- 1 Music Dynamics Laboratory, Center for Complex Systems and Brain Sciences, Florida Atlantic University, Boca Raton, FL, USA
- 2 EEG/ERP Laboratory, Vanderbilt Kennedy Center, Vanderbilt University, Nashville, TN, USA
- 3 Brain and Language Laboratory, Psychology Department and PhD in Literacy Studies, Middle Tennessee State University, Murfreesboro, TN, USA
Song composers incorporate linguistic prosody into their music when setting words to melody, a process called “textsetting.” Composers tend to align the expected stress of the lyrics with strong metrical positions in the music. The present study was designed to explore the idea that temporal alignment helps listeners to better understand song lyrics by directing listeners’ attention to instances where strong syllables occur on strong beats. Three types of textsettings were created by aligning metronome clicks with all, some or none of the strong syllables in sung sentences. Electroencephalographic recordings were taken while participants listened to the sung sentences (primes) and performed a lexical decision task on subsequent words and pseudowords (targets, presented visually). Comparison of misaligned and well-aligned sentences showed that temporal alignment between strong/weak syllables and strong/weak musical beats were associated with modulations of induced beta and evoked gamma power, which have been shown to fluctuate with rhythmic expectancies. Furthermore, targets that followed well-aligned primes elicited greater induced alpha and beta activity, and better lexical decision task performance, compared with targets that followed misaligned and varied sentences. Overall, these findings suggest that alignment of linguistic stress and musical meter in song enhances musical beat tracking and comprehension of lyrics by synchronizing neural activity with strong syllables. This approach may begin to explain the mechanisms underlying the relationship between linguistic and musical rhythm in songs, and how rhythmic attending facilitates learning and recall of song lyrics. Moreover, the observations reported here coincide with a growing number of studies reporting interactions between the linguistic and musical dimensions of song, which likely stem from shared neural resources for processing music and speech.
Introduction
Song is an ecological model for studying the complex relationship between music and speech, and has been examined in recent studies with cognitive neuroscience methods that have found interactions between various aspects of the linguistic and musical dimensions of songs (Lidji et al., 2009; Gordon et al., 2010; Sammler et al., 2010; Schön et al., 2010). In both music and speech, temporal patterning of events gives rise to hierarchically organized rhythms that are perceived as metrical (Palmer and Hutchins, 2006). Songs are created through the process of textsetting, in which the prosodic features of speech are combined with musical melody, uniting their separate metrical structures and forming one rhythmic pattern (Halle and Lerdahl, 1993). Musicologists have long noted that in the process of textsetting, song composers often align linguistically strong syllables with musically strong beats (Kimball, 1996), an observation that has been confirmed in analyses of corpuses of English songs (Palmer and Kelly, 1992). In other words, stressed syllables, or syllables that one would expect to be stressed in speech (“strong” syllables), are more likely than unstressed (“weak”) syllables to occur on hierarchically prominent musical beats. Musicians intuitively emphasize this correspondence, by lengthening well-aligned syllables more than misaligned ones. Furthermore, non-musicians have predictable, consistent intuitions about aligning lyrics to musical rhythms when asked to sing novel lyrics (i.e., new verses) to a familiar melody (Halle and Lerdahl, 1993) or chant lyrics without knowledge of the original melody (Hayes and Kaun, 1996).
It has been proposed that certain goals of music performance, including communication and memorization, may be fulfilled by the temporal alignment of strong syllables with musically strong beats (Palmer and Kelly, 1992). In fact, Johnson et al. (in revision) studied comprehension of sung lyrics in a sentence context by asking listeners to transcribe well-aligned words (strong syllables occurring on strong beats), and misaligned words (weak syllables occurring on strong beats). They found that intelligibility of sung lyrics was better when strong syllables aligned with strong metric positions in the music. Behavioral results from several studies suggest that songs that are well-aligned are also easier to memorize. For instance, Gingold and Abravanel (1987) reported that children had more difficulty learning songs with misaligned textsettings than songs with well-aligned textsettings. Experiments with adults also point to the efficacy of emphasizing both the linguistic (Wallace and Rubin, 1988; Wallace, 1994) and musical (Purnell-Webb and Speelman, 2008) rhythm during song learning. To summarize, composers, performers, and naïve listeners appear to prefer well-aligned textsettings, which may be associated with more efficient encoding mechanisms.
One explanation for these observations is that attending and comprehension are facilitated when strong syllables occur on strong beats. It thus follows that intelligibility of sung lyrics would be degraded when word stress does not align with strong beats (Johnson et al., in revision). Misaligned words could be perceived as prosodically incongruous sung language, which disrupts attending and hinders comprehension, similar to the way that rhythmic incongruities in speech disrupt intelligibility (Tajima et al., 1997; Field, 2005). The present study was designed to uncover the brain mechanisms underlying the preference for metrical alignment, by testing the hypothesis that well-aligned textsettings help listeners to better understand song lyrics, by directing temporal attention to instances where strong syllables occur on strong beats.
Our approach is based on previous work on perception of speech prosody and musical meter. Several studies have shown that prosody is important for speech segmentation in adults (e.g., Cutler and Norris, 1988; Smith et al., 1989; Mattys et al., 2005) and children (e.g., Jusczyk et al., 1993). It has been proposed that word stress facilitates segmentation of the speech signal by directing temporal attention toward salient events in speech (Shields et al., 1974; Pitt and Samuel, 1990; Quené and Port, 2005): listeners attend more to strong syllables than weak syllables. Rhythmic expectations generated by metrical and syntactic cues also play an important role in predicting word stress (Kelly and Bock, 1988; Pitt and Samuel, 1990). Likewise, when the timing of musical notes is organized to aid the listener in predicting the timing of upcoming events (Schmidt-Kassow et al., 2009), attention is preferentially allocated to strong beats in music (Large and Jones, 1999).
Therefore, it is likely that listeners’ temporal attention is focused by well-aligned, predictable textsettings in song, in which strong syllables and strong beats coincide. Cortical rhythms entrain to the temporal structure of acoustic signals (Snyder and Large, 2005; Zanto et al., 2005; Fujioka et al., 2009; Geiser et al., 2009; Nozaradan et al., 2011), and entrainment of neuronal oscillations can function as a mechanism of attentional selection (Lakatos et al., 2008; Stefanics et al., 2010). Entrainment of intrinsic neural processes explains temporal expectancy and perceptual facilitation of events that occur at expected points in time (Large and Jones, 1999; Jones, 2010). The experience of meter is posited to arise from interaction of neural resonances at different frequencies. In light of suggestions of similar mechanisms for perceiving meter in speech and music (Port, 2003; Patel et al., 2006; Patel, 2008; Marie et al., 2011), we sought to probe a possible influence of linguistic rhythm on neural correlates of musical rhythm by investigating prosodically driven changes in these neural responses to sung stimuli.
An experiment was thus designed to explore the idea that alignment of stressed syllables with strong beats in music helps to temporally focus listeners’ attention, which in turn facilitates syllable segmentation and comprehension of the lyrics of a song. Using a cross-modal priming paradigm, listeners were presented with sung sentences (primes) in which all, none, or some of the strong syllables occurred on strong musical beats. Strong beats were determined by a series of isochronous metronome clicks occurring on alternating syllables before and during the sentences. Three kinds of sentence alignment were used: well-aligned (regular stress pattern; all strong syllables occur on strong beats), misaligned (regular stress pattern; all weak syllables occur on strong beats), varied (irregular stress pattern: some strong syllables occur on strong beats, some occur on weak beats). To test the influence of different kinds of sung sentence alignment on a subsequently presented stimulus, each sentence was followed by a target word or pseudoword, presented visually, on which listeners were asked to perform a lexical decision task (i.e., discerning real words from nonsense words).
This approach exploits the ability of a metronome to induce different metrical interpretations (i.e. shifting of strong beats; see Iversen et al., 2009; Geiser et al., 2010; Vlek et al., 2011) on identical melodies, sung with words. An isochronous metronome click train was used to set up metrical expectancies before and during sung sentences, such that the perceived strong musical beats (marked by the clicks) were either aligned or not aligned with the expected linguistic stress of each sung syllable. Alignment was manipulated by shifting the same sung sentence by one quarter note (or shifting the metronome clicks by one quarter note; see Figure 1).
