- 1 Istituto di Scienze e Tecnologie della Cognizione, Consiglio Nazionale delle Ricerche, Roma, Italy
- 2 Istituto di Linguistica Computazionale “Antonio Zampolli”, Consiglio Nazionale delle Ricerche, Pisa, Italy
- 3 Department of Psychology, Emory University, Atlanta, GA, USA
- 4 School of Computing and Mathematics, University of Plymouth, Plymouth, UK
- 5 School of Psychology, University of Dundee, Dundee, Scotland, UK
- 6 Department of Psychology, University of Western Ontario, London, ON, Canada
- 7 School of Social Sciences, Humanities and Arts, University of California, Merced, CA, USA
Embodied theories are increasingly challenging traditional views of cognition by arguing that conceptual representations that constitute our knowledge are grounded in sensory and motor experiences, and processed at this sensorimotor level, rather than being represented and processed abstractly in an amodal conceptual system. Given the established empirical foundation, and the relatively underspecified theories to date, many researchers are extremely interested in embodied cognition but are clamoring for more mechanistic implementations. What is needed at this stage is a push toward explicit computational models that implement sensorimotor grounding as intrinsic to cognitive processes. In this article, six authors from varying backgrounds and approaches address issues concerning the construction of embodied computational models, and illustrate what they view as the critical current and next steps toward mechanistic theories of embodiment. The first part has the form of a dialog between two fictional characters: Ernest, the “experimenter,” and Mary, the “computational modeler.” The dialog consists of an interactive sequence of questions, requests for clarification, challenges, and (tentative) answers, and touches the most important aspects of grounded theories that should inform computational modeling and, conversely, the impact that computational modeling could have on embodied theories. The second part of the article discusses the most important open challenges for embodied computational modeling.
Introduction
Embodied cognition is a theoretical stance which postulates that sensory and motor experiences are part and parcel of the conceptual representations that constitute our knowledge. This view has challenged the longstanding assumption that our knowledge is represented abstractly in an amodal conceptual network of formal logical symbols. There now exist a large number of interesting and intriguing demonstrations of embodied cognition. Examples include changes in perceptual experience or motor behavior as a result of semantic processing (Boulenger et al., 2006; Meteyard et al., 2008), as well as changes in categorization that reflect sensory and motor experiences (Smith, 2005; Ross et al., 2007). These demonstrations have received a great deal of attention in the literature, and have spurred many researchers to take an embodied approach in their own work. There are also a number of theoretical accounts of how embodied cognition might work (Clark, 1998; Lakoff and Johnson, 1999). One influential proposal is “perceptual symbols system” theory (Barsalou, 1999), according to which the retrieval of conceptual meaning involves a partial re-enactment of experiences during concept acquisition. However, to a large extent, embodied theories of cognition are still developing, particularly in terms of their computational implementations, as well as their specification with regard to moment-by-moment online processing.
Given the established empirical foundation, and the relatively underspecified theories to date, many researchers are extremely interested in embodied cognition but are clamoring for more mechanistic implementations. What is needed at this stage is a push toward explicit computational models that implement sensorimotor grounding as intrinsic to cognitive processes. With such models, theoretical descriptions can be fleshed out as explicit mechanisms, idiosyncratic patterns across experiments may be explained, and quantitative predictions for new experiments can be put forward.
In this article, six authors from varying backgrounds and approaches address issues concerning the construction of embodied computational models, and illustrate what they view as the critical current and next steps toward mechanistic theories of embodiment. We propose the use of cognitive robotics to implement embodiment, and discuss the main prerequisites for a fruitful cross-fertilization between empirical and robotics research. Cognitive robotics is a broad research area, whose central aim is realizing complete robotic architectures that, on the one hand, include principles and constraints derived from animal and human cognition and, on the other hand, learn to operate autonomously in complex, open-ended scenarios (possibly interacting with humans) and have realistic embodiment, sensors, and effectors.
The relationship between theories of grounded cognition and cognitive robotics is twofold. On the one hand, theories and findings in research on grounded cognition imply that robot design should take into account the fact that a robot’s cognitive capacities should not be independent of its design and the modalities it uses for interacting with the external environment. This poses opportunities and challenges for robotics research (Pfeifer and Scheier, 1999). On the other hand, computational modeling in cognitive robotics can contribute to the development of better theories of embodied cognition by clarifying and testing some of its critical aspects, such as the extent to which embodied phenomena exert a causal influence on cognitive processing, thereby suggesting new avenues of research. Note that we are interested in computational models of embodied cognition in general, and not only for modeling human cognition, although we often use human cognitive abilities as examples in this article.
The article is structured as follows. We begin by clarifying the usage of some terms. The second section takes the form of a dialog between two fictional characters: Ernest, the “experimenter,” and Mary, the “computational modeler.” The dialog consists of an interactive sequence of questions, requests for clarification, challenges, and (tentative) answers. The dialog touches on the most important aspects of grounded theories that should inform computational modeling and, conversely, the impact that computational modeling could have on grounded theories. In the final section, we discuss the most important open challenges for embodied computational modeling, and suggest a roadmap for future research.
The use of terms such as “grounded” and “situated” is somewhat arbitrary, and these terms are used often interchangeably with “embodied.” Because of this issue in the current literature, we introduce some definitions at the outset of this article (cf. Myachykov et al., 2009; Fischer and Shaki, 2011). Together with these definitions, we also provide examples that specifically pertain to numerical cognition because this area of knowledge representation has traditionally been considered as a domain par excellence for abstract and amodal concepts, a view we wish to challenge.
Grounding
At the most general level, cognition has a physical foundation and it is, first and foremost, grounded in the physical properties of the world, such as the presence of gravity and celestial light sources, and constrained by physical principles (at least until we have evidence of life and cognition in a virtual reality). One example of grounding in the domain of numerical cognition is the fact that we associate smaller numbers with lower space and larger numbers with upper space (Ito and Hatta, 2004; Schwarz and Keus, 2004). This association is presumably universal because it reflects the physical necessity that the aggregation of more objects leads to larger piles. The recognition of the physical foundation of cognition has led researchers to challenge traditional theories of cognitive science and AI, in which cognitive operations were conceived as unconstrained manipulations of arbitrary and amodal symbols. The philosophical debate on how concepts and ideas have any meaning and are linked to their referents was revitalized by Searle’s (1980) Chinese room argument and by Harnad’s (1990) paper on symbol grounding, in which he argued that language-like symbols traditionally used in AI are meaningless because they lack grounding and reference to the external world. Harnad argued that the solution to this problem lies in the grounding of symbols in sensorimotor states; in this way, internal manipulations are constrained by the same laws that govern sensory and motor processes. Successively, grounded cognition has become the label for a methodological approach to the study of cognition that sees it as “grounded in multiple ways, including simulations, situated action, and, on occasion, bodily states” (Barsalou, 2008a, p. 619). As such, grounded cognition is different from, and wider than, embodied or situated cognition, because on occasion “cognition can indeed proceed independently of the specific body that encoded the sensorimotor experience (Barsalou, 2008a).” Rather, embodied and situated effects on representation and cognition can be conceptualized as a cascade.
Embodiment
On top of conceptual grounding, embodied representations are shaped by sensorimotor interactions, and consequently by the physical constraints of the individual’s body. Thus, embodiment is a consequence of the filtering properties of our sensory and motor systems, but this input is already structured and shaped in accord with physical principles, and these provide the grounding of cognition. For an example of embodiment in the domain of numerical cognition, consider the ubiquitous fact that small numbers are responded to faster with the left hand and larger numbers with the right hand – the spatial–numerical association of response codes (SNARC) effect. This effect is weaker in people who start counting on the fingers of their right hand compared to those who start counting on their left hand (Fischer, 2008; Lindemann et al., inpress), presumably because right-starters associate small numbers with their right side. This shows that the systematic use of one’s body influences the cognitive representation of numbers. Note that “embodied cognition” is often used metonymically so as to refer to “grounded cognition;” the former label is much more popular than the latter, and there is nothing wrong with its use providing that one keeps in mind that its literal meaning is restrictive.
Situatedness
Finally, situated cognition refers to the context dependence of cognitive processing and reflects the possibility that embodied signatures in our performance are context-specific and can be modified through experience. This can be a simple change of posture, as in the crossing of arms that reveals the dominance of allocentric over egocentric spatial coding in the Simon effect (Wallace, 1971). The SNARC effect offers two illustrations of this idea. First, a given number can be associated with either left or right space depending on the range of other numbers in the stimulus set (Dehaene et al., 1993; Fias et al., 1996). Second, turning one’s head alternatingly to the left and right while generating random numbers leads to a bias, such that left turns evoke more smaller numbers than do right turns (Loetscher et al., 2008). Both examples illustrate how the specific situation modulates the grounded and embodied representation of numbers (see also Fischer et al., 2009, 2010).
Although most contemporary theories of grounded cognition focus only on a subset of the phenomena that we have described here, future theories should tell a coherent story of how all of the relevant grounded, embodied, and situated phenomena constitute and constrain cognition.
Embodied Cognition and Computational Modeling: A Discussion
Topic 1. What Qualifies as an “Embodied” Computational Model and What are its Most Important Requirements?
Recent research has shown that grounded, embodied, and situated phenomena have a great impact on cognitive processing at all levels rather than being confined to the sensory and motor peripheries. In particular, beyond basic response production, intelligent action coupled with perception epitomizes embodied approaches. This poses significant challenges for computational models in all traditions (symbolic, connectionist, etc.). The first and foremost challenge is that cognition cannot be studied as a module independent from other modules (sensory and motor), as suggested by the “cognitive sandwich” metaphor. Instead, cognition is deeply interrelated with sensorimotor action and affect. Evidence indicates that even complex cognitive operations such as reasoning and language rely on and recruit perceptual and motor brain areas, and that imposing interference in these sensorimotor areas significantly impairs (or enhances) a person’s ability to execute cognitive tasks. Embodiment plausibly exerts its influence also by shaping development; thus, complex cognitive operations are learned based on simpler sensorimotor skills, which provide a ready neural and functional substrate. This implies that cognitive processes cannot be divorced from the sensorimotor processes that provided the scaffold for their development.
Consider a few examples of embodiment signatures in cognition. Spatial associations are frequently used to ground abstract conceptual knowledge, such as numerical magnitudes. This has been documented extensively in the SNARC effect (for a recent meta-analytic review, see Wood et al., 2008). Briefly, smaller magnitudes are associated with left space and larger magnitudes with right space, but this mapping is sensitive to contextual and cultural factors. More recently, the manipulation of magnitudes (arithmetic) has been shown to be mapped onto space, with addition inducing right biases and subtraction inducing left biases (Pinhas and Fischer, 2008; Knops et al., 2009). Another significant example of embodiment signatures in cognition is attention deployment, which plays a central role in forming concepts and directing reasoning within a grounded cognition framework (Grant and Spivey, 2003). In line with the embodied cognition approach, bodily constraints impose corresponding constraints on cognitive functioning and vice versa. Consider first how body postures affect attentional processing. With regard to one’s own postures, attention cannot be cued more laterally if the observer’s eyes are already at their biomechanical limit (Craighero et al., 2004). Similarly, pre-shaping one’s hand influences the selection of large or small objects in a visual search task (Symes et al., 2008), and planning to either point or grasp modulates the space- and object-based deployment of attention (Fischer and Hoellen, 2004) as well as the selection of object features (Bekkering and Neggers, 2002). With regard to perceiving other people’s postures, a large body of work on joint attention has discovered behavioral and neural evidence of rapid and automatic ability to process another person’s gaze direction (Frischen et al., 2007), head orientation (Hietanen, 2002), and hand aperture (Fischer et al., 2008) to deploy one’s own attention to a likely action goal. Body postures also affect one’s higher-level cognition. For example, adopting a particular posture will improve one’s recollection of events that involved similar postures, such as reclining on a chair and the associated experience of a dental visit (Dijkstra et al., 2008).
This body of work seems to confirm the tight coupling between sensory and motor maps on the one hand and conceptual processing on the other hand, as postulated by the embodied cognitive approach. It does, however, also raise an architectural challenge for computational modeling because it seems to require persistent cross-talk between domain-specific systems to account for the wide range of embodiment effects on performance. In terms of computational modeling, the main implication of this view is that the specific way in which robots act, perceive their external environment, and strive to survive and obtain reward, must have a significant impact on their cognitive representations and skills, and on how they develop. Indeed, this insight has close relations with a limitation that has been widely recognized in AI research, namely that cognitive processes were implemented by manipulating abstract symbols that were not “grounded” in the external world, and were unrelated to the robot’s action repertoire and perception (Harnad, 1990).
Research in grounded cognition makes an even stronger case for the influence of embodiment on cognition. Not only should representations be grounded, but their processing should essentially be fully embodied as well, such that there is no central processing independent of sensorimotor processes and/or affective experience. For instance, if we consider again the examples mentioned for spatial reasoning and attention, this leads to difficult issues for modelers, such as how spatial relations could be transferred to other domains (e.g., the temporal domain), or how spatial associations of abstract concepts could be simulated. The grounded perspective opens new avenues in robotics research, although a number of open research issues remain to be addressed.
One general set of issues relative to the realization of embodied cognitive models concerns the computational architecture, aside from whether it takes the form of neural networks, Bayesian approaches, production systems, classic AI architectures, or another form. Ernest, an experimenter, and Mary, a computational modeler, discuss these topics.
Requirement 1. Modal versus amodal representations
Mary: What are the most important constraints that grounded cognition pose for computational modeling and robotics? And, conversely, what are the essential features that computational models should have for them to be recognized as being “grounded cognitive models”?
Ernest: Perhaps the first and foremost attribute of a grounded computational model is the implementation of cognitive processes (e.g., memory, reasoning, and language understanding) as depending on modal representations and associated mechanisms for their processing [e.g., Barsalou’s (1999) simulators] rather than on amodal representations, transductions, and abstract rule systems.
Mary: This is a key departure point from most models in AI, which use amodal representations. However, don’t you think that modal representations, such as for instance visual representations, are too impoverished to support cognition? Take for example the visual representation of an apple. It could be sufficient to support decisions on how to grasp the apple, but maybe not for processing the word “apple” or for reasoning about apple market prices.
Ernest: Well, there is a big gap between the visual representation of an apple and the kind of reasoning you have in mind. However, note that embodied cognition does not claim that the brain is a recording system, or that objects are represented as “pictures” in the brain. Rather, the key idea is that the format of representations is still modal when they are manipulated in reasoning, memory, and linguistic tasks. For instance, it is now increasingly recognized that linguistic objects are stored as sensorimotor codes (Pulvermüller, 2005). Since language processing recruits the same circuits as action representation and planning (bidirectional) interference effects occur that have been observed experimentally. There is increasing evidence that the modal nature of representations creates “interferences” with memory and reasoning tasks as well (see Barsalou, 2008a for a review). Note also that to implement a truly embodied cognitive system, multiple modalities are essential. In addition to sensory and motor modalities, internal modalities, including affect, motivation, and reward, are essential from the embodied perspective.
