- 1 Department of Political Science and Economics, Waseda University, Shinjuku-ku, Tokyo, Japan
- 2 Faculty of Intercultural Studies, Tomakomai Komazawa University, Tomakomai, Hokkaido, Japan
Since the discovery of the “framing effect” by Kahneman and Tversky, the sensitivity of the “framing effect” – its appearance and in some cases its disappearance – has long been an object of study. However there is little agreement as to the reasons for this sensitivity. The “ambiguity-ambivalence hypothesis” (Wang, 2008) aims to systematically explain the sensitivity of this effect by paying particular attention to people’s cue priority: it states that the framing effect occurs when verbal framing is used to compensate for the absence of higher prioritized decision cues. The main purpose of our study is to examine and develop this hypothesis by examining cue priority given differences in people’s “group experience.” The main result is that the framing effect is absent when the choice problem is presented in a group context that reflects the actual size of the group that the participant has had experience with. Thus, in order to understand the choices that people make in life and death decisions, it is important to incorporate the decision maker’s group experience explicitly into the ambiguity-ambivalence hypothesis.
1 Introduction
Over the last three decades, empirical research on human cognition and decision-making behavior has shown a systematic bias in a number of decision-making areas. Needless to say, one of the pioneering studies in this field was performed by Kahneman and Tversky: on the framing effect in life and death decision problems (e.g., Kahneman and Tversky, 1979; Tversky and Kahneman, 1981).
In their study, subjects were presented with a cover story, which explained that 600 people were suspected to be infected with a fatal Asian disease for which only two curative plans are available. Specifically, Plan A has a deterministic outcome, while Plan B has a probabilistic outcome. The deterministic outcome ensures the survival of one-third of the patients (i.e., 200 survivors), while the probabilistic outcome results in a one-third probability that all of the patients will survive, and a two-thirds probability that no one will survive. After the subjects read the cover story, they were asked to choose one of the two plans.
The classic demonstration of the framing effect (Tversky and Kahneman, 1981) is as follows: when this problem was represented in terms of saving lives (a “positive frame”), most subjects (72%) were risk-averse: the certain survival of 200 lives was more attractive than the risky choice, that is, a one-third chance of saving all 600 lives. When this problem was represented in terms of losing lives (a “negative frame”), in contrast, most subjects (78%) favored the risky choice: the assured death of 400 people was less attractive than the two-thirds probability that all 600 could die.
A number of studies have been conducted to test the reliability and generality of Tversky and Kahneman’s original work on the framing effect. On the one hand, using the standard cover story, strong framing effects have been replicated not only in different kinds of respondents, such as university faculty staff, students, and physicians (McNeil et al., 1982) but also in various applied areas (Burton and Babin, 1989; Kramer, 1989; Travis et al., 1989). On the other hand, some studies have shown little or no framing effect when the context or cover story was manipulated. This suggests that the framing effect may not be as general and as robust a choice phenomenon as one thought, because it is sensitive to both the context in which the problem is described (Fagley and Miller, 1987; Schneider, 1992; Wang and Johnston, 1995; Wang, 1996a,b; Takemura, 2001; Wang et al., 2001) and to various cognitive and social variables (Roszkowski and Snelbecker, 1990; Miller and Fagley, 1991; Shoorman et al., 1994).
In support for context-dependent line of inquiry, a series of studies by Wang et al. (2001) observed an obvious effect of contextual group size on people’s attitude to risk. Table 1 summarizes Wang’s previous research, almost all of which used a sample of university students, showing how people’s attitude to risk changes when the contextual group size is manipulated in the life and death decision problem. By closely examining these results, we can emphasize one major finding: an absence of the framing effect. The framing effect is seen only when the decision problem was presented in large group contexts, in this case, 6000 or 600 people. However, this bias was eliminated when the same problem was posed in terms of small groups, consisting of 6 or 60 people.
