains largely unexplained. Some of the relevant cortical structures have been identified in recent years through microelectrode recordings in non-human primates and by using neuroimaging techniques in humans. It has been shown repeatedly that structures surrounding the primary (or core) areas of auditory cortex are critically involved in speech perception. In particular, research conducted over the last 10 years has demonstrated consistently that the anterior and lateral parts of the superior temporal gyrus (STG) and superior temporal sulcus (STS) are activated more rigorously by speech sounds than by non-speech noise or pseudo-speech of similar acoustic complexity (Binder et al., 2000; Scott et al., 2000; Davis and Johnsrude, 2003; Liebenthal et al., 2005; Warren et al., 2005; Obleser et al., 2006; Rauschecker and Scott, 2009). However, there is an ongoing debate as to whether these anterior–lateral areas actually house abstract and categorical representations of speech sounds. Other authors have argued for the importance of posterior STG/STS in phonetic–phonological processing (e.g., Okada et al., 2010). A third position would be that the neural speech sound code is completely distributed and does not have a defined locus of main representation at all.
We hypothesize that local activation patterns providing segregation of acoustic–phonetic features occur most frequently in higher areas of auditory cortex (Wang, 2000; Tian et al., 2001; Read et al., 2002; Zatorre et al., 2004). A robust approach to test this hypothesis would be to analyze the anatomical distribution and mean accuracy of local classifying patterns across areas of the superior temporal cortex. Although functional magnetic resonance imaging (fMRI) is a technique that averages over a vast number of neurons with different response behaviors in each sampled voxel, it can be used to detect complex local patterns that extend over millimeters of cortex, especially when comparably small voxels are sampled (here less than 2 mm in each dimension) and multivariate analysis methods are used (Haxby et al., 2001; Haynes and Rees, 2005; Kriegeskorte et al., 2006; Norman et al., 2006). Particularly relevant to phoneme representation, these methods are capable of exploiting the richness and complexity of information across local arrays of voxels rather than being restricted to broad BOLD amplitude differences averaged across large units of voxels (for discussion see Obleser and Eisner, 2009). Notably, a seminal study by Formisano et al. (2008) demonstrated in seven subjects robust above-chance classification for a set of isolated vowel stimuli (around 65% correctness when training and testing the classifier on single trials of data) in temporal areas ranging from lateral Heschl’s gyri anterior and posterior, down into the superior temporal sulcus. The study demonstrated the power of the multivariate method and its applicability to problems of speech sound representation. However, one might expect regional variation in accuracy of classification across superior temporal cortex, an issue not specifically addressed by the study of Formisano et al. (2008). Moreover, in order to approach the robustness with which speech sounds are neurally encoded, it is important to consider that such sounds are rarely heard in isolation. Two next steps follow immediately from this, covered in the present report.
First, the direct comparison of the information contained in activation patterns for speech versus noise (here, consonant-vowel syllables versus band-passed noise classification) to the information for within-speech activation patterns (e.g., vowel classification) will help understand the hierarchies of the subregions in superior temporal cortex. Second, the systematic variation of acoustic–phonetic features not in isolated vowels, but in naturally articulated syllables will put any machine-learning algorithm presented with such complex data to a more thorough test.
To this end, we chose naturally produced syllables, built from two categories of stop-consonants ([d] vs. [g]) and two broad categories of vowels ([u:, o:] vs. [i:, e:]). Importantly, such an orthogonal 2 × 2 design allows to disentangle, within broad activations of the superior temporal cortex by speech, local voxel activation patterns most informative for decoding the heard vowel quality (back vowels [u:, o:] vs. front vowels [i:, e]; see Figure 1; see also Obleser et al., 2004b, 2006) from patterns relevant for decoding the heard stop-consonant quality of a syllable. Fortunately, the acoustic correlates of the “place of articulation” feature, for instance, are well defined for both vowels and consonants (e.g., Blumstein and Stevens, 1980; Lahiri et al., 1984) and are therefore suitable to test this hypothesis. By including a conventional contrast (speech versus band-passed noise), results from this design will be able to compare the relative gain offered by multivariate analysis methods over classical (univariate) analyses and help to resolve questions of hierarchical processing in the auditory brain.
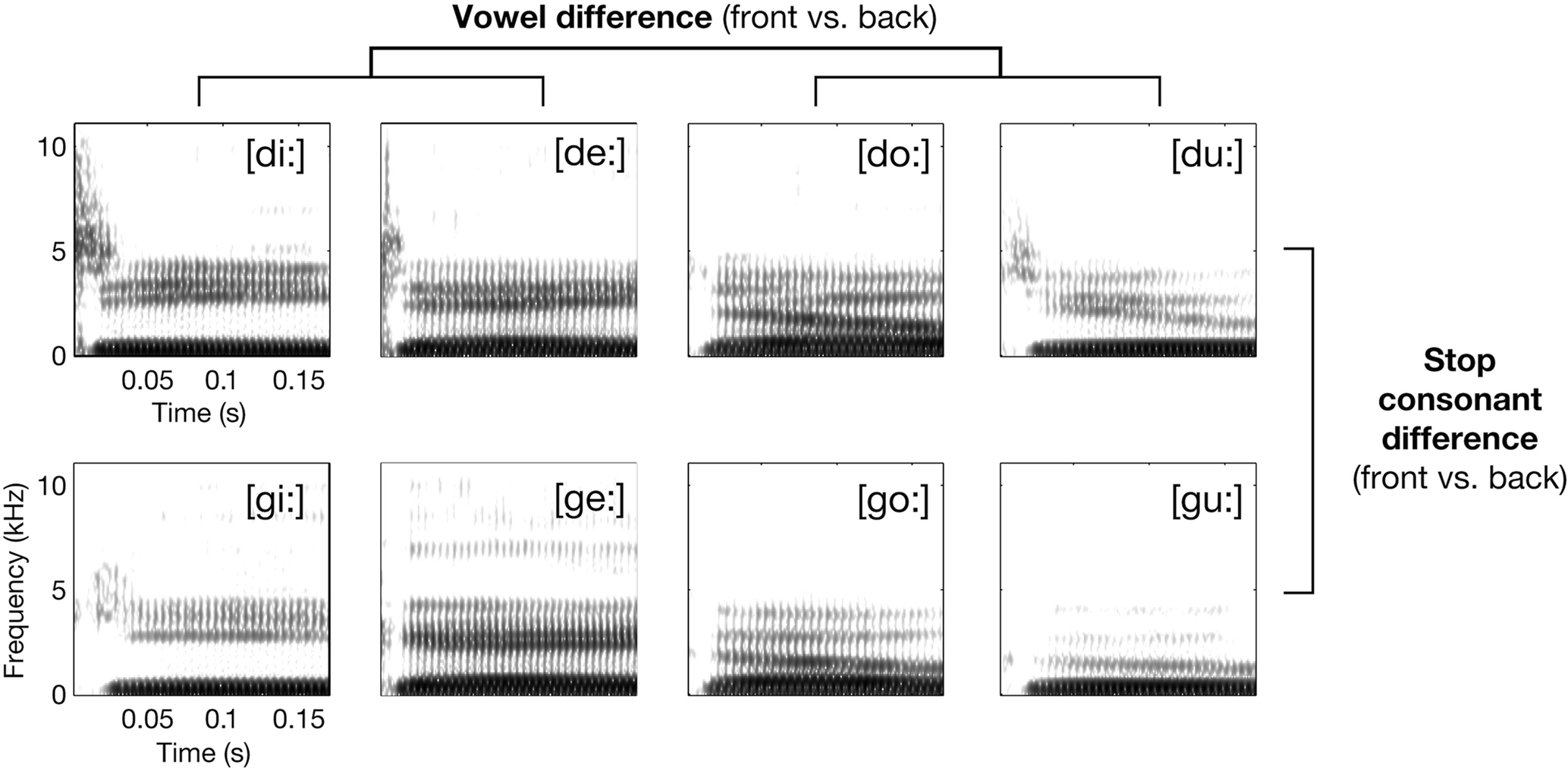
Figure 1. Exemplary spectrograms of stimuli from the respective syllable conditions (waveform section of consonantal burst has been amplified by +6 dB before calculating spectrogram for illustration only). The principal 2 × 2 design realized by the selection of phonetic features for the syllables is also shown.
By first training a classifier on the auditory brain data from a set of participants (independent observations) and testing it then on a new set of data (from another subject; repeating this procedure as many times as there are subjects), and by using the responses to natural consonant–vowel combinations as data, this challenging classification problem is most suited to query the across-subjects consistency of neural information on speech sounds for defined subregions of the auditory cortex. Also, we will compare the speech–speech classifier performance to speech–noise classifier performance in select subregions of the superior temporal cortex in order to establish a profile of these regions’ response specificities.
Materials and Methods
Subjects
Sixteen Subjects (8 females, mean age 24.5 years, SD 4.9) took part in the study. All of the subjects were right-handed monolingual speakers of American English and reported no history of neurological or otological disorders. Informed consent was obtained from all subjects, and they received a financial compensation of $20.
