- 1 Center for Research on Individual Development and Adaptive Education, German Institute for International Educational Research, Frankfurt am Main, Germany
- 2 Department of Psychology, University of Konstanz, Konstanz, Germany
- 3 Department of Psychology, National Institute of Mental Health Center for the Study of Emotion and Attention, University of Florida, Gainesville, FL, USA
The ability to direct cognitive resources to target objects despite distraction by competing information plays an important role for the development of mental aptitudes and skills. We examined developmental changes of this ability in a cross-sectional design, using the “attentional blink” (AB) paradigm. The AB is a pronounced impairment of T2 report, which occurs when a first (T1) and second target (T2) embedded in a rapid stimulus sequence are separated by at least one distractor and occur within 500 ms of each other. Two groups of children (6- to 7-year-olds and 10- to 11-year-olds; ns = 21 and 24, respectively) were asked to identify green targets in two AB tasks: one using non-linguistic symbols and the other letters or words. The temporal distance or stimulus-onset asynchrony (SOA) between T1 and T2 varied between no intervening distractor (Lag 1, 116-ms SOA) and up to 7 intervening distractors (Lag 8, 928-ms SOA). In the symbol task, younger children linearly increased T2 identification with increasing lag. Older children, however, displayed a hook-shaped pattern as typically seen in adults, with lowest identification reports in T2 symbols at the critical blink interval (Lag 2, 232-ms SOA), and a slight performance gain for the Lag 1 condition. In the verbal task, the older group again exhibited a prominent drop in T2 identification at Lag 2, whereas the younger group showed a more alleviated and temporally diffuse AB impairment. Taken together, this pattern of results suggests that the control of attention allocation and/or working memory consolidation of targets among distractors represents a cognitive skill that emerges during primary school age.
Introduction
The effective selection of perceptual information for in-depth processing, at the cost of competing sensory input, is essential for adaptive behavior. The capability to selectively attend to relevant visual events and to protect the attended information against continued distraction has been associated with the development of higher-order cognitive skills and with indices of academic achievement (Rueda et al., 2005b; Heim et al., 2006). Recently, advances in the cognitive neurosciences have opened avenues for studying the many facets of such attentional selection in the context of increasingly complex tasks, enhancing ecological validity while maintaining experimental control of the stimuli and task. Research designs are now available that tap into complex aspects of attention selection such as resource sharing among multiple attended objects or the temporal competition among targets and distractor items. Work in this area has highlighted the role of strategic processes of attention regulation, as well as the flexible and dynamic nature of attention allocation across spatial and temporal dimensions. Although not much is known about the cognitive development of such strategic and dynamic aspects of attention allocation, there is an impressive body of research describing the development of attentional capacity and selection versus distraction across childhood and into adolescence (for a review, see Ridderinkhof and van der Stelt, 2000).
One traditional and robust measure of the ability to attentively select for a particular stimulus dimension is the distractor effect: Behavioral accuracy and response speed in a primary task suffer from the presence of task-irrelevant stimulus. This effect diminishes as age progresses from childhood to adolescence, which has been taken as a developmental index in the ability to better focus attention (Enns and Girgus, 1985). In a typical study (Cowan et al., 2006), 8- to 12-year-old children, younger (college-aged), and older adults (65–85 years) responded to color changes of one square embedded in a multi-object visual array. Results revealed that children and older adults had more difficulty binding visual features to locations than younger adults. This supports the notion that the ability to protect selected information against interference shows a pronounced developmental trajectory, and varies between individuals. Accordingly, Rueda et al. (2005b) reported that 5 days of attention training with preschoolers had not only beneficial effects on behavioral and neural parameters of resisting to attentional interference, but also on more general cognitive abilities, such as abstract reasoning.
Age-related differences in susceptibility to distraction by task-irrelevant stimuli were observed in the auditory domain as well (Wetzel et al., 2006): Electrophysiological measures indicated that the stages of early change detection, sensory facilitation, and orienting were more strongly affected by distractors in 6- to 8-year-old children, than in young adults. Conversely, a recent study of training effects on language processing demonstrated improvements in neural indices of auditory selective attention in 6- to 8-year-old language-learning impaired individuals, and to a lesser extent in their typically developing age-mates (Stevens et al., 2008). Importantly, this training regime (Fast ForWord-Language®, http://www.scilearn.com/index.php) aimed to enhance auditory rate processing through shaping and reinforcement in identification and discrimination tasks, and resulted in gains on a standardized language assessment. To summarize, previous work on the development of attentional selection across childhood and adolescence has highlighted that the vulnerability of attended information to distraction is greater in younger than older children, and that this difference is related to other higher-order skills as well.
In the present article, we extend this research to a specific skill in the context of selective attention and distraction, namely the ability to strategically allocate and distribute cognitive resources across multiple stimuli over time. Specifically, we examine the dynamic process of resource allocation to target events embedded in rapidly presented distractors. To be successful, this process needs to encompass effective attentive selection of a stimulus as well as protection/maintenance of the selected information against distraction. Both components can be operationalized using a rapid serial visual presentation (RSVP) task. In RSVP experiments, stimuli are presented sequentially at a high rate, for instance 10 items per second (see Raymond et al., 1992). In a typical experimental design, participants search the stimulus stream for specified target items. Attending to a first target (T1) embedded in the distractor stream often leads to a transient impairment in detecting or identifying a subsequent second target stimulus (T2). This so-called “attentional blink” (AB) effect (Raymond et al., 1992) has been demonstrated for a variety of stimuli such as symbols, letters, digits, and words (e.g., Raymond, 2003). Report rates for the second target are usually reduced for intertarget intervals between 200 and 500 ms.
A number of theoretical views of the AB attribute the impairment for T2 stimuli to decreased availability of cognitive resources (e.g., Chun and Potter, 1995; Jolicoeur et al., 2006) or attentional capacity (Vul et al., 2008), which is assumed to occur as a consequence of encoding/selecting the T1 item. In this perspective, over-allocation of resources to T1 is associated with lack of resources available for T2 in a trade-off fashion. Several accounts have explained this as a failure of working memory consolidation of the target due to limited resources (Jolicoeur et al., 2006). With regard to the present developmental study, this raises the question if AB performance is related to developmental changes on more traditional measures of working memory and attentional capacity such as the digit span test. In these procedures, participants listen to series of strings of digits read aloud, and are asked to repeat them back in the same – or reverse – order of presentation.
In the resource sharing account of the AB, resources over-allocated to T1 are at the expense of T2 processing, thus predicting overall better performance for the T1 stimuli occurring in trials in which T2 is missed, compared to correct T2 trials. Consistent with this prediction, relative increases in T1-related neural activity during RSVP as measured by means of magnetoencephalography have been related to impaired T2 report (Shapiro et al., 2006). Sustained reduction of brain activity during correct trials was also observed in a study using dense array electroencephalography (Keil and Heim, 2009). A group of alternative theoretical notions of the AB have emphasized non-trade-off aspects during RSVP target identification (Di Lollo et al., 2005; Olivers et al., 2007). For instance, work examining the so-called Lag 1 sparing effect showed that two or more targets may be reported at high accuracy, if delivered in a row, without intermittent distractor items present (Di Lollo et al., 2005), or if target-distractor-target sequences are presented within 100 ms (Potter et al., 2002). In the same vein, AB effects have been observed even in the absence of any distractors, with two targets separated by a blank interval (Nieuwenstein et al., 2009b). In addition, robust evidence exists to demonstrate that over-investment of resources into the stimulus (or distractor) stream of an RSVP task may lead to increased suppression of T2 accuracy, i.e., the AB effect (Olivers et al., 2007). A similar account states that repeated selection of a time segment in the stream results in suppression, delay, and diffusion of selective attention available for a second target (Vul et al., 2008). These theoretical perspectives on the AB highlight the need of temporal control of the attention system, when rapid stimuli are processed. Importantly, computational (Wyble et al., 2009a) and empirical evidence (Shapiro et al., 2006) suggest that such control can be altered by the participants’ strategic control. This latter property makes the AB an interesting target for developmental research in the context of academic achievement. Thus, although it is currently impossible for us to highlight one specific cognitive process reflected by the variability in the AB effect, there is good evidence that the AB is sensitive to the sequence of mental processes of interest, including attention selection and successful consolidation of attended information, despite distraction.
