- Cognition and Interaction Lab, School of Humanities and Informatics, University of Skövde, Skövde, Sweden
In this article, we review the nature of the functional and causal relationship between neurophysiologically/psychologically generated states of emotional feeling and action tendencies and extrapolate a novel perspective. Emotion theory, over the past century and beyond, has tended to regard feeling and action tendency as independent phenomena: attempts to outline the functional and causal relationship that exists between them have been framed therein. Classically, such relationships have been viewed as unidirectional, but an argument for bidirectionality rooted in a dynamic systems perspective has gained strength in recent years whereby the feeling–action tendency relationship is viewed as a composite whole. On the basis of our review of somatic–visceral theories of feelings, we argue that feelings are grounded upon neural-dynamic representations (elevated and stable activation patterns) of action tendency. Such representations amount to predictions updated by cognitive and bodily feedback. Specifically, we view emotional feelings as minimalist predictions of the action tendency (what the agent is physiologically and cognitively primed to do) in a given situation. The essence of this point is captured by our exposition of action tendency prediction–feedback loops which we consider, above all, in the context of emotion regulation, and in particular, of emotional regulation of goal-directed behavior. The perspective outlined may be of use to emotion theorists, computational modelers, and roboticists.
Historical Background – On Feelings and Actions
“[c]ommon sense says, we lose our fortune, are sorry and weep; we meet a bear, are frightened and run; we are insulted by a rival, are angry and strike … this order of sequence is incorrect … the more rational statement is that we feel sorry because we cry, angry because we strike, afraid because we tremble,”
(James, 1890, p. 449, James’ italics).
The above view of the pioneering emotions researcher William James provided a landmark in the understanding of the role of bodily feedback in feelings and decision making in the context of overt behavior. It has been considered counter-intuitive to the psychological interpretation of emotional experience1. The Jamesian view has since been conceived as a pioneering somatic theory of emotion, contrasting with the more abundant cognitive theories of contemporary emotion research, since bodily changes were considered compositional to, rather than independent of (and secondary to), the emotion. The Jamesian perspective can also be considered a “(somatic) feeling theory of emotion” (Prinz, 2004, p. 5) where feelings are comprised of bodily changes that follow “perception of the exciting fact.” The purported causal role of action-instigated bodily feedback in feeling together with the concept of “feeling as the emotion” had at least two important implications for the functional role of emotions: firstly, if the emotion follows action, what useful role, if any, does it have in higher cognitive and behavioral activity, e.g., decision making? Secondly, if there is no role for cognition in triggering the bodily changes that comprise the emotional state, what is the trigger mechanism?
Following the behaviorist and cognitivist revolution of the early to mid twentieth century, interest in affective and emotional processes and their role in conscious experience and behavior waned (cf. LeDoux, 1996) as they were not considered functional to classically conceived rational cognitive processes, e.g., planning, decision making, attention, learning. Nevertheless, James had seeded a prospective debate that would be embarked on in the second half of the twentieth century concerning the causal and functional relationship existing between emotional feelings and their associated actions. From the 1960s, interest in emotion per se was galvanized, and a popular conception viewed them as part and parcel of functional behavioral activity in the context of cognitive appraisals (Arnold, 1960). Early “appraisal theory,” however, still had a very much cognitivist flavor whereby emotions were considered hot action responses or tendencies triggered by, and independent from (secondary to), cold cognitive perceptual judgments of the significance of stimuli to the well-being of the organism (effective triggers).
In recent years, appraisal theory has evolved to incorporate a bidirectional perspective on the relationship between (cognitive) appraisal and emotion rooted in the dynamics and neural representation of action tendency. Such views include: (1) embodied appraisals (cf. Prinz, 2004, 2005), and (2) process models of appraisal (cf. Scherer, 1984, 2000, 2009; Ellsworth and Scherer, 2003). In the case of perspective (1), neural patterns reflective of changes in the internal milieu and skeletomusculature serve as embodied appraisals (perceptions and feelings). These appraisals track the relevance of an external stimulus to the well-being of the organism thereby establishing organism–environment relations – “core relational themes” (Lazarus, 1991) – and are informative insofar as they register bodily changes that constitute action tendencies (see Lowe et al., 2007 for discussion of this perspective). In the case of (2), affective action tendencies contribute to ongoing and context-elaborated appraisals. These appraisals manifest according to a dynamic relationship (reciprocal modulation) among the constitutive components that include “monitoring/feeling state” and “motivation/action tendencies.” Such process models are, nevertheless, purely cognitive regarding the early stages of stimulus appraisal processing. Recently, Lewis (2005) also posited a dynamic systems (DS) approach whereby feeling and action tendencies are intricately interwoven2, producing synchronized and stable global-orienting states (emotion–appraisal amalgams) the “substrate” on which higher emotional–cognitive activity (e.g., attentional orientation, learning) operates. In this view, no causal precedence for either purely cognitive or emotion processes exists.
Mirroring the development of embodied/somatic and DS appraisal theoretic accounts of emotional–cognitive activity, much research into the neurophysiology underlying affective phenomena has emphasized the complex and integrated role of feelings and action tendencies. In the spirit of James, somatic emotion theorists have identified feelings as being developmentally grounded in bodily changes that prefigure behavior. Some of these theorists have emphasized the significance of skeletomuscular feedback to the generation of feeling. In line with James’ central argument, Bem (1972), Ekman (1972, 2003), Laird (2007) have argued for the sufficiency to feeling generation of feedback from facial, vocal, and postural expression. In the view of Laird, error-based feedback can provide a critical “cybernetics control process” function for “shaping” behavior. Damasio (1994, 1999) and Bechara (2004), however, have been the chief architects of re-establishing the Jamesian-somatic (or, perhaps more accurately, somatic–visceral) feeling perspective in psychology and neuroscience. Their perspective converges with that of the above-mentioned theorists. Nevertheless, (somatic–visceral) bodily feedback is not considered necessary for feeling generation. In their view, consistent with the feedback–feeling sufficiency argument, bodily changes to the organism (above all internal milieu and skeletomusculature), in some circumstances, precede registrations in the brain that correlate with emotional experience (feelings) – Damasio termed this stimulus processing route the “body loop” (Damasio, 1994). This loop is enacted in circumstances of uncertainty or prior to learning stimulus significance. Critically, however, Damasio posited that emotional feeling states can occur prior to such bodily changes following learning. In this case, brain areas implicated in providing the neural substrate for feelings are activated in parallel with (or in the absence of) the slower dynamics of bodily change activated and fed back (to the central nervous system) through the conduit of the peripheral nervous system. Damasio termed this processing route the “as-if body loop” (Damasio, 1994, 1999, 2003, 2010; also see Bechara, 2004). In the Damasio/Bechara perspective, therefore, emotional feelings can be both primary, and secondary, to somatic–visceral bodily changes.
On the basis of the evidence outlined above and in contradistinction to James, emotional feelings may be derived from both feedback and anticipation of bodily change involved in overt behavioral–expressive activity. In line with these findings, contemporary researchers of emotion regulation, have considered both feedback and anticipation as mechanisms critical to context-appropriate behavior and expression (particularly with respect to social context). For example, Baumeister et al. (2007) have suggested that emotion serves as an anticipatory-feedback system the primary role of which is to facilitate learning through feedback since “feed-forward” bodily emotional activity operates on too slow a time scale to be of cognitive/informational value to online behavior. Emotional feelings have also been seen, consistent with Baumeister et al., as a means for anticipating other emotions – Mellers et al. (1999), Anderson (2003), Krueger et al. (2005), as cited in Gross (2007) – where actions are chosen that are expected to promote the onset of positive emotions and reduce the likelihood of negative emotions. This agrees with views of emotions researchers that hold that “emotions are motivating” (Rolls, 1999), and that “[e]motions are closely and intimately related to action by way of their nature as motivational states” (Frijda, 2004, p. 159). As a general mechanism, anticipation may also go some way to offsetting the above-mentioned latency of the emotional (action tendency) response (cf. Gross, 2007). Leventhal (1980) has viewed emotion in terms of the generation of prediction–feedback mismatch “errors.” He posits the existence of emotion schemata that are “integrations of separate perceptual codes of the visual, auditory, somesthetic, expressive, and autonomic reactions that are reliably associated with emotional experiences” (p. 171) and that “man and other primates clearly respond emotionally to the disconfirmation of schematic expectations. The violation of schemata is a critical source of affective experiences and reactions” (p. 187).
If such perceptual schemata exist that permit predictive–feedback processing, however, their computational dynamics and underlying neural representation have yet to be identified. Notwithstanding, dynamic control processes have been viewed as fundamental to emotion regulation functionally realized through predictive/primary-responses and feedback/secondary-responses (e.g., Koole, 2009). In the case of the former response, emotion elicitation sensitivity may be malleable to experience while in the latter response, emotion states are regulated. In this sense, emotion regulation comprises an initial estimate of emotion relevance and also down- or up-regulated emotional activity contingent on the monitored feedback that precipitates the secondary response.
The present state of the art of emotion science, summarized briefly above, therefore, is such that, contra James, emotional feeling is viewed as both preceding and following action or action tendency as it affords online behavior and learning. In the remainder of this article, we discuss the relationship between emotional feeling and action tendency. We investigate the extent to which they can be viewed in terms of a composite whole according to a dynamic bidirectional relationship with the neural-dynamic substrate of the former state affording a prediction of the latter state in the context of a particular emotional event. The functional and causal relationship between feeling and action tendency is discussed, above all, in relation to homeostatic- and event-based contingencies that impact on goal-directed (or more generally reinforcement–contingent) behavior (cf. Simon, 1967; Frijda, 1986, 1995, 2004, 2007, 2010; Rolls, 1986, 1999, 2005; Oatley and Johnson-Laird, 1987; Dickinson and Balleine, 1994; Cañamero, 2003; Koole, 2009; Boureau and Dayan, 2010). Complementary to James, such contingencies or junctures provide scope for addressing the question of the nature and form of non-cognitive triggers in emotional episodes.
The rest of the article breaks down as follows: in Section “The Relationship Between Emotional Feeling and Action Tendency: A review,” we review classical and contemporary perspectives on action tendencies, feelings, and the feeling–action tendency relation according to existing evidence gleaned from research in neurophysiology and psychology. In Section “Emotional Feelings as Predictions of Action Tendency: A Position,” we discuss the feeling–action tendency relation through the lens of our postulation that emotional feelings function as predictions of action tendency. We expound our notion of action tendency prediction–feedback loops (ATPFL) that regulate emotion episodes according to a neural-dynamic stable representational feeling substrate. In Section “ATPFL in Goal-Directed Behavior: An Application,” we examine the role of ATPFL according to ongoing and prospective goal-directed behavior with respect to triggers rooted in reinforcement contingencies (or goal junctures, GJs) and in the context of homeostatic regulation of an existing goal/need set. In this section we make specific reference to work undertaken in AI and robotics that has utilized emotion-like mechanisms in the service of adaptive goal-regulated behavior. Finally, in Section “Conclusion,” we offer some final remarks.
The Relationship between Emotional Feeling and Action Tendency: A Review
Broadly, theories of emotion can be categorized according to their emphasis on one or more of triggers, action and action tendencies, and perceptual states that regard the body and may or may not integrate information from the outside world – we may broadly label such states as feelings. Emotion theories may focus more or less on a given constituent.
Certain theorists focus on the primacy of pre-conscious triggers in the emotion episode, often in the context of appraised dimensions (cf. Arnold, 1960; Zajonc, 1980, 1984; Lazarus, 1984, 1991; Scherer, 1984, 2009; Frijda, 1986, 2007) or alternatively in terms of reinforcement or/and goal-directed behaviors (Simon, 1967; Frijda, 1987, 2010, Oatley and Johnson-Laird, 1987; LeDoux, 1996; Rolls, 1999, 2005; Kreibig et al., 2010), that may relate to a “primary reinforcing stimulus” i.e., that has some pain or pleasure value, or a secondarily reinforcing stimulus, i.e., that is predictive of the primary reinforcer. Other theorists place strong emphasis on action and action tendency where individual emotions are either considered relatively prescribed and rigid action programs (cf. Damasio, 1994, 2010; Panksepp, 1998, 2000, 2007) of ancestral survival relevance (Tooby and Cosmides, 2008) and may involve an expressive–communicative component (cf. Ekman, 1972, 2003) or alternatively considered motivating and preparatory to, though not determining, action (cf. Frijda, 1986, 2004, 2010; Rolls, 1999, 2005; Bradley and Lang, 2000; Lang and Bradley, 2010). Finally, some theorists emphasize the role of feelings in emotions at subconscious and conscious perceptual levels and that may or may not concern bodily feedback (James, 1884; Damasio, 1994, 1999, 2003, 2010; Prinz, 2004, 2005; Laird, 2007; Friedman, 2010).
In the remainder of this section we will attempt, according to a broad review of (embodied; cf. Ziemke, 2008; Ziemke and Lowe, 2009) emotions, to clarify better the feeling and action tendency components of emotion as well as their temporal and functional relationship in the context of a given emotion episode that is triggered by a nebulus emotionally competent stimulus (ECS; Damasio, 1994)3. We reserve discussion of triggers, in the context of goal-directed and reinforcement–contingent behaviors, to Section “ATPFL in Goal-Directed Behavior: An Application.”
What is an (Emotional) Action Tendency and What is Its Relation to (Overt) Action?
Discrete action programs versus motivation-grounded action tendencies
The link between emotion and action or the “impulses” to action has been apprehended since the time of Aristotle (cf. Aristotle, 1984, also see Oatley et al., 2006, pp. 11–13, for discussion). A key area of debate among emotion theorists is whether bodily activity that precipitates overt emotional behavior can be characterized according to one of two main perspectives. The first of these holds that discrete action- (or affect-) programs exist with relatively detailed and stereotyped autonomic nervous system (ANS) profiles. For simplicity, we will label this the discrete action program perspective. The second perspective declares that bodily activity is expressed in relatively unspecified ANS states whose influence on behavior is structurally and functionally grounded upon, and constrained by, motivational and environmental contextual factors. We label this latter view the motivation-grounded perspective.
As a stereotypical description, the discrete action program perspective, endorsed by protagonists such as Ekman (1972), Levenson et al. (1992), Levenson (2003, 2011), Panksepp (1998, 2000, 2007), Friedman (2010), Stephens et al. (2010), holds that emotions: are “hard-wired,” “natural kinds,” have discrete ANS-specified profiles, have evolved to enable organisms to cope with specific survival challenges, directly map/output to behavioral and expressive states, are identifiable as subjectively reported feelings (see Levenson, 2011, for summary of discrete basic emotion properties). Levenson (2011) has indicated the existence of, and requisite need for (for basic emotion theory to hold), a central organizing mechanism that “(searches) continuously for meaningful patterns in incoming sensory information, recognizing survival-critical situations, and activating the appropriate emotion, which recruits and orchestrates the optimal behavioral and physiological responses”. This “executive system” is conceived as an affect program (cf. Tomkins, 1962). Criticism of the discrete action program perspective has focused on the relatively weak evidence concerning reliable somatic–visceral profiles of activity demarcating even the basic emotions and that a (low) dimension perspective, e.g., concerning valence and arousal, offers a more reliable indicator of ANS–emotion relations (see Cacioppo et al., 2000; Feldman-Barrett, 2006; Larsen et al., 2008; Koole, 2009; Mauss and Robinson, 2009 for critical reviews). Further criticism, important for the discussion in the rest of this section as it concerns the action–action tendency relation, is that affective/emotional activity can only be understood in terms of the dynamic interchange of behavioral, physiological, and experiential components (cf. Mauss and Robinson, 2009; Lang and Bradley, 2010) rather than with recourse to a centralized system.
In contrast to the discrete action program position, the motivation-grounded position posits that emotional systems structurally (in terms of neural circuitry) and functionally build upon networks for “appetitive” and “aversive/defensive” responding (cf. Frijda, 2010). These two systems function as (tree-like) cascade networks (e.g., see Frijda, 2010; Lang and Bradley, 2010) that afford approach- and withdrawal-action possibilities. The path along this cascade may be more or less habitually traversed or require deliberation (Daw et al., 2005; Frijda, 2010, Gläscher et al., 2010). The applicable motivational states may be considered, above all, “extrinsic” as opposed to “intrinsic” (cf. Schmidhuber, 1991; Oudeyer and Kaplan, 2007, Baldassarre, 2011) where the former type is evoked by homeostatic imbalances, as a form of “negative feedback control” (Frijda, 2010, p. 573), and the latter concerns learning for the sake of learning (e.g., artificial curiosity – Schmidhuber, 2010; Luciw et al., 2011) where no specific aim or goal is necessary. The “tendency” to act is often equated with the emotion itself: “emotions are often dispositions to act rather than the actions themselves: when a stimulus of threat or appetite prompts the execution of an action procedure, preparatory metabolic changes occur in muscles and glands” (Bradley and Lang, 2000, p. 244), and “[e]motion, by its very nature, is change in action readiness to maintain or change one’s relationship to an object or event” (Frijda, 2004, p. 158). The tendency, fundamentally, is rooted in a “striving” following obstructions or facilitations to goal- or need-directed behaviors (cf. Frijda, 1986, 2010; Cacioppo et al., 2000; Kreibig et al., 2010).
