- 1 Brain and Cognition, Faculty of Social and Behavioural Sciences, University of Amsterdam, Amsterdam, Netherlands
- 2 Social Psychology, Faculty of Social and Behavioural Sciences, University of Amsterdam, Amsterdam, Netherlands
In this paper we sketch a new framework for affect elicitation, which is based on previous evolutionary and connectionist modeling and experimental work from our group. Affective monitoring is considered a local match–mismatch process within a module of the neural network. Negative affect is raised instantly by mismatches, incongruency, disfluency, novelty, incoherence, and dissonance, whereas positive affect follows from matches, congruency, fluency, familiarity, coherence, and resonance, at least when an initial mismatch can be solved quickly. Affective monitoring is considered an evolutionary-early conflict and change detection process operating at the same level as, for instance, attentional selection. It runs in parallel and imparts affective flavor to emotional behavior systems, which involve evolutionary-prepared stimuli and action tendencies related to for instance defensive, exploratory, attachment, or appetitive behavior. Positive affect is represented in the networks by high-frequency oscillations, presumably in the gamma band. Negative affect corresponds to more incoherent lower-frequency oscillations, presumably in the theta band. For affect to become conscious, large-scale synchronization of the oscillations over the network and the construction of emotional experiences are required. These constructions involve perceptions of bodily states and action tendencies, but also appraisals as well as efforts to regulate the emotion. Importantly, affective monitoring accompanies every kind of information processing, but conscious emotions, which result from the later integration of affect in a cognitive context, are much rarer events.
Introduction
An organism with the ability to discern adaptive from maladaptive conditions has a much higher chance of transmitting its genes than an organism without this ability. Brains possessing the capacity to distinguish these conditions, and to steer behavior in more adaptive directions, must therefore have developed early in evolutionary history. This fundamental ability has been linked by Johnston (2003) to the most basic quality of emotions: positive and negative affect are generated by the nervous system “to those aspects of the environment that were a consistent benefit or threat to gene survival in ancestral environments” (p. 173). Johnston’s reasoning presupposes a neural mechanism for determining whether a situation is advantageous for gene survival, which first translates the organism’s environmental conditions into internal representations and then compares them to “desirable” states. We will argue here that this affective monitoring provides a generic mechanism for affect elicitation. In our simple connectionist implementation, changes in level of competition within network modules are monitored, resulting in low-frequency oscillations of neural activity in the case of mismatch, and high-frequency oscillations in matching conditions. Similar competitive network modules have formerly been used to model competitive learning (Murre et al., 1992; Phaf, 1994) and attentional selection (Phaf et al., 1990; Duncan, 1996). The analysis of these functions in terms of competition suggests that organisms capable of attentional selection should also be able to monitor processing affectively, and that affective processing should not be limited to humans. The elementary nature of the affective monitoring modules, moreover, implies that in its most basic form affect constitutes a non-conscious process, which only through more elaborate constructive processing may develop into a conscious emotional experience (cf. Phaf and Wolters, 1997).
Affect, sometimes referred to as “core affect,” is often considered an irreducible component of emotion that cannot be analyzed further (e.g., Frijda, 1986; Ortony and Turner, 1990). This may stem in part from the behaviorist tradition where reward and punishment represent biologically given unconditioned stimuli that resist any analysis in terms of internal processes. At the other side of the spectrum, affect is uniquely associated with, perhaps the most basic, conscious states (i.e., feelings) that according to some (e.g., Chalmers, 2004) are almost impossible to capture in a mechanistic analysis. New simulations of the evolutionary development of nervous systems and findings of interactions between affect and, seemingly non-emotional, “cognition,” however, suggest that affect can be analyzed in terms of non-emotional information processing. In some experiments affective influences may even occur when the affective nature is not recognized either in the independent or in the dependent variable. The affective monitoring hypothesis offers a mechanistic account of affect elicitation and postulates that affect does not need to be conscious, or open to introspection (see also Berridge, 2003; Berridge and Winkielman, 2003).
Which stimuli signal adaptivity, or a lack of it, to the brain? There is probably a small class of stimuli that through large parts of evolutionary history were consistently related to adaptive benefits or costs. For Johnston (2003) the existence of such evolutionary-prepared stimuli seems inescapable: “If toxins tasted sweet, and sugar evoked a bitter taste, then survival would be in jeopardy” (p. 175). Besides tastes and smells, also relatively intense (i.e., painful) stimuli are probably evolutionary prepared (e.g., the startle reflex; Lang, 1995). Whether more complex stimuli, such as snakes, spiders, and emotion faces (e.g., Öhman, 1986) are also evolutionary prepared still remains a matter of scientific debate (e.g., Blanchette, 2006). Little is known about which specific simple stimulus characteristics would be able to drive affect directly (but see Vuilleumier et al., 2003). In isolation, most stimuli are ambiguous with respect to their evolutionary and affective value. We will argue here that such evolutionary-prepared stimuli can directly activate behavior programs (cf. Panksepp, 1998), and may only indirectly elicit affect through the monitoring of activity in these programs.
Other even simpler processing characteristics that are not stimulus specific have been available from the start of the evolutionary development of neural networks. Affective monitoring focuses on conflicts in processing and the subsequent resolution of conflicts (i.e., change detection). The detection of both conflict, implemented by neural inhibition, and change, constituting the first-order derivative of neural excitation, requires less complicated neural machinery even than identifying a stimulus, which proceeds through a progressive combination of stimulus features (cf. Hubel and Wiesel, 1977). In the approximation and averaging process performed by evolution, the quick resolution of conflict was associated with relatively beneficial circumstances, whereas lasting obstructions and interruptions were linked with challenges and potential threats to survival. The latter generally imply that priority should be given to steering behavior in more adaptive directions (cf. the evolutionary simulations by Heerebout and Phaf, 2010a,b), which may be avoided if the conflict can be relieved rapidly.
Nervous systems are likely capable of analyzing changes in all constituent features of a stimulus, separately. The representations for these features mostly result from learning processes, so the match with memory representations (i.e., familiarity) may play an important role in affect elicitation. The scope of this match–mismatch detection (cf. Williams and Gordon, 2007) likely extends to everything that can be represented by the brain. The correspondence of automatized bodily actions with stimulus features, for instance, may be a strong source of affect (e.g., Beilock and Holt, 2007; see also Cannon et al., 2010). In addition, if arrow direction agrees with habitual eye movements made in the reading direction (i.e., the habitual direction of attentional shift) this raises positive affect, even when the person is not aware of these influences or the affect itself (Phaf and Rotteveel, 2009). Conversely, the inhibition of non-selected stimuli induces negative affect (Raymond et al., 2003). At a semantic level, moreover, word triads with a remote associate raise more facial muscle activity indicative of positive affect than word triads without such an associate, even though the participants were ignorant of the underlying structure (Topolinski et al., 2009). The pluriformity of potential to-be-matched representations has led to a large variety of terms for the match (e.g., smooth, fluent, familiar, congruent, resonant, coherent) and mismatch (e.g., obstructed, disfluent, novel, incongruent, dissonant, incoherent). To emphasize the generality of the affect elicitation process, we propose the term affective monitoring. Match–mismatch is determined locally in aggregates of closely connected nodes, which we have previously called modules (Murre et al., 1992). Which representational feature is being processed by the module depends on its interconnections to other modules within the network. Only when there is a convergence of match–mismatch determinations in many modules, an affective state arises, which may be elaborated into a full emotion. Affective monitoring occurs continuously on all active representations in the neural network, but only now and then transforms into a conscious emotion. According to this view, “cognitive” and affective processing cannot be separated, though the latter is often not experienced consciously, and affective monitoring represents one of the most elementary operations performed by the brain.
The Model
A Neural Implementation
Affective monitoring essentially comprises a conflict-detection mechanism within a network module. High levels of conflict elicit negative affect, whereas the swift resolution of conflict, resulting in “smooth” functioning due to matching representations, signals positive affect. At the neural level conflicts are often modeled in terms of mutual inhibition and competitive processes (e.g., Rumelhart and Zipser, 1985). Competitive models have been applied successfully to self-organization of visual representations (e.g., von der Malsburg, 1973), implicit and explicit memory performance (Murre et al., 1992; Phaf, 1994), attentional selection (Phaf et al., 1990), and even fear conditioning (Armony et al., 1995). A role for competitive processes in the elicitation of affect has first been suggested by the evolutionary simulations of Heerebout and Phaf (2010b). The fact that neural competition emerges so readily when optimizing evolutionary fitness suggests that it may be a basic building block of the neural networks responsible for many kinds of information processing, including cognitive and emotional functioning.
Neural processes are generally envisaged in the language of neuron activations and activation transfer via connections. Connections can be either excitatory, increasing the activation of the receiving neuron, or inhibitory, decreasing the activation. A suitable formalism for building process models can be found in artificial neural networks or connectionist models (e.g., see Murre et al., 1992). The latter term emphasizes that complex function arises from connecting many very simple processors in a specific manner. Network models formulated in the connectionist language, however, represent extreme simplifications, which cannot capture the full range of complexities of biological neural networks. Despite these limitations, we think that the connectionist formalism provides good opportunities for casting the affective monitoring hypothesis in a mechanistic model. Not only can such simplified models capture core processes essential for this function, but developing concrete computational models may also lead to new insights into affect.
In evolutionary computation the structure of the models is not designed by the modeler to fit some set of empirical data, but emerges autonomously from the optimization performed by the evolutionary algorithm (cf. Holland, 1975) under a specific set of environmental conditions. den Dulk et al. (2003, see Figure 1), for instance, simulated agents that could increase their fitness by attending selectively to either plants or predators. In the computational evolution, the weights of the agents’ artificial neural networks developed in such a way that the agents showed organized behavior by avoiding predators and approaching plants. The resulting networks had a dual-processing architecture (cf., LeDoux, 1996) with avoidance taking priority over approach and predator and food only being differentiated in the indirect route. This architecture emerged autonomously in the simulations under the conditions set out by LeDoux’ evolutionary reasoning and thus made it more plausible that it had actually developed in this manner during evolution. Evolutionary computation also possesses a capacity of generating new hypotheses that have not been previously thought of by psychologists or cognitive neuroscientists.
