- 1 Department of Diagnostic and Interventional Neuroradiology, MR Research Group, University of Tübingen, Tübingen, Germany
- 2 Department of English Studies, Centre of Linguistics, University of Tübingen, Tübingen, Germany
- 3 Former Section of Experimental MR of the CNS, Centre for Integrative Neuroscience, University of Tübingen, Tübingen, Germany
- 4 Department of General Neurology, Hertie Institute for Clinical Brain Research, University of Tübingen, Tübingen, Germany
- 5 Laboratory of Clinical Biochemistry and Molecular Biology, Department of Experimental Pathology, Medical Biotechnologies, Infectivology and Epidemiology, University of Pisa, Pisa, Italy
- 6 Neuroimaging Laboratory, Santa Lucia Foundation, Rome, Italy
- 7 Department of Psychiatry, Psychotherapy and Psychosomatics University Clinic Aachen, Aachen, Germany
An unanswered question in adult language learning or late bi and multilingualism is why individuals show marked differences in their ability to imitate foreign accents. While recent research acknowledges that more adults than previously assumed can still acquire a “native” foreign accent, very little is known about the neuro-cognitive correlates of this special ability. We investigated 140 German-speaking individuals displaying varying degrees of “mimicking” capacity, based on natural language text, sentence, and word imitations either in their second language English or in Hindi and Tamil, languages they had never been exposed to. The large subject pool was strictly controlled for previous language experience prior to magnetic resonance imaging. The late-onset (around 10 years) bilinguals showed significant individual differences as to how they employed their left-hemisphere speech areas: higher hemodynamic activation in a distinct fronto-parietal network accompanied low ability, while high ability paralleled enhanced gray matter volume in these areas concomitant with decreased hemodynamic responses. Finally and unexpectedly, males were found to be more talented foreign speech mimics.
Introduction
There are considerable individual differences when it comes to the pronunciation of a foreign language, especially if it is learned in adolescence. While some of the so-called “late learners” have excellent mimicking capacities and pass easily as a native speaker (e.g., Abrahamsson and Hyltenstam, 2008), others retain a heavy native accent (sometimes referred to as the “Joseph Conrad” phenomenon). Research in the field of second language (L2) learning ability/aptitude (Obler and Fein, 1988; Skehan, 2011) has established that individuals can either have what the authors call a “talent for accent” (phonetic/phonological domain) or a “talent for grammar” (syntactic–semantic domain, Nauchi and Sakai, 2009). Those who have a talent for accent can imitate foreign speech up to a native level, despite their late age of onset of learning (AOL). They seem not to be affected by a “critical/sensitive period” for learning pronunciation – contrary to the usual line of thinking (Birdsong, 2006). Various researchers acknowledge that the prevalence of this outstanding ability – which adopts Gaussian distribution – is rather low, amounting only to about 5% of adults (Selinker, 1972; Wells, 1985). However, as with all abilities, there is a continuum rather than a sharp demarcation, with individuals possessing varying degrees of a certain ability. Despite a widespread interest in the causal underpinnings of this phenomenon, it has remained a neglected research topic. Apart from other, more cognitive attempts to explain the phenomenon, or on the basis of case studies, it has been suggested earlier (Geschwind and Galaburda, 1985; Obler and Fein, 1988) that the behavioral foreign accent differences could arise from underlying functional–neuro-anatomical individual differences. Most published accounts or reviews of this however leave open exact descriptions of the nature of these neural underpinnings. Recently, a very few attempts to clarify the neural correlates of parts of foreign language imitation capacity have been undertaken (Golestani et al., 2002; Amunts et al., 2004), but 1. no integration of the anatomical and functional bases in one and the same subject sample has been reported so far, 2. collateral variables such as previous language experience have not been controlled rigorously, 3. single cases were reported, or small to medium sample sizes which did not include the upper and lower percentiles of the ability spectrum (very high versus very low ability), 4. sample stimuli employed were confined to single phonemes only (which do not reflect accent imitation in its full range), and 5. in most cases the phonetic level of speech-sound imitation/production capacity was not investigated in isolation (thus being confounded with other levels of language).
Concerning brain anatomy, one research team (Golestani and Pallier, 2007; Golestani et al., 2007) could relate speed in speech-sound production learning (a foreign Persian sound) of a single phoneme to white matter (WM) changes (more WM density for better learners) located in bilateral inferior parietal areas as well as left insula and prefrontal structures. Another study (Amunts et al., 2004, albeit not directly investigating the phonetic level of speech imitation) could correlate cytoarchitectonic differences in Broca’s area to outstanding giftedness in foreign language learning in general (the learning of 60 languages). Concerning the anatomical level, a range of studies so far could establish a relationship between GM/WM density or volume and general speech/language skills. Usually lower amounts of gray matter (GM) volume are reported to be neuro-anatomical signatures of lower performance in a specific skill. For example, McAlonan et al. (2008) found a correlation between GM volume (GMV) around Brodmann Area 44 (BA 44, opercular part of Broca’s area in the inferior frontal gyrus) and language skills in high-functioning autistic individuals. Another study using voxel-based morphometry (VBM; Mechelli et al., 2004) reported higher L2 proficiency to be correlated with more GM density in the left IPL (Inferior Parietal Lobe). However, these last two studies mentioned were not specific to foreign language speech production/pronunciation.
Neuro-functionally, one study (Golestani and Zatorre, 2004) suggested that the degree of success in learning to perceive the differences is accompanied by more efficient neural processing in classical frontal speech regions. In this study the researchers used a training paradigm for learning to passively perceive differences in a difficult foreign phonetic contrast.
Thus, to re-examine this issue of individual differences in accent imitation ability and its neuro-cognitive bases, we adopted an extensive pre hoc search paradigm. We screened 200 mother-tongue (L1) German-speaking individuals, who were either “talented,” “mid-range,” or “low-talented” foreign language imitators (L2, second language, English) to include also the extreme upper and lower percentiles of this normally distributed ability.
To get rid of the confounding variable of training or linguistic experience, we (additionally to using multiple standardized tests of language proficiency, aptitude, and language experience questionnaires) tested all informants of the pre-search pool on the imitation of completely foreign and unknown languages (L0) that none of the participants had any previous experience with: Hindi and Tamil. Furthermore, we strictly controlled for age of onset of second language learning and invited only late learners (age 10) to participate in the study, excluding “early” bilinguals. As robust stimuli to elicit the foreign language speech imitation capacity of the individuals we incorporated imitations on the word, sentence, and text level in four languages: 1. L1 German, 2. L2 English, 3. L0 Hindi and Tamil.
Our aim was to investigate the exact interplay between the behavioral, the neuro-functional (tested by functional magnetic resonance imaging, fMRI), and the neuro-anatomical/structural level (tested by VBM) in one and the same sample. Based on our own previous research (Reiterer et al., 2005a,b; Ackermann, 2008; Ackermann and Ziegler, 2010) and the literature (Just et al., 1996; Golestani et al., 2002, 2007; Amunts et al., 2004; Golestani and Zatorre, 2004; Mechelli et al., 2004; Perani and Abutalebi, 2006; Golestani and Pallier, 2007; Díaz et al., 2008; Moser et al., 2009; Orban et al., 2010) we hypothesized that higher ability individuals which would have reduced task effort which would be reflected in less extensive and less intense activation (consumption of less global workspace) in the areas most relevant for speech imitation/production (Fox et al., 2001; Clark and Wagner, 2003; Golestani and Zatorre, 2004; Ackermann, 2008; Cunillera et al., 2009; Eickhoff et al., 2009; Moser et al., 2009; Ackermann and Ziegler, 2010), the areas of the left prefrontal/premotor cortex, and left IPL. The left IPL has repeatedly been implicated as being an important relay station involved in multilingual language learning, proficiency, success, and even talent in second language learning, (Mechelli et al., 2004; Catani et al., 2005; Perani and Abutalebi, 2006; Golestani and Pallier, 2007; Richardson et al., 2010). On the neuro-anatomical level, we hypothesized that the opposite of this “less is more” principle would apply, so that increases in GM/WM would correlate with higher ability scores (“more is more”).