Attempts have been made to reconcile the discrete action program and motivation-grounded perspectives on emotional action and action tendency. Christie and Friedman (2004), for example, found that the “basic”4 emotions (anger, fear, sadness, amusement, contentment, disgust) mapped onto the continuous dimensions of valence and activation in the case of subjective reports of emotion following presentation of emotion-inducing film clips. They found that these basic emotions, however, mapped significantly better onto the dimensions of approach–withdrawal and activation with respect to ANS activity. This result indicates that low-dimensional ANS specificity or/and neural representation may exist for arousal/activation, affective valence, and action tendencies (approach–withdrawal orientation). Mauss and Robinson (2009) also point out that discrete emotions might be envisioned according to combinations of two or more dimensions, e.g., anger = negative valence, high arousal, and high approach tendency, whereas fear = negative valence, high arousal, and high withdrawal tendency. More discussion of the dimensional perspective on emotions and action tendency will be provided in Section “Emotional Feelings: The Neural-Dynamic Representation of Action Tendency.”
From the alternative angle, a given theoretical position, naturally, may not easily fit into the discrete action program or motivation-grounded emotion camp. The position of Damasio, for example, while suggesting that emotions are hierarchically grounded upon homeostatic and motivation-based neurophysiological “machinery” (Damasio, 2003), nevertheless describes emotions as action programs:
“[e]motions are complex, largely automated programs of actions concocted by evolution. The actions are complemented by a cognitive program that includes certain ideas and modes of cognition, but the world of emotions is largely one of actions carried out in our bodies, from facial expressions and postures to changes in viscera and internal milieu,”
(Damasio, 2010, p. 109).
This tightly coupled emotion–behavior relation is, however, one to which the motivation-grounded advocates object. Frijda (2010) suggests that differences in autonomic activity may not so much reflect automated emotional action patterns but constitute contingent patterns of action readiness. With reference to Kreibig et al. (2010), Frijda points out that many different behaviors can manifest subsequent to a given emotion-relevant event. A threat stimulus, for example, commensurate with a fear state, may elicit fight or flight tendencies. Furthermore, a given behavior may be elicited according to different emotional events though its expression may alternately owe to enaction of the appetitive or defensive systems. Fight (or aggressive approach), for example, may be triggered in either a defensive, or a non-threatening appetitive, context. Following Van Hoof (1972), a cascade of broad appetitive and defensive behaviors has been identified by Frijda (2010) as providing elaborated states of action tendency. Appetitive behaviors include approach, watch, open up, body contact; defensive behaviors include withdraw, go against, submit, detach the latter in turn branching to subsets of possibilities – physical, verbal, or turn back.
The above-mentioned perspective on the role of ANS activation in the constitution of the emotion state can be understood with reference to Cacioppo et al.’s (1992, 2000) terms of tactical versus strategic emotions, made with reference to Bradley (2000). A Strategy pertains to “underlying (ANS) organizations that direct actions in the pursuit of broad end goals,” p. 183. They are centered around appetitive and aversive systems and the chief responsibility of the ANS is to “mobilize metabolic resources in response to hostile and hospitable stimuli […] crucial to survival,” p. 183. Tactics, on the other hand, refer to “specific context-bound patterns of actions.” Broadly, tactical emotions are those advocated by the discrete action program position whereas strategic emotions are those purported by the motivation-grounded position. For the former position, however, and similar to Frijda, Cacioppo et al. suggest that ANS specificity may be compromised as the same emotion may relate to many behaviors. From the motivation-grounded perspective, the discrete action program position confuses tactics-based patterns of action with the broad, strategic emotional biasing of branches of cascaded behavioral possibilities. On this basis, ANS activity relevant to particular branched subsets of behaviors is not synonymous with the underlying emotion.
The exact nature of appetitive–defensive motivation systems is complex but appears to be rooted in diffusive neuromodulatory projections involving, above all, dopamine and serotonin. Gray (1982; see also Gray and McNaughton, 2000; McNaughton and Gray, 2000) proposed two types of neural system for dealing with appetitive and defensive survival challenges, the behavioral activation system (BAS) and the behavioral inhibition system (BIS), respectively. The BAS is largely composed of mesolimbic dopaminergic projections from the ventral tegmentum area to the ventral striatum, the BIS is composed of serotonergic projections in amygdala and septohippocampal regions (also see Boureau and Dayan, 2010 for perspective). The systems are seen to work largely independently and in opposition to each other where the BIS is implicated more in withdrawal tendencies and the BAS in approach tendencies. However, the extent of the existence of opponency in such systems is contended. The separate appetitive and aversive quantifying systems may work in opposition (Daw et al., 2002; Cools et al., 2010) but also in co-activation (cf. Norris et al., 2010) augmenting behavioral arousal.
Attempts to map motivational valence onto action tendencies has led to criticism of the validity of the motivation-grounded perspective on emotion. Stemmler et al. (2007) found somatovisceral activation (“structural”) independence concerning anger–fear states and approach–withdrawal tendencies and concluded functional independence between motivation and emotion-based systems. There are several comments that can be made regarding Stemmler et al.’s conclusions: (1) as mentioned, appetitive–defensive systems do not map directly (linearly or monotonically) to approach–withdrawal systems. Defensive systems may incline withdrawal responses but can nevertheless activate fight-like approach defensive responses (cf. Boureau and Dayan, 2010; Frijda, 2010). On this basis, it may be challenging to establish an exact relationship between anger–fear and approach–withdrawal based on ANS profiles of activity; (2) the finding of only weak somatovisceral activation somewhat undermines the relevance of the results. Furthermore, even if structural independence is the case, it does not preclude functional interdependence. Emotions may have evolved for several functions (see Koole, 2009 for review of core emotion functions) and therefore some structural independence in the multi-serving emotion systems may be expected.
The dynamics of action and action tendency
The dynamic mapping of action tendency to overt action/behavior highlights difficulties in sustaining a strong discrete action program position. Actions, and tendencies to act, invariably involve sequences of responding which may be qualitatively different regarding profiles of physiological activation and/or approach–withdrawal tendency. Fanselow (1994) identified three classes of behavior, structured according to a cascade of possibilities, appropriate to a defensive behavior system – pre-encounter, post-encounter, circa-strike. The pre-encounter class pertains to changes in behavioral organization affecting homeostasis, e.g., feeding habits, and behaviors that may pre-empt hostile encounters, e.g., protective nest maintenance. The post-encounter class amounts to immediate threat management where BIS-like activity is enacted (e.g., freezing, potentiated startle). In the circa-strike phase animals choose from a behavioral repertoire pertinent to active coping (similar to BAS employment). Fight, flight, and display behaviors can be utilized at this threat engagement stage.
The dynamic flow concerning action tendencies, as physiological (ANS-governed) states, and overt actions has been investigated in depth by Bradley, Lang, and co-workers (cf. Bradley and Lang, 1994; Lang, 1995; Bradley and Lang, 2000, 2007; Löw et al., 2008; Lang and Bradley, 2010). Lang and Bradley (2010) illuminated that the dynamic flow of emotional action tendency and behavior is so complex as to render “impossible” the ANS specificity of emotions and action programs as retroactively attributed, as a position, to James. The defense cascade model (Lang et al., 1997) demonstrates the importance of viewing ANS activity preparatory to action as a dynamic process. The model (Figure 1) consists of three distinct phases, similar to those identified by Fanselow (1994), that map the relation between the organism and the ECS according to action – pre-encounter, post-encounter, overt behavior. However, the mapping of the physiological variables that capture preparatory aspects to a behavioral response (e.g., sweat gland activity, heart rate, startle reflex) have a non-monotonic relation to each other and to overt behavior: heart rate, for example, initially slows as the organism attends to the encountered stimulus (presumably to evaluate threat relevance) prior to an acceleration preparatory to a fight–flight response.
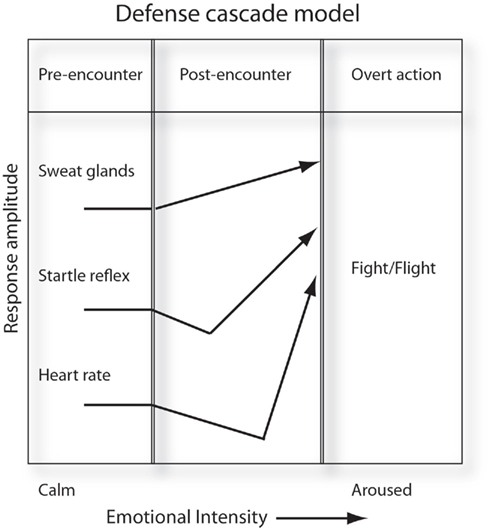
Figure 1. Action as a function of action tendency variables – the defense cascade model. The relationship between bodily variable activity (arousal-relevant) antecedent to action is non-monotonic. The action tendency therefore is dynamic and changes as a function of time and/or perception of the emotion eliciting stimulus. Adapted from Bradley and Lang (2000).
More specifically, the adapted diagram of the Lang et al. (1997) model (Figure 1) highlights the non-monotonicity of the arousal constituents of the motive state (emotional response). The manner in which such tendency components: constitute emotions in themselves or/and instigate overt behaviors, however, comprise issues subject to ongoing debate. Mauss and Robinson (2009), in their extensive meta-analysis of ANS specificity of emotion, concluded that of the many physiological components proffered to distinguish among basic emotions cross-study correlations between emotion experience and individual components are weak. Instead, a clearer relationship is apparent between sets of physiological variables comprising a particular affect dimension, e.g., arousal, and a given emotion.
Similar to the action tendency dynamic flow, the nature of the emotional action tendencies and how they map to overt behavioral response is not merely a “feed-forward” relation but is instead of a complex dynamic nature. Schauer and Elbert (2010), for example, have produced a six stage action–action tendency model according to fear activity that evolves into a traumatic stress response. The model is conceived according to a cascade of “Freeze–Flight–Fight–Fright–Flag–Faint” behaviors (Figure 2) that functions to provide the best chance for an organism to overcome a severe survival threat.
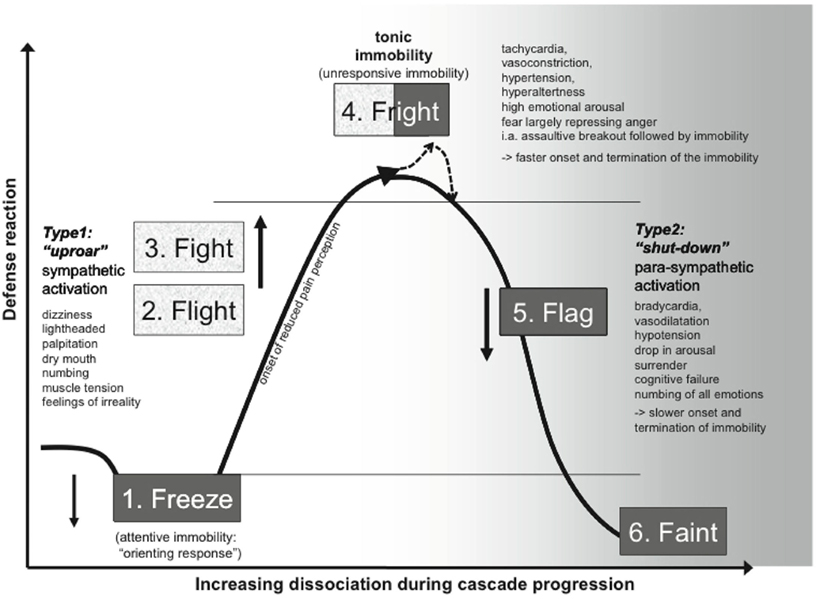
Figure 2. Action and action tendency mapping in fear–trauma responding. Here, is provided an example of the complex non-linear relationship between bodily variables constitutive of the evolving action tendencies and the concomitant overt behaviors. Taken from Schauer and Elbert (2010). Reprinted with permission.
Both Figures 1 and 2 demonstrate stages of inhibition (BIS activation) and active coping (cf. Waldstein et al., 1997; BAS activation). It is clear however, that even within this action–action tendency dynamic the BIS and BAS systems may be alternately activated during the threat episode that requires the emotional response. We may schematize the relation between action tendency and overt behavior in Figure 3 where the gray arrows overlaying a feed-forward model serve to implement the more dynamic perspective that best fits the action–action tendency relation according to the above-discussed research.
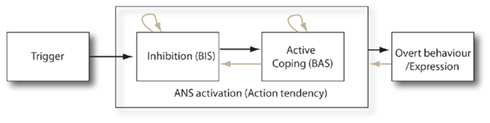
Figure 3. The relation between action tendency and action. The gray arrows superimpose a dynamic perspective onto an otherwise conventional feed-forward model of emotional behavior. Only the “dynamic” model is in line with the motivation-grounded perspective.
While correlations in neurophysiological states and particular subjective ratings of emotions may exist, it is not obvious how the individual action and action tendency components cohere into a global categorizable state that is greater than the sum of its parts. Such a gestalt would help rebuff the accusation of emotions as being epiphenomenal. This lack of componential coherence has been claimed to be a problem for the discrete action program position (Mauss and Robinson, 2009). However, it is also of concern for the motivation-grounded position. Bradley and Lang (2000) acknowledge that “no system can be defined by a single subsystem measure” (p. 245) where subsystem refers to a given single dimension of behavior–physiology-language emotional action and action tendency space but where “the three response systems have no obvious common metric.” For Prinz (2004), this represents an example of the “problem of plenty” in emotion theory: “if all parts [emotion constituents] are essential, how do they hang together into a coherent whole? … the Problem of Plenty asks for an essential function of emotions in virtue of which they may have several essential components” (p. 18).
Naturally, a relative lack of tractability does not preclude the existence of coherence which may necessarily only be conceived in complex dynamic terms, e.g., pertinent to stabilizations and transitions between sequenced sensorimotor components. Lewis (2005) has posited a (psychoneurophysiological) dynamic systems (DS) account of emotional states, incorporating action tendency components that stabilize through their interaction with appraisal constituents culminating in global attention orienting states (“emotion–appraisal amalgams”). On this basis, appraisal is considered the end point of the emotional episode that, through stabilized neural activity, enables learning of enduring action (e.g., goal-directed) plans (cf. Lewis, 2005, p. 177). Carver and Scheier (1998) – see also Carver (2006) and Carver and Harmon-Jones (2009) – have proposed a motivation-grounded DS perspective on emotional activation. Carver (2005) criticized Lewis’ assertion of the existence of a psychoneurophysiological stabilization mechanism for emotion. In the Carver and Scheier perspective, emotions and motivations serve as control (feedback) systems whose activations stabilize but in accordance with behavioral as well as (psycho-) neurophysiological activity.
Coherence among action tendency components may also occur through a process of development and learning which may constrain self-organized activation patterns. Camras (2011), for example, claims that peripheral motor system components may become associated following learning (also see Berkowitz, 2000). For Camras affective (facial) expressions are considered motor coordinated structures – the coincident activity of groups of muscles – that via hebb-like processes, come to recruit/entrain activity in other muscles or muscle groups such that a coordinated expression develops. Furthermore, according to Laird, who also refers to the same three action and action tendency systems as Bradley and Lang(2000; i.e., behavior, physiology, and language), the deployment of these systems may have been coordinated as a consequence of both learning and Darwinian evolutionary pressures.
Dynamic neural activity may, therefore, enable coherence in the physiological domain as it concerns action tendency components across a sequence of behavioral phases constitutive of an emotion episode. The specific dynamic processes that enable this coherence offer a means of providing a firm mechanistic and functional foundation to a motivation-grounded perspective of emotion. Another fundamental means of component synchronization may be the regulation of emotion. This is the major topic of Sections “Emotional Feelings as Predictions of Action Tendency: A Position” and “ATPFL in Goal-Directed Behavior: An Application.”
What is an (emotional) feeling and what is its relation to action tendency?