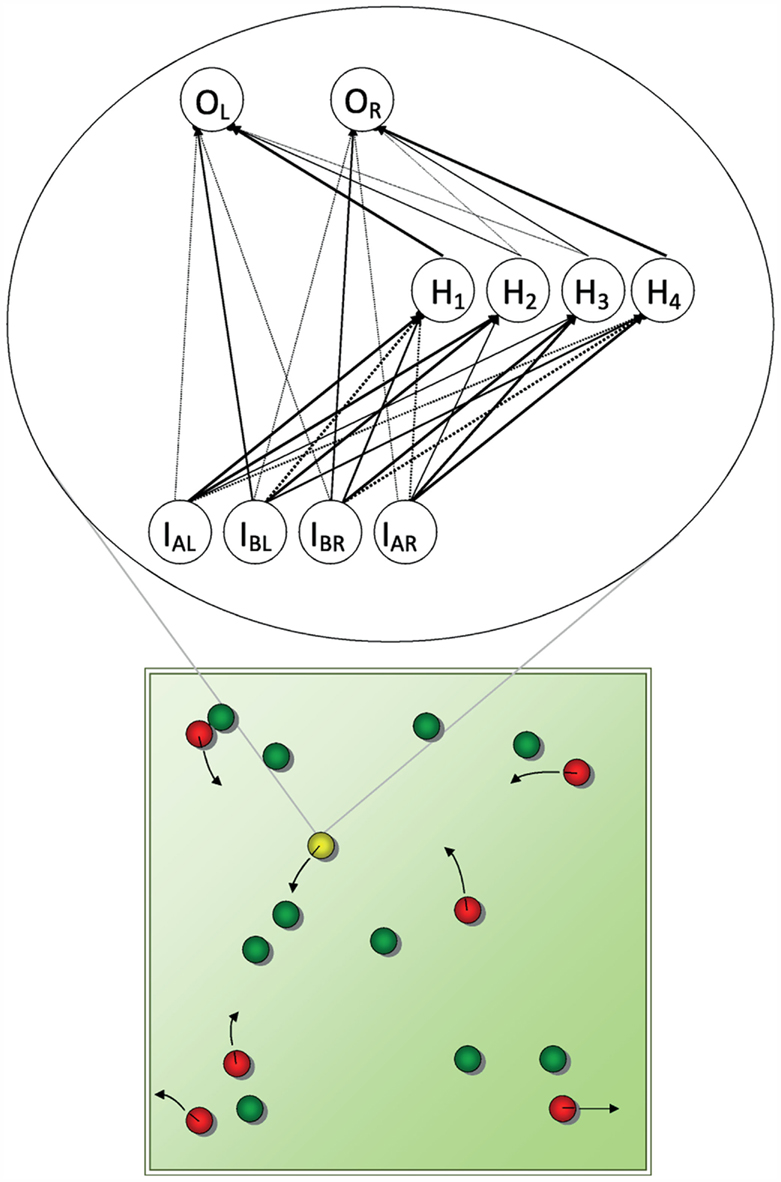
Figure 1. A schematic representation of the torus-shaped (i.e., without edges) virtual world in the evolutionary simulations of den Dulk et al. (2003). Predators (red), food patches (green), and agents (yellow) emit different scents. Agents have receptors for the predator and food scents, predators for the agent scents only. Predators and agents have left and right actuators and move according to the laws of classical mechanics. Collisions between predator and agent result in the agent being “eaten,” between agent and food in the disappearance of the food patch. The predator is controlled by a fixed neural network, so that it moves toward the agent. The agent’s network (an example resulting from the den Dulk et al. simulations is shown at the inset) is subject to mutations and crossovers to its genes (i.e., the connection weights). Starting out with random connection strengths, eventually after hundreds of generations organized behavior (i.e., avoidance of predators, approach of food) emerges, due to the selection of the fittest agents for reproduction.
More recently, after including the possibility of recurrent connections between nodes, oscillations emerged spontaneously in these networks, which nearly doubled the agents’ fitness (Heerebout and Phaf, 2010a). Although neural oscillations have been investigated extensively (e.g., Buzsáki and Draguhn, 2004), the function suggested by the evolutionary simulations appears to be new. The oscillations facilitated attentional switching from plants to predators, and thus led to quicker and more forceful avoidance reactions when a predator suddenly showed up. In addition, high-frequency oscillations occurred with fitness-increasing stimuli (i.e., food), whereas low-frequency oscillations were evoked by fitness-reducing stimuli (i.e., predators; Heerebout and Phaf, 2010b).
The networks of the evolved agents revealed strong inhibitory influences between neighboring nodes, which is characteristic of competitive networks (Heerebout and Phaf, 2010b). Lateral inhibition and competition have traditionally served as an explanatory mechanism for selective attention (e.g., Duncan, 1996; see also Phaf et al., 1990), and these simulations indicate that oscillations act as a supplementary mechanism to selection. According to the analysis of Heerebout and Phaf, the oscillations did not specifically influence the type of response that would be selected in the competitive networks, but modulated the speed of selection and of switching between selections. The trough in an oscillation (i.e., when the “winner” is least active) is of course the perfect time to switch “winners” in a competitive process. Most importantly, however, both selection by competition and oscillations emerged autonomously from the simulations and, as reflected in the fitness levels, appeared to have separate adaptive functions. Extending on these simulations, we argue that affect can be dissociated from the selection of approach and avoidance responses (see also Gable and Harmon-Jones, 2010), and that positive and negative affect are not stimulus-bound but are derived from the processing dynamics in the nervous system.
Internal monitoring did not yet arise from the evolutionary simulations, but may be derived from the competitive networks we previously designed for the purpose of modeling implicit and explicit memory effects (e.g., Phaf, 1994; Phaf et al., 1994, 2001). The memory models consisted of separate modules (i.e., CALM modules; see Murre et al., 1992) capable of detecting the local level of competition and thus distinguishing familiar (i.e., matching) from novel (i.e., non-matching) input to the module. As a consequence of this competition monitoring, CALM modules can exhibit two different modes of learning, which have been invoked to account for implicit and explicit memory performance (Graf and Mandler, 1984). Novel input results in elaboration learning, which is characterized in CALM by an increased learning rate combined with the distribution of non-specific, random activations over the module. Elaboration leads the input pattern to become associated with a node that is not yet committed by any other pattern. When familiar input is presented, however, the absence of competition keeps the learning rate low and only the existing representation is strengthened (i.e., activation learning).
Connection weights in biological networks are subject to change on two different time-scales: during phylogenesis and during ontogenesis. Presumably, both the gross network architecture of module interconnections and the internal connection scheme of a module have largely been put into place by evolution. Fine-tuning of this gross connection structure takes place by learning from experiences during ontogenesis, for instance through Hebbian learning (see Murre et al., 1992). The networks, generally, start out with exuberant connections (for a review see Innocenti and Price, 2005). During development, the number of connections is selectively reduced through the physical elimination of weak synapses (i.e., pruning) and specific connection patterns arise through learning processes. Both genetically pre-programmed pruning and selective pruning of weak and unused connections increase the specificity of the connection schemes (see Innocenti and Price, 2005). The network architecture responsible for affective monitoring has, in our opinion, been largely installed by evolution, but is similarly fine-tuned by pruning and learning during ontogenesis.
The competitive mechanism (see Figure 2) was built from a few architectural principles. First, two basic node types are distinguished that can give off only excitatory or only inhibitory connections. We called the former ones representation nodes (R-nodes) and the latter ones veto nodes (V-nodes). Secondly, we defined modules as regions with dense, excitatory and inhibitory, intramodular connections and sparser, long-range, only excitatory, intermodular connections (cf. Phaf et al., 1990; Murre et al., 1992). The inhibitory effects exerted by the V-nodes, generally, result in a competitive working of the module. Due to reciprocal inhibition, two simultaneously active V-nodes will try to suppress one another. The most strongly activated node wins the competition, resulting in a single winner (“winner takes all,” see Murre et al., 1992), or an activated neighborhood of only weakly inhibited nodes (see Phaf et al., 2001). V-nodes can only get activated by the excitatory connections from R-nodes within the same module. We have assumed that there is a tight coupling between specific R-nodes and V-nodes, so that the winner actually consists of an R–V node pair. If the V-node wins, the coupled R-node from which it receives its excitation, will also win the competition.
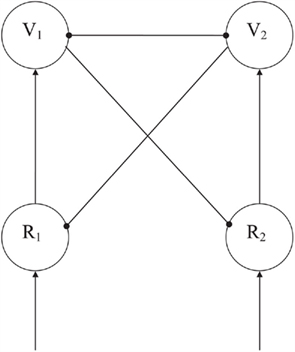
Figure 2. A two input node competitive network with separate excitatory (R) and inhibitory (V) nodes. Arrows denote excitatory connections, globules inhibitory connections. The network is a simplified variant (i.e., without oscillations occurring) of the schematic network that evolved in the simulations of Heerebout and Phaf (2010b). Competition is implemented by strong mutual inhibition of the V-nodes. The winning V-node eventually suppresses its competitor and the corresponding R-node, and releases its own R-node from the inhibition by the competing V-node.
The specific function of novelty detection in CALM modules constitutes a straightforward extension of the above competitive principles. Novel, not previously encountered and stored, input will simultaneously activate many R-nodes and subsequently initiate much competition. Novelty detection works by determining the amount of competition in the module. The activation of the negative monitoring Rne node (see Figure 3) is determined by subtracting the total amount of inhibition by the V-nodes from the total amount of excitation from their paired R-nodes. Due to the mutual inhibition of the V-nodes, the balance will swing toward excitation of the Rne node when many V-nodes are simultaneously active. If only one V-node is active, however, inhibition of the Rne node will dominate. In the CALM module, enhanced Hebbian learning, or elaboration learning, will help settle the competition, and will lead to a strengthening of intermodular connections to the winning nodes and to a weakening of the connections to losing nodes. With representation of the input, which then has been committed to a R-node and thus become familiar, much less competition is evoked.
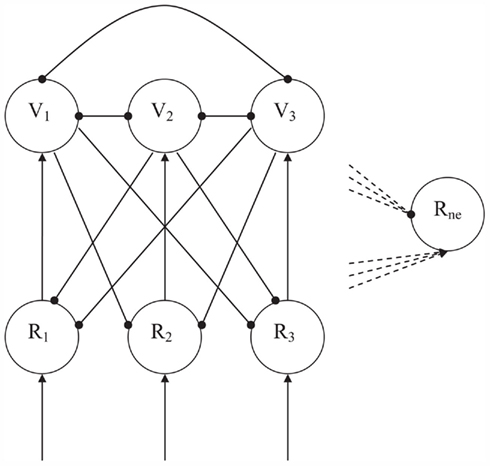
Figure 3. The CALM module with monitoring ability of level of competition. Arrows denote excitatory connections, globules inhibitory connections. Input is represented on the representation nodes (Rn). The inhibitory Veto nodes (Vn) enable competition between potential representations. When there is much competition, the sum of activations from the R-nodes and inhibitions from the V-nodes to the Negative node (Rne) will be positive. With little competition this sum will be negative and the Rne node will not be active.
Positive Affect
With a variation on Whittlesea and Williams’ (1998) famous observation that encountering one’s spouse in the kitchen does not raise much familiarity, but unexpectedly meeting her in a crowded stadium does, we expect that the former situation would also not elicit particularly much positive affect, but the latter would indeed. The quick resolution of conflict in the latter case is a prerequisite both for familiarity and positive affect. Despite the processing of the spouse being massively fluent in the kitchen, the initial conflict is missing here. The laughter raised by quick tension release (cf. Sroufe and Waters, 1976), as is the case in humorous jokes, may be an extreme case of such an initial conflict. In many cases more subtle uncertainties are evoked by task instructions, as for instance in our arrow experiment (Phaf and Rotteveel, 2009), which then can be settled quite easily, or not, by task execution. Another example of such subtle initial incongruities can be found in the mere-exposure task when the participant is asked to select one from two test stimuli. In this type of task effects are largest when the fluency is unexpected (Willems and Van der Linden, 2006), or discrepant after a change in fluency (Hansen et al., 2008). From an evolutionary point of view, continuous fluent processing without any change in conditions would also not expected to signal particularly fitness-enhancing opportunities.
Positive affect arises when the matching process initially raises competition, and the competition can be solved quickly. For this purpose, also a solution-of-competition detector node is required. In the CALM module, presence-of-competition was implemented by a dedicated R-node, collecting the excitations and inhibitions from the other R- and V-nodes in the model. A similarly connected V-node (Vne node) that inhibits a positive monitoring R-node (Rpo node) would allow this Rpo node to become active only after the resolution of competition (see Figure 4). The Rpo node itself is driven by the Rne node, reflecting that positive affect needs to be preceded by some level of competition in the matching process. Because the Vne node decays more quickly that the Rne node, the simple three-node network of Figure 4 functions as a change detector. With a rapid decrease of competition the Rne node will remain activated longer than the Vne node, so that the Rpo activation will be released. Basic assumptions in this network design are thus that positive affect can only follow after some initial level of competition, and that positive affect occurs later in time than negative affect.