Mary: Then, one can ask: what modalities are critical to include in a model of grounded cognition?
Ernest: Well, the answer depends, I suppose, on the empirical phenomena you want to model and on the specific embodiment of the robots you use. In general, however, it seems obvious that an embodied model of human cognition has to include the perceptual modalities, including, if possible, various aspects of vision, hearing, taste, smell, and touch, as all of them are relevant to human cognition. Note that the different modalities could be organized differently in the brain, and could contribute differently to action control. Excluding task-specific weighting of relevance, it seems important to implement visual dominance over the other modalities because it has frequently been demonstrated in sensory conflict paradigms, as in Calvert et al. (2004), for example. Embodied models of non-human cognition should also consider that non-human animals use multiple modalities as well.
Mary: Implementing multimodality in robots is not simple. There are nowadays many robotic platforms in the market; if we also consider that some of them can be customized, this offers (at least in principle) an ample choice of modalities to be included. However, simply including more modalities does not guarantee better performance, because an important issue concerns the associations among them. How should representations of different modalities be associated into patterns?
Ernest: In principle, this could be done directly, via connections from one modality to another, or indirectly, via association areas that function as hubs, linking modalities.
Mary: In computational terms, a simple, but certainly not unique, method for implementing direct connections from one modality to another is designing robot controllers composed of multiple, interlinked modal “maps” [e.g., Kohonen’s (2001) self-organizing maps], such as for instance motor maps, visual maps, and auditory maps, and see how they become related so as to support cognitive processing. For instance, a robot controller composed of multiple maps can learn coordinated motor programs such as looking at objects, pointing at objects, hearing their sound, etc., and the maps could develop strong associations between object-specific (motor, perceptual) features. Association areas can be designed as well within the same framework, by introducing maps that group the outputs of multiple maps. However, I don’t see any principled criterion for deciding what association areas should be included and how should they be organized.
Ernest: This problem is complicated because modalities seem to have a hierarchical rather than a flat structure. However, we still have an incomplete knowledge of the hierarchical structure of feature areas and association areas, and the connectivity patterns among them. Also important are the unique areas associated with bottom-up activation versus top-down simulation, along with shared areas. Regarding perception, most researchers believe that at least one association area or convergence zone is required for integrating information from different modalities (Damasio, 1989; Simmons and Barsalou, 2003). These ideas offer a starting point for computational modeling, which could be useful for answering many open questions. One question is whether a single area exists in which all types of information are integrated, as proposed by Patterson et al. (2007), or whether there are many, possibly hierarchically organized convergence zones. This question seems ripe for modeling because we have only begun to explore the consequences of various configurations in experimental work. For example, is more than one association area required to account for patterns of conceptual impairment?
Mary: I believe that the computational methodology could help in this regard by assessing the computational advantages that association areas and hierarchies provide, and assessing their costs (in computational terms). In addition, by using computational modeling it becomes possible to investigate the factors that regulate the patterns of connectivity among modalities. For instance, it has been proposed that “far-” senses, such as vision, are often predictive of “near-” senses, such as touch (Verschure and Coolen, 1991), and this would constrain associations and hierarchies. A third important issue concerns investigating the relations between sensory and motor codes. Indeed, in complete robot architectures, not only sensory information, but also motor information, in terms of both planning and execution of movements, would be required, and an important issue for computational modeling is how to integrate them.
Ernest: In cognitive neuroscience and psychology, there is a wide interest on how sensory and motor information is integrated in the brain. Traditional theories of planning tend to see sensory, cognitive, and motor codes as different; this implies a transduction from (modal) sensory to (amodal) cognitive codes, in which the latter guides cognitive processing and activates motor codes (Newell and Simon, 1972). By contrast, ideomotor theories of action provide support for the common coding of action and perception (Prinz, 1997; Hommel et al., 2001), which requires no transduction and could provide a better substrate for computational modeling of embodied phenomena. Similar ideas have rapidly gained importance in (social) cognitive neuroscience, due to the discovery of multimodal neurons, such as mirror neurons (Rizzolatti and Craighero, 2004). Many researchers believe that both perception and action rely on a principle of feature binding, whose anatomical and functional aspects are only partly understood. At the functional level, Körding and Wolpert (2004) proposed that the central nervous system combines, in a nearly optional fashion, multiple sources of information, such as visual, proprioceptive and predicted sensory states, to overcome sensory and motor noise. One advantage here is that neuroscience research is advancing rapidly and continues to provide useful information on how to represent and integrate these types of information.
Mary: This is indeed a very relevant point for computational modeling of embodiment. In traditional AI and vision research, internal representations are typically defined as functions of the input, and perceptual learning is formulated as the problem of extracting useful “features” from passively received perceptual (mainly visual) stimuli. Even in robotics, most studies (e.g., using reinforcement learning) use fixed sets of representations, which define for instance the current location and pose of the robot. Not only are these representations predefined (by the programmer), but also they are “generic,” or not specifically tied to the motor repertoire of the robot. Conversely, researchers in cognitive robotics increasingly recognize that perception and action form a continuum, and perceptual learning cannot disregard what is behaviorally relevant for the robot (see, e.g., Weiller et al., 2010); or, in other words, that representations should be shaped by the motor repertoire of the robot rather than being generic descriptions of the external world. This has led to a renaissance of the construct of object affordances (Gibson, 1979). Yet another formulation of the same idea is that learning is not a passive process, but is governed by the properties of the learning system. Because robots can actively control their inputs by means of their motor commands, their perceptual representations become dependent on motor skills and imbued with motor information as they explore their environment.
Ernest: Robotics seems to be a good starting place for investigating the mutual relations among the sensory modalities, and between sensory and motor modalities. Going even further in this direction, internal representations should be imbued with value representation, or information from the “internal” modalities, such as affect, interoception, motivation, and reward.
Mary: In robotic scenarios, adding internal motivations to robot architectures offers a natural way to link actions and value. The study of motivational systems is recently re-gaining importance in robotic settings (see, e.g., Fellous and Arbib, 2006).
Ernest: This stream of research could have additional advantages. Indeed, not only is value information essential for indicating significance to a robot’s actions, but current research in embodied cognition is revealing that affect, emotion, and the internal states that result from them could play a key role in shaping high-level cognition in a fully embodied system. One possibility is that understanding and producing abstract concepts, such as love or fear, depends on knowledge acquired from introspection (Craig, 2002; Barsalou and Wiemer-Hastings, 2005).
Requirement 2. From sensorimotor experience to cognitive skills: abstraction and abstract thought on the top of a modal system
Mary: This leads us to another important topic. Even if we understand how the modalities interact, from a computational viewpoint, we do not know how modal representations can support cognitive processing, nor the wide range of cognitive tasks embodied theories can potentially tackle. Is there any theory of how grounded representations could do that?
Ernest: One of the most “organic” proposals so far is Barsalou’s (1999) idea of simulation. A simulation is “the re-enactment of perceptual, motor, and introspective states acquired during experience with the world, body, and mind” (Barsalou, 2008a, pp. 618–619). The ability to map simulations onto sensorimotor states by using overlapping systems is essential, and permits implementing the top-down construal that characterizes all cognitive activity. A big challenge for computational modeling is realizing simulation mechanisms. This permits testing whether and how they can support cognitive operations ranging from memory tasks to categorization, action planning and symbolic operations, and producing both abstractions and exemplars, both of which are central to cognition.
Mary: Then, it seems to me that an embodied cognition picture of cognitive processing could be the following (see Figure 1). First, grounded models are formed based on situated interaction of the robot with its environment (including other robots or humans). These symbols are multimodal and link perceptual, motor and valence information related to the same learning episodes. Second, cognitive processing is performed through the re-enactment of grounded symbols: a process that is called “situated simulation.” During situated simulation, what becomes active anew includes not only the relevant episode-specific representations, but also the associated bodily resources and sensorimotor strategies, and so cognition operates under the same constraints and situatedness of action.
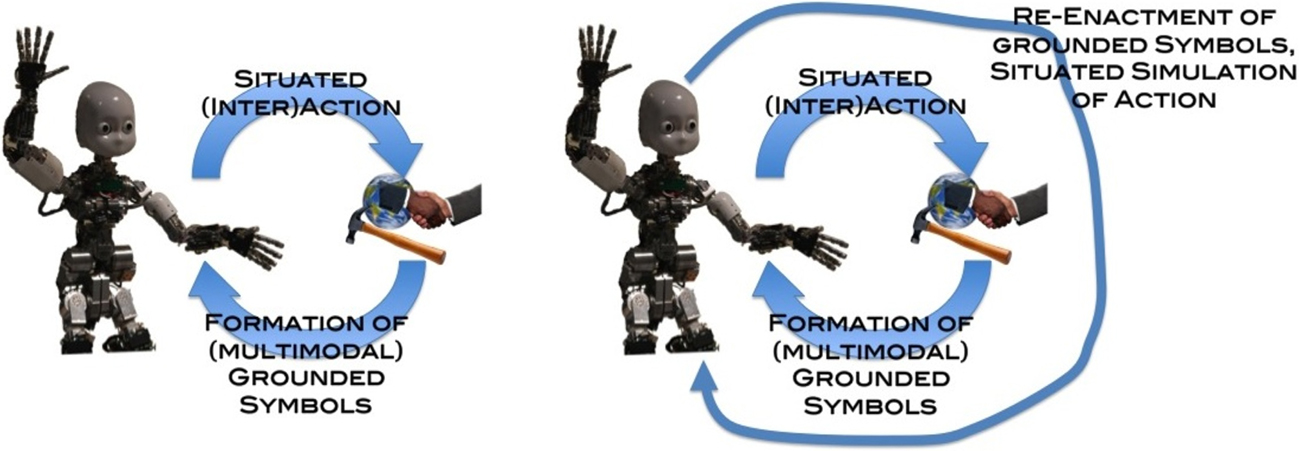
Figure 1. A grounded cognition perspective on how grounded (modal) symbols are firstly formed based on situated interactions with the external environment, and therefore re-enacted as situated simulations that afford higher-level cognitive processing (having the same characteristics and constraints as embodied and situated action).
Ernest: This seems to me quite an appropriate blueprint. How different is it from standard computational models?
Mary: Well, some existing systems, for instance connectionist architectures, already encode sensorimotor patterns in some form. What is more novel is how sensorimotor patterns are reused, and the idea that grounded symbols can be re-enacted so as to produce grounded cognitive processes. From a computational viewpoint, one interesting aspect of this theory is that a single mechanism, simulation, could underlie a wide range of cognitive phenomena. However, despite the potentialities of this idea, it raises many feasibility issues, such as how quick and accurate simulations should be to be really useful, how many computational resources are required to run simulations in real time, and how simulations of different aspects of the same situation can merge. Feasibility issues are of primary importance for computational modeling; if the idea of situated simulation successfully permeates cognitive robotics, a lot of effort will be required to bridge the gap between its current conceptualization and the full specification of efficient simulated mechanisms. In addition, we still have an incomplete picture of how simulation works. Even if we have a complete architecture provided with multiple modalities, it is still unclear what should be re-enacted that constitutes a simulation.
Ernest: As a first approximation, simulations could be automatic processes that simply re-enact the content of previously stored perceptual symbols, although there could be deliberate uses of simulations as well.
Mary: This simply shifts the problem from the re-enactment to the formation of simulators, and more in general to how the different modalities contribute to specific cognitive tasks. Take categorization as an example. It is difficult to see how individual concepts are extracted from rich multimodal experience, and which mechanisms are responsible for their formation. How should these mechanisms work in practice?
Ernest: Psychologists and neuroscientists have often focused on pattern association in associative areas, which could encode increasingly “abstract” concepts. Nevertheless, how (and which) patterns are associated and classified is only partly understood. One recent idea (Barsalou, 1999) is that categorical representations might emerge when attention is focused repeatedly on the same kind of thing in the world, by utilizing associative mechanisms among modalities, which, in turn, might permit re-enactment and simulation when needed. To the best of my knowledge, this mechanism has never been tested in computational models and would certainly be a valuable contribution to embodied cognition research because it would represent the development of alternative computational mechanisms.
Mary: This is a very good example of what grounded models can offer concerning longstanding questions, like the acquisition of abstract concepts and abstract thought. Also, your example highlights the “style” of embodied cognitive models compared to traditional computational modeling. What seems to me to be crucial here is that the acquisition of representations and skills is itself an embodied and situated process, is grounded in the sensorimotor abilities and bodily resources of the learner, and thus is modulated by the same environmental and cultural circumstances.
Ernest: You are right. Not only should grounded models refrain from using amodal symbols, but also from modeling the acquisition and use of concepts and reasoning skills as abstract processes, or processes that are not subject to the same constraints and laws that govern sensorimotor skills, as has been done in traditional computational modeling of psychological phenomena. This is not to say that all concepts originate in experience, given that there could be nativist contributions as well, only that the empirical contributions to concepts reflect the constraints of actual experience.
Mary: This is a good starting point for a research program in embodied computational modeling. However, computer scientists also have to deal with the soundness and feasibility of their approach; and unfortunately, from a computational viewpoint, the powers and limitations of simulations are still unclear. For instance, similar to traditional theories of conceptual representations, simulations could be too rigid to account for the variety of experience. If simulations and concepts collect (or perhaps average on) experience, how do they adapt to novel simulations and how do they get framed around background situations?
Ernest: One possible answer to this question is that simulations are not expected to replay all collected information; instead, they merge with perception to form completely situated experiences and can re-enact different content depending on current goals, sensorimotor, social, and affective states, all of which make (only) some content relevant. In a series of articles, Barsalou (1999, 2008a, 2009) presented various arguments and data indicating that simulators can be considered dynamical systems that produce simulations in a context-specific way that changes continually with experience.
Mary: A second possible limitation of simulations and re-enactment is that they seem to be prima facie related to the here-and-now. Therefore, it is difficult to see how they relate to something outside the present situation.