Emotions and feelings are popularly conflated terms in everyday parlance. James put forward the counter-intuitive perspective of feelings as following rather than preceding actions. Nevertheless, feelings, for James, are perceptions of body states that are synonymous with the emotion states. One of the most oft-cited perspectives on feelings is offered by James:
“[o]ur natural way of thinking about these standard emotions is that the mental perception of some fact excites the mental affection called the emotion, and that this latter state of mind gives rise to the bodily expression. My thesis on the contrary is that the bodily changes follow directly the PERCEPTION of the exciting fact, and that our feeling of the … same changes as they occur IS the emotion”
(James, 1884, p. 191).
The perception of bodily changes preparatory to action is at the core of somatic–visceral theories of emotion and emotional feeling as pioneered by James. A century on from James, a number of somatic–visceral theorists that broadly fall into the discrete action program and motivation-grounded camps have put forward different accounts of the relation between feeling and: (a) conscious or unconscious perception, (b) emotions, and (c) action tendency. The precise causal and functional nature of the relation between (conscious or unconscious) emotional feelings and actions and action tendencies, however, requires recourse to the underlying neural dynamics that may permit a representational substrate on which such feelings can manifest.
Emotional feelings: The perception of action tendency
Extending the James’ (1884)5 position, Damasio (1994, 2010), Prinz (2004, 2005), and Laird (2007; see also Ekman, 1972, 2003 and Friedman, 2010) are among the key discrete action program theorists that posit that emotional feelings are (neurally) registered states capturing somatovisceral changes comprising action states/patterns. However, depending on the theorist in question, feelings may be thought of as conscious or unconscious, and may be synonymous with, or separable from, emotions. The Jamesian view on feelings is that they are synonyms for emotions and thus not separable (see Prinz, 2004 for discussion); they are also consciously experienced. Damasio’s perspective on feelings and emotions, however, differs in both respects. Firstly, Damasio views feelings and emotions as separable, distinct phenomena. Simply, emotions are about action programs (see previous sub-section). Emotional feelings, on the other hand, are primarily perceptions of action commands or programs:
“[f]eelings of emotion […] are composite perceptions of what happens in our body and mind when we are emoting. As far as the body is concerned, feelings are images of actions rather than actions themselves; the world of feelings is one of perceptions executed in brain maps”
(Damasio, 2010, p. 110).
Both Damasio and Prinz, as somatic/behavior emotion theorists, do not view emotional perceptions as being necessarily consciously experienced. James, contrarily, uses labels for internal bodily “perceptions,” “feelings,” and (self-) “consciousness” interchangeably. However, whilst Damasio does not contend that feelings need be conscious, Prinz views them as experiential. Finally, for Prinz, similar to James, perceptions are emotions which, as stated above, differs from Damasio:
“[w]hen emotions are felt, the feeling is the emotion: the emotion is a conscious perception of a patterned change in the body. But emotions can go unfelt: they can be unconscious perceptions of patterned changes in the body”
(Prinz, 2005, p. 17).
Laird (2007), on the other hand, distinguishes between feelings, as types of “self-perception” and consciousness in his somatic/behavioral feeling theory: “Self-perception theory assumes that feelings like these (happiness, hunger) are higher order integrations of various kinds of cues. As with depth perception, the process of detecting and integrating these cues is automatic and occurs outside consciousness,” (p. 10).
Notwithstanding these contentions, a perspective that unites discrete action program theorists is that emotional feelings can be engendered and modulated by bodily feedback. Emphases of modern theorists, however, diverge with respect to the extent to which proprioceptive and interoceptive processes are involved. According to Damasio (2010), proprioceptive processing entails “images of specific body components such as joints, striated musculature, some viscera” and interoceptive processing entails neural activation “mapping” of “the functional condition of body tissues such as the degree of contraction/distension of smooth musculature; parameters of internal milieu state,” (p. 76). Ekman has focused on the role of proprioceptive feedback6 (initially embraced by James but later subordinated to interoceptive feedback) especially in facial expression: forcing oneself to smile can literally make one happier. Laird (2007) has similarly pushed a proprioceptive feedback – feeling theory: “self-perception theory.” On this account, emotional feelings are primarily caused by bodily feedback. Prinz (2004, 2005), on the other hand, emphasizes interoception as a means of somatic feeling constitution. Similar to James (and Laird), Prinz views the bodily state changes intrinsic to the emotion as preceding the feeling state which are then registered and represented in neural states.
While Damasio (1994, 1999, 2003, 2010), also holds a “strong” Jamesian-somatic, i.e., predominantly interoceptive, view of the primacy of body in emotional feelings, in his view, feeling states need not always be subsequent to the bodily changes underlying emotional activity. According to Damasio (2010), there are three means by which feelings can be constructed: (1) by registrations of bodily changes induced by ECS in the brain-stem and cerebral cortex, (2) by an “as-if” body loop (Damasio, 1994; Bechara, 2004), (3) by misrepresentative “hallucinations” of bodily changes. In the case of (2), “after emotions have been expressed and experienced at least once” (Bechara, 2004, p. 38), those brain areas that ordinarily trigger bodily changes constitutive of the emotional feeling become able to activate parts of the brain responsible for registering bodily changes. This “simulation” of bodily change may happen (a) prior to, but in parallel with, those bodily changes, (b) in the absence of the bodily changes. In the case of (3), Damasio suggests in certain instances the brain can be duped into misrepresenting the body and ECS-induced changes therein. Examples of this phenomenon include cases in which analgesic medicine is provided to temporarily alleviate pain “fooling” the brain into a feeling percept of non-bodily irritation or where, in the service of promoting survival related behaviors, the brain-stem may “disengage” from the body regarding the representation of pain.
Many motivation-grounded emotion theorists have considered the relation among action, action tendency and feeling emotional components. Frijda suggests, contra Damasio, that tendency to act rather than acting per se is at the heart of emotional experience:
“emotional feeling is to a very large extent awareness, not of the body, but of the body striving, and not merely of the body striving, but the body striving in the world … [e]motional experience is, to a large extent, experienced action tendency or experienced state of action readiness,”
(Frijda, 2004, p. 161).
Cacioppo et al. (1992, 2000) – also see Larsen et al. (2008) – have argued that the relation between action tendency (as manifested in ANS patterns of activity) and experiential feeling states can be understood as a function of cognitive elaboration/disambiguation of ANS activation patterns. The researchers’ “Somatovisceral Afference Model of Emotion” (SAME) describes three (historically) prototypical cases: (1) “unambiguous” patterns where a hypothetical highly specified ANS pattern for a discrete emotion may be experienced without cognitive deliberation beyond pattern recognition (the pro-James perspective); (2) “general arousal” which requires a cognitive extraction of context for emotional experience to occur (essentially the proposal of Schachter and Singer, 1962); (3) “ambiguous” which requires a combination of cognitive “priming” and (ANS) pattern recognition in order for emotion experience to ensue. In the case of (3), an ANS-activated pattern of somatic and visceral change may be ambiguous, i.e., consisting of only a few physiological dimensions/variables, affective “valence” being primary. The ambiguity may be resolved by recourse to cognitive processing or biasing (“priming” relating to context) giving rise to the differentiated emotional experience. This experience is considered a “somatovisceral illusion” in that a fuller somatovisceral state that may map to a given subjectively experienced/reported discrete emotion may not initially exist and the full gamut of physiological responses associated with discrete emotional states may in fact only be galvanized by the experiential feeling following cognitive discernment. This notion is somewhat similar to Damasio’s “as-if body loop” insofar as feeling can precede full emotion-relevant somatovisceral activation.
Emotional feelings: The neural-dynamic representation of action tendency
In relation to the previous sub-section, it is incumbent on this review to illuminate how the felt action tendency corresponds to neural-dynamic activity. A key question, in line with the above-mentioned perspective of Prinz (2004), is: to what extent can neural-dynamic activity be considered representative of the underlying action tendency? According to the philosopher Dretske (1981, 1986) for a phenomenon to count as representational it must be fallible. Prinz (2004), with reference to Dretske’s philosophy, offers the example of a dog representation that might, on occasion, mistake a wolf, for example, for a dog. The fundamental point is that the “dog concept is a mental state that is reliably caused by dogs and was acquired for that purpose […] After that state is formed, it carries information about dogs, foxes, and wolves, because all these things can cause it to activate, but it only represents dogs, because it was set up as a result of dog encounters” [authors’ italics]. The point made here is that for a neural pattern to constitute a representation of action tendency that is a substrate for feelings, it should have the purpose of (e.g., have evolved for) representing action tendency. Essentially, the feeling neural pattern would comprise an effective and fallible prediction of what action tendency will occur following emotion event triggers. From the point of view of minimizing error, a parsimonious representation of action tendency is desirable where it is sufficient to distinguish functional (i.e., emotional) states from non-functional states; that is, states that are of informational benefit to the organism. Appeal to parsimony also respects the need for swift and efficient processing in the face of dynamic environmental threats or/and appetitive opportunities. Naturally, a corollary of holding this perspective is that the discrete action program position postulating relatively high dimensional ANS specificity for basic emotions may be subject to great potential for misclassification of somatovisceral patterns7.
An alternative view of emotions is that they can indeed be captured according to just a few dimensions. As mentioned in the previous section, it has been suggested that basic emotions may be mapped onto a low-dimensional space where affective valence, arousal/activation, and approach–withdrawal tendency are at the core (cf. Christie and Friedman, 2004). Russell (1980, 2003) has provided a model of “core affect” whereby affective valence and arousal account for basic emotions according to a circumplex. However, it has been noted that two dimensions may be insufficient to account for differences in particular negative emotions, e.g., fear and anger. The approach–withdrawal dimension has been championed by Davidson (1993), Watson et al. (1999), Carver (2006), Mauss and Robinson (2009), Koole (2009) such that anger–fear emotions may be at least partially discerned by ANS activity – diastolic blood pressure difference (see Sinha et al., 1992) – or by differential left–right brain hemispheric EEG activity (Mauss and Robinson, 2009). The qualitatively similar dimension of dominance has also been posited (Russell and Mehrabian, 1977; Mehrabian, 1996, 1997) – this dimension has otherwise been conceived as “potency” (Osgood et al., 1957). The three dimensions of Russell and Mehrabian (1977) – pleasantness, arousal, dominance – referred to as PAD, is a convenient acronym for referencing valence, arousal, and orientation (approach–withdrawal) dimensions. The fact that these three dimensions, that appear necessary and sufficient to discriminate among the basic emotions, have been extrapolated according to factor analyses using self-report measures is indicative of their importance in emotion and, more speculatively, in (conscious or unconscious) feeling.
As mentioned in the previous sub-section with reference to the work of Cacioppo et al., it may also be possible that emotional feeling states are grounded in low-dimensional neural-dynamic activity that stimulate more elaborated somatovisceral responses. Insofar as these neural-dynamic patterns should be considered representational, the feelings would come to represent somatovisceral states over the emotion episode. Whether such a dynamic process should be considered representational, however, is arguable given the purported brain–body transformative nature of the somatovisceral state. It may be more confidently asserted that the nascent feeling state represents valenced motive states (cf. Frijda, 2010) or core affective phenomena that is then elaborated into fuller emotional bodily and feeling states the constituent activity of which being distributed across brain and body. On the other hand, whether or not a feeling state comes to represent somatovisceral activity may depend on whether a self-organized process, such as that inherent to Cacioppo et al.’s view, culminates in stable activity, i.e., elevated (above baseline) neural activation levels persistent in the face of perturbations (e.g., noise). As previously mentioned, Lewis (2005) has posited such an emotion episode whereby following an emotion trigger, emotion–cognition components integrate in a self-amplification process culminating in a stable state. Stability may thereafter allow for persistent action orientations and availability of learning affordances.
In this view, stability is a critical pre-condition to higher cognitive and behavioral activity. It may also be a requisite feature of a full or functional emotional response. Stable activation patterns may not just be a self-organized outcome of emotion–cognition component integration but a pre-condition to further integration of those somatovisceral afferents whose temporal dynamics manifest on a slower time scale to emotion-relevant neural–cognitive processing. It is such stability that may lay the foundation for functional representations – in the sense of Dretske – of action tendency.
The DS perspective of Scherer, broadly, exemplifies the above view. Scherer (1984, 2004, 2009) suggests feelings can simultaneously monitor and trigger emotional change whilst registering integrated inputs from motor expressive, cognitive, physiological, and action tendency systems: “I propose to view the feeling component of emotion as a monitoring system that consists of a central representation of the response organization, including the underlying cognitive processes in an emotion episode … it integrates the representation of changes in the other components during the duration of an emotion episode” (Scherer, 2004, p. 137). Furthermore, for Scherer: “[t]he feeling component has a special status in the emotion process, as it integrates and regulates the component process” (Scherer, 2004, p. 138). In this sense, action tendency and feelings have a bidirectional relation in the emotion episode but feeling simultaneously monitors and participates in the full emotion episode.
Of what might the neurocomputational properties of stabilization dynamics8 be comprised? Stabilization dynamics have been mathematically and computationally formalized through the differential equations of Amari (1977) initially deployed to model the topographic spatial representations in the visual cortex according to neural fields. Dynamic field theory (DFT) has since been particularly noteworthy in capturing infant cognitive–behavioral phenomena (cf. Thelen et al., 2001). However, it has also been posited to be of relevance to modeling emotional phenomena in the context of Bechara et al.’s (1994) Iowa gambling task (Lowe and Ziemke, 2010; Lowe et al., 2010b). The DFT approach has spawned a perspective on representations in the brain that map cognitive phenomena to continuous dimensions. Stabilization occurs where activation on a given site on the field exceeds a given threshold consequent to local excitation the degree of which being determined by an interaction kernel. Schöner (2008) has listed the different types of stable attractor dynamics that neural field theory permits. Fundamentally, self-stable states occur contingent on the presence of input. In this sense, there is a bistable attractor dynamic since activation will gravitate toward the stable suprathreshold level or to a baseline of activation as a function of input. On the other hand, self-sustainable states require initial input to achieve suprathreshold activity but stability is thereafter resistant to the withdrawal of the stimulus. It is only the sites on a field that have suprathreshold activation that are considered units of representation (cf. Schöner, 2008; Sandamirskaya et al., 2011) which may also be considered units of cognition to the extent that they are then capable of impacting on the activity of sites in different fields. Field activation is non-representational and non-cognitive when subthreshold since it does not influence other field site activation (it is not “for” anything at such a stage). Nevertheless, activated sites on a field may be more or less subthreshold depending on memory inputs that effectively prime or predispose activation at particular sites.
Since emotional states are generally postulated to require an ECS (or event) trigger whose offset (withdrawal) is antecedent to emotional dampening the more reasonable stabilization dynamic for emotional feelings is the self-stable state. The onset and offset dynamics (gain/slope) of the feeling state, however, could be modulated consequent to experience, personality, and the degree of above-threshold activation following ECS input. Experience of a self-sustainable state might pertain to one of a number of pathological conditions where following ECS input the agent fails to destabilize emotional feeling (e.g., in the case of “sham rage”). One could imagine an ontogenetically emergent attractor landscape that is comprised of multi- (low-) dimensional fields (e.g., representing core affect or perhaps PAD space). The landscape might predispose particular sites on the field to be active (to produce self-stable states) according to personality-dependent past experience, while low-level somatovisceral inputs would predispose (suprathreshold) emotional feeling states following ECS trigger onset constituting something like “moods.” Through the provision of a non-smooth/non-linear continuous space an observable mapping from dimensional to discrete emotion space is thereby afforded. This attractor landscape could thereby suggest a mechanism to explain the Christie and Friedman (2004) result of continuous dimension–discrete emotion mapping. The computational investigation of stability dynamics in the context of an emotion episode may unveil an important bridge between dimension theory and discrete emotion theory.
Finally, at a neural–anatomic level of description, Lewis has cited the existence of interacting object evaluation, monitoring, and action loops centering on key hub neural structures, e.g., amygdala, prefrontal cortex, and brain-stem, that permit stability dynamics. These areas are also critical to Damasio’s account of emotion feeling generation and are oft-cited in theories of emotion (cf. LeDoux, 1996; Rolls, 1999, 2005; Davidson et al., 2007; Quirk, 2007; Pessoa, 2008, 2010). Damasio has identified convergence–divergence zones (CDZs) in the brain for neural exteroceptive, interoceptive, and proprioceptive maps (the feeling substrate within the brain). At the top levels of the hierarchy, CDZs are said to represent “dispositions” of how to construct stable representations. Through a mechanism of “time-locked retroactivation” (Damasio, 2010) coincidence of activity in brain sites activating during the mapping of particular features enables learning and recalling of complex representational contexts. Another key feature for promoting stabilization processes is phase synchronization9. Damasio has suggested that this can be observed in sub-cortical regions that might be responsible for “primordial” feelings which consist of coarse mappings of body changes: “the superior colliculus (a sub-cortical convergence zone station for visual, auditory, and body states inputs) is the only brain region outside the cerebral cortex known to exhibit gamma-range oscillations,” (Damasio, 2010, p. 86).