Materials and Methods
Participants – Behavioral Group
We pre-searched for late learning second language speakers with high, medium, and poor foreign speech imitation skills. All participants of the pre-search pool (N = 138) were German native speakers who learned English as their first L2 at around age 10 (all late learners). The age range of actual age at the time of investigation was between 20 and 40, with a mean overall age of 25.94 years. All were students or young academics and their educational background/field of study was balanced for linguistic experience, i.e., approximately half of them came from a language studying background (Table 1). They all knew at least one foreign language, which was English, 24% knew only one L2, 30% knew two L2s, 22% knew three, 17.5% knew four, 3.5% five, 2% six, and 1% nine foreign languages. Their mean exposure to formal school instruction in L2 (English) was 9.8 years. Most of them (73%) were clearly right-handed (Edinburgh Laterality Quotient, LQ: 1) with the overall mean LQ assuming 0.87, and the remaining 27% being dispersed over all LQ increments from −1 to +0.89. For all details of these parameters, see summary Table 1. The participants had no neurological disorders, and received financial remuneration for their participation after having given informed written consent to participate in the study. The study was approved by the local Ethics Committee and was in accordance with the Helsinki declaration.
Participants – MR Imaging Groups
After performing various behavioral tests, the Hindi imitation scoring and questionnaires (explained under the headings “behavioral testing”), 70 subjects were willing to and allowed to participate in further MR scanning (structural and/or functional). Six dropouts due to scanning artifacts were discarded. Of the remaining 64, we determined the upper and lower 15% to extract two extreme groups (N = 18), one for high ability (N = 9), and one for low ability (N = 9). The high and low ability groups are rather small, because these individuals are rare in the general population as well (e.g., Birdsong (1999, 2005) suggested that 15% of all adult L2 learners can be considered native-like). Thirty-six successfully completed the sentence and word imitation tasks (see fMRI task description below) in the scanner. Due to scanning time limitations, not all 64 MR scanned subjects were able to finish all the tasks. Thus smaller groups were selected for specific tasks (reaching 36 participants from the pool of 64 for “word and sentence imitation”).
All fMRI participants were strongly right-handed (see also Table 1). The behavioral parameters for the two extreme groups and the remaining participants are summarized in Table 1. Statistically significant differences between the extreme groups (High–Low) for these parameters are reduced to two scores: Hindi score (p = 0.000**) and score from English raters (English score) of their L2 English pronunciation (p = 0.024*, trend level considering multiple comparisons).
Behavioral Testing 1
Speech recordings and assessment
We recorded 138 participants in a sound-proof room at a phonetics laboratory while they performed different speech imitation, pronunciation, or reading tasks in German (L1), English (L2), and Hindi (L0). (For details of the different task types and elicitation techniques see Dogil and Reiterer, 2009). The task to elicit the English pronunciation skills of the participants was to read the well known story of the international phonetic alphabet (IPA) “The North wind and the Sun” in the best English accent they could “do.” They were free to choose/imitate the variant of English (either General American or British English, Received Pronunciation) they were most comfortable with, if they were able to discriminate between the two. For the unknown foreign language Hindi (L0) they had to repeat a model Hindi speaker who had previously been recorded in the sound-proof room. The imitations were based on four Hindi sentences of different length and phonetic complexity (7/7/9/11 syllables long) which had to be repeated immediately (direct imitation) after having been presented binaurally for three times. We repeated the stimulus sentences three times before imitation to ensure that everybody would produce the sentences or at least parts of them. A pilot experiment had shown that performance was very low for average individuals (N = 10) after only a single exposure to the stimulus sentence.
Assessment of the quality of speech imitation of these stimuli was performed with online (blind) native speaker ratings of the participants’ speech productions. Recordings were originally saved in wave format, but for the sake of speed of online access we transformed them into MP3 format when inserting them into the internet evaluation database. Feedback of the native raters was very positive and no loss in acoustic quality was reported. The raters were naïve with regard to phonetic or linguistic background and were instructed to transmit their global intuitive impression of whether the sample he/she was listening to could be spoken by a native speaker of English/Hindi or not. In order to confuse the raters and ensure the quality of the evaluation procedure, we randomly inserted recordings of native speakers who had imitated the speech samples into the database. English natives N = 13, Hindi natives N = 18. The speech samples were presented in random order. For the intuitive rating scale (Jilka, 2009) we used a rating bar to be clicked which ranged from “10” to “0” (most to least representation of “native-speaker-likeness”). In the case of English, 30 gender-balanced (15 females) English natives, and in the case of Hindi, 30 gender-balanced (15 females) Indian native speakers rated the all samples online using earphones.
Behavioral Testing 2
Additional behavioral questionnaires and tests were performed either online, where possible (e.g., questionnaires) or on site together with the recordings and MR scans. For language learning experience, participants had to provide a kind of “language learning resume” for each of their foreign languages known. They were subjected to the following further tests: 1. Non-verbal IQ (Raven Advanced Progressive Matrices; Raven et al., 1998); 2. Verbal IQ (Multiple Word Choice Test, MWT-B; Lehrl et al., 1995); 3. TOEFL subtest on English grammar (25 multiple choice questions on the “structure” of English); 4. Behavioral Inhibition System (from the BIS/BAS Test; Carver and White, 1994; see also Dogil and Reiterer, 2009); 5. Auditory Working Memory (Digit Span, Tewes, 1991) and German Non-word Repetition test, taken from an in-house syllable database developed according to German phonotactic rules, at the Institute of Natural Language Processing, University of Stuttgart (Benner, 2005).
FMRI Paradigm and Stimulus Material
In the event-related fMRI paradigm, two tasks (20 min each) were preformed, a “Sentence imitation” task (Sentence or “SIMI”) and a “Word imitation” task (“WORD”). The sentence imitation task was subdivided into two sub-conditions: (A) German (L1) and (B) English (L2) sentences, and the WORD task into the two sub-conditions (A) English (L2) and (B) Tamil (L0). We used Tamil inside the scanner (instead of Hindi) to again present a new unknown foreign language the subjects had never been exposed to. The auditorily presented sentences were all 11 syllables long and were balanced for syntactic complexity and semantic content. Fifty stimulus sentences were divided into 25 German and 25 English sentences (split into 13 with American and 12 with British accent). Mean sentence duration was 2.53 s. The 48 total stimulus words were all four-syllable nouns (mean length 0.80 s), matched for semantic content, and split into 24 Tamil words and 24 English (12 American, 12 British accent). In both tasks the requirement was to immediately imitate the presented stimulus with the best mimicking capacity at command. For acquisition, a sparse sampling paradigm was used (TR = 12 s, TA = 3 s, delay or “pause” = 9 s) with sentences/words presented and imitated during the scanner pauses. For a detailed and schematic description of the fMRI paradigm please see also Figure 1.