Stimuli
The speech sound set consisted of 64 syllable tokens; they were acoustically variant realizations of eight American English consonant-vowel (CV) syllables: [di:] (e.g., as in “deeper”), [de:] (daisy), [du:] (“Doolittle”), [do:] (“dope”), [gi:] (“geezer”), [ge:] (“gait”), [gu:] (“Google”), and [go:] (“goat”; these words were articulated with exaggerated long vowels to allow for coarticulation-free resulting edits). Each syllable was used in four different versions, edited from single-word utterances of four monolingual native speakers (two females and two males, recorded using a DAT-recorder and a microphone in a sound-proof chamber). Figure 1 gives an overview over the spectro-temporal characteristics of the syllables. The CV syllables were selected to test for possible mapping mechanisms of speech sounds in the auditory cortices. See Appendix for extensive description of acoustic characteristics of the entire syllable set.
Experimental Design and Scanning
The paradigm contrasted the syllables in four conditions: [di:/de:], [du:/do:], [gi:/ge:], and [gu:/go:] (Figure 1). All syllables were instantly recognized as human speech and correctly identified when first heard by the subjects. Please note that there was considerable acoustic intra-conditional variance due to the four different speakers and the combined usage of [u:, o:] and [i:, e:].
As an additional non-speech reference condition, a set of eight different noise bursts was presented, comprising four different center frequencies (0.25, 0.5, 2, and 4 kHz) and two different bandwidths (one-third and one-octave), expected to activate mainly early (core and belt) areas of the auditory cortex (Wessinger et al., 2001).
All audio files were equalized with respect to sampling rate (22.05 kHz), envelope (180 ms length; no fade-in but cut at zero-crossing for syllables, 3 ms Gaussian fade-in for noise bursts; 10 ms Gaussian fade-out) and RMS intensity. Stimuli were presented binaurally using Presentation software (Neurobehavioral Systems Inc.) and a customized air-conduction sound delivery system at an average sound pressure level of 65 dB.
Functional magnetic resonance imaging was performed on a 3-Tesla Siemens Trio scanner using the standard volume head coil for radio frequency transmission. After positioning the subject, playing back a 16-item sequence of exemplary syllables from all four conditions and ensuring that subjects readily recognized all items as speech, a 42-min echo planar image acquisition period with small voxel sizes and a sparse sampling procedure started (TR = 10 s, TA = 2.48 s, 25 axial slices, resolution 1.5 mm × 1.5 mm × 1.9 mm, no gap, TE = 36 ms, 90° flip angle, 192 mm × 192 mm field of view, 128 × 128 matrix). The slices were positioned such as to cover the entire superior and middle temporal gyri and the inferior frontal gyrus, approximately parallel to the AC–PC line. In total, 252 volumes of interest were acquired; 42 volumes per condition (four syllable conditions; band-passed noises; silent trials).
Subjects were instructed to listen attentively to the sequence of sounds: Between volume acquisitions, 7500 ms of silence allowed presentation of eight acoustically different stimuli of one condition (either eight stimuli of a given syllable condition or eight noise bursts) with an onset asynchrony of 900 ms. To exemplify, for the /d/–front vowel condition in a given trial, a sequence of, e.g., [di:]male1, [de:]male2, [di:]female1, [di:]male2, [di:]female2, [de:]female2, [de:]male1, and [de:]female2 was presented in silence, followed by volume acquisition; as evident from such an exemplary sequence, many salient acoustic effects (e.g., pitch; idiosyncracies in consonant–vowel coarticulation, etc.) will average out and the volume acquisition will primarily capture an “average” activation related to the “abstract” spectro-temporal features of the syllables’ stop-consonant (alveolar /d/ or velar /g/) and vowel category (front vowels /i:, e:/ or back vowel /u:, o:/) only.
Presentation of conditions was pseudo-randomized. Four different randomization lists were used counterbalanced across participants, avoiding any unintended systematic order effects. After functional data acquisition, a 3-D high-resolution anatomical T1-weighted scan (MP-RAGE, 256 mm3 field of view, and resolution 1 mm × 1 mm × 1 mm) was also acquired. Total scanning time amounted to 55 min.
Data Analysis
All data were analyzed using SPM8 (Wellcome Department of Imaging Neuroscience). The first volume was discarded, and all further volumes were realigned to the first volume acquired and corrected for field inhomogeneities (“unwarped”). They were co-registered to the high-resolution anatomical scan. For further reference, a spatial normalization was performed (using the gray-matter segmentation and normalization approach as implemented in SPM). For further analysis strategies, mildly smoothed images (using a 3 mm × 3 mm × 4 mm Gaussian kernel) as well as entirely non-smoothed images were retained.
In order to arrive at univariate estimates of activation in all conditions against silence, the native-space image time series of individuals were modeled in a general linear model using a finite impulse response (length 1 s, first order). Scaling to the grand mean and 128-s high-pass filtering were applied. The resulting contrast images (especially the sound greater than silence contrast, the speech greater than noise contrast, as well as all four syllable greater silence contrasts) were transformed to MNI space using the normalization parameters derived in each individual earlier. Figure A1A of Appendix shows two examples of individuals’ non-smoothed activation maps in native space for the contrast sound greater than silence. Random-effects models of the univariate data were thresholded at p < 0.005 and a cluster extent of 30; a Monte Carlo simulation (Slotnick et al., 2003) ensured that this combination, given our data acquisition parameters, protects against inflated type-I errors on the whole brain significance level of α = 0.05.
Univariate fMRI analyses focus on differences in activation strength associated with the experimental conditions. Pattern or multivariate analysis, by contrast, focuses on the information contained in a region’s activity pattern changes related to the experimental conditions, which allows inferences about the information content of a region (Kriegeskorte and Bandettini, 2007; Formisano et al., 2008). For our classification purposes, we used the un-smoothed and non-thresholded but MNI-normalized maps of individual brain activity patterns, estimated as condition-specific contrasts of each condition (i.e., the SPM maps of non-thresholded t-estimates from the condition-specific contrasts, e.g., /d/–front against silence, /g/–front against silence, and so forth; see Misaki et al., 2010 for evidence on the advantageous performance of t-value-based classification; note, however, that this approach is different from training and testing on single trial data (cf. Formisano et al., 2008).
In order to ensure absolute independence of testing and training sets, we decided to pursue an across-participants classification approach. As data in our experiment had been acquired within one run, single trials of a given individual would have been too dependent on each other, and we chose to pursue an across-participants classification instead: We split our subject sample into n − 1 training data sets and a n = 1-sized testing data set. This procedure was repeated n times, yielding for each voxel and each classification task n = 16 estimates, from which an average classification accuracy could be derived. Please note that successful (i.e., significant above-chance) classification in such an approach is particularly meaningful, as it indicates that the information coded in a certain spatial location (voxel or group of voxels) is reliable across individuals.
A linear support vector machine (SVM) classifier was applied to analyze the brain activation patterns (LIBSVM Matlab-toolbox v2.89). Several studies in cognitive neuroscience have recently reported accurate classification performance using a SVM classifier (e.g., Haynes and Rees, 2005; Formisano et al., 2008), and SVM is one of the most widely used classification approaches across research fields. For each of our three main classification problems (accurate vowel classification from the syllable data; accurate stop-consonant classification from the same data; accurate noise versus speech classification), a feature vector was obtained using the t-estimates from a set of voxels (see “search-light” approach below) as feature values. In short, a linear SVM separates training data points x for two different given labels (e.g., consonant [d] vs. consonant [g]) by fitting a hyperplane wT x + b = 0 defined by the weight vector w and an offset b. The classification performance (accuracy) was tested, as outlined above, by using a leave-one-out cross validation (LOOCV) across participants’ data sets: The classifier was trained on 15 data sets, while one data set was left out for later testing the classifier in “predicting” the labels from the brain activation pattern, ensuring strict independence of training and test data. Classification accuracies were obtained by comparing the predicted labels with actual data labels and averaged across the 16 leave-one-out iterations afterward, resulting in a mean classification accuracy value per voxel.
We chose a multivariate so-called “search-light” approach to estimate the local discriminative pattern over the entire voxel space measured (Kriegeskorte et al., 2006; Haynes, 2009): multivariate pattern classifications were conducted for each voxel position, with the “search-light” feature vector containing t-estimates for that voxel and a defined group of its closest neighbors. Here, a search-light radius of 4.5 mm (i.e., approximately three times the voxel length in each dimension) was selected, comprising about 60 (un-smoothed) voxels per search-light position. Thus, any significant voxel shown in the figures will represent a robust local pattern of its 60 nearest neighbors. We ensured “robustness” by constructing bootstrapped (n = 1000) confidence intervals (CI) for each voxel patch’s mean accuracy (as obtained in the leave-one-out-procedure). Thus, we were able to identify voxel patches with a mean accuracy above chance, that is, voxel patches whose 95% CI did not cover the 50% chance level. The procedure is exemplified in Figure A2 of Appendix.