Concerning developmental studies with the AB design, 8- to 10-year-old children belonging to the upper half readers of their grade were reported to exhibit an overall superior accuracy in second target identification (McLean et al., 2009). Heim et al. (2006) observed two robust relationships between parameters of resource sharing and reading/spelling skills in fifth and sixth graders: First, the ability to optimize target processing at the cost of flexible resource sharing (i.e., a pronounced AB effect) predicted better performance in controlled language production tasks such as pseudoword reading. Second, the temporal capacity over time predicted automatized language processing such as reading/spelling of familiar words and sentences.
Several authors have addressed experimental questions related to developmental disorders. For instance, Mason et al. (2005) found that children with a diagnosis of attention-deficit/hyperactivity disorder showed an overall reduced performance during RSVP with letters and were more susceptible to distractors. In a similar vein, dyslexic individuals ranging in age from 10 to 15 years showed increased and prolonged impairment in a non-verbal AB task, compared to age-matched controls (Visser et al., 2004).
Taken together, there is mounting evidence that basic parameters of attentional resource sharing are related to more complex cognitive skills. Cognitive changes upon entering the academic environment are a major developmental milestone of higher-order intellectual functions. It is unclear however, if such dramatic change in cognitive performance is accompanied by changes in elemental building blocks of cognition such as attentional control. Primary curricula in many developed countries aim inter alia to establish a range of skills in areas, such as literacy and mathematics, the automatized execution of which enables more diverse and sophisticated secondary education (Hall et al., 2008). To explore this putative role, a cross-sectional design is desirable, which compares younger children, entering the academic environment, with older children after several years of basic education.
The present study used such a design in the context of the German education system. In Germany, one major milestone of compulsory education is related to entering first grade of primary school, when children are aged 6 or 7 years (different schools exist for children with special educational needs). Typically, primary school covers four grades. The next major milestone is associated with beginning (lower) secondary education, when most children are around ages 10 or 11 years. Lower-level secondary education includes grades 5 to 9/10 and prepares students for courses of education at upper secondary level, which is necessary for vocational or university entrance qualification (Secretariat of the Standing Conference of the Ministers of Education and Cultural Affairs of the Länder in the Federal Republic of Germany, 2010). Comparing these two groups of children enables us to investigate change in academic performance levels and mental aptitudes vis-à-vis specific parameters of attention and working memory, as they change between two important cognitive milestones.
Here, two specific aspects of attentional control over time were examined in German students of early primary and lower secondary level education: First, we quantified the amount of Lag 1 sparing as a measure of overall capacity for rapidly presented information. Second, we investigated the students’ sensitivity to disruptive intervening distractors when focusing on a set of target features, operationalized as the size of the AB effect. Under the assumption of general non-specific attentional maturation, both measures should show marked improvement in the older children. If attention development is characterized by multiple trajectories for specific sub-processes of attention control, then interactions of group and task measure would be expected. Overall, we predicted that younger children show a flat and linear profile for the AB task, on an overall lower performance level than older children. This would indicate less ability to control the allocation of cognitive resources over time, which paradoxically leads to lower sensitivity to distractors. With increased ability to control the selection and consolidation of target objects in a rapid stream, the AB effect is expected to be greater in older children. Such a difference suggests a developmental trend from a high-capacity, low control system in younger children, to more control at the cost of interference in the older group. In addition, we were interested in the relationship between indices of resource sharing and attentional capacity with higher-order measures of cognitive functioning such as general intelligence and digit span.
Materials and methods
Study Participants
Two groups of healthy participants volunteered in this study: 21 younger children (seven girls) aged between 6 and 7 years (M = 6.86 years, SD = 0.36) and 24 older children (eight girls) aged between 10 and 11 years (M = 10.79 years, SD = 0.41). Study groups attended early regular classes of primary (first grade) and secondary schools (fifth and sixth grade) in the catchment area of Konstanz and were native speakers of German. The gender ratio was a constant 2:1 in each age group. Although the excess of boys might have limited the external validity of the present findings, post hoc analyses did not reveal significant gender differences in terms of the AB and psychometric test performance (see Experimental and Psychometric Assessment; p-values varied between <0.210 and 0.988). This converged with studies in children involving lower male to female ratios, where sex effects were also absent in the AB, reading and spelling measures, or general cognitive factors (re-analysis of data presented in Heim et al., 2006; McLean et al., 2010).
None of the children were reported to suffer from developmental disorders (in particular language-based learning impairments and attention-deficit/hyperactivity disorder), any psychiatric or neurological diseases or taking medication that might affect central nervous system functioning. All children had normal or corrected-to-normal vision. Because stimuli in the AB paradigm were presented very rapidly, only seizure-free participants with a negative family history of epilepsy were examined. The protocol was approved by the institutional review board of the University of Konstanz. Written informed consent was obtained from the parents of the children prior to the experimental session; children gave their verbal assent. Each child received a shopping voucher or cinema ticket for participation.
Experimental and Psychometric Assessment
Children worked on four tasks: a non-verbal and a verbal AB task tapping into attentional capacity and resource sharing, as well as Raven’s Progressive Matrices (Heller et al., 1998; Bulheller and Häcker, 2002) and the Digit Span subtest of the German version of the Wechsler Intelligence Scale for Children (WISC III; Tewes et al., 1999) to assess general intellectual functioning. Being more challenging, the Matrices always were followed by Digit Span, and both tests were administered in between the two AB tasks, whose presentation order (non-verbal versus verbal) was counterbalanced among participants. This procedure allowed us to control for fatigue and interference imposed by the different task demands. Each child was tested individually in a quiet room. Including breaks, sessions lasted from 1.5 to 2 h.
Attentional blink experiment
In both AB tasks, stimuli appeared centrally on a black computer screen with a retrace rate of 60 Hz, at a distance of 50 cm from the observer. A script written using the Presentation software (Neurobehavioral Systems, Inc., Albany, CA, USA) controlled stimulus presentation and response registration. Task requirement involved identification of two green-colored target stimuli (T1 and T2) interspersed amidst a stream of white distractors, shown at a rate of 8.7 items per second. Observers were instructed to guess when unsure about the stimulus. No feedback was provided. Each item in the stream subtended a vertical visual angle of 0.82° and had a luminance of approximately 24.9 cd/m2. The rapid presentation rate was implemented by displaying each stimulus for 50 ms, followed by a blank screen for 66 ms.
Intertarget intervals varied to contain none, one, two, four, or seven intervening distractor stimuli (i.e., Lag 1, Lag 2, Lag 3, Lag 5, and Lag 8). Accordingly, stimulus-onset asynchronies (SOAs) were 116 ms (Lag 1), 232 ms (Lag 2), 348 ms (Lag 3), 580 ms (Lag 5), and 928 ms (Lag 8). To avoid anticipation of T1 (first target) occurrence, trials started with a randomized number of 5–25 distractor items. T2 was followed by 10 distractors. Presentation mode was pseudo-randomized, avoiding immediate repetitions of the same target as well as immediate repetitions of trials belonging to the same lag condition. Each AB version comprised 100 trials (20 trials per lag), which were equally divided into two blocks, allowing the child to take a short break. Prior to testing, at least three practice trials per task were administered to demonstrate the procedure and make sure that all children understood the task correctly. A schematic of an example trial for both the non-verbal and verbal AB paradigm is shown in Figure 1.