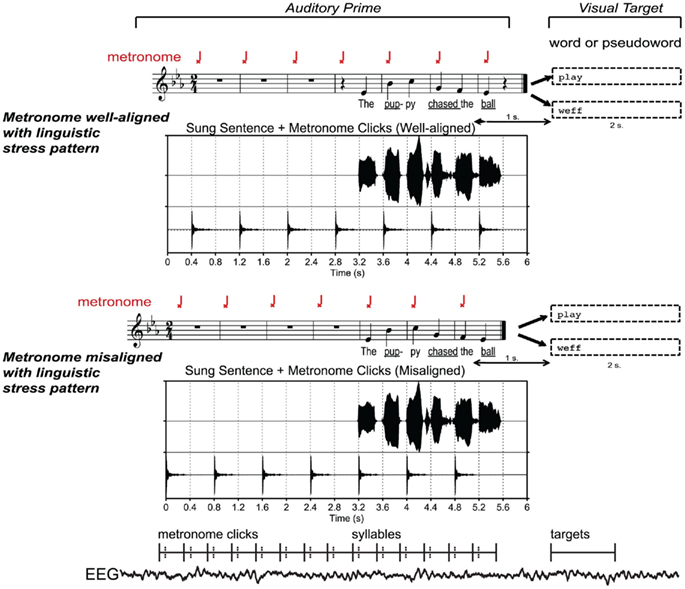
Figure 1. Experimental paradigm and stimuli. Auditory sentence primes were created by aligning a sequence of isochronous metronome clicks with strong syllables in sung sentences (underlined), and shifting by one beat relative to metronome clicks on downbeats. The waveforms show that the same recording of sung sentence can be aligned with metronome clicks on strong syllables to form well-aligned sentences, or with weak syllables to form misaligned sentences. Targets consisted of words or pseudowords presented visually following each auditory prime. The lower section of the figure gives a schematic view of time windows analyzed for the EEG responses timelocked to strong and weak beats (metronome clicks and syllables) and targets. Bold lines show the boundaries of each latency window, and dashed lines show zero points. See Section “Data Analysis – EEG” for further detail.
Our first hypothesis was that well-aligned and misaligned syllables would elicit different neural responses. It was expected that strong metronome beats would elicit synchronized rhythmic fluctuations in beta and gamma activity, in light of previous studies suggesting that high frequency EEG/MEG fluctuates with endogenous temporal and metrical expectancies, and persists even in the absence of a tone (Snyder and Large, 2005; Fujioka et al., 2009). If similar mechanisms are at work for perceiving rhythm in speech, then high frequency neural activity may coordinate with strong beats when they align with strong syllables. On the other hand, when a weak syllable is aligned with a strong beat, beat tracking could be disrupted, and therefore typical beta and gamma responses would be attenuated or suppressed. If the anti-phase relationship between strong syllables and strong beats in the misaligned sentences is perceived as syncopation, misaligned syllables may elicit decreased beta power, as reported for sensori-motor syncopation (Jantzen et al., 2001; Mayville et al., 2001).
The second hypothesis was that both alignment and regularity facilitate lexical access. If well-aligned and misaligned syllables are attended to differently online, during the sung sentence primes, then the interplay between linguistic and musical rhythm may also have a cumulative effect on attention to and perception of target words and pseudowords. This may influence the lexico-semantic search process, as shown in previous work on perception of spoken language (Schirmer et al., 2002; Dilley and McAuley, 2008). Thus, we predicted that misaligned and varied sentence primes would hinder target processing, and would be associated with poorer task performance [slower reaction times (RTs), more errors], compared to well-aligned sentence primes. It was therefore predicted that misaligned and varied primes would be more difficult to process than well-aligned primes and thus recruit more cognitive resources for their respective targets. These priming effects could stem from differences in domain–general memory mechanisms involved in lexical search (such as changes reported in alpha band activity by Doppelmayr et al., 2005; Grabner et al., 2007; Klimesch et al., 2007) and selective attention to linguistic stimuli, reflected in alpha and beta band suppression (Shahin et al., 2009; Van Elk et al., 2010).
Materials and Methods
Participants
Nineteen volunteers participated in this experiment that lasted about 2 h including preparation time. The study was approved by the IRB committees at Florida Atlantic University and Middle Tennessee State University, and all participants gave written informed consent. By self-report, all participants had normal hearing, no known neurological problems, and were right-handed, native speakers of English. Data from three participants was discarded due to excessive ocular artifacts and line noise, thus the final group of subjects consisted of 16 subjects (nine males, mean age = 21.4 years, age range 18–34, mean number years of formal music lessons = 1.9).
Stimuli
Sung sentences
First, a corpus of 144 English sentences was created based on the type of sentences used in a previous study of meter in speech (Kelly and Bock, 1988). Each sentence contained six syllables that formed three types of metrical patterns: trochaic-regular (strong–weak–strong–weak–strong–weak); iambic-regular (weak–strong–weak–strong–weak–strong); and mixed-irregular (e.g., weak–strong–strong–weak–weak–strong). See Table 1 for examples of sentences used. The irregular sentences were controlled so that the total number of strong and weak syllables in each syllable position (first through sixth) was balanced over sentences.
Each sentence from each of the three linguistic conditions was paired with a melody from a corpus of 24 tonal, isochronous pitch sequences composed for this experiment. The first and last notes of each melody were always the tonic, and the second through fifth notes followed an overall contour of rising then falling. Because pitch accents can contribute to the perception of linguistic stress and musical meter (Hannon et al., 2004; Jones, 2009), each melody was used once in each of the six experimental conditions. As shown in Figure 2, the peak in melodic contour in a given melody was held constant across experimental conditions and types of syllable alignment (strong or weak syllables on strong or weak beats), so any global effects of metrical alignment could not be attributed to differences in melodic accents across syllables.
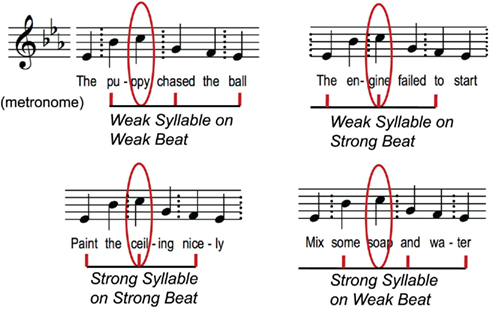
Figure 2. Melodic contour of sung sentence stimuli. In these four sentences sung to the same melody, the same pitch (circled in red) can belong to different alignment conditions: well-aligned (weak syllable on weak beat; strong syllable on strong beat) or misaligned (weak syllable on strong beat; strong syllable on weak beat).
Using the MIDI Toolbox (Eerola and Toiviainen, 2004), the melodies were exported as MIDI (Musical Instrument Digital Interface) files in preparation for the digital recording session with the software GarageBand (Apple, Cupertino, CA, USA). The recording session took place in the IAC chamber in the soundproof booth in the Music Dynamics Lab at FAU. Each sentence was sung by a trained singer (the first author, a soprano) using a metronome set at 150 beats-per-minute, targeting a duration of 400 ms per syllable. The sentences were sung with as little variation as possible in loudness or duration between syllables, regardless of their expected linguistic stress. Vowels in unstressed syllables were not reduced, as they would be in speech; instead, they were pronounced fully, as called for by traditional vocal pedagogy. Each of the 144 sung sentences were edited in Praat (Boersma and Weenink, 2007). Syllable and vowel boundaries were marked by hand for acoustic analyses, by visually inspecting the waveform and spectrogram, and by listening to the segments. The wave files containing the sung sentences were normalized in intensity by equalizing the maximum root-mean-squared (RMS) amplitude across wave files.
Next, acoustic analyses were performed to determine whether strong and weak syllables differed in duration and amplitude. There was no overall difference in RMS amplitude between strong (mean = 0.079, SD = 0.029) and weak (mean = 0.077, SD = 0.028) syllables [t(359) = 0.759, p = 0.449], measured with two-tailed paired t-tests. Next, duration of the first five syllables in each sentence was computed by calculating the inter-vocalic interval (IVI; the interval between the onsets of vowels in adjacent syllables). The analyses showed that strong syllables were slightly shorter1 (mean = 396 ms, SD = 30) than weak syllables (mean = 401 ms, SD = 29) [t(359) = −2.458, p = 0.014].
An isochronous metronome click train (woodblock sound; seven clicks) with an IOI of 800 ms was generated in Praat. The wave file containing the metronome click sounds was normalized in intensity to a level that sounded appropriate when played simultaneously with the normalized sung sentences. Two versions of each sung sentence were created by aligning the metronome click train on alternating sung syllables, from either the vowel onset of the first syllable of each sentence, or 400 ms post-vowel onset (corresponding to the onset of the second syllable). This process created two versions of each sentence, as illustrated in Figure 1, so that precisely the same syllable in each sentence, defined by a single set of boundaries, would be heard with and without a click, across subject lists. Each stimulus was then checked aurally to make sure that clicks were appropriately synchronized with the intended syllables. The alignment of metronome clicks and sung sentences yielded 96 well-aligned sentences, 96 misaligned sentences, and 96 varied sentences. Audio examples of stimuli can also be accessed on the online Supplementary Materials.