As an additional control for a possible inflated alpha error due to multiple comparisons (which is often neglected when using CI; Benjamini and Yekutieli, 2005), we used a procedure suggested in analogy to the established false discovery rate (FDR; e.g., Genovese et al., 2002), called false coverage-statement rate (FCR). In brief, we “selected” those voxels whose 95% CI for accuracy did not cover the 50% (chance) level in a first pass (see above). In a second correcting pass, we (re-)constructed FCR-corrected CI for these select voxels at a level of 1 − R × q/m, where R is the number of selected voxels at the first pass, m is the total number of voxels tested, and q is the tolerated rate for false coverage statements, here 0.05 (Benjamini and Yekutieli, 2005). See also Figure A2 of Appendix. Effectively, this procedure yielded FCR-corrected voxel-wise confidence limits at α ∼ 0.004 rather than 0.05; approximately two-thirds of all voxels declared robust classifiers at the first pass also survived this correcting second pass.
Note that all brain map overlays of average classification accuracy are thresholded to show only voxels with a bootstrapped CI lower limit not covering 50% (chance level; 1000 repetitions), while all bar graphs or numerical reports and ensuing inferences are based on the FCR-corrected data. Significance differences, as indicated by asterisks in the bar graphs, were assessed using Wilcoxon signed-rank tests, corrected for multiple comparisons using false discovery rate (FDR, q = 0.05) correction.
Results
Univariate Contrasts Analysis
All 16 subjects were included in the analysis, as they exhibited bilateral activation of temporal lobe structures when global contrasts of any auditory activation were tested. Examples are shown in Figure A1 of Appendix.
As predicted, the global contrast of speech sounds over band-pass filtered noise (random effects, using the mildly smoothed images) yielded focal bilateral activations of the lateral middle to anterior aspects of the STG extending into the upper bank of the STS (Figure 2).
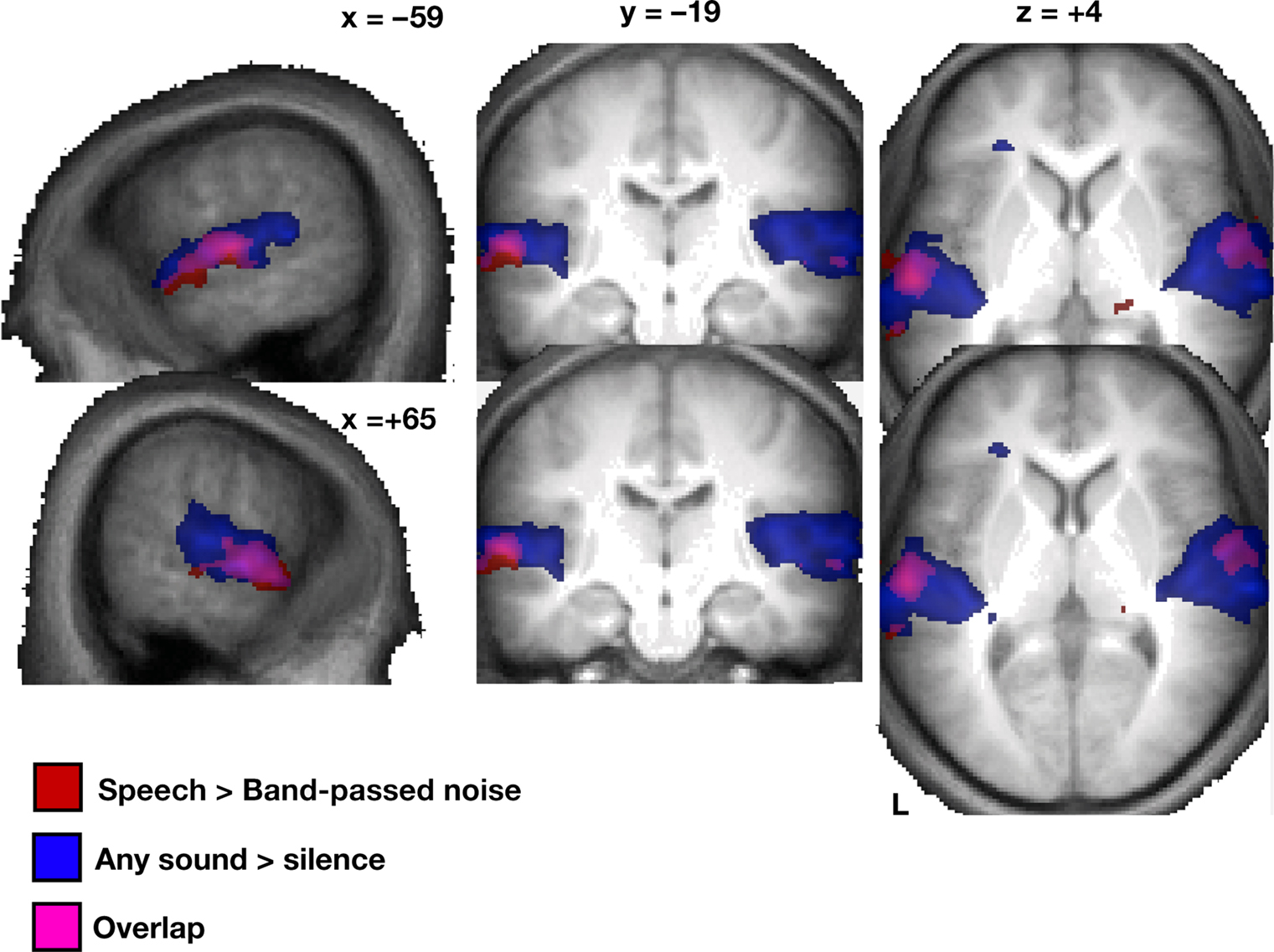
Figure 2. Random effects (N = 16) of the univariate analysis for speech > band-passed noise (red) and sound > silence (blue; overlay purple) based on smoothed and normalized individual contrast maps, thresholded at p < 0. 005 at the voxel level and a cluster extent of at least 30 voxels; displayed on an average of all 16 subjects’ T1-weighted images (normalized in MNI space).
In order to reveal cortical patches that might show stronger activation of one vowel type over another or one stop-consonant type over another, we tested the direct univariate contrasts of syllable conditions or groups of conditions against each other. However, none of these contrasts yielded robust significant auditory activations at any reasonable statistical threshold (p < 0.001 or p < 0.005 uncorrected). This negative result held true on a whole-volume level as well as within a search volume restricted by a liberally-thresholded (p < 0.01) “sound greater than silence” contrast. This outcome is to be taken as safe indication that, at this “macroscopic” resolution which contains tens or hundreds of voxels, the different classes of speech stimuli used here do not exert broad differences in BOLD amplitudes. All further analyses thus focused on studying local multi-voxel patterns of activation (using the Search-light Approach described in Materials and Methods) rather than massed univariate tests on activation strengths.
Multivariate Pattern Classification Results
Figures 3–6 illustrate the results for robust (i.e., significantly above-chance) vowel–vowel, stop–stop-consonant, as well as noise–speech classification from local patterns of brain activity. In Figure 3, all voxels marked in color represent centroids of local 60-voxel clusters, from which correct prediction of vowel (red) or stop-consonant (blue) category of a heard syllable was possible.