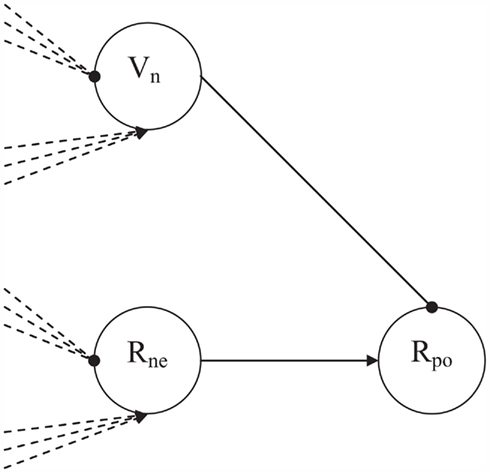
Figure 4. The affective monitoring submodule with the Rne, Vne, and Rpo nodes. Both Rne and Vne receive excitatory input from the other R-nodes and inhibitory input from the other V-nodes. Vne activation decays quicker than Rne activation, so that Rpo will get activated particularly after fast reductions in level of competition.
Affective monitoring distinguishes three types of internal functioning of the module. If the input to the module evokes initial competition, but this competition can be solved quickly, the faster decay of the Vne than of the Rne node will evoke Rpo activation (Figure 5A). If on the other hand the input sustains the competition and it cannot be settled quickly, for instance because no unified representation can be formed for conflicting inputs, Rne and Vne activations remain high. The strong inhibition by the Vne node will then suppress any Rpo activation (Figure 5B). Finally, the inputs to the module may match directly, without evoking much competition. In this case neither Rne nor Rpo node will be activated (Figure 5C).
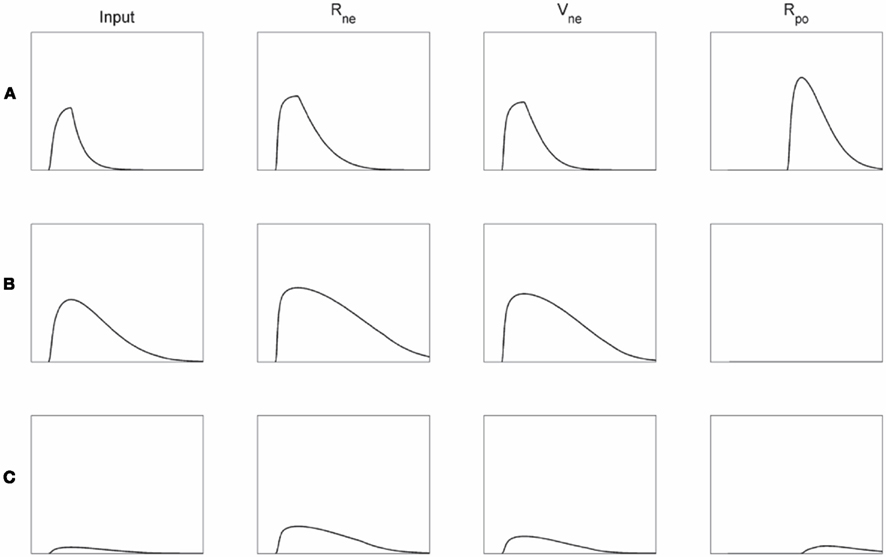
Figure 5. Activation plots of the network presented in Figure 4. The left column depicts the input (i.e., level of competition in the module) to the Rne and Vne nodes, subsequent columns the Rpe, Vne, and Rpo activations, respectively. (A) Initially much competition arises due to the conflicting module input, but this can be solved quickly, which then leads to considerable Rpo activation. (B) If the competition cannot be resolved quickly, the Rne and Vne nodes remain active for longer periods resulting in a net inhibition of the Rpo node. (C) If the module input does not lead to much competition from the start (i.e., a direct match), neither Rne nor Rpo will become activated very strongly.
From a classical empirical study on the development of the smile and laughter, Sroufe and Waters (1976) concluded much earlier to a similar origin of positive affect: the tension–release hypothesis. Although these authors did not want to identify the initial tension, which they also associated with incongruity or discrepancy, with negative affect, they postulated that the quick release from tension could evoke smiles and laughter. The network of Figure 4, which of course stems from a different source, could be seen as a connectionist implementation of this tension–release hypothesis. Tension is represented by the level of competition between nodes in the module. If the competition can be resolved quickly, positive affect arises. If it cannot, negative affect will remain activated. If there is no initial competition, neither positive nor negative affect is evoked. Affective monitoring thus extends on the tension–release hypothesis by specifying also the conditions for negative affect, when the tension holds on and inhibits the release of positive affect.
Temporal Order of Positive and Negative Affect
The network implementation of affective monitoring entails that positive affect arises later than negative affect, because the former can appear only after the latter has disappeared. Williams and Gordon (2007) earlier postulated this order, which they deduced from their ERP findings with emotional facial expressions (Williams et al., 2006). They showed that the potentials distinguishing fearful faces from neutral conditions precede the potentials distinguishing happy faces from neutral. In addition, Williams and collaborators obtained larger and more distributed activations with fearful expressions than with happy expressions. They concluded that signals of danger gain precedence and therefore are processed earlier than other stimuli. According to affective monitoring, the higher levels of competition with negative than with positive affect could account for the earlier, larger, and more distributed activations, but interpretations in terms of differential pattern classification are also possible (Schyns et al., 2007). There also appears to be a large variability in the kind and direction of effects of facial emotion on EEG and MEG responses (e.g., Astikainen and Hietanen, 2009; Straube et al., 2011). In our view, moreover, positive and negative affect are not evoked directly by happy and fearful expressions, but result from subsequent monitoring of activity elicited by the faces. The fearful faces may, for instance, interfere with the task instruction of maintaining vigilance for post-experimental testing in the Williams et al. study, whereas happy faces indicated that everything was going smoothly. Affective monitoring predicts that further ERP research using fluency manipulations that directly elicit affect would show the temporal order more consistently.
Neuroimaging research focusing on memory performance and the medial temporal lobe (MTL) has yielded converging evidence for the order of positive and negative affect predicted by affective monitoring (Daselaar et al., 2006). In our view, memory matches during retrieval result in high levels of familiarity and positive affect, whereas memory mismatches correspond to novelty and negative affect. In previous modeling efforts from our group (Phaf, 1994; Murre, 1996; Phaf et al., 2001) we have assumed that the hippocampus, which together with the parahippocampal gyrus is located in MTL, is involved in novelty detection of episodic memories. This assumption appears to be borne out by neuroimaging work (Strange et al., 1999; Daselaar et al., 2006), but other regions, such as prefrontal cortex, have also been implicated in novelty detection (e.g., Yamaguchi et al., 2004). Indeed, according to affective monitoring, novelty detection should be a distributed process, with different regions involved in different forms of novelty. The converging neuropsychological and neuroimaging evidence strongly points to the specific involvement of MTL in the monitoring of memory representations (e.g., Köhler et al., 2002).
In the study of Daselaar et al. (2006) activations of anterior and posterior parts of MTL were determined in a word recognition task for Hits (H), Misses (M), Correct Rejections (CR), and False Alarms (FA), separately. True oldness was reflected by activation levels associated with combined H and M performance, as compared to CRs and FAs. Because the participant does not need to be aware of the memory match, activations corresponding to true oldness can be said to reflect overall level of familiarity (i.e., match with stored memory representations. Novelty was evidenced by higher activation levels on CRs and Ms than on Hs and FAs. The activation of posterior parts of MTL, which includes parahippocampal cortex, was highly correlated with true oldness of stimuli. Anterior parts of the hippocampus were particularly sensitive to perceived novelty. Similar results were also obtained with the recognition of visual scenes. Köhler et al. (2002) for instance showed an involvement of the posterior parahippocampal place area only when the recognition could be based on familiarity.
Interestingly, posterior parahippocampal regions have also been linked to positive affect. Yue et al. (2007) found that activity in posterior parts of MTL after presentation of visual scenes correlated positively with subjective scene preferences. According to these authors the (posterior) parahippocampal cortex is particularly rich in endorphine receptors, which seem to be related to perceptual pleasure. Also more conventional reward regions (i.e., ventral striatum) showed higher activity levels with preferred than less-preferred scenes, which in our view may result from synchronization across different neural regions. Daselaar et al. (2006) finally investigated the functional connectivity between anterior and posterior parts of MTL. Importantly, and in agreement with our model (see Figure 4), they observed a negative coupling from anterior MTL to posterior MTL, but not vice versa. This finding implies that activity induced by novelty processing can inhibit activity involved with familiarity processing, but not the other way around. Extending this connection structure outside the memory domain, the affective monitoring view posits that negative affect inhibits the activation of positive affect, but not vice versa, and that therefore positive affect occurs later in time than negative affect.
What is Evoked?
Neural codes for positive and negative affect emerged from the evolutionary simulations of Heerebout and Phaf (2010b). When the agent in these simulations encountered fitness-increasing stimuli (i.e., food patches), its network nodes oscillated with higher frequencies than when it was confronted with fitness-reducing stimuli (i.e., predators). According to Johnston (2003), the latter stimuli should be called negative and the former positive. The simulations also yielded clues about how the oscillations enhanced fitness. Troughs in the oscillations, which have the lowest activation in the cycle, provide the best opportunity for competitors to topple the previous winner after a change in input. When there are many troughs over time, switches will be made earlier than when there are only few. High-frequency oscillations with food stimuli, therefore, allow for fast attentional switches from food to predator when the threat appears suddenly. With lower frequencies, the locking of attention to the predator ensures the highest fitness levels. The evolutionary simulations inspired the attentional switching hypothesis (Heerebout, 2011), which argues that positive affect facilitates attentional flexibility (cf. Dreisbach and Goschke, 2004; Fredrickson, 2004; van Wouwe et al., 2011), whereas negative affect prevents disengagement of attention (cf. Yiend and Mathews, 2001; Phaf and Kan, 2007).
The evolutionary simulations also demonstrated how oscillations could arise within a competitive setup (Heerebout and Phaf, 2010b). Over generations a flip–flop, or push–pull, mechanism evolved with feed-forward excitatory and feedback inhibitory connections between nodes. Figure 6 shows how this oscillation generator can be implemented in the network of Figure 4 by adding a Vpo node, which is solely connected to the Rpo node. The R-node excites the V-node, which subsequently inhibits this R-node, so that in the next time step the V-node activation will go down and inhibit the R-node less. If the R-node receives non-zero input from other sources (i.e., the Rne node), this flip–flop process will repeat itself over and over again, and an oscillation results. In the CALM module (Murre et al., 1992), which acts as a template for the present affective monitoring model, activation of the Rne node already resulted in randomly distributed activations to the other R-nodes of the module to break symmetry. Similarly, we propose here that the Rpo node distributes its oscillatory activity among these R-nodes and thus entrains them to the oscillation. Rne node activation should spread lower-frequency, more incoherent spikes among the R-nodes.
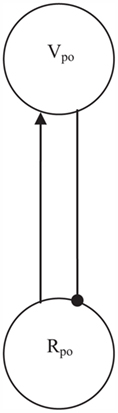
Figure 6. Activation of Rpo results in oscillations through a flip–flop mechanism. Excitation of Vpo by Rpo is followed by inhibition of Rpo from Vpo in the next time step. With a constant input to Rpo this push–pull process repeats itself indefinitely.