Ernest: According to Barsalou (1999), simulations re-enact perceptual experience, and the same neural codes implied in the initial experience with the actual objects. However, this does not preclude running simulations that represent future states of affairs. The re-enactment notion should not be confused with passive recollection of past states. Many recent studies have highlighted the importance of simulating future states of affairs to coordinate with the world as it will be, not simply with how it is now, and have argued that preparing for future action, not just recalling it, could be the main adaptive advantage of re-enactment, simulation, and memory systems (Glenberg, 1997; Schacter et al., 2007; Pezzulo, 2008; Bar, 2009; Barsalou, 2009). The richness and multimodality of simulations is useful to produce predictions across domains. A study by Altmann and Kamide (1999) shows that subjects started to look at edible objects more than inedible objects when listening to “the boy will eat” but not “the boy will move,” indicating that people can combine linguistic and non-linguistic cues to generate predictions. (Note that here the terminology is somewhat ambiguous because sometimes “simulation” is used as a synonym of re-enactment and sometimes as a synonym of long-term prediction.). Studies that involve imagination of future states of affairs also report that (visual and motor) simulations and imagery share neural circuits with actual perceptions and actions (Kosslyn, 1994; Jeannerod, 1995) and are subject to the same timing and general constraints. For instance, visual images and perception have the same metric spatial information and are subject to illusions in the same way. Performed and imagined actions respect Fitts’s law of motor control and its occasional violations (Eskenazi et al., 2009; Radulescu et al., 2010). It has been proposed that detachment from the here-and-now of experience, which, for example, is required for planning actions in the future, could be realized as a sophistication of the predictive and prospective abilities required in motor control and could recruit the same brain areas, rather than being processed in segregated brain areas with abstract representations (Pezzulo and Castelfranchi, 2007, 2009). This view is supported by the close relationship between the neural circuits that underlie motor imagery and motor preparation (Cisek and Kalaska, 2004).
Mary: Still, we have been talking about non-present circumstances related to the senses and the modalities (future or past states of affairs). It is even harder to imagine how simulations might relate to non-observable circumstances, such as, for example, “transcendental” concepts like space and time. How could space and time be implemented in a grounded system? And how would these implementations allow the system to run simulations of non-present experience with some fidelity to the representation of space and time in actual perception and action? A second issue concerns abstract concepts, including how they can support a full-fledged symbolic system.
Ernest: Well, these are all difficult questions, and I believe that cross-fertilization between empirical research and computational modeling could contribute to clarifying them. One tenet of grounded cognition is that all processes are situated and use modality-specific information rather than being processed in an abstract, amodal, logical space. This means that the representations of space and time in grounded systems, in all their manifestations, draw significantly on the processing of space and time in actual experience. Perception, cognition, and action must be coupled in space and time, and simulations of non-present situations must be implemented in space and time, perhaps using overlapping systems. Internal simulations do not escape this rule; so if abstract concepts and symbolic manipulations are grounded in introspective simulations, they should be sensitive to external space and time, too, and retain sensorimotor aspects. Although realizing how to implement a full-fledged symbolic system is a complex issue, some ideas useful for modelers have been presented. For example, Barsalou (2003) argued that selective attention and categorical memory integration are essential for creating a symbolic system. Once these functions are present, symbolic capabilities can be built upon them, including type-token propositions, predication, categorical inference, conceptual relations, argument binding, productivity, and conceptual combination.
Requirement 3. Realistic linkage of cognitive processes with the body, the sensory and motor surfaces, the environments in which cognition happens, and brain dynamics
Mary: These ideas at least provide some initial direction for creating novel grounded architectures and models. However, we have mostly discussed the modality of representations: do you think that there are additional factors that embodied models should include?
Ernest: According to grounded cognition theories, not only the modalities, but also sensorimotor skills and bodily resources influence cognitive processing, even in abstract domains. For instance, visuomotor strategies and eye movements are reused for abstract thinking; finger movements can be employed for counting; spatial navigation skills can be reused for reasoning in the temporal domain; motor planning processes can be re-enacted for imagining future events, understanding actions executed by others, or as an aid to memory. In these cases, expressions such as “taking a perspective on a problem” or “putting oneself into another’s shoes” or “grasping a concept” have to be taken more literally than normally assumed. Overall, due to their coherent learning processes and to re-enactment, grounded cognitive processes have the same powers, but also the same constraints, as bodily actions.
Mary: I wonder how your examples could be treated in a sound cognitive robotics design methodology. Ideally, rather than focusing on the abstract nature of cognitive problems, modelers should ask first what sensorimotor processes could support them in embodied agents. An emblematic example is temporal reasoning via spatial skills: a somewhat novel way to approach this topic could be learning spatial navigation first, and then reasoning in the temporal domain on top of the spatial representations, by re-enacting similar bodily processes. Clearly, this should be done within an embodied and situated research program rather than generically via computational modeling. I am increasingly more inclined to propose cognitive robotics as the primary methodology with which this and other grounded phenomena could be studied, as it emphasizes the importance of sensorimotor processes, situated action, and the role of the body. Still, it is unclear to me how realistic the bodies of robots should be. What kind of embodiment is necessary to study grounded phenomena? Is the specific embodiment of our models really important for embodied phenomena to happen? Can we study embodied cognition in agents that do not have their own “body” (as in general cognitive agents) or that are just computer simulations of robots’ bodies?
Ernest: Well, we know that most embodied effects are not only due to the way task-related information is represented, re-enacted, and processed, but are also due to the fact that the body is the medium of all cognitive operations, whether they are as simple as situated action or as complex as reasoning. Contrary to traditional cognitive theory, researchers in embodied cognition (e.g., Lakoff and Johnson, 1999) have argued that the body shapes cognition during development and continues to exert an influence at all stages of cognitive processing. Embodiment could have subtle and unexpected effects on cognitive processing. For instance, Glenberg and Kaschak (2002) showed that the action system influences sentence comprehension [the action–sentence compatibility (ACE) effect] and that subjects needed more time to understand sentences when the action required to signal comprehension was in the opposite direction than the target sentence (e.g., upward direction when the sentence referred to downward actions). From all these considerations, I would say that being realistic about embodiment is a must, at least in certain domains. Then, I see the point of your last question: a paradoxical consequence of taking embodiment claims literally is that cognitive robots could not be good models of human cognition because their bodies are too different from the body of humans, and have different computational, sensory, memory, and motor resources. What are the currently available platforms in cognitive robotics, and how well embodied are they?
Mary: Within embodiment research, cognitive models can be based on a variety of tools and platforms ranging from general cognitive agent systems (including multi-agent systems), to robot simulation models, up to physical robot platforms in cognitive robotics.
• Cognitive agents. Through these models we can typically simulate only selected features of the agent’s embodied system. For example an agent can have a retina-like visual system, and a motor control system to navigate the environment. This is the case for models of environment navigation as in foraging tasks. Moreover, these models are suitable for multi-agent simulation where we also investigate social and interaction aspects of cognitive processing. For example, Cangelosi (2001) implemented a multi-agent model of the evolution of communication. In it, a population of simulated abstract agents have to perform a foraging task. They can perceive the visual properties of objects (“mushrooms”) that determine their category of edible and inedible objects. Moreover, agents have a motor system to navigate the 2D world and approach/avoid foods. The perceptual and motor systems are implemented through a connectionist network, which also includes information relevant to the agent’s basic drives, such as hunger. Through this essential modeling of the agent’s sensorimotor system, it has been possible to investigate the symbol grounding problem in language learning and evolution (see also Cangelosi, 2010).
• Simulated robotic agents. These include realistic models of an existing robot, such as simulation models of the iCub humanoid platform (Tikhanoff et al., 2008), which is an open source robotic platform specifically developed for cognitive research, and of mobile robots such as khepera (Nolfi and Floreano, 2000). Moreover, it is possible to build physics-realistic models that do not correspond to living systems, such as in studies of the evolution of morphology (Pfeifer and Bongard, 2006). Typically these simulation models are based on physics engines that simulate the physics of object–object interaction dynamics with a high degree of fidelity. Despite the fact that the use of a simulation might not provide a full model of the complexity present in the real environment and might not assure fully reliable transferability of the controller from the simulation environment to the real one, robotic simulations are of great interest for cognitive scientists (Ziemke, 2003). For example, a simulator for the iCub robot (Metta et al., 2008; Tikhanoff et al., 2008) magnifies the value a research group can extract from the physical robot by making it more practical to share a single robot between several researchers. The fact that the simulator is free and open makes it a simple way for people interested in the robot to begin learning about its capabilities and design, with an easy “upgrade” path to the actual robot due to the protocol-level compatibility of the simulator and the physical robot. And for those without the means to purchase or build a humanoid robot, such as small laboratories or hobbyists, the simulator at least opens a door to participate in this area of research.
• Physical robot platforms in cognitive robotics. This is for embodied models of cognitive capabilities directly implemented and tested in the physical platform such as the iCub robot (Metta et al., 2008; Macura et al., 2009). Physical robot models are important when one wants to study the detailed physics of interaction dynamics of specific configurations of sensors and actuators. The main field of cognitive modeling relying on physical robot platforms is that of cognitive robotics (Metta and Cangelosi, in press). In particular, cognitive robotics regards the use of bio-inspired methods for the design of sensorimotor, cognitive, and social capabilities in autonomous robots. Robots are required to learn such capabilities (e.g., attention and perception, object manipulation, linguistic communication, social interaction), through interaction with their environment, and via incremental developmental stages. Cognitive robotics, especially approaches that focus on the modeling of developmental stages (aka developmental and epigenetic robotics), can be very beneficial in investigating the role of embodiment, from the early stages of cognitive development to well developed cognitive systems, and to study how bodies and cognitive abilities co-evolve and exert significant influences on one another.
The choice of the most suitable modeling approach from the three methodologies listed above depends on the specific aims of the research, the availability of resources, and the consideration of the technical issues specific to the chosen methodology. For example, the first two approaches based on cognitive agents and on simulated robot agents are useful when the details of the whole embodiment system are not crucial, but rather it is important to investigate the role of specific sensorimotor properties in cognitive modeling. Moreover, the practical and technical requirements of the first two methods are limited as they are mostly based on software simulations. Instead, the work with physical robot platforms, as in cognitive and developmental robotics, has the important advantage of considering the constraints of a whole, integrated embodiment architecture. In addition, robotics experiments can demonstrate that what has been observed in simulation models can actually be extrapolated to real robot platforms. This enhances the potential scientific and technological impact of the research, as well as further demonstrating the validity of cognitive theories and hypotheses.
Ernest: I see that there is a range of possibilities here. Do you think that, to study cognition, the bodies of robots should be the same, or very similar to, the bodies of humans or of other animals?
Mary: The kind of embodiment and the constraints that have to be taken into consideration depend on what you expect from embodied computational modeling. On the one hand, modeling can help to find novel ways of understanding phenomena that are potentially applicable to cognition in general, such as the idea that sensory processing, categorization, and action planning are interdependent rather than separate processing stages. On the other hand, if one aims to produce specific predictions about, say, humans, then she should aim at replicating the same bodies and the same constraints (e.g., environmental and social), or at least a useful approximation – which could be difficult to define a priori.
Ernest: One could argue that this is not the whole story, though, since there are additional constraints that could be potentially central to embodied cognitive modeling, such as brain dynamics and their peculiarities and limitations, which are could also be part of the robot embodiment in some sense.
Mary: I see your point here. Modeling in general is about finding useful abstractions, but it is difficult to define a priori which constraints should be included in embodied computational models, and which should not. There is a debate on this topic in the cognitive robotics community, with positions that range from defenders of biologically constrained methods to the less demanding artificial life approach. Although this is still an open point, it is necessary to recognize that, compared to traditional AI methodology that focuses on “abstract” or “general” intelligence or problem solvers, embodied cognitive modeling suffers more from the idiosyncrasies of what is meant to be modeled, be it a human or another living organism, because it takes a theoretical stance on the role of the “substratum” of cognition and the body.
Ernest: I see another problem of embodied computational models compared to traditional ones. Indeed, one important aspect of embodied models is that they should be coherent at the architectural level; or, in other terms, that their functions should not be developed by fully encapsulated models that work in isolation. This is especially true for the realization of higher-level cognitive abilities, such as reasoning, language, and categorical thinking, which cannot be totally disjoint from the neural systems that, say, direct eye movements and attention, regulate posture, or prepare actions to be executed.
Mary: This seems to rule out the hybrid approaches that are popular in robotics, in which complex cognitive skills are juxtaposed to basic sensorimotor skills, with a minimal (and predefined) interface. In addition, this poses a challenge to any kind of modular design in which functionalities are partly or completely encapsulated and do not interact, calling overall for a truly integrative theory. Understanding to what extent modelers can use modular design and what functionalities actually interact in any given cognitive process is both an important research aim and is crucial for the realization of working robotic systems. Indeed, to achieve the latter aim, it would be very difficult to simply connect all components, but rather the design of their coordination is crucial (Barsalou et al., 2007).
Interim conclusion. Novelty of grounded cognitive modeling and cognitive robotics
Ernest: We have discussed many important ingredients of embodied computational models, but I can easily imagine that some of them are already used in computational modeling and robotics. In your opinion, what are the most novel elements?
Mary: There are a few points that circulate to some extent in the literature, but to which embodied computational modeling should give extra emphasis: (1) Representations (grounded modal symbols) and cognitive abilities are not “given” but learned through sensorimotor interaction and on the top of sensorimotor skills and genetically specified abilities. Take as an example spatial abilities. A natural way to implement them using early connectionist (PDP) models is to encode spatial relations in the input nodes, and them let the agent learn navigation on top of them by capturing relevant statistics in the input. Rather, in this methodology even spatial relations should be autonomously acquired. (2) Higher-level cognitive abilities (in the individual and social domains) develop on top of the architecture for sensorimotor control. The re-enactment of modal representations rather than the re-coding in amodal format determines them, and they typically reuse existing sensorimotor competences in novel, more cognitive domains (e.g., visuomotor strategies for the temporal domain; counting with fingers) rather than using novel components. (3) Embodied cognitive modeling should go beyond isolated models, for example, attention models, memory models, and navigation models, to focus on complete architectures that develop their skills over time (see, e.g., Anderson, 2010). (4) Embodied cognitive modeling should emphasize the fact that robots and agents are naturally oriented to action. Other abilities (e.g., representation ability, memory ability, categorization ability, attention ability) could be in the service of action themselves, rather than having disconnected functions (e.g., vision as a re-coding of the external world). This latter point would have an impact on the traditional conceptualization of cognition as a stage in the perception–cognition–action pipeline.
Ernest: I see that all these are important points, and I am sure that future research will point out other relevant ingredients as well. Concerning the impact that your research could have, I expect that if a strong case can be made for the efficacy of embodied cognitive models, it would contribute to the success of grounded theories in general (as it was the case for the adoption of modal representations in cognitive science under the influence of early AI systems based on the manipulation of logic rules). So, my question is: what is the equivalent of the grounded perspective in computational modeling?
Mary: Unfortunately, cognitive modeling does not yet have standard, off-the-shelf solutions for implementing grounded phenomena, but there are several lines of research that could lead to convincing solutions. As a reaction to the conceptual and technical limitations of early AI symbolic systems, connectionism emphasized that cognition is based on distributed representations and processing (e.g., statistical processing) rather than on the manipulation of amodal symbols and abstract rules. Similarly, Bayesian systems showed the full effect of statistical manipulations on representations and structures (most of the time, however, this has been shown on predefined representations). Although connectionist and Bayesian systems might provide a good starting point for modeling grounded phenomena, they are not complete answers per se. In most connectionist and Bayesian architectures, processing occurs in modular systems separated from the modalities (similar conceptually to a transduction from modal to amodal representations), and the processing of cognitive tasks is specialized rather than shared with perception and action (similar conceptually to the manipulation of abstract rules, except that they are not explicitly represented but are implicitly encoded in the weights of the networks). This means that sensory and motor modalities do not affect cognition during processing, even though they can do so during development. This would be a weak demonstration of grounding and embodiment, showing that sensorimotor and bodily processes are affected by cognition, but not vice versa. Another extremely interesting research approach is dynamic systems, which emphasize situated action and the importance of a tight coupling with the external environment for the realization of all perceptual, action, and cognitive phenomena, as well as for their development (see, e.g., Thelen and Smith, 1994).