Emotional Feelings as Predictions of Action Tendency: A Position
Based on the brief review in Section “The Relationship Between Emotional Feeling and Action Tendency: A review” of emotional: (1) actions and action tendencies, (2) feelings, and (3) feelings–action (tendencies) causal relations, in this section we discuss a possible role for emotional feelings of action tendencies as predictive states that serve homeostatic emotion regulation. We start the section by providing a context of prediction as core to function of whole brain activity which thereby may generalize to emotional activity as captured by the action tendency – feeling relation.
Prediction in the Brain
Some recent theories of core brain (above all neocortical) functioning have highlighted prediction as underlying neural and psychological processing. Hawkins (2004), for example, when addressing his theory of human intelligence being rooted in a (neo-) cortical hierarchy, suggests “(prediction) is the primary function of the neocortex and the foundation of intelligence” (p. 89). Hawkins suggests that (“invariant representational”) memory provides a critical substrate for thinking through the mechanism of prediction: “What we perceive is a combination of what we sense and of our brains’ memory derived predictions” (p. 87). One reason why prediction based on memory is crucial is simply processing time and thus “educated guesses” are a requisite feature of neural processing. Classical Artificial Intelligence methods have invoked listing all attributes of scenes in lookup tables which affords a processing technique – searching through the table of properties to find a match or optimal solution – which is not amenable to the processing of neurons, it would take far too long. Another reason for the necessity of prediction is functional. Tracking the trajectory of a ball, either purely visually or with respect to attempting to position oneself for catching, based solely on (sensory) feedback ensures failure at the task. This is essentially an exteroceptive problem of the delay inherent in neural processing time with respect to the real-time dynamics of world events10. Downing (2009) also points out that basic locomotion requires prediction and that reliance on control theoretic techniques in engineering based only on sensory feedback ensures a mismatch between motor capabilities and processing speed.
The focus of Hawkins, following Mountcastle (1978, 1998), has been on sensory and motor (primarily exteroceptive and proprioceptive) processing. However, Hawkins argues that all neocortical brain function deals in the currency of prediction:
“[a]ll regions of your neocortex are simultaneously trying to predict what their next experience will be. Visual areas make predictions about edges, shapes, objects, locations, and motions. Auditory areas make predictions about tones, direction to source, and patterns of sound. Somatosensory areas make predictions about touch, texture, contour, and temperature,”
(Hawkins, 2004, p. 88/89).
Hawkins has proposed that hierarchically structured layers of cortical columns receive a combination of bottom-up, top-down, and lateral inputs. The latter inputs are critical to producing a type of attentional winner-take-all effect whilst the top-down versus bottom-up processing establishes a comparison between a high-level (invariant) prediction of the contextual nature of the stimulus processing at a particular level of abstraction (relative to the hierarchy) which is compared against bottom-up inputs from a number of cortical column inputs that feed back specific details relevant to the stimulus processing. Predictive error signals can occur where the processed details do not meet with the high-level contextual expectations and such signals are then sent up the hierarchy (with the hippocampus sitting atop of the cortical hierarchy as the predictive beacon) in order to establish whether the input detail can fit within a broader (higher level) context.
Downing (2009) has identified a number of structures that partake in predictive processing: cerebellum, basal ganglia, and thalamocortical loops, the latter of which he suggests is a mechanism by which Hawkins’ theory of error signals relaying up the cortical hierarchy may be achieved. Thalamocortical loops are understood to be a key means by which such predictions can occur and be updated through ongoing sensorial feedback and allow for predictions of sequences of states (cf. Rodriguez et al., 2004; Granger, 2006, Sherman and Guillery, 2006; Downing, 2009). Hesslow (2002), has argued, that sequences of sensory perceptual states and also motoric activations involve internal simulation of overt behavior that constitute ever more distal forms of prediction of consequences of sensory and motoric activity as an organism relates to its environment (see also Jeannerod, 1994). In a similar vein to Damasio (1994), regarding simulation of the interoceptively processed body, motor structures may be activated in the absence of overt expression as may sensory cortex in the absence of external sensory stimulation.
Simulation has also been invoked as a concept to explain predicting sequences of sensory states according to a dynamic systems (DS) perspective (Friston and Kiebel, 2009) and in the context of predicting social situations (inferring intentionality of others from behaviors). The predictive coding hypothesis (Friston, 2002, 2003; see also Kilner et al., 2007) proposes the existence of hierarchies of DS in the brain (cortical hierarchy) that implement an empirical Bayesian inferencing network. Within this framework it is proposed that states (e.g., goal states of others) can be inferred through a process of prediction error (PE) minimization at all levels of the cortical hierarchy. The focus on PE minimization, through reciprocal or/and recurrent interactions between levels of the hierarchy, distinguishes the predictive coding perspective from that of Hawkins (2004). Another difference is that predictive coding does not signify forecasting (i.e., of future events) which is the property of prospective coding (cf. Prinz, 2007) but rather refers to predictions of current states based on present information. Similar to Hawkins, on the other hand, is the notion that higher levels of the cortical hierarchy represent, or come to represent, predictions in lower levels of the hierarchy. In the predictive coding framework, predictions from lower levels of the hierarchy can feed back to higher levels and update existing predictions. This self-organization prediction–feedback process only stabilizes at the point at which PE minimization is achieved and probable (goal) state can be inferred from (action) input.
Prediction error minimization appears to be a key mechanism for all forms of learning. Theoretically well grounded learning rules have been established providing normative models (i.e., that guide functional understanding) in the context of motivational systems such as the Rescorla and Wagner (1972) model and a mathematically similar model that accounts for temporal dynamics – the temporal difference (TD) learning algorithm (Sutton and Barto, 1990, 1998). TD learning can explain much animal neurobehavioral data (e.g., Suri and Schultz, 1998; Suri, 2002; Roesch et al., 2007) but also higher order learning in humans (cf. Seymour et al., 2004). The predictive dynamics of appetitive and aversive/defensive networks in the brain are thought to be organized around key neuromodulators emanating from brain-stem regions that act in accordance with TD learning (cf. Wörgötter and Porr, 2005; Niv, 2009; Samson et al., 2010 for reviews). Above all, dopamine (DA) is implicated in reward and action signaling (cf. Schultz, 1998, 2007) and serotonin (5HT) in punisher and inhibition/withdrawal signaling. It has been suggested that the two types of signal may provide the key outputs of an opponent process system (e.g., Daw et al., 2002; Dayan and Huys, 2008; Cools et al., 2010; Norris et al., 2010) critical to arbitrating appetitive and defensive behaviors. It might be speculated that these signals parsimoniously provide compressed information to feeling representational networks concerning affective state as rooted in reinforcement contingencies. For example: a pleasantness dimension (“P”) may simply imply a negation of dopamine and serotonin signals where DA negative PEs and high 5HT will tend toward an overall representation of negative P; an arousal dimension (“A”) may imply a conjunction of dopamine and serotonin magnitude signals reflecting overall strength of activity of the appetitive and defensive networks; an approach–withdrawal or dominance (“D”) dimension may imply a negation of signal magnitudes since DA positive and negative prediction errors statistically concern appetitive contexts relevant to approach behaviors even though the relation between such action orientation tendencies and appetitive–defensive systems is not absolute (as mentioned with respect to Frijda, 2010).
Other recent theory in neuroscience and physiology indicates that interoceptive (somatovisceral/motivation-based) processing may also be of a predictive nature insofar as it concerns homeostatic activity. The notion of allostasis (Eyer and Sterling, 1977; Schulkin, 2003; Sterling, 2004; Woods and Ramsay, 2007) concerns a rethink of the classical control theoretic perspective on homeostasis revolving around feedback loops respecting set points that demarcate “ideal” states. According to Sterling (2004), allostasis can be conceived in terms of prediction where brain areas implicated in planning and decision making (for Sterling, above all prefrontal cortex and amygdala) are viewed as supplying inputs that may override other inputs that signal errors from ideal homeostatic balance. Such overriding of “basal” homeostasis operates in the service of supplying the organism with the resources previously learned to be necessary to meet predicted environmental pressures. Sterling considers allostasis as a means of permitting adaptive bodily regulation according to “stability through change” which accounts for both internal needs and external pressures (or opportunities) and compares to the Bernard notion of “stability through constancy.”
Emotional feelings: Action tendency prediction–Feedback Loops
In this section, we make explicit a potential role for prediction in the emotional feeling of action tendency. The position we put forward is extrapolated according to the review provided in the previous sections. We postulate that somatovisceral prediction–feedback loops are essential for ongoing learning and online behavior across an emotion episode. Stable (suprathreshold) activation in a neural-dynamic substrate is suggested to provide a critical foundation on which prediction–feedback loops can operate. Subthreshold activation may come to represent (through self-stabilization) action tendency following a) strong predictions of action tendency, or b) in the absence of relative certainty, according to bodily feedback given appropriate priming at neural activity registration sites. This process can enable efficient emotion regulation where mismatch between neural representations and actual action tendencies can be down-regulated by bodily feedback and relatively strong action tendencies may up-regulate or instigate suprathreshold activation in the “feeling” neural-dynamic substrate. This is the raw essence of the ATPFL perspective. We will discuss it in relation to emotion regulation in the remainder of this section. Section “ATPFL in Goal-Directed Behavior: An Application” focuses specifically on how the theoretical position we put forward can explain regulation of goal (or need) directed behavior. From this point, we will use the acronym ATPFL in place of “action tendency prediction–feedback loop(s).”
The “As-if body loop” as an ATPFL
To what extent can emotional feelings be viewed as being based on predictions of action and action tendency? The idea of emotional feelings predicting behaviors is implicit in many perspectives. Frijda (1986, 2004, 2007), for example, advocates that emotional feelings are concerned, above all, with action tendencies that anticipate actions though he also notes that the relationship between action tendency and action is complex: “The link between emotion and action is intimate; yet it is weak. Anger has intimate links to aggression, but few angers actually go that far” (Frijda, 2004, p. 163).
The Damasio (1994) and Bechara (2004) perspective on emotions and emotional feelings also may be interpreted in terms of prediction. In regard to Section “Emotional Feelings: The Perception of Action Tendency,” we might extract from this view the following functions that underlie emotional feelings: (1) the as-if body loop predicts how the body will be affected by a given ECS; (2) the body loop provides feedback that may confirm or disconfirm the effective as-if body loop prediction; (3) the hallucinatory aspect of feeling is, partially, a side effect of attention filtering whereby those body changes predicted to be important to survival will be attended to, e.g., pain during flight will tend not to be felt as it affords no benefit to the escape act.
The above, arguably, combines, and extends perspectives put forward by Laird (2007) and Prinz (2004) concerning the functions of emotional feelings. For Laird, neural-dynamic represented bodily states provide a means for comparing predicted and actual (feedback) states as a type of cybernetics control process. For Prinz, stable representations (or stabilized activation states that come to represent the body) allow for tracking of core relational themes via filtering noisy sensory input. According to this extrapolation, the sequence of events in an emotion episode can be described as follows (visualized in Figure 4). The ECS is perceived (e.g., the snake). Brain areas implicated in evaluating the significance of the stimulus (e.g., amygdala, ventral-medial prefrontal cortex) activate elicitor sites in the brain-stem and thereby simultaneously instigate emotional bodily changes and activate regions of the brain responsible for registering bodily feeling states. The first sites to be activated in the brain-stem register coarse feeling activation patterns and those in turn activate parts of the brain (e.g., somatosensory cortices, anterior cingulate cortex) that register more context-elaborated feeling activation patterns (according to convergent exteroceptive, interoceptive, and proprioceptive inputs). However, by this stage, in accordance with the as-if body loop hypothesis, the more context-based feeling states have already been triggered by the ECS evaluation sites. This means an early, contextual prediction of body state can be constructed while input from coarse patterns in the brain-stem constitutive of early stages of body change registrations provide (1), a coarse prediction–feedback loop, and (2), the initial phases of feedback control to the contextual prediction–feedback loop in the cortex. Bodily feedback can be seen as an ongoing process of comparing neurally registered body states to actual body states. This allows the organism access to an embodied dynamic comprising a representation of a prediction of how its body will be perturbed by the perceived ECS.
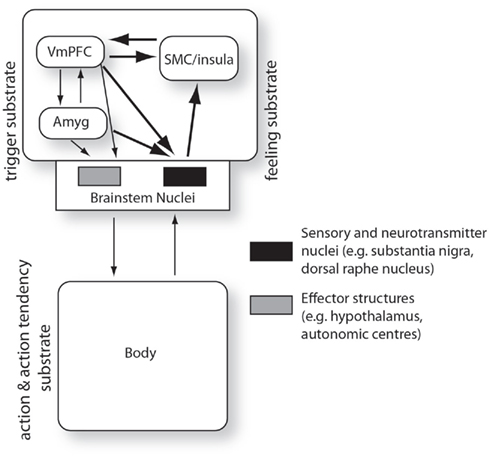
Figure 4. Brain–body prediction–feedback loops: adaptation of Damasio’s brain–body loop theory of emotion and emotional feeling. The “as-if body loop” of Damasio is superimposed (thick arrows) on the “body loop.” The diagram can be seen to encapsulate at least two nested prediction–feedback regulatory loops implemented by VmPFC and Amyg projections: firstly, the brain-stem nuclei are provided a coarse predictive representation of body state; secondly, the SMC/insula is provided a more contextual predictive representation of body state as it relates to exteroceptive and proprioceptive late stages of processing in relation to the emotionally competent stimulus. Abbrev: VmPFC (ventral-medial prefrontal cortex), Amyg (amygdala), SMC (somatosensory cortices). Adapted from Lowe and Ziemke (2010).
This description of an emotion event broadly captures the essence of the ATPFL. However, it may be the case that subthreshold activation exists in body registering brain sites. Such activity comes to represent the body to the extent that it moves to a suprathreshold state, e.g., following input from the body that strengthens the neural activation. The ATPFL explanation suggests, therefore, that the as-if body loop, consistent with Damasio, is functional in situations of relative certainty concerning the relation of the ECS to the agent whereas uncertainty requires bodily feedback (a type of somatic marker).
Emotion regulation – the function of ATPFL
Why should the ATPFL be of functional value? The straight answer is it affords efficient emotion regulation. The above-mentioned “As-if body loop” interpretation appears consistent with the exposition of Koole (2009) concerning the primary and secondary emotional responses the latter of which permitting up- or down-regulated emotional activity. Koole suggests: “People’s primary emotional response presumably reflects their emotional sensitivity, whereas their secondary emotional response presumably reflects emotional regulation” (p. 7). In this case “sensitivity” can be interpreted as “prediction11,” i.e., predisposition to emotional response based on past experience (or ontogenetically developed tendency) while the secondary regulatory component can be interpreted as “feedback” (see Figure 5 for visualization of this up/down regulatory feedback perspective). From a computational perspective (neural field theoretic perspective – see last section), following a suprathreshold primary response this “prediction” might amount to an OR gate whereby PEs concerning the somatovisceral/action tendency representation will either amplify or destabilize (down-regulate) neural-dynamic activity. Where activation is subthreshold, the primary response might be better described in terms of an AND gate such that somatovisceral feedback, insofar as it matches primed activation sites on the field, may only induce suprathreshold activation. In the latter case, the uncertain (unlearned) situation requires bodily feedback to disambiguate the emotional context (cf. Damasio, 1994).
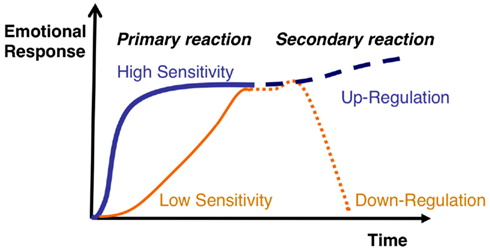
Figure 5. Model of emotional sensitivity versus emotional regulation: taken from Koole (2009). The emotional episode may be viewed as a neural-dynamic representation (our argument) where the primary reaction serves as the prediction of the emotion state and may become stabilized depending on the strength of the prediction. Stabilization would thus occur following some parameterized suprathreshold activation and at such a point activation may impact on behavioral and cognitive systems over an emotion episode. Weaker predictions may not be stabilized. In either case, feedback from the secondary reaction may lead to down- or up-regulation (self-amplification, also see Lewis, 2005). Reprinted with permission.