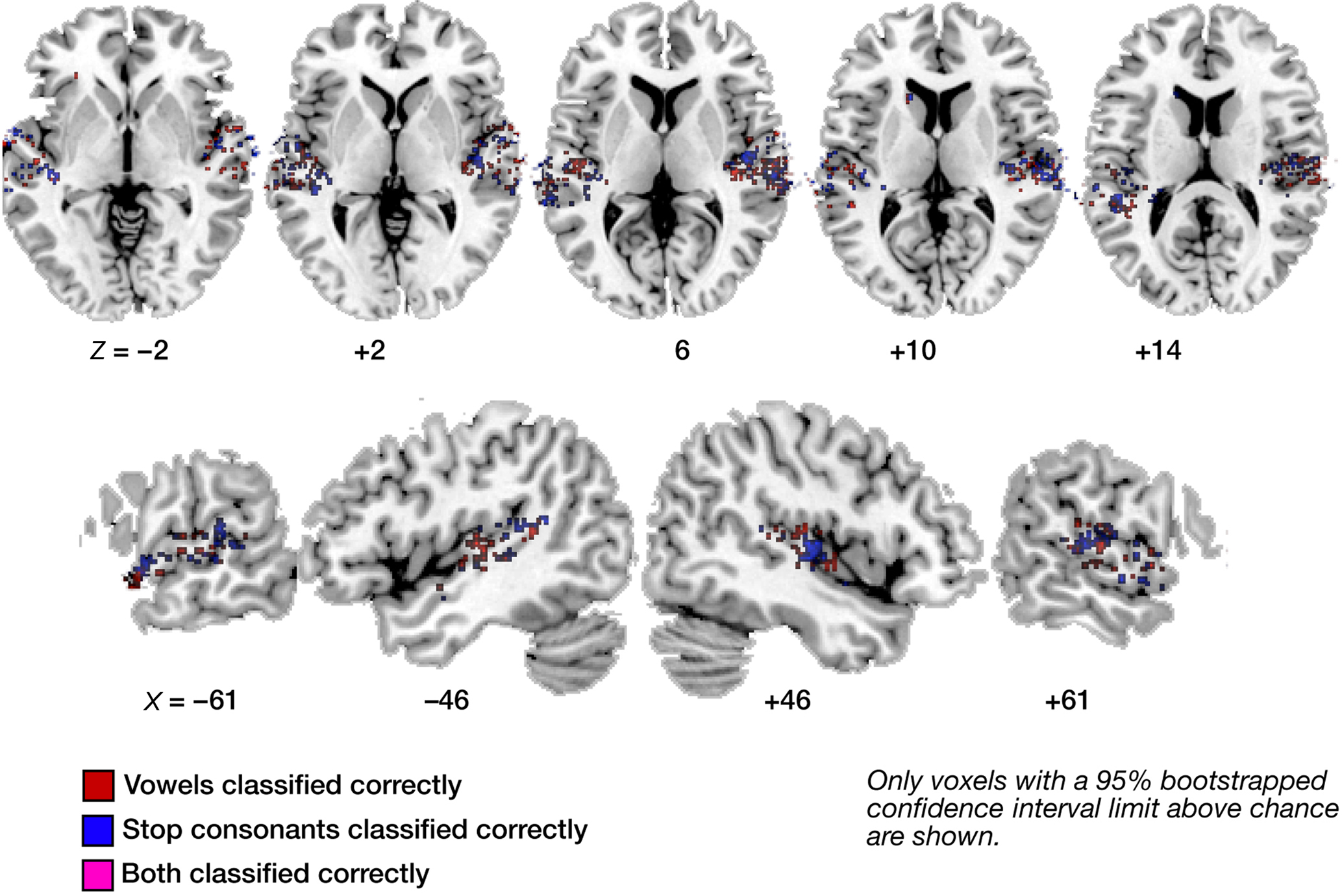
Figure 3. Maps of correct classification for vowel and stop category. Display of significantly above-chance classification voxels from the leave-one out across-subjects classification. Axial (top panels) and sagittal slices (bottom panels) are shown, arranged for left and right hemisphere view, and displayed on a standard T1 template brain. Note the sparse overlap in voxels significantly classifying vowel information (red) and stop information (blue; voxels allowing correct classification of both are marked in purple).
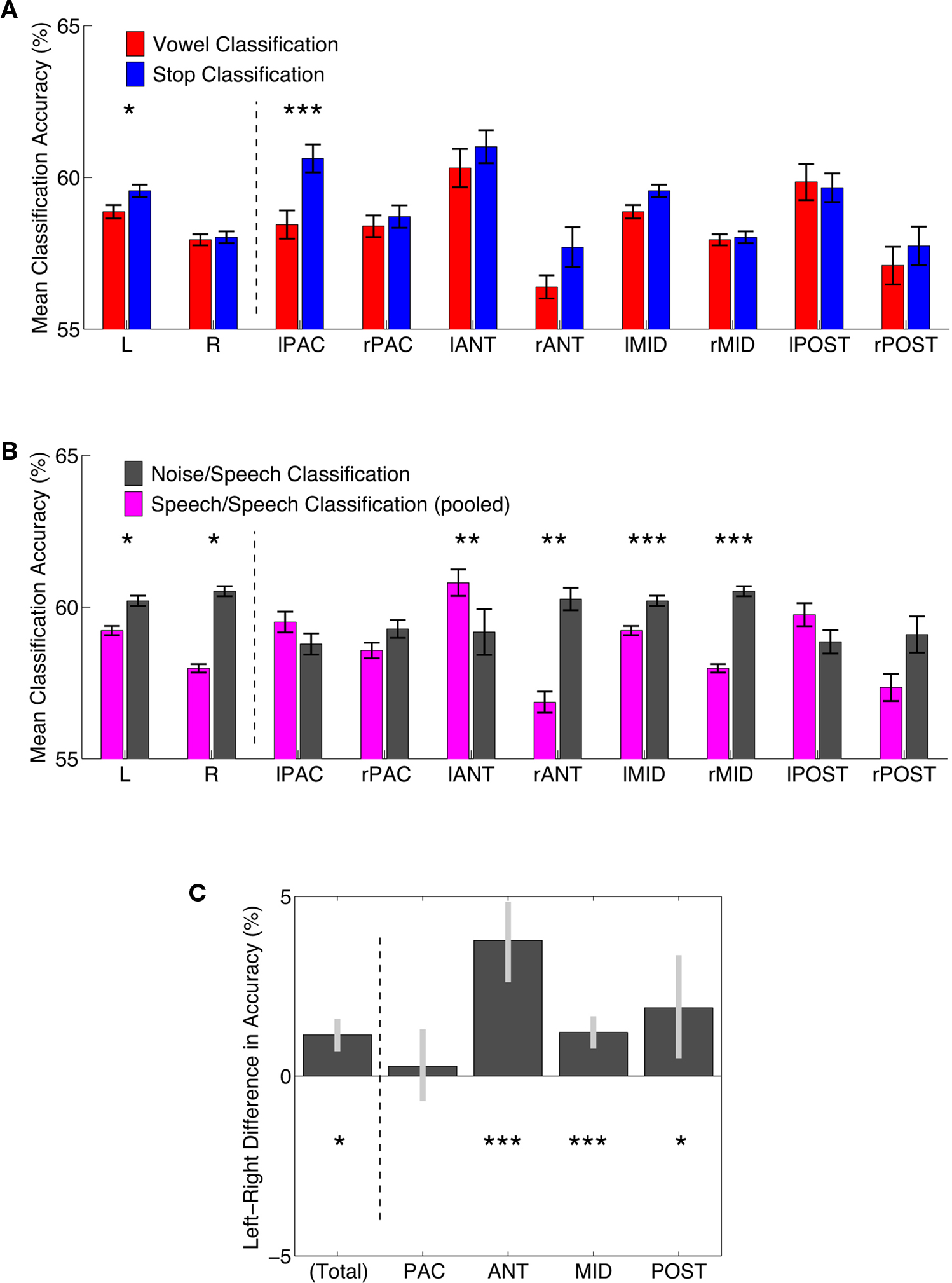
Figure 4. Comparisons of classification accuracy in regions of interest. (A) Classification accuracies for vowel (red) and stop-consonant (blue) classification. Means of all FCR-corrected above-chance voxels within a region ±1 standard error of the mean are shown. Asterisks indicate a FDR-corrected significant difference; *p < 0.05, **p < 0.01, ***p < 0.001. L, R – total average of significant left and right hemisphere voxels, respectively; for anatomical definition of subregions see Figure A3 of Appendix. (B) Classification accuracies for speech–speech classification (accuracies averaged across vowel and stop; slate gray) and noise–speech classification (purple). Means of all FCR-corrected above-chance voxels within a region ±1 standard error of the mean are shown. Asterisks indicate a FDR-corrected significant difference; *p < 0.05, **p < 0.01, ***p < 0.001. (C) Left–Right hemisphere differences for speech–speech classification (accuracies averaged across vowel and stop). Mean differences in accuracy ±95% confidence limits are shown. Asterisks indicate a FDR-corrected significant difference; *p < 0.05, **p < 0.01, ***p < 0.001. NB, the anterior regions show the most distinct left-bias for mean classification accuracy, while the primary areas show no significant bias.