Within dynamic systems and dynamic fields thinking, cognition is mediated by the dynamics of sensorimotor coordination, and is sensitive to its parameters (e.g., activation level of dynamic fields, and their timing), rather than being separated from the sensory and motor surfaces. For this reason, dynamic systems could be an ideal starting point for modeling grounded cognition (Schöner, 2008). In addition, dynamic systems could potentially offer explanations that span multiple levels, including brain dynamics, sensorimotor interactions, and social interaction, all using the same language and theoretical constructs. However, the full potential of this approach has not been shown yet. First, we need increasingly more dynamic systems models of the higher-level cognitive phenomena that interest psychologists, and which provide novel accounts of existing data. An interesting example is Thelen et al.’s (2001) model, which offers a novel explanation of children’s behavior in the A-not-B paradigm. However, much remains to be done in this direction if dynamic systems want to become a paradigm for implementing complex operations on modal systems. Second, these systems should be increasingly embodied, instantiated for instance in robotic architectures, and tested in increasingly realistic situated (individual and social) scenarios, in order to tell a more complete story about the passage from realistic sensorimotor processing to realistic higher-level cognitive and social tasks. Third, dynamic systems tend to de-emphasize (or reformulate) internal representation and related notions, which are common currency in psychological and neuroscientific explanations, in favor of a novel ontology that includes conceptual terms such as “stability,” “synchronization,” “attractor,” and “bifurcation.” Besides the adequacy of these ideas, there is clearly a more sociological issue, and a new theoretical synthesis is required. It is logically possible that the new ontology replaces the old one (but then it is necessary that it provides higher explanatory power, and this is clearly acknowledged by psychologists and neuroscientists), that it re-explains the old one, offering novel and potentially more interesting theories of traditional concepts such as “representation,” or that the two ontologies can be harmonized to some extent, but clearly the foundational aspects of a “dynamicist” cognitive science should be clarified before it can really offer itself as a novel candidate paradigm (Spivey, 2007). Although I have emphasized dynamic systems research, different research traditions, including for instance Bayesian approaches and connectionist networks, offer a good starting point for developing embodied cognitive models as well, providing that they successfully face the same challenges that I outlined before. However, I believe that a necessary complement to all these methodologies is to increasingly adopt cognitive robotics as their experimental platform, rather than designing models of isolated phenomena, or relaxing too many constraints about sensorimotor processing and embodiment. Indeed, it seems to me that cognitive robotics offer a key advantage to the aforementioned methodologies, because it emphasizes almost all of the components of grounded models: the importance of embodiment, the loop among perceptual, motor and cognitive skills, and the mutual dependence of cognition and sensorimotor processes.
Topic 2. What can Embodied Cognition Learn From Computational Modeling and the Synthetic Methodology?
We have discussed what aspects of grounded cognition theories should inform computational modeling and the realization of robots informed by embodied cognitive abilities. Apart from the obvious scientific and technological achievements that these robots could provide, we have argued that computational models could help to answer open questions in the grounded cognition literature, and we have offered a few examples of this potential cross-fertilization. Here we focus on methodological issues: the role that computational modeling could play in developing grounded theories of cognition, and how it can complement theoretical and empirical research.
Ernest: It seems to me that computational modeling, as a methodology, is highly complementary to empirical research. It can help shed light on some aspects of grounded theories that are difficult to assess with empirical means only, and in doing so it can help to formulate better theories and specific predictions that can be tested empirically, and to even falsify current views (or at least lower our confidence level) by showing that they are computationally untenable. However, prima facie it is different to imagine how designing efficacious robots could be a convincing argument for psychologists and neuroscientists for or against a certain theory.
Mary: The primary role of embodied cognition models is not necessarily that of designing physical robots, such as the iCub, that are capable of reproducing human embodiment phenomena, although this is also a crucial benefit, as demonstrated by the advantages of biologically inspired systems. Rather, for cognitive scientists the robotic and computer simulation models are a way to verify and extend their hypotheses and theories. A simulation model can be viewed as the implementation of a theory in a computer or robot platform. A theory is a set of formal definitions and general assertions that describe and explain a class of phenomena. Examples of general cognitive theories are the ones on embodied cognition (as discussed in this article) but also other general, and hard to test theories such as in language evolution research that hypothesize a specific ability as the major factor explaining the origins of language (e.g., gestural communication for Armstrong et al., 1995; and tool making for Corballis, 2003). Theories expressed as simulations possess three characteristics that may be crucial for progress in the study of cognition (Cangelosi and Parisi, 2002). First, if one expresses one’s theory as a computer program, the theory cannot help but be explicit, detailed, consistent, and complete because, if it lacks these properties, the theory/program would not run in the computer and would not generate results. Second, a theory expressed as a computer program helps generate detailed predictions because, as we have said, when the program runs in the computer, the simulation results are the predictions (even predictions not thought of by the researcher) for human behavior derived from the theory. And finally, computer simulations are not only theories but also virtual experimental laboratories. As in a real experimental laboratory, a simulation, once constructed, allows the researcher to observe phenomena under controlled conditions, to manipulate the conditions and variables that control the phenomena, and to determine the consequences of these manipulations. Indeed, this is one of the main advantages of computational modeling over other techniques. Computer simulations answer questions that cannot be addressed directly by empirical research; for instance, because the addressed phenomena cannot be observed directly or replicated (e.g., evolutionary phenomena) or are simply too difficult, risky, unethical, or expensive to test in the real world (e.g., the consequences of different learning episodes or model architectures for development). In addition, certain empirical phenomena depend on systemic and computational constraints on the behaving (or cognitive) system, irrespective of whether these constraints are posed by the system itself (e.g., bounded resources) or by external factors (e.g., situatedness). For instance, in a computational study reviewed below, Pezzulo and Calvi (in press) investigated what simulators could emerge in a perceptual symbol system due to limited resources and other computational constraints.
Ernest: This is indeed interesting, and I see how computational modeling could contribute to the development and refinement of embodied theories of cognition. For instance, one issue that is widely debated is how to interpret the activation of the motor system during “cognitive” tasks, such as language understanding; or, in other terms, assessing if embodied phenomena are causal or epiphenomenal. So far, the most common methodology consists of measuring the time course of activation of the brain areas; for instance, of motor areas during language perception (Pulvermüller, 2005). In brief, early activations are more compatible with the view that embodied cognition plays a causal role. A more direct approach to the understanding of causality consists of interfering with the cognitive process, such as in TMS studies, but also with behavioral paradigms that create interference between tasks (e.g., a motor and a higher-level cognitive task).
Mary: Computational modeling can in principle help to resolve the aforementioned debate by providing principled ways to assess causality in cognitive processes, or at least provide a “sufficiency proof” that certain cognitive tasks, whose functioning is still unclear, can be explained on the basis of embodied phenomena. For instance, it is possible to compare how competing (epiphenomenal versus causal) computational models explain motor involvement during perception of language (Pulvermüller, 2005; Garagnani et al., 2007) or affordances (Tucker and Ellis, 2001, see later). In “epiphenomenal” models, representations (e.g., linguistic representations) are amodal and their processing is modular (i.e., separated from the sensory and action cortices), and when the “central” processing affects the “peripheries,” this is an epiphenomenon without causal influence. On the contrary, in “causal” models all processing involves simulations and manipulation of modal representations. Implementing causal and epiphenomenal theories in computational terms, and embodying them into robot or agent architectures, can help to disambiguate their explanatory power, and to compare their empirical predictions.
Ernest: Another important issue for which computational models can provide insight is the apparently contradictory evidence on facilitatory or inhibitory roles of embodied processes. For instance, observation of actions performed by others can either facilitate or inhibit one’s own motor actions, depending not only on the degree of convergence between the observed and executed actions, but also on the time course of the interference, and in some cases on the localization of the processing in the brain. The conflicting facilitatory versus inhibitory effects in the literature could, for instance, reflect the hierarchical nature of perceptual and motor systems, with different kinds of effects reflecting different levels in these systems, or alternatively, they could depend on the time course of the interference. What is lacking is a specific model of when and how various processes produce facilitation or inhibition, which could serve to test different hypotheses.
Mary: Relative to this issue, computational modeling can implement competing theories that aim at explaining interference, such as theories in which timing or competition for shared resources is viewed as the key element for modulating the interaction. Note that in different tasks and domains, the mechanisms and the effect could be different, so computational models should be endowed with precise details and contextual constraints. At the same time, it is desirable that common principles emerge, and thus the promise of computational modeling lies in providing a comprehensive framework for explaining interference within cognitive processing and reconciling the puzzling findings.
Ernest: You have argued convincingly that cognitive robotics could be a good starting point for modeling embodied phenomena, but I see a potential drawback in its method: when modeling cognitive functions, we should not forget that the way they are realized depends on the way they develop. Indeed, issues associated with the architecture’s development and plasticity are important, too, including morphological, genetic, experiential, and social contributions, and how epigenesis is realized (Elman et al., 1996). For instance, as sensorimotor skills mature over time, cognitive abilities also develop in coordination with the acquisition of action skills (Rosenbaum et al., 2001). Also, social factors contribute substantially to development. Unfortunately, these dynamics are quite problematic to study empirically (but see Thelen and Smith, 1994). Again, however, here computational modeling can really help, addressing, for instance, the following questions: To what extent is a developmental trajectory necessary to “build” a grounded system? If it is necessary, what sorts of learning regimens are critical, and why?
Mary: This also seems like a nice place for modeling, at least in terms of the development or learning of knowledge in some specific domains. So, one could investigate whether you need to begin with some number of association areas already in place, for example. Or possibly this might be an interesting situation for using constructive algorithms like cascade correlation (Fahlman and Lebiere, 1990) or evolutionary techniques (Nolfi and Floreano, 2000) to see how “hidden units,” or convergence zones, develop with experience. Overall, I would definitely agree that the dynamics of situated experience play an essential role in the shaping of cognitive abilities, and this is why I see developmental and epigenetic robotics approaches as extremely promising approaches for the construction of grounded systems (Weng et al., 2001).
Ernest: Overall, from this discussion I see significant potential for collaboration and cross-fertilization between the theoretical, empirical, and synthetic methodologies. However, I have recently participated in many good conferences, such as SAB, EpiRob, and ALife, in which I have seen many computational systems at work. Although most scientists in these conferences have similar motivations as you, that is, designing computational models that can tell us something about cognition (and in particular higher-level cognition), and using similar methods as you describe, including cognitive robotics, I am still unsure of what the results are. I don’t deny that most of the things that I have seen are inspiring; still I fail to see how they can really influence my work. My guess is that most current computational models are mere “proofs of concept” and lack the adequate level of detail to start deriving precise predictions, or to simply be considered as useful tools by psychologists and neuroscientists. To realize the full impact of cognitive modeling on current (and future) theories, one not only has to develop computational systems that are generically informed by embodied cognition principles, but systems that target specific functionalities and experimental data.
Mary: This is indeed a necessary step. It is worth noting that although we still need a solid methodology for comparing empirical and synthetic data, various approaches have been proposed that compare modeling and empirical data both qualitatively and quantitatively. For example, within the literature on computational and robotic models of embodiment, there are studies where reaction times collected in psychology experiments have been directly compared with other time-related measurements in computational agents. Caligiore et al. (2010) directly compare the reaction times of Tucker and Ellis’s (2001) stimulus response compatibility experiments with the time steps required by the simulated iCub robot’s neural controller to reach a threshold that initiates the motor response. In Macura et al. (2009), a more indirect comparison for reaction time is used, based on the neural controller’s error measurements in the production of the response. In the context of neuroscience, specific quantitative methods have been developed to compare brain-imaging data from human participants (e.g., from fMRI and PET methodologies), with “synthetic brain-imaging” data from computational models ranging from computational neuroscience models (Horwitz and Tagamets, 1999; Arbib et al., 2000) to connectionist models (Cangelosi and Parisi, 2004). The direct comparison between empirical and modeling data remains a major challenge for multi-agent models of cognition. This is the case for evolutionary models (e.g., language evolution models, Cangelosi and Parisi, 2002) where only general qualitative comparisons are possible due to the lack of data, or due to less realistic implementation of the sensorimotor and behavioral systems (e.g., multi-agent models of foraging). There are, however, other important reasons why our ideas fail to permeate cognitive science as a whole. On the one hand, there is a clear “sociological” problem of different languages and different conferences. On the other hand, there are more serious methodological differences that have to be bridged to some extent. It is often the case that modelers and empiricists have different research questions in mind, or use different lexicons. In addition, modelers in the communities that you mentioned tend to emphasize complete architectures and the fact that many processes interact, whereas empirical research often adopts a divide-and-conquer strategy and tends to study brain and behavior as if there were specialized processes, such as memory, attention, and language, with specialized neural representations.
Ernest: I would agree that the methodological differences make collaboration harder, and then that “empiricists” have to change as much as “modelers.” The last point you mentioned is the most important one to me. Although you would rarely meet a cognitive scientist who claims to be a modularist, still some modularism (and localizationism) leaks into experimental paradigms in practice. Indeed, there is a tendency to study cognitive processes in isolation, as if they had separable neural substrates and encapsulated representations, a clear “objective” target that can be readily disconnected from the organism’s behavior and goals (e.g., memory is for storage and retrieval the maximum number of elements, attention is for selecting stimuli), and as if they had specialized resources, inputs, and outputs that are clearly separable from those implied in other processes that take place at the same time. Overall, an added value of collaboration with embodied computational modelers could be a push toward integrated theories of cognition rather than theories of isolated functions.
Topic 3. Current Embodied Computational Models: Successes and Limits
As we have briefly mentioned, there have been many attempts to model embodied and situated phenomena, especially in the connectionist and dynamic systems traditions. In these areas, it is widely recognized that the body, environment, and internal neural dynamics of agents are highly interconnected and shape one another (Clark, 1998; Pfeifer and Scheier, 1999). However, although these models fit the general framework of grounded cognition generically, most of them do not incorporate its specific predictions. In addition, only a few of them explicitly address complex (or even moderately complex) cognitive abilities, which are a true “benchmark” for grounded theories.