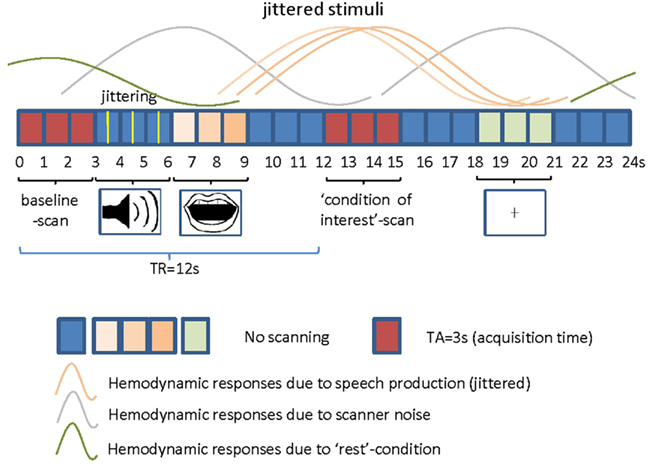
Figure 1. Functional magnetic resonance imaging task and paradigm: this figure shows the timing characteristics and jittered stimulus presentation of the sentence imitation task using a sparse sampling event-related paradigm. The timeline is given in seconds, TR (repetition time) = 12 s, TA (acquisition time) = 3 s, auditory sentence presentation = 3 s, sentence repetition following hearing the model sentence (yellowish color boxes) = 3 s. The yellow color boxes denote also the “condition of interest” which are captured at their BOLD peaks by the subsequent TA (red color boxes). Green colored boxes denote the scanned baseline/rest condition. The condition of interest (auditory presented sentences) was jittered at three different possible time points (3.5/4.5/5.5 s), see the yellow vertical lines in blue colored boxes. Different hemodynamic responses to the different events occurring during task are indicated by colored waves above the time line. Waves in yellowish colors denote hemodynamic responses due to speech production (condition of interest, jittered); wave in gray color denotes responses due to scanner noise and wave in green denotes hemodynamic responses due to “rest” condition.
The sparse sampling method was employed to avoid movement artifacts and to allow auditory control during sentence and word imitation. Stimuli were jittered and presented in pseudo-randomized order. Interstimulus baseline trials were inserted alternatingly every second TR accompanied by fixating a white cross on black screen. Each starting of a sentence was visually prepared by a different color screen and the imitation (speech production period) was visually co-triggered by a mouth symbol. The stimulus material was programmed and presented on the commercially available software “E prime” using a presentation laptop and a standard MR-compatible white screen the participants looked into via an inbuilt mirror system. Stimuli were binaurally presented over MR-compatible earphones (Sennheiser) and the produced speech was recorded by a commercially available MR-compatible optical microphone (company1). Before the start of the fMRI scanning session subjects were familiarized with sample stimuli.
MR Image Acquisition
For MR image acquisition, a Siemens Vision 1.5 T scanner was used. We did not go to higher field strength to reduce image artifacts induced by field inhomogeneity to obtain more reliable speech production data. For functional imaging (fMRI) of the blood oxygen level dependent (BOLD) signal, we used an EPI (echo planar imaging) Gradient Echo sequence with sparse sampling method set at the following parameters: TR = 12 s, TA = 3 s, delay in TR (pause) = 9 s, TE = 48 ms, slice number = 36 transversal, Flip angle (FA): 90°, Slice thickness = 3 mm + 1 mm gap, Voxel Size: 3 mm × 3 mm × 4 mm, field of view (FoV) = 192 mm × 192 mm × 143 mm, matrix = 64 × 64. The first three EPI data sets of each session were discarded prior to analysis to allow for T1-saturation effects.
For structural (anatomical) image acquisition, structural MRI scans of all subjects were performed on the same scanner, using a high resolution T1-weighted MDEFT sequence (Modified Driven-Equilibrium Fourier Transform), scan time = 12 min, repetition time (TR) = 7.92 ms; echo time (TE) = 2.48 ms; inversion time (TI) = 910 ms; FA = 16°; voxel size: 1 mm × 1 mm × 1 mm, FoV = 176 mm × 256 mm × 256 mm, slices per slab = 176 sagittal, matrix = 256 × 256. An eight-channel head coil was used.
fMRI Statistical Analysis
Functional magnetic resonance imaging images were analyzed using the free software packet SPM5 (Statistical Parametric Mapping2). Data pre-processing: each fMRI data set underwent spatial realignment by aligning the first scan from each session with the first scan of the first session and aligning the images within sessions with the first image of a particular session. The realigned data were spatially normalized to the standard Montreal neurological institute (MNI) T1 template, with the coregistered individual T1 image as a reference. Volumes were resliced to a voxel size of 3 mm × 3 mm × 3 mm, motion corrected and spatially smoothed using a 10-mm full-width at half-maximum Gaussian kernel and prepared for later random effects analyses.
At the first level, design matrices of individual general linear models incorporated two regressors of language type (English, Tamil) for the session word imitation, and two regressors of language type (English, German) for the session sentence imitation. Additional six regressors of movement parameters were added for each session as well. Regressors were defined with onsets at the time of appearance of the corresponding event and convolved with the canonical hemodynamic response function. At the second level, group analysis was performed using analysis of variance (ANOVA), with one between subject factor “ability group” (high versus low ability group) and one within-subjects factor “language type” (L1, L2) for each session. A third factor, “subject,” was added to the design matrix in order to remove variability as a result of differences in the participants’ average responses. Main effects for group and language type and the interaction effect of group by language type were calculated separately for each session. A statistical threshold of p < 0.05 (whole-brain cluster level correction for multiple comparisons) was obtained. Results were overlaid on the mean anatomical image and the rendered image of an SPM5 sample brain template.
VBM Structural Statistical Analysis
Image pre-processing
Structure images were first pre-processed by a skull-stripping software [Brain extraction tool v.2.1 (BET2) in FSL3], so that only the brain tissues remained in the images. Pre-processing and the statistical procedure was the same as in a previous paper (Hu et al., 2011). The following steps of image processing were performed by SPM5 executed in Matlab5 (MathWorks, Inc.). The origin of each image was manually set at the anterior commissure (AC). Then images were segmented into GM and WM using the unified segmentation (Ashburner and Friston, 2005) algorithm with a medium hidden Markov random field (HMRF) option in voxel-based-morphometry5 (VBM5) toolbox. The parameters of segmented images were used to generate a DARTEL template of the total sample (N = 68) by DARTEL toolbox (Ashburner, 2007). Then each segmented GM and WM map was modulated by this custom DARTEL template and also modulated by Jacobian determinant. Afterward, all the images as well as the DARTEL template were normalized to MNI space. As a final step, all normalized, segmented, modulated images were smoothed with an 8-mm FWHM isotropic Gaussian kernel.
VBM Statistics
Voxel-based multiple regression analysis (based on GLM) was carried out by SPM5 with voxel-wise GMV or WM volume (WMV) as dependent variable, the Hindi imitation score as a covariate of interest, with and without age, or total GMV (TGMV) as nuisance covariates in separated gender subgroups (male and female). Region of interest (ROI) analysis based on the fMRI results were performed. A statistical threshold of p < 0.05 (FWE corrected) was obtained. Results were overlaid on the mean anatomical image of the whole sample (N = 68).
Behavioral Statistical Analyses
For statistical analysis by means of Student’s t-tests for independent samples and bivariate correlation analyses of the behavioral data, the statistical software package SPSS was used.
For alpha level adjustments, we employed the Holm–Bonferroni correction procedure for multiple comparisons.
Results
Behavioral Results
First of all, we obtained evaluations of the Hindi imitations of our German test subjects (N = 138) by 30 gender-balanced Indian native speaker judges and found the scores of “imitating ability” to be normally distributed (see Figure 2, test for normality using Kolmogorov Smirnov, p = 0.74).
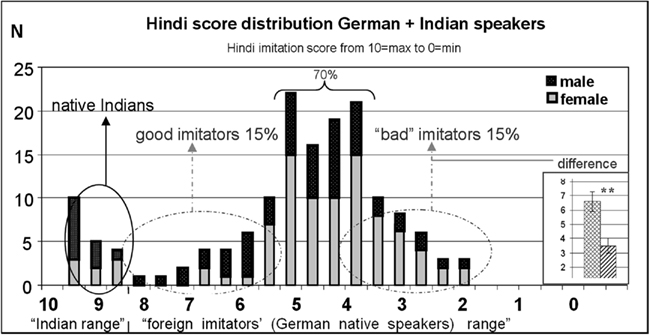
Figure 2. Hindi score distribution: Hindi speech imitation score (reflecting degree of “native-speaker-likeness”) distribution (as rated by 30 Indian native judges, 15 females) including the scores for the native Hindi speakers immersed into the German subject pool. German subjects, N = 138; immersed Indian natives N = 18; overall N = 156. Maximum score for sounding “native-like” Indian = 10; minimum vote = 0. 30 Indian (online) judges had to click an intuitive rating bar between 0 and 10 without demarcated increments to ensure intuitive and quick rating. The 18 Indian natives scored on the first 18 places. More German males (black) are amongst the good imitators (15%). Most German subjects scored average (70%). The score difference between the low ability “unsuccessful” 15% and the upper range or high ability 15% “successful” German imitators was significant at p = 0.000** (SD ±0.3 upper group; SD ±0.6 lower group).