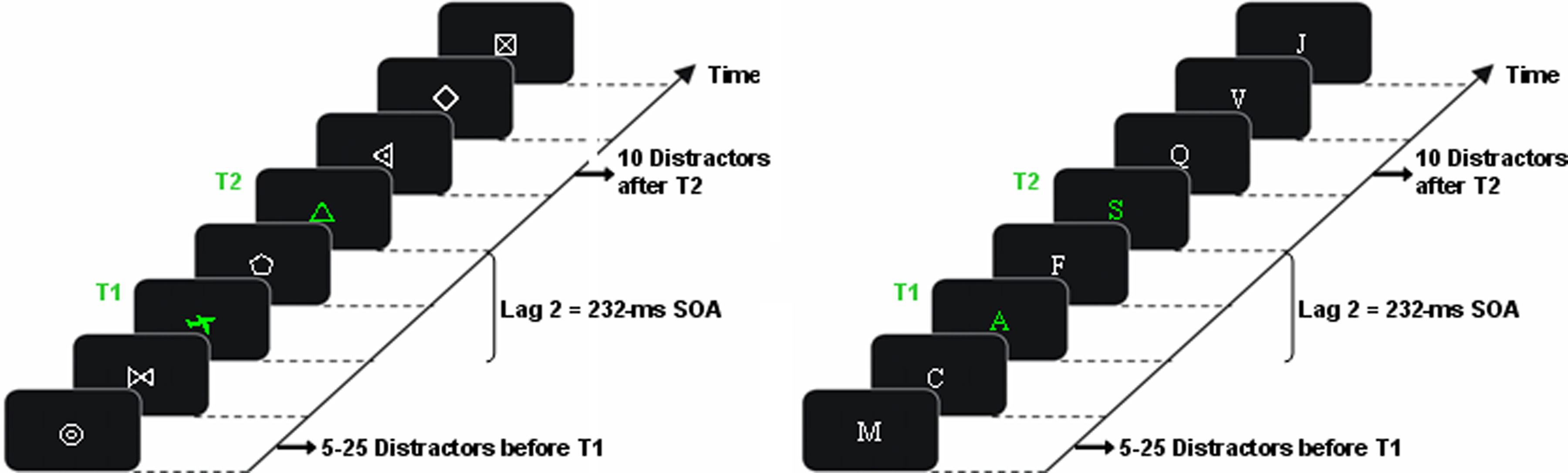
Figure 1. Schematic of the attentional blink design involving either non-linguistic symbols (left panel) or verbal stimuli (right panel). Rapid stimulus presentation was implemented by displaying each stimulus for 50 ms, followed by a blank screen for 66 ms, resulting in a stimulation frequency of 8.7 Hz. Children were asked to indicate the identity of two targets (T1 and T2) shown in green font amidst a series of white distractor items. In the symbol task (left), sketches of a car, airplane, or boat served as T1, and a circle, triangle, or square as T2; other shapes acted as distractors. Verbal stimulus sequences (right) were realized by letters of the alphabet in the younger group and simple nouns (not shown here) in the older group. Each of the present examples illustrates a trial with one intervening distractor between T1 and T2, i.e., Lag 2 with a stimulus-onset asynchrony (SOA) of 232 ms.
In terms of the (non-verbal) symbol task, T1 stimuli were sketches of means of transport (car, airplane, and boat) and T2s were geometric shapes (circle, triangle, and square), selected from the SPSS Marker Set (True Type). Twenty different geometric figures and shapes of the same typeface served as distractor items. All events were presented in 40-point Arial font. After the end of the rapid stimulus stream, participants indicate their response selection by mouse clicking the appropriate T1 and T2, all of which consecutively appear among three alternatives. They initiated the next trial with an additional mouse click.
As to the verbal task, stimuli were selected according to the academic achievement of primary and secondary students. In order to maximize familiarity with the stimulus set, older participants dealt with simple nouns, drawn from prevailing fourth-grade reading books, and younger participants worked on letters of the alphabet. Thus, we used stimuli that the children in each age group knew very well. Knowledge of the target items was also tested by having the child read aloud the list of letters or words once in a fluent manner at the beginning of the experimental session. All children successfully completed this initial test.
In the younger group, 10 selected letters (A, B, K, L, N, O, S, T, W, and Z) served as targets and the remaining 16 letters as distractors (C, D, E, F, G, H, I, J, M, P, Q, R, U, V, X, and Y). Items were presented in capitals, using 36-point Times New Roman font. In the older group, 11 out of 20 target words were monosyllabic (e.g., Dorf = village, Mund = mouth), and nine disyllabic (e.g., Foto = photo, König = king). Their lemma-frequency varied between 216 and 1137 per one million words in the CELEX database (Baayen et al., 1995), thus being considered high-frequent (Mohr et al., 2001). The distractor set (n = 60) included 39 two-syllable words (e.g., Fenster = window, Sommer = summer) and 21 three-syllable exemplars (e.g., Kartoffel = potato, Nachmittag = afternoon). Having a mean length of 7.25 letters, distractors were longer than target items, comprising on average 4.65 letters, which enabled sufficient masking of the targets (Anderson and Phelps, 2001). As is customary for all true nouns in the German language, words were presented with capital initial letters. A Times New Roman font, point-size 28 was used. At the end of each trial, children reported aloud the green letters or words they had seen, which in turn were recorded by the experimenter. After response completion, participants started the next trial, using the control key of the computer keyboard.
In both AB tasks, target identification was expressed as the percentage of correct responses for each of the five lags. Only trials with a correct T1 report were considered for determining T2 accuracy.
Psychometric tests
Younger children received the colored form of Raven’s Progressive Matrices (CPM), designed to assess the capability to form perceptual relations and to reason abstractly from non-verbal stimuli. The CPM includes three sets of 12 colored stimulus designs, for a total of 36 problems, arranged in order of increasing difficulty. Each problem involves the completion of continuous or discrete patterns by choosing the correct missing part from among six response options. Since the CPM spreads out the scores of the bottom 20 percent of the general population (Bulheller and Häcker, 2002), it is less appropriate to use them for representative children of 8 years and above. Older students thus completed the standard form of the Matrices (SPM), consisting of five sets of 12 black-and-white stimulus designs, for a total of 60 items. Test instructions to the study participants were given in accordance with the guidelines presented in the CPM and SPM manuals. The children indicated their response (by pointing to the selected alternative, often enhanced by naming its number) to the experimenter, who filled in the response sheet. We converted the number of correct items via age-appropriate percentile ranks to T-scores (mean = 50, SD = 10), subsequently used for statistical comparison of the younger and older group. Because standard values intrinsically even out developmental differences, Raven raw scores were preferred to examine the relationship with experimental indices of resource sharing and attentional capacity. Raw scores of the CPM were therefore transformed to SPM equivalents, using a table established by Andrich and Dawes (Bulheller and Häcker, 2002).
The procedure of the Digit Span subtest was performed as suggested in the manual of the WISC III. Participants listened to the experimenter reading lists of digits of increasing length, with a rate of one item per second and were asked to immediately repeat each list back in order (forward digit span). Two series of each length were presented, starting with two digits up to a maximum of nine. The task was terminated when the child failed to repeat both series of the same digit length. In a second condition, digit strings were recalled in reverse order (backward digit span), beginning with a digit length of 2 up to a maximum length of 8. The criterion to stop the procedure was the same as in the forward condition. To increase the sensitivity of the digit span measures to small performance differences (e.g., in items solved on each level), a mean span for both forward and backward conditions was calculated across item lists for each student in the following manner: In the forward condition, we linearly transformed the results for each item list (binary variable: solved/not solved) to a scale with a maximum span of nine digits (see above). Because the first two item lists (digit length 2 and 3) have low difficulty and low discriminative value, they were weighted with a factor of 0.5, whereas subsequent item lists (digit length 4–9) were weighted with 1. In a similar manner, performance in the backward condition was linearly transformed onto a scale with a maximum span of eight digits. Here, already digit lists from a length of 3 were weighted with the higher factor of 1, reflecting the higher complexity (auditory sequencing, temporary storage plus mental manipulation) of the backward task (e.g., Jensen, 1980; Gardner, 1981; Reynolds, 1997).
Statistical Analyses
In both AB tasks, the percentages of correct responses on T1 and conditional T2 were evaluated separately in mixed design analysis of variance (ANOVA), crossing the between-subjects factor Group (2; younger, older) and the within-subjects factor Lag (5; 1 = 116-ms SOA, 2 = 232-ms SOA, 3 = 348-ms SOA, 5 = 580-ms SOA, 8 = 928-ms SOA). T2 report was considered correct only on trials with accurate T1 identification (T2|T1 accuracy). This is generally assumed to emphasize specific effects of limited resources across the two targets (Raymond et al., 1992). Contrast analyses were used to follow up significant ANOVA results.
To assess group differences in regard to the AB profiles in greater detail, Pearson product–moment correlations were calculated between participants’ age and two difference measures extracted from conditional T2 responses (see below). Because chronological age (in months) shows a bimodal distribution across the two study groups, we rank transformed this variable with the smallest age value assigned rank one. Mean ranking was used in the case of ties. In both the symbol and verbal task, two difference measures (termed E and L) of mean T2 identification contingent upon T1 report at the following lags were considered: Esymbol or verbal = ΔT2|T1 (Lag 1–Lag 2) and Lsymbol or verbal = ΔT2|T1 (Lag 8–Lag 2). Value E from the first equation reflects early competition between T1 and T2, being positive when Lag 1 sparing is observed, and negative when the T2 presented at Lag 2 is better identified than the T2 at Lag 1. Thus, a positive E indicates intertarget interference at Lag 2, often taken as a consequence of sharing costs induced by successful T1 identification (Shapiro et al., 2006; Keil and Heim, 2009). L characterizes the difference between T2 report at the late Lag 8 beyond the AB interval, where accuracy was high and interference low, and performance at the interference-sensitive Lag 2. We used this difference measure as an index of performance range, with small values indicating minor differences between early and late interference, and high values indicating poor performance in the AB window, and effective recovery with longer intertarget intervals.