Targets
For each of the 144 sentences, a monosyllabic target word that was different from, but semantically related to, the content of the sentence was designated. Words were four or five letters in length, were either nouns or verbs, and part of speech was balanced over conditions. A corpus of 72 monosyllabic pseudowords was selected from the ARC database (Rastle et al., 2002). Pseudowords were orthographically plausible and were also matched in number of letters to the real words. Two experimental lists were created, such that half of the prime sentences in each condition, and in each list, were assigned to pseudoword targets, and half were assigned to (semantically related) real word targets. The same list of pseudowords appeared in both experimental lists but with different prime sentences, and in addition, word frequency was equalized across the two lists (frequency of occurrence in verbal language was derived from the London-Lund Corpus of English Conversation by Brown, 1984).
A Latin Square design ensured that real words and pseudowords would appear with sung sentences with both metronome click patterns across subjects, and no sentence would be heard in more than one condition within the list of stimuli heard by one participant. This counterbalancing created 144 sung sentences × 2 metronome click patterns × 2 lists of targets, for a total of 576 different pairs of primes and target, to yield four different experimental lists.
To summarize, an orthogonal manipulation of the factors “Prime Alignment” (well-aligned, misaligned, and varied) and “Target Lexicality” (Word, Pseudoword) yielded six experimental conditions listed in Table 1, across which the 144 sentences were divided evenly to yield 24 sentences per condition.
Procedure
To familiarize participants with the task, each experimental session began with a block of practice trials consisting of sample stimuli representing each of the experimental conditions; the examples were not heard during the actual experiment. Participants were instructed to fixate on the cross that appeared at the beginning of each trial and to listen to the sung sentence, paying attention to the words and not to worry about the melody or rhythm, and then to decide whether the visual target shown on the screen after the sung sentence was a real word or a nonsense word. They were instructed to respond as quickly and accurately as possible by pressing one of two buttons on the response pad with the index and middle finger of their right hand. Each trial consisted of: a fixation period of 1000 ms, followed by wave file containing the sung sentence prime (6000 ms), and the visual target (2000 ms), which started 1000 ms after the onset of the final beat (see Figure 1 for trial timing).
Participants then listened through earbuds, adjusted slightly to comfortable volume level for each individual, to the 144 trials from the six experimental conditions, presented in a pseudorandom order, and broken up into three blocks of 48 trials with breaks between each block. They were asked to avoid blinking until a series of X’s appeared on the computer screen, at the end of each trial. Response key–finger association and stimulus lists were counterbalanced across participants. The software E-Prime (Psychology Software Tools Inc., Pittsburgh, PA, USA) was used for stimulus presentation and to record behavioral responses [RTs and percent correct (PC)].
EEG Data Acquisition
Electroencephalogram was recorded continuously from 64 Ag/AgCl electrodes embedded in sponges in the Hydrocel Geodesic Sensor Net (EGI, Eugene, OR, USA) placed on the scalp with Cz at the vertex, connected to a NetAmps 300 high-impedance amplifier, using a MacBook Pro computer. Data was referenced online to Cz. All electrical equipment was properly grounded. The sampling frequency was 500 Hz with an anti-aliasing lowpass Butterworth filter of 4 kHz, and impedances were kept below 50 kΩ.
Data Analysis – Behavioral (Targets)
For each of the six experimental conditions listed in Section “Targets,” PC was computed on all responses. Mean RTs were computed on correct behavioral responses only. Analyses of behavioral data were conducted with e-prime and MATLAB (The MathWorks Inc., Natick, MA, USA) and the statistical software CLEAVE2 was used to conduct a 3 × 2 ANOVA with factors: Alignment (well-aligned vs. misaligned vs. varied), Lexicality (word vs. pseudoword). The Bonferroni threshold was applied to pairwise post hoc tests on significant interactions to correct for multiple comparisons.
Data Analysis – EEG
EEG preprocessing
Electroencephalographic preprocessing was carried out with NetStation Viewer and Waveform tools. The EEG data was filtered offline with a pass band from 0.5 to 100 Hz. The data were then re-referenced offline to the algebraic average of the left and right mastoid sensors. To analyze responses to the lead-in metronome clicks in the primes, data were epoched at ±750 ms from click onset (strong beats) and from the midpoint between clicks (weak beats) and then divided into two conditions: strong beats and weak beats. To analyze the EEG recorded during the sung sentences, data were epoched from −450 to +1050 ms from the onset of each syllable, which was defined as described in Section “Sung Sentences” (note that long epoch windows are required for wavelet calculation, but statistical analyses are not performed on overlapping time windows of the time–frequency representations (TFRs), as shown in Figure 1 and described in Cluster Randomization Analysis). Epochs corresponding to prime syllables were divided into the following three conditions: strong syllable on strong beat; weak syllable on strong beat; strong syllable on weak beat. The irregular sentences (varied condition) were excluded from the syllable analysis because they became irregular beginning at different syllable positions. To analyze responses to the targets, data were segmented into epochs of −1000 to +2000 ms from the onset of the target word/pseudoword. Epochs corresponding to targets were then divided into three conditions: targets following well-aligned primes, misaligned primes, and varied primes. Artifact rejection was performed on epoched data, such that trials containing movement or ocular artifacts, or amplifier saturation were discarded, and likewise, several sensor channels containing excessive noise (in the occipital area) were excluded from further analysis in all subjects. Following artifact rejection, the mean (SD in parentheses) number of remaining trials per participant, in each dataset was as follows. For metronome click dataset: strong beats – 353(68), weak beats –362(67); for the syllable dataset: Strong Syllable on Strong Beat –130(20), Weak Syllable on Strong Beat – 130(17), Strong Syllable on Weak Beat 129(16); for the target dataset: Well-aligned – 38(8), Misaligned –38(8), Irregular 38(8).
Time–frequency representations – evoked and induced
Time–frequency analysis was conducted using the FieldTrip open source toolbox3 (Oostenveld et al., 2011) to calculate both evoked and induced representations of EEG4. To obtain the evoked (phase-locked) response, TFRs were computed on the average waveform for each condition and each subject, at each time point and frequency, by convolving the average waveform with a Morlet wavelet that had a width of six cycles (see Tallon-Baudry et al., 1996, and Herrmann et al., 2005 for more details on wavelet analysis of EEG data). The resulting frequency resolution was sf = f/6, and the temporal resolution was st = 1/sf, where f is the center frequency for each wavelet, sf is SD in the frequency domain, and st is the SD in the temporal domain. The convolution of wavelets was done between 8 and 50 Hz, with a frequency step of 1 Hz and a time step of 2 ms, between −750 and +750 ms from the zero point of metronome click data; between −250 and +500 ms for the sung syllables; and from −200 to +1000 ms for the targets. Only low gamma band (30–50 Hz) was analyzed, as measuring high gamma band activity would likely have required more trials per condition (to establish an acceptable signal-to-noise ratio, due to heightened effects of small-latency jitter in high frequencies) than were present in this study. To obtain the induced response (phase-invariant), TFRs were computed by convolving single trial data with a Morlet wavelet that had the same parameters as described for the evoked analysis, and averaging power over trials for each subject and condition.
In order to compensate for inter-individual variability in absolute power, a normalization procedure was used for both evoked and induced analyses. For the targets, the spectra for all six conditions were averaged together for each subject (and averaged over time across the entire time period for which power values were computed as described above), to obtain a value for each frequency at each channel, which then served as a baseline. For the metronome clicks and the syllables from the sung sentences, normalization was conducted by using the average power (across epochs) of the response to introductory metronome clicks as a baseline. The power spectra for each condition and each subject were then normalized with respect to these baseline values, resulting in normalized power, expressed as the percent change from the baseline.
Cluster-randomization analysis
Statistical significance between the normalized power spectra in different conditions was tested using cluster-randomization procedure (Maris and Oostenveld, 2007), to identify consistent trends of activity in time–frequency clusters of electrodes. This is a data-driven approach that is particularly useful for spatial localization without having to specify regions of interest a priori. This method utilizes planned comparisons between pairs of conditions using cluster-based permutation tests, a solution to the multiple comparisons problem for EEG/MEG data that is prevalent in recent studies of oscillatory activity in language cognition (see Bastiaansen et al., 2010 for a discussion of the advantages of this method). Clustering was performed by identifying power values (for each channel, at each frequency and time point) that showed a similar direction of effect, and then testing the significance of a cluster in a given condition compared to another condition in relation to clusters computed from a random permutation of values drawn from both conditions.