The German test subjects’ mean score was 4.62, SD ±0.99, ranging from 2.42 (lowest score) to 7.74 (highest score) on a range from 0 = min to 10 = max. None of our German subjects ranged within the “native speaker” range (8–10 points) and none of them was at the lowest end (between 0 and 2). This shows that we did not test any speech impaired individuals and that the task was indeed extremely difficult so that no one could “fool” the native listener’s ear constantly over all four sentences (although for single sentences the Germans achieved scores up to 9.82). For defining our high and low ability groups for the further investigations we used the uppermost and lowest 15% of all participants, which resulted in extreme groups of 20 subjects, corresponding to the range between the first and second SD above and below the mean score (upper group: 5.7–8 points, lower group: 2–3.6 points). Seventy percent of the subjects (N = 97) formed the average group within 1 SD below and above the mean. To ensure the quality of the entire rating and detect outliers we had interspersed 18 native Hindi speakers into our speech database which was subjected to the online evaluation done by different (blind) Indian judges in India (N = 30). The 18 Hindi native speakers who imitated their own language were ranked along the first 18 places of the evaluation scoring between 8.07 and 9.9, SD ±0.6, mean: 9.5, females (N = 7) mean score: 9.4 (SD ±0.74), males (N = 11) mean score: 9.5 (SD ±0.5) showing no significant gender difference (t-test for independent samples, p = 0.44). For the German participants, however, a significant difference (p = 0.005, F = 2.12) between the group of females and males was found for mimicking capacity of Hindi (see Table 1; Figures 3 and 4). Amongst the highest scoring 10 subjects, the female/male ratio was 3/7, for the lowest 10 it was 7/3. For our internet based native speaker rating (30 English natives) based on L2 English text reading speech samples by our same subjects we also found this difference, namely the female participants scored lower than the males. In the case of English, 11 Germans (8%) came into the native range and succeeded in “fooling” the native ears.
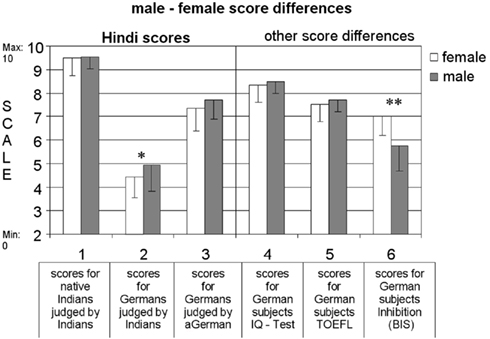
Figure 3. Gender differences: Hindi speech imitation (“Hindi scores,” 1–3) and other score differences [4–6; 4 = non-verbal IQ score; 5 = TOEFL grammar score; 6 = Behavioral Inhibition Score (BIS)] with respect to the male (gray bars)/female (white bars) differences. Scale for Hindi scores (1–3): 0 (min)–10 (max); scales of other scores (4–6) normalized. Mean Hindi score (y-axis) averaged over (1) 18 Indian native speakers (11 males/7 females) and in (2) + (3) over 138 German natives (53 males/85 females). In the case of the other scores (4–6) mean scores were averaged over N = 113 (German natives). Significant gender differences were obtained for (2), the Hindi score by the Indian native judges (see Figure 2): mean score males: 4.92 (SD ±1.0); females: 4.44 (SD ±0.89); group difference (t-test of independent samples): p = 0.005*; and for (6) the BIS score: mean score males: 5.6 (SD ±1.2); females: 7 (SD ±0.9); group difference: p = 0.000**. A minor difference at trend level resulted for (3) – the Hindi score as judged by a German expert phonetician. Mean score males: 7.7 (SD ±0.8); mean score females: 7.3 (SD ±0.9), group difference: p = 0.028.
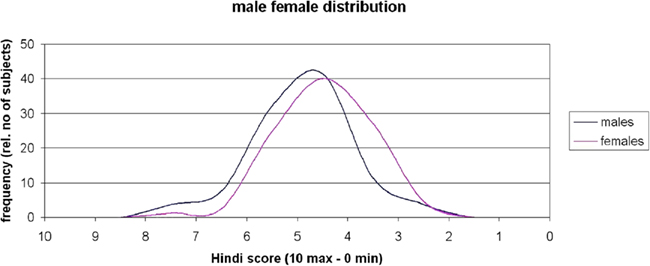
Figure 4. Gender differences distribution: this figure shows the score distributions of mimicking capacity of the Hindi sentences, separately for males and females. The distribution curve of the females is shifted slightly toward the lower score range, whereas the males’ distribution is shifted toward the higher scores. Scores as rated by native Hindi speakers are provided on the x-axis, relative number of participants (frequency) is given on the y-axis.
Additionally, an independent phonetic expert, a German phonetician, rated all our participants’ Hindi imitations: mean score 7.5 (range 4–9.2, SD ±0.9), mean score females: 7.3 (SD ±0.9), mean score males: 7.7 (SD ±0.8), resulting in a gender difference (p = 0.028, F = 1.7) at trend level (considering multiple comparisons). Although in absolute scores, he gave the participants much higher scores than did the Indian natives, the inter-rater correlation between his and the Indian natives’ evaluation is high: r = 0.6 (p = 0.000**), thus replicating the results of the native speaker rating.
Further linguistic and psychological control variables yielded the following results. From a sample of N = 113, we obtained additional test scores on the following variables: auditory working memory, general non-verbal IQ, verbal IQ, behavioral inhibition, number of foreign languages spoken, general experience with languages “linguistic expertise,” English grammar (TOEFL), English pronunciation as rated by native English speakers. Working memory (non-word repetition and digit span) correlated most significantly with the Hindi score: non-word repetition r = 0.37, p = 0.000**, digit span r = 0.36, p = 0.000**, followed by the English imitation skills as rated by native English judges: r = 0.3, p = 0.001**, and the results of the English grammar (TOEFL subtest) with r = 0.27, p = 0.004**. No significant (or low) correlations were obtained for Hindi score with non-verbal IQ (r = 0.1, p = 0.29); general linguistic experience (r = 0.01, p = 0.99); behavioral inhibition (r = 0.11, p = 0.22); number of foreign languages spoken (r = 0.16, p = 0.09); verbal IQ (r = 0.17, p = 0.07). The only variable amongst these additional ones (and not already reported above), which yielded a significant gender difference was the “behavioral inhibition score” (p = 0.000**, females: 21.8, SD ±3.6; males: 17.7, SD ±4, see Figure 3).
Brain Imaging Results
Neuro-functional (fMRI) results
According to the global main effects for each group during sentence processing (L1 and L2 mixed), a large bilateral speech–language network is activated in both groups comprising the auditory cortices (superior temporal gyri, Wernicke’s area), the inferior parietal areas, the postcentral “somatosensory” cortices, the motor and premotor areas surrounding the representation for the “mouth” area, including Broca’s area BA 44 and 45 as well as portions of the middle frontal gyri and insular cortex, the supplementary motor areas, the basal ganglia system (globus pallidus, putamen, and caudates), thalamus, the upper part of the cerebellar cortices, and parts of visual cortex.
One can already see by visual inspection only that the two groups (N = 9 + 9) do not differ so much in localization, but in the extent of activation, with the low ability imitation group showing more extended activation clusters, especially in the left-hemisphere.