Lag conditions entering the difference measures were selected both on the basis of earlier AB work with children (Heim et al., 2006) and ANOVA results obtained in the current study. ANOVA interactions revealed reliable differences between groups for the Lag 1/Lag 2 gradient and for Lag 8 (see Performance in the Attentional Blink Experiment). Using the same lag differences for the younger and older students imply that both phenomena (i.e., Lag 1 sparing and the AB) can be indexed in either group at the same lags. This assumption would for instance be invalid if the younger children showed a pronounced blink at a later time than the older children. To examine if such artifacts of lag selection are possible in our analysis, we plotted the E (L) difference measure against alternative difference scores formed between Lags 1 versus 3 and Lags 2 versus 3 (Lags 3 versus 8 and Lags 5 versus 8), searching for significant group disparities of the sparing (AB) pattern as a function of lag choice. We did not find systematically changing differences between the age groups as we swapped lags entering the difference scores. It is conceivable that inclusion of a longer lag, such as Lag 10 or 12 would have led to better recovery in the younger participants, potentially qualitatively changing the correlation patterns observed here, but such an assumption cannot be tested with the present data.
E and L values inherent in the symbol and verbal AB profiles described any correlational relationships with Raven Matrices raw scores and Digit Spans. To complete the picture of the current cross-sectional approach, Pearson correlations were run between the psychometric scores and rank-transformed age variable. For all analyses, results were deemed significant when p < 0.05.
Results
Performance in the Attentional Blink Experiment
As illustrated in Figure 2A, older participants were generally more accurate in identifying T1 symbols than the younger children, F(1,43) = 13.85, p < 0.001. This was also true for the verbal task, F(1,43) = 19.91, p < 0.001 (Figure 2B). In addition, report of verbal T1s was lag-dependent, F(4,172) = 33.06, p < 0.001, with lowest accuracy at Lag 1. A linear trend analysis on the Lag × Group interaction, F(4,172) = 3.30, p < 0.05, pointed to a significant difference in the steepness of the T1 gradients, indicating that relative impairment in first target identification at Lag 1 was more pronounced for the younger students, F(1,43) = 10.73, p < 0.01.
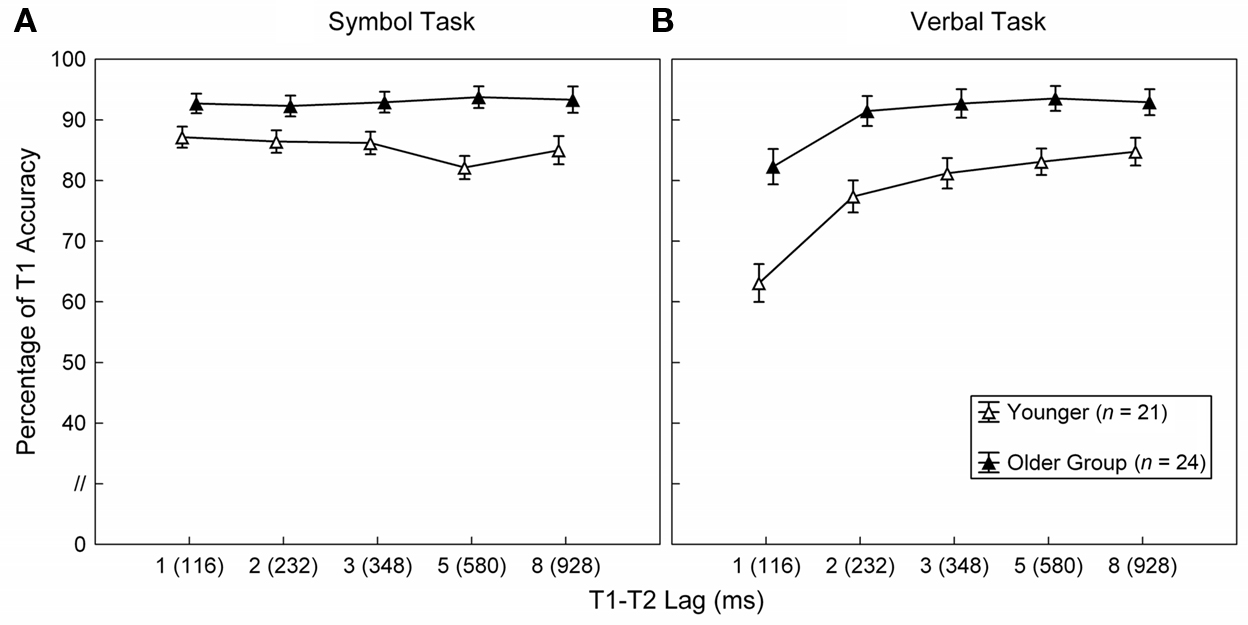
Figure 2. Percentage of accurate first target (T1) report at each intertarget interval (T1–T2 lag) of the symbol and verbal tasks (A and B, respectively). Values represent means of 21 younger children (open triangles) and 24 older children (filled triangles). Vertical bars indicate SE of mean.
Figure 3A depicts the mean percentage of accurate T2 report contingent on the correctly identified T1 in the symbol task at each lag for the two participant groups. Older participants outperformed the younger overall, F(1,43) = 49.01, p < 0.001. Furthermore, Group membership interacted with Lag, F(4,172) = 3.86, p < 0.01: while the younger children linearly increased T2 report with increasing lag, F(1,43) = 29.43, p < 0.001, the older children exhibited a quadratic pattern, F(1,43) = 21.50, p < 0.001. Planned comparisons confirmed significant group differences at two positions in the non-verbal AB profile: first, only older participants showed sparing of T2 report (∼16%) at Lag 1 versus Lag 2, F(1,43) = 10.18, p < 0.01; second, the decrease in performance by the AB effect (Lag 2) relative to T2 symbol identification at the latest intertarget interval (Lag 8) was larger in the older (∼32%) than in the younger students (∼16%), F(1,43) = 9.03, p < 0.01.
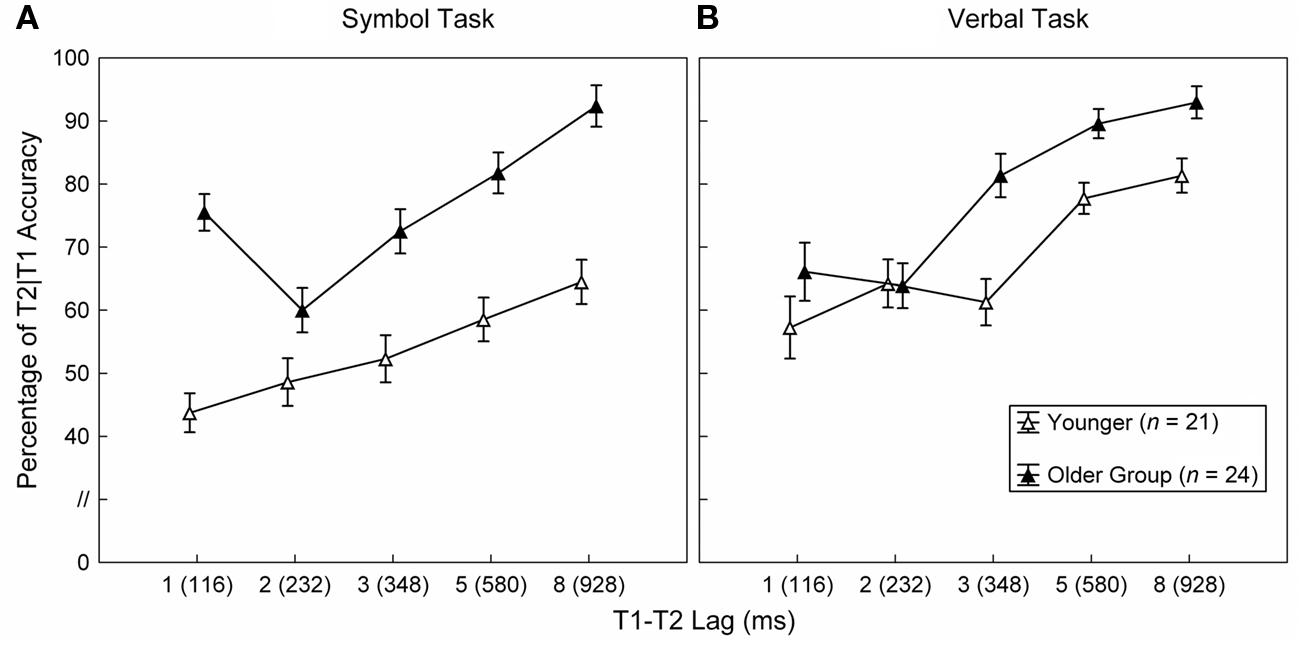
Figure 3. Percentage of accurate second target (T2) report given first target (T1) identification at five T1–T2 lags of the symbol and verbal tasks (A and B, respectively). Values represent means of 21 younger children (open triangles) and 24 older children (filled triangles). Vertical bars indicate SE of mean.