The power spectra were first divided into frequency bands: alpha (8–12 Hz), beta (13–29 Hz), and low gamma (30–50 Hz), and time windows of interest were defined (−100 to 300 ms for the metronome clicks and prime syllables, and 0–800 ms for the targets). Frequency bands for the analysis were chosen based on hypotheses described in the Introduction (primes: beta and gamma; targets: alpha and beta). In each comparison, within a given frequency band5, the resulting power values at each time point and channel underwent two-tailed dependent t-tests, for each subject. Then, a cluster-level statistic was calculated by taking the sum of the t-statistics within every cluster. All data points that did not exceed the preset significance level (p = 0.025) were zeroed, and clusters were created by grouping together adjacent non-zero points. The next step was to compute significance probability of the clusters using the (non-parametric) Monte Carlo method6. The Monte Carlo significance probability (p-value) was determined by calculating the proportion of clusters from random partitions of the data that resulted in a larger test statistic than the clusters on the observed test statistic. p-Values < 0.05 were considered significant. Only pairwise comparisons are possible with the clustering method; these planned comparisons are presented in Table 2.
Results
Behavioral Results: Influence of Prime Alignment on Target Lexical Decision
In the Reaction Time data (see Table 3), there was a main effect of Lexicality [F(1,15) = 24.89, p < 0.001]. As predicted, participants were always faster at detecting words than pseudowords. There was also a significant interaction of Alignment × Lexicality [F(2,30) = 4.12, p = 0.0262]: RT differences were greater on targets following varied and misaligned primes vs. well-aligned primes (each pairwise post hoc p < 0.001). A follow-up ANOVA was then conducted on the Reaction Time difference (Word minus Pseudoword; see Figure 3A). There was a main effect of Alignment [F(2,30) = 4.12, p = 0.0262]. Post hoc tests revealed that misaligned led to a larger difference in the RTs (p = 0.009) compared to well-aligned, and varied vs. well-aligned also approached significance (post hoc p = 0.0535). Thus, alignment affects the speed of lexical decision.
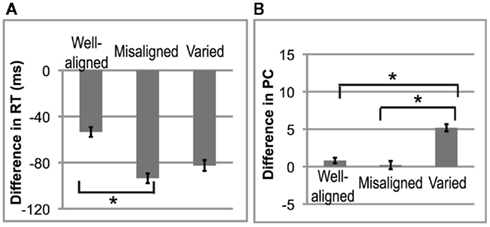
Figure 3. Behavioral data. (A) Reaction Times. The difference in lexical decision was computed by subtracting the mean RT for pseudoword from the mean RT for word for each type of prime alignment. Error bars indicate SE. (B) Percent correct. The difference in lexical decision on accuracy scores.
In the PC data, performance was nearly at ceiling (see Table 3), and there were no main effects. However, the Alignment × Lexicality interaction was significant [F(2,30) = 4.11; p = 0.0264]; participants were less accurate for pseudoword than word targets, but only for varied primes (pairwise post hoc p < 0.001). A follow-up one-way ANOVA was then conducted on the difference in PC scores (see Figure 3B), showing a main effect of Alignment [F(2,30) = 4.11; p = 0.0264]. Post hoc tests on PC revealed that the difference was significantly larger for varied than for misaligned (p = 0.013) and for varied vs. well-aligned (p = 0.028). Thus, predictability of stress patterns in prime sentences affects the detection of pseudowords.
Results – EEG
Metronome clicks
Time–frequency analyses of the EEG recorded during the lead-in metronome clicks (prior to each sung sentence), averaged together over all the trials and over the clicks within each trial, showed differences in evoked and induced activity between strong beats (clicks) and weak beats (half-way point between clicks). Two widespread clusters of electrodes (both cluster ps < 0.001) showed increased evoked power in the beta band (13–29 Hz) from −100 to 300 ms and in the gamma band (30–50 Hz) from −30 to 122 ms (see Figure 4A). Similarly, a cluster of electrodes (p = 0.044) over frontal–central regions showed increased induced beta (13–24 Hz) power for strong beats (clicks) vs. decreased power for weak beats, from −100 to 124 ms (see Figure 4B).
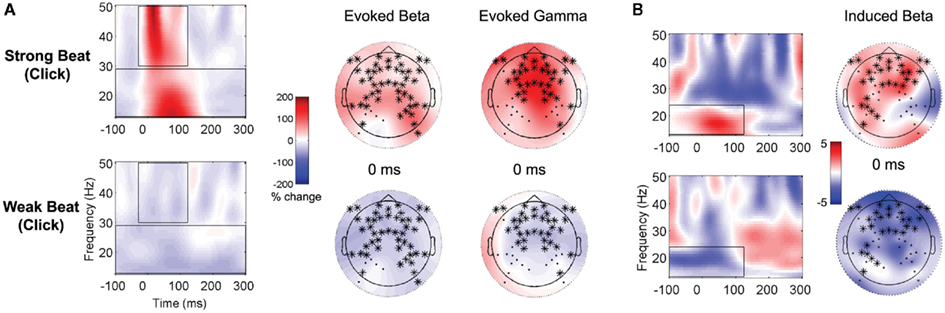
Figure 4. Time-frequency representations to lead-in metronome clicks. In each panel, results of clustering analysis shown on grand averages of each of the two significantly different conditions in the cluster. Electrodes belonging to the significant cluster at the time point above each topographic plot (right) are represented with *, and overlay average activity for the data used in the contrast. The scale (middle) is the percent change from baseline (i.e., normalized power), with red representing increased power and blue representing decreased power. Time–frequency representations (left) were calculated by averaging together all of the electrodes belonging to the significant cluster; the same power scale applies to both topographic plots and TFRs. (A) Evoked EEG to the lead-in metronome clicks – strong beats (top) and weak beats (bottom) in the beta band (13–29 Hz) and gamma band (30–50 Hz). (B) Induced EEG to the lead-in metronome clicks in the beta band (13–24 Hz) – strong beats (top) and weak beats (bottom).
Syllables in sung sentence primes
Well-aligned syllables were compared to misaligned syllables by comparing strong and weak syllables that occurred on strong beats. Results from the clustering analysis of the prime syllable conditions are described in the following section and clusters significant at the p < 0.05 level are reported. Times are given relative to a beat onset time at 0 ms.
A cluster of electrodes (a tendency was found, p = 0.051) showed increased induced beta power for strong syllables vs. weak syllables (on strong beats), from −100 to 54 ms, over mostly frontal and central regions (see Figure 5A). This result was expected based on Snyder and Large (2005). Thus, similar to the strong beats during the lead-in metronome, strong syllables appear to be associated with increased early-latency high frequency power. This response was not observed when weak syllables occurred on strong beats; instead, the paired comparison showed they were associated with decreased early-latency power.
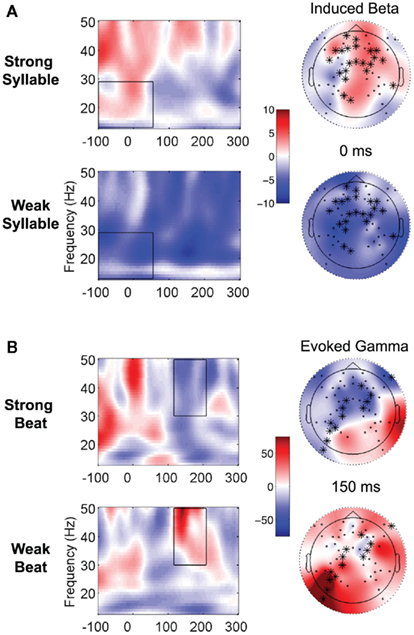
Figure 5. Time-frequency representations to well-aligned and misaligned syllables. The temporal and frequency boundaries of the cluster are demarcated in the black box. See legend of Figure 4 for description of plotting. (A) Contrast showing differences in induced beta power between brain responses to strong vs. weak syllables, on strong beats. (B) Contrast showing differences in evoked gamma power to strong vs. weak beats, on strong syllables.
Next, we considered strong syllables, asking whether responses differed depending on whether they occurred to strong vs. weak beats. Weak and strong beats were compared, holding syllable strength constant (always strong). A significant cluster (p = 0.035) of electrodes mostly over left parietal, central, and right fronto-temporal regions (see Figure 5B) showed increased evoked gamma power for weak vs. strong beats (on strong syllables), from 116 to 208 ms.