While this absence of the framing effect in small group contexts has been replicated with university students (e.g., Bloomfield, 2006; Zhang and Miao, 2008), other researchers (e.g., Shimizu and Udagawa, 2011) continued to report a robust framing effect when using a general public sample. Their data (Shimizu and Udagawa, 2011) is shown in Table 2. How does a shift in participant populations affect the appearance and disappearance of the framing effect? The main purpose of this study is to answer that question in terms of the “ambiguity–ambivalence hypothesis,” explained in the next section. The point we want to make, is that in order to understand people’s choice patterns in life and death decision problems, it is important to take into account not only their inherent biases but also their personal experience of groups in real life. The rest of the paper will be structured as follows, Section 2 explains the essence of the ambiguity-ambivalence hypothesis. Section 3 reframes the ambiguity-ambivalence hypothesis in terms of our predictions about personal group experience. Section 4 presents results of an experiment supporting this view. Implications for these results will be discussed in Section 5.
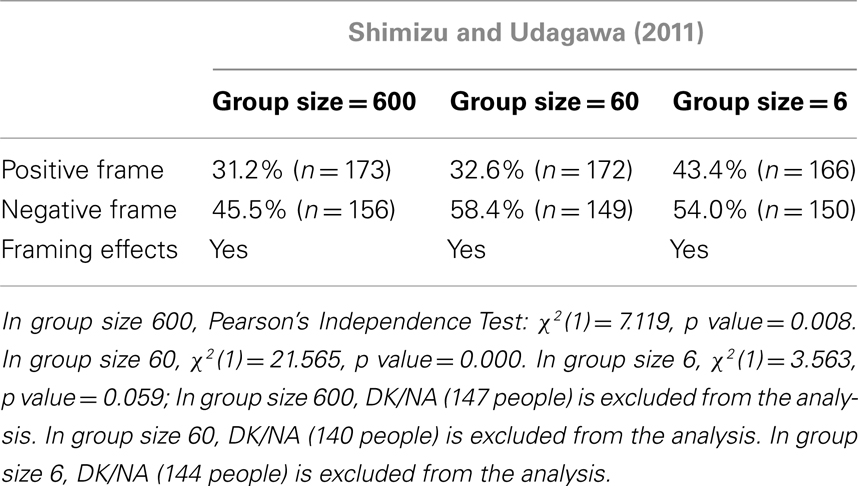
Table 2. Percentages of the probabilistic alternative of the life-death decision problem across three sizes in a national survey.
2 Ambiguity–Ambivalence Hypothesis
The ambiguity–ambivalence hypothesis (Wang, 1996a, 2008) aims to systematically explain the disappearance of the framing effect. This hypothesis, drawing on evolutionary concepts, proposes the following assumptions: “(1) Decision cues are selected and used in accordance to their priorities. (2) Cue priority reflects the evolutionary and ecological validity of a cue in predicting specific risks. (3) Primary cues in risk communication carry evolutionary, ecological, and social significance and anchor decision reference points, while secondary cues of verbal communication fine-tune the settings of reference points. (4) Inconsistent decision biases tend to occur as a result of secondary cue use when primary cues are absent in risk communication (i.e., an ambiguity condition) or when primary cues elicit conflicting preferences (i.e., an ambivalence condition)” (Wang, 2008, p. 82).
According to this hypothesis, the framing effect was evident in both the 6000 people and 600 people groups because the life and death problem was presented in a large, anonymous, and hence ambiguous context: group size variables (6000 and 600) then, cannot function as primary cues. On the other hand, the framing effect was absent in groups of 60 and 6 people, because these group sizes indicate a high level of interdependency, and is likely to have evoked familial, kinship relationships in the subjects. Size variables (60 and 6), then, can function as primary cues. Generally speaking, this hypothesis states that verbal framing as a secondary cue has the most obvious effect only when a choice is presented in an evolutionarily novel and ambiguous context in which primary cues are absent or produces conflicting risk preferences.
We agree that an evolutionary perspective sufficiently captures the reason for the seemingly inconsistent decision biases observed in a context-dependent choice phenomena. However, there is room for further investigation as to what “evolutionary and ecological validity of a cue” means and how this affects people’s cue choice. Our interpretation of this is outlined in the following section.
3 People’s Experiences and Evolved Decision-Making Rules
Evolutionary psychology suggests that the human mind consists of psychological mechanisms derived from the human Environment of Evolutionary Adaptedness (EEA). For example, the size of a Pleistocene hunter-gatherer society is estimated to consist of around 100–200 people (Cosmides and Tooby, 1989, 2000; de Waal, 1996)1. A considerable number of studies support that figures around 150 are frequently observed among a wide range of contemporary human societies, including farming communities, sub-disciplines of academic communities, and basic army units (Mange and Mange, 1980; Hardin, 1988; Becher, 1989).