In the verbal task, conditional T2 identification was again less accurate in the younger observers than in the older, F(1,43) = 8.62, p < 0.01 (Figure 3B). Linear trend analyses on the Lag × Group interaction, F(4,172) = 3.54, p < 0.01, indicated that verbal T2 performance increased from early to late lags in both the younger and older children, Fs(1,43) = 24.62 and 46.66, ps < 0.001. Group differences, however, emerged when conducting focused contrasts on Lag 8 versus Lag 2, F(1,43) = 5.17, p < 0.05, and Lag 8 versus Lag 3, F(1,43) = 4.55, p < 0.05). Similar to the symbol task, the impairment in reporting verbal T2 identity at Lag 2 compared to those at Lag 8 was greater in the older (∼29%) than in the younger group (∼17%; Figure 3B). The opposite pattern occurred for the latter contrast, showing a more pronounced decline for the younger children (∼20 versus ∼12% in the older).
Psychometric Test Performance
Participants performed in the average or above-average age range on Raven’s Progressive Matrices, with T-scores varying between 43 and 78. Non-verbal intellectual functioning (M ± SEM) of the younger (55.86 ± 1.80) and older group (54.29 ± 1.64) did not differ significantly, t(43) = 0.65, p > 0.5. ANOVA on digit span scores using Recall Condition (2; forward, backward) as within-subjects factor and Group (2; younger, older) as between-subjects factor showed significant main effects: both groups recalled longer digit lists forward than backward, F(1,43) = 24.97, p < 0.001, but older children outperformed the younger overall, F(1,43) = 12.77, p < 0.001. Mean forward and backward spans were 3.11 (SEM = 0.29) and 2.29 (SEM = 0.16) for the younger group; respective values in the older group amounted to 4.37 (SEM = 0.26) and 3.23 (SEM = 0.29).
Age-Related Correlations of Attentional and Psychometric Assessment Scores
Pearson product–moment correlations of the rank-transformed age variable, attentional parameters, and psychometric assessment scores across the entire study sample are listed in Table 1.
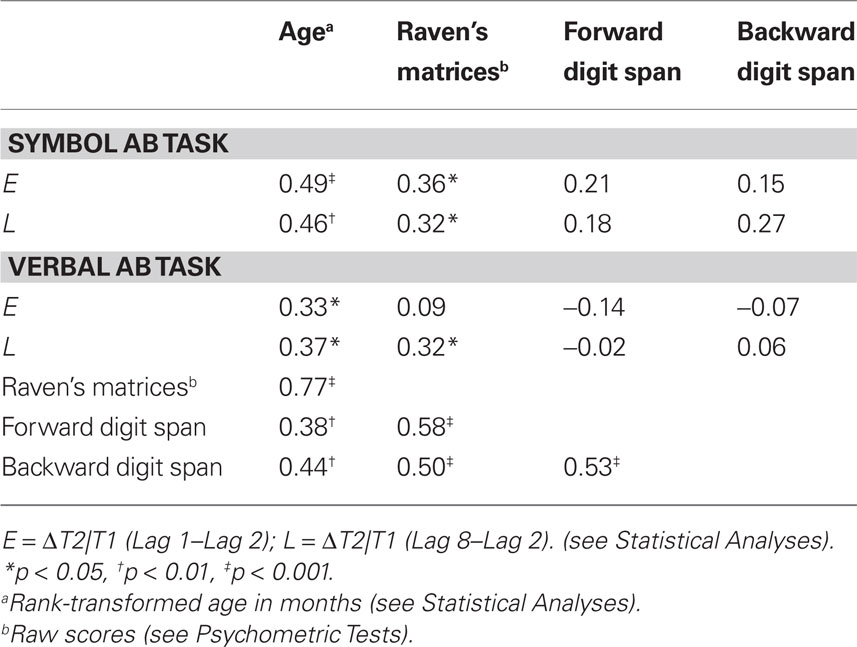
Table 1. Pearson product–moment correlations (r) of age, difference measures E and L inherent in the AB profile, and psychometric assessment variables in the study sample (n = 45).
Children’s age was significantly correlated with the difference measure E of both the symbol and verbal AB profiles. As depicted in Figure 4, older children tended to exhibit more positive E values, indicating that the report of two targets presented at Lag 1 is superior to the report at Lag 2 (i.e., Lag 1 sparing). Furthermore, the older the students, the more positive L values for the two AB tasks were observed (Figure 5). This suggests that an increase in age was accompanied by impaired performance in the AB window (Lag 2) and effective recovery when T2 followed T1 at greater temporal distance (Lag 8).
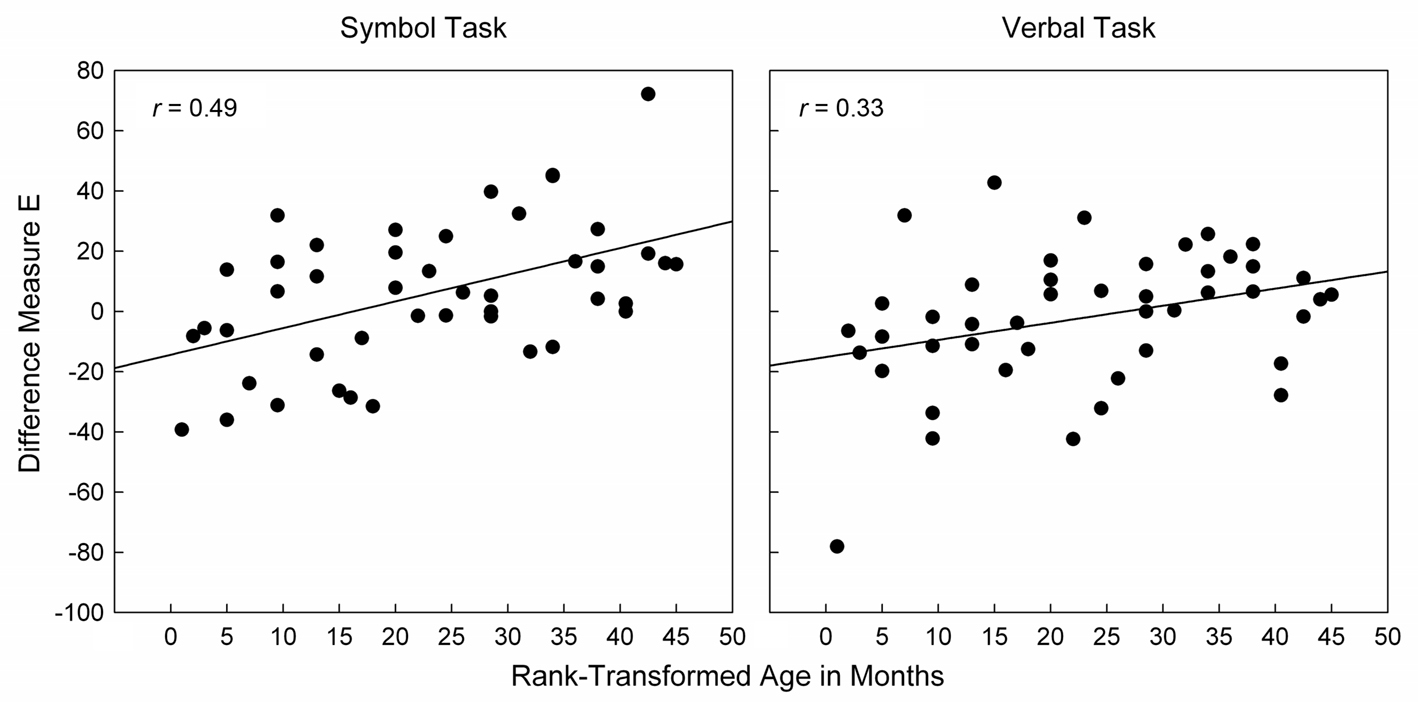
Figure 4. Pearson product–moment correlations (r) between the rank-transformed age variable and difference measure E = ΔT2. T1 (Lag 1–Lag 2) extracted from conditional T2 responses of the symbol and verbal tasks across the entire sample of 45 children.