Targets
Contrasting responses to targets preceded by well-aligned vs. misaligned vs. varied primes yielded four significant clusters for induced alpha and beta activity. All conditions showed increased power followed by decreased power relative to baseline (see middle column of Figure 6), but contrasting the conditions revealed differences in the degrees of increased and decreased power. Between 238 and 654 ms (cluster p = 0.006) from target onset, greater induced alpha power overall was observed for targets preceded by well-aligned compared to varied sentences, over right hemisphere regions. In a similar latency band (208–650 ms; cluster p = 0.01), well-aligned primes also led to greater induced alpha power in a widespread cluster of electrodes, compared to misaligned primes (see Figure 6, left column).
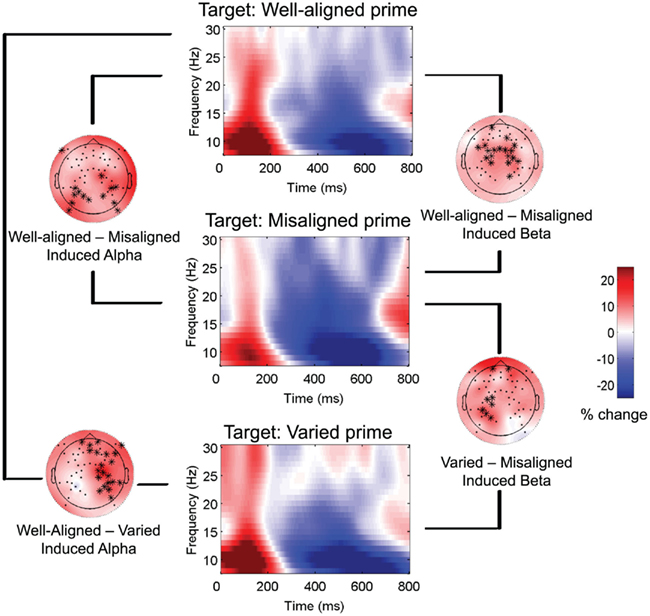
Figure 6. Time-frequency representations to targets: Induced alpha and beta. Brain responses to targets (words and pseudowords combined), primed by three types of sentences: Well-aligned, Misaligned, and Varied. Time–frequency representations (center column) for each condition were calculated by averaging together all channels. Results of clustering pairs of conditions are shown for induced alpha (left column) and induced beta (right column): topographic plots show difference in scalp power and topography of cluster at 400 ms, between the pair of conditions indicated.
Next, well-aligned primes led to greater early increased induced beta power for targets compared to misaligned primes, which led to greater mid-latency decreased power (see Figure 6, right column). The activity associated with this cluster of electrodes began in posterior and spread to central regions, stretching from 0 to 526 ms (cluster p = 0.002). Finally, varied compared to misaligned primes led to greater induced beta power, between 228 and 552 ms (cluster p = 0.03), starting over right hemisphere regions and then shifting to left hemisphere around 400 ms. Overall, well-aligned primes led to greater alpha and beta power on targets, compared to misaligned and varied. Thus, the pattern of increased followed by decreased alpha and beta power on visual linguistic targets seems to be modulated by the sentence alignment of the preceding prime. No significant clusters were found for the evoked data.
Discussion
In this study, participants listened to sung sentences with different kinds of alignment between linguistic stress and musical meter, and performed a lexical decision task in which they discerned words from pseudowords. Time–frequency analysis of EEG data acquired during the introductory metronome clicks showed expected patterns of increased high frequency power (evoked beta and gamma, and induced beta), and during the sung sentences showed increased induced beta power for well-aligned syllables (strong syllables on strong beats). However, these patterns of brain activity were modified for misaligned syllables. Notably, decreases in induced beta power were associated with the onsets of weak syllables that occurred on strong beats. Moreover, when strong syllables occurred on weak beats, a delayed peak in evoked gamma power was observed. Visual targets primed by misaligned and varied sentences were associated with greater decreases in induced alpha and beta power compared to well-aligned sentences, primarily over central and right regions of the scalp.
Beat Tracking
The lead-in metronome clicks were associated with increased induced beta, evoked beta and evoked gamma power at their onsets, as predicted from the literature on rhythmic tone sequences. The evoked beta and gamma may reflect the perceived onsets and metrical strength of tones (Zanto et al., 2005; Iversen et al., 2009), while the induced beta likely reflects top-down temporal expectancies (Snyder and Large, 2005). By reproducing previous findings, we were able to show that neural attending rhythms are set up during these isochronous metronome clicks prior to the sentence onsets.
Effect of Linguistic and Musical Alignment
When strong syllables occurred on strong beats, increased induced beta power was observed relative to weak syllables on strong beats. This finding of increased power at strong beats in the lead-in metronome, and at strong syllables when they occurred on strong beats during the sung sentences, are consistent with the literature showing that the timing of high frequency bursts entrains to the beat in rhythmic tone sequences (e.g., Zanto et al., 2005; Fujioka et al., 2009). Thus, weak syllables, in this contrast, were associated with decreased induced beta power, despite the fact that they occurred on strong musical beats. What is surprising and novel about this finding is that the high frequency response appears not to be entrained to the isochronous metronome clicks during the misaligned sentences. One interpretation of these observations is that beat tracking is sustained when strong syllables occur on strong beats, whereas it is disturbed when weak syllables occur on strong beats. However, the decreased induced beta power on weak syllables also agrees with results of Mayville et al. (2001) and Jantzen et al. (2001) showing beta desynchronization while participants performed syncopated finger movements compared to synchronized finger movements. Yet if the strong syllables were perceived as syncopated in relation to metronome clicks (strong beats), one might still expect to observe some relative increase in induced beta power on weak syllables that occur on strong beats (Iversen et al., 2009). Instead, beat tracking itself seems to have been disrupted to the point that beta and gamma responses were no longer timelocked to the isochronous metronome, providing an interesting exception to an otherwise consistent phenomenon in studies using tone sequences (Snyder and Large, 2005; Zanto et al., 2005; Fujioka et al., 2009; Iversen et al., 2009).
If beat tracking was disrupted when weak syllables occur on strong beats, would syllable tracking also be disrupted when strong syllables occurred on weak beats? Strong syllables on strong beats were thus contrasted with strong syllables on weak beats. A decrease in evoked gamma power was observed for strong syllables on strong beats at around 150 ms post-click-onset, consistent with the literature showing periodic high frequency synchronization followed by desynchronization during perception of rhythmic patterns (Fujioka et al., 2009). Furthermore, this cluster showed that strong syllables on weak beats were associated with increased evoked gamma power at around 150 ms. Here both conditions have strong syllables; therefore, syllable tracking seems to be affected by misaligned syllable and beats. This finding suggests that the brain shifts attending rhythms toward strong syllables rather than strong beats during perception of misaligned sung sentences. It remains to be determined whether the addition of singing is specifically responsible for the change in brain response to syncopation, or whether similar results would be observed in more complex syncopated sequences consisting of several instruments, as in orchestral music.
Induced beta activity seems to be involved in predicting the timing of tones in ongoing musical rhythms (Snyder and Large, 2005; Zanto et al., 2005), which are likely related to beta-mediated communication between auditory and motor areas (Fujioka et al., 2009; Fujioka et al., in revision). Evoked beta and gamma fluctuate in response to the presentation of a tone (Snyder and Large, 2005), with evoked beta showing power changes proportional to perceived metrical strength, even in the absence of physical differences in the intensity of tones (Iversen et al., 2009). Evoked gamma responses also discriminate between speech and non-speech sounds within 50 ms from stimulus onset (Palva et al., 2002). Thus, the current findings fit with the literature by differentiating functional roles of induced and evoked EEG. Here induced beta appears to reflects rhythmic anticipation of alignment of strong syllables with strong beats, while evoked EEG for strong syllables appears to be involved in mediating expectations and perception of the onsets of strong syllables.
Taken together, when the syllables and beats are in-phase, the neural response appears to easily entrain to the strong beats/syllables, which occur in alignment. By showing that attentional pulses synchronize to temporally predictable input, this finding is predicted by the dynamic attending theory (Large and Jones, 1999), and corroborated by recent studies on dynamic attending in music (e.g., Snyder and Large, 2005; Geiser et al., 2009, 2010; Nozaradan et al., 2011) and language (e.g., Port, 2003). These observations also coincide with phoneme monitoring data showing that attention is preferentially allocated to strong syllables (Shields et al., 1974; Pitt and Samuel, 1990; Quené and Port, 2005; Wang et al., 2005), which aid in segmenting the speech signal (e.g., Smith et al., 1989). Analyses of speech production and sensori-motor synchronization have also indicated that syllable stress acts as an attractor that captures attention and facilitates production of periodic, perceptually salient events (Kelso et al., 1983; Port, 2003; Lidji et al., 2011). In the present study, the metronome seems to build up expectations that in turn influence the processing of syllable stress in the sung sentences. Given the differences observed here in prime sentences, the subsequently presented targets were then analyzed to uncover potential differences in targets primed by sentences with different types of alignment.
