- 1 Auditory and Language Neuroscience Laboratory, Center for Language Sciences, Center for Cognitive Neuroscience, Department of Cognitive Sciences, University of California Irvine, Irvine, CA, USA
- 2 Department of Cognitive Sciences, Institute for Mathematical Behavioral Sciences, University of California Irvine, Irvine, CA, USA
Recent evidence suggests that the speech motor system may play a significant role in speech perception. Repetitive transcranial magnetic stimulation (TMS) applied to a speech region of premotor cortex impaired syllable identification, while stimulation of motor areas for different articulators selectively facilitated identification of phonemes relying on those articulators. However, in these experiments performance was not corrected for response bias. It is not currently known how response bias modulates activity in these networks. The present functional magnetic resonance imaging experiment was designed to produce specific, measureable changes in response bias in a speech perception task. Minimal consonant-vowel stimulus pairs were presented between volume acquisitions for same-different discrimination. Speech stimuli were embedded in Gaussian noise at the psychophysically determined threshold level. We manipulated bias by changing the ratio of same-to-different trials: 1:3, 1:2, 1:1, 2:1, 3:1. Ratios were blocked by run and subjects were cued to the upcoming ratio at the beginning of each run. The stimuli were physically identical across runs. Response bias (criterion, C) was measured in individual subjects for each ratio condition. Group mean bias varied in the expected direction. We predicted that activation in frontal but not temporal brain regions would co-vary with bias. Group-level regression of bias scores on percent signal change revealed a fronto-parietal network of motor and sensory-motor brain regions that were sensitive to changes in response bias. We identified several pre- and post-central clusters in the left hemisphere that overlap well with TMS targets from the aforementioned studies. Importantly, activity in these regions covaried with response bias even while the perceptual targets remained constant. Thus, previous results suggesting that speech motor cortex participates directly in the perceptual analysis of speech should be called into question.
Introduction
Recent research and theoretical discussion concerning speech perception have focused considerably on the language production system and its role in perceiving speech sounds. More specifically, it has been suggested – in varying forms and with claims of variable strength – that the cortical speech motor system supports speech perception directly (Rizzolatti and Craighero, 2004; Galantucci et al., 2006; Hasson et al., 2007; Pulvermüller and Fadiga, 2010). Classic support for this position comes from studies involving patients with large frontal brain lesions (i.e., Broca’s aphasics), which demonstrate that these patients are impaired on syllable discrimination tasks (Miceli et al., 1980; Blumstein, 1995), including worse performance when discriminating place of articulation versus voicing (Baker et al., 1981), and perhaps mildly impaired (and significantly slowed) in auditory word comprehension (Moineau et al., 2005). Indeed, more recent evidence demonstrates unequivocally that the cortical motor system is active during speech perception (Fadiga et al., 2002; Hickok et al., 2003; Watkins et al., 2003; Wilson et al., 2004; Skipper et al., 2005; Pulvermüller et al., 2006). Perhaps the strongest evidence for motor system involvement comes from recent transcranial magnetic stimulation (TMS) studies. For example, one study demonstrated that repetitive TMS applied to a speech region of premotor cortex impaired syllable identification but not color discrimination (Meister et al., 2007), while another found that TMS of primary motor areas for different vocal tract articulators selectively facilitated identification of phonemes relying on those articulators (D’Ausilio et al., 2009). Additional TMS studies demonstrated that disruptive TMS applied to motor/premotor cortex significantly altered performance in discrimination of synthesized syllables (Möttönen and Watkins, 2009) and phoneme discrimination (Sato et al., 2009).
Despite the evidence listed above, neuropsychological data seem to dispel the notion that the speech motor system is critically involved in speech perception (Hickok, 2009; Venezia and Hickok, 2009). In short, Broca’s aphasics generally have preserved word-level comprehension (Damasio, 1992; Goodglass, 1993; Goodglass et al., 2001; Hillis, 2007), as do patients with bilateral lesions to motor speech regions (Levine and Mohr, 1979; Weller, 1993). Further, two recent studies of patients with radiologically confirmed lesions to motor speech areas including Broca’s region and surrounds, failed to replicate earlier findings that Broca’s aphasics have substantial speech discrimination deficits (Hickok et al., 2011; Rogalsky et al., 2011). Additionally, children that fail to develop motor speech ability (as a result of congenital or acquired anarthria) are able to develop normal receptive speech (Lenneberg, 1962; Bishop et al., 1990; Christen et al., 2000). Lastly, anesthesia of the entire left hemisphere, producing complete speech arrest (mutism), leaves speech sound perception proportionately intact (Hickok et al., 2008).