On the basis of this discussion, it is natural to suppose that people tend to consider a small sized group to be a community held together emotionally by fate. Thus, they might be more likely to prioritize small group sizes as ecologically valid cues rather than large group sizes in the life and death decision problem, so that the framing effect disappears in the small group context, containing 6 and 60 people. Let us paraphrase this argument by focusing on the fact that human minds evolve through the interplay of biology and culture.
As mentioned in the introduction, while the absence of the framing effect appears to be stable in experiments using university student samples (Wang and Johnston, 1995; Wang, 1996a,b,c, 2008; Wang et al., 2001; Bloomfield, 2006; Zhang and Miao, 2008), this is not the case for experiments using the general public (Shimizu and Udagawa, 2011). However, if decision-making is influenced by acquired elements, such as group experience in real life, the general public may have a different cue priority from students. This is the point that we intend to add to the original ambiguity– ambivalence hypothesis.
From the perspective of evolutionarily inherited biases, groups of 6 and 60 can be valid cues, because they are smaller than the EEA size; groups of 600 and 6000 cannot, because they are greater. This argument is valid for both university students and the general public. This is also supported by personal experience, groups of 600 and 6000 are unlikely to be a primary cue both for university students and for the general public, because experience with groups of such a large size is uncommon in our society. However, this is not the case for groups of 6 and 60.
On the one hand, groups of 6 and 60 can serve as valid cues for university students, due to the fact that 6 corresponds to the size of families, and 60 is not far from group sizes encountered in their school lives. On the other hand, it is possible that the general public does not focus on small group sizes such as those of 6 or 60 people as closely as students, since the general public is more varied than university students in terms of group experience. Although family remains an important group for them, we may assume that some of them are living independently from the families in which they were born/raised, and that they may have other important group experiences such as working for a company, or union activities, in which group sizes can be larger than 6 or 60. In short, compared to university students, their various experiences can prevent them from giving immediate priority to smaller group sizes.
This intuition that one’s group experience can affect his/her cue priority is supported by several lines of inquiry. On the one hand, it is well known in both cognitive and social psychology that our cognitive processes are frequently influenced by our sense of group membership (e.g., Tajfel, 1970, 1981; Brewer, 1979). On the other hand, a considerable number of studies in various fields have shown that prior experiences affect the decision makers’ attitude toward risk (e.g., Levin and Chapman, 1990, 1993; Roszkowski and Snelbecker, 1990; Zhang and Miao, 2008). Little attention, however, has been paid to the prior experience of group membership on cue priority. Rothman and Salovey (1997) provided a focused review of framing effects in health-related decisions, showing that although a message is advocated by a particular frame, such as gain or loss, the framed information is not automatically accepted by the receiver; “an individual’s knowledge and experiences with an issue can play important roles to determine whether a particular frame is accepted or deflected” (p. 16). It seems reasonable to extend their argument about one’s willingness to adopt a particular frame to one’s tendency to prioritize a particular cue.
4 Experiment: Effect of Experience on Cue Priority
The main purpose of this experiment was to examine how the experience of groups in real life affects people’s cue priority. Participants were quizzed on questions that not only revealed their degree of family experience, but also their group experiences beyond that of family.
4.1 Subjects
For this study, we consigned a web experiment through a private research company and used the members pooled by that company as subjects. These members voluntarily apply for membership to the company, and can choose to answer the administered survey questions diffused over the internet. Participation can be done in their homes, as the experimental instructions are presented on their computer screens. After the experiment, the company randomly extracts the collected data and pays these respondents a fee. The experiment took place between 18/02/2010 and 26/02/2010, with 1893 subjects: 860 female (45.4%) and 1033 male (54.6%), excluding DK (don’t know)/NA (no answer) responses. The mean age was 42.4 years (standard deviation: 12.6 years, range: 20–69 years). The student sample was very small, representing only 0.5% of the total sample.