To elucidate the exact group differences, we compared the groups by means of a two way ANOVA flexible factorial design (see Figure 5). When performing the comparison high versus low ability group, we found no significant “suprathreshold” voxels, because no BOLD activation remains for the high ability group. Only when performing the reverse comparison, low versus high ability group, did significant suprathreshold activation remain. The low ability group shows these clusters of activations primarily in a left dominant fronto-parietal network comprising the left motor/premotor cortex (predominantly BA 6) and left inferior frontal areas of Broca, the triangular, opercular as well as orbital parts (BA 44, 45, 47) in the frontal part of the network and the left inferior parietal lobe (ventral part of supramarginal gyrus, BA 40) plus adjacent dorsal areas in the inferior parietal lobule along the postcentral gyrus [somatosensory cortex (BA 1,2)] for the parietal part. The activations are relatively consistent and similar across the different languages (L1, L2, L0) and conditions (sentence and word imitation). The only notable difference is that for the less familiar language within each condition (i.e., L2 English in the sentence task or L0 Tamil in the word task, see section Materials and Methods), the low ability group activated an additional right hemispheric centro-parietal cluster around the rolandic operculum.
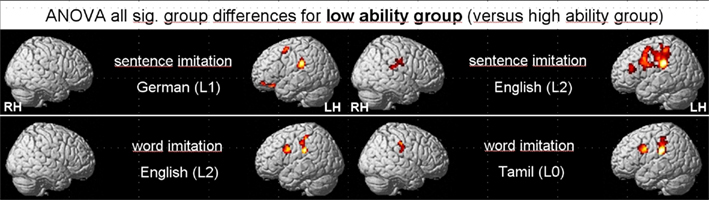
Figure 5. Functional magnetic resonance imaging differential effects for the low ability group (group versus group comparisons): upper panel: during (overt) “sentence imitation” in L1 (first language) German and L2 (second language) English. Lower panel: during (overt) “word imitation” in L2 English and “L0” (unknown language) Tamil. Flexible factorial ANOVA was used to perform the group versus group analyses. The comparison “high ability versus low ability group” yielded no significant remaining activations for the “high” (N = 9) group (no brain maps presented). The comparison depicted here represents “low ability versus high ability”: significant suprathreshold activations emerging for the low ability group (N = 9) – in “sentence imitation” (also in the case of L1 German), as well as in “word imitation.” A typical left-hemisphere dominant network comprising inferior parietal, premotor, and inferior frontal regions, emerges. In case of English in “sentence imitation” and Tamil in “word imitation” a right hemispheric centro-parietal cluster is additionally recruited. Statistical threshold: p < 0.05, whole-brain corrected for multiple comparisons at cluster level; cluster extent threshold: k = 60 voxels (p = 0.05).
In order to ensure the quality of the analyses comparing the extreme groups only, we performed an additional correlational analysis comprising a further 18 participants that we randomly selected from the mid-range group to counterbalance the 18 extreme group participants [N(total) = 36, 20 males]. In the correlational analyses (Figure 6) we obtained only significant negative correlations between BOLD signal changes (fMRI activation) and the Hindi imitation scores, but no significant positive correlations. In other words, the lower the scores in imitation ability, the higher the activation in certain areas, but with increasing ability scores (“accent talent”) we found no significantly activated areas. The locations of these activations exactly matched the areas in which we also found the individual differences in the group versus group comparison (Figure 5), featuring the network of a premotor/Broca cluster (BA 44/6) together with the left inferior parietal/postcentral cluster (BA 40).
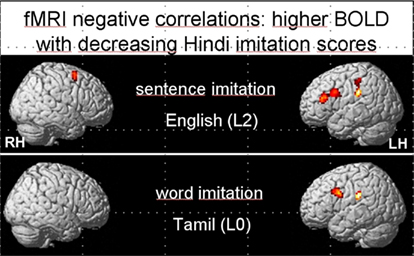
Figure 6. Functional magnetic resonance imaging correlation effects: when correlating the Hindi imitation scores with fMRI BOLD activation on a mixed ability group (N = 36), a significant negative correlation effect is obtained. Significant activations depicted here correlate negatively with the Hindi imitation score (decreasing imitation scores yield higher BOLD activation). The activated areas are largely overlapping with the activated areas found in the previous analyses (Figure 5). They are obtained in both tasks, “sentence imitation” (example upper panel) and “word imitation” (example lower panel). Statistical threshold: p < 0.05, whole-brain corrected for multiple comparisons at cluster level; cluster extent threshold: k = 64 voxels (p = 0.05).
Neuro-anatomical VBM results
Based on the results of the fMRI study and our basic hypothesis that the differences between the groups would be expected in a left fronto-parietal speech imitation network, we created a ROI for the more fine-grained VBM analyses (Figure 7). The ROI comprised one sphere (r = 12 mm) around the peak voxel of the frontal part of the network we found most activated: BA 44/6 (MNI coordinates [−54/6/30]) and one sphere (r = 15 mm) around the peak voxel of the inferior parietal cluster: BA 40 (MNI coordinates [−66/−30/27]. Because of reported differences between the male and the female brains, we analyzed the anatomical MRI data differently for each gender group (males N = 20, females N = 16). Within this ROI, we found a significant positive correlation between GMV and increased Hindi imitation scores in exactly the same cluster that we have also found to be more significantly activated in the low ability group in the functional analyses: the premotor (BA 6)/Broca, opercular part (BA 44), in combination with the left IPL, supramarginal gyrus (BA 44). The significant [after FWE (Family Wise Error) correction for multiple comparisons] increase of GMV with higher imitation scores occurred only in the subgroup of males. This result remained the same for several variants of covariates of non-interest analyzed: if controlled for 1. “0” covariates, 2. “age,” and 3. “TGMV” (TGMV) as covariate. For WM differences in the male group, or either white/GM differences in the female subgroup, we found no further significant correlations within this region.
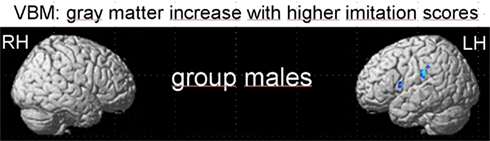
Figure 7. Structural MR correlation effects (VBM): correlation of Hindi imitation score with gray matter volume yields a positive correlation in the male (N = 20) subgroup; i.e., higher imitation scores correlate with increased gray matter volume in the left inferior parietal cortex (supramarginal gyrus; peak MNI voxel coordinates [−68/−24/29]) and left inferior frontal–premotor area (BA 44/BA 6; peak voxel coordinates [−59/8/11]). Statistical threshold: p < 0.05, corrected for multiple comparisons at FWE level within two regions of interest (ROIs) based on our fMRI results, an inferior left frontal ROI-sphere (r = 12 mm) around [−54/6/30] and a left inferior parietal ROI-sphere (r = 15 mm) around [−66/−30/27].
Summarizing the results of our study, both on the behavioral, neuro-functional, and -anatomical level, we found the most striking differences between the high and low ability accent imitators – according to the untrained language “Hindi” imitation scores – within a network comprising the left premotor cortex (BA 6) plus Broca, opercular part (BA 44), as well as the left inferior parietal area (BA 40, supramarginal gyrus). The low ability imitators activated this left hemispheric network significantly more than their “talented” counterparts. A correlation of BOLD activation with the Hindi imitation scores demonstrated that decreased scores (lower ability) evoked more activation in these left hemispheric areas, with females showing a slightly higher (negative) correlation for the fronto-premotor (BA 44/6) cluster than males (Figure 8). However, the correlational analysis based on the neuro-anatomical data showed the reverse pattern, increased GMV with higher imitation scores (higher ability). This significant positive correlation was only evident for the male subgroup in both clusters of the network: the inferior parietal and the fronto-premotor peak area. Thus, an increase in the anatomical measure “GMV” was accompanied with a decrease of activation on the functional level in motor speech areas, reflecting individual differences in speech imitation ability.