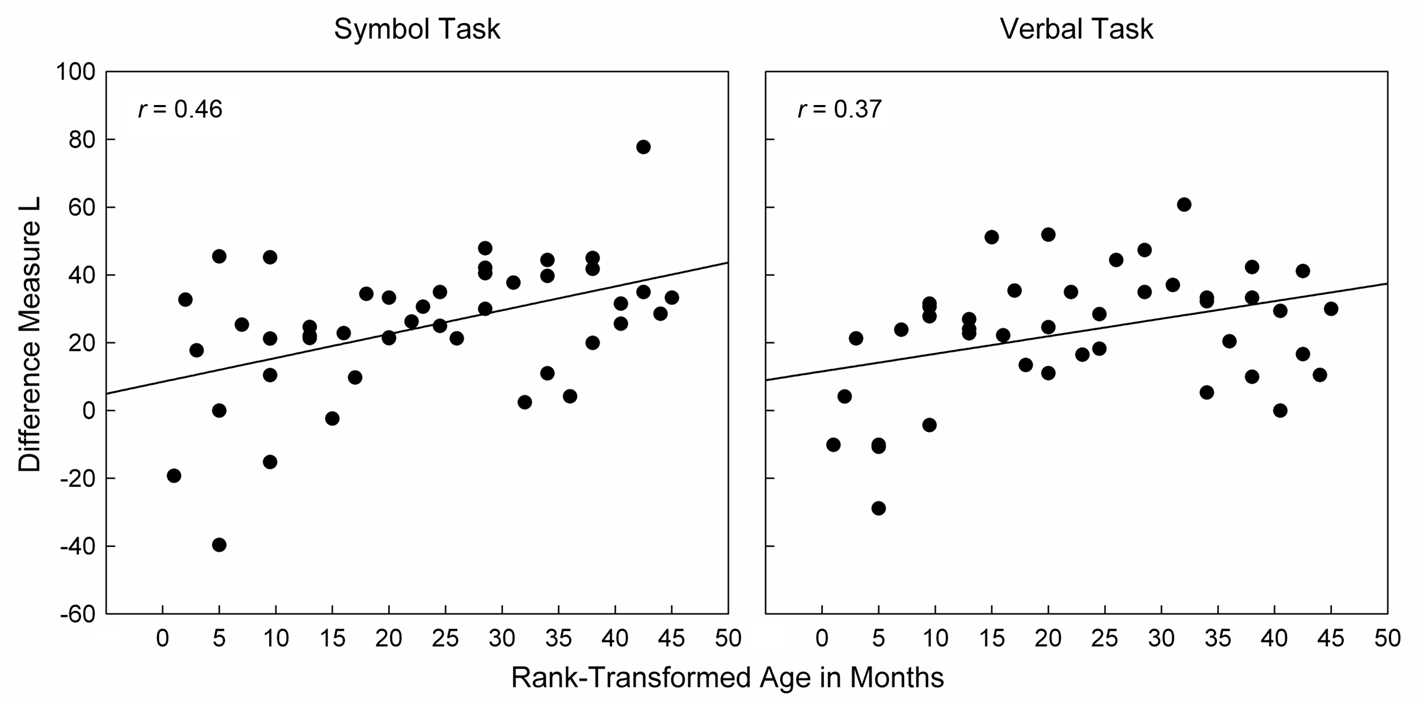
Figure 5. Pearson product–moment correlations (r) between the rank-transformed age variable and difference measure L = ΔT2. T1 (Lag 8–Lag 2) extracted from conditional T2 responses of the symbol and verbal tasks across the entire sample of 45 children.
Participants’ age was also statistically related to psychometric test performance (Table 1). Unsurprisingly, younger children tended to achieve lower raw scores in Raven’s Progressive Matrices and to recall shorter digit lists forward and backward.
Correlations of Attentional Parameters and Psychometric Test Scores
As is apparent from Table 1, the relative amount of Lag 1 sparing (difference measure E) in the symbol AB task correlated positively to the accuracy in abstract reasoning (Matrices raw scores). This was not the case for the verbal E. Greater conditional T2 impairment at Lag 2 or a more positive L value, however, was associated with higher Matrices scores for both AB tasks. In contrast, individual differences in E and L measures did not show significant correlations with forward and backward digit spans (Table 1).
Because indices of memory span were positively linked to Matrices scores and all psychometric variables systematically varied with children’s age, the observed relationships were subsequently explored by using partial correlations, controlled for chronological age in months. Correlations between Matrices and digit span forward (r = 0.43, p < 0.01) and backward (r = 0.30, p < 0.05) still reached the alpha level of 5%. In contrast, none of the associations with E and L difference measures remained significant (range: r = −0.19 to r = 0.07, all ps > 0.2), indicating that the AB profile is related to age, but not to developmental change in terms of non-verbal intellectual capacity.
Discussion
The present cross-sectional study set out to explore developmental differences in the AB paradigm and its relationship with mental aptitudes in German first-grade school children, compared to students in early secondary education (grades 5 and 6). Working on two AB tasks including either non-linguistic symbols or verbal stimuli, the younger group was outperformed by the older overall. The analyses focused on T2 accuracy contingent upon correct T1 report. In the symbol version, older children showed a hook-shaped T2 identification pattern as found in numerous AB studies in adults (Visser et al., 1999). Conditional T2 report was highest at Lag 8 (928-ms SOA) and decreased linearly with shorter intervals, having its minimum at Lag 2 (232-ms SOA) as expected. At the earliest intertarget interval (Lag 1, 116-ms SOA) accuracy increased by about 16%, indexing Lag 1 sparing (see Figure 3A). In contrast, younger students did not exhibit Lag 1 sparing relative to Lag 2. They scored lowest when the second target symbol followed immediately the first and highest when the target doublet was separated by a maximum of seven distractors, reflecting a linear trend over lags (Figure 3A). For both participant groups, accurate report of T1 symbols did not vary as a function of the temporal display position (see Figure 2A).
In the verbal AB task, older children again demonstrated a prominent drop in conditional T2 identification at Lag 2 relative to those at Lag 8. Although there was no significant difference in accuracy between the second and first intertarget interval, means suggested that some sparing at Lag 1 emerged in their performance pattern (see Figure 3B). In the younger group, verbal T2s were subject to a more alleviated and temporally diffuse impairment in report for the early Lags 1–3 (Figure 3B). Relative to accuracy scores at the longest interval, first graders showed a lesser decrement at Lag 2 than students attending secondary school. However, relative T2 identification remained similarly mitigated at Lag 3 in the younger individuals, while the older already exhibited considerable relief. In both groups, analysis of performance of the preceding verbal T1 revealed accuracy losses at Lag 1 (see Figure 2B), which were more pronounced for the younger children. In terms of our hypotheses, this pattern of findings suggests that younger children have overall less capacity for processing rapidly presented visual information than the older students. In the older group however, greater capacity for a specific set of target features comes at the cost of higher sensitivity to intervening distractors or to a second target.
The developmental trajectories of the T2|T1 performance patterns in the symbol and verbal tasks were also confirmed by correlational analyses of children’s age with two difference measures extracted from the AB profiles. Greater relative amounts of Lag 1 sparing (measure E) and T2 impairment at Lag 2 (measure L) were related to an advanced age in the study sample. This was most prominent when children were asked to identify symbols in the RSVP paradigm (see Figures 4 and 5).