One oftnoted drawback of such web experiments is sample bias, resulting from issues such as the digital divide. Although the company shuffles its members every year and we tried to use as widely distributed a sample as possible, the problem of digital division may not be negligible when the results are generalized. However, to explicitly consider experience-related variables, it is necessary to use a subject sample whose range of experience is greater than those of than a university student sample. Thus, we decided to conduct a web experiment with a general population rather than a laboratory experiment using university students.
4.2 Experimental Design
For this study, we principally used the same experimental design described in the introduction, apart from the number of group sizes. While group size previously consisted of 3 categories (600, 60, and 6), or 4 categories (6000, 600, 60, and 6), in this experiment we used 6 categories: 60000, 6000, 600, 150, 60, and 6. The additional group sizes sought to explore the size effect a little further and to directly compare the estimated threshold of EEA group size–namely 150. Subjects were randomly presented with one of the different versions of the life and death problem and were asked to choose a medical plan.
Since the subject’s experience in groups of different sizes is hypothesized to influence his or her way of considering group size as a primary cue in life-death decisions, we had prepared, after their choice of Plan A or Plan B, not only a demographics questionnaire (including sex, age, marital status, residential status, profession, and revenue) but also a question about their group experiences. While questions about marital and residential status of the former set were mainly used to query their family situation, the latter targeted how deeply committed they are to group(s) besides their family. The latter question was, “To which groups or organizations do you commit enthusiastically or quite enthusiastically? Choose all that apply from the following list.” The list of groups/organizations consisted of “trade associations, agricultural cooperatives, labor unions, consumer cooperatives, NGOs, religious organizations, groups for study or lessons, groups for hobbies or pleasure, volunteer groups, alumni (alumnae) associations, neighborhood self-governing bodies, and others2.” While the first 5 groups/organizations are considered to be closely related to the subjects’ economic interests, the last 6 categories are less closely so. Further, subjects were asked to provide the size of each group or organization to which they were committed. In this answer, 1 corresponds to 2 ∼ 4 members; 2 to 5 ∼ 9; 3 to 10 ∼ 29; 4 to 30 ∼ 99; 5 to 100 ∼ 199; 6 to 200 ∼ 299; 7 to 300 ∼ 999; 8 to 1000 ∼ 4999; 9 to 5000 ∼ 9999; and 10 to over 10000 (see Table 3)3.
4.3 Predictions
Based on previous research and the evolutionary approach we put forward in Section 3, we hypothesized that the framing effect would not be observed in a group size which is prioritized both by evolutionary inheritance and by acquired experience.
That is, we predict that the framing effect disappears in group size 6 for subjects who are responsible for managing the family; and disappears in group size 60 for those who are deeply involved in groups or organizations whose size is also around 60.
4.4 Results1: Family Experience and Cue Priority
Table 4 reveals the presence of the framing effect in all 6 group sizes. The critical question motivated by the main research interest of this experiment is whether this framing effect interacts with group experience, guiding differences in people’s cue priority.
In this web experiment, as well as in Shimizu and Udagawa (2011), the framing effect was not observed even for group size 6 (p value is 0.001). Thus, we re-examined the framing effect for every group size with a selected sample that we hypothesized would give high priority to a group size variable of 6 people. As we argued in Section 3, the framing effect should disappear in the group size 6 condition with a sample selected to prioritize these cues because they have had experience with groups of this size. However, we cannot logically predict the direction of effect in the other group sizes.
Firstly, by using the participant’s demographic data, we selected a subset of 723 subjects (hereafter sample S), who were heads of households or homemakers living with children. Although most humans develop experiences of family and kinship, sample S might prioritize cues about 6 people more than others because they are, intuitively, those responsible for managing a family. Because of their commitment to the family, we expect them to focus on this specific cue.
Table 5 reveals an absence of the framing effect for sample S in group size 6 (p value is 0.289)4. This is because the difference in risk choices between the two frames is smaller for sample S compared to the whole sample (the difference between the two frames is 20.2% for the whole sample and 10.2% for sample S). It follows that the framing effect is highly significant in group size 6 for the subset of participants that did not belong to sample S (hereafter sample SC; p value is 0.000).