Nonetheless, it remains to explain why acute disruption and/or facilitation of speech motor cortex via TMS significantly alters performance on speech perception tasks. The following observations are relevant. First, the aforementioned TMS studies either utilized degraded or unusual (synthesized) speech stimuli (Meister et al., 2007; D’Ausilio et al., 2009; Möttönen and Watkins, 2009), or failed to produce an effect unless the phonological processing load was unusually high (Sato et al., 2009). Several studies indicate that speech motor areas of the inferior frontal cortex are more active with increasing degradation of the speech signal (Davis and Johnsrude, 2003; Binder et al., 2004; Zekveld et al., 2006). Additionally, Broca’s aphasics showed poor auditory comprehension when stimuli were low-pass filtered and temporally compressed (Moineau et al., 2005). Indeed, syllable identification (as in Meister et al., 2007; D’Ausilio et al., 2009) was not impaired nor were reaction times facilitated in TMS studies using clear speech stimuli (Sato et al., 2009; D’Ausilio et al., 2011).
Second, the effects of applying TMS to speech motor cortex are often small (Meister et al., 2007) and/or confined to reaction time measures (D’Ausilio et al., 2009; Sato et al., 2009). A recent functional magnetic resonance imaging (fMRI) study utilizing a two-alternative forced choice syllable identification task at varying signal-to-noise ratios (SNR) demonstrated that hemodynamic activity correlated with identification performance (percent correct) in superior temporal cortex and decision load (reaction time) in inferior frontal cortex (Binder et al., 2004). Additionally, Broca’s aphasics exhibit increased reaction times on an auditory word comprehension task relative to older controls and patients with right hemisphere damage (Moineau et al., 2005). Together these findings suggest that speech motor brain regions may be preferentially involved in decision-level components of speech perception tasks.
An important component of decision-level processes in standard syllable- or single-word-level speech perception assessments is response bias – i.e., when changes in a participant’s decision criterion lead to a more liberal or conservative strategy that biases the participant toward a particular response [see discussion of signal detection theory (SDT) below]. Response bias is not properly accounted for in standard measures of performance on speech perception tasks (percent correct, reaction time, error rates) such as those reported in the studies above that appear to implicate speech motor cortex in speech perception ability (Meister et al., 2007; D’Ausilio et al., 2009). For example, a recent study in which disruptive TMS was applied to the lip region of motor cortex reported that cross-category discrimination of synthesized syllables was impaired for lip-tongue place of articulation continua (/ba/-/da/, /pa/-/ta/) but not for voice onset time or non-lip place of articulation continua (/ga/-/ka/ and /da/-/ga/, respectively; Möttönen and Watkins, 2009). However, the performance measure in this study was simply the change in proportion of “different” responses in the same-different discrimination task after application of TMS. This effect could simply be due to changes in response bias induced by application of TMS to speech motor cortex (there is no reason to believe that an effect on response bias, like an effect on accuracy, should not be articulator-specific). Indeed, another recent study demonstrated that use-induced motor suppression of the tongue resulted in a larger response bias toward the lip-related phoneme in a syllable identification task with lip- and tongue-related phonemes (/pa/ and /ta/, respectively; Sato et al., 2011). The opposite effect held for use-induced suppression of the lips. Importantly, suppression had no effect on identification performance (d′) in any condition, it only modulated response bias.
Classic lesion data suggesting a speech perception deficit in Broca’s aphasics may also be contaminated by response bias. A study by Miceli et al. (1980) demonstrated that patients with a phonemic output disorder (POD+; fluent and non-fluent aphasics) were impaired on a same-different syllable discrimination task versus patients without disordered phonemic output (POD−). However, both groups made more false identities than false differences, and POD+ patients were more likely to make a false identity than POD− patients (see Miceli et al., 1980; their Table 1), indicating the presence of a response bias toward “same.” Similarly, a single-word, minimal pair discrimination study conducted by Baker et al. (1981) showed that Broca’s aphasics were more impaired at discriminating place of articulation than voicing, but this effect was driven by a higher error rate for “different” trials. In other words, Broca’s aphasics were again more likely to make a false identity. An informal analysis of the data (inferred from the error rates in same and different trials relative to the overall number of trials) indicates that overall performance on the discrimination task was quite good in Broca’s aphasics when response bias is accounted for (d′ = 3.78; Hickok et al., 2011).