This section discusses a few robotic and agentive systems that exemplify current efforts toward the realization of embodied models of higher-level cognitive abilities, and specifically concepts and language understanding. This short review, which is undoubtedly biased by the authors’ knowledge, is by no means representative of the most successful systems in technological terms, but is intended to illustrate current directions toward embodied cognitive models, together with their powers and limitations.
Ernest: Two intertwined areas in which embodied cognition currently is central are concepts and the expression and comprehension of those concepts via language. For example, it seems pretty clear at the moment that multiple perceptual modalities are intrinsic to object concepts. Lots of experiments and imaging studies suggest this. One clear demonstration of embodied cognition for the link between visual and motor processes is the stimulus response compatibility effect studied by Ellis and colleagues (Tucker and Ellis, 2001; Ellis et al., 2007). They have consistently demonstrated that when we perform visual categorization tasks (e.g., identifying artifact versus natural objects), the micro-affordances linked to the objects (e.g., power grasp for a large apple or precision grip for a small cherry) affect the visual categorization task.
Mary: These embodied phenomena have been recently implemented in iCub. Macura et al.’s (2009) model also implements the stimulus compatibility effects demonstrated by Tucker and Ellis (2001) in a simulation model of iCub (Tikhanoff et al., 2008). The experiments focus on training the robot to grasp objects using different responses, such as precision versus power grips for small and large objects, respectively. They also replicate the psychological experiments in which the objects can be categorized using different grips (e.g., precision grip for artifacts and power grip for natural objects). Specifically, in the simulation experiments there are four objects: two larger objects (“big-ball” and “big-cube”) for power grips; and two smaller objects (“small-ball” and “small-cube”) for precision grips. In this simulation, the round objects (big and small balls) are viewed as natural objects, whereas the cubes are viewed as artifacts. The training data consist of a set of grasping sequences for each object. A connectionist network is used to learn and guide the robot’s behavior and to acquire embodied representations of objects and actions. The neural architecture, based on the Jordan recurrent architecture, has recurrent connections to permit information integration and the execution of actions such as grasping. The robots successfully learn to handle and categorize the objects as per the two tasks.
One important test of this model of object grasping and micro-affordances is the comparison of the congruent condition (where the categorization grip is in agreement with the natural grip) and the incongruent ones (where there is mismatch between the categorization grip and the natural grip). The trained neural networks were presented each object in turn, where the desired target depended on the task being performed. The network test error was used as an equivalent of the participant’s reaction time performance. Test results are highly consistent with psychological experiments where categorization latencies are shorter in congruent than in incongruent trials. In addition, the reaction times for larger objects were faster than for smaller objects, as was also the case in psychological experiments. This indicates that the robot was able to generalize a grasping sequence for each task and object from the four grasping sequences used in training, hence learning to appropriately grasp and categorize objects based on their shapes and sizes.
This computational model of Tucker and Ellis’s (2001) compatibility effect demonstrates that it is possible to build robots capable of performing object manipulation tasks using the same constraints and mechanisms observed in human embodied cognition. Moreover, related models of microaffordance effects have been developed using a neurally plausible organization of the robot’s neural architecture (Caligiore et al., 2010), with an extension of this model to simulate other compatibility effects, such as those studied by Borghi et al. (2004), which are also language-related.
Ernest: This is indeed very interesting, and I am curious to see how these studies evolve toward a comprehensive design methodology. However, most scientists, even those not convinced by embodied theories might admit that certain concepts, and especially concepts for manipulable objects, are partially represented in motor terms, and might recruit the motor system (even if they would not admit that motor processes are necessary for their understanding). What is definitely less clear is how you might model abstract concepts, such as objects that have no clear reference to observable or manipulable entities, or concepts that seem to be essentially “linguistic.” How might we do this?
Mary: Currently, there are few embodied models of cognition that address the issue of how to develop concepts that depart from the most immediate sensations and actions, and grounded processes for their manipulation. One important line of research touches on the issue of how language and the conceptual system interact. Cangelosi and Riga (2006) present a simulated robotic agent model of the combination of sensorimotor categories, paired with language learning, to autonomously generate new action concepts. This is achieved through a connectionist implementation of the mental simulations in Barsalou’s perceptual symbol system. The model is based on two humanoid robotic agents: the demonstrator and the imitator. The demonstrator (teacher) shows the correct performance of basic motor primitives (e.g., close the left arm, go forward, etc.) and also names the actions being demonstrated. The researcher programs this robot to perform these basic actions. The second agent, the imitator (learner), learns the actions by imitating the demonstrator’s behavior. This agent is equipped with an artificial neural network that can learn to perform the basic actions by predicting the demonstrator’s movement trajectories. The robot’s neural controller also learns the words associated with the actions, so that when the imitator “hears” a word, it can perform the corresponding action. This training phase based on the visual demonstration and simultaneous naming of actions is called the “direct grounding” phase and resembles the way in which children acquire new concepts while an adult comments on their actions (Pulvermüller, 2005). Subsequently the demonstrator teaches new composite, higher-order actions solely through language instructions. The demonstrator utters sentences such as “grab is close_left_arm and close_right_arm” (no visual demonstration of the grab action is given). The learner’s neural network uses a new learning algorithm that allows it to transfer the sensorimotor grounding of the basic words (“close_left_arm”) to the new linguistic concept of “grab.” This is achieved by first (internally) simulating the individual actions and by later reusing its own predicted output motor states as teaching inputs for the new linguistic concepts. This is an operational neural network implementation of a mental simulation mechanism in perceptual symbol system theory. Through this internal (mental) simulation, the imitator agent learns to perform and demonstrate the higher-order motor concept of grabbing. This training phased is called “grounding transfer.” This model is an example of a higher-level (i.e., language-related) cognitive model of embodiment theory. A related robotic model, implemented by Madden et al. (2009), uses situated simulation as a “middle layer” for connecting propositional representations of sentences and the robot’s sensorimotor experience. This system permits the temporal unfolding of propositions under the guidance of situated simulations and, at the same time, successfully demonstrates grammatical control of aspects of the simulation, beginning to tackle the broad issue of language comprehension and its neural bases. This approach could shed light on the relations between simulations and language, and how the linguistic system can be used to control simulations.
Other robotics models of language learning, which have a direct impact on the embodied literature, are Steels and Kaplan’s (2000, 2002) models of the cultural evolution of language. In these computational studies, the focus has been on the social interaction between robotics agents that leads to the self-organization of shared lexicons.
Another relevant line of research touches on the issue of how concepts can be grounded in anticipated action or interaction with objects. For example, Moller and Schenck (2008) have studied how navigation-related concepts such as “far” or “closed path” could derive from the internal simulation of robot navigation. Interestingly, these concepts are grounded in the robot’s anticipated perception, but go far beyond mere perception and include action possibilities, suggesting a route toward the development of more abstract knowledge. In a similar vein, Roy (2005a) has proposed a schema-based robot architecture in which the meaning of words and sentences in natural language are grounded in expectations relative to the robot’s sensorimotor flow. For example, simple words such as “red” that refer to perceptual features have their grounding in expected sensory information in the robot’s sensors. Concepts and words that refer to reachable and graspable objects are grounded in perceptual and motor schemas and in the expectations they produce during action planning and performance. For instance, the meaning of “sponge” is a set of expected action outcomes (e.g., an anticipated softness).
Finally, the emergence of grounded categories for motivation-related concepts such as “prey” and “predator” has been studied by adopting a simulated robotic agent methodology (Pezzulo and Calvi, in press). The computational architecture first learns multiple perceptual symbols in the form of action schemas that couple perception of the entities’ features and action patterns that are more useful in the presence of the entities, such as escape or approach. Successively, as the agent interacts with the entities in its environment and learns to adaptively find food and escape from predators, associations are created within the perceptual symbols and between the perceptual symbols and the agent’s internal motivational states, namely hunger and fear. In this way, entire simulators develop that cluster (or categorize) external entities and events in terms of the integration of possible associated perceptual events, actions, and motivational value for the agent, and which form reusable “situated conceptualizations.” Pezzulo and Calvi (in press) observed that many simulators emerge and become encoded within the architecture’s associative links. On the one hand, simulators cluster entities that have similar perceptual appearance or behavior; on the other hand, two more simulators emerge that correspond to the two families of “preys” and “predators,” and determine highly coherent motivational dynamics (related to hunger and fear, respectively). Importantly, the simulators are not (only) memory structures, but support simulations and the dynamic reactivation of perceptual symbols (Barsalou, 1999). Simulators, with all their associated perceptual symbols, are acquired (partially or in their entirety) even from partial observations of salient events, or from changes in the agent’s motivational state. In other words, when formed, simulators become tuned to types and not only tokens (and the exemplars which they were originally developed), simultaneously providing abstraction abilities and graded effects. This occurs because, depending on the circumstances, perceptual symbols in the simulators can be re-enacted to different degrees, or for different periods of time. For this reason, they can be considered categories that are grounded in the agent’s sensorimotor and motivational repertoire. In addition, the study shows that the development of simulators produces an increase in the agent’s adaptivity and performance rate.
All these are examples of recent efforts in the modeling of embodied phenomena. However, many of them can be considered preliminary investigations that do not derive precise predictions, but instead explore possible, novel ways to conceptualize and model cognitive phenomena, which are broadly inspired by embodied cognition research, but have not yet reached the level of detail that is required for a fruitful dialog with the empirical sciences. In addition, up to now most cognitive abilities, including for instance reasoning and memory abilities, and the realization of a full-fledged symbolic system, have not been addressed. In the rest of the article we discuss these and other related challenges for embodied computational modeling, and suggest promising directions of research.
Embodied Computational Modeling: Challenges for Future Research
We have argued that embodied cognitive theories and computational modeling are complementary, and we have briefly described a few implemented systems that begin to show embodied cognitive abilities. In the remainder of the article, we discuss the most important challenges for future research in embodied computational modeling, and offer a few views on how to tackle them.
Challenge 1. Taking a Developmental Viewpoint to Explore Why and How Embodied Cognition Could have Originated
From an engineering standpoint, the smart way to build an intelligent organism would be to use a modular linear-systems approach to learn sensorimotor regularities to the greatest possible extent, and then to perform exhaustive computations on that input until a single optimal motor action could be selected and executed. The “motor babbling” of infants could be seen as supporting such a mechanism. Under such a rubric, one should restrict the motor system to generating and executing movement plans only for actions that have been confidently chosen as the appropriate effector output given the array of sensor inputs. In such a scenario, the motor-movement module is essentially a patient tele-operations system enslaved to the finalized commands of the cognition module, an approach that dominates the cognitive study of human motor control today.
However, the primate nervous system was not engineered by designers with such a linear-systems bias. Instead it evolved over millions of years, from quite different ancestors, through varying environmental niches, with substantial non-linear co-evolution among its many subsystems. The result is that the functional neuroanatomy of the human brain shares none of the feed-forward reasoning that makes a computer circuit-board understandable to an engineer. The human cerebral cortex is rife with top-down feedback projections and lateral connections that quickly scuttle a purely linear-systems analysis (see Carandini et al., 2005, for review). For example the orbito-frontal cortex, which is one of many sensory-integration regions in frontal cortex, has a direct functional projection back to visual cortex (Kveraga et al., 2007). This may allow expectations from multiple sensory sources to prepare visual cortex to process its afferent input in a richly contextualized manner (Mumford, 1992; Rao and Ballard, 1999).
Not only does feedback cause problems for a modular account of cognition, but the continuous flow of information among brain areas tends to blur the boundary that one might wish to daw between cognition and action. In other words, embodied cognition may be unavoidable given the dynamics of the brain, which was not designed as a whole, but underwent a complex evolutionary history in which successive adaptive solutions (to the same evolutionary problems) were implemented by adding on to earlier ones.
Evidence that the motor system is not in fact a “patient and obedient slave” that blindly follows the cognition system’s finalized commands comes from a variety of studies that measure continuous motor output and multi-cell recording in premotor cortex. For example, Cisek and Kalaska (2005) report partial activation of two non-overlapping population codes in premotor cortex when the monkey is considering two possible reaching destinations. When Gold and Shadlen (2000) presented motion stimuli to a monkey and induced an eye movement via micro-stimulation of the frontal eye fields, the response-based eye movement (with which the monkey indicated the direction of perceived motion) and the micro-stimulated eye movement tended to average together into a single saccade that (with varying stimulus exposure times) revealed the gradual accrual of sensory information apparent in neural activity in the frontal eye fields. That is, neurons in the frontal eye fields (an oculomotor region in frontal cortex) were accumulating partial information about the perceptual process before it had been allowed to reach completion. Similarly, eye movements in humans often “jump the gun” and fixate objects that correspond to partially active representations that in the end play no role in the person’s planned action (Tanenhaus et al., 1995; Allopenna et al., 1998). Likewise, reaching movements will systematically curve toward multiple potential targets during the course of the reach (Tipper et al., 1997; Spivey et al., 2005; Song and Nakayama, 2009). Even repetitive bimanual rhythmic coordination can show effects, in its relative phase dynamics, of changes in a cognitive process “leaking” into the motor system (Shockley and Turvey, 2005). Furthermore, when two people are conversing, their motor systems can become entrained with one another, such that their postural sway becomes coordinated (Shockley et al., 2003), and their eye movements become coordinated (Richardson and Dale, 2005). These rich interactions among multiple sensory and motor subsystems in the brain are especially robust in children (Karmiloff-Smith, 1992), and may even lead to a child’s early formation of concepts being undergirded by sensorimotor representations (Mandler, 1992; Lakoff and Johnson, 1999).
The architectural and developmental perspective points to a possible roadmap for studying embodied cognition through epigenetic, evolutionary, or developmental robotics. One could investigate whether organisms provided with simple sensorimotor circuits (Braitenberg, 1984) develop behavioral strategies for facing their adaptive problems (e.g., discriminating good from poisonous food) that can be considered precursors of cognitive abilities (e.g., categorization abilities). Importantly, one could also examine whether these cognitive abilities maintain vestigial (embodied) aspects of the earlier sensorimotor skills. The literature on evolutionary robotics offers examples of how flexible abilities (e.g., categorization abilities) can be developed, which have always been studied under the rubric of cognitive processing and without reference to behavior, and that rely on behavioral strategies only, without rich internal representations. For instance, Nolfi (2005; see also Beer, 2003) has studied how robots equipped with simple sensors can learn to discriminate circles from squares without any memory mechanism, by simply moving toward the most informative points so as to produce a different sensory flow for each shape. Such studies offer an “intuition pump” and a fresh view of how behavioral strategies could actually implement basic forms of cognition, suggesting that they could be reused (at least partially) even in more sophisticated ones – thus making the case that evolution constrained our higher-level cognitive abilities to be embodied. Less studied in this literature is, in general, an analysis of how increasingly complex abilities (e.g., human-level) could have developed on the basis of their putative evolutionary precursors.