Secondly, we tested whether the framing effect for sample S (i.e., the difference between the positive and negative frames: 61.1% − 50.9% = 10.2%) was significantly different from that for sample SC’s (i.e., 65.4% − 39.8% = 25.6%). The interaction coefficient β3 should be significant in the following model if the framing effect is stronger for sample SC than sample S5:

The results show that while both β1 and β2 are not significant (p values 0.290 and 0.185 respectively), β3 is significant (exp β3 is 2.047, p value of z-test 0.007 and standard error 0.268). Thus, the two samples significantly differed in the size of their framing effect in the group size 6 condition.
4.5 Results 2: Group Experience and Cue Priority
So far, the results of statistical analysis show an absence of the framing effect in a sample that might adopt a group size of 6 as a primary cue. If experience shapes cue priority, the same phenomenon should be observed in other group sizes.
As shown in Table 4, the framing effect is evident in group size 60 for the whole sample. If we focus on people who are likely to give high cue priority to groups of size 60, the framing effect should disappear for that group (we cannot logically predict the presence or absence of the framing effect in the other sizes). From their questionnaire answers concerning the subjects’ group experiences, we selected subjects who were deeply involved in groups or organizations at a scale that is greater than that of the family, and below that of the EEA maximum: i.e., those who answered (3) 10 ∼ 29, (4) 30 ∼ 99, or (5) 100 ∼ 199 for any groups or organizations (the total of this sample is 670)6. For this sample (hereafter sample T), we hypothesized that they have inherited a deep involvement in groups/organizations of a scale that reminds them of a community, hence allowing them to focus on group size 60 as a primary cue when also faced with group size variable of 60.
Consistent with our prediction, the statistical analysis of Table 6 revealed an absence of the framing effect for a group size of 60 for sample T (p value is 0.266). Within the total sample, the difference in risk choice between the two frames was significantly different at 13.2%, while the difference in sample T was 10.3%7. For the remaining participants (sample TC), the framing effect is significant (p value is 0.041)
Similar to Section 4.5, we also tested whether the difference in sample T’s choice in positive and negative frames (i.e., 43.6% − 33.3% = 10.3%) was significantly different from that of sample TC’s difference between both frames (i.e., 49.5% − 35.6% = 13.9%). Again, we expect that the interaction coefficient β3 would be significant, such that the framing effect is stronger with sample TC than with sample T8:

The result of this analysis showed that while both β1 and β2 were not significant (p value is 0.278 and 0.859 respectively), β3 was significant (p value of z-test 0.028 and standard error 0.241). Thus, there were significant differences in the framing effect between sample T and TC.
The combination of results from Sections 4.4 and 4.5, support our prediction about the framing effect: the framing effect is nullified in contextual group sizes of both 6 and 60 for a sample that has selectively had real experience with these group sizes.
It is worth pointing out how people, who categorically belong to both S and T at the same time, prioritize their cues. Following the argument in 4.4 and this section, we can suppose that the framing effect should not appear for these people in both the 6 and 60 group conditions. Table 7 supports this prediction and additionally shows an interesting finding. The framing effect does not appear in any of the categories for these people. We cannot reasonably explain here why and how the framing effect disappears for this sample, for example this may be partly due to an insufficient number of participants in the statistical analysis, but it may be important to keep this finding in mind.
5 Conclusion and Discussion
Given that human perception is hardwired through an evolutionary process, the results of this experiment support the argument that one needs to consider not only people’s inherent biases but also their acquired experiences in order to understand the way in which cues are prioritized in life and death decisions. To explore the further possibilities of this research, we should clarify the points that call for discussion.
The first is related to the questionnaire we prepared probing subjects’ experiences in social groups, in order to classify their group experience. We recognize at least two problems with this attempt.
Firstly, it was difficult to test the effect of personal group experience on cue priority for the life-death decision problem when the contextual group size is greater than 100. This is due to the fact that subjects clustered on the smaller sized groups/organizations (see Table 3). In other words, in conducting a standard laboratory or web experiment, there are insufficient numbers of participants with large group experiences. In this case, field experiments may be helpful, particularly if conducted in an area where subjects are working or living in a large group, such as a factory, military base, or farming collective.
Secondly, the present question only quizzed group experience on 11 groups or organizations considered to be important in Japanese social life. Thus, it excludes other important organizations, such as production groups represented by companies or factories. Since, from an evolutionary standpoint, it is plausible to suppose that this kind of experience would affect cue priority, we need to know more about subjects’ group experience in production sites, in order to measure their level of group experience more precisely.