Frijda (2004) has suggested that the value of emotional feeling is in its (social) behavioral and homeostatic predictive and regulatory effects. From the perspective of (social) behavioral predictive regulation, emotional feeling furnishes the organism with information concerning the social acceptability of a particular action. For example, aggression might provide short-term benefits concerning the weakening of a perceived competitor but at the cost of social respect and possible previously existing friendship. Down-regulating (dampening) emotional activity associated with inappropriate action may therefore be a useful option consequent to an ability to represent (feel) the emotion as action tendency. From the perspective of homeostatic regulation, Frijda points to the predictive importance of behavior availability. Behaviors that tend to be evoked by particular emotions, e.g., aggressive actions following anger, may just not be feasible to the organism according to physical and energetic resources. The competitor may be perceived as physically too strong, or the perceiving organism may be fatigued. Again, down-regulating the emotion or otherwise modulating the emotion may be appropriate in such circumstances. Frijda suggests: “[i]f there seems to be nothing one can do in a given emotional contingency, emotion tends to change – from fear or anger, to despair, for instance. Not seeing the possibility for meaningful action can deeply affect emotional motivation” (Frijda, 2004, p. 166). The opposite may also be true where emotions are “up-regulated” (augmented) “[b]y contrast, if actions are readily available, motivation may be enhanced, say, from irritation to outright rage.”
The same arguments Frijda posits for being able to foresee possible future actions according to acceptability and availability also applies to predicting possible action tendencies: in terms of acceptability, action tendencies increase the likelihood of producing inappropriate behavior as well as expressing emotional states that may (socially) inappropriately belie the underlying activity; in terms of availability, readiness to action is metabolically costly, and energetic resources used on actions that may not be acceptable or available are lost that might otherwise be used serving the organism’s “total set” of needs and concerns. Moreover, an emotion episode rooted in a tendency to act reduces the capacity and speed with which cognitive–behavioral programs – that concern more deliberative processing, e.g., planning, decision making, declarative knowledge construction – appropriate to the situation may be invoked. Koole (2009), with particular reference to negatively valenced emotions, also alludes to the above-mentioned points: “Negative emotional states are costly, because they mobilize a wide array of mental and physical resources within the individual […] emotion regulation may thus be adaptive, by allowing individuals to conserve these resources by promoting a rapid return to hedonically agreeable states” (p. 15).
Allostatic regulation – ATPFL and resource mobilization
On the basis of the above discussion, regulation of emotion state occurring through ongoing prediction–feedback loops of motivation-grounded action tendency across an emotion episode seems apt. We suggest that the key neurophysiological substrate by which this may occur is the “as-if” body loop proposed and evidenced by Damasio and Bechara, utilizing phase-synchronized neural activity (representations) in zones of convergent proprioceptive, exteroceptive, and interoceptive processing routes (cf. Damasio, 2010). We suggested that, emotional feelings rooted in such a neural-dynamic representational substrate, provide the foundation on which ongoing prediction–feedback loops can operate.
Using the ATPFL position we may accommodate and even reconcile perspectives previously referred to in this article. For example, we can identify two PE (prediction error) scenarios requisite to ATPFL – (1) overestimation of action tendency, (2) underestimation of action tendency – that may precipitate “down-regulation” and “up-regulation” or “stabilization/maintenance,” respectively (cf. Koole, 2009). In the case of (1) overestimation, following learning, a particular ECS could induce a pattern of activation in areas of the brain that enable representation of action tendencies (i.e., somatovisceral changes that are signaled by neuromodulatory activity in appetitive and defensive networks). The bodily response invoked by the trigger brain areas, however, will not exactly reproduce the predicted action tendency. It may be overestimated by the neural-dynamic representation according to availability of energetic or skeletomusculature resources – the organism may be fatigued through lack of sleep, nutrition, water, or intense physical activity. The mismatch (error) may then serve to down-regulate the action tendency. This will occur in the case of lack of resources and provoke the recruitment of other cognitive and behavioral programs following termination, or redirection (e.g., from one emotion state to a less costly emotion state – Frijda, 1986, 2007), of the emotional episode. In the case of (2) underestimation, the relationship between the organism and the ECS may not have been well learned and consequently the bodily response that is activated may be stronger than that which is anticipated in the neural representation. In this case, consistent with Damasio (cf. Bechara and Damasio, 2005), the body guides decision making where outcomes are uncertain or not well learned. Such activity may lead to a relative loss of (volitional) control (cf. Leventhal, 1980) where “the spontaneous motor system overrides the control of the voluntary system” (Leventhal, 1980, p. 169). Such error-triggered activation might be viewed in terms of a secondary emotional response (Koole, 2009) leading to a down-regulation of emotional activity in order to reassert volitional control.
Sterling’s (2004) notion of allostasis might also be understood in terms of ATPFL, particularly concerning up-regulation of emotional responsivity. For example, suprathreshold neural representation of action tendency (predictions/feelings) can recruit metabolic resources in the service of emotional behavior. This would precipitate up-regulation at the secondary response constrained, nevertheless, by availability and acceptability concerns. The perceived availability of the action tendency is dependent on prediction–feedback loops but whilst Sterling’s view suggests that basal homeostatic activity may be compromised according to an agent–environmental pressure to recruit metabolic resources to act, a relative lack of available resources (overestimation), or alternatively an unanticipated abundance of metabolic resources (underestimation), may serve to modulate the prediction (modify associations between trigger and feeling neural-dynamic patterns). This adaptation is schematized in Figure 6 and is broadly consistent with the predictive coding perspective of Friston (2002; also see Kilner et al., 2007), i.e., that prediction minimization is a self-organizing process following reciprocal interactions between higher and lower processing levels where in the case of emotion the body provides the “lower level” proffering feedback to the “higher level” that is the brain.
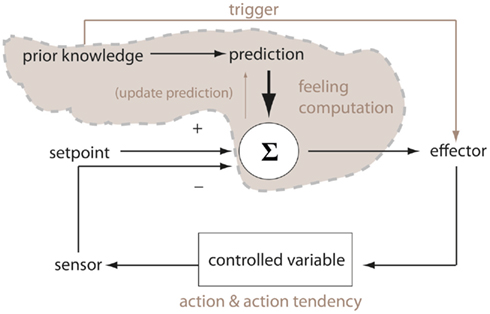
Figure 6. Prediction–feedback control loop: adaptation of Sterling’s control theoretic perspective of allostasis. The additional feedback arrow relating to “update prediction” highlights our perspective on the relation between emotional feeling and action tendency. All labels and arrows in gray are additions to the original diagram of Sterling (2004). The primary emotional response concerns a prediction (emotional feeling) that may override homeostatic set points – make less sensitive to negative error – in order that metabolic resources may be recruited, e.g., in an emergency. The “effector” in this case amounts to parts of the brain, e.g., hypothalamus, brain-stem, that instigate somatovisceral changes preparatory to action. The “controlled variable” pertains to the metabolic resources that are aroused/galvanized. The (desensitized) “setpoint” determines whether sufficient resources are available for the particular action tendency (e.g., an active response based on approach or withdrawal). At such a point a secondary emotional response ensues (regulation, cf. Koole, 2009) where up- or down-regulation of the action tendency occurs and prediction may be updated. For example, negative feedback would lead to down-regulation of emotional response and the prediction schema being updated such that set points are not so desensitized in the future. This, however, may also be overridden by higher (contextual) levels of processing. This control loop diagram can be mapped onto the Damasio neural-anatomy diagram in Figure 4.
The ATPFL is, therefore, best apprehended as an allostatic regulatory mechanism since neural patterns representative of anticipated body states also exert a top-down modulatory effect as in allostasis. This can also be inferred from Damasio (2010): “[t]he brain states, which correspond to certain mental states, cause particular body states to occur; body states are then mapped in the brain and incorporated into the ongoing mental states. A small alteration on the brain side of the system can have major consequences for the body states … likewise, a small change on the body side … can have a major effect on the mind once the change is mapped and perceived,” (p. 96).
ATPFL in Goal-Directed Behavior: An Application
In the previous section, the notion of emotional feeling as a predictive mechanism in a dynamically realized control system, an ATPFL, was proposed. It was suggested that being able to anticipate one’s future bodily changes that are preparatory to action might circumvent:
1. Increased likelihood of triggering inappropriate behaviors and expressions,
2. Misuse of resources – to the detriment of:
a. Basic homeostatic maintenance, and …
b. Time and efficiency of processing according to invocation of cognitive and behavioral programs.
These two aspects were related to Frijda’s notions of acceptability and availability, respectively, as they concern, according to his perspective, emotional feeling as prediction of action outcome. We then suggested that this perspective could be adapted in order to posit a functional role of feeling in the context of predicting action tendency.
In order to make clearer still the importance of the two above points to organismic adaptivity and viability (functionality), we discuss emotions and emotional feelings in relation to point 2. but with respect to the specific case of emotion regulation of multiple needs or goals and with respect to both biological and artificial (robotic) agents.
ATPFL as a Mechanism for Mediating among Multiple Goals
Goal-directed behavior is viewed as one of three primary functions that is served by efficient emotion regulation according to Koole (2009) the others being “satisfaction of hedonic need12” and maintenance of personality integrity. The pertinence of goal-directed behavior in emotion regulation has been interpreted in terms of feedback, above all, in the service of learning, e.g., Baumeister et al. (2007) – also see Carver and Scheier (1990), and Carver (2003). In the view of Baumeister et al., emotions are motivating – organisms seek to act to foster positive emotions and reduce negative emotions – and learning affords tailored predictions of contexts in which positive or negative emotions are likely. Frijda notes that trading off all the concerns (including goals) of the organism (homeostatic balancing) is a critical mediating influence in action selection: “[a]ction is the result of the cost-benefit balance over the consequences of the action for the total set of the individual’s concerns” (2004, p. 164). Koole (2009) similarly notes this trade off: “Some of the functions of emotion regulation may extend beyond single goals. In particular, emotion regulation may allow people to balance multiple goals” (p. 16).
The term “goal-directed” has to be used with caution since it is considered often misrepresented. Frijda (1987) in his critique of the work of Oatley and Johnson-Laird (1987), for example, suggested that the authors’ use of the term was inaccurate: “it is confusing when the word ‘goal’ is used for what we commonly designate by ‘wish’ or ‘interest,’ or ‘concern”’ (Frijda, 1987, p. 53/54). Essentially, goal-directed behavior refers to outcome expectation based on plans arrived at through deliberative processing (cf. Daw et al., 2005; Frijda, 2010). This is to be distinguished from habitual activity – largely stimulus-driven behavior that, unlike goal-directed behavior, is relatively inflexible following changes in reinforcement outcomes (cf. Daw et al., 2005). Goal-directed behavior is also to be distinguished from impulsive activity (Frijda, 2010) which: (1) comprises automatic emotional responses that are unconscious, (2) occurs at an early (and possibly premature) contextual processing stage (e.g., responding aggressively to insult to someone that is bigger than you), (3) entails urges to act on the expectancy of gain following behavior completion. For Frijda (2010), habits and impulses pertain to stimulus-driven “aims” whereas goal-directed behavior starts with the goal in mind and requires deliberation as to how to achieve this (somewhat) irrespective of stimulus presence.
Many researchers consider emotion functionality in terms of states elicited following interrupts on goal-directed behavior (Simon, 1967; Toda, 1982; Oatley and Johnson-Laird, 1987; Rolls, 1999; Kreibig et al., 2010). Such “goals,” however, may be interpreted as being alternatively based on deliberated action plans or motivated states regarding present needs divorced from detailed action schemata. Rolls (1986, 1999, 2000, 2005) has adopted a reinforcement learning perspective on emotions and their role in goal (or perhaps “need”)-directed behavior. He suggests that emotions can be defined as “states elicited by rewards and punishments, including changes in rewards and punishments” (2000, p. 178). For Rolls, different emotions are elicited by different primary and secondary reinforcers. The specific reinforcement contingency (RC) also determines the particular triggered emotion. Anger, for example, is a state elicited by the omission of a previously expected positive reinforcer; fear pertains to the presence of a negative reinforcer; happiness relates to the presence of a positive reinforcer (see Figure 8 – left-side – for visualization of the dimensional structure of Rolls’ perspective). The emotion that is triggered by the RC may thereafter “help to produce persistent and continuing motivation and direction of behavior, to help achieve a goal or goals,” (Rolls, 2000, p. 181, authors’ italics). This perspective might also be extended to incorporate more fully the notion of prediction. As mentioned in Section “Prediction in the Brain,” Schultz (1998, 2007) made pioneering discoveries on the role of dopamine as a prediction and learning signal that enables organisms to temporally associate (primary and secondary) reinforcers. Anger, on this basis, may be seen as a state elicited by the omission of a positive reinforcer predicted to occur at a certain point in time, i.e., feedback provides a negative/omission error. Happiness and fear, on the other hand, are states elicited by positive and negative reinforcers, respectively, according to time-sensitive learned expectations.
In “classical” AI (or perhaps “enlightened” AI), the relevance of emotions to goal/need-directed behavior has been noted. Simon (1967) likened central nervous system activity to a serial information processor where emotions have two essential functions: (1) as “goal”-terminating mechanisms, (2) as interruption mechanisms. These mechanisms were considered to allow organisms to achieve a number of goals whilst simultaneously meeting urgent needs in real-time. More recently, Cañamero (2003), taking inspiration above all from Frijda and Damasio, has proposed that emotions offer important means for action selection where homeostatic regulation of fulfillment of multiple needs is considered critical to the long-term viability of the (robotic) agent. This follows the thinking of McFarland and Spier(1997; McFarland and Bösser, 1993; McFarland, 2008) who have advocated the need for robots to react to real-time environmental opportunities (opportunism) when considering homeostatic regulation of multiple internal needs and goal/need directives. Avila-Garcìa (2004), Avila-Garcìa and Cañamero (2005) have suggested that emotion-relevant synthetic hormones allow robotic agents to trade off the need for opportunism with the need to persist in the pursuit of a particular need-fulfilling goal. More recently, work by Lowe et al. (2010a), Montebelli et al. (2010) has considered the importance of different types of energy constraints to the homeostatic regulation of adaptive behavior of robotic agents with multiple goals. Lowe et al. (2008) allude to the fact that agents’ goals may require different metabolic/physiological resources in order to be successfully carried out and such resources must also be distributed appropriately to those effector systems that are prioritized during the particular behavior. This is true of the elaborated action tendencies that are seen to underlie emotions. For example, as Ekman (2003) points out, when we are angry we tend to feel a rush of blood in our upper arms and torso, presumably preparing us for an aggressive response (approach tendency) appropriate, for example, to neutralizing the obstacle to our present goal(s). In the case of fear, on the other hand, we feel a sensation of coldness in our upper body as blood drains away from the upper body areas implicated in aggressive responses so as to provide energetic resources to our legs priming a potential flight response.
On the basis of the above, an estimate of energy costs to carrying out particular behaviors, or to galvanize action tendencies, pertains not only to the goal in mind or available need but must account for all goals relevant to agent viability and well-being. If a particular goal is too costly to achieve in respect to the goal set, it may not serve the agent to persist in the goal/need-directed activity. We propose that ATPFL allows for mediation among goal-directed behaviors in the sense that predictions of action tendencies may be down-regulated if goal/aim-directed behaviors are not considered available regarding energy costs with respect to the total goal set.
How do goal/need junctures or reinforcement contingencies, and the emotional states that they provoke, inform agents how to act? Oatley and Johnson-Laird (1987) consider emotional activity as guiding actions permitting transitioning between goal/need-based states. In their “cognitive theory of emotion,” Oatley and Johnson-Laird list five of the basic emotions (they do not consider “surprise” as an emotion) for which goal/need-based behavior may be modulated. The emotion states, the conditions of their elicitation, and the goal/aim-(re)directed behavior they promote are listed in Table 1.
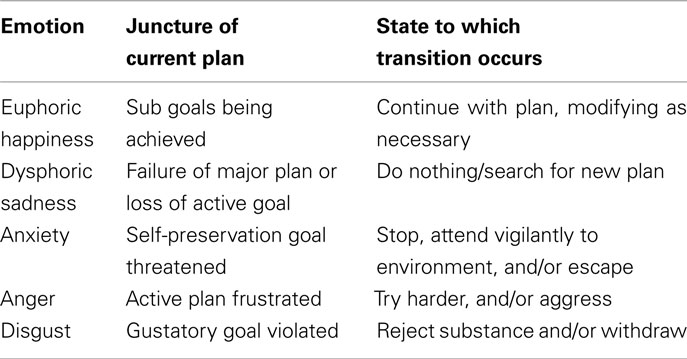
Table 1. Oatley and Johnson-Laird’s, 1987) list of basic emotions and their relevance to goal/need-directed behavior.