Differences between the two AB tasks are apparent: whereas Lag 1 sparing was seen in the symbol task, a more linear pattern was evinced in the letter/word versions. Several factors may contribute to the discrepancy. First, the chance level of correct responses was substantially higher in the symbol task, in which one of three symbols was a target in a given trial, for T1 and T2 respectively. Second, T1 and T2 belonged to predictable and distinct categories (T1, means of transportation and T2, geometric shapes) in the symbol, but not the verbal task. According to recent theoretical work (Wyble et al., 2009a), such categorical change may facilitate the separation of the two targets that enter attentive processing together and thus are subject to merging or to temporal confusion errors (Potter et al., 2002). Because participants know the categories a priori and because both categories are distinct from the distractors, T1–T2 pairs in the symbol task may trigger temporally circumscribed increases in attention (Wyble et al., 2009b), which may help distinguish pairs that are especially close in temporal proximity (Craston et al., 2009). Third, earlier research has suggested that AB suppression during RSVP may be seen even in the absence of distractor items, if the T2 task is difficult, and if a gap is present between T1 and T2 (Nieuwenstein et al., 2009a). Because our design included a 66-ms temporal gap between items, one would be led to predict that the more difficult verbal task should show less Lag 1 sparing than the symbol version. This prediction is in line with the findings of our study (see Figure 3).
As a methodological concern, it is important to note that although groups digressed in their overall performance, any effects of Lag are likely to be related to interindividual differences in attentional control, rather than to overall performance differences. Because we used conditional T2 accuracy, taking into account only trials with correct T1 report, it seems unlikely that younger students’ difficulty with rapid tasks in general has led to the present pattern of results. Only systematic and extremely large T1 differences would distort the T2|T1 findings, and such differences were not evident in the current data set (see Figure 2).
As outlined in the introduction, several AB models highlight the role of engaging and disengaging attention within short epochs of time (Vul et al., 2008). Developmental models of attentional control based on the theory proposed by Posner and colleagues (e.g., Posner and Rothbart, 1998) emphasize the development of executive control as one key aspect of attention, which strongly involves facets of inhibition and strategic control of resources. Experimental work with tasks that are sensitive to interference and distraction effects has suggested that performance profiles show substantial change within the age range examined in the current study (Rueda et al., 2004), and that executive attention predicts other aspects of self-regulation and well-being later in life (Rueda et al., 2005a).
Our data are compatible with most of these perspectives and cannot speak as to their validity. Rather, it is interesting to see how the current developmental data can be informed by conceptual work on the AB mechanism. The AB paradigm represents a potential avenue to examining time dynamics of attentional engagement and disengagement, as well as working memory consolidation together in one task. Specifically, the AB may capture features of competition between attended events ( Keil et al., 2006; Ihssen et al., 2007). It is also sensitive to the strategic distribution of resources across multiple stimuli and time periods, intentional, or non-intentional (Shapiro et al., 2006). Thus, the present data are relevant for our understanding of how and when individuals are capable of applying strategies to the deployment of cognitive resources over time, during competition. Here, we found strong evidence for costs associated with correctly identifying T1 in older children, particularly in the Lag 2 condition, which is most susceptible to the interference induced by the combination of the T1 and the T1 + 1 distractor. Younger children did not show such a specific profile and also demonstrated less accuracy for the T1 overall. This is in line with the finding that younger children evinced greater trade-off between T1 and T2 accuracy at Lag 1 than older children. The latter group showed small cost effects of correct T2 processing on T1 and vice versa, whereas the younger students displayed a performance decrease specifically at Lag 1 for the T1, suggesting that there was a linear cost of processing speed, which was stronger than the Lag 2 decrease seen in older children and adults.
In light of theoretical views of the AB, this pattern of findings points to several important aspects of attention development during childhood. Impairment for T2 stimuli during the AB time window has been attributed to decreased availability of cognitive resources (e.g., Chun and Potter, 1995; Jolicoeur et al., 2006) or attentional capacity (Vul et al., 2008), which is assumed to be a result of effortful encoding/selection of the T1 item. From this perspective, over-allocation of resources to T1 is associated with a lack of resources available for the second target in a trade-off fashion. Overspending resources to the T1 then prevents T2 from being transformed into a durable and reportable working memory representation. Neurophysiological research on the AB in adults has supported this notion, showing that trials with correct T2 responses are characterized by relative reduction in the resource allocation to the first target, compared to incorrect T2 trials (Shapiro et al., 2006). A complementary interpretation may focus on the temporal sequence of attention episodes in the current task: as students acquire more control over the time course of attention deployment, they become more susceptible to the AB deficit, while showing improvement for the T1 stimulus across lags. It is therefore interesting to consider whether these changes are part of a general trajectory of cognitive functioning.
Results of the psychometric tests served as measures to parameterize variations in cognitive abilities. On the non-verbal CPM/SPM, all of the participants yielded standardized scores appropriate for their chronological age, and these scores did not differ between primary and secondary students. Consistent with the memory span literature (e.g., Gardner, 1981; Brocki and Bohlin, 2004), both groups recalled more digits forward than backward, with generally higher performance for the older group. The two indices of memory span were positively linked to Matrices raw scores in the whole sample of children (see Table 1). This is a typical finding given that the immediate serial recall of digits is part of several standardized tests of general intelligence. From a theoretical perspective, the Digit Span scale and Raven’s Progressive Matrices can be arrayed on a continuum of apparent processing complexity (based on their variance accounted for by general ability, G) with the former falling toward the border from simple to intermediate complexity and the latter in the complex range (Marshalek et al., 1983). Correlation analyses between these psychometric measures and attentional parameters (Table 1) seemed to suggest that higher achievement in the Matrices was accompanied by Lag 1 sparing when non-linguistic targets have to be identified. Additionally, larger AB impairment and greater resistance to interference at the latest temporal lag in both the symbol and verbal task were associated with superior scores in the Matrices. Such relationships were not evident for the forward and backward spans. Because Matrices raw scores increased with participants’ age, partial correlations were conducted to more closely examine the role of age versus general intellectual ability in attentional resource sharing and capacity. These analyses showed consistently that general ability did not manifest in linear relationships with measures of attention after chronological age was controlled for. Importantly, Matrices raw scores and digit span results still shared a significant amount of variance despite the procedure under consideration. Taken together, the findings imply that changes in parameters of attention inherent to the AB profile were linked to age-related development.
Considering the correlational and experimental results of the present study, we suggest that the ability to allocate mental resources to multiple events under competition emerges as a cognitive skill, independent of general intellectual functions. Because resource sharing across multiple targets has been shown to be associated with other cognitive skills, namely reading and spelling (Heim et al., 2006), future work may aim to clarify a potential causal role of resource sharing capacity for academic achievement.
Conflict of Interest Statement
The authors declare that the research was conducted in the absence of any commercial or financial relationships that could be construed as a potential conflict of interest.
Acknowledgments
This work was supported by grants from the German Research Foundation (Deutsche Forschungsgemeinschaft) and by the National Institute of Mental Health (R01 MH084932). We would like to thank Ina Wäldin for help in data acquisition and Niklas Ihssen for technical support. We are grateful for comments provided by Forrest Gruss on an earlier version of this manuscript. Special thanks to the children, who volunteered their time to participate in this research, as well as to the parents for their commitment.
References
Anderson, A. K., and Phelps, E. A. (2001). Lesions of the human amygdala impair enhanced perception of emotionally salient events. Nature 411, 305–309.
Baayen, R. H., Piepenbrock, R., and Gulikers, L. (1995). The CELEX Lexical Database (Version 2.0) [CD-ROM]. Philadelphia: Linguistic Data Consortium, University of Pennsylvania.
Brocki, K. C., and Bohlin, G. (2004). Executive functions in children aged 6 to 13: a dimensional and developmental study. Dev. Neuropsychol. 26, 571–593.
Bulheller, S., and Häcker, H. (2002). Raven’s Progressive Matrices (CPM) und Vocabulary Scales, German Adaptation. Frankfurt: Swets and Zeitlinger.
Chun, M. M., and Potter, M. C. (1995). A two-stage model for multiple target detection in rapid serial visual presentation. J. Exp. Psychol. Hum. Percept. Perform. 21, 109–127.
Cowan, N., Naveh-Benjamin, M., Kilb, A., and Saults, J. S. (2006). Life-span development of visual working memory: when is feature binding difficult? Dev. Psychol. 42, 1089–1102.
Craston, P., Wyble, B., Chennu, S., and Bowman, H. (2009). The attentional blink reveals serial working memory encoding: evidence from virtual and human event-related potentials. J. Cogn. Neurosci. 21, 550–566.