In addition to these limitations, there are two interesting points. One is an alternative explanation of disappearance/occurrence of the framing effect. This explanation highlights individual differences in cognitive abilities, such as numeracy. Peters and Levin (2008) reveals that the less numerate showed a more direct effect of framing, compared to the highly numerate. Assuming that the general public is lower than university students in terms of numeracy, this explanation appears to be consistent with the pattern of the framing effects in our sample. In a similar vein, Frederick (2005) stated that people with lower cognitive ability generally tend to prefer the deterministic choice to the probabilistic choice. Risk-averseness, due to low cognitive ability, might prevent our sample from being risk-seeking in both frames when the contextual group size is small, resulting in the framing effect across all conditions. Since these studies (i.e., Frederick, 2005; Peters and Levin, 2008) do not focus on the manipulation of contextual group size in the life and death problem, it is premature to attribute the existence of the framing effect in small group sizes to differences in the sample’s cognitive ability. Future research in group size manipulations might seek to also measure participant’s cognitive ability.
The other is the possibility of another nurture element beyond group experience affecting decision-making under uncertain conditions. If we compare the percentages of the subjects that choose the probabilistic choice between our sample (Japanese) and those percentages found in previous research (American or Belgian), the former sample is consistently more risk-averse than the latter sample. In comparison with Wang (1996b) and Wang et al. (2001), the percentage of Japanese subjects who chose the probabilistic choice is usually smaller than those of American or European subjects by 10 ∼ 30% across every contextual size. Olivola and Sagara (2009) showed in a cross-national study of risk preference that the tendency to be risk-seeking in the life and death decision problem is lower in countries in which high-mortality events are more frequently observed. It is debatable whether mortality-related events are more often observed in Japan than in the U.S.A and Belgium. However it is important to bear in mind that cross-national differences in the social environment, such as human fatalities, may influence people’s risk attitude.
Conflict of Interest Statement
The authors declare that the research was conducted in the absence of any commercial or financial relationships that could be construed as a potential conflict of interest.
Acknowledgments
We would like to thank the participants of the 20th Annual Meeting of the Human Behavior and Evolution Society (2008) for their helpful suggestions. This paper owes much to the thoughtful and helpful comments of Professor X. T. Wang and Professor Jonathan Baron. We gratefully acknowledge financial support from the Global COE program of Political Economy and Institutional Construction (I-13) and the Grant-in-Aid for Scientific Research on Priority Areas (19046002). All support was provided by the Japanese Ministry of Education, Culture, Sports, Science and Technology.
Footnotes
- ^According to neuroscience, the size of a social animal’s neocortex is causally related to that of group size. Dunber (1995) calculated the size of hunter-gatherer societies by regressing group size with the size of human neocortex. The predicted group size was 147.8, and the 95% confidence limits around this prediction were from 100.2 to 231.1.
- ^We modeled this list on a standard Japanese social opinion survey. The survey considers these 11 groups and organizations as important to ordinary Japanese social life.
- ^In making this scale, we ensured that each contextual group size – 60000, 6000, 600, 150, 60 and 6 – is represented by a different number. The estimated EEA group size from 100.2 to 231.1 (Dunber, 1995) is generally covered by the answer 5 (100 ∼ 199).
- ^There is an unexpected observation which needs to be mentioned. The framing effect is also absent in group size 150 (p value is 0.504). If group experience affects cue priority as we predicted, sample S might have also tended to commit to some groups/organizations, whose size would also be around 150 people. However, we cannot say for certain whether our guess is valid, because our question about group experience is not complete enough to detect people’s group experience in real life.
- ^If the probability of the ith respondent’s probabilistic outcome choice is written by pi, the dependent variable is defined as logit (pi); that is, log pi/1 − pi. β is a standardized binomial logit regression coefficient. Framing (Framei) is dummy coded as 1 if subject i responded to the negative frame problem, otherwise, 0. Family (Familyi) is also dummy coded as 1 if subject i belonged to sample SC and otherwise, 0.
- ^The number of participants in sample T (670) is not the sum of participants who responded to groups of size “10 ∼ 29,” “30 ∼ 99,” and “100 ∼ 199” in Table 3. This is because participants could choose several groups or organization at the same time. For example, they can participate in a Religious organization of size 30–99 as well as a Volunteer group of size 100–199.