Similar to Damasio (1994), Oatley and Johnson-Laird (1987) suggest that emotions can limit a search subspace and circumvent problems inherent in traditional Artificial Intelligence (AI) planning programs. Emotional states are important for generating modes whereby a suite of goals, action possibilities, and skills may be invoked. Frijda (1987), in his emotions as action tendencies perspective, in a direct response to Oatley and Johnson-Laird’s paper, largely concurred with the authors’ “goal”-directed perspective on emotion whereby both event-context and resource competition constrain action selection in the service of a particular goal:
“[e]motions, one can say, clamour for control precedence for the actions that they motivate, or for the abandonment of actions or plans under way … [c]ontrol precedence means claiming exclusive access to available resources for the goal under execution, or readiness to interrupt work on the goal under execution on behalf of the precedent one,” (p. 52).
However, Frijda indicated that the presumed innate predisposition to perceive goal junctures (GJs; as emotion triggers) and associations between them and transition states (effective detailed action plans) was at odds with existing research that “could find the elementary qualities of pleasure and pain, and no more” (Frijda, 1987, p. 52). Frijda instead posits an appraisal theoretic angle according to two phases of emotion elicitation: “The first phase is assessment of the fact that a goal is achieved or promises to be so, or on the contrary is threatened or has failed; I call this ‘relevance appraisal.’ The second phase is assessment of the particular type of juncture at which this happens; I call this ‘context appraisal”’ (Frijda, 1987, p. 53). This description implies a secondary deliberative processing phase and can be likened to the Koole (2009) and Kuhl (2008) notion of emotional responses consisting of primary (sensitive) and secondary (regulatory) stages, as discussed in Section “Emotional Feelings: Action Tendency Prediction–Feedback Loops.” Reconciling these two views we can say that the second phase/stage of the emotional response concerns a deliberation of availability and acceptability (see Emotional Feelings: Action Tendency Prediction–Feedback Loops) which induces up- or down-regulated emotion. This deliberation must necessarily account for the multiple goals that the agent is required to mediate among. A key output of a neural-dynamic representation (prediction) of action tendency has been suggested to be with regard to the biasing of available behaviors in appetitive and defensive networks.
On the basis of the above, a full emotion episode may require the presence of deliberative processing. It is, however, conceivable that a secondary emotional response could be induced simply by delayed bodily feedback and therefore deliberation may concern Frijda’s acceptability, but not availability, estimations. However, the computation concerning availability must also take into account whether or not carrying out the particular behavior is energetically tenable not just for the present goal/need-directed behavior but also with respect to the need to utilize resources for other goals/needs, i.e., the total goal/need set. On the other hand, it is not clear that deliberation is always necessary for a primary emotion response to occur (e.g., see LeDoux, 1996). Rather, in the spirit of Oatley and Johnson-Laird (1987), this may merely necessitate a particular sensitivity to reinforcement contingencies particularly if learning may be simplified to (conjunctions and negations of) phasic signals emanating from appetitive and defensive networks13. Such an emotional system need not induce more or less hard-wired action transitions but rather bias sub-trees of action tendencies within appetitive and defensive networks. Such a perspective amounts to a compromise between the discrete action program and motivation-grounded perspective therefore. In reference to Section “What is An (Emotional) Action Tendency and What is its Relation to (Overt) Action?” we might envisage the emotion episode as governed by ATPFL according to Figure 7. In this case a “RC” or “GJ” triggers the initial emotional response as captured by a low-dimensional feeling neural-dynamic representational substrate – presented here in the form of pleasantness, arousal, dominance (approach–withdrawal) or “PAD” dimensions. The feeling state has a fundamental role in regulating the action tendencies it represents (or comes to represent). Following feedback from somatovisceral and event-context perception/appraisal PAD is updated and then induces a secondary, regulatory, emotional response. As referred to above, the deliberative processing phase (“acceptability” and “availability” feedback) preceding the secondary emotional response also includes assessment of how action tendencies impact on resource availability that affects the goal/need set. Deliberation may be particularly relevant for mediating among goals (in mind) rather than needs (more stimulus-driven) where, in the case of the former, energetically costly behavioral persistence may be required in order to obtain any value from the sequences of steps that lead to the ultimate goal.
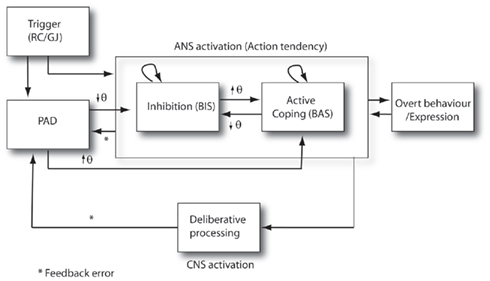
Figure 7. Schema of emotion regulation of goal-directed behavior. Following Figure 3, PAD (pleasantness, arousal, dominance) has a key regulatory effect on action tendency and is a fundamental component of the full emotion episode. Feedback, in the case of suprathreshold activity may produce acceptability/availability mismatch errors, or in the case of subthreshold activity, galvanize neural activation that comes to represent the action tendency. See main text for details.
The emotion episode in the context of goal-directed behavior, as depicted in Figure 7, can be described sequentially as follows:
1. Trigger: an emotional stimulus (ECS) in the context of a RC/GJ is perceived;
2. Primary emotion response (sensitivity/priming): changes in emotional feeling representational space occur simultaneously in neural-dynamic representational regions in the brain (e.g., in somatosensory cortices as captured by PAD dimensional space) – and in the ANS via trigger cites in the brain (e.g., amygdala, hypothalamus, brain-stem);
3. Secondary emotion response (regulation): bodily and cognitive–behavioral feedback which may be more or less automatic or deliberative (evaluation/appraisal of action tendency). This ensues as a function of certainty of outcomes concerning:
a. Availability: internal resources that impact on not just individual goals but the entire goal/need “set,”
b. Acceptability: (social) event/GJ, e.g., actual or relative reward or punishment,
4. Strategic/tactical action selection (cf. Cacioppo et al., 2000): goal-persistence or goal-abandonment tendencies and actions may be affectively or emotionally marked – PAD will regulate a persistent bias of appetitive–defensive networks and specific branches of the search space. This occurs:
a. After 2. when suprathreshold but may be up- or down-regulated
b. After 3. when subthreshold, i.e., following context (including somatovisceral) disambiguation,
5. Overt action selection: active coping strategy (BAS – see McNaughton and Gray, 2000 and see Section What is An (Emotional) Action Tendency and What is its Relation to (Overt) Action), inhibition/attention–orientation (BIS);
6. Feedback from behavior will modulate all of the above over the emotion episode.
In the next sub-section, we will describe in more detail the means by which inhibition and active coping might be emotionally regulated according to goal contexts.
ATPFL: Inhibition and Active Coping at Goal Junctures
The view of emotion regulation of goal-directed behavior that we propose is somewhat complementary to that of Baumeister et al. (2007) that concerns anticipation and feedback. However, Baumeister et al. (2007) suggest that emotions primarily function as feedback systems that promote the elicitation of behaviors that anticipate future emotional states. For these researchers, “automatic affective responses” guide online behavior, while feedback (supporting an emotionally reinforced learning) allows for the updating of such affective responses according to a priming of, above all, cognitive systems for context evaluation. Here, something like core affect (pleasantness, arousal) is of adaptive guidance to online behavior and directly triggered by relevant stimuli (ECSs) but full emotions are, for the most part, dysfunctional to behavior in Baumeister et al.’s view and instead serve only for learning purposes (feedback). To illustrate the comparison, we refer to Baumeister et al.’s perspective as exemplified in the context of aging:
“[f]indings of aging also seem to support the feedback theory of emotion rather than direct causation. Carstensen et al. (1999) have proposed that as people get older, they shift from emphasizing acquiring knowledge toward emphasizing regulating emotion. Carstensen et al.’s broader assumption is that the value of acquiring knowledge is inversely proportional to the time one has left in life, and so as the person begins to recognize that time is growing shorter, he or she will downplay that goal,” (p. 180).
Alternatively, or complementarily, as individuals/organisms age, they tend to have less metabolic/physiological resources to expend on emotions and emotional overt behavior. A tendency to be less emotional (to down-regulate at the secondary stage of the emotional response, cf. Koole, 2009) as one ages, therefore, may be explained according to overestimation of action tendency following the initial representation of an emotional contingency in the service of goal/need-directed behavior. On the one hand, therefore, aging organisms have more knowledge to draw upon regarding how to respond behaviorally to junctures in goals, and on the other hand, they have less resources available to produce emotion states (rooted in action tendency changes) that would promote flexible online use of cognitive and behavioral programs as well as learning from feedback of the enduring emotional state. As the organism ages, it is also less likely to underestimate its emotional activity rooted in action tendency as availability of metabolic/physiological resources are less likely to overwhelm learned predictive and represented states. Aging, on this basis, can be seen as a process of moving gradually from up-regulation of emotion states to down-regulation of emotion states with respect to goal-directed behavior. Essentially, an active coping response is less required as the individual ages. In general, efficient full emotion guided behavior requires the learning – through ATPFL – of efficient emotion regulation.
Recent research by Boureau and Dayan (2010) provides further context within which we might view emotions according to reinforcement contingencies or GJs. In relation to the phasic neuromodulatory signals of dopamine (DA) and serotonin (5HT) that appear to be central to propelling learning and action, Boureau and Dayan note that contrary to popular understanding DA neurons phasically respond in some aversive/defensive contexts while 5HT neurons may phasically respond in appetitive contexts. The researchers produced a dimensional model of DA and 5HT influence in relation to appetitive and aversive contexts, on the one hand, and to active and inhibitory contexts on the other. They suggested that DA neurons fire in the context of appetitive expectation (a positive valence signal) but also with respect to action tendency (invigoration). In the latter case, the invigorated response can be seen as indicative of an expected relative reward in the sense that through action, punishment is expected to be avoided. 5HT, on the other hand, is observed where punishment is expected but also where inhibition of ongoing behavior occurs. Since the latter case can also occur in an appetitive context (expected omission of reward), to make consistent with the popular understanding of 5HT function the appetitive–inhibitory context, signaling is considered indicative of expectation of relative punishment, i.e., failure to achieve reward. This failure might be interpreted as occurring either as a consequence of continuing a behavior where inhibition is appropriate (Boureau and Dayan, 2010), e.g., in a social context, but it may also conceivably occur in the absence of finding an alternative active coping response.
The junctures presented by Boureau and Dayan (2010) concern: appetitive reward expectation; an aversive punishment expectation; omission of expected reward; omission of expected punishment. As such, these junctures can be compared to Rolls’ dimensional approach describing emotion elicitation at reinforcement contingencies (see Figure 8). Boureau and Dayan do not refer to emotion elicitation but we suggest that expected relative or actual punishment inducing an inhibition of ongoing behavior might precipitate an active response phase that is galvanized by an emotional action tendency. Consistent with Lang and Bradley (2010) an emotion episode may, in the context of motivated behavior, be characterized by an inhibition stage (step 1 in Figure 8 – right; activating the BIS system, McNaughton and Gray, 2000) where uncertainty resides concerning the appropriate action tendency or emotional response (a prediction is not fully formed according to a neural-dynamic representation based on ATPFL). Following a stage of more or less deliberative processing (Step 2 in Figure 8 – right; somatovisceral and environmental contextual disambiguation), where up-regulation occurs (emotion regulation, Koole, 2009), an appropriate action response may be initialized permitting the agent to move into an active coping phase leading to expected actual or relative reward. Down-regulation on the other hand, following deliberation, may lead to continued inhibition or less active coping responses, e.g., calling for help. As a simplification, a magnitude negation of DA and 5HT might inform of the appropriate response orientation in this case and, following learning, be of statistical predictive value, i.e., noting that the mapping between approach–avoidance to appetitive–defensive networks (behavioral possibilities) is not entirely unambiguous (again see Frijda, 2010).
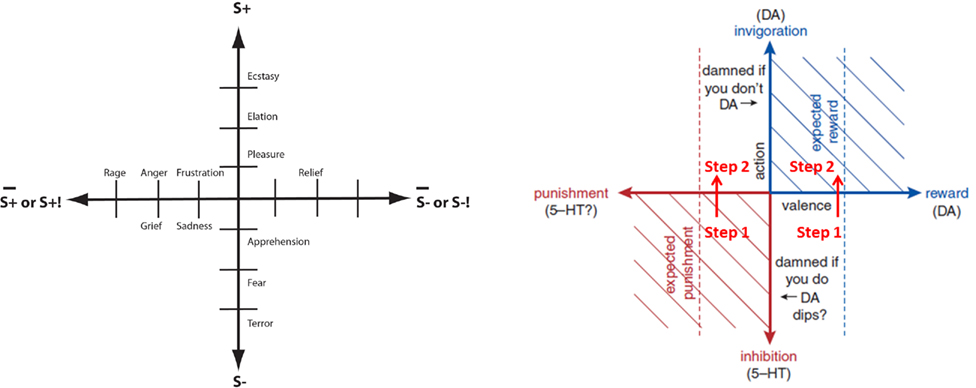
Figure 8. Reinforcement–contingent emotional dimensional models. Left: Rolls’ (1999) dimensional model of emotion-based on omission (! | −) or fulfillment (+ | −) of reinforcement. Reprinted with permission. Right: adaptation of Boureau and Dayan (2010) model (step 1 and step 2, and red arrows denoting step transitions have been added). The Rolls model can be mapped onto the Boureau and Dayan model where activation spans outward from the origin (center) and cuts across the diagonals of the four quadrants in the Boureau and Dayan model. In this manner, the upper right quadrant provides the S+ dimension, the lower left quadrant the S− dimension, the upper right quadrant the S− omission/termination dimension, the bottom right quadrant the S+ omission/termination dimension. Reprinted with permission.
This ATPFL interpretation of Boureau and Dayan’s (2010) model according to active coping provides an elaboration of the Oatley and Johnson-Laird (1987) explanation. Emotion regulation provides insight into the dynamics of behavior that may occur according to GJs as a function of comparison of expected (relative and actual) rewards and punishments. This offers an, above all, motivation-grounded example of the facility of the ATPFL whilst simultaneously proffering a tentative bridge to discrete action program perspectives of (basic) emotions.
Conclusion
In this article we have gone through three main stages of enquiry:
1. what is the considered view of the relationship between emotional feeling and action tendency?
2. can the considered view be understood according to a perspective that likens emotional processing to other types of processing in the brain, i.e., one that involves prediction and feedback enabled by the mechanism of neural-dynamic representation?
3. how can this new perspective be understood with respect to a purported key function of emotion: regulating goal/need-directed behavior?
In relation to 1., we distinguished between discrete action program and motivation-grounded theorists of emotions who have investigated the relation between emotional feeling and somatovisceral changes constitutive of action program or tendency, respectively. This coarse division was made to render more explicable existing controversies in the field. We outlined in 2. the notion that emotional feeling is functionally critical (insofar as it reliably maps to neural-dynamic stable representations) as it provides an operational foundation for “action tendency prediction-feedback loop(s)” which we abbreviate to ATPFL. In relation to the perspective elucidated in point 2., however, emotional activity as it pertains to action tendencies tracked by interoceptive (and proprioceptive) neural-dynamic representational processes may operate differently to sensorimotor modes of processing. Other senses in being focused on the outside world are less subject to the messy and latent dynamic effects of ongoing bodily changes to emotion evoking stimuli. Nevertheless, we have suggested that, using mechanisms not dissimilar to sensory and motoric perceptual systems in the brain, i.e., comprising prediction and feedback, emotional feelings might best be understood as predictive mechanisms for regulating action tendencies. This perspective has been put forward on the basis of neural–anatomic and computational/functional feasibility and can also be seen as an extension to other perspectives that implicitly or explicitly attribute a predictive or/and regulatory role to emotion (e.g., Leventhal, 1980; Rolls, 1999; Frijda, 2007; Laird, 2007; Koole, 2009; Damasio, 2010). Mechanistically, it is argued that the perspective posited requires a neural-dynamic representational substrate upon which predictions and feedback can be compared over the varying brain and bodily time scales inherent in the emotion episode, as it (the emotion), for example, tracks and organizes, or recalibrates, a goal/need-directed behavior. The top-down/bottom-up predictive–feedback loops thereby permitted offer a means by which energetic resources may be appropriately allocated among the constituents of a goal/need-directed behavior set and also guards against the elicitation of inappropriate behaviors and expressions. In relation to the ATPFL explicated and exemplified in points 2 and 3., respectively, advocates of the (motivation-grounded) view of emotion might suggest that positing the existence of more than two dimensions represented in feeling neural-dynamic substrates is not parsimonious given existing evidence – that more unequivocally argues for the dimensions of valence (pleasantness) and arousal. However, the existence of a single dimension additional to the core affect perspective (embraced by, e.g., Russell, 1980, 2003; Frijda, 1986, 2010; Cacioppo et al., 1992, 2000; Baumeister et al., 2007) concerning approach–withdrawal tendency or dominance: (1) has received strong evidential support where existing measures to track it may be unreliable (e.g., Russell and Mehrabian, 1977; Davidson, 1993; Mehrabian, 1996; Mauss and Robinson, 2009), (2) may still be considered to offer a low-dimensional ANS-specification of emotion broadly consistent with the motivation-grounded perspective (rooted in core affect; e.g., see Krieglmeyer et al., 2010), and (3) may be conceived according to core learning and behavior guidance signals in appetitive and defensive networks (cf. Boureau and Dayan, 2010). Furthermore, importantly, a core affect + perspective affords a bridge between dimension theory and basic emotion theory (as also usefully studied by Christie and Friedman, 2004) which may be necessary to furthering the theoretically dense field of emotion science.