Di Lollo, V., Kawahara, J., Shahab Ghorashi, S. M., and Enns, J. T. (2005). The attentional blink: resource depletion or temporary loss of control? Psychol. Res. 69, 191–200.
Enns, J. T., and Girgus, J. S. (1985). Developmental changes in selective and integrative visual attention. J. Exp. Child. Psychol. 40, 319–337.
Gardner, R. A. (1981). Digits forward and digits backward as two separate tests: normative data on 1567 school children. J. Clin. Child Adolesc. Psychol. 10, 131–135.
Hall, K., Murphy, P., and Soler, J. (2008). Pedagogy and Practice: Culture and Identities. London: Sage.
Heim, S., Keil, A., and Ihssen, N. (2006). Der Zusammenhang zwischen zeitlicher Aufmerksamkeitsallokation und Lese-Rechtschreibleistungen im frühen Sekundarschulalter (The Relationship between Temporal Attention and Literacy Skills in Classroom Children). Z. Psychol. 214, 196–206.
Heller, K. A., Kratzmeier, H., and Lengfelder, A. (1998). Raven-Matrizen-Test, Standard Progressive Matrices (SPM), German Adaptation. Göttingen: Beltz.
Ihssen, N., Heim, S., and Keil, A. (2007). The costs of emotional attention: affective processing inhibits subsequent lexico-semantic analysis. J. Cogn. Neurosci. 19, 1932–1949.
Jolicoeur, P., Sessa, P., Dell’Acqua, R., and Robitaille, N. (2006). On the control of visual spatial attention: evidence from human electrophysiology. Psychol. Res. 70, 414–424.
Keil, A., and Heim, S. (2009). Prolonged reduction of electrocortical activity predicts correct performance during rapid serial visual processing. Psychophysiology 46, 718–725.
Keil, A., Ihssen, N., and Heim, S. (2006). Early cortical facilitation for emotionally arousing targets during the attentional blink. BMC Biol. 4, 23. doi: 10.1186/1741-7007-4-23
Marshalek, B., Lohman, D. F., and Snow, R. E. (1983). The complexity continuum in the radex and hierarchical models of intelligence. Intelligence 7, 107–127.
Mason, D. J., Humphreys, G. W., and Kent, L. (2005). Insights into the control of attentional set in ADHD using the attentional blink paradigm. J. Child Psychol. Psychiatry 46, 1345–1353.
McLean, G. M. T., Castles, A., Coltheart, V., and Stuart, G. W. (2010). No evidence for a prolonged attentional blink in developmental dyslexia. Cortex 46, 1317–1329.
McLean, G. M. T., Stuart, G. W., Visser, T. A. W., and Castles, A. (2009). The attentional blink in developing readers. Sci. Stud. Read. 13, 334–357.
Mohr, B., Heim, S., Pulvermüller, F., and Rockstroh, B. (2001). Functional asymmetry in schizophrenic patients during auditory speech processing. Schizophr. Res. 52, 69–78.
Nieuwenstein, M., Van der Burg, E., Theeuwes, J., Wyble, B., and Potter, M. (2009a). Temporal constraints on conscious vision: on the ubiquitous nature of the attentional blink. J. Vis. 9, 18.1–18.14.
Nieuwenstein, M. R., Potter, M. C., and Theeuwes, J. (2009b). Unmasking the attentional blink. J. Exp. Psychol. Hum. Percept. Perform. 35, 159–169.
Olivers, C. N., van der Stigchel, S., and Hulleman, J. (2007). Spreading the sparing: against a limited-capacity account of the attentional blink. Psychol. Res. 71, 126–139.
Posner, M. I., and Rothbart, M. K. (1998). Attention, self-regulation and consciousness. Philos. Trans. R. Soc. Lond. B Biol. Sci. 353, 1915–1927.
Potter, M. C., Staub, A., and O’Connor, D. H. (2002). The time course of competition for attention: attention is initially labile. J. Exp. Psychol. Hum. Percept. Perform. 28, 1149–1162.
Raymond, J. E. (2003). New objects, not new features, trigger the attentional blink. Psychol. Sci. 14, 54–59.
Raymond, J. E., Shapiro, K. L., and Arnell, K. M. (1992). Temporary suppression of visual processing in an RSVP task: an attentional blink? J. Exp. Psychol. Hum. Percept. Perform. 18, 849–860.
Reynolds, C. R. (1997). Forward and backward memory span should not be combined for clinical analysis. Arch. Clin. Neuropsychol. 12, 29–40.
Ridderinkhof, K. R., and van der Stelt, O. (2000). Attention and selection in the growing child: views derived from developmental psychophysiology. Biol. Psychol. 54, 55–106.
Rueda, M. R., Fan, J., McCandliss, B. D., Halparin, J. D., Gruber, D. B., Lercari, L. P., and Posner, M. I. (2004). Development of attentional networks in childhood. Neuropsychologia 42, 1029–1040.
Rueda, M. R., Posner, M. I., and Rothbart, M. K. (2005a). The development of executive attention: contributions to the emergence of self-regulation. Dev. Neuropsychol. 28, 573–594.
Rueda, M. R., Rothbart, M. K., McCandliss, B. D., Saccomanno, L., and Posner, M. I. (2005b). Training, maturation, and genetic influences on the development of executive attention. Proc. Natl. Acad. Sci. U.S.A. 102, 14931–14936.
Secretariat of the Standing Conference of the Ministers of Education and Cultural Affairs of the Länder in the Federal Republic of Germany. (2010). The Education System in the Federal Republic of Germany 2008. Available at: http://www.bildungsserver.de/innovationsportal/zeigen_e.html?seite=6066
Shapiro, K. L., Schmitz, F., Martens, S., Hommel, B., and Schnitzler, A. (2006). Resource sharing in the attentional blink. Neuroreport 17, 163–166.
Stevens, C., Fanning, J., Coch, D., Sanders, L., and Neville, H. (2008). Neural mechanisms of selective auditory attention are enhanced by computerized training: electrophysiological evidence from language-impaired and typically developing children. Brain Res. 1205, 55–69.
Tewes, U., Rossmann, P., and Schallberger, U. (1999). Hamburg Wechsler Intelligenztest für Kinder – Dritte Version, HAWIK-III. Bern: Huber.
Visser, T. A. W., Bischof, W. F., and Di Lollo, V. (1999). Attentional switching in spatial and nonspatial domains: evidence from the attentional blink. Psychol. Bull. 125, 458–469.
Visser, T. A. W., Boden, C., and Giaschi, D. E. (2004). Children with dyslexia: evidence for visual attention deficits in perception of rapid sequences of objects. Vision Res. 44, 2521–2535.
Vul, E., Nieuwenstein, M., and Kanwisher, N. (2008). Temporal selection is suppressed, delayed, and diffused during the attentional blink. Psychol. Sci. 19, 55–61.
Wetzel, N., Widmann, A., Berti, S., and Schröger, E. (2006). The development of involuntary and voluntary attention from childhood to adulthood: a combined behavioral and event-related potential study. Clin. Neurophysiol. 117, 2191–2203.
Wyble, B., Bowman, H., and Nieuwenstein, M. (2009a). The attentional blink provides episodic distinctiveness: sparing at a cost. J. Exp. Psychol. Hum. Percept. Perform. 35, 787–807.
Keywords: attention, development, children, rapid serial visual processing, attentional blink
Citation: Heim S, Wirth N and Keil A (2011) Competition for cognitive resources during rapid serial processing: changes across childhood. Front. Psychology 2:9. doi:10.3389/fpsyg.2011.00009
Received: 26 August 2010;
Accepted: 07 January 2011;
Published online: 27 January 2011.
Edited by:
David Sobel, Brown University, USAReviewed by:
Nicholas B. Turk-Browne, Princeton University, USADima Amso, Cornell University Weill Medical College, USA
Copyright: © 2011 Heim, Wirth and Keil. This is an open-access article subject to an exclusive license agreement between the authors and Frontiers Media SA, which permits unrestricted use, distribution, and reproduction in any medium, provided the original authors and source are credited.
*Correspondence: Sabine Heim, Center for Research on Individual Development and Adaptive Education, German Institute for International Educational Research, Solmsstrasse 73-75, D-60486 Frankfurt am Main, Germany. e-mail:aGVpbUBkaXBmLmRl