The evolutionary simulations did not specify which frequencies (i.e., number of cycles per time unit) are negative and which are positive. For one thing, the relation of the time unit, which is the time needed to update all activations once, in artificial neural networks to actual time in biological neural networks is unknown. In addition, the many simplifications made in connectionist modeling also preclude a direct translation of model time into actual time. For more precise ideas about these frequency bands we therefore had to turn to research into neural oscillations and affect. Because the two do not seem to have been associated before, only a few studies are available with more or less coincidental findings of a relation between oscillations and affect. Much more work has, however, been done on oscillations and attention (Herrmann, 2001; Womelsdorf and Fries, 2006, 2007; Bauer et al., 2009), which may support the attentional switching hypothesis emerging from the evolutionary simulations.
The specific association of gamma (20–70 Hz) oscillations to positive affect only came up from a conditioning study of Tsai et al. (2009) with their innovative optogenetic method, which entails the regulation of cellular activity by light pulses in genetically modified animals. They established a causal relationship between gamma stimulation and positive affect by showing that selective 50 Hz stimulation of dopamine neurons served as a strong reward signal in a place preference task performed by genetically modified mice. They controlled the timing of dopamine release by neurons in the ventral tegmental area through light pulses. The neurons were stimulated with high-frequency light pulses (50 Hz) in one room and with low-frequency light pulses (1 Hz) in another. The mice developed a strong preference for the room that had been reinforced by gamma, even though the total number of light flashes was equal in both frequency conditions. Gamma stimulation, however, elicited phasic increases in dopamine release that were more than 50 times higher than after low-frequency light pulses. The gamma resonance in these dopaminergic cells enables a broader range of modulatory effects than of attentional flexibility by the oscillations alone. The production of neuromodulators, such as dopamines and endorphins, may add to the specific consequences of gamma oscillations by evoking a broader range of physiological reactions and action tendencies. Long-range synchronization (e.g., Gregoriou et al., 2009) of gamma, elicited anywhere in the network, can thus turn gamma oscillations into a more global positive state modulating many different aspects of behavior.
The core attentional switching effect of gamma was supported by another optogenetic study from the same group. Sohal et al. (2009) showed that gamma induced in the prefrontal cortices of the mice enhanced information transmission through the network. This transmission was defined as the difference between response entropy, which measures variability of output, and noise entropy, which reflects how much output variability is unrelated to input. It thus constitutes the degree to which the output follows the input, and would in our terms depend upon attentional flexibility. The higher the flexibility, the more information can be transmitted through the network. The authors of these parallel optogenetic studies did not explain why they expected these remarkable findings or how they were related. According to the hypotheses emerging from the evolutionary simulations, however, synchronized gamma oscillations both signal positive affect and facilitate attentional flexibility, thereby increasing information transmission (Heerebout and Phaf, 2010a,b).
Both affective and attentional consequences of gamma oscillations induced by brightness variations on the screen (i.e., flicker) were observed in a recent study from our group (Heerebout, 2011). The entrainment of neural activations by visual gamma flicker has been demonstrated in visual areas of occipital cortex (Herrmann, 2001; Williams et al., 2004), but the oscillations may well spread to other areas (Gregoriou et al., 2009). We adapted the setup of Bauer et al. (2009), who showed that subliminal presentation of 50 Hz visual flicker facilitated attentional shifting to the flicker position. A stimulus display contained gray-scale images of male and female, emotionally neutral, faces and consisted of either two males (i.e., distracters) and one female (i.e., target), or two females (i.e., distracters) and one male (i.e., target). A circular patch flickering at 50, 25, or 0 Hz preceded the target. Participants decided on the gender of the target face (Block 1), and whether they felt the target face was positive or negative (Block 2). A flicker-detection task was performed in a third block. Two-alternative-forced-choice responses in the first two blocks were made with the approach-avoidance button stand of Rotteveel and Phaf (2004). In the latter study we found that happy faces speeded approach actions and slowed avoidance actions, whereas these action tendencies reversed with angry faces.
The main finding of Heerebout (2011) was that 50 Hz gamma flicker evoked positive affect, both when measured implicitly (i.e., Block 1) with the approach–avoidance task and when faces were evaluated explicitly (i.e., Block 2). If the target was preceded by a 50-Hz flicker, the participants’ rating of the neutral target went up to 55% positive compared to 48% in the no-flicker condition. The implicit affective measures revealed the predicted shifts in approach and avoidance reaction times, even though participants did not perform an affective evaluation task, and were not aware of the gamma flicker or its affective value. Overall, reactions times to targets were shorter after 50 Hz flickers than after 25 or 0 Hz flickers, thus confirming the attentional facilitation first observed by Bauer et al. (2009). With respect to no-flicker (0 Hz), 25 Hz flicker did not have an effect on either attention or affect, despite being the only type of flicker that was detected above chance in Block 3. Even though gamma flicker was not detected by these participants, it thus modulated both attention and affect.
Additionally, the results of other studies could be re-interpreted along the lines of our hypotheses. Jung-Beeman et al. (2004), for instance, measured high-frequent, gamma band, neural activity with a scalp electroencephalogram while subjects were solving verbal problems. This activity, which was distributed over right anterior temporal positions, occurred 300 ms prior to the moment of sudden insight. The authors called these moments Aha! experiences, which stand in contrast to the slower solutions of more systematic search strategies. We think that the gamma burst in this study is not so much associated with the process of gaining insight, but rather with the positive affect evoked by the sudden shift from difficult to smooth processing. According to affective monitoring (see also Sroufe and Waters, 1976), the sudden insight leads to strong positive affect, similar to when, for instance, the punch line of a joke “breaks through.” In terms of our network model, the quick resolution of competition evokes Rpo activation, which then gets involved in a push–pull process with the Vpo node resulting in a gamma oscillation.
The absence of negative priming by 25 Hz flicker in Heerebout’s (2011) experiment may indicate that the postulated lower-frequency negative oscillations lie in another frequency band. In this domain, however, even fewer studies are available than with gamma oscillations. We know of only one study that suggests an association of lower-frequency theta oscillations (4–8 Hz) with negative affect. Using magnetoencephalography, Maratos et al. (2009) demonstrated differences in theta activity in the amygdala when either blurry, containing only low spatial frequencies, fear faces or blurry neutral faces were presented. This activity was accompanied by similar power differences in visual and frontal regions, which were apparently synchronized to the amygdala. Support for the identification of the negative lower-frequency oscillations with theta thus seems limited to only one study and certainly much more research into this issue would be needed. Indirect support for our association of affect with oscillations in different frequency bands comes from EEG research in memory. It has been claimed that memory familiarity is reflected in the gamma band and recollection in the theta band (Gruber et al., 2008). If recollection performance indeed results from laborious memory search, and familiarity from fluent memory activation, we indeed expect these to correspond to negative and positive affect, respectively (cf. Phaf and Rotteveel, 2005). In sum, we propose that affect first elicits oscillations in different frequency bands and that synchronization of these oscillations to other neural regions than where they have been evoked may have broader effects, possibly mediated by the release of affect-specific neuromodulators, on physiology and action tendencies.
Affect and Behavior Systems
Emotions are generally believed to evoke expressions, action tendencies, and specific modes of information processing (e.g., Frijda, 1986; Oatley and Johnson-Laird, 1987, 1995; Panksepp, 1998). In many theories, these emotional effects are organized in “hard-wired” behavior systems that, similar to affective monitoring, have an evolutionary origin. Throughout evolutionary history the organism was confronted with recurring situations, such as escaping from threats, finding mates, and caring for infants, for which adaptations have developed to optimize gene survival. The behavior systems can be activated by a repertoire of evolutionary-prepared stimuli and provide outline scripts of previously fitness-enhancing behavior. Panksepp, for instance, distinguishes FEAR, SEEKING, RAGE, PANIC, LUST, CARE, and PLAY systems, which are in capitals to emphasize that they concern emotion systems and not only conscious emotional feelings or single brain locations. Because the systems have been given emotion names, it seems pretty clear whether positive or negative affect is associated with a system, perhaps with the exception of the SEEKING system. Although originally devised by Panksepp (see also Berridge, 2003) as a positive emotion system, the appetitive goal-directed behavior elicited by this system can both serve to search for fitness-enhancing stimuli and to try to avoid fitness-reducing stimuli. Later Panksepp and Watt (2011) conceded that even the SEEKING system may be associated with negative affect if escape from a potential threat is sought. Oatley and Johnson-Laird(1987, 1995) also couple their basic emotions to evolutionary-prepared behavior systems, but they do not seem to conclude to a one-to-one relationship between these systems and specific emotions. Oatley and Jenkins(1996, see Table 3.2.), for instance, link the attachment behavior system to either happiness, love, distress, relief, anger, or anxiety, depending on the recurring theme being addressed. In our view, when ongoing activity matches the goals of the behavior system, for instance being with the attached person, positive affect is elicited. When however a mismatch occurs, for instance interruption of attachment, activation of the same behavior system may lead to negative affect. Affective monitoring thus imparts affective flavor on the behavior systems. We propose here that, although there may be a preferential association with one type of affect, this association is not exclusive for any behavior system.
There are both similarities and differences between affective monitoring and the behavior systems. Similar to the behavior systems, also affective monitoring probably has a repertoire of evolutionary-prepared stimuli that may trigger it directly and also a repertoire of evolutionary-prepared action tendencies, from which it may select a response. For affective monitoring we postulate that match–mismatch, which is a dynamical property of processing, serves as the only evolutionary-prepared signal, whereas the behavior systems may be directly activated by definite classes of evolutionary relevant stimuli, for instance intense stimuli, snakes, spiders, faces, babies, etc., as well as by stimuli learned during ontogenesis. The consequence of this assumption is that the latter stimuli have no immediate affective value, but only acquire one indirectly through affective monitoring of the activity in the behavior system they are associated with.
The large range of situations and stimuli that are able to evoke affect suggests that this process cannot be localized in a single or even a few neural regions. Affective monitoring is, moreover, not restricted to activity in these behavior systems, but applies to many more types of internal processing, which may not be explicitly related to emotion (e.g., Phaf and Rotteveel, 2009). In the proposed implementation, moreover, well-functioning networks have fewer active nodes and thus would be less easily detected with neuroimaging techniques than networks suffering from a lot of competition. A parallel finding can be observed in skill acquisition. As skills are acquired, global brain activation declines (Haier et al., 1992) and, moreover, shifts from cortical to intermediate cortical or subcortical regions (Saling and Phillips, 2007). If neuroimaging primarily detects areas with much competition, this implies that automaticity corresponds to a relative decrease in competition in cortical areas, but an increase at lower levels which are perhaps involved in task execution. The lower levels of activity in positive than in negative affect may also be one of the reasons why less effort has been spent to investigate positive than negative emotions, at least with neuroimaging tools (e.g., see Berridge, 2003).
Behavior systems may be subject to more limited localization than affective monitoring, but without explicit process models the contributions of different neural areas to any function may be very hard to determine with neuroimaging methods. Any neuroscientific approach to mental functioning should in our opinion emphasize procedural instead of localization aspects. The knowledge of where a particular function resides helps little in understanding how that function works. Perhaps the prime example of an integrative process model for a behavior system has been presented by LeDoux (1996) in his well-known dual-route model. LeDoux specified the connections in a larger fear network, and identified the amygdala as the hub in the wheel of fear processing.