The present article began with an allusion to how the proposed dynamic interdependence of feeling–action tendency reflects a relative inseparability between cognition and emotion – emotion as a form of action tendency regulation involves prediction, memory, representation and, in some cases, planning, i.e., anticipation over longer-time scales or anticipation of other emotion episodes14. In this sense, emotional activity bears the hallmarks of classically conceived cognitive processes. It was also alluded to, however, in Section “The Relationship Between Emotional Feeling and Action Tendency: A Review,” that any full-fledged theory of emotion should account for the role of triggers, action and action tendencies, and feelings. In this article, on the other hand, we have focused on the latter two emotional phenomena. In the previous section, however, we made reference to reinforcement and goal-directed behavior contingencies (e.g., Oatley and Johnson-Laird, 1987; Rolls, 1999; Boureau and Dayan, 2010) as effective emotion triggers which need not, in all circumstances, be the result of deliberative or cognitive appraisal processes. We suggested that the use of conjunctions and negations of the neuromodulator learning/action selection signals key to TD computations in appetitive and defensive (i.e., motivational) systems may encode goal/need contextual information critical to triggering appropriate active coping responses. However, the exact means by which emotion elicitation occurs may involve complex ontogenetic development of cognitive and behavioral programs. We intuit that the relation between stimuli and instrumental approach–avoidance activity as mediated by core affective components (Russell, 1980), are indeed central to such development. However, it has not been the aim of this paper to attempt to address the issue of emotional development, though clearly an important area. Rather, as a first step to better understanding emotions, their relation to cognition and their involvement in goal-directed behavior, it is suggested that a clearer elucidation of the relationship between feelings (both conscious and unconscious) and actions and action tendencies in emotion regulation is required. This article has described a perspective that makes tentative steps in this direction. Emotional feeling states and their constituents are notoriously difficult to track using standard neuroscientific and psychological methods. As Scherer remarks regarding feeling states: “[s]o far, we have little hope of getting even close to measuring the processes represented by (non-communicated feelings)” (2004, p. 139). Postulating global principles of brain–body functioning may help researchers in emotion science to see the wood for the trees. By framing the issue of emotional–cognitive activity according to (1) predictive regulation, (2) goal-directed behavior, (3) use of artificial agents, and suitable mechanisms for studying emotional constituents (e.g., machine learning and DFT), it may be possible to arrive at a computationally tractable means of understanding how emotions are, or how they could be, with respect to organisms that have multiple needs and goals and that are required to exist in the real world.
Conflict of Interest Statement
The authors declare that the research was conducted in the absence of any commercial or financial relationships that could be construed as a potential conflict of interest.
Footnotes
- ^Though for a review on the cultural-constructivist nature of the “intuitive” alternative perspective see Laird (2007).
- ^Here “cognitive” components perception, attention, reflection, and evaluation reciprocally interact with “emotion” components arousal, action tendency, and feeling tone.
- ^Scherer (2004) has criticized Damasio for his focus on the ECS as an innate emotion differentiator. Damasio (2004), however, has suggested that an ECS is underdetermined by evolution – it may acquire the ECS status over the organism’s lifetime and may or may not be viewed in cognitive appraisal theoretic terms. For convenience, it is to this broad definition that we subscribe for the bulk of this article – but see Section “ATPFL in Goal-Directed Behavior: An Application.”
- ^The basic emotions identified by Ekman (1972) and Levenson et al. (1992) are: anger, fear, happiness, disgust, sadness, and surprise. The latter emotion, omitted by Christie and Friedman (2004), has perhaps been most often contended regarding its status (e.g., Oatley and Johnson-Laird, 1987). In the case of the Christie and Friedman study, the basic emotions were derived from a pattern classification analysis of a number of emotion-relevant ANS-activating physiological variables.
- ^James did not advocate that feelings are captured by, or synonymous with, particular neural patterns.
- ^Ekman and colleagues have, however, investigated physiological (interoceptively processed) correlates of emotion – see, for example, Levenson et al. (1992).
- ^Mauss and Robinson (2009) identify at least seven dimensions regularly cited according to discrete action program sympathizers where only a comparatively narrow range of values for each dimension is permissible for a given emotion categorization.
- ^The term stabilization dynamics may appear as a contradiction in terms; however, it captures the neural-dynamic essence of a non-static stabilization–destabilization phenomenon based on the existence of attractors. A stable state will resist perturbatory inputs but strong inputs may destabilize activity shifting the state into another region (e.g., another attractor).
- ^This is somewhat abstractly captured by the population dynamics (local excitation/global inhibition) of DFT.
- ^Damasio (2010) refers to exteroceptive neural “maps” in the brain which track external events a given object of which “engages a sensory probe such as the retina, the cochlea, or the mechanoreceptors of the skin” (p. 76).
- ^According to a DFT neurocomputational account, however, “prediction” might only relate to suprathreshold activation. “Sensitivity” would perhaps better capture the possibility of DFT states being either sub- or suprathreshold.
- ^Emotions will tend to promote future likelihood of experiencing positive affective states and reduce the likelihood of experiencing future negative emotion (also see Baumeister et al., 2007).
- ^Goal junctures as captured by such signals in low-dimensional, e.g., PAD, space also appears tenable when considering the work of Krieglmeyer et al. (2010) who suggest that associative links between valence and approach–avoidance (i.e., a not classically core affective dimension) do not require appraisal but may be automatic and therefore appear grounded upon motivational (appetitive–defensive) systems.
- ^Though the cognitive–behavioral programs to which we allude and that follow stable feeling states may be considered in terms of more deliberative, e.g., linguistic/declarative, forms of processing that are somewhat underdetermined, though not independent from, the emotional feelings.
References
Amari, S. (1977). Dynamics of pattern formation in lateral-inhibition type neural fields. Biol. Cybern. 27, 77–87.
Anderson, C. J. (2003). The psychology of doing nothing: forms of decision avoidance result from reason and emotion. Psychol. Bull. 129, 139–166.
Aristotle, N. (1984). Complete Works [Revised Oxford Translation in 2 Volumes, ed. J. Barnes]. Princeton, NJ: Princeton University Press.
Avila-Garcìa, O. (2004). Towards Emotional Modulation of Action Selection in Motivated Autonomous Robots. Ph.D. thesis, Department of Computer Science, University of Hertfordshire, Hatfield.
Avila-Garcìa, O., and Cañamero, L. (2005). “Hormonal modulation of perception in motivation-based action selection architectures,” in Proceedings of Agents that Want and Like: Motivational and Emotional Roots of Cognition and Action, Symposium of the AISB’05 Convention, (Hatfield: University of Hertfordshire), 9–17.
Baldassarre, G. (2011). “What are intrinsic motivations? A biological perspective,” in IEEE 10th International Conference on Development and Learning (ICDL), Frankfurt.
Baumeister, R. F., Vohs, K. D., DeWall, C. N., and Zhang, L. (2007). How emotion shapes behaviour: feedback, anticipation, and reflection, rather than direct causation. Pers. Soc. Psychol. Rev. 11, 167–203.
Bechara, A. (2004). The role of emotion in decision-making: evidence from neurological patients with orbitofrontal damage. Brain Cogn. 55, 30–40.
Bechara, A., and Damasio, A. R. (2005). The somatic marker hypothesis: a neural theory of economic decision. Games Econ. Behav. 52, 336–372.
Bechara, A., Damasio, A. R., Damasio, H., and Anderson, S. W. (1994). Insensitivity to future consequences following damage to human prefrontal cortex. Cognition 50, 7–15.
Bem, D. J. (1972). “Self-perception theory,” in Advances in Experimental Social Psychology, Vol. 6, ed. L. Berkowitz (New York: Academic Press), 1–62.
Berkowitz, L. (2000). The Causes and Consequences of Feelings. Cambridge: Cambridge University Press.
Boureau, Y.-L., and Dayan, P. (2010). Opponency revisited: competition and cooperation between dopamine and serotonin. Neuropsychopharmacol. Rev. 1, 1–24.
Bradley, M. M. (2000). “Emotion and motivation,” in Handbook of Psychophysiology, eds J. T. Cacioppo, L. G. Tassinary, and G. G. Berntson (New York: Cambridge University Press), 602–643.
Bradley, M. M., and Lang, P. J. (1994). Measuring emotion: the self-assessment manikin and the semantic differential. J. Behav. Ther. Exp. Psychiatry 25, 49–59.
Bradley, M. M., and Lang, P. J. (2000). “Measuring emotion: behavior, feeling, and physiology,” in Cognitive Neuroscience of Emotion, eds R. D. Lane and L. Nadel (New York: Oxford University Press), 242–276.
Bradley, M. M., and Lang, P. J. (2007). “Emotion and motivation,” in Handbook of Psychophysiology, eds J. T. Cacioppo, L. G. Tassinary, and G. Berntson (New York: Cambridge University Press), 581–607.
Cacioppo, J. T., Berntson, G. G., and Klein, D. J. (1992). What is an emotion? The role of somatovisceral afference, with special emphasis on somatovisceral “illusions.” Rev. Pers. Soc. Psychol. 14, 63–98.
Cacioppo, J. T., Berntson, G. G., Larsen, J. T., Poehlmann, K. M., and Ito, T. A. (2000). “The psychophysiology of emotion,” in The Handbook of Emotions, eds M. Lewis and J. M. Haviland-Jones (New York: Guildford Press), 173–192.
Camras, L. A. (2011). Differentiation, dynamical integration and functional emotional development. Emot. Rev. 3, 138–146.
Cañamero, D. (2003). “Designing emotions for activity selection,” in Emotions in Humans and Artifacts, eds R. Trappl, P. Petta and S. Payr (Cambridge MA: The MIT Press), 115–148.
Carstensen, L. L., Isaacowitz, D. M., and Charles, S. T. (1999). Taking time seriously: a theory of socioemotional selectivity. Am. Psychol. 54, 165–181.
Carver, C. S. (2003). Pleasure as a sign you can attend to something else: placing positive feelings within a general model of affect. Cogn. Emot. 17, 241–261.
Carver, C. S. (2005). Emotion theory is about more than affect and cognition: taking triggers and actions into account. Behav. Brain Sci. 28, 198–199.
Carver, C. S. (2006). Approach, avoidance, and the self-regulation of affect and action. Moitv. Emot. 30, 105–110.
Carver, C. S., and Harmon-Jones, E. (2009). Anger is an approach-related affect: evidence and implications. Psychol. Bull. 135, 183–204.
Carver, C. S., and Scheier, M. F. (1990). Origins and functions of positive and negative affect: a control-process view. Psychol. Rev. 97, 19–35.
Carver, C. S., and Scheier, M. F. (1998). On the Self-regulation of Behavior. Cambridge: Cambridge University Press.
Christie, I., and Friedman, B. (2004). Autonomic specificity of discrete emotion and dimensions of affective space a multivariate approach. Int. J. Psychophysiol. 51, 143–153.
Cools, R., Nakamura, K., and Daw, N. D. (2010). Serotonin and dopamine: unifying affective, activational, and decision functions. Neuropsychopharmacology 36, 98–113.
Damasio, A. R. (1994). Descartes’ Error: Emotion, Reason, and the Human Brain. New York: GP Putnam’s Sons.
Damasio, A. R. (1999). The Feeling of What Happens: Body, Emotion and the Making of Consciousness. London: Vintage.
Damasio, A. R. (2003). Looking for Spinoza: Joy, Sorrow and the Feeling Brain. Orlando, FL: Harcourt.
Damasio, A. R. (2004). “Emotions and feelings: a neurobiological perspective,” in Feelings and Emotions, eds A. S. R. Manstead, N. Frijda, and A. Fischer (Cambridge: Cambridge University Press), 49–57.
Damasio, A. R. (2010). Self Comes to Mind: Constructing the Conscious Brain. New York: Pantheon Books.
Davidson, R. J. (1993). Parsing affective space: perspectives from neuropsychology and psychophysiology. Neuropsychology 7, 464–475.
Davidson, R. J., Fox, A., and Kalin, N. H. (2007). “Neural bases of emotion regulation in nonhuman primates and humans,” in Handbook of Emotion Regulation, ed. J. J. Gross (New York: The Guilford Press), 47–68.
Daw, N. D., Kakade, S., and Dayan, P. (2002). Opponent interactions between serotonin and dopamine. Neural Netw. 15, 603–616.
Daw, N. D., Niv, Y., and Dayan, P. (2005). Uncertainty-based competition between prefrontal and dorsolateral striatal systems for behavioural control. Nat. Neurosci. 8, 1704–1711.
Dayan, P., and Huys, Q. M. (2008). Serotonin, inhibition, and negative mood. PLoS Comput. Biol. 4, e4. doi:10.1371/journal.pcbi.0040004
Dickinson, A., and Balleine, B. (1994). Motivational control of goal-directed action. Anim. Learn. Behav. 22, 1–18.
Dretske, F. (1986). “Misrepresentation,” in Belief: Form, Content and Function, ed. R. Bogdan (Oxford: Oxford University Press), 17–36.
Ekman, P. (1972). “Universals and cultural differences in facial expression of emotion,” in Nebraska Symposium on Motivation, ed. J. Cole Lincoln (Nebraska: University of Nebraska Press), 207–283.
Ekman, P. (2003). Emotions Revealed: Recognizing Faces and Feelings to Improve Communication and Emotional Life. New York: Times Books.
Ellsworth, P. C., and Scherer, K. R. (2003). “Appraisal processes in emotion,” in Handbook of Affective Sciences, eds R. J. Davidson, H. Goldsmith, and K. R. Scherer (New York: Oxford University Press), 572–595.
Eyer, J., and Sterling, P. (1977). Stress-related mortality and social organization. Rev. Radic. Polit. Econ. 9, 1–44.
Fanselow, M. S. (1994). Neural organization of the defensive behaviour system responsible for fear. Psychon. Bull. Rev. 1, 429–438.
Friedman, B. H. (2010). Feelings and the body: the Jamesian perspective on autonomic specificity of emotion. Biol. Psychol. 84, 283–293.
Frijda, N. H. (1987). Comment on Oatley and Johnson-Laird’s “Towards a cognitive theory of emotion.” Cogn. Emot. 1, 51–58.
Frijda, N. H. (1995). “Emotions in robots,” in Comparative Approaches to Cognitive Science, eds H. L. Roitblat and J.-A. Meyer (Cambridge, MA: The MIT Press), 501–516.
Frijda, N. H. (2004). “Emotions and action,” in Feelings and Emotions, eds A. S. R. Manstead, N. Frijda, and A. Fischer (Cambridge: Cambridge University Press), 158–173.
Friston, K. J. (2002). Functional integration and inference in the brain. Prog. Neurobiol. 68, 113–143.
Friston, K. J., and Kiebel, S. (2009). Predictive coding under the free-energy principle. Philos. Trans. R. Soc. Lond. B Biol. Sci. 364, 211–1221.
Gläscher, J., Daw, N., Dayan, P., and O’Doherty, J. P. (2010). States versus rewards: dissociable neural prediction error signals underlying model-based and model-free reinforcement learning. Neuron 66, 585–595.
Granger, R. (2006). Engines of the brain: the computational instruction set of human cognition. Artif. Intell. Mag. 27, 15–32.
Gray, J. A. (1982). The Neuropsychology of Anxiety: An Enquiry into the Functions of the Septo-hippocampal System. New York, NY: Clarendon Press/Oxford University Press.