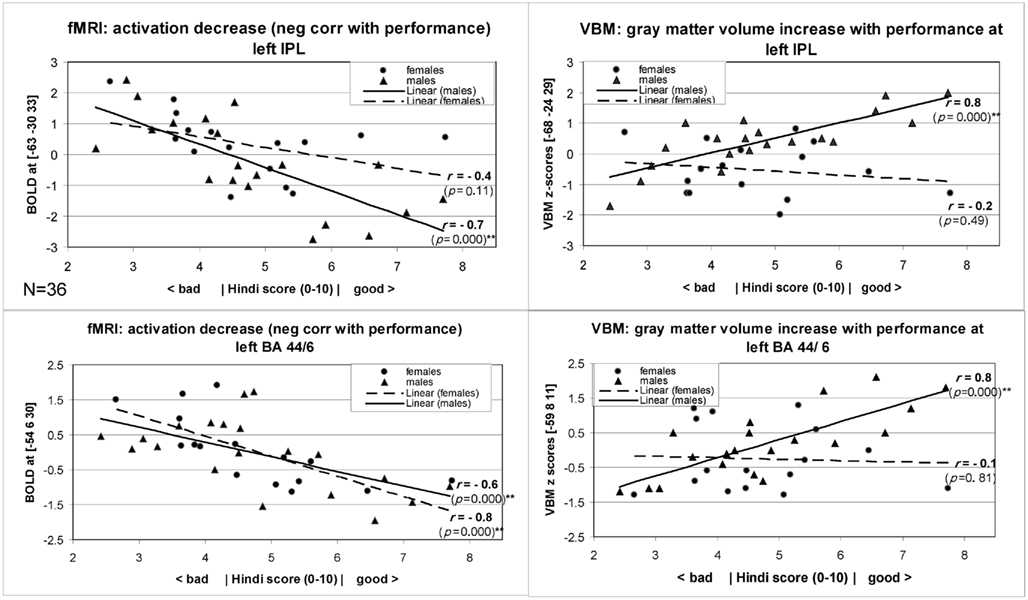
Figure 8. Functional (fMRI) and structural (VBM) correlation effects in the peak clusters: left side of figure shows fMRI (BOLD) activation decrease with higher Hindi imitation scores at left inferior parietal lobe (IPL, upper picture) and left inferior frontal lobe (BA 44/6) respectively (lower picture). Contrastingly, right side of figure shows the structural (VBM) results; i.e., a gray matter volume increase, within the same clusters (left IPL - upper picture, left IFG lower picture), with Hindi imitation scores, however, is significant only for the male group (black solid lines). Males are represented by regression lines in black and black triangles, females by gray dashed lines and gray dots.
Discussion
The results of our study point to a distinct neuro-functional/neuro-anatomical signature of speech imitation ability (aptitude): “pronunciation/speech imitation talent” was found to be associated with less hemodynamic activation together with higher amounts of GMV within a left-hemisphere perisylvian network, including premotor cortex (Broca) and inferior parietal lobe.
At the neuro-functional level (fMRI), we observed a clear-cut difference between low and high ability speakers as a function of their imitation ability: low ability imitators showed significantly higher amounts of activation and more extended clusters during sentence and word imitation. These findings are in accord with previous studies suggesting increased ”cortical effort” in lower proficiency L2 speakers in terms of “neuro-functional compensation mechanisms” or “consumption of global workspace” (Just et al., 1996; Reiterer et al., 2005b; Moser et al., 2009). As a novel aspect, all languages tested (L1, L2, and L0) seem to be affected by this principle in similar ways with a gradual increase from the “easiest” (L1, German) to the most “difficult” language (L0, Tamil). Conceivably, thus, even the native tongue was neuro-functionally differently processed by the poor (Hindi) mimics, pointing to a general underlying articulation capacity less dependent on immediate training, since our participants had had no prior experience with Hindi. Evidence is accumulating that there are high similarities between L1 and L2 phonetic processing dependent on either level of expertise or the pre-existential ability/capacity of the speaker (Golestani and Zatorre, 2004; Díaz et al., 2008; Grogan et al., 2009; Skehan, 2011). This corroborates our finding that individually different processing strategies are reflected (important) more strongly neuro-functionally than the different languages being processed distinctly in the brain, even if they are systems typologically as diverse as German and Hindi.
Employing fMRI we could show that individual differences in speech imitation ability are reflected by increased activation in the speech motor relevant areas. Our data point to considerable individual differences in the way the speech motor network is engaged during actual speech imitation and production. We found two areas to be most relevant: a premotor cluster, reflecting the speech motor execution of the articulatory movements (the “parroting part”) and second, the phonological loop mechanism of the acoustic working memory which integrates the phonological stream with the articulation output, located in the left inferior parietal area (the “phonology part”). The phonological loop is used for short term retention of verbal information and is a necessary prerequisite for later imitation of verbal material (Gathercole, 2006). We do not want to dissect these two components/areas, the frontal and the parietal cluster, into a production and perception component, because it becomes increasingly clear that there is extensive overlap between production and perception in each of these areas (Price et al., 2005; Hickok and Poeppel, 2007; Reiterer et al., 2008; Eickhoff et al., 2009).
An alternative line of discussion regarding these two clusters in speech perception/production and imitation comes from the concept of the so-called “mirror neuron system,” increasingly used to explain speech processing as well as language evolution in humans. Recent evidence (Aziz-Zadeh and Ivry, 2009; D’Ausilio et al., 2009; Gazzola et al., 2006) points to an existence of a specific left lateralized auditory mirror neuron system engaged in auditorily triggered speech imitation comprising predominantly and exactly these two clusters we found to be more active in “poor” speech imitators.
The left IPL is not only an eminent hub area for phonological working memory, phonemic awareness, speech production/perception integration, but has also been found to play an essential role in foreign language learning, even once explicitly called a “language talent area” (Poetzl, 1929; Perani and Abutalebi, 2006).
As far as the neuro-anatomical results of our study are concerned, it becomes increasingly clear that higher skills or ability are accompanied by increases in either white or GM density or volume and the reverse, i.e., decreased volume is reported to be a marker of lower abilities or even neurological disorders (either generally as well as specifically with respect to second language skills, Mechelli et al., 2004; Golestani and Pallier, 2007; Golestani et al., 2007; McAlonan et al., 2008; Richardson et al., 2010). Correlations between neuro-anatomical structures and higher performance skills in foreign languages have been reported. For example, an exceptional general language learning “talent,” as exemplified by a hyper polyglot (60 languages spoken) post mortem male brain was reported to show significantly diverse cytoarchitectural (cell) structures in Broca’s area as a signature of his outstanding foreign language skills (Amunts et al., 2004). More specifically increased GM/WM density or volume (especially within the inferior parietal areas) have been reported to reflect higher performance related to either increased proficiency levels in a second language (Mechelli et al., 2004) or capacity/success in perceiving (Golestani et al., 2002, 2007) or producing (Golestani and Pallier, 2007) foreign language speech sounds.
However, part of our results, the increased GMV in left inferior parietal and prefrontal regions, was only found for the male subgroup. Whether this reflects a simple sample problem, meaning that by chance we had too few high talented females in our random, but already huge sample pool and therefore the effect of imitation talent could only emerge in the male subgroup, or there are biological gender differences at the basis of speech imitation capacity is unclear. Yet another possibility of interpretation emerges, namely, whether this result is a consequence of lack of gender-balanced educational or even social systems in Germany. It is noteworthy in this context to repeat that females also scored higher on the “inhibition score.” The gender difference result of our study would remain to be clarified by future research.
Since our behavioral speech imitation data showed a significant gender difference (higher scores for the male imitators), we would like to provide a few possible lines of explanation for this phenomenon. This result was unexpected since traditionally the literature attributed an advantage for second language learning to females (for critical reviews of this issue see Ullman et al., 2008; Chiarello et al., 2009; Wallentin, 2009). However, here we did not investigate language learning in all linguistic subsystems, but focused only on speech-sound audio–vocal imitation. The task essentially required a speech motor imitation skill which did not involve “language” planning (e.g., semantics, declarative memory). It was almost devoid of syntactic and semantic operations. When testing for pure motor skill learning, recent evidence (Dorfberger et al., 2009) could show that males have a significant advantage over females in motor skill learning.