A related line of research is the attempt to find basic (computational and neural) mechanisms that could have facilitated the development of cognitive abilities. For instance, some researchers have made the case that prediction abilities, originally developed for the sake of action control, could have bootstrapped higher-level cognitive and social abilities and prospection (Pezzulo and Castelfranchi, 2007, 2009; Moller and Schenck, 2008).
Overall, a first significant challenge for cognitive robotics research consists in studying the development of embodied cognition and how sensorimotor information interfaces with cognition. In other words, a possible lesson for cognitive robotics research is to pay attention to how an ability could have been developed, and not only to its end state. Developmental studies have played an important role in forming our understanding or a continuity of sensorimotor action and cognition (e.g., the dynamical system perspective of Thelen and Smith, 1994; see also von Hofsten, 2004). Cognitive robotics can contribute by systematically manipulating a robot’s knowledge and skills, in order to understand what are the necessary prerequisites for the development of a particular cognitive ability, and by studying the environmental conditions that facilitate or prevent cognitive development.
Challenge 2. Exploring the (Causal) Influence of Embodied Phenomena for Cognitive Processes
As suggested in the previous section, cognitive processes appear to “leak” into the motor system. Therefore, it appears that the very reason we can measure cognitive processes via nearly continuous dense-sampling recordings of motor movement (e.g., eye movements, reaching movements, bimanual rhythmic movements, postural sway) originates from the fact that the neural subsystems implementing those cognitive processes cannot help but “leak” their patterns of neural activation continuously into the various motor subsystems. That is, the very fact that we can learn about cognition by recording continuous motor output strongly implies that cognition is embodied. When those neural subsystems are dynamically coupled, a signal arising in one of them routinely is detectable as a signal in the other. Importantly for computational implementations of embodied cognition, this should be expected to happen in both directions – otherwise embodied phenomena would have no causal impact on cognitive processing.
Bidirectional synaptic pathways are the rule in cortex (Churchland and Sejnowski, 1992), and unidirectional projections between cortical areas are quite rare. Therefore, from a neurophysiological standpoint, it would seem unlikely that cognitive subsystems (in frontal cortex) could “leak” their patterns of neural activation out to sensory subsystems (in occipital and temporal cortices) and motor subsystems (in frontoparietal areas), but not the inverse. However, in formulating a defense for the amodal symbolic cognition framework, Mahon and Caramazza (2008) have proposed exactly that. To their credit, they acknowledge the preponderance of existing laboratory evidence supporting the spreading of activation from cognitive processes to sensory and motor processes. They propose an account in which cognition itself may be “disembodied” in the traditional sense that it conducts its business via amodal rules and abstract symbols (unlike perception and action), and the activation of those symbols then spreads to sensorimotor areas to produce the kind of results found in much of embodied cognition research. If the directionality of that spreading activation is such that the symbolic processes modulate sensorimotor processes, but not vice versa, then computations within the cognition module would not be influenced by whether or not those connections to sensorimotor processes existed (see also Pylyshyn, 1974). Adhering to that unidirectional influence is crucial in Mahon and Caramazza’s (2008) proposal because if sensorimotor processes can directly influence the algorithms being used in the symbolic processes, then those symbolic processes would not be purely amodal and abstract.
Mahon and Caramazza’s (2008) argument is a powerful one because most of the evidence for embodied cognition can fit nicely into their spreading activation account, which preserves a role for purely abstract symbolic processing at some level. However, evidence for the other direction of influence, which compromises the purity of abstract symbolic processing, is beginning to accumulate (reviewed in Barsalou et al., 2003).
More embodiment experiments need to explore this directionality of effect: sensory and motor perturbations influencing central cognitive processes. Studies that show an early influence of perceptual and motor processes on cognition (see later on timing issues), as well as studies that suggest causality (e.g., TMS studies) are particularly relevant because these are difficult to explain as a by-product of a spreading activation and reverberation phenomenon. For example, Pulvermüller (1999) reported a collection of neuroimaging findings demonstrating that comprehension of action-based language triggers activation not only of language areas of the brain but also of limb-appropriate areas of motor cortex. Those findings epitomize the typical directionality of effect in embodiment studies. To show the reverse, Pulvermüller et al. (2005) conducted a transcranial magnetic stimulation study showing that mild TMS potentiation of the leg region of motor cortex improved reaction times to leg-action words (compared to arm-action words) in a lexical decision task (see also D’Ausilio et al., 2009). Behavioral studies have demonstrated this type of phenomena as well. After Richardson et al. (2003) showed that the image-schematic orientation of certain verbs influences visual attention in an object discrimination task, Toskos et al. (2004) showed that a controlled regime of repeated horizontal or vertical eye movements influenced memory for the (vertical or horizontal) verbs that were heard during those eye movements. In an elegant pair of studies, Meteyard et al. (2007) first showed that hearing directional motion verbs influenced d-prime in a motion detection task, and then Meteyard et al. (2008) showed that watching subtle visual motion signals influenced reaction times to directional verbs in a lexical decision task. Finally, one of the few examples of embodiment influencing high-level cognitive reasoning comes from a problem-solving experiment where the burgeoning onset of insight into the solution (the Aha! moment) for a difficult diagrammatic problem was correlated with a particular pattern of spontaneous eye movements which seemed to “participate” in the generation of the solution (Grant and Spivey, 2003). Thomas and Lleras (2007) then used the same problem and diagram, but enforced that particular pattern of eye movements as a secondary task, and participants were suddenly able to discover the solution with a significantly higher frequency. Both Smith (2005) and Ross et al. (2007) showed that repeated pairing of objects with actions influences their cognitive representation, as measured with a stronger congruency effect in object classification after training compared to before training. This reverse directionality of motor processes influencing cognitive processes is not merely another instance of Mahon and Caramazza’s (2008) spreading activation idea. It shows that those cognitive processes do not “go about their business” the same way they always would have irrespective of the motor constraints. It shows that the cognitive algorithms for word reading, speech recognition, object recognition, and even problem-solving, all incorporate information from motor algorithms when producing their results.
Because these directionality effects, and their time courses, add further nuance and structure to the embodied cognition literature, it becomes especially important for theories of embodied cognition to be computationally implemented in order to make quantitative predictions of laboratory results explicit, and to study rigorously what is the role of perceptual and motor processes in cognitive tasks by comparing systems that include or exclude them (for instance, simulating lesions or “virtual lesions” such as TMS). If we are to understand embodied cognition as a natural consequence of rich and continuous recurrent interactions among neural subsystems, then building interactivity into models of cognition should have embodiment fall out of the simulation naturally. A number of neural network models (Howell et al., 2005; Mayberry et al., 2009; Anderson et al., 2010), computational simulations (Joyce et al., 2003; Scheutz et al., 2004; Cangelosi and Riga, 2006; Pezzulo and Calvi, in press) and robots (Brooks and Stein, 1994; Roy, 2005b) have begun to implement such simulations of embodied cognition, and further research along these lines is required.
Challenge 3. Specifying the Time Course of Activation for Embodied Concepts
We have discussed that processing models of embodied cognitive phenomena are needed at this stage. A key issue for assessing the suitability of such models is their ability to explain the unfolding in time of cognitive processes, and how they relate to the timing of motor and perceptual processes in the brain. Indeed, assessing the time course of activation of motor processes during cognitive tasks is key to arguments regarding their causal role. Take as an example the processing of language. Embodied language processing (both comprehension and production) has now been studied in a number of ways, covering neuroscientific as well as behavioral approaches. Several empirical findings constrain the temporal dynamics of embodiment processes and should thus also advise their computational modeling. One well-known finding is the rapid and somatotopic activation of motor- and premotor cortical areas while passively viewing action verbs (Pulvermüller, 2005). This finding suggests that the meaning of words is available as early as 150 ms after visual stimulus onset, a result that has been corroborated by electrophysiological evidence (Sereno et al., 1998; Sereno and Rayner, 2003). More importantly, it means that a body-specific representation of word meaning has been created within such a short time that it is unlikely that strategic factors will have contributed to the effect. How does a computational architecture for language comprehension include access to bodily representations? Moreover, how does the comprehension process in turn affect the use of motor structures, as is suggested by the kinematic recordings of Boulenger et al. (2006)? These authors found that action verb meaning selectively interferes with action execution within 200 ms of action onset, but only when the action is initiated prior to lexical processing. When lexical processing precedes motor activity, the effect is facilitatory. In a similar vein, the incremental reading work by Zwaan and Taylor (2006) suggests that there is an immediate but time-limited activation of motor congruency effects that can be expressed in faster knob turning when reading about a directionally corresponding action. Interestingly, this congruency effect can then be re-instantiated when referring back to the described action with an adjective (Taylor and Zwaan, 2008).
The time course of embodiment effects is also of interest as an internal validation of the embodied cognition view of conceptual representation. One would expect that concrete concepts that have had a more direct grounding in sensorimotor experiences should show more rapid embodiment signatures when compared to more abstract concepts which require metaphorical mapping and indirect grounding. But even when controlling for word frequency, this comparison may be flawed, due to the differential age of acquisition which favors concrete words. In addition, as we have already remarked, timing issues could be critical for explaining apparently contradictory findings on the interference (facilitation or inhibition) of embodied processes.
In summary, there is now a detailed body of work on the time course of activation of concepts. What is needed now are integrative models that make specific predictions regarding the time course of embodied cognitive processes. One example is Chersi et al. (2010) who studied the precise dynamics underling the relation between language and action. Their model predicts interference or facilitation effects across linguistic and action tasks as depending on the time course of activation of associated representations.
Challenge 4. Developing Embodied Computational Models of Symbolic and Linguistic Operations
It is a common view that a true “benchmark” for embodied theories of cognition is explaining symbolic operations, which have been the province of amodal theories since the beginning of cognitive science (with few exceptions). This is certainly a difficult challenge for computational models as well; AI systems have tackled symbolic operations from the very beginning, but with little success. The wide domain of symbolic manipulations, which loosely includes reasoning and abstract thinking, predication, conceptual combination, language and communication, and which is typical of humans and possibly few other species, is both a challenge and a huge opportunity. Could embodied cognition be the right route for explaining computational symbolic processing? And, at the same time, could computational modeling provide strong evidence in favor of embodied cognitive theories? Our introductory examples of embodied arithmetic suggest a positive answer.
However, developing embodied approaches to symbolic operations requires a rethinking of most of the basic assumptions of traditional symbolic processing, in which symbols were taken as input, represented and outputted as symbols (with the notable exception of a few connectionist models). Indeed, it is still unclear how, from an embodied perspective, basic symbolic operations should be implemented. Barsalou’s (1999, 2003, 2005) articles make the case that perceptual symbols can implement symbolic (or symbolic-like) operations, and provide initial thoughts on how this could be possible, but there are still many open questions, as the following examples attest. What specific computational mechanisms underlie the type-token propositions that result from categorization? What specific computational mechanisms produce the basic inferences that follow from categorization and produce anticipation? What specific computational mechanisms integrate and combine concepts into larger conceptual structures as needed to comprehend the world and achieve goals? What specific computational mechanisms underlie top-down construals of the world that are mapped into online bottom-up sensorimotor experience, producing the fusions that characterize experience? To what extent are these operations implemented explicitly in the system versus emerging implicitly? Preliminary empirical evidence for these accounts of symbolic operations are reviewed in Barsalou (2008b).
As already remarked, symbolic processing is often considered to be highly related to abstraction on the one hand, and to language on the other hand. This leads to five additional important questions. How are abstract concepts represented and processed in a grounded system? What roles do they play in a grounded system? What is the role of language in learning and using them? To what extent should language or other communicative mechanisms be included in a grounded system? Should robotics researchers currently try to build a human (with language) or a non-human (with simple communication)? Recent research on language development and communication in robotics (reviewed above) has begun to elucidate these topics, but there clearly exist numerous avenues for future research.
Challenge 5. Realizing Situated and Complete Architectures Without Losing Contact with Data
One could ask what specific scenarios should be studied to cause embodied cognitive modeling research to advance most rapidly. On the one hand, if one aims to replicate specific experimental results, the scenario studied is constrained by the original experimental set-up. On the other hand, building many micro-simulations, one for each task to be modeled, could lead to a proliferation of disconnected models. Therefore, it would be equally valuable to create “unified” scenarios, or scenarios that could support the modeling and testing of many embodied phenomena. To do so, it is necessary to review critically what are the most important (and general) characteristics of environments, embodiment, and tasks that could be included.
Because (goal-directed) situated action in the environment is fundamental for all organisms, implementing embodied cognition that supports intelligent activity in a few critical situations (e.g., related to the organism’s life and death) may be a good place to start (Robbins and Aydede, 2008). This viewpoint is not novel, as most recent research in artificial cognitive systems has focused on the realization of situated agents, or agents that dwell in complex environments (however, simplified with respect to the real environment), and must “close the loop” from (real) perception to (real) action so as to satisfy their internal needs and motivations (which, in most cases, are quite simplified as well). This set-up is beneficial for embodied cognitive modeling for many reasons. First, it forces modelers to build complete embodied architectures for achieving goals in specific situations rather than implementing specific capabilities, such as goal setting, planning, perception, action, cognition, affect, reward, and learning. This permits us to study how perceptual, motor, affective, and cognitive abilities interact effectively, and how advanced abilities can emerge from the coordination of simpler ones (Barsalou et al., 2007). Indeed, because a central point of embodiment concerns the interactions among, and integration of, these systems, building larger scale models appears to be necessary. Second, in their attempt to build situated agents, modelers have widely recognized that the ways in which agents interact with their environments profoundly modify their representations and cognitive abilities, and that indeed agents cannot be studied in complete isolation from the environments in which they acquire their skills, and without including realistic details of their embodiment. Thus, this approach points quite directly toward grounded and embodied approaches to cognition.
However, if, on the one hand, generality of scenarios is desirable, this procedure comes at the risk of losing contact with human and animal data. Indeed most models developed under the hat of “situated cognition” (or artificial life, or AI) are indeed only loosely related to what is currently known about animal cognition. In addition, the scenarios that are currently employed in artificial cognitive systems research are more related to the basic survival of the organisms, but make it difficult to tackle higher-level cognitive abilities. One challenge for future research in embodied cognitive modeling is the realization of design principles (for architectures, scenarios, and embodiment) that are general enough to study many phenomena, but at the same time are specific enough to avoid losing contact with data and animal or human experiments.
Challenge 6. Realizing Realistic Social Scenarios for Studying Collaborative, Competitive, Communication, and Cultural Abilities
In the previous section, we focused on the realization of (possibly complete) goal-directed agent architectures. On the other hand, the realization of social scenarios, which involve human–robot interactions or coordinated interaction of multi-agent teams, is important as well. Although most theories of embodied cognition tend to more strongly emphasize the individual than the social aspects of cognition, they are not at odds with acknowledging the essentially social nature of learning and life of most animal species (including, of course, humans), and on the cultural origin of their representations and behaviors.