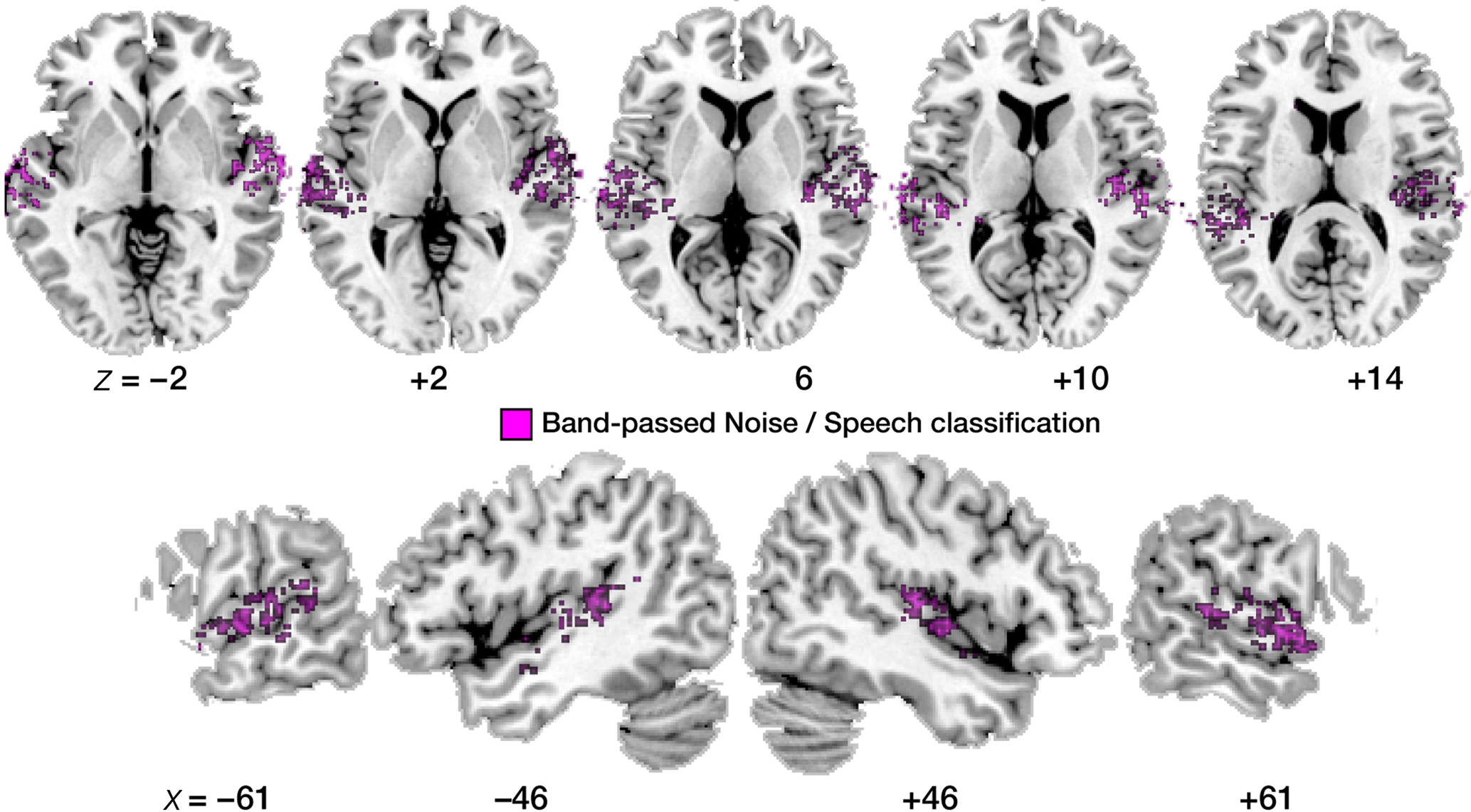
Figure 5. Maps of correct noise versus speech classification. Display of significantly above-chance classification voxels from the leave-one-out across-subjects classification. Axial slices are shown, arranged for left and right hemisphere view, and displayed on a standard T1 template brain.
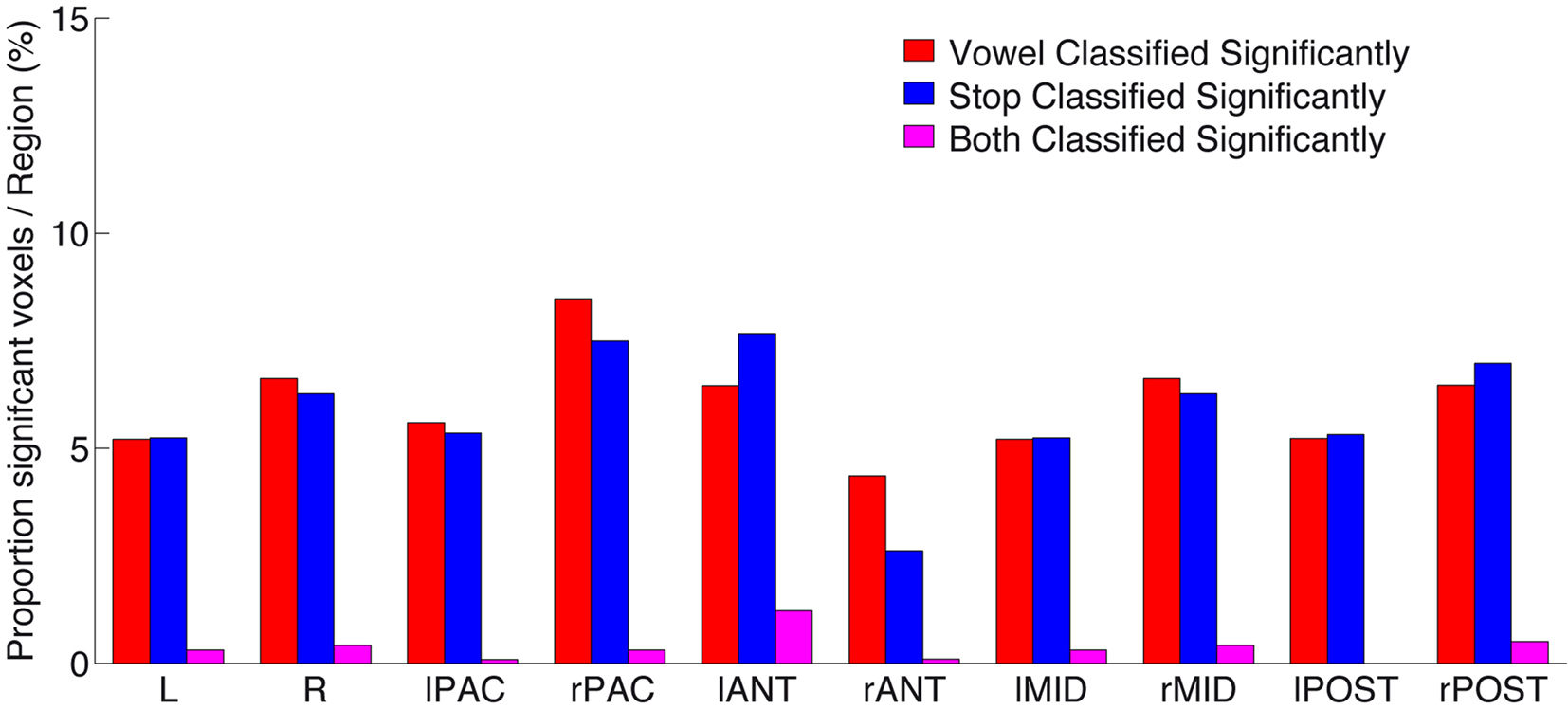
Figure 6. Proportion of significantly above-chance voxels per region, for vowel (red) classification, stop (blue) classification, as well as voxels (and their immediate neighbors; see Materials and Methods) that significantly classified both speech sound categories (“overlap,” shown in purple). Note the low number of such “overlap” voxels across all subregions. For anatomical definition of subregions see Figure A3 of Appendix.
The displayed mean accuracies per voxel resulted from averaging the results of all 16 leave-one-out classifications per voxel; a 1000-iterations bootstrap resampling test was used to retain only those voxels where the 95%-CI of the mean accuracy did not include chance level (50%). As can be seen in Figure 3, voxels that allow for such robust classification are, first, distributed throughout the lateral superior temporal cortex. Second, these voxels appear to contain neural populations that are highly selective in their spectro-temporal response properties. This is evident from the fact that voxels robustly classifying both vowel and stop-consonant categories (i.e., steady-state, formant-like sounds with simple temporal structure versus non-steady-state, sweep-like sounds with complex temporal structure) are sparse (see also Figure 6). Also, subareas of auditory core cortex (here using the definition of Morosan et al., 2001; Rademacher et al., 2001; see Figure A3 of Appendix) appear relatively spared in the left (but see noise versus speech classification results below); in the right, stop-consonant classification is above-chance in TE 1.0 and vowel classification in TE 1.1. (Figure A3A of Appendix). Figure A1B of Appendix also shows individual vowel and stop-consonant classification results for four different subjects.
These observations were followed up with a quantitative analysis of mean accuracy within regions of interest (Figure 4). Differences in mean accuracy between vowel and stop classification; differences in speech classification (average vowel and stop classification) and noise versus speech classification; and the proportion of significantly classifying voxels were tested in pre-defined regions of interest. The outline of these regions is shown in Figure A3 of Appendix. It directly resulted from the distribution of broadly “sound-activated” voxels (T15 > 3 in the “sound > silence” contrast); left and right voxels likely to be located in primary auditory cortex (PAC; TE 1.0–1.2; Morosan et al., 2001) were separated from regions anterior (ant) and posterior to it (post) as well as lateral to it (mid).
Vowels and stops were classified significantly above chance throughout these various subregions (Figure 4). In each patch, the difference in mean accuracy between vowel and stop classification was also assessed statistically to find any potential classification accuracy differences between the two broad speech sound categories. Particularly in the left auditory core region, stop-consonants were classified significantly better than vowels (Figure 4A).
Next, we directly compared accuracy in a speech versus band-passed noise classification analysis with the average accuracy in the two speech versus speech classification analyses (i.e., vowel categorization, stop-consonant classification; Figure 5). Three findings from this analysis deserve to be elaborated.
First, the noise versus speech classification (accomplished by randomly choosing one of the four speech conditions and presenting it alongside the band-passed noise condition to the classifier, in order to ensure balanced training and test sets) yielded somewhat higher average classification performances than the speech versus speech classifications described above (Figure 4B). This lends overall plausibility to the multivariate analysis, as the band-passed noise condition differs from the various syllable conditions by having much less detailed spectro-temporal complexity, and its neural imprints should be distinguished from neural imprints of syllables quite robustly by the classifier, which was indeed the case.