Priming Effects on Targets
The brain response to target stimuli was analyzed to investigate whether different types of rhythmic alignment in the primes would affect the perception of visual words and pseudowords. Varied and misaligned sentences, compared to well-aligned sentences, led to lower levels of induced alpha power during presentation of the targets. The decrease in alpha power could be due to increased top-down attentional demands (Fan et al., 2007; Klimesch et al., 2007) when some or all of the syllables in the prime sentence are misaligned and therefore do not satisfy the type of prosodic expectancies that typically facilitate speech comprehension (e.g., Dilley et al., 2010). Alpha band (de)synchronization is likely related to domain–general mechanisms, such as attention and memory, that play an important role in lexico-semantic processing (Klimesch et al., 1999; Bastiaansen and Hagoort, 2006; Krause et al., 2006). This induced alpha activity seen for the alignment effects on the targets is primarily over the right hemisphere, perhaps related to fMRI data showing right hemispheric lateralization for prosodic aspects of speech (Glasser and Rilling, 2008).
Induced beta suppression (i.e., decreased power) was observed when contrasting targets primed by misaligned vs. well-aligned sentences. This cluster was already significant at the onset of the target stimulus and stretched past 500 ms over central regions, thus beginning earlier and lasting for longer than the induced alpha effects of alignment on the targets for well-aligned vs. misaligned and varied. As seen with the induced alpha in this same pair of conditions, this cluster of beta activity included more electrodes over the right than left hemisphere; however, the difference in lateralization was slight, and only significant at the earlier latencies. The fact that the differences in beta power were still present around the onset of the target stimulus strongly suggests that it reflects an ongoing process of rhythmic cognition in which the brain responds differently to the misaligned primes than the well-aligned primes, over a sustained time period that continues after the offset of the auditory stimulus. Furthermore, another cluster of electrodes showed greater beta suppression for targets primed by misaligned compared to varied primes, suggesting that the proportion of misaligned syllables (all, in the misaligned prime) modulates beta power more than the less predictable alignment and the irregular stress patterns in the varied sentences do.
Induced alpha and beta suppression have been specifically linked to the attention-related modulations required to carry out a semantic task on spoken words (Shahin et al., 2009). In the present study, misaligned sentences may have required greater attentional resources as participants attempted to understand them and search for semantically related targets in the lexicon, leading to the alpha and beta suppression on the targets. Interestingly, listeners’ highly focused attentional pulses to the metronome and the lyrics seem to work together in the well-aligned to actually require fewer cognitive resources to understand sentences and conduct lexical search on the targets. That efficient use of periodic fluctuations in well-aligned linguistic and musical rhythms stands in contrast to the less efficient strong beats and stress in opposition, in the misaligned and varied, which seem to lead to heavier overall attentional load and more labored processing of the targets.
Taken together, these results could be interpreted as follows. In the well-aligned sentences, it is easy for participants to attend to strong beats and strong syllables, because they occur together (in-phase). Unified accent structure helps the listener to track beats (induced beta) and strong syllables (evoked gamma) more efficiently, which may facilitate word segmentation in the prime sentences. These well-aligned primes facilitate early stages of processing of targets, reflected in increased alpha and beta power. In the misaligned sentences, strong syllables and strong beats occur in anti-phase, which may interfere with beat tracking (induced beta) and weaken/delay syllable tracking (evoked gamma). Syncopated structure between linguistic and musical rhythm may hinder segmentation of misaligned words, thus affecting task performance. If intelligibility, and thus clarity of the linguistic content, is weakened by the misalignment, then attentional demands would be greater for the lexical decision task, shown by alpha and beta suppression. Overall, these results of this study suggest that well-aligned textsettings improve rhythmic attending and linguistic comprehension7. In fact, Magne et al. (2007) reported that metrical stress violations in French words modulated lexico-semantic processing, even when participants were not attending to the prosodic dimension. The present results may thus be related to other electrophysiological evidence that prosodic expectations are involved in lexico-semantic retrieval at the local (word) level.
Perhaps the most intriguing aspect of the present findings is that induced beta modulations in both the prime syllables and target stimuli differentiate between well-aligned and misaligned conditions. Strong syllables on strong beats are associated with anticipatory beta increased power; increased beta power is also present at the onset of target stimuli preceded by those same well-aligned sentences. Weak syllables on strong beats show anticipatory decreased power, and relatively decreased beta power also characterizes the onset of targets that are preceded by misaligned sentences. The overall increase in beta power for well-aligned sentences may thus carry over into the presentation of its targets. This interpretation would be consistent with results on sensori-motor syncopation (Jantzen et al., 2001; Mayville et al., 2001), suggesting that induced beta suppression on the targets is specifically linked to syncopation between strong syllables and strong beats in misaligned sentences. Non-proximal, or distal, prosodic cues in word sequences can also affect not only perception of subsequently presented, lexically ambiguous target items, but also visual recognition of previously presented words (Dilley and McAuley, 2008; Dilley et al., 2010). In interpreting these effects, the authors discuss the possibility that internal oscillators are involved in building up periodic expectancies that form prosodic context and affect grouping, as in music (Dilley et al., 2010). Future work could utilize song prosody to bias grouping of lexically ambiguous syllables, by manipulating linguistic and musical meter in the “song” modality, thus shedding light on the extent to which metrically driven hierarchical grouping is shared by language and music.
Conclusion
We found that beta power increases when strong syllables align with strong beats, supporting the idea that metrical attending is facilitated by alignment; this increase on strong beats disappears when weak syllables are aligned with strong beats, suggesting that misalignment disrupts metrical attending. Overall, these results may provide a first step toward an explanation for the widespread tendency to align strong syllables and strong beats in songs. Periodic peaks in attentional energy (e.g., as described by Large and Jones, 1999), manifested in neural responses, would be facilitated when linguistic stress and musical beats are in-phase, and disrupted when they are in anti-phase. Typical textsetting behaviors on the part of composers, such as placing stressed syllables on strong metrical positions, and on the part of performers, such as lengthening well-aligned syllables more than misaligned ones (Palmer and Kelly, 1992) could be accounted for by the present findings. Moreover, by showing that alignment modulates lexical decision-making, these results are an encouraging first step in understanding why good textsettings are easier to learn and memorize (Gingold and Abravanel, 1987) and how the rhythmic dimension of lyrics facilitates memorization (Wallace, 1994; Purnell-Webb and Speelman, 2008).
Many further studies on song prosody will need to be undertaken to tease apart these influences; for example, sung words (in a given grammatical category) whose stress is well-aligned or misaligned with the musical meter, could be presented in isolation, or in a consistent word position within sentences, to reduce syntactical processing demands. The comprehension and lexical access of sung words with varying types of alignment and rhythms could be tested directly, while controlling for other linguistic and musical factors that influence intelligibility in songs (Johnson et al., in revision). Caution should be exerted in generalizing these tendencies to different linguistic systems, as certain languages may be more permissible than English toward misalignment in songs (e.g., Spanish: Rodríguez-Vázquez, 2006, and French: Dell and Halle, 2005). In addition to stress-to-beat alignment, alignment is usually achieved through durational accents (Palmer and Kelly, 1992), which of course, are important markers of stress in speech prosody (Ramus et al., 1999) and should thus be a focus of future studies on the neural basis of song prosody. The present work does provide a starting place for using techniques such as metrical strength manipulations in songs, time–frequency analysis of EEG data, and cross-modal priming paradigms, to investigate the neural correlates of textsetting and better understand the interplay between linguistic and musical rhythm in song. Continuing research in this area may shed light on the idea that shared mechanisms for processing linguistic rhythm and musical rhythm contribute to the universal appeal of song.
Conflict of Interest Statement
The authors declare that the research was conducted in the absence of any commercial or financial relationships that could be construed as a potential conflict of interest.
Acknowledgments
R. L. Gordon was supported by a Dissertation Fellowship from the American Association for University Women in 2007–2008 and the Daniel B. Newell and Aurel B. Newell Doctoral Fellowship in 2008–2009. E. W. Large was supported by the Air Force Office of Scientific Research (AFOSR FA9550-07-C0095). The authors gratefully acknowledge Steven Bressler, Caroline Palmer, Betty Tuller, and Alexandra Key for helpful comments on earlier versions of the manuscript. Portions of this study were adapted from R. L. Gordon’s doctoral dissertation.