A popular research field in robotics and human–robot interaction concerns imitation, mindreading, intersubjectivity, and tool use, with an emphasis on their reliance on subpersonal processes such as prediction and mental simulation, and their sensorimotor roots (Demiris and Khadhouri, 2005; Oztop et al., 2005; Arbib et al., 2009). Other studies have focused on the affective dimension of human–robot interaction (Breazeal, 2003), and could provide interesting insights for embodied cognition research, in which this topic is seldom studied.
Unfortunately, although social and cultural robotics are being increasingly studied (Breazeal, 2004), it is difficult to combine social, cultural, and embodied aspects in the same endeavor. However, this is potentially very interesting for embodied cognition research, since it enables testing the relative importance of embodied and social (or cultural) phenomena in shaping learning and behavior. For instance, one of the promises of the research program of Steels and Kaplan (2000, 2002) is providing insights on how embodiment and situatedness could have constrained language acquisition. Collective robotics studies on the combined evolutionary learning of (collective) behavior and language (Marocco et al., 2003) could be informative as well, as these dynamics would be difficult to test experimentally. These two examples, together with the other models of symbol grounding and language learning discussed above (e.g., Cangelosi and Riga, 2006), provide a computational framework for investigating the embodiment and situated cognition phenomena of language and communication. The double function of language, as a social/communicative means, and as an individual/cognitive capability, derives from its fundamental property that allows us to internally re-represent the world we live in. This is possible through the mechanism of symbol grounding, that is, the ability to associate entities and states in the external and internal world with internal categorical representations. The symbol grounding mechanism, as our language, has both an individual and a social component (Cangelosi, 2006). The individual component, called “Physical Symbol Grounding,” refers to the ability of each individual to create an intrinsic link between world entities and internal categorical representations. The social component, called “Social Symbol Grounding,” refers to collective negotiation for the selection of shared symbols (words) and their grounded meanings. The extensive evidence on the mirror neuron system in both individual and social cognition provides support for the hypothesis of a link between the social (e.g., imitation) components of action production/recognition and language and communication (Rizzolatti and Arbib, 1998). This hypothesis can be computationally investigated through social and cognitive robotics experiments, as in Tani et al.’s (2004) model of mirror neuron system in language learning. (The modeling of mirror neurons has attracted a lot of attention in the last years; we refer the reader to Oztop et al., 2006 for a detailed review).
Overall, robotic studies of interactive, social, linguistic, and cultural dynamics, along with their interrelations, are particularly important for the extension of current embodied theories, which have not sufficiently incorporated these aspects, instead mostly focusing on individual cognition. One reason for this lack of attention is that all these dynamics are extremely difficult and expensive to study experimentally. Here the synthetic methodology offers a significant contribution because it involves many fewer constraints than empirical studies with living organisms. To make this cross-fertilization possible, however, it is desirable to design social robotic studies that incorporate increasingly more complex social dynamics (imitation, cooperation, joint action, and possibly the dynamics of whole societies of agents) and aim to reproduce social grounding and symbolic learning phenomena. A competing constraint is that it is necessary for these studies to make explicit and testable predictions, which is rare at this time.
Conclusion
Embodied effects have been consistently found in many cognitive tasks, including, for instance, action and object observation, memory, and language processing. Comprehensive theories that advocate the importance of grounding, embodiment, and situatedness have been proposed to explain these findings, which are corroborated by empirical data, but in most cases lack a precise computational or mathematical formalization. Computational modeling of embodied phenomena could contribute to the development of embodied theories of cognition by having the same kind of impact that early AI concepts (such as symbols, plans, or chunking) had on early theories in cognitive science. In addition, compared to early AI studies, embodied computational models have the potential to derive more precise predictions because they typically involve more realistic agent–environment interactions. Furthermore, embodied theories of cognition could provide insights for the realization of robots with sophisticated cognitive abilities that other design methodologies have not been able to realize.
Throughout this article, we have proposed that cognitive robotics is an ideal platform for studying embodied phenomena (including developmental and social aspects), and vice versa that embodied theories can provide insights for the realization of novel, more efficacious, and reliable artificial cognitive systems. To help the realization of embodied computational models, this article has clarified the most important components and processes that such models should include, highlighted how the synthetic methodology could help research in embodied cognition, and proposed six challenges for modelers. Our hope is that, in 5 or 10 years, we will see another special issue on “Embodied and grounded cognition” that describes success stories in tackling these challenges, and that in turn this progress will inspire novel and more complete cognitive theories, and ultimately a novel paradigm for (individual and social) cognition that has grounding, embodiment, and situatedness at its heart.
Conflict of Interest Statement
The authors declare that the research was conducted in the absence of any commercial or financial relationships that could be construed as a potential conflict of interest.
Acknowledgments
We thank the reviewers for their precious suggestions. The effort of Giovanni Pezzulo was supported by the European Union FP7 project HUMANOBS. The effort of Angelo Cangelosi was supported by the European Union FP7 projects ITALK and ROBOTDOC. Angelo Cangelosi and Martin H. Fischer also acknowledge the support of the UK Engineering and Physical Sciences Research Council, through the project VALUE.
References
Allopenna, P. D., Magnuson, J. S., and Tanenhaus, M. K. (1998). Tracking the time course of spoken word recognition using eye movements: evidence for continuous mapping models. J. Mem. Lang. 38, 419–439.
Altmann, G., and Kamide, Y. (1999). Incremental interpretation at verbs: restricting the domain of subsequent reference. Cognition 73, 247–264.
Anderson, M. L. (2010). Neural reuse: a fundamental organizational principle of the brain. Behav. Brain Sci. 33, 245–266.
Anderson, S. E., Huette, S., Matlock, T., and Spivey, M. J. (2010). On the temporal dynamics of negated perceptual simulations. In F. Parrill, M. Turner and V. Tobin (Eds.), Meaning, Form, and Body. Stanford: CSLI Publications.
Arbib, M., Bonaiuto, J., Jacobs, S., and Frey, S. (2009). Tool use and the distalization of the end-effector. Psychol. Res. 73, 441–462.
Arbib, M. A., Billard, A., Iacoboni, M., and Oztop, E. (2000). Synthetic brain imaging: grasping, mirror neurons and imitation. Neural Netw. 13, 975–997.
Armstrong, D., Stokoe, W., and Wilcox, S. (1995). Gesture and the nature of language. Cambridge: Cambridge University Press.
Bar, M. (2009). The proactive brain: memory for predictions. Philos. Trans. R. Soc. Lond., B, Biol. Sci. 364, 1235–1243.
Barsalou, L. W. (2003). Abstraction in perceptual symbol systems. Philos. Trans. R. Soc. Lond., B, Biol. Sci. 358, 1177–1187.
Barsalou, L. W. (2005). “Abstraction as dynamic interpretation in perceptual symbol systems,” in Building Object Categories. Carnegie Symposium Series, eds L. Gershkoff-Stowe and D. Rakison (Mahwah, NJ: Erlbaum), 389–431.
Barsalou, L. W. (2008b). “Grounding symbolic operations in the brain’s modal systems,” Embodied Grounding: Social, Cognitive, Affective, and Neuroscientific Approaches, eds G. R. Semin and E. R. Smith (New York: Cambridge University Press), 9–42.
Barsalou, L. W. (2009). Simulation, situated conceptualization, and prediction. Philos. Trans. R. Soc. Lond., B, Biol. Sci. 364, 1281–1289.
Barsalou, L. W., Breazeal, C., and Smith, L. B. (2007). Cognition as coordinated non-cognition. Cogn. Process. 8, 79–91.
Barsalou, L. W., Niedenthal, P. M., Barbey, A., and Ruppert, J. (2003). “Social embodiment,” in The Psychology of Learning and Motivation, Vol. 43, ed. B. Ross (San Diego: Academic Press), 43–92.
Barsalou, L. W., and Wiemer-Hastings, K. (2005). “Situating abstract concepts,” in Grounding Cognition: The Role of Perception and Action in Memory, Language, and Thought, eds D. Pecher and R. Zwaan (New York: Cambridge University Press), 129–63.
Beer, R. D. (2003). The dynamics of active categorical perception in an evolved model agent. Adapt. Behav. 11, 209–243.
Bekkering, H., Neggers, S. F. W. (2002). Visual search is modulated by action intentions. Psychol. Sci. 13, 370–374.
Borghi, A. M., Glenberg, A., and Kaschak, M. (2004). Putting words in perspective. Mem. Cogn. 32, 863–873.
Boulenger, V., Roy, A. C., Paulignan, Y., Deprez, V., Jeannerod, M., and Nazir, T. A. (2006). Cross-talk between language processes and overt motor behavior in the first 200 ms of processing. J. Cogn. Neurosci. 18, 1607–1615.
Caligiore, D., Borghi, A. M.., Parisi, D., and Baldassarre, G. (2010). TRoPICALS: a computational embodied neuroscience model of experiments on compatibility effects. Psychol. Rev. 117, 1188–1228.
Calvert, G. A., Spence, C., and Stein, B. E. (2004). The Handbook of Multisensory Processes. Cambridge, MA: MIT Press.
Cangelosi, A. (2001). Evolution of communication and language using signals, symbols and words. IEEE Transactions on Evolutionary Computation. 5, 93–101.
Cangelosi, A. (2010). Grounding language in action and perception: from cognitive agents to humanoid robots. Phys. Life Rev. 7, 139–151.
Cangelosi, A., and Parisi, D. (eds). (2002). Simulating the Evolution of Language. London: Springer.
Cangelosi, A., and Parisi, D. (2004). The processing of verbs and nouns in neural networks: insights from synthetic brain imaging. Brain Lang. 89, 401–408.
Cangelosi, A., and Riga, T. (2006). An embodied model for sensorimotor grounding and grounding transfer: experiments with epigenetic robots. Cogn. Sci. 30, 673–689.
Carandini, M., Demb, J., Mante, V., Tolhurst, D., Dan, Y., Olshausen, B., Gallant, J., and Rust, N. (2005). Do we know what the early visual system does? J. Neurosci. 25, 10577–10597.
Chersi, F., Thill, S., Ziemke, T., and Borghi, A. M. (2010). Sentence processing: linking language to motor chains. Front. Neurorobot. 4:4. doi: 10.3389/fnbot.2010.00004
Cisek, P., and Kalaska, J. F. (2004). Neural correlates of mental rehearsal in dorsal premotor cortex. Nature 431, 993–996.
Cisek, P., and Kalaska, J. F. (2005). Neural correlates of reaching decisions in dorsal premotor cortex: specification of multiple direction choices and final selection of action. Neuron 45, 801–814.
Corballis, M. C. (2003). From hand to mouth: the origins of language. New York, NY: Princeton University Press.
Craig, A. D. (2002). How do you feel? Interoception: the sense of the physiological condition of the body. Nat. Rev. Neurosci. 3, 655–666.
Craighero, L., Nascimben, M., and Fadiga, L. (2004). Eye position affects orienting of visuospatial attention. Curr. Biol. 14, 331–333.
Damasio, A. R. (1989). Time-locked multiregional retroactivation: a systems-level proposal for the neural substrates of recall and recognition. Cognition 33, 25–62.
D’Ausilio, A., Pulvermüller, F., Salmas, P., Bufalari, I., Begliomini, C., and Fadiga, L. (2009). Motor somatotopy of speech perception. Curr. Biol. 19, 381–385.
Dehaene, S., Bossini, S., and Giraux, P. (1993). The mental representation of parity and number magnitude. J Experimental Psychology: General, 122, 371–396.
Demiris, Y., and Khadhouri, B. (2005). Hierarchical attentive multiple models for execution and recognition (hammer). Robot. Auton. Syst. J. 54, 361–369.
Dijkstra, K., Kaschak, M. P., and Zwaan, R. A. (2008). Body posture facilitates retrieval of autobiographical memories. Cognition 102, 139–149.
Ellis, R., Tucker, M., Symes, E., and Vainio, L. (2007). Does selecting one visual object from several require inhibition of the actions associated with non-selected objects? J. Exp. Psychol. Hum. Percept. Perform. 33, 670–691.
Elman, J. L., Bates, E. A., Johnson, M. H., Karmiloff-Smith, A., Parisi, D., and Plunkett, K. (1996). Rethinking Innateness: A Connectionist Perspective on Development. Cambridge, MA: MIT Press.
Eskenazi, T., Grosjean, M., Humphreys, G. W., and Knoblich, G. (2009). The role of motor simulation in action perception: a neuropsychological case study. Psychol. Res. 73, 477–485.
Fahlman, S. E., and Lebiere, C. (1990). “The cascade-correlation learning architecture,” in Advances in Neural Information Processing Systems, Vol. 2, ed. D. S. Touretzky (Los Altos, CA: Morgan Kaufmann Publishers Inc.), 524–532.
Fellous, J.-M., and Arbib, M. (eds). (2006). Who Needs Emotions? The Brain Meets the Robot, Vol. 24. New York: Oxford University Press, 271–272.
Fias, W., Brysbaert, M., Geypens, F., and d’Ydewalle, G. (1996). The importance of magnitude information in numerical processing: Evidence from the SNARC effect. Mathematical Cognition, 2, 95–110.
Fischer, M. H. (2008). Finger counting habits modulate spatial-numerical associations. Cortex, 44, 386–392.
Fischer, M. H., and Hoellen, N. (2004). Space-based and object-based attention depend on motor intention. J. Gen. Psychol. 131, 365–377.
Fischer, M. H., Mills, R. A., and Shaki, S. (2010). How to cook a SNARC: number placement in text rapidly changes spatial–numerical associations. Brain Cogn. 72, 333–336.
Fischer, M. H., Prinz, J., and Lotz, K. (2008). Obligatory attention to action goals. Quarterly Journal of Experimental Psychology, 61, 860–868.
Fischer, M. H., Shaki, S., and Cruise, A. (2009). It takes only one word to quash the SNARC. Exp. Psychol. 56, 361–366.
Fischer, M. H., and Shaki, S. (2011). Predilection or preconception? A reply to Treccani and Umilta. Brain Cogn. (in press).
Frischen, A., Bayliss, A. P., and Tipper, S. P. (2007). Gaze cueing of attention: visual attention, social cognition, and individual differences. Psychol. Bull. 133, 694–723.
Garagnani, M., Wennekers, T., and Pulvermuller, F. (2007). A neural model of the language cortex. Neurocomputing 70, 1914–1919.
Glenberg, A. M., and Kaschak, M. P. (2002). Grounding language in action. Psychon. Bull. Rev. 9, 558–565.
Gold, J., and Shadlen, M. (2000). Representation of a perceptual decision in developing oculomotor commands. Nature 404, 390–394.
Grant, E. R., and Spivey, M. J. (2003). Eye movements and problem solving: guiding attention guides thought. Psychol. Sci. 14, 462–466.
Hietanen, J. K. (2002). Social attention orienting integrates visual information from head and body orientation. Psychol. Res. 66, 174–179.