Second, the topographic distribution of local patterns that allowed noise versus speech classification (Figure 5) strikingly resembles the result from the univariate BOLD contrast analysis (Figure 2); most voxels robustly classifying noise from speech map to anterior and lateral parts of the superior temporal cortex; primary auditory areas also appear spared more clearly than was the case in the within-speech (vowel or consonant) classification analyses.
Third, we compared the average accuracy of vowel–vowel and stop–stop classification (speech–speech classification; purple bars in Figure 4B) and compared it statistically to noise–speech classification (gray bars). Only in the left anterior region, speech–speech classification accuracy was statistically better than noise–speech classification (p < 0.01). In PAC as well as the posterior regions, speech–speech classification accuracies were not statistically different from noise–speech classification accuracies.
A comparison of hemispheres in mean accuracy did not yield a strong hemispheric bias in accuracy. However, when again averaging accuracies across stop and vowel classification and testing for left–right differences, a lateralization to the left was seen across regions (leftmost bar in Figure 4C; p < 0.05). Also, Figure 4 shows a strong advantage in both vowel and stop classification accuracy for the left anterior region of interest (both mean stop and mean vowel accuracy >60%), when compared to its right hemisphere homolog region of interest (both <60%).
Figure 6 gives a quantitative survey of the relative sparse overlap in voxels that contribute accurately to both vowel and stop classification (cf. Figure 3). Plotted are the proportions of voxels in each subregion that allow above-chance classification (i.e., number of FCR-corrected above-chance voxels divided by number of all voxels, in % per region) of vowels (red), stop-consonants (blue), or of both these classification problems (purple). As can be seen, a proportion of less than 10% of voxels in all regions contribute significantly (corrected for multiple comparisons) to the accurate classification of speech sounds. However, more importantly, the proportion of voxels contributing accurately to both speech sound classification problems (i.e., the “overlap”) remains surprisingly low. This provides strong evidence in favor of local distributed patterns (“patches”) of spectro-temporal analysis that are most tuned either to vocalic or to consonantal features, across several subregions of human auditory cortex.
Discussion
Having participants listen to a simple 2 × 2 array of varying stop-consonant and vowel features in natural spoken syllables in a small-voxel fMRI study, we tested the superior temporal cortex for the accuracy by which its neural imprints allow the decoding of acoustic–phonetic features – across participants.
First, the univariate or by now “classic” approach of directly contrasting speech with non-speech noise stimuli yielded a clear-cut hierarchy (Figure 2), where all syllables more than band-passed noise bursts activate the anterior and lateral parts of the superior temporal cortex. This is in line with most current models of hierarchical processing in central auditory pathways (e.g., Hickok and Poeppel, 2007; Rauschecker and Scott, 2009). The peak coordinates of the univariate speech > band-passed noise contrast concur with areas in the lateral and somewhat anterior sections of the STS previously labeled as voice-sensitive as well as those labeled speech-sensitive (Belin et al., 2000; Petkov et al., 2009). This is not surprising as the literature review shows that there is, to date, no clear separation of speech versus voice-sensitive areas, and our design was not tuned toward disentangling any voice versus speech sensitivity.
However, the more critical observation here is the inability of these subtraction-based analyses to yield consistent (i.e., across participants) evidence for broad activation differences for acoustic–phonetic categories against each other. At an acceptable level of thresholding and using mildly smoothed data, no consistent activation differences for the various syllables, that is, between vowels or between consonants, could be revealed. This speaks to (i) a good overall matching of acoustic parameters that could have influenced the auditory cortical BOLD amplitudes (e.g., loudness, stimulus length), and (ii) a more microscopic level of encoding for the critical information of consonants and vowels, presumably distributed over various stages of the central auditory processing “hierarchy.” Previous MEG source localization studies, using isolated vowels or syllables, had proposed such comparably broad topographic differences, albeit at very isolated time steps (i.e., for a brief period 100 ms after vowel onset; Obleser et al., 2003, 2004b; Shestakova et al., 2004); however, no broad amplitude differences could be observed there either. Using rather complex vowel-alternating design and specific vowel-detection tasks, similar shifts in topography had been elicited in MEG as well as in fMRI (Obleser et al., 2004a, 2006). In the latter study, we had observed hints to a vowel feature separation in the anterior temporal cortex using standard univariate group statistics, but the vowel material used there had much less acoustic variance, used isolated vowels, and – as mentioned above – a very specific vowel-detection task. However, the carefully balanced syllable material, the highly increased acoustic variance in stimulus tokens, and the – arguably pivotal – absence of a task (other than attentive listening) in the current study revealed the limits of univariate subtraction analysis in studying human speech sound representation.
Second, the success of the multivariate pattern classifier stands in contrast to this and provides good evidence toward a model of speech sound representation that includes clusters of activity specific to particular speech sound categories distributed over several areas of the superior temporal cortex. To reiterate, the classifier was trained on activation data from various participants and tested on an independent, left-out set of data from another participant and had to solve a challenging task (classifying broad vowel categories or stop-consonant categories from acoustically diverse syllables).
It was only in this type of analysis that voxels (or, patches of voxels, following from the “search-light” approach) could be identified that allowed robust above-chance and across-individuals classification of vowel or stop category. The overall accuracy in classification was far from perfect, yet in a range (∼55–65%) that is comparable to the few previous studies of speech-related fMRI classification reports (Formisano et al., 2008; Okada et al., 2010; Herrmann et al., in press). However, in the current study the classifier arguably had to solve a harder problem, being trained on a variety of independent subjects and tested on another, also independent subject. Moreover, vowel and stop categories had to be classified from naturally coarticulated syllables. Adding further plausibility to these conclusions, the classifier performed significantly better overall in the acoustically simpler problem of classifying band-passed noise (i.e., uniform and simple spectro-temporal shape) from speech sound information (Figure 4B). It is also very likely that through additional sophisticated algorithms, for example, recursive feature elimination (De Martino et al., 2008), the performance of the classifier could be improved further. The “search-light” approach employed here has the further limitation of ignoring the information entailed in the co-variance (connectivity) of voxels in remote regions or hemispheres. For the purposes of this study, however, the argument stands that a patch of voxels in the left anterior region of the STG, for example, which carries information to accurately classify, for example, /d/ from /g/ in more than 60% of all (independent) subjects above chance can be taken as good evidence that the cortical volume represented by these voxels encodes relevant neural information for this stop-consonant percept.
Third, to go beyond the observation of such above-chance voxel patches across a wide range of superior temporal cortex (which has also been reported in the vowels-only study by Formisano et al., 2008), we ran a set of region-specific analyses for the average classification accuracy. The main findings from this region-specific analysis can be summarized as follows:
All eight subregions (left and right primary auditory cortex [PAC]; anterior; mid [lateral to PAC]; and posterior) carry substantial information for correctly classifying vowel categories as well as stop-consonant categories, and also the more salient noise–speech distinction (Figures 3–6). Thus, differentiation of abstract spectro-temporal features may already begin at the core and belt level, which is in line with recordings from non-human primates and rodents (e.g., Steinschneider et al., 1995; Wang et al., 1995; Tian et al., 2001; Engineer et al., 2008). Between regions, however, differences in average accuracy for vowels, stops, and noise–speech classification were observed (Figure 4). All findings taken together single out the left anterior region (i.e., left-hemispheric voxels that were (i) activated by sound in a random-effects analysis and (ii) anterior to a probabilistic border of the PAC, as suggested by Morosan et al., 2001; see Figure A3 of Appendix).
To list a conjunction of these findings, the left anterior region
• showed the highest average classification accuracy for the vowel and stop classification (>60%; Figure 4A);
• was the only region to show an average speech–speech classification accuracy that was statistically superior to the less specific noise–speech classification (Figure 4B).
• showed the most pronounced leftward lateralization, when based on average accuracy (Figure 4C, yielding a ∼4% leftward bias). This might be taken as corroborating evidence to a 2-FDG PET study on left-lateralized monkey vocalizations processing; Poremba et al., 2004.
In sum, our results for the left anterior region fit well with previous results on comparably high levels of complexity being processed in the (left) anterior parts of the superior temporal cortex (for imaging results from non-humans see, e.g., Poremba et al., 2004; Petkov et al., 2008; for imaging results in humans see, e.g., Binder et al., 2004; Zatorre et al., 2004; Obleser et al., 2006; Leaver and Rauschecker, 2010; a list that can be extended further if studies on more complex forms of linguistic information, mainly syntax, are taken into account, e.g., Friederici et al., 2000; Rogalsky and Hickok, 2009; Brennan et al., 2010). Recall, however, that the current data do not allow to draw any conclusions upon possibly discriminative information being available in the inferior frontal or inferior parietal cortex (e.g., Raizada and Poldrack, 2007), as these regions were not activated in the broad “sound > silence” comparison and were not fully covered by our chosen slices, respectively.