Fear mostly has a negative valence, but components of fear, such as surprise and sudden changes may also figure in positive emotions. A smiling facial expression, for instance, may well be a fear expression signaling that the sender poses no threat. Smiling expressions have indeed been found to also activate the amygdala (e.g., Fitzgerald et al., 2006). The amygdala seems most involved in coding emotional intensity, arousal, or relevance with a small bias toward negativity (Cunningham et al., 2008). The amygdala through its connections with the brain stem, the hypothalamus, and cortical regions evokes the arousal, endocrine, behavioral, and information processing effects associated with fear. Heerebout and Phaf (2010a) suggested that the amygdala is mainly involved in the preparation an prioritization of both approach and avoidance tendencies. Frijda (1986) considered “control precedence” one of the defining features of emotion. Activation of the amygdala may be responsible for this precedence by boosting emotional processing elsewhere. In a similar vein as with biased competition (Phaf et al., 1990; Duncan, 1996), the amygdala pre-activates, either through direct connections or through neuromodulatory control, whole regions enabling swift selection of an appropriate action tendency. Subsequent monitoring of the resulting internal and information processing states would then determine what specific affect is elicited.
The amygdala example strengthens the case for a dissociation between the emotional behavior systems and affect. Patients with selective amygdala damage, for instance, show surprisingly few affective consequences (Damasio, 1999). In this view, moreover, defensive behavior can occur in the absence of negative affect. On the other hand, negative affect may enhance such defensive behavior, as in the startle reflex (e.g., Lang, 1995). Although both affective monitoring and the behavior systems contribute to full emotions, they do not exclusively map onto each other. In the next paragraph we will argue that their combined workings are not even a sufficient prerequisite for a conscious emotion.
The Construction of Conscious Emotions
At every moment in time, some of the many modules in the vast network will give off affective signals, which only rarely develop into conscious emotions. Distributed processing of specific features across the network will mostly result in contradictory positive and negative signals. If, however, one type of oscillation dominates, synchronization over large areas may occur, and an affective state with a particular valence may arise, which does not need to be conscious. Resonance of these oscillations in areas responsible for the production of neuromodulators, physiological reactions, and action tendencies may further extend the affective reactions. Synchronization in our view is not a sufficient prerequisite for the transformation of non-conscious affect into conscious emotion. According to constructivist theory (cf. Mandler, 1996; see also Barrett, 2009), consciousness arises from the building of an internal model in terms of sensori-motor representations (Phaf and Wolters, 1997; see also Hesslow, 2002). Only faint informational signals for these constructions, mainly through the observation of the person’s own behavior, are available from affect, because it has developed primarily in evolution to steer behavior into fitness-promoting directions without the intervention of slower conscious processes. An affective state may thus occur but remain non-conscious (cf. Berridge and Winkielman, 2003) due to the inability to incorporate it in an internal model.
Constructivism opposes the identity assumption, which according to Mandler (1996) “postulates that some preconscious state ‘breaks through’, ‘reaches’, ‘is admitted’, ‘crosses a threshold’, ‘enters’, into consciousness. A constructivist position states, in contrast, that most conscious states are constructed out of preconscious structures in response to the requirements of the moment” (p. 482). Whereas affect can be passively activated in our network model, conscious feelings need active constructions. These feelings not only include the perception of the person’s own bodily and physiological states, facial and postural expressions, and action tendencies, but also reconstructions of the events leading up to the emotion (cf. Parkinson and Manstead, 1992). They generally present an interpretation of the person’s situation, in which current concerns, emotional schemata, and also plans for the future are involved. The actively constructed compounds differ qualitatively from their passively activated non-conscious constituents, and therefore require extensions to the network models suggested above.
Biological and artificial networks are generally equipped with input and output modalities, so that they can react to external stimuli. Responses enacted externally in turn change the input, which may again result in adjustment of the output. Activations in the network thus accurately “model” the external situation by closing the external loop between output and input. What is needed for “imagining” situations that are not actually present in the environment? Phaf and Wolters, 1997, (see also Phaf et al., 1994) have suggested that the internalization of the output–input loop, through the installation by evolution of long-range recurrent connections between output and input modalities, presents the organism with a capacity to represent states that could potentially, but need not, exist in the outside environment. Most often, however, there is close correspondence between the internal and external loops, which run in parallel. Phaf and Wolters argued that the ability to construct internal models in terms of one’s own perceptions and actions, which may be disconnected from actual actions and perceptions, constitutes consciousness.
A similar internal-loop concept, which similar to the external loop operates sequentially, has been proposed in the renowned working-memory model of Baddeley (1986). He actually distinguished two such loops: the articulatory–auditory loop and the visuo-spatial sketchpad. Still other input–output modalities may have been coupled by evolution in this way, however. Given the large role bodily states are assumed to play in emotional feelings, also an internal loop between bodily output and input has likely developed. Damasio (1994), for instance, postulated “as–if” loops, in which internal models of bodily states could be maintained and manipulated. In the neo-Jamesian view of Damasio, both internal and actual bodily states can lie on the basis of the conscious feelings. Baddeley’s two types of working memory thus probably need to be supplemented by a third, somato-sensory, working memory, to accommodate theories on conscious emotions (Phaf and Wolters, 1997). We do not agree with Damasio that representations in somato-sensory working memory are solely responsible for feelings, but think that constructions in all three types of working memory may contribute (Phaf and Wolters, 1997). Every type possesses long-range recurrent connections between specific input–output modalities around a central network. In this network the working memories interact and transformations from one modality to the other take place. Processing in this network is also responsible for the combination and elaboration of representations that are simultaneously maintained in working memory. If, for instance, your way is blocked by another person, you may construct an internal model of not being able to continue walking in somato-sensory working memory, direct your visual attention and plan toward a possible passage in visuo-spatial working memory, and finally construct an angry appraisal in articulatory–auditory working memory by verbally reasoning that the person is responsible for the obstruction.
In this paper on the elicitation of non-conscious affect, we will not further elaborate upon constructivist theories of consciousness, but instead present an intriguing example of a qualitative dissociation between conscious and non-conscious processing. The Jacoby and Whitehouse (1989) paradigm compares two conditions in which the fluency resulting from a matching word is either incorporated in the conscious reconstruction of the memory for that word (i.e., the aware condition) or not (i.e., the unaware condition). In the classical list-learning setup, a list of words is first studied and a forced-choice recognition test on old, previously studied, words and new words is later administered. Test words are preceded by either matching (i.e., identical) context words, non-matching context words, or a meaningless string of letters. The level of consciousness is manipulated by presenting the context word either for a short, suboptimal, or a longer, optimal, duration between a premask and a postmask.
Matching words raise processing fluency of the test word on a number of different features, such as the visual and auditory word forms, and help settle the initial competition set up by the instruction to decide whether the test word is old or not. Non-matching context words on the other hand elicit competition with the test word on these features. Recognition requires the reconstruction of the memory status of the word, in which also influences at the time of testing (e.g., of the prime) may be incorporated. The higher processing fluency at test is involved in this reconstruction as a higher likelihood of the word being presented at study. The more difficult processing at test due to non-matching context words is interpreted as a higher likelihood of novelty. This is exactly the pattern of results Jacoby and Whitehouse obtained, but only in unaware conditions. Matching words increased correct recognition of old, actually studied, words and false recognition of new words relative to non-matching words, if context words were presented suboptimally. In aware conditions, the recognition advantage with matching words reversed into a recognition disadvantage. Still similar fluency priming should occur as in the unaware condition. With optimal priming, however, the matching context word is incorporated in the conscious experience of the test trial and identified as the probable source of the enhanced fluency. Non-matching words are similarly discounted as the source of conflict. The separation of the two words into two conscious experiences and the counteracting of the context words even reverses their influence. Discounting and source attribution effects, such as in affective priming (Murphy and Zajonc, 1993; Rotteveel et al., 2001) and mere exposure (Bornstein, 1989), are in our view representative for conditions, where primes are processed consciously.
Affective monitoring predicts that matching suboptimal primes also raise positive affect, which indeed was demonstrated with pictures by Reber et al. (1998; for a review of these and similar effects, see Fazendeiro et al., 2007). Affective monitoring, moreover, adds the further prediction that non-matching words not only elicit disfluency and conflict but also negative affect. The close correspondence between fluency/disfluency and positive/negative affect, at least at a non-conscious level, was shown in a study where we replaced the matching/non-matching context words with positive/negative context words in the Jacoby–Whitehouse paradigm (Phaf and Rotteveel, 2005). In suboptimal conditions, positive words led to more correct and false recognition than negative words, but the effect was only diluted but not reversed in optimal conditions. A second experiment in this study showed that negative affect can reduce familiarity as much as positive affect can increase it. Positive and negative affect were induced by covert instructions to contract facial zygomaticus and corrugator muscles, respectively. Relative to a neutral induction by juggling a pen with the fingers of the non-preferred hand, the positive condition showed an increase in correct and false recognition, whereas a decrease resulted from the negative condition. The absence of strong discounting or attribution effects due to conscious processing of the affective context words may well be explained by the fact that a link between affective words and familiarity was not obvious to the participants. The varying results in conscious conditions lead us to the conclusion that the most straightforward evidence for affective monitoring can be found when confounding influences of conscious constructions have been minimized.
Discussion
Three main types of arguments will probably be raised against affective monitoring.
(i) In many cases even strongly fluent processing does not elicit much positive affect, or may even induce boredom,
(ii) Exploration, or the seeking of novelty, motivates many human activities and is mostly evaluated positively,
(iii) Affect reflects personal meanings based on appraisal processes which compare situations to individual concerns and goals.
When is Fluency Neutral, or Even Negative?
The prime experimental paradigm in psychology supporting a fluency–positivity relationship is probably mere exposure (Kunst-Wilson and Zajonc, 1980), which entails that the “non-reinforced” presentation of a stimulus increases liking. Although mere exposure is by now well-established (Zajonc, 2001), many factors can moderate the effect. Shifts in preference ratings generally decrease with level of consciousness for the previous exposure (Bornstein, 1989). Even after suboptimal previous exposure, detailed, analytic, processing at test eliminates familiarity effects both on preference and recognition (Whittlesea and Price, 2001). In addition, when fluency is expected, not much exposure effects can be found on feelings of familiarity (e.g., Whittlesea and Williams, 1998) or on positive feelings (Willems and Van der Linden, 2006). A high level of fluency due to many repetitions may even induce boredom and negative affect (Bornstein et al., 1990).
The absence of fluency effects when the fluency is expected (e.g., Whittlesea and Williams, 1998; Willems and Van der Linden, 2006; see also Hansen et al., 2008) is explained in a straightforward manner within the affective monitoring framework. In our network model (see Figure 4), positive activation always requires some initial competition, which may not be there when the expectation matches the actual outcome. Tentatively, a similar account may apply to the recent findings of de Vries et al. (2010; cf. Freitas et al., 2005) that a happy mood diminishes the fluency effects in the “beauty-in-averages” phenomenon. The positive mood may set up expectations of high fluency, which prevents the elicitation of any affect.
The observation that fluency is not always positive has led to a split into “cognitive” and affective interpretations of mere exposure. The cognitive account assumes that mere exposure is a non-affective implicit memory effect (e.g., Seamon et al., 1984; Mandler et al., 1987; Whittlesea and Price, 2001), in which the increased fluency is attributed to a higher liking of the stimulus, particularly when its source is not consciously recognized. Zajonc (1980, 2001), however, postulates primacy for affect and holds that exposure evokes genuine positive affect. Evidence showing that mere exposure also has diffuse mood effects (Monahan et al., 2000), and that mere exposure is accompanied by contractions of the facial zygomaticus muscle (Harmon-Jones and Allen, 2001; see also Winkielman and Cacioppo, 2001), seems to have clearly tipped the balance in favor of Zajonc’ position.