- ^While it is established that the maximum size of EEA is around 150, some scholars insist that typical group sizes in EEA were often around 30 (e.g., Marlowe, 2007). On the basis of the latter idea, we reran the same analysis with the 58 subjects who answered (3) 10 ∼ 29 or (4) 30 ∼ 99. The result remained non-significant (p value is 0.190). Thus, the result does not essentially change. However, this result is tentative due to the small number of participants in the analysis.
- ^In this model, Group (Groupi) is dummy coded as 1 if subject i belonged to sample TC and otherwise, 0. The other variables were coded as described in model A.
References
Bloomfield, A. N. (2006). Group size and the framing effect: threats to human beings and animals. Mem. Cognit. 34, 929–937.
Brewer, M. B. (1979). Ingroup bias in the minimal intergroup situation: a cognitive-motivational analysis. Psychol. Bull. 86, 307–324.
Burton, S., and Babin, L. A. (1989). Decision-framing helps make the sale. J Consum. Mark. 6, 15–24.
Cosmides, L., and Tooby, J. (1989). Evolutionary psychology and the generation of culture: part II. A computation theory of social exchange. Ethol. Sociobiol. 10, 51–97.
Cosmides, L., and Tooby, J. (2000). “Toward mapping the evolved functional organization of mind and brain,” in The New Cognitive Neurosciences, 2nd Edn, ed. M. S. Gazzaniga (Cambridge, MA: MIT Press), 1167–1178.
de Waal, F. (1996). Good Natured: The Origin of Right and Wrong in Humans and Other Animals. Cambridge: Harvard University Press.
Dunber, R. I. M. (1995). Neocortex size and group size in primates: a test of the hypothesis. J. Hum. Evol. 28, 287–296.
Fagley, N. S., and Miller, P. M. (1987). The effects of decision framing on choice of risky vs certain options. Organ. Behav. Hum. Decis. Process. 39, 264–277.
Kahneman, D., and Tversky, A. (1979). Prospect theory: an analysis of decisions under risk. Econometrica 47, 313–327.
Kramer, R. M. (1989). Windows of vulnerability or cognitive illusions? Cognitive processes and the nuclear arms race. J. Exp. Soc. Psychol. 25, 79–100.
Levin, I. P., and Chapman, D. P. (1990). Risk taking, frame of reference, and characterization of victim groups in AIDS treatment decisions. J. Exp. Soc. Psychol. 26, 421–434.
Levin, I. P., and Chapman, D. P. (1993). Risky decision making and allocation of resources for leukemia and AIDS programs. Health Psychol. 12, 110–117.
Marlowe, F. W. (2007). Hunting and gathering: the human sexual division of foraging labor. Cross Cult. Res. 41, 170–195.
McNeil, B. J., Pauker, S. G., Sox, H. C. Jr., and Tversky, A. (1982). On the elicitation of preferences for alternative therapies. N. Engl. J. Med. 306, 1259–1262.
Miller, P. M., and Fagley, N. S. (1991). The effects of framing, problem variations, and providing rationale on choice. Pers. Soc. Psychol. Bull. 17, 517–522.
Olivola, C. Y., and Sagara, N. (2009). Distributions of observed death tolls govern sensitivity to human fatalities. Proc. Natl. Acad. Sci. U.S.A. 106, 22151–22156.
Peters, E., and Levin, I. P. (2008). Dissecting the risky-choice framing effect: numeracy as an individual-difference factor in weighting risky and riskless options. Judgm. Decis. Mak. 3, 435–448.
Roszkowski, M. J., and Snelbecker, G. E. (1990). Effects of “framing” on measures of risk tolerance: financial planners are not immune. J. Behav. Econ. 19, 237–246.
Rothman, A. J., and Salovey, P. (1997). Shaping perceptions to motivate healthy behavior: the role of message framing. Psychol. Bull. 121, 3–19.
Schneider, S. L. (1992). Framing and conflict: aspiration level contingency, the status quo, and current theories of risky choice. J. Exp. Psychol. Learn. Mem. Cogn. 18, 1040–1057.
Shimizu, K., and Udagawa, D. (2011). A re-examination of the effect of contextual group size on people’s attitude to risk. Judgm. Decis. Mak. 6, 156–162.