Additionally, there is reported anecdotal superiority of males over females when rare and exceptional high talent in foreign language learning (including native-like accent) is concerned. So-called “hyper polyglots” (Erard, in press) who know between 10 and 50 languages fluently, or parodists, mimics, and impersonators are usually predominantly male. Hypothetically speaking, this phenomenon also reminds one of evolutionary Darwinian theories of speech origin, namely, sexual selection bearing a possible hidden driving force behind predominantly male song performance, as is the case in most songbird species (Fitch, 2005, 2010).
The gender bias, however, would be consistent with the emerging evidence in the field of giftedness research which shows that gender differences are observed to be larger and more pronounced in gifted (the upper end of the scale) than in average ability individuals (Preckel et al., 2008). This fits well with evolutionary theories which see males as more represented in the extremes of the normal distribution curve, whereas females form the main representation toward the mean (with respect to any kinds of abilities). Like male predominance in the upper end of the ability scale in gifted populations, but in the opposite direction, many developmental and acquired disorders, like, for example – disorders of the voice and tone-deafness – are more prevalent in males than in females (Howard and Angus, 1998).
Whether this discrepancy is still the effect of a bias of educational traditions in our societies or rooted in biology requires future clarification.
Conclusion
In this combined behavioral and brain imaging study we investigated the neuro-functional and neuro-anatomical correlates of individual differences in speech imitation/pronunciation ability.
Having excluded the confounding factors of age of onset of foreign language learning and exposure/linguistic experience as influencing variables by extensive pre hoc behavioral testing, we could pin down the neurological signatures related to individual differences in speech imitation talent to two areas in the brain on a functional as well as anatomical level. Lower amounts of activation, accompanied by increased volumes in GM in a left premotor cluster including Broca’s area (BA 44/6) and the left inferior parietal lobe (BA 40) characterized high ability in second language speech imitation.
Conflict of Interest Statement
The authors declare that the research was conducted in the absence of any commercial or financial relationships that could be construed as a potential conflict of interest.
Acknowledgments
Special thanks go to our Indian collaborators who helped with the realization of the Hindi rating procedures: Vinod Kumar (University of Tübingen), Susanta Roy (Dimapure, Bioexplore India), Nandini C Singh (NBRC India). We are particularly grateful to Ardith Meier for reading through the manuscript from the viewpoint of a native speaker. We want to further thank Mathias Röger, Maike Borutta, and Franziska Hösl for their medical technical assistance with MR data acquisition, Arev Anus for her special help with organization of the participants, Kurt Prangenberg, Bernd Kardatzki, and André Blessing for database assistance, Henrike Baumotte, Natalie Lewandowska, Olga Anufryk, and Jagoda Sieczkowska for assisting with speech recordings at the phonetics lab of the institute for Natural Language Processing, Experimental Phonetics Group and the head of the department himself, Grzegorz Dogil, University of Stuttgart). Further thanks go to Simon Eikhoff for methodological assistance, and to Ingo Hertrich and Jens Maier for discussions. This research has been funded by the German Research Foundation (DFG grant AC 55/7-1). Author contributions: Susanne Reiterer, Hermann Ackermann, Susanne Maria Winkler (manuscript writing and organization), Xiaochen Hu, Susanne Maria Reiterer, Michael Erb (data analysis), Susanne Maria Reiterer, Xiaochen Hu, M. J., Giuseppina Rota, Davide Nardo, Wolfgang Grodd, Hermann Ackermann (contributing to planning and preparations of the experiments and study designs).
Footnotes
References
Abrahamsson, N., and Hyltenstam, K. (2008). The robustness of aptitude effects in near-native second language acquisition. Stud. Second Lang. Acquis. 30, 481–509.
Ackermann, H. (2008). Cerebellar contributions to speech production and speech perception: psycholinguistic and neurobiological perspectives. Trends Neurosci. 31, 265–272.
Ackermann, H., and Ziegler, W. (2010). “Brain mechanisms underlying speech motor control,” in The Handbook of Phonetic Sciences, 2nd Edn, eds W. J. Hardcastle and J. Laver (Malden, MA: Blackwell), 202–250.
Amunts, K., Schleicher, A., and Zilles, K. (2004). Outstanding language competence and cytoarchitecture in Broca’s speech region. Brain Lang. 89, 346–353.
Aziz-Zadeh, L., and Ivry, R. B. (2009). The human mirror neuron system and embodied representations. Adv. Exp. Med. Biol. 629, 355–376.
Benner, U. (2005). Syllables in Speech Production – A Study of the Mental Syllabary. Linguistic Master Thesis at the University of Stuttgart, Stuttgart.
Birdsong, D. (1999). “Introduction: whys and why nots of the critical period hypothesis for second language acquisition,” in Second Language Acquisition and the Critical Period Hypothesis, ed. D. Birdsong (Mahwah, NJ: Erlbaum), 1–22.
Birdsong, D. (2005). “Interpreting age effects in second language acquisition,” in Handbook of Bilingualism: Psycholinguistic Perspectives, eds J. Kroll and A. De Groot (New York: Cambridge University Press), 109–127.
Birdsong, D. (2006). Age and second language acquisition and processing: a selective overview. Lang. Learn. 56, 9–49.
Carver, C. S., and White, T. L. (1994). Behavioral inhibition, behavioral activation, and affective responses to impending reward and punishment: the BIS/BAS scales. J. Pers. Soc. Psychol. 67, 319–333.
Catani, M., Jones, D. K., and ffytche, D. H. (2005). Perisylvian language networks of the human brain. Ann. Neurol. 57, 8–16.
Chiarello, C., Welcome, S. E., Halderman, L. K., Towler, S., Julagay, J., Otto, R., and Leonard, C. M. (2009). A large-scale investigation of lateralization in cortical anatomy and word reading: are there sex differences? Neuropsychology 23, 210–222.
Clark, D., and Wagner, A. D. (2003). Assembling and encoding word representations: fMRI subsequent memory effects implicate a role for phonological control. Neuropsychologia 41, 304–317.
Cunillera, T., Càmara, E., Toro, J. M., Marco-Pallares, J., Sebastián-Galles, N., Ortiz, H., Pujol, J., and Rodríguez-Fornells, A. (2009). Time course and functional neuroanatomy of speech segmentation in adults. Neuroimage 48, 541–553.
D’Ausilio, A., Pulvermüller, F., Salmas, P., Bufalari, I., Begliomini, C., and Fadiga, L. (2009). The motor somatotopy of speech perception. Curr. Biol. 19, 381–385.
Díaz, B., Baus, C., Escera, C., Costa, A., and Sebastián-Gallés, N. (2008). Brain potentials to native phoneme discrimination reveal the origin of individual differences in learning the sounds of a second language. Proc. Natl. Acad. Sci. U.S.A. 105, 16083–16088.
Dogil, G., and Reiterer, S. (2009). Language Talent and Brain Activity. Berlin, NY: Mouton de Gruyter.
Dorfberger, S., Adi-Japha, E., and Karni, A. (2009). Sex differences in motor performance and motor learning in children and adolescents: an increasing male advantage in motor learning and consolidation phase gains. Behav. Brain Res. 198, 165–171.
Eickhoff, S. B., Heim, S., Zilles, K., and Amunts, K. (2009). A systems perspective on the effective connectivity of overt speech production. Philos. Transact. A Math. Phys. Eng. Sci. 367, 2399–2421.
Fitch, W. T. (2005). The evolution of music in comparative perspective. Ann. N. Y. Acad. Sci. 1060, 29–49.
Fox, P. T., Huang, A., Parsons, L. M., Xiong, J. H., Zamarippa, F., Rainey, L., and Lancaster, J. L. (2001). Location-probability profiles for the mouth region of human primary motor-sensory cortex: model and validation. Neuroimage 13, 196–209.