Affective monitoring integrates affective and cognitive processing by arguing that the processing of familiarity/novelty coincides with affective processing at the earliest stages of processing (cf. Phaf and Rotteveel, 2005). It conforms to Zajonc’ idea that genuine affect is evoked by the repeated exposure. In contrast to affective primacy, however, this affect cannot effectively be distinguished from cognitive processing. The illusory distinction between affect and cognition only arises when conscious experiences are probed (cf. Rotteveel and Phaf, 2007). Whether the previous exposure is incorporated in the construction of the conscious feeling depends on whether exposure can be recollected consciously. If it can, the positive affect will mostly be discounted and attributed to this exposure. We argue here that attribution and discounting are expressions of conscious processing, and that the most direct affective influences can be found when conscious recollection is impeded.
Boredom, which represents a form of negative affect, clearly covers larger time spans than, for instance, with subliminal presentation and requires many repetitions. Under those conditions mere-exposure is indeed limited by an effect of boredom (cf. Berlyne, 1970). In Experiment 2 of Bornstein et al. (1990), for instance, affect ratings increased after the first five presentations, but clearly decreased after 10 and more presentations. In their Experiment 1, moreover, only low boredom–prone individuals showed the mere-exposure effect. Similar individual differences have been obtained by Hansen and Topolinski (2011) in the prototypicality effect, which entails a preference for prototypes over exemplars. Participants with an exploratory mindset in this experiment showed a reduced preference for prototypes and an increased preference for novel exemplars.
The role of individual differences in these fluency-affect findings provides important clues for an explanation in terms of evolutionary-prepared behavior systems, which function in parallel to affective monitoring. Many theoreticians assume an exploratory behavior system (e.g., the PLAY system, Panksepp, 1998), which allows the organism to refine its abilities to deal with the physical and social environment. With only a few repetitions, or with subliminal presentation, positive affect is evoked by fluent stimuli, even in high-boredom, or very exploratory, individuals. After many repetitions the positive affect habituates, however, because it is fully expected and no initial competition occurs anymore. In these monotonous conditions the exploratory system will take over, particularly when the exploratory system is highly active, as it may be in high-boredom and highly exploratory persons. The very fluent stimuli are then clearly in conflict with these exploratory tendencies, and negative affect will arise. In sum, the affective monitoring account postulates a fluency–positivity link primarily in non-conscious conditions and only after there has been some initial competition. In the long run, fluency effects may peter out because they are no longer unexpected and the affective monitoring of activity in an exploratory behavior system may turn fluency into a negative property.
Can Novelty be Positive?
Also in our view novelty can evoke positive affect, but this does not constitute the most direct reaction. In the experiments of Berlyne (1970), for instance, novel stimuli were evaluated more positively than familiar stimuli, when they were presented in sequences with very long, supraliminal, durations (9 s), sometimes after extensive prefamiliarization. Novel stimuli may have constituted a welcome relief from boredom and may have satisfied exploratory tendencies evoked by the repetitive task. Humans often also actively seek novelty to avoid boredom. Biederman and Vessel (2006) have posited that pleasure arises from “infovore” behavior which maximizes the rate at which people acquire knowledge under conditions where there may be no immediate need for the information. Counter to affective monitoring, but also invoking a connectionist novelty detection mechanism similar to Murre et al. (1992; see also Phaf et al., 2001), they assumed that novelty corresponds to positive affect and familiarity to boredom and negative affect. They moreover noted (see Yue et al., 2007) that preferred stimuli resulted in larger activations than neutral stimuli in, particularly the posterior portion of, the parahippocampal cortex, a region rich in opioid receptors.
There is more reason to believe that the parahippocampal cortex is linked to familiarity than to novelty. Daselaar et al. (2006; see also Strange et al., 1999; Köhler et al., 2002 have, for instance, found that activation in posterior parts of the MTL, which includes the parahippocampal cortex, increases with the true oldness of the stimulus, irrespective of whether the participant consciously recollects the stimulus. Novelty on the other hand was coded by anterior parts of the MTL, which inhibited the posterior parts in a unidirectional fashion. The neuroimaging results of Yue et al. (2007) thus seem to support a link between preference and familiarity, rather than one between preference and novelty.
The hypothesis of Biederman and Vessel (2006) on the operation of the opioid neurotransmitters (i.e., endorphins) also points toward a link between smooth processing and positive affect rather than between novelty and positive affect. According to Biederman and Vessel, the brain is immersed in an inhibitory “GABA-bath.” The release of the GABA neurotransmitter is in turn inhibited by the opioids, so that competition decreases and activation can flow more smoothly through the network. In that case, however, also competitive learning is reduced, so that there is less opportunity to develop focused representations, and stimuli would remain relatively novel. If opioids were released due to the processing of novel stimuli, this would seriously undermine the (competitive) learning capability and would hamper the development of sparse representations for these novel stimuli. If, however, opioids are released when competition has subsided, as we propose, these neurotransmitters would lower mutual inhibition even further and foster attentional flexibility. The seeking of novelty thus only takes place after longer periods of smooth processing. Positive affect may then be elicited by novelty because it matches the exploratory tendencies set up by this particular behavior system.
Affective Monitoring and Appraisal
Appraisal is the central concept of emotion elicitation in classical emotion psychology (Frijda, 1986; Lazarus, 1991). It represents the individual’s evaluation of a current or future event with respect to personal well-being. At first sight, there is a close connection between primary appraisal and affective monitoring. Lazarus and Folkman (1984) distinguished primary appraisal, secondary appraisal, and reappraisal. The former refers to whether the situation has relevance for personal well-being or, to put it in another way, “Am I in trouble or being benefited, now or in the future, and in what way?” (Lazarus and Folkman, 1984, p. 31). Congruence between a real or imagined situation and a personal concern evokes positive affect, whereas incongruence leads to negative affect. Secondary appraisal differentiates between different emotions of the same affect (see also Parkinson and Manstead, 1992) and determines “What if anything can be done about it?” (p. 31). Finally, reappraisal is the renewed evaluation of the original encounter in the light of the success or failure of the coping strategies resulting from secondary appraisal.
The concept of affective monitoring owes much to Frijda (2007): “Pleasure is the positive outcome of constantly monitoring one’s functioning” (p. 82), and “Emotions monitor and regulate progress toward concern satisfaction. They signal when goal shifts are needed or urgent” (p. 127). It is tempting to consider primary appraisal a high level, conceptual, form of affective monitoring. For appraisal it is necessary that the concerns are activated first, probably in the same manner as other meanings and concepts can be set up for further processing. In the connectionist language, concerns are represented as active nodes or nodes assemblies. Environmental situations or events result in stimulus input that is split up along partly the same representational components as the concerns. The presence, or alternatively the quick resolution, of competition between these nodes elicits negative or positive affect, respectively. We argue here, however, that affective monitoring is not limited to the comparison with personal goals and concerns, but is more general covering all kinds of bodily and cognitive functioning.
Many appraisal theoreticians (e.g., Lazarus, 1991) would also consider the non-conscious instances of affective monitoring a form of primary appraisal. Many of these, however, do not clearly involve personal concerns and there is no obvious connection to personal well-being, such as in the matching of attentional direction (Phaf and Rotteveel, 2009), or in mere exposure (Zajonc, 2001). An arrow to the right does not increase personal well-being in left-to-right readers, but represents an impersonal byproduct of the evolutionary basic process of affective monitoring. Appraisal is about the pursuit of personal, and often short-term, well-being, whereas affective monitoring results from the optimization of gene survival. Evolutionary development leads to behavior systems or generic mechanisms that have a net adaptive value, but that may also result in behavior without obvious fitness benefits (cf. “spandrels”; Gould and Lewontin, 1979), or rarely even maladaptive behavior. The fact that all behavior has been shaped by evolution simply does not mean that all behavior is adaptive. Affect has evolved as a gross code for adaptive value, but in a similar manner not all positive affect signals specific benefits to the individual. At best, affective monitoring and appraisal represent partly overlapping processes, particularly when conceptual concerns are monitored, but they are certainly not identical.
The nature of empirical appraisal research suggests an altogether different conceptualization of appraisal. This research (e.g., Smith and Ellsworth, 1985; Shaver et al., 1987; Frijda et al., 1989) has almost exclusively relied on subjective reports of conscious emotional contents. The role of consciousness in appraisal has sparked an acrimonious debate between Zajonc (1980) and Lazarus (1981, 1982). Zajonc held that non-conscious affect preceded conscious cognition whereas, according to Lazarus, the two are completely intertwined. Although this debate may have gotten bogged down in semantics and variable definitions, particularly of “cognition,” there seems to be at least one substantial issue in the debate. Lazarus did not deny, and even emphasized, non-conscious appraisal, but did not think that it mattered much whether appraisal was conscious or non-conscious. Zajonc held open the possibility of dissociations between early, non-conscious, and diffuse affect and later conscious emotions. The method of subjective report for finding out the structure of both conscious and non-conscious appraisal implies adherence to an identity position (e.g., Mandler, 1996). Identity assumes, implicitly or explicitly, that conscious and non-conscious processes do not differ qualitatively, and that conscious report can thus be used to probe the fundamental structure of non-conscious appraisal. In all fairness, Lazarus (1995) later acknowledged this paradox in appraisal research and even called it a “vexing” problem. In our opinion (see also Rotteveel and Phaf, 2007), the qualitative dissociation between non-conscious and conscious emotional processes was the real issue in the Zajonc–Lazarus debate.
If one wants to hold that all emotion involves appraisal in the face of the elicitation of at least a diffuse form of emotion (i.e., affect) by a different mechanism, one has to concede that appraisal can occur after the initial causation of an emotion. Increasingly, appraisal has indeed been considered a consequence rather than a cause of emotion (see Parkinson and Manstead, 1992; Frijda, 1993; Frijda and Zeelenberg, 2001). A prime feature of non-conscious processes, moreover, is that they may not be accurately represented in the conscious contents (e.g., Nisbett and Wilson, 1977). The subjective reports of emotional appraisals are, therefore, likely post hoc reconstructions, possibly fitting commonsense causal schemata, which may or may not reflect the underlying emotional processing. This, of course agrees very well with constructionist accounts of consciousness (e.g., Mandler, 1996).
Phaf and Wolters (1997) have argued that constructions are responsible for all conscious contents by combining representations that are temporarily activated in working memory. We distinguished three types of working memory, somato-sensory, visuo-spatial, and auditory–articulatory working memory, which are probably all involved in the conscious experience of emotion. Verbal reports of appraisal will, however, mainly be constructed in the auditory–articulatory type. Not only representations of underlying affective processes will be active but also general schemata, demand characteristics, current concerns, and future plans may be involved in the construction. The schema “that there must be a reason for my behavior” will induce a reconstruction in terms of a comparison of the emotional situation with my current goals and concerns. If the emotional event was some time ago there is even a chance that the appraisal will be constructed with my present goals instead of with my goals at the time of the event (Levine, 1997).
For theoretical clarity, it would be best to fully separate affective monitoring from appraisal by reserving the latter term for the conscious constructions of emotional experiences in working memory. Appraisal thus coincides with the creation of conscious emotional contents in verbal working memory. Appraisal is no longer the elicitor of affect, but the constructor of emotional consciousness. In our view, affect is elicited and modulates cognition and behavior predominantly in a non-conscious manner. If this affect is elaborated into a conscious emotion, the resulting experience runs the risk of being inaccurate with respect to its non-conscious sources, even to the point that, very similar to the more readily investigated false memories (e.g., Loftus, 1997), false emotions bearing no relation to the non-conscious affect may occur.