Shoorman, F. D., Mayer, R. C., Douglas, C. A., and Hetrick, C. T. (1994). Escalation of commitment and the framing effect: an empirical investigation. J. Appl. Soc. Psychol. 24, 509–528.
Tajfel, H. (1981). Human Groups and Social Categories: Studies in Social Psychology. Cambridge: Cambridge University Press.
Takemura, K. (2001). “Contingent decision making in the social world,” in Decision Making: Social and Creative Dimensions, eds C. M. Allwood and M. Selart (Dordrecht: Kluwer Academic), 153–173.
Travis, C. B., Phillippi, R. H., and Tonn, B. E. (1989). Judgment heuristics and medical decisions. Patient Educ. Couns. 13, 211–220.
Tversky, A., and Kahneman, D. (1981). The framing of decisions and the psychology of choice. Science 211, 452–458.
Wang, X. T. (1996a). Evolutionary hypotheses of risk-sensitive choice: age differences and perspective change. Ethol. Sociobiol. 17, 1–15.
Wang, X. T. (1996b). Framing effects: dynamics and task domains. Organ. Behav. Hum. Decis. Process. 68, 145–157.
Wang, X. T. (1996c). Domain-specific rationality in human choices: violations of utility axioms and social contexts. Cognition 60, 31–63.
Wang, X. T. (2008). Risk communication and risky choice in context ambiguity and ambivalence hypothesis. Ann. N. Y. Acad. Sci. 1128, 78–89.
Wang, X. T., and Johnston, V. S. (1995). Perceived social context and risk preference: a re-examination of framing effects in a life-death decision problem. J. Behav. Decis. Mak. 8, 279–293.
Wang, X. T., Simons, F., and Bredart, S. (2001). Social cues and verbal framing in risky choice. J. Behav. Decis. Mak. 14, 1–15.
Zhang, Y., and Miao, D. (2008). Social cues and framing effects in risky decisions among Chinese military students. Asian J. Soc. Psychol. 11, 241–246.
Appendix
Versions of the Life-Death Decision Problem in Experiments
Positive Frame Version
Imagine that 6 (60, 150, 600, 6000, or 60000) people are infected by a fatal disease. Two alternative medical plans to treat the disease have been proposed. Assume that the exact scientific estimates of the consequences of the plans are as follows:
• If plan A is adopted, 2 (20, 50, 200, 2000, or 20000) people will be saved.
• If plan B is adopted, there is a one-third probability that all 6 (60, 150, 600, 6000, or 60000) people will be saved, and a two-thirds probability that none of them will be saved.
Which of the two plans would you favor?
Negative Frame Version
Imagine that 6 (60, 150, 600, 6000, or 60000) people are infected by a fatal disease. Two alternative medical plans to treat the disease have been proposed. Assume that the exact scientific estimates of the consequences of the plans are as follows:
• If plan A is adopted, 4 (40, 100, 400, 4000, or 40000) people will die.
• If plan B is adopted, there is a one-third probability that none of them will die, and a two-thirds probability that all 6 (60, 150, 600, 6000, or 60000) will die.
Which of the two plans would you favor?
Keywords: framing effect, group size, cue priority, experience
Citation: Shimizu K and Udagawa D (2011) How can group experience influence the cue priority? A re-examination of the ambiguity-ambivalence hypothesis. Front. Psychology 2:265. doi: 10.3389/fpsyg.2011.00265
Received: 04 March 2011;
Accepted: 25 September 2011;
Published online: 12 October 2011.
Edited by:
Xiao-Tian Wang, University of South Dakota, USAReviewed by:
Sarah E. Hill, Texas Christian University, USAShenghua Luan, Singapore Management University, Singapore
Copyright: © 2011 Shimizu and Udagawa. This is an open-access article subject to a non-exclusive license between the authors and Frontiers Media SA, which permits use, distribution and reproduction in other forums, provided the original authors and source are credited and other Frontiers conditions are complied with.
*Correspondence: Kazumi Shimizu, Department of Political Science and Economics, Waseda University, 1-6-1 Nishi-Waseda, Shinjuku-ku, Tokyo 169-8050, Japan. e-mail:c2thenVtaTE5NjFAZ21haWwuY29t