Supplementary Material
The Supplementary Material for this article can be found online at http://www.frontiersin.org/Auditory_Cognitive_Neuroscience/10.3389/fpsyg.2011.00352/abstract
Footnotes
- ^In English, strong syllables typically have longer durations than weak syllables (Ramus et al., 1999); here the IVI for strong syllables are only 5 ms shorter than for weak syllables. This small difference, if perceived, could only bias the results in a direction opposite the hypotheses, i.e., the increased duration would lead to weak syllables being perceived as stressed despite their expected linguistic stress.
- ^www.nitrc.org/projects/cleave
- ^http://www.ru.nl/neuroimaging/fieldtrip
- ^Evoked activity is calculated by averaging EEG/MEG activity across trials and then performing a time–frequency transformation on the average waveform (ERP), thus preserving only the oscillations whose phases are time-locked to the stimulus. Induced activity, on the other hand, is calculated by first performing time-frequency transformations on the EEG activity in single trials, and then averaging together the resulting oscillatory power for each single trial, thus preserving the average amplitude envelope (see Tallon-Baudry and Bertrand, 1999 for more information). Through this process, the induced activity includes oscillations that are not necessarily phase-locked and thus would have been eliminated from the evoked during averaging.
- ^There is a statistical trade-off involved in specifying whether the clustering procedure would be carried out in a each frequency band without averaging over frequencies, in order to identify only a subset of frequencies showing effects; this was possible on the metronome click data due to the high signal to noise ratio afforded by the number of epochs on the metronome clicks (see EEG Preprocessing for number of epochs kept after artifact rejection). As many fewer epochs were available for each type of prime syllables and targets, the signal-to-noise ratio was less favorable, and therefore, power was averaged within each frequency band to optimize statistical power when carrying out the clustering.
- ^First, the data from both experimental conditions in a given comparison were collected into a single set. Then, random draws were taken from the combined data set to form two subsets, on which the test statistic was computed by summing together the individual t-statistics for each data point in the cluster. This process of random partition and computing the test statistic was repeated 2500 times, and those results were collected into a histogram of test statistics, that was then compared to the test statistic of the actual data.
- ^While the results do strongly suggest that comprehension is better in the well-aligned condition, the present experiment does not allow us to determine whether linguistic comprehension during the well-aligned condition is actually facilitated, or whether comprehension of well-aligned is similar to speech (which does not usually have a musical rhythm), but comprehension in the misaligned and varied conditions is in fact hindered. This is often the challenge in the devising experiments to demonstrate how speech prosody facilitates segmentation.
References
Bastiaansen, M., and Hagoort, P. (2006). “Oscillatory neuronal dynamics during language comprehension,” in Progress in Brain Research, eds C. Neuper and W. Klimesch (Amsterdam: Elsevier), 179–196.
Bastiaansen, M., Magyari, L., and Hagoort, P. (2010). Syntactic unification operations are reflected in oscillatory dynamics during on-line sentence comprehension. J. Cogn. Neurosci. 22, 1333–1347.
Boersma, P., and Weenink, D. (2007). Praat: Doing Phonetics by Computer. Available at http://www.praat.org [accessed February 6, 2007].
Brown, G. D. A. (1984). A frequency count of 190,000 words in the London-Lund Corpus of English Conversation. Behav. Res. Methods Instrum. Comput. 16, 502–532.
Cutler, A., and Norris, D. (1988). The role of strong syllables in segmentation for lexical access. J. Exp. Psychol. Hum. Percept. Perform. 14, 113–121.
Dell, F., and Halle, J. (2005). “Comparing musical textsetting in French and in English songs,” in Conference Proceedings from Typology of Poetic Forms, ed. J. L. Aroui, Paris.
Dilley, L. C., Mattys, S. L., and Vinke, L. (2010). Potent prosody: comparing the effects of distal prosody, proximal prosody, and semantic context on word segmentation. J. Mem. Lang. 63, 274–294.
Dilley, L. C., and McAuley, J. D. (2008). Distal prosodic context affects word segmentation and lexical processing. J. Mem. Lang. 59, 294–311.
Doppelmayr, M., Klimesch, W., Hodlmoser, K., Sauseng, P., and Gruber, W. (2005). Intelligence related upper alpha desynchronization in a semantic memory task. Brain Res. Bull. 66, 171–177.
Eerola, T., and Toiviainen, P. (2004). MIDI Toolbox: MATLAB Tools for Music Research. Available at http://www.jyu.fi/musica/miditoolbox/[accessed June 30, 2005].
Fan, J., Byrne, J., Worden, M. S., Guise, K. G., Mccandliss, B. D., Fossella, J., and Posner, M. I. (2007). The relation of brain oscillations to attentional networks. J. Neurosci. 27, 6197–6206.
Field, J. (2005). Intelligibility and the listener: the role of lexical stress. TESOL Q. 39, 399–423.
Fujioka, T., Trainor, L. J., Large, E. W., and Ross, B. (2009). Beta and gamma rhythms in human auditory cortex during musical beat processing. Ann. N. Y. Acad. Sci. 1169, 89–92.
Geiser, E., Sandmann, P., Jancke, L., and Meyer, M. (2010). Refinement of metre perception – training increases hierarchical metre processing. Eur. J. Neurosci. 32, 1979–1985.
Geiser, E., Ziegler, E., Jancke, L., and Meyer, M. (2009). Early electrophysiological correlates of meter and rhythm processing in music perception. Cortex 45, 93–102.
Gingold, H., and Abravanel, E. (1987). Music as a mnemonic: the effects of good- and bad-music setting on verbatim recall of short passages by young children. Psychomusicology 7, 25–39.
Glasser, M. F., and Rilling, J. K. (2008). DTI tractography of the human brain’s language pathways. Cereb. Cortex 18, 2471–2482.
Gordon, R. L., Schön, D., Magne, C., Astésano, C., and Besson, M. (2010). Words and melody are intertwined in perception of sung words: EEG and behavioral evidence. PLoS ONE 5, e9889. doi:10.1371/journal.pone.0009889
Grabner, R. H., Brunner, C., Leeb, R., Neuper, C., and Pfurtscheller, G. (2007). Event-related EEG theta and alpha band oscillatory responses during language translation. Brain Res. Bull. 72, 57–65.
Hannon, E. E., Snyder, J. S., Eerola, T., and Krumhansl, C. L. (2004). The role of melodic and temporal cues in perceiving musical meter. J. Exp. Psychol. Hum. Percept. Perform. 30, 956–974.
Hayes, B., and Kaun, A. (1996). The role of phonological phrasing in sung and chanted verse. Linguist. Rev. 13, 243–303.
Herrmann, C. S., Grigutsch, M., and Busch, N. A. (2005). “EEG oscillations and wavelet analysis,” in Event-Related Potentials: A Methods Handbook, ed. T. C. Handy (Cambridge, MA: MIT Press), 229–259.
Iversen, J. R., Repp, B. H., and Patel, A. D. (2009). Top-down control of rhythm perception modulates early auditory responses. Ann. N. Y. Acad. Sci. 1169, 58–73.
Jantzen, K. J., Fuchs, A., Mayville, J. M., Deecke, L., and Kelso, J. A. (2001). Neuromagnetic activity in alpha and beta bands reflect learning-induced increases in coordinative stability. Clin. Neurophysiol. 112, 1685–1697.
Jones, M. R. (2009). “Musical time,” in Oxford Handbook of Music Psychology, eds S. Hallam, I. Cross, and M. Thaut (New York: Oxford University Press), 81–92.
Jones, M. R. (2010). “Attending to sound patterns and the role of entrainment,” in Attention and Time, eds A. C. Nobre and J. T. Coull (New York: Oxford University Press), 317–330.
Jusczyk, P. W., Cutler, A., and Redanz, N. J. (1993). Infants’ preference for the predominant stress patterns of English words. Child Dev. 64, 675–687.
Kelly, M. H., and Bock, J. K. (1988). Stress in time. J. Exp. Psychol. Hum. Percept. Perform. 14, 389–403.
Kelso, J. A. S., Tuller, B., and Harris, K. S. (1983). “A ‘dynamic pattern’ perspective on the control and coordination of movement,” in The Production of Speech, ed. P. F. Macneilage (New York: Springer-Verlag), 138–173.
Klimesch, W., Doppelmayr, M., Schwaiger, J., Auinger, P., and Winkler, T. (1999). ‘Paradoxical’ alpha synchronization in a memory task. Brain Res. Cogn. Brain Res. 7, 493–501.
Klimesch, W., Sauseng, P., and Hanslmayr, S. (2007). EEG alpha oscillations: the inhibition-timing hypothesis. Brain Res. Rev. 53, 63–88.
Krause, C. M., Gronholm, P., Leinonen, A., Laine, M., Sakkinen, A. L., and Soderholm, C. (2006). Modality matters: the effects of stimulus modality on the 4- to 30-Hz brain electric oscillations during a lexical decision task. Brain Res. 1110, 182–192.