Gathercole, S. (2006). Nonword repetition and word learning: the nature of the relationship. Appl. Psycholinguist. 27, 513–543.
Gazzola, V., Aziz-Zadeh, L., and Keysers, C. (2006). Empathy and the somatotopic auditory mirror system in humans. Curr. Biol. 16, 1824–1829.
Geschwind, N., and Galaburda, A. M. (1985). Cerebral lateralization: Biological mechanisms, associations, and pathology. 2. a hypothesis and a program for research. Arch. Neurol. 42, 521–552.
Golestani, N., Molko, N., Dehaene, S., LeBihan, D., and Pallier, C. (2007). Brain structure predicts the learning of foreign speech sounds. Cereb. Cortex 17, 575–582.
Golestani, N., and Pallier, C. (2007). Anatomical correlates of foreign speech sound production. Cereb. Cortex 17, 929–934.
Golestani, N., Paus, T., and Zatorre, R. J. (2002). Anatomical correlates of learning novel speech sounds. Neuron 35, 997–1010.
Golestani, N., and Zatorre, R. J. (2004). Learning new sounds of speech: reallocation of neural substrates. Neuroimage 21, 494–506.
Grogan, A., Green, D. W., Ali, N., Crinion, J., and Price, C. (2009). Structural correlates of semantic and phonemic fluency ability in first and second languages. Cereb. Cortex 19, 2690–2698.
Hickok, G., and Poeppel, D. (2007). The cortical organization of speech processing. Nat. Rev. Neurosci. 8, 393–402.
Howard, D. M., and Angus, J. A. (1998). A comparison between singing pitching strategies of 8 to 11 year olds and trained adult singers. Logoped. Phoniatr. Vocol. 22, 169–176.
Hu, X., Erb, M., Ackermann, H., Martin, J., Grodd, W., and Reiterer, S. (2011). Voxel-based morphometry studies of personality: issue of statistical model specification – effect of nuisance covariate. Neuroimage 54, 1994–2005.
Jilka, M. (2009). “Assessment of phonetic ability,” in Language Talent and Brain Activity, eds G. Dogil and S. M. Reiterer (Berlin, NY: Mouton de Gruyter), 17–66.
Just, M. A., Carpenter, P., Keller, T., Eddy, W., and Thulborn, K. (1996). Brain activation modulated by sentence comprehension. Science 274, 114–116.
Lehrl, S., Triebig, G., and Fischer, B. (1995). Multiple choice vocabulary test MWT as a valid and short test to estimate premorbid intelligence. Acta Neurol. Scand. 91, 335–345.
McAlonan, G. M., Suckling, J., Wong, N., Cheung, V., Lienenkaemper, N., Cheung, C., and Chua, S. E. (2008). Distinct patterns of grey matter abnormality in high-functioning autism and Asperger’s syndrome. J. Child Psychol. Psychiatry 49, 1287–1295.
Mechelli, A., Crinion, J., Noppeney, U., O’Doherty, J., Ashburner, J., Frackowiak, R., and Price, C. (2004). Neurolinguistics: structural plasticity in the bilingual brain. Nature 431, 757.
Moser, D., Fridriksson, J., Bonilha, L., Healy, E. W., Baylis, G., Baker, J. M., and Rorden, C. (2009). Neural recruitment for the production of native and novel speech sounds. Neuroimage 46, 549–557.
Nauchi, A., and Sakai, K. L. (2009). Greater leftward lateralization of the inferior frontal gyrus in second language learners with higher syntactic abilities. Hum. Brain Mapp. 30, 3625–3635.
Obler, L. K., and Fein, D. (1988). The Exceptional Brain: The Neuropsychology of Talent and Special Abilities. New York: Guilford Press.
Orban, P., Peigneux, P., Lungu, O., Albouy, G., Breton, E., Laberenne, F., Benali, H., Maquet, P., and Doyon, J. (2010). The multifaceted nature of the relationship between performance and brain activity in motor sequence learning. Neuroimage 49, 694–702.
Perani, D., and Abutalebi, J. (2006). The neural basis of first and second language processing. Curr. Opin. Neurobiol. 15, 202–206.
Poetzl, O. (1929). Aphasie und mehrsprachigkeit. Zeitschrift für die gesamte Neurologie und Psychiatrie 124, 145–162.
Preckel, F., Goetz, T., Pekrun, R., and Kleine, M. (2008). Gender differences in gifted and average-ability students. Gift. Child Q. 52, 146–159.
Price, C., Thierry, G., and Griffiths, T. (2005). Speech specific auditory processing: where is it? Trends Cogn. Sci. 9, 271–276.
Raven, J., Raven, J. C., and Court, J. H. (1998). Manual for Raven’s Advanced Progressive Matrices. Oxford: Psychologists Press.
Reiterer, S., Berger, M. L., Hemmelmann, C., and Rappelsberger, P. (2005a). Decreased EEG coherence between prefrontal electrodes: a correlate of high language proficiency? Exp. Brain Res. 163, 109–113.
Reiterer, S., Hemmelmann, C., Rappelsberger, P., and Berger, M. L. (2005b). Characteristic functional networks in high- versus low-proficiency second language speakers detected also during native language processing: an explorative EEG coherence study in 6 frequency bands. Brain Res. Cogn. Brain Res. 25, 566–578.
Reiterer, S., Erb, M., Grodd, W., and Wildgruber, D. (2008). Cerebral processing of timbre and loudness: fMRI evidence for a contribution of Broca’s area to basic auditory discrimination. Brain Imaging Behav. 2, 1–10.
Richardson, F. M., Thomas, M. S., Filippi, R., Harth, H., and Price, C. (2010). Contrasting effects of vocabulary knowledge on temporal and parietal brain structure across lifespan. J. Cogn. Neurosci. 22, 943–954.
Skehan, P. (2011) “Language Aptitude,” in The Routledge Handbook of Second Language Acquisition, Chap. 23, eds S. Gass and A. Mackay (London: Routledge). (in press).
Ullman, M. T., Miranda, R. A., and Travers, M. L. (2008). “Sex differences in the neurocognition of language,” in Sex Differences in the Brain: From Genes to Behavior, eds J. Becker, K. J. Berkley, N. Geary, E. Hampson, J. P. Herman, and E. Young (Oxford, NY: Oxford University Press), 291–310.
Keywords: speech imitation ability, language aptitude, fMRI, VBM, second language acquisition, individual differences, late bilinguals, foreign accent
Citation: Reiterer SM, Hu X, Erb M, Rota G, Nardo D, Grodd W, Winkler S and Ackermann H (2011) Individual differences in audio-vocal speech imitation aptitude in late bilinguals: functional neuro-imaging and brain morphology. Front. Psychology 2:271. doi: 10.3389/fpsyg.2011.00271
Received: 18 February 2011;
Accepted: 28 September 2011;
Published online: 28 October 2011.
Edited by:
Guillaume Thierry, Bangor University, UKReviewed by:
Stefano F. Cappa, Vita-Salute San Raffaele University, ItalyCesar Avila, Universitat Jaume I, Spain
Copyright: © 2011 Reiterer, Hu, Erb, Rota, Nardo, Grodd, Winkler and Ackermann. This is an open-access article subject to a non-exclusive license between the authors and Frontiers Media SA, which permits use, distribution and reproduction in other forums, provided the original authors and source are credited and other Frontiers conditions are complied with.
*Correspondence: Susanne Maria Reiterer, Section of Experimental MR of the CNS, Centre for Integrative Neuroscience, University of Tübingen, Hoppe Seyler Strasse 3, 72076 Tübingen, Germany. e-mail:c3VzYW5uZS5yZWl0ZXJlckBtZWQudW5pLXR1ZWJpbmdlbi5kZQ==;cy5yZWl0ZXJlckBnbXguZGU=;c3VzYW5uZS5yZWl0ZXJlckB1bml2aWUuYWMuYXQ=