Gray, J. A., and McNaughton, N. (2000). The Neuropsychology of Anxiety. Oxford: Oxford University Press.
Hesslow, G. (2002). Conscious thought as simulation of behaviour and perception. Trends Cogn. Sci. (Regul. Ed.) 6, 242–247.
Jeannerod, M. (1994). The representing brain: neural correlates of motor intention and imagery. Behav. Brain Sci. 17, 187–245.
Kilner, J. M., Friston, K. J., and Frith, D. (2007). Predictive coding: an account of the mirror neuron system. Cogn. Process. 8, 159–166.
Koole, S. L. (2009). The psychology of emotion regulation: an integrative review. Cogn. Emot. 23, 4–41.
Kreibig, S. D., Gendolla, G. H., and Scherer, K. R. (2010). The psychophysiological effects of emotional responding to goal attainment. Biol. Psychol. 84, 474–487.
Krieglmeyer, R., Deutsch, R., De Houwer, J., and De Raedt, R. (2010). Being moved: valence activates approach-avoidance behavior independently of evaluation and approach-avoidance intentions. Psychol. Sci. 21, 607–613.
Krueger, J., Wirtz, D., and Miller, D. T. (2005). Counterfactual thinking and the first instinct fallacy. J. Pers. Soc. Psychol. 88, 725–735.
Kuhl, J. (2008). Einführung in die Persönlichkeitspsychologie: 4. Coping [Introduction into Personality Psychology: 4. Coping]. Osnabrück: Lecture at the University of Osnabrück.
Lang, P. J., and Bradley, M. M. (2010). Emotion and the motivational brain. Biol. Psychol. 84, 437–450.
Lang, P. J., Bradley, M. M., and Cuthbert, M. M. (1997). “Motivated attention: affect, activation and action,” in Attention and Orienting: Sensory and Motivational Processes, eds P. J. Lang, R. F. Simons, and M. T. Balaban (Hillsdale, NJ: Lawrence Erlbaum Associates), 97–135.
Larsen, J. T., Berntson, G. G., Poehlmann, K. M., Ito, T. A., and Cacioppo, J. T. (2008). “The psychophysiology of emotion,” in The Handbook of Emotion, eds M. Lewis and J. M. Haviland-Jones (New York: Guildford Press).
Levenson, R. W. (2003). “Autonomic specificity and emotion,” in Handbook of Affective Sciences, eds R. J. Davidson, K. R. Scherer, and H. H. Goldsmith (New York: Oxford University Press), 212–224.
Levenson, R. W., Carstensen, L. L., Friesen, W. V., and Ekman, P. (1992). Emotion, physiology and expression in old age. Psychol. Aging 6, 28–35.
Leventhal, H. (1980). “Toward a comprehensive theory of emotion,” in Advances in Experimental Social Psychology, ed. L. Berkowitz (London: Academic Press), 149–207.
Lewis, M. (2005). Bridging emotion theory and neurobiology through dynamic systems modeling. Behav. Brain Sci. 28, 169–194.
Löw, A., Lang, P. J., Smith, J. C., and Bradley, M. M. (2008). Both predator and prey: emotional arousal in threat and reward. Psychol. Sci. 19, 865–873.
Lowe, R., Herrera, C., Morse, T., and Ziemke, T. (2007). “The embodied dynamics of emotion, appraisal and attention,” in Attention in Cognitive Systems: Theories and Systems from an Interdisciplinary Viewpoint, eds L. Paletta and E. Rome (Berlin: Springer), 1–20.
Lowe, R., Montebelli, A., Ieropoulos, I., Greenman, J., Melhuish, C., and Ziemke, T. (2010a). “Grounding motivation in energy autonomy: a study of artificial metabolism constrained robot dynamics,” in Artificial Life XII, eds H. Fellermann, M. Dörr, M. Hanczyc, L. Laursen, S. Maurer, D. Merkle, P.-A. Monnard, K. Sty, and S. Rasmussen (Odense: The MIT Press), 725–732.
Lowe, R., Duran, B., and Ziemke, T. (2010b). “A dynamic field theoretic model of iowa gambling task performance,” in IEEE 9th International Conference on Development and Learning (ICDL), Michigan, 297–304.
Lowe, R., Philippe, P., Montebelli, A., Morse, A., and Ziemke, T. (2008). “Affective modulation of embodied dynamics,” in The Role of Emotion in Adaptive Behaviour and Cognitive Robotics, Electronic Proceedings of SAB Workshop, Osaka.
Lowe, R., and Ziemke, T. (2010). Towards a cognitive robotics methodology for reward-based decision-making: dynamical systems modelling of the Iowa Gambling Task. Connect. Sci. 22, 247–289.
Luciw, M., Graziano, V., Ring, M., and Schmidhuber, J. (2011). “Artificial curiosity with planning for autonomous perceptual and cognitive development,” in IEEE 10th International Conference on Development and Learning (ICDL), Frankfurt.
McFarland, D., and Spier, E. (1997). Basic cycles, utility and opportunism in self-sufficient robots. Rob. Auton. Syst. 20, 179–190.
McNaughton, N., and Gray, J. A. (2000). Anxiolytic action on the behavioural inhibition system implies multiple types of arousal contribute to anxiety. J. Affect. Disord. 61, 1161–1161.
Mehrabian, A. (1996). Pleasure-arousal-dominance: a general framework for describing and measuring individual differences in temperament. Curr. Psychol. 14, 261–292.
Mehrabian, A. (1997). Comparison of the PAD and PANAS as models for describing emotions and for differentiating anxiety from depression. J. Psychopathol. Behav. Assess. 19, 331–357.
Mellers, B., Schwartz, A., and Ritov, I. (1999). Emotion-based choice. J. Exp. Psychol. Gen. 128, 332–345.
Montebelli, A., Lowe, R., Ieropoulos, I., Greenman, J., Melhuish, C., and Ziemke, T. (2010). “Microbial fuel cell driven behavioral dynamics in robot simulations,” in Artificial Life XII, eds H. Fellermann, M. Dörr, M. Hanczyc, L. Laursen, S. Maurer, D. Merkle, P.-A. Monnard, K. Sty, and S. Rasmussen (Odense: The MIT Press), 749–756.
Mountcastle, V. B. (1978). “An organizing principle for cerebral function: the unit model and the distributed system,” in The Mindful Brain, G. M. Edelman and V. B. Mountcastle (Cambridge, MA: The MIT Press), 7–50.
Mountcastle, V. B. (1998). Perceptual Neuroscience: The Cerebral Cortex. Cambridge, MA: Harvard University Press.
Norris, C. J., Gollan, J., Berntson, G. G., and Cacioppo, J. T. (2010). The current status of research on the structure of evaluative space. Biol. Psychol. 84, 422–436.
Oatley, K., and Johnson-Laird, P. N. (1987). Towards a cognitive theory of emotions. Cogn. Emot. 1, 29–50.
Oatley, K., Keltner, D., and Jenkins, J. M. (2006). Understanding Emotions, 2nd Edn. Oxford: Blackwell Publishing.
Osgood, C. E., Suci, G. J., and Tannenbaum, P. H. (1957). The Measurement of Meaning. Urbana, IL: University of Illinois Press.
Oudeyer, P., and Kaplan, K. (2007). What is intrinsic motivation? a typology of computational approaches. Front. Neurorobot. 1:6. doi:10.3389/neuro.12.006.2007
Panksepp, J. (2000). “The neurodynamics of emotions: an evolutionary neurodevelopmental view,” in Emotion, Development, and Self-organization: Dynamic Systems Approaches to Emotional Development, eds M. D. Lewis and I. Granic (New York: Cambridge University Press), 236–264.
Panksepp, J. (2007). Emotional feelings originate below the neocortex: toward a neurobiology of the soul. Behav. Brain Sci. 30, 101–103.
Pessoa, L. (2008). On the relationship between emotion and cognition. Nat. Rev. Neurosci. 9, 148–158.
Pessoa, L. (2010). Emotion and cognition and the amygdala: from “what is it?” to “what’s to be done?” Neuropsychologia 48, 3416–3429.
Prinz, J. J. (2004). Gut Reactions: A Perceptual Theory of Emotion. New York: Oxford University Press.
Quirk, G. J. (2007). “Prefrontal-amygdala interactions in the regulation of fear,” in Handbook of Emotion Regulation, ed. J. J. Gross (New York: The Guilford Press), 27–46.
Rescorla, R. A., and Wagner, A. R. (1972). “A theory of pavlovianconditioning: variations in the effectiveness of reinforcement and non-reinforcement,” in Classical Conditioning II: Current Research and Theory, eds A. H. Black and W. F. Prokasy (New York: Appleton- Century-Crofts).
Rodriguez, A., Whitson, J., and Granger, R. (2004). Derivation and analysis of basic computational operations of thalamocortical circuits. J. Cogn. Neurosci. 16, 856–877.
Roesch, M. R., Calu, D. J., and Schoenbaum, G. (2007). Dopamine neurons encode the better option in rats deciding between differently delayed or sized rewards. Nat. Neurosci. 10, 1615–1624.
Rolls, E. T. (1986). “A theory of emotion and its application to understanding the neural basis of emotion,” in Emotions. Neural and Chemical Control, ed. Y. Oomura (Tokyo: Japan Scientific Societies Press), 325–344.
Russell, J. A. (2003). Core affect and the psychological construction of emotion. Psychol. Rev. 110, 145–172.
Russell, J. A., and Mehrabian, A. (1977). Evidence for a three-factor theory of emotions. J. Res. Pers. 11, 273–294.
Samson, R. D., Frank, M. J., and Fellous, J.-M. (2010). Computational models of reinforcement learning: the role of dopamine as a reward signal. Cogn. Neurodyn. 4, 91–105.
Sandamirskaya, Y., Richter, M., and Schöner, G. (2011). “A neural-dynamic architecture for behavioural organization of an embodied agent,” in IEEE 10th International Conference on Development and Learning (ICDL), Frankfurt.
Schachter, S., and Singer, J. E. (1962). Cognitive, social and physiological determinants of emotional state. Psychol. Rev. 69, 379–399.
Schauer, M., and Elbert, T. (2010). Dissociation following traumatic stress: etiology and treatment. J. Psychol. 218, 109–127.
Scherer, K. R. (1984). “On the nature and function of emotion: a component process approach,” in Approaches to Emotion, eds K. R. Scherer and P. Ekman (Hillsdale, NJ: Erlbaum), 293–317.
Scherer, K. R. (2000). “Emotions as episodes of subsystem synchronization driven by non-linear appraisal processes,” in Emotion, Development, and Self-organization, eds M. D. Lewis, and I. Granic (Cambridge: Cambridge University Press), 70–99.
Scherer, K. R. (2004). “Feelings integrate the central representation of appraisal-driven response organization in emotion,” in Feelings and Emotions, eds A. S. R. Manstead, N. H. Frijda, and A. Fischer (Cambridge: Cambridge University Press), 136–157.
Scherer, K. R. (2009). Emotions are emergent processes: they require a dynamic computational architecture. Philos. Trans. R. Soc. Lond. B Biol. Sci. 364, 3459–3474.
Schmidhuber, J. (1991). “Curious model-building control systems,” in Proceedings of the International Joint Conference on Neural Networks, Singapore, Vol. 2, 1458–1463.
Schmidhuber, J. (2010). Formal theory of creativity, fun, and intrinsic motivation (1990–2010). IEEE Trans. Auton. Ment. Dev. 2, 230–247.
Schöner, G. (2008). “Dynamical systems approaches to cognition,” in The Cambridge Handbook of Computational Psychology, ed. R. Sun (New York: Cambridge University Press), 101–125.
Schulkin, J. (2003). Rethinking Homeostasis: Allostatic Regulation in Physiology and Pathophysiology. Cambridge MA: MIT Press.
Schultz, W. (2007). Multiple dopamine functions at different time courses. Annu. Rev. Neurosci. 30, 259–288.
Seymour, B., O’Doherty, J. P., Dayan, P., Koltzenberg, M., Jones, A. K., Dolan, R. J., Friston, K. J., and Frackowlak, R. S. (2004). Temporal difference models describe higher-order learning in humans. Nature 429, 664–667.
Sherman, S., and Guillery, R. (2006). Exploring the Thalamus and its Role in Cortical Function. Cambridge, MA: The MIT Press.
Sinha, R., Lovallo, W. R., and Parsons, O. A. (1992). Cardiovascular differentiation of emotions. Psychosom. Med. 54, 422–435.
Stemmler, G., Aue, T., and Wacker, J. (2007). Anger and fear: separable effects of emotion and motivational direction on somatovisceral responses. Int. J. Psychophysiol. 66, 141–153.
Stephens, C., Christie, I. C., and Friedman, B. H. (2010). Autonomic specificity of basic emotions: evidence from pattern classification analysis and cluster analysis. Biol. Psychol. 84, 463–473.
Sterling, P. (2004). “Principles of allostasis: optimal design, predictive regulation, pathophysiology and rational therapeutics,” in Allostasis, Homeostasis, and the Costs of Adaptation, ed. J. Schulin (Cambridge: Cambridge University Press).
Suri, R. E. (2002). TD models of reward predictive responses in dopamine neurons. Neural Netw. 15, 523–533.
Suri, R. E., and Schultz, W. (1998). Dopamine-like reinforcement signal improves learning of sequential movements by neural network. Exp. Brain Res. 121, 350–354.
Sutton, R. S., and Barto, A. G. (1990). “Time-derivative models of Pavlovian reinforcement,” in Learning and Computational Neuroscience: Foundation of Adaptive Networks, eds M. Gabriel and J. Moore (Cambridge, MA: MIT Press), 497–537.
Sutton, R. S., and Barto, A. G. (1998). Reinforcement Learning: An Introduction. Cambridge, MA: The MIT Press.
Thelen, E., Schöner, G., Scheier, C., and Smith, L. (2001). The dynamics of embodiment: a dynamic field theory of infant perseverative reaching. Behav. Brain Sci. 24, 1–86.
Tomkins, S. S. (1962). Affect, Imagery, Consciousness. The Positive Affects, 1. New York, NY: Springer.
Tooby, J., and Cosmides, L. (2008). The evolutionary psychology of the emotions and their relationship to internal regulatory variables,” in Handbook of Emotions, eds M. Lewis, J. M. Haviland-Jones, and L. F. Barrett (New York: Guilford), 114–137.
Van Hoof, J. A. R. A. M. (1972). “A structural analysis of the social behaviour of a semi-captive group of chimpanzees,” in Social Communication and Movement, eds M. van Cranach and J. Vine (New York: Academic Press), 75–162.
Waldstein, S. R., Bachen, E. A., and Manuck, S. B. (1997). Active coping and cardiovascular reactivity: a multiplicity of influences. Psychosom. Med. 59, 620–625.
Watson, D., Wiese, D., Vaidya, J., and Tellegen, A. (1999). The two general activation systems of affect: structural findings, evolutionary considerations, and psychobiological evidence. J. Pers. Soc. Psychol. 76, 820–838.
Wörgötter, F., and Porr, B. (2005). Temporal sequence learning, prediction, and control: a review of different models and their relation to biological mechanisms. Neural. Comput. 17, 245–319.
Zajonc, R. B. (1980). Feeling and thinking: preferences need no inferences. Am. Psychol. 35, 151–175.
Ziemke, T. (2008). On the role of emotion in biological and robotic autonomy. Biosystems 91, 401–408.
Keywords: feeling, action tendency, prediction, feedback, neural-dynamic representations, reinforcement, homeostasis, goal-directed behavior
Citation: Lowe R and Ziemke T (2011) The feeling of action tendencies: on the emotional regulation of goal-directed behavior. Front. Psychology 2:346. doi: 10.3389/fpsyg.2011.00346
Received: 04 August 2011;
Accepted: 04 November 2011;
Published online: 27 December 2011.
Edited by:
Tom Verguts, Ghent University, BelgiumReviewed by:
Angelos-Miltiadis Krypotos, Universiteit van Amsterdam, NetherlandsLi Su, University of Cambridge, UK
Copyright: © 2011 Lowe and Ziemke. This is an open-access article subject to a non-exclusive license between the authors and Frontiers Media SA, which permits use, distribution and reproduction in other forums, provided the original authors and source are credited and other Frontiers conditions are complied with.
*Correspondence: Robert Lowe, Cognition and Interaction Lab, School of Humanities and Informatics, University of Skövde, Skövde, Box 408, 541 28 Skövde, Sweden. e-mail:cm9iZXJ0Lmxvd2VAaGlzLnNl