Lakatos, P., Karmos, G., Mehta, A. D., Ulbert, I., and Schroeder, C. E. (2008). Entrainment of neuronal oscillations as a mechanism of attentional selection. Science 320, 110–113.
Large, E. W., and Jones, M. R. (1999). The dynamics of attending: how we track time varying events. Psychol. Rev. 106, 119–159.
Lidji, P., Jolicoeur, P., Moreau, P., Kolinsky, R., and Peretz, I. (2009). Integrated preattentive processing of vowel and pitch: a mismatch negativity study. Ann. N. Y. Acad. Sci. 1169, 481–484.
Lidji, P., Palmer, C., Peretz, I., and Morningstar, M. (2011). Listeners feel the beat: entrainment to English and French speech rhythms. Psychon. Bull. Rev. doi:10.3758/s13423-011-0163-0
Magne, C., Astésano, C., Aramaki, M., Ystad, S., Kronland-Martinet, R., and Besson, M. (2007). Influence of syllabic lengthening on semantic processing in spoken French: behavioral and electrophysiological evidence. Cereb. Cortex 17, 2659–2668.
Marie, C., Magne, C., and Besson, M. (2011). Musicians and the metric structure of words. J. Cogn. Neurosci. 23, 294–305.
Maris, E., and Oostenveld, R. (2007). Nonparametric statistical testing of EEG- and MEG-data. J. Neurosci. Methods 164, 177–190.
Mattys, S. L., White, L., and Melhorn, J. F. (2005). Integration of multiple speech segmentation cues: a hierarchical framework. J. Exp. Psychol. Gen. 134, 477–500.
Mayville, J. M., Fuchs, A., Ding, M., Cheyne, D., Deecke, L., and Kelso, J. A. (2001). Event-related changes in neuromagnetic activity associated with syncopation and synchronization timing tasks. Hum. Brain Mapp. 14, 65–80.
Nozaradan, S., Peretz, I., Missal, M., and Mouraux, A. (2011). Tagging the neuronal entrainment to beat and meter. J. Neurosci. 31, 10234–10240.
Oostenveld, R., Fries, P., Maris, E., and Schoffelen, J. M. (2011). FieldTrip: open source software for advanced analysis of MEG, EEG, and invasive electrophysiological data. Comput. Intell. Neurosci. 2011, 156869.
Palmer, C., and Hutchins, S. (2006). “What is musical prosody?” in Psychology of Learning and Motivation, ed. B. H. Ross (Amsterdam: Elsevier Press), 245–278.
Palmer, C., and Kelly, M. (1992). Linguistic prosody and musical meter in song. J. Mem. Lang. 31, 525–542.
Palva, S., Palva, J. M., Shtyrov, Y., Kujala, T., Ilmoniemi, R. J., Kaila, K., and Naatanen, R. (2002). Distinct gamma-band evoked responses to speech and non-speech sounds in humans. J. Neurosci. 22, RC211.
Patel, A. D., Iversen, J. R., and Rosenberg, J. C. (2006). Comparing the rhythm and melody of speech and music: the case of British English and French. J. Acoust. Soc. Am. 119, 3034–3047.
Pitt, M. A., and Samuel, A. G. (1990). The use of rhythm in attending to speech. J. Exp. Psychol. Hum. Percept. Perform. 16, 564–573.
Purnell-Webb, P., and Speelman, C. P. (2008). Effects of music on memory for text. Percept. Mot. Skills 106, 927–957.
Quené, H., and Port, R. F. (2005). Effects of timing regularity and metrical expectancy on spoken-word perception. Phonetica 62, 1–13.
Ramus, F., Nespor, M., and Mehler, J. (1999). Correlates of linguistic rhythm in the speech signal. Cognition 73, 265–292.
Rastle, K., Harrington, J., and Coltheart, M. (2002). 358,534 nonwords: the ARC nonword database. Q. J. Exp. Psychol. 55A, 1339–1362.
Rodríguez-Vázquez, R. (2006). The metrics of folk song: a comparative study of text-setting in Spanish and English. Rhythmica Revista Española de Métrica Comparada 3–4, 253–281.
Sammler, D., Baird, A., Valabregue, R., Clement, S., Dupont, S., Belin, P., and Samson, S. (2010). The relationship of lyrics and tunes in the processing of unfamiliar songs: a functional magnetic resonance adaptation study. J. Neurosci. 30, 3572–3578.
Schirmer, A., Kotz, S. A., and Friederici, A. D. (2002). Sex differentiates the role of emotional prosody during word processing. Brain Res. Cogn. Brain Res. 14, 228–233.
Schmidt-Kassow, M., Schubotz, R. I., and Kotz, S. A. (2009). Attention and entrainment: P3b varies as a function of temporal predictability. Neuroreport 20, 31–36.
Schön, D., Gordon, R., Campagne, A., Magne, C., Astesano, C., Anton, J. L., and Besson, M. (2010). Similar cerebral networks in language, music and song perception. Neuroimage 51, 450–461.
Shahin, A. J., Picton, T. W., and Miller, L. M. (2009). Brain oscillations during semantic evaluation of speech. Brain Cogn. 70, 259–266.
Shields, J. L., Mchugh, A., and Martin, J. G. (1974). Reaction time to phoneme targets as a function of rhythmic cues in continuous speech. J. Exp. Psychol. 102, 250–255.
Smith, M. R., Cutler, A., Butterfield, S., and Nimmo-Smith, I. (1989). The perception of rhythm and word boundaries in noise-masked speech. J. Speech Hear. Res. 32, 912–920.
Snyder, J. S., and Large, E. W. (2005). Gamma-band activity reflects the metric structure of rhythmic tone sequences. Cogn. Brain Res. 24, 117–126.
Stefanics, G., Hangya, B., Hernadi, I., Winkler, I., Lakatos, P., and Ulbert, I. (2010). Phase entrainment of human delta oscillations can mediate the effects of expectation on reaction speed. J. Neurosci. 30, 13578–13585.
Tajima, K., Port, R., and Dalby, J. (1997). Effects of temporal correction on intelligibility of foreign-accented English. J. Phon. 25, 1–24.
Tallon-Baudry, C., and Bertrand, O. (1999). Oscillatory gamma activity in humans and its role in object representation. Trends Cogn. Sci. (Regul. Ed.) 3, 151–162.
Tallon-Baudry, C., Bertrand, O., Delpuech, C., and Pernier, J. (1996). Stimulus specificity of phase-locked and non-phase-locked 40 Hz visual responses in human. J. Neurosci. 16, 4240–4249.
Van Elk, M., Van Schie, H. T., Zwaan, R. A., and Bekkering, H. (2010). The functional role of motor activation in language processing: motor cortical oscillations support lexical-semantic retrieval. Neuroimage 50, 665–677.
Vlek, R. J., Schaefer, R. S., Gielen, C. C., Farquhar, J. D., and Desain, P. (2011). Sequenced subjective accents for brain-computer interfaces. J. Neural Eng. 8, 036002.
Wallace, W. T. (1994). Memory for music: effect of melody recall on text. J. Exp. Psychol. 20, 1471–1485.
Wallace, W. T., and Rubin, D. C. (1988). “‘The Wreck of the Old 97’: a real event remembered in song,” in Remembering Reconsidering: Ecological and Traditional Approaches to the Study of Memory, eds U. Niesser and E. Winograd (Cambridge: Cambridge University Press), 283–310.
Keywords: rhythm, EEG, beta/gamma oscillations, song perception, textsetting, prosody, language, music
Citation: Gordon RL, Magne CL and Large EW (2011) EEG correlates of song prosody: a new look at the relationship between linguistic and musical rhythm. Front. Psychology 2:352. doi: 10.3389/fpsyg.2011.00352
Received: 02 April 2011;
Accepted: 09 November 2011;
Published online: 29 November 2011.
Edited by:
Lutz Jäncke, University of Zurich, SwitzerlandReviewed by:
Stefan Debener, University of Oldenburg, GermanyMartin Meyer, University of Zurich, Switzerland
Copyright: © 2011 Gordon, Magne and Large. This is an open-access article distributed under the terms of the Creative Commons Attribution Non Commercial License, which permits use, distribution, and reproduction in other forums, provided the original authors and source are credited.
*Correspondence: Reyna L. Gordon and Edward W. Large, Music Dynamics Laboratory, Center for Complex Systems and Brain Sciences, Florida Atlantic University, 777 Glades Road, Boca Raton, FL, USA. e-mail: reyna.gordon@vanderbilt.edu; large@ccs.fau.edu