Conclusion
Building on earlier modeling and experimental work, we presented a mechanistic view on how affect is elicited, how it is represented, and how it modulates cognition and behavior. Elements of this view, such as competition and oscillations, emerged from evolutionary simulation, but others (e.g., match–mismatch detection) extended upon design choices that were made in earlier models. Together they are consistent with a large range of experimental findings, of which only a small selection could be discussed in the present paper.
Core tenets of the affective monitoring view are:
1. Affective monitoring is an evolutionary-early mechanism working at the same basic level as the formation of representations, attentional selection, and memory storage.
2. The constituent features of a representation are monitored locally, provided they have an active counterpart against which they can be matched.
3. If representations addressing the same module evoke much competition, negative affect will arise.
4. If competition can be solved quickly, negative affect will decay quickly and positive affect will ensue.
5. If fluent processing is not preceded by initial competition, neither positive nor negative affect will arise.
6. In parallel to affective monitoring separate mechanisms have evolved linking specific evolutionary-prepared stimulus repertoires to specific evolutionary-prepared action repertoires, such as in defensive, exploratory, attachment, and SEEKING (or wanting) behavior systems. These behavior systems are predominantly, but not exclusively, linked to one type of affect.
7. Positive affect locally induces gamma oscillations, whereas negative affect probably corresponds to more incoherent activity in the lower-frequency theta band.
8. When there is sufficient oscillatory activity, particularly of gamma, synchronization across different neural regions enables more global affective states.
9. Oscillatory activity in either band is associated with specific types of attentional modulation, neuromodulatory activation, action tendencies, and facial muscle activation.
10. Affect is primarily non-conscious but may be elaborated by constructive processes into conscious emotions, encompassing positive or negative feelings.
11. Constructive processes also entail regulatory and attributional processes, which may dilute or even invert the non-conscious match-positive and mismatch-negative relations.
Considering positive and negative valence basic, irreducible, entities, such as reward and punishment or feeling good and bad, has resulted in an artificial distinction between affective and cognitive (i.e., non-affective) processes. In the affective monitoring point of view all processing is continuously monitored and accompanied by a mixture of positive and negative affect. Only when one type of affect dominates and the oscillations resonate throughout the network and an internal model (Phaf and Wolters, 1997; Hesslow, 2002) that is consistent with only one type of affect, is built, will a clear-cut affective experience arise. All processing, therefore, has affective qualities, but only when the affect is involved in conscious constructions are the processes experienced as affective.
Conflict of Interest Statement
The authors declare that the research was conducted in the absence of any commercial or financial relationships that could be construed as a potential conflict of interest.
Acknowledgments
We are grateful to Bram T. Heerebout, A. E. Yoram Tap, and William H. Thompson for their help in various stages of this work.
References
Armony, J. L., Servan-Schreiber, D., Cohen, J. D., and LeDoux, J. E. (1995). An anatomically constrained neural network model of fear conditioning. Behav. Neurosci. 109, 246–257.
Astikainen, P., and Hietanen, J. K. (2009). Event-related potentials to task-irrelevant changes in facial expressions. Behav. Brain Funct. 5, 30.
Barrett, L. F. (2009). Variety is the spice of life: a psychological construction approach to understanding variability in emotion. Cogn. Emot. 23, 1284–1306.
Bauer, F., Cheadle, S. W., Parton, A., Müller, H. J., and Usher, M. (2009). Gamma flicker triggers attentional selection without awareness. Proc. Natl. Acad. Sci. U.S.A. 106, 1666–1671.
Beilock, S. L., and Holt, L. E. (2007). Embodied preference judgments: can likeability be driven by the motor system? Psychol. Sci. 18, 51–57.
Berridge, K. C., and Winkielman, P. (2003). What is an unconscious emotion? The case for unconscious ‘liking’. Cogn. Emot. 17, 181–211.
Blanchette, I. (2006). Snakes, spiders, guns, and syringes: how specific are evolutionary constraints on the detection of threatening stimuli? Q. J. Exp. Psychol. 59, 1394–1414.
Bornstein, R. F. (1989). Exposure and affect: overview and meta-analysis of research, 1968–1987. Psychol. Bull. 106, 265–289.
Bornstein, R. F., Kale, A. R., and Cornell, K. R. (1990). Boredom as a limiting condition on the mere exposure effect. J. Pers. Soc. Psychol. 58, 791–800.
Buzsáki, G., and Draguhn, A. (2004). Neuronal oscillations in cortical networks. Science 304, 1926–1929.
Cannon, P. R., Hayes, A. E., and Tipper, S. P. (2010). Sensorimotor fluency influences affect: evidence from electromyography. Cogn. Emot. 24, 681–691.
Chalmers, D. J. (2004). “How can we construct a science of consciousness?” in The Cognitive Neurosciences III, ed. M. S. Gazzaniga, (Cambridge, MA: MIT Press), 1111–1119.
Cunningham, W. A., Van Bavel, J. J., and Johnsen, I. R. (2008). Affective flexibility: evaluative processing goals shape amygdala activity. Psychol. Sci. 19, 152–160.
Daselaar, S. M., Fleck, M. S., Prince, S. E., and Cabeza, R. (2006). The medial temporal lobe distinguishes old from new independently of consciousness. J. Neurosci. 26, 5835–5839.
de Vries, M., Holland, R. W., Chenier, T., Starr, M. J., and Winkielman, P. (2010). Happiness cools the warm glow of familiarity: psychophysiological evidence that mood modulates the familiarity-affect link. Psychol. Sci. 21, 321–328.
den Dulk, P., Heerebout, B. T., and Phaf, R. H. (2003). A computational study into the evolution of dual-route dynamics for affective processing. J. Cogn. Neurosci. 15, 194–208.
Dreisbach, G., and Goschke, T. (2004). How positive affect modulates cognitive control: reduced perseveration at the cost of increased distractibility. J. Exp. Psychol. Learn. Mem. Cogn. 30, 343–353.
Duncan, J. (1996). “Cooperating brain systems in selective perception and action,” in Attention and Performance, Vol. XVI. Information integration in perception and communication, eds T. Inui and J. L. McClelland (Cambridge, MA: MIT Press), 549–578.
Fazendeiro, T., Chenier, T., and Winkielman, P. (2007). “How dynamics of thinking create affective and cognitive feelings: psychology and neuroscience of the connection between fluency, liking, and memory,” in Social Neuroscience, eds E. Harmon-Jones and P. Winkielman (New York: The Guilford Press), 271–289.
Fitzgerald, D. A., Angstadt, M., Jelsone, L. M., Nathan, P. J., and Phan, K. L. (2006). Beyond threat: amygdala reactivity across multiple expressions of facial affect. Neuroimage 30, 1441–1448.
Fredrickson, B. L. (2004). The broaden-and-build theory of positive emotions. Philos. Trans. R. Soc. Lond. B Biol. Sci. 359, 1367–1377.
Freitas, A. L., Azizian, A., Travers, S., and Berry, S. A. (2005). The evaluative connotation of processing fluency: inherently positive or moderated by motivational context? J. Exp. Soc. Psychol. 41, 636–644.
Frijda, N. H., Kuipers, P., and Ter Schure, E. (1989). Relations among emotion, appraisal, and emotional action readiness. J. Pers. Soc. Psychol. 57, 212–228.
Frijda, N. H., and Zeelenberg, M. (2001). “Appraisal: what is the dependent?” in Appraisal Processes in Emotion: Theory, Methods, Research, eds K. R. Scherer, A. Schorr, and T. Johnstone (Oxford: Oxford University Press), 141–155.
Gable, P. A., and Harmon-Jones, E. (2010). The motivational dimensional model of affect: implications for breadth of attention, memory, and cognitive categorization. Cogn. Emot. 24, 322–337.
Gould, S. J., and Lewontin, R. C. (1979). The spandrels of San Marcos and the Panglossian program: a critique of the adaptationist programme. Proc. R. Soc. Lond. B Biol. Sci. 205, 581–598.
Graf, P., and Mandler, G. (1984). Activation makes words more accessible, but not necessarily more retrievable. J. Verbal Learn. Verbal Behav. 23, 553–568.
Gregoriou, G. G., Gotts, S. J., Zhou, H., and Desimone, R. (2009). High-frequency, long-range coupling between prefrontal and visual cortex during attention. Science 234, 1207–1210.
Gruber, T., Tsivilis, D., Giabbiconi, C.-M., and Müller, M. M. (2008). Induced electroencephalogram oscillations during source memory: familiarity is reflected in the gamma band, recollection in the theta band. J. Cogn. Neurosci. 20, 1043–1053.
Haier, R. J., Siegel, B. V. Jr., MacLachlan, A., Soderling, E., Lottenberg, S., and Buchsbaum, M. S. (1992). Regional glucose metabolic changes after learning a complex visuospatial/motor task: a positron emission tomographic study. Brain Res. 570, 134–143.
Hansen, J., Dechêne, A., and Wänke, M. (2008). Discrepant fluency increases subjective truth. J. Exp. Soc. Psychol. 44, 687–691.
Hansen, J., and Topolinski, S. (2011). An exploratory mindset reduces preference for prototypes and increases preference for novel exemplars. Cogn. Emot. 25, 709–716.
Harmon-Jones, E., and Allen, J. J. B. (2001). The role of affect in the mere exposure effect: evidence from psychophysiological and individual differences approaches. Pers. Soc. Psychol. Bull. 27, 889–898.
Heerebout, B. T. (2011). Getting Emotional with Evolutionary Simulations: The Origin of Affective Processing in Artificial Neural Networks. Saarbrücken: LAP Lambert Academic Publishing.
Heerebout, B. T., and Phaf, R. H. (2010a). Emergent oscillations in evolutionary simulations. J. Cogn. Neurosci. 22, 807–823.
Heerebout, B. T., and Phaf, R. H. (2010b). Good vibrations switch attention: an affective function for network oscillations in evolutionary simulations. Cogn. Affect. Behav. Neurosci. 10, 217–229.
Herrmann, C. S. (2001). Human EEG responses to 1–100 Hz flicker: resonance phenomena in visual cortex and their potential correlation to cognitive phenomena. Exp. Brain Res. 137, 346–353.
Hesslow, G. (2002). Conscious thought as simulation of behaviour and perception. Trends Cogn. Sci. (Regul. Ed.) 6, 242–247.
Holland, J. H. (1975). Adaptation in Natural and Artificial Systems. Ann Arbor: University of Michigan Press.
Hubel, D. H., and Wiesel, T. N. (1977). Functional architecture of macaque monkey visual cortex. Proc. R. Soc. Lond. B Biol. Sci. 198, 1–59.
Innocenti, G. M., and Price, D. J. (2005). Exuberance in the development of cortical networks. Nat. Rev. Neurosci. 6, 955–965.
Jacoby, L. L., and Whitehouse, K. (1989). An illusion of memory: false recognition influenced by unconscious perception. J. Exp. Psychol. Gen. 118, 126–135.
Jung-Beeman, M., Bowden, E. M., Haberman, J., Frymiare, J. L., Arambel-Liu, S., Greenblatt, R., Reber, P. J., and Kounios, J. (2004). Neural activity when people solve verbal problems with insight. PLoS Biol. 2, 500–510. doi:10.1371/journal.pbio.0020097
Köhler, S., Crane, J., and Milner, B. (2002). Differential contributions of the parahippocampal place area and the anterior hippocampus to human memory for scenes. Hippocampus 12, 718–723.
Kunst-Wilson, W. R., and Zajonc, R. B. (1980). Affective discrimination of stimuli that cannot be recognized. Science 207, 557–558.