As for the ongoing debate whether posterior (see, e.g., Okada et al., 2010) or anterior (see evidence listed above) aspects of the central auditory processing pathways have a relatively more important role in processing spectro-temporal characteristics of speech, i.e., vowel and consonant perception, our study reaffirms the evidence for the anteriority hypothesis. The left posterior region did not show a statistically significant advantage for the speech–speech over noise–speech classification, and its voxels showed overall weaker classification accuracies than the left anterior region. The evidence presented in this study, therefore, adds compellingly to the existing data on a predominant role of left anterior regions in decoding, analyzing and representing speech sounds.
Our conclusions on the differential distribution of voxel patches that accurately classify speech sound features gains plausibility by two side findings we have reported above. First, above-chance classification in both hemispheres (combined with a moderate leftward bias in overall accuracy, which is in essence present across the entire superior temporal cortex) is a likely outcome given the mixed evidence on left-dominant versus bilateral speech sound processing (see Hickok and Poeppel, 2007; Obleser and Eisner, 2009; Petkov et al., 2009 for reviews). Second, left primary auditory cortex and the region lateral to it (“mid”), which probably includes human belt and parabelt cortex (Wessinger et al., 2001; Humphries et al., 2010), showed a significantly better accuracy in classifying stop-consonants than vowels. This is in line with a wide body of research suggesting a left-hemispheric bias toward a better temporal analysis of the signal, which has been claimed to be especially relevant for the analysis of stop-consonant formant transitions (e.g., Schwartz and Tallal, 1980; Zatorre and Belin, 2001; Poeppel, 2003; Schönwiesner et al., 2005; Obleser et al., 2008).
Lastly, what can we infer from these data about the functional organization of speech sounds in the superior temporal cortex across participants? Microscopic (i.e., below-voxel-size) organization in the superior temporal areas is expected to be quite variable across participants. However, our data imply that there is enough spatial concordance within local topographical maps of acoustic–phonetic feature sensitivity to produce classification accuracies above chance. Also recall that we did not submit single voxels and single trial data to the classifier, but patches of neighboring voxels (which essentially allows for co-registered and normalized participant data to vary to some extent and still contribute to the same voxel patch) and statistical t-values (see Misaki et al., 2010), respectively. Therefore, the reported classification accuracies across participants form a lower bound of possible classification accuracy. What these data will not answer is the true “nature” or abstractness of features that aided successful classification in these various subareas of the auditory cortex. It is conceivable (and, in fact, highly likely) that different areas are coding different and differentially abstract features of the syllable material; which, again, would be testimony to the redundant or multi-level neural implementation of speech sound information.
Conclusion
In sum, the reported results show a widely distributed range of local cortical patches in the superior temporal cortex to encode critical information on vowel, consonant, as well as non-speech noise. Yet it assigns a specific role to left anterior superior temporal cortex in the processing of complex spectro-temporal patterns (Leaver and Rauschecker, 2010). In this respect, our results extend previous evidence from subtraction-based designs.
Univariate analyses of broad BOLD differences and multivariate analyses of local patterns of small-voxel activations are converging upon a robust speech versus noise distinction. The wide distribution of information on the vowel and stop category across regions of left and right superior temporal cortex accounts well for previous difficulties in pinpointing robust “phoneme areas” or “phonetic maps” in human auditory cortex. A closer inspection of average classification performance across select subregions of auditory cortex, however, singles out the left anterior region of the superior temporal cortex as containing the highest proportion of voxels bearing information for speech sound categorizations (Figure 6) and yielding the strongest lateralization toward the left (Figure 4). The consistent and non-overlapping classification into vowels and consonants in the current study was surprisingly robust and resonates with patient studies reporting selective deficits in processing of vowels and consonants (Caramazza et al., 2000). How exactly even finer phoneme categories or features (different types of vowels and consonants) are represented within these subregions escapes the current technology and may have to await future new approaches with even better resolution.
In sum, multivariate analysis of natural speech sounds opens a new level of sophistication in our conclusions on the topography of human speech sound processing in auditory cortical areas. First, it converges with subtraction-based analysis methods for broad, that is, acoustically very salient comparisons (as reported here for the speech versus noise comparisons). Second, it demonstrates that local activation patterns throughout auditory subregions in the superior temporal cortex contain robust (i.e., significant above-chance and sufficiently consistent across participants) encodings of different categories of speech sounds, with a special emphasis on the role of the left anterior STG/STS region. Third, it yields, across a wide area of superior temporal cortex, a surprisingly low overlap of those local patterns best for classification of vowel information and those best for stop-consonant classification. Given the knowledge about the hierarchical nature of non-primary auditory cortex derived from non-human primate studies, we can propose that complex sounds, including speech sounds as studied here, are represented in hierarchical networks distributed over a wide array of cortical areas. How these distributed “patches” communicate with each other to form a coherent percept will require further studies that can speak to dynamic functional connectivity.
Conflict of Interest Statement
The authors declare that the research was conducted in the absence of any commercial or financial relationships that could be construed as a potential conflict of interest.
Acknowledgments
This study was supported by research grants from the National Science Foundation (BCS 0519127 and OISE-0730255; Josef P. Rauschecker) and from the German Research Foundation (DFG, SFB 471; University of Konstanz), and by a post-doctoral grant from the Baden-Württemberg Foundation, Germany (Jonas Obleser). Jonas Obleser is currently based at the Max Planck Institute for Human Cognitive and Brain Sciences, Leipzig, and is funded through the Max Planck Society, Germany. The authors are grateful to Laura E. Girton (Georgetown), Carsten Eulitz and Aditi Lahiri (Konstanz), Björn Herrmann (Leipzig), and Angela D. Friederici (Leipzig) for their help and comments at various stages of this project. Elia Formisano and Lee Miller helped considerably improve this manuscript with their constructive suggestions.
References
Belin, P., Zatorre, R. J., Lafaille, P., Ahad, P., and Pike, B. (2000). Voice-selective areas in human auditory cortex. Nature 403, 309–312.
Benjamini, Y., and Yekutieli, D. (2005). False discovery rate – adjusted multiple confidence intervals for selected parameters. J. Am. Stat. Assoc. 100, 71–81.
Binder, J. R., Frost, J. A., Hammeke, T. A., Bellgowan, P. S., Springer, J. A., Kaufman, J. N., and Possing, E. T. (2000). Human temporal lobe activation by speech and nonspeech sounds. Cereb. Cortex 10, 512–528.
Binder, J. R., Liebenthal, E., Possing, E. T., Medler, D. A., and Ward, B. D. (2004). Neural correlates of sensory and decision processes in auditory object identification. Nat. Neurosci. 7, 295–301.
Blumstein, S. E., and Stevens, K. N. (1980). Perceptual invariance and onset spectra for stop consonants in different vowel environments. J. Acoust. Soc. Am. 67, 648–662.
Brennan, J., Nir, Y., Hasson, U., Malach, R., Heeger, D. J., and Pylkkanen, L. (2010). Syntactic structure building in the anterior temporal lobe during natural story listening. Brain Lang. doi:10.1016/j.bandl.2010.04.002. [Epub ahead of print].
Caramazza, A., Chialant, D., Capasso, R., and Miceli, G. (2000). Separable processing of consonants and vowels. Nature 403, 428–430.
Davis, M. H., and Johnsrude, I. S. (2003). Hierarchical processing in spoken language comprehension. J. Neurosci. 23, 3423–3431.
De Martino, F., Valente, G., Staeren, N., Ashburner, J., Goebel, R., and Formisano, E. (2008). Combining multivariate voxel selection and Support Vector Machines for mapping and classification of fMRI spatial patterns. Neuroimage 43, 44–58.
Engineer, C. T., Perez, C. A., Chen, Y. H., Carraway, R. S., Reed, A. C., Shetake, J. A., Jakkamsetti, V., Chang, K. Q., and Kilgard, M. P. (2008). Cortical activity patterns predict speech discrimination ability. Nat. Neurosci. 11, 603–608.
Formisano, E., De Martino, F., Bonte, M., and Goebel, R. (2008). “Who” is saying “what”? Brain-based decoding of human voice and speech. Science 322, 970–973.
Friederici, A. D., Meyer, M., and von Cramon, D. Y. (2000). Auditory language comprehension: an event-related fMRI study on the processing of syntactic and lexical information. Brain Lang. 74, 289–300.
Genovese, C. R., Lazar, N. A., and Nichols, T. (2002). Thresholding of statistical maps in functional neuroimaging using the false discovery rate. Neuroimage 15, 870–878.
Haxby, J. V., Gobbini, M. I., Furey, M. L., Ishai, A., Schouten, J. L., and Pietrini, P. (2001). Distributed and overlapping representations of faces and objects in ventral temporal cortex. Science 293, 2425–2430.
Haynes, J. D. (2009). Decoding visual consciousness from human brain signals. Trends Cogn. Sci. 13, 194–202.
Haynes, J. D., and Rees, G. (2005). Predicting the orientation of invisible stimuli from activity in human primary visual cortex. Nat. Neurosci. 8, 686–691.
Herrmann, B., Obleser, J., Kalberlah, C., Haynes, J. D., and Friederici, A. D. (in press). Dissociable neural imprints of perception and grammar in auditory functional imaging. Hum. Brain Mapp.