Hommel, B., Musseler, J., Aschersleben, G., and Prinz, W. (2001). The theory of event coding (TEC): a framework for perception and action planning. Behav. Brain Sci. 24, 849–878.
Horwitz, B., and Tagamets, M.-A. (1999). Predicting human functional maps with neural net modeling. Hum. Brain Mapp. 8, 137–142.
Howell, S., Jankowicz, D., and Becker, S. (2005). A model of grounded language acquisition: sensorimotor features improve lexical and grammatical learning. J. Mem. Lang. 53, 258–276.
Ito, Y., and Hatta, T. (2004). Spatial structure of quantitative representation of numbers: evidence from the SNARC effect. Mem. Cogn. 32, 662–673.
Joyce, D., Richards, L., Cangelosi, A., and Coventry, K. R. (2003). “On the foundations of perceptual symbol systems: specifying embodied representations via connectionism,” in The Logic of Cognitive Systems. Proceedings of the Fifth International Conference on Cognitive Modeling, eds F. Detje, D. Dörner, and H. Schaub (Bamberg: Universitätsverlag), 147–152.
Karmiloff-Smith, A. (1992). Beyond Modularity: A Developmental Perspective on Cognitive Science. Cambridge, MA: MIT Press.
Knops, A., Thirion, B., Hubbards, E. M., Michel, V., and Dehaene, S. (2009). Recruitment of an area involved in eye movements during mental arithmetic. Science 324, 1583–1585.
Körding, K. P., and Wolpert, D. M. (2004). Bayesian integration in sensorimotor learning. Nature 427, 244–247.
Kveraga, K., Ghuman, A. S., and Bar, M. (2007). Top-down predictions in the cognitive brain. Brain Cogn. 65, 145–168.
Lindemann, O., Alipour, A., and Fischer, M. H. (in press). Finger counting habits in Middle-Eastern and Western individuals: an online survey. J. Cross Cult. Psychol.
Loetscher, T., Schwarz, U., Schubiger, M., and Brugger, P. (2008). Head turns bias the brain’s random number generator. Curr. Biol. 18, R60–R62.
Macura, Z., Cangelosi, A., Ellis, R., Bugmann, D., Fischer, M. H., and Myachykov, A. (2009). “A cognitive robotic model of grasping,” in Proceedings of the Ninth International Conference on Epigenetic Robotics, Venice, November 12–14, 2009.
Madden, C. J., Hoen, M., and Dominey P. F. (2009). A cognitive neuroscience perspective on embodied language for human–robot cooperation. Brain Lang. 112, 180–188.
Mahon, B. Z., and Caramazza, A. (2008). A critical look at the embodied cognition hypothesis and a new proposal for grounding conceptual content. J. Physiol. Paris 102, 59–70.
Marocco, D., Cangelosi, A., and Nolfi, S. (2003). The emergence of communication in evolutionary robots. Philos. Trans. A Math. Phys. Eng. Sci. 361, 2397–2421.
Mayberry, M., Knoeferle, P., and Crocker, M. (2009). Learning to attend: a connectionist model of situated language comprehension. Cogn. Sci. 33, 449–496.
Meteyard, L., Bahrami, B., and Vigliocco, G. (2007). Motion detection and motion words: language affects low-level visual perception. Psychol. Sci. 18, 1007–1013.
Meteyard, L., Zokaei, N., Bahrami, B., and Vigliocco, G. (2008). Visual motion interferes with lexical decision on motion words. Curr. Biol. 18, R732–R733.
Metta, G., and Cangelosi, A. (in press). “Cognitive robotics,” in Encyclopedia of the Sciences of Learning, ed. N. M. Seel (Springer).
Metta, G., Sandini, G., Vernon, D., Natale, L., and Nori, F. (2008). “The iCub humanoid robot: an open platform for research in embodied cognition,” in PerMIS: Performance Metrics for Intelligent Systems Workshop, August 19–21, 2008, Washington, DC.
Moller, R., and Schenck, W. (2008). Bootstrapping cognition from behavior – a computerized thought experiment. Cogn. Sci. 32, 504–542.
Mumford, D. (1992). On the computational architecture of the neocortex II. The role of cortico-cortical loops. Biol. Cybern. 66, 241–251.
Myachykov, A., Platenburg, W., and Fischer, M. H. (2009). Non-abstractness as mental simulation in the representation of number. Behav. Brain Sci. 32, 343–344.
Nolfi, S. (2005). “Categories formation in self-organizing embodied agents,” in Handbook of Categorization in Cognitive Science, eds H. Cohen and C. Lefebvre (Elsevier), 869–889.
Oztop, E., Kawato, M., and Arbib, M. (2006). Mirror neurons and imitation: a computationally guided review. Neural Netw. 19, 254–271.
Oztop, E., Wolpert, D., and Kawato, M. (2005). Mental state inference using visual control parameters. Cogn. Brain Res. 22, 129–151.
Patterson K., Nestor P. J., and Rogers T. T. (2007). Where do you know what you know? The representation of semantic knowledge in the human brain. Nat. Rev. Neurosci. 12, 976–987.
Pezzulo, G. (2008). Coordinating with the future: the anticipatory nature of representation. Minds Mach. 18, 179–225.
Pezzulo, G., and Calvi, G. (in press). Computational explorations of perceptual symbol systems theory. N. Ideas Psychol. doi: 10.1016/j.newideapsych.2009.07.004
Pezzulo, G., and Castelfranchi, C. (2007). The symbol detachment problem. Cogn. Process. 8, 115–131.
Pezzulo, G., and Castelfranchi, C. (2009). Thinking as the control of imagination: a conceptual framework for goal-directed systems. Psychol. Res. 73, 559–577.
Pfeifer, R., and Bongard, J. (2006). How the Body Shapes the Way We Think: A New View of Intelligence. Cambridge, MA: MIT Press.
Pinhas, M., and Fischer, M. H. (2008). Mental movements without magnitude? A study of spatial biases in symbolic arithmetic. Cognition 109, 408–415.
Pulvermüller, F. (2005). Brain mechanisms linking language and action. Nat. Rev. Neurosci. 6, 576–582.
Pulvermüller, F., Hauk, O., Ilmoniemi, R., and Nikulin, V. (2005). Functional links between motor and language systems. Eur. J. Neurosci. 21, 793–797.
Pylyshyn, Z. (1974). Minds, machines and phenomenology: some reflections on Dreyfus’ “What computers can’t do.” Cognition 3, 57–77.
Radulescu, P. V., Adam, J. J., Fischer, M. H., and Pratt, J. (2010). Fitts’s law violation and motor imagery: are imagined movements truthful or lawful? Exp. Brain Res. 201, 607–611.
Rao, R., and Ballard, D. (1999). Predictive coding in the visual cortex: a functional interpretation of some extra-classical receptive-field effects. Nat. Neurosci. 2, 79–87.
Richardson, D., and Dale, R. (2005). Looking to understand: the coupling between speakers’ and listeners’ eye movements and its relationship to discourse comprehension. Cogn. Sci. 29, 1045–1060.
Richardson, D., Spivey, M., Barsalou, L., and McRae, K. (2003). Spatial representations activated during real-time comprehension of verbs. Cogn. Sci. 27, 767–780.
Rizzolatti, G., and Craighero, L. (2004). The mirror-neuron system. Annu. Rev. Neurosci. 27, 169–192.
Robbins, P., and Aydede, M. (eds). (2008). Cambridge Handbook of Situated Cognition. New York: Cambridge University Press.
Rosenbaum, D. A., Carlson, R. A., and Gilmore, R. O. (2001). Acquisition of intellectual and perceptual-motor skills. Annu. Rev. Psychol. 52, 453–470.
Ross, B. H., Wang, R. F., Kramer, A. F., Simons, D. J., and Crowell, J. A. (2007). Action information from classification learning. Psychon. Bull. Rev. 14, 500–504.
Roy, D. (2005a). Semiotic schemas: a framework for grounding language in action and perception. Artif. Intell. 167, 170–205.
Roy, D. (2005b). Grounding words in perception and action: computational insights. Trends Cogn. Sci. 9, 389–386.
Schacter, D. L., Addis, D. R., and Buckner, R. L. (2007). Remembering the past to imagine the future: the prospective brain. Nat. Rev. Neurosci. 8, 657–661.
Scheutz, M., Eberhard, K., and Andronache, V. (2004). A real-time robotic model of human reference resolution using visual constraints. Connect. Sci. 16, 145–167.
Schöner, G. (2008). “Dynamical systems approaches to cognition,” in Toward a Unified Theory of Development: Connectionism and Dynamic Systems Theory Re-Considered, eds J. P. Spencer, M. S. Thomas, and J. L. McClelland (New York: Oxford University Press).
Schwarz, W., and Keus, I. (2004). Moving the eyes along the mental number line: comparing SNARC effects with manual and saccadic responses. Percept. Psychophys. 66, 651–664.
Sereno, S. C., and Rayner, K. (2003). Measuring word recognition in reading: eye movements and event-related potentials. Trends Cogn. Sci. 7, 489–493.
Sereno, S. C., Rayner, K., and Posner, M. I. (1998). Establishing a time-line of word recognition: evidence from eye movements and event-related potentials. Neuroreport 9, 2195–2200.
Shockley, K., Santana, M., and Fowler, C. (2003). Mutual interpersonal postural constraints are involved in cooperative conversation. J. Exp. Psychol. Hum. Percept. Perform. 29, 326–332.
Shockley, K., and Turvey, M. T. (2005). Encoding and retrieval during bimanual rhythmic coordination. J. Exp. Psychol. Hum. Percept. Perform. 31, 980–990.
Simmons, W. K., and Barsalou, L. W. (2003). The similarity-in-topography principle: reconciling theories of conceptual deficits. Cogn. Neuropsychol. 20, 451–486.
Song, J., and Nakayama, K. (2009). Hidden cognitive states revealed in choice reaching tasks. Trends Cogn. Sci. 13, 360–366.
Spivey, M. J., Grosjean, M., and Knoblich, G. (2005). Continuous attraction toward phonological competitors. Proc. Natl. Acad. Sci. U.S.A. 102, 10393–10398.
Steels, L., and Kaplan, F. (2000). AIBO’s first words, the social learning of language and meaning. Evol. Commun. 4, 3–32.
Steels, L., and Kaplan, F. (2002). “Bootstrapping grounded word semantics,” in Linguistic Evolution Through Language Acquisition: Formal and Computational Models, ed. T. Briscoe (Cambridge: Cambridge University Press), 53–73.
Symes, E., Tucker, M., Ellis, R., Vainio, L., and Ottoboni, G. (2008). Grasp preparation improves change detection for congruent objects. J. Exp. Psychol. Hum. Percept. Perform. 43, 854–871.
Tanenhaus, M., Spivey-Knowlton, M., Eberhard, K., and Sedivy, J. (1995). Integration of visual and linguistic information during spoken language comprehension. Science 268, 1632–1634.
Tani, J., Ito, M., and Sugita, Y. (2004). Self-organization of distributedly represented multiple behavior schemata in a mirror system: reviews of robot experiments using RNNPB. Neural Netw. 17, 1273–1289.
Taylor, L. J., and Zwaan, R. A. (2008). Motor resonance and linguistic focus. Q. J. Exp. Psychol. 61, 869–904.
Thelen, E., Schöner, G., Scheier, C., and Smith, L. (2001). The dynamics of embodiment: a field theory of infant perseverative reaching. Brain Behav. Sci. 24, 1–33.
Thelen, E., and Smith, L. (1994). A Dynamic Systems Approach to the Development of Cognition and Action. Cambridge, MA: MIT Press.
Thomas, L., and Lleras, A. (2007). Moving eyes and moving thought: on the spatial compatibility between eye movements and cognition. Psychon. Bull. Rev. 14, 663–668.
Tikhanoff, V., Cangelosi, A., Fitzpatrick, P., Metta, G., Natale, L., and Nori, F. (2008). “An open-source simulator for cognitive robotics research: the prototype of the iCub humanoid robot simulator,” in Proceedings of IEEE Workshop on Performance Metrics for Intelligent Systems Workshop (PerMIS’08), eds R. Madhavan and E. R. Messina (Washington, DC: IEEE), 57–61.
Tipper, S., Howard, L., and Jackson, S. (1997). Selective reaching to grasp: evidence for distractor interference effects. Vis. Cogn. 4, 1–38.
Toskos, A., Hanania, R., and Hockema, S. (2004). “Eye scanpaths influence memory for spoken verbs,” in Proceedings of the 26th Annual Meeting of the Cognitive Science Society, eds K. Forbus, D. Gentner, and T. Regier (Mahwah, NJ: Erlbaum), 1643.
Tucker, M., and Ellis, R. (2001). The potentiation of grasp types during visual object categorization. Vis. Cogn. 8, 769–800.
Verschure, P., and Coolen, A. (1991). Adaptive fields: distributed representations of classically conditioned associations. Network 2, 189–206.
Wallace, R. J. (1971). Stimulus–response compatibility and the idea of a response code. J. Exp. Psychol. 88, 354–360.
Weiller, D., Märtin, R., Dähne, S., Engel, A. K., and König, P. (2010). Involving motor capabilities in the formation of sensory space representations. PLoS ONE 5, e10377. doi: 10.1371/journal.pone.0010377
Weng, J., McClelland, J., Pentland, A., Sporns, O., Stockman, I., Sur, M., and Thelen, E. (2001). Autonomous mental development by robots and animals. Science 291, 599–600.
Wood, G., Nuerk, H.-C., Willmes, K., and Fischer, M. H. (2008). On the cognitive link between space and number: A meta-analysis of the SNARC effect. Psychology Science Quarterly, 50, 489–525.
Ziemke, T. (2003). On the role of robot simulations in embodied cognitive science. AISB J. 1, 389–399.
Keywords: grounded cognition, embodiment, simulation, cognitive robotics, computational modeling, situated cognition, embodied cognition
Citation: Pezzulo G, Barsalou LW, Cangelosi A, Fischer MH, McRae K and Spivey MJ (2011) The mechanics of embodiment: a dialog on embodiment and computational modeling. Front. Psychology 2:5. doi: 10.3389/fpsyg.2011.00005
Received: 25 June 2010;
Accepted: 04 January 2011;
Published online: 31 January 2011.
Edited by:
Anna M. Borghi, University of Bologna, ItalyCopyright: © 2011 Pezzulo, Barsalou, Cangelosi, Fischer, McRae and Spivey. This is an open-access article subject to an exclusive license agreement between the authors and Frontiers Media SA, which permits unrestricted use, distribution, and reproduction in any medium, provided the original authors and source are credited.
*Correspondence: Giovanni Pezzulo, Istituto di Scienze e Tecnologie della Cognizione, Consiglio Nazionale delle Ricerche, Via S. Martino della Battaglia, 00185, Roma, Italy. e-mail:Z2lvdmFubmkucGV6enVsb0Bpc3RjLmNuci5pdA==