Lang, P. J. (1995). The emotion probe: studies of motivation and attention. Am. Psychol. 50, 372–385.
Lazarus, R. S. (1981). A cognitivist’s reply to Zajonc on emotion and cognition. Am. Psychol. 36, 222–223.
Lazarus, R. S. (1982). Thoughts on the relations between emotion and cognition. Am. Psychol. 37, 1019–1024.
Lazarus, R. S. (1995). Vexing research problems inherent in cognitive-mediational theories of emotion – and some solutions. Psychol. Inq. 6, 183–196.
Mandler, G. (1996). “Consciousness redux,” in Scientific Approaches to Consciousness: The Twenty-Fifth Carnegie Symposium on Cognition, eds J. C. Cohen and J. W. Schooler (Hillsdale, NJ: Erlbaum), 479–498.
Mandler, G., Nakamura, Y., and Van Zandt, B. J. S. (1987). Nonspecific effects of exposure on stimuli that cannot be recognized. J. Exp. Psychol. 13, 646–648.
Maratos, F., Mogg, K., Bradley, B., Rippon, G., and Senior, C. (2009). Coarse threat images reveal theta oscillations in the amygdala: a magnetoencephalography study. Cogn. Affect. Behav. Neurosci. 9, 133–143.
Monahan, J. L., Murphy, S. T., and Zajonc, R. B. (2000). Subliminal mere exposure: specific, general, and diffuse effects. Psychol. Sci. 11, 462–466.
Murphy, S. T., and Zajonc, R. B. (1993). Affect, cognition and awareness: affective priming with optimal and suboptimal stimulus exposures. J. Pers. Soc. Psychol. 64, 723–739.
Murre, J. M. J. (1996). TraceLink: a model of amnesia and consolidation of memory. Hippocampus 6, 674–684.
Murre, J. M. J., Phaf, R. H., and Wolters, G. (1992). CALM: categorizing and learning module. Neural Netw. 5, 55–82.
Nisbett, R. E., and Wilson, T. D. (1977). Telling more than we can know: verbal reports on mental processes. Psychol. Rev. 84, 231–259.
Oatley, K., and Johnson-Laird, P. N. (1987). Towards a cognitive theory of emotion. Cogn. Emot. 1, 29–50.
Oatley, K., and Johnson-Laird, P. N. (1995). “The communicative theory of emotions: empirical tests, mental models, and implications for social interaction,” in Striving and Feeling: Interactions Among Goals, Affect, and Self-Regulation, eds L. L. Martin and A. Tesser (Mahwah, NJ: Erlbaum), 363–380.
Öhman, A. (1986). Face the beast and fear the face: animal and social fears as prototypes for evolutionary analyses of emotion. Psychophysiology 23, 123–145.
Panksepp, J. (1998). Affective Neuroscience: The Foundations of Human and Animal Emotions. New York: Oxford University Press.
Panksepp, J., and Watt, D. (2011). What is basic about basic emotions? Lasting lessons from affective neuroscience. Emot. Rev. 3, 387–396.
Parkinson, B., and Manstead, A. S. R. (1992). “Appraisal as a cause of emotion,” in Emotion: Review of Personality and Social Psychology, Vol. 13, ed. M. S. Clark (London: Sage), 122–149.
Phaf, R. H. (1994). Learning in Natural and Connectionist Systems. Dordrecht: Kluwer Academic Publishers.
Phaf, R. H., den Dulk, P., Tijsseling, A., and Lebert, E. (2001). Novelty-dependent learning and topological mapping. Conn. Sci. 13, 293–321.
Phaf, R. H., and Kan, K. J. (2007). The automaticity of emotional Stroop: a meta-analysis. J. Behav. Ther. Exp. Psychiatry. 38, 184–199.
Phaf, R. H., Mul, N. M., and Wolters, G. (1994). “A connectionist view on dissociations,” in Attention and Performance XV, Conscious and Nonconscious Information Processing, eds C. Umiltà and M. Moscovitch (Cambridge, MA: MIT Press), 725–751.
Phaf, R. H., and Rotteveel, M. (2005). Affective modulation of recognition bias. Emotion 5, 309–318.
Phaf, R. H., and Rotteveel, M. (2009). Looking at the bright side: the affective monitoring of direction. Emotion 9, 729–733.
Phaf, R. H., Van der Heijden, A. H. C., and Hudson, P. T. W. (1990). SLAM: a connectionist model for attention in visual selection tasks. Cogn. Psychol. 22, 273–341.
Phaf, R. H., and Wolters, G. (1997). A constructivist and connectionist view on conscious and nonconscious processes. Philos. Psychol. 10, 287–307.
Raymond, J. E., Fenske, M. J., and Tavassoli, N. T. (2003). Selective attention determines emotional responses to novel visual stimuli. Psychol. Sci. 14, 537–542.
Reber, R., Winkielman, P., and Schwarz, N. (1998). Effects of perceptual fluency on affective judgments. Psychol. Sci. 9, 45–48.
Rotteveel, M., de Groot, P., Geutskens, A., and Phaf, R. H. (2001). Stronger suboptimal than optimal affective priming? Emotion 1, 348–364.
Rotteveel, M., and Phaf, R. H. (2004). Automatic affective evaluation does not automatically predispose for arm flexion and extension. Emotion 4, 156–172.
Rotteveel, M., and Phaf, R. H. (2007). Mere exposure in reverse: mood and motion modulate memory bias. Cogn. Emot. 21, 1323–1346.
Rumelhart, D. E., and Zipser, D. (1985). Feature discovery by competitive learning. Cogn. Sci. 9, 75–112.
Saling, L. L., and Phillips, J. G. (2007). Automatic behaviour: efficient not mindless. Brain Res. Bull. 73, 1–20.
Schyns, P. G., Petro, L. S., and Smith, M. L. (2007). Dynamics of visual information integration in the brain for categorizing facial expressions. Curr. Biol. 17, 1580–1585.
Seamon, J. G., Marsh, R. L., and Brody, N. (1984). Critical importance of exposure duration for affective discrimination of stimuli that are not recognized. J. Exp. Psychol. Learn. Mem. Cogn. 10, 465–469.
Shaver, P., Schwartz, J., Kirson, D., and O’Connor, C. (1987). Emotion knowledge: further exploration of a prototype approach. J. Pers. Soc. Psychol. 52, 1061–1086.
Smith, C. A., and Ellsworth, P. C. (1985). Patterns of cognitive appraisal in emotion. J. Pers. Soc. Psychol. 48, 813–838.
Sohal, V. S., Zhang, F., Yizhar, O., and Deisseroth, K. (2009). Parvalbumin neurons and gamma rhythms synergistically enhance cortical circuit performance. Nature 459, 698–702.
Sroufe, L. A., and Waters, E. (1976). The ontogenesis of smiling and laughter: a perspective on the organization of development in infancy. Psychol. Rev. 83, 173–189.
Strange, B. A., Fletcher, P. C., Henson, R. N. A., Friston, K. J., and Dolan, R. J. (1999). Segregating the functions of human hippocampus. Proc. Natl. Acad. Sci. U.S.A. 96, 4034–4039.
Straube, T., Mothes-Lasch, M., and Miltner, W. H. R. (2011). Neural mechanisms of the automatic processing of emotional information from faces and voices. Br. J. Psychol. 102, 830–848.
Topolinski, S., Likowski, K. U., Weyers, P., and Strack, F. (2009). The face of fluency: semantic coherence automatically elicits a specific pattern of facial muscle reactions. Cogn. Emot. 23, 260–271.
Tsai, H., Zhang, F., Adamantidis, A., Stuber, G. D., Bonci, A., de Lecea, L., and Deisseroth, K. (2009). Phasic firing in dopaminergic neurons is sufficient for behavioral conditioning. Science 324, 1080–1084.
van Wouwe, N. C., Band, G. P. H., and Ridderinkhof, K. R. (2011). Positive affect modulates flexibility and evaluative control. J. Cogn. Neurosci. 23, 524–539.
von der Malsburg, C. (1973). Self-organization of orientation sensitive cells in the striate cortex. Kybernetik 14, 85–100.
Vuilleumier, P., Armony, J. L., Driver, J., and Dolan, R. J. (2003). Distinct spatial frequency sensitivities for processing faces and emotional expressions. Nat. Neurosci. 6, 624–631.
Whittlesea, B. W. A., and Price, J. R. (2001). Implicit/explicit memory versus analytic/nonanalytic processing: rethinking the mere exposure effect. Mem. Cognit. 29, 234–246.
Whittlesea, B. W. A., and Williams, L. D. (1998). Why do strangers feel familiar, but friends don’t? A discrepancy-attribution account of feelings of familiarity. Acta Psychol. 98, 141–165.
Willems, S., and Van der Linden, M. (2006). Mere exposure effect: a consequence of direct and indirect fluency-preference links. Conscious. Cogn. 15, 323–341.
Williams, L. M., and Gordon, E. (2007). Dynamic organization of the emotional brain: responsivity, stability, and instability. Neuroscientist 13, 349–370.
Williams, L. M., Palmer, D., Liddell, B. J., Song, L., and Gordon, E. (2006). The ‘when’ and ‘where’ of perceiving signals of threat versus non-threat. Neuroimage 31, 458–467.
Williams, P. E., Mechler, F., Gordon, J., Shapley, R., and Hawken, M. J. (2004). Entrainment to video displays in primary visual cortex of macaque and humans. J. Neurosci. 24, 8278–8288.
Winkielman, P., and Cacioppo, J. T. (2001). Mind at ease puts a smile on the face: psychophysiological evidence that processing facilitation elicits positive affect. J. Pers. Soc. Psychol. 81, 989–1000.
Womelsdorf, T., and Fries, P. (2006). Neuronal coherence during selective attentional processing and sensory–motor integration. J. Physiol. 100, 182–193.
Womelsdorf, T., and Fries, P. (2007). The role of neuronal synchronization in selective attention. Curr. Opin. Neurobiol. 17, 154–160.
Yamaguchi, S., Hale, L. A., D’Esposito, M., and Knight, R. T. (2004). Rapid prefrontal-hippocampal habituation to novel events. J. Neurosci. 24, 5356–5363.
Yiend, J., and Mathews, A. (2001). Anxiety and attention to threatening pictures. Q. J. Exp. Psychol. 54A, 665–681.
Yue, X., Vessel, E. A., and Biederman, I. (2007). The neural basis of scene preferences. Neuroreport 18, 525–529.
Zajonc, R. B. (1980). Feeling and thinking: preferences need no inferences. Am. Psychol. 35, 151–175.
Keywords: affect, emotion, consciousness
Citation: Phaf RH and Rotteveel M (2012) Affective monitoring: a generic mechanism for affect elicitation. Front. Psychology 3:47. doi: 10.3389/fpsyg.2012.00047
Received: 01 November 2011;
Accepted: 08 February 2012;
Published online: 01 March 2012.
Edited by:
Jack Van Honk, Utrecht University, NetherlandsReviewed by:
Eddie Harmon-Jones, Texas A&M University, USABarak Morgan, University of Cape Town, South Africa
Dennis Hofman, Utrecht University, Netherlands
Copyright: © 2012 Phaf and Rotteveel. This is an open-access article distributed under the terms of the Creative Commons Attribution Non Commercial License, which permits non-commercial use, distribution, and reproduction in other forums, provided the original authors and source are credited.
*Correspondence: R. Hans Phaf, Brain and Cognition Program, Department of Psychology, Faculty of Social and Behavioural Sciences, University of Amsterdam, Weesperplein 4, 1018 XA Amsterdam, Netherlands. e-mail:ci5oLnBoYWZAdXZhLm5s