Hickok, G., and Poeppel, D. (2007). The cortical organization of speech processing. Nat. Rev. Neurosci. 8, 393–402.
Humphries, C., Liebenthal, E., and Binder, J. R. (2010). Tonotopic organization of human auditory cortex. Neuroimage 50, 1202–1211.
Kriegeskorte, N., and Bandettini, P. (2007). Combining the tools: activation-and information-based fMRI analysis. Neuroimage 38, 666–668.
Kriegeskorte, N., Goebel, R., and Bandettini, P. (2006). Information-based functional brain mapping. Proc. Natl. Acad. Sci. U.S.A. 103, 3863–3868.
Lahiri, A., Gewirth, L., and Blumstein, S. E. (1984). A reconsideration of acoustic invariance for place of articulation in diffuse stop consonants: evidence from a cross-language study. J. A. coust. Soc. Am. 76, 391–404.
Leaver, A. M., and Rauschecker, J. P. (2010). Cortical representation of natural complex sounds: effects of acoustic features and auditory object category. J. Neurosci. 30, 7604–7612.
Liebenthal, E., Binder, J. R., Spitzer, S. M., Possing, E. T., and Medler, D. A. (2005). Neural Substrates of Phonemic Perception. Cereb. Cortex 15, 1621–1631.
Misaki, M., Kim, Y., Bandettini, P. A., and Kriegeskorte, N. (2010). Comparison of multivariate classifiers and response normalizations for pattern-information fMRI. Neuroimage 53, 103–118.
Morosan, P., Rademacher, J., Schleicher, A., Amunts, K., Schormann, T., and Zilles, K. (2001). Human primary auditory cortex: cytoarchitectonic subdivisions and mapping into a spatial reference system. Neuroimage 13, 684–701.
Norman, K. A., Polyn, S. M., Detre, G. J., and Haxby, J. V. (2006). Beyond mind-reading: multi-voxel pattern analysis of fMRI data. Trends Cogn. Sci. 10, 424–430.
Obleser, J., Boecker, H., Drzezga, A., Haslinger, B., Hennenlotter, A., Roettinger, M., Eulitz, C., and Rauschecker, J. P. (2006). Vowel sound extraction in anterior superior temporal cortex. Hum. Brain Mapp. 27, 562–571.
Obleser, J., and Eisner, F. (2009). Pre-lexical abstraction of speech in the auditory cortex. Trends Cogn. Sci. 13, 14–19.
Obleser, J., Eisner, F., and Kotz, S. A. (2008). Bilateral speech comprehension reflects differential sensitivity to spectral and temporal features. J. Neurosci. 28, 8116–8123.
Obleser, J., Elbert, T., and Eulitz, C. (2004a). Attentional influences on functional mapping of speech sounds in human auditory cortex. BMC Neurosci. 5, 24. doi: 10.1186/1471-2202-5-24.
Obleser, J., Lahiri, A., and Eulitz, C. (2004b). Magnetic brain response mirrors extraction of phonological features from spoken vowels. J. Cogn. Neurosci. 16, 31–39.
Obleser, J., Lahiri, A., and Eulitz, C. (2003). Auditory-evoked magnetic field codes place of articulation in timing and topography around 100 milliseconds post syllable onset. Neuroimage 20, 1839–1847.
Okada, K., Rong, F., Venezia, J., Matchin, W., Hsieh, I. H., Saberi, K., Serences, J. T., and Hickok, G. (2010). Hierarchical organization of human auditory cortex: evidence from acoustic invariance in the response to intelligible speech. Cereb. Cortex. doi: 10.1093/cercor/bhp318. [Epub ahead of print].
Petkov, C. I., Kayser, C., Steudel, T., Whittingstall, K., Augath, M., and Logothetis, N. K. (2008). A voice region in the monkey brain. Nat. Neurosci. 11, 367–374.
Petkov, C. I., Logothetis, N. K., and Obleser, J. (2009). Where are the human speech and voice regions, and do other animals have anything like them? Neuroscientist 15, 419–429.
Poeppel, D. (2003). The analysis of speech in different temporal integration windows: cerebral lateralization as ‘asymmetric sampling in time’. Speech Commun. 41, 245–255.
Poremba, A., Malloy, M., Saunders, R. C., Carson, R. E., Herscovitch, P., and Mishkin, M. (2004). Species-specific calls evoke asymmetric activity in the monkey’s temporal poles. Nature 427, 448–451.
Rademacher, J., Morosan, P., Schormann, T., Schleicher, A., Werner, C., Freund, H. J., and Zilles, K. (2001). Probabilistic mapping and volume measurement of human primary auditory cortex. Neuroimage 13, 669–683.
Raizada, R. D., and Poldrack, R. A. (2007). Selective amplification of stimulus differences during categorical processing of speech. Neuron 56, 726–740.
Rauschecker, J. P., and Scott, S. K. (2009). Maps and streams in the auditory cortex: nonhuman primates illuminate human speech processing. Nat. Neurosci. 12, 718–724.
Read, H. L., Winer, J. A., and Schreiner, C. E. (2002). Functional architecture of auditory cortex. Curr. Opin. Neurobiol. 12, 433–440.
Rogalsky, C., and Hickok, G. (2009). Selective attention to semantic and syntactic features modulates sentence processing networks in anterior temporal cortex. Cereb. Cortex 19, 786–796.
Schönwiesner, M., Rubsamen, R., and von Cramon, D. Y. (2005). Hemispheric asymmetry for spectral and temporal processing in the human antero-lateral auditory belt cortex. Eur. J. Neurosci. 22, 1521–1528.
Schwartz, J., and Tallal, P. (1980). Rate of acoustic change may underlie hemispheric specialization for speech perception. Science 207, 1380–1381.
Scott, S. K., Blank, C. C., Rosen, S., and Wise, R. J. (2000). Identification of a pathway for intelligible speech in the left temporal lobe. Brain 123, 2400–2406.
Shestakova, A., Brattico, E., Soloviev, A., Klucharev, V., and Huotilainen, M. (2004). Orderly cortical representation of vowel categories presented by multiple exemplars. Brain Res. Cogn. Brain Res. 21, 342–350.
Slotnick, S. D., Moo, L. R., Segal, J. B., Hart, J., and Jr. (2003). Distinct prefrontal cortex activity associated with item memory and source memory for visual shapes. Brain Res. Cogn. Brain Res. 17, 75–82.
Steinschneider, M., Reser, D., Schroeder, C. E., and Arezzo, J. C. (1995). Tonotopic organization of responses reflecting stop consonant place of articulation in primary auditory cortex (A1) of the monkey. Brain Res. 674, 147–152.
Tian, B., Reser, D., Durham, A., Kustov, A., and Rauschecker, J. P. (2001). Functional specialization in rhesus monkey auditory cortex. Science 292, 290–293.
Wang, X. (2000). On cortical coding of vocal communication sounds in primates. Proc. Natl. Acad. Sci U.S.A. 97, 11843–11849.
Wang, X., Merzenich, M. M., Beitel, R., and Schreiner, C. E. (1995). Representation of a species-specific vocalization in the primary auditory cortex of the common marmoset: temporal and spectral characteristics. J. Neurophysiol. 74, 2685–2706.
Warren, J. D., Jennings, A. R., and Griffiths, T. D. (2005). Analysis of the spectral envelope of sounds by the human brain. Neuroimage 24, 1052–1057.
Wessinger, C. M., VanMeter, J., Tian, B., Van Lare, J., Pekar, J., and Rauschecker, J. P. (2001). Hierarchical organization of the human auditory cortex revealed by functional magnetic resonance imaging. J. Cogn. Neurosci. 13, 1–7.
Zatorre, R. J., and Belin, P. (2001). Spectral and temporal processing in human auditory cortex. Cereb. Cortex 11, 946–953.
Keywords: auditory cortex, speech, multivariate pattern classification, fMRI, syllables, vowels, consonants
Citation: Obleser J, Leaver AM, VanMeter J and Rauschecker JP (2010) Segregation of vowels and consonants in human auditory cortex: evidence for distributed hierarchical organization. Front. Psychology 1:232. doi:10.3389/fpsyg.2010.00232
Received: 13 August 2010;
Paper pending published: 30 October 2010;
Accepted: 08 December 2010;
Published online: 24 December 2010
Edited by:
Micah M. Murray, Université de Lausanne, SwitzerlandReviewed by:
Lee M. Miller, University of California Davis, USAElia Formisano, Maastricht University, Netherlands
Copyright: © 2010 Obleser, Leaver, VanMeter and Rauschecker. This is an open-access article subject to an exclusive license agreement between the authors and the Frontiers Research Foundation, which permits unrestricted use, distribution, and reproduction in any medium, provided the original authors and source are credited.
*Correspondence: Jonas Obleser, Max Planck Institute for Human Cognitive and Brain Sciences, Stephanstrasse 1a, 04103 Leipzig, Germany. e-mail:b2JsZXNlckBjYnMubXBnLmRl