In light of this information, we set out to determine whether changes in response bias modulate functional activity in speech motor cortex. Thus, the present fMRI experiment was designed to produce specific, measureable changes in response bias in a speech perception task using degraded speech stimuli. Minimal consonant-vowel stimulus pairs were presented between volume acquisitions for same-different discrimination. Speech stimuli were embedded in Gaussian noise at the threshold SNR as determined via 2-down, 1-up staircase. We manipulated bias by changing the ratio of same-to-different trials: 1:3, 1:2, 1:1, 2:1, 3:1. Ratios were blocked by run and subjects were cued to the upcoming ratio at the beginning of each run. In order to measure response bias, we modeled the data using a modified version of SDT. Briefly, SDT attempts to disentangle a participant’s decision criterion from true perceptual sensitivity (which should remain constant under unchanging stimulus conditions, regardless of shifts in criterion). In the classic case of a “yes-no” detection experiment, the participant is tasked with identifying a signal in the presence of noise (e.g., a tone in noise, a brief flash of light, or a tumor on an X-ray). Two conditional, Gaussian probability distributions – one for noise trials and another for signal + noise trials – are used to model the likelihood of observing a particular level of internal (sensory) response on a given trial. The normalized distance between the means of the two distributions, known as d′, is taken to be the measure of perceptual sensitivity (i.e., ability to detect the signal), where this distance is an intrinsic (fixed) property of the sensory system. However, the participant must set a response criterion – a certain position on the internal response continuum – for which trials that exceed the criterion response are classified as “signal.” The position of the criterion is referred to as c, and can change in response to a number of factors, both internal and/or external to the observer. The values for d′ and c can be estimated from the proportion of response types. We have extended this analysis to our same-different design. In brief (see Materials and Methods below for an extended discussion), we have modeled the decision space as six separate conditional Gaussian distributions that represent each of the six possible stimulus pairs presented on a same-different trial. The internal response continuum is a single perceptual statistic (standard normal units) that represents the stimulus pair, where negative values are more likely to be a “same” pair (e.g., ba-ba) and positive values are more likely to be a “different” pair (e.g., ba-da). The listener sets a single criterion value on the internal response continuum, where trials that produce a response above the criterion yield a “different” response, while responses below the criterion yield a “same” response (see Figure 2A).
Based on the properties of our design – in particular, the maintenance of a constant SNR and otherwise identical stimulus conditions across runs – we assumed that the distances between the means (analogous to d′) of the six stimulus distributions were fixed across bias ratio conditions. The criterion value, here called C, was allowed to vary across conditions. To be explicit, d′ would not be expected to change because the sensory properties of the stimuli remained constant across conditions, while C would be expected to change because the same-different ratio was manipulated directly in each condition. We expected changes in response bias to correlate with changes in the blood-oxygen level dependent (BOLD) signal in motor (i.e., frontal) brain regions, but not sensory (i.e., temporal) brain regions. This is precisely what we observed – response bias, C, varied significantly across conditions and a group-level regression of overall bias on percent signal change (PSC) revealed a network of motor brain regions that correlated with response bias. To the best of our knowledge, this is the first study to demonstrate a direct relationship between response bias and functional brain activity in a speech perception task. The significance of this relationship is discussed below along with details of the particular network of brain areas that correlated with response bias.
Materials and Methods
Participants
Eighteen (9 female) right-handed, native-English speakers between 18 and 32 years of age participated in the study. All volunteers had normal or corrected-to-normal vision, no known history of neurological disease, and no other contraindications for MRI. Informed consent was obtained from each participant in accordance with UCI Institutional Review Board guidelines.
Stimuli and Procedure
Participants were presented with same-different discrimination trials involving comparison of 250 ms-duration consonant-vowel syllables (/ba/, /da/, or /ga/) embedded in a broadband Gaussian noise masker (independently sampled) of equal duration. Auditory stimuli were recorded in an anechoic chamber (Industrial Acoustics Company, Inc.). During recording, a male, native-English speaker produced approximately 20 samples of each syllable using natural timing and intonation, pausing briefly between each sample over the course of a single continuous session. A set of four tokens was chosen for each syllable based on informal evaluation of loudness, clarity, and quality of the audio recording (see Figure 1A for a representative member from each speech sound category). Syllables were digitally recorded at a sampling rate of 44.1 kHz and normalized to equal root-mean-square amplitude. The average A-weighted level of the syllables was 66.3 dB SPL (SD = 0.5 dB SPL). Since natural speech was used, several tokens (i.e., separate recordings) of each syllable were selected so that no artifact of the recording process could be used to distinguish between speech sound categories. This also increased the difficulty of the task such that discrimination relied on subjects’ ability to distinguish between speech sound categories rather than identify purely acoustic differences in the stimuli (i.e., both within- and between-category tokens differed acoustically). Throughout the experiment, the level of the noise masker was held constant at approximately 62 dB(A). All sounds were presented over MR-compatible, insert-style headphones (Sensimetrics model S14) powered by a 15-W-per-channel stereo amplifier (Dayton model DTA-1). This style of headphone utilizes a disposable “earbud” insert that serves as both an earplug and sound delivery apparatus, allowing sounds to be presented directly to participants’ ear canals. During scanning, a secondary protective ear cover (Pro Ears Ultra 26) was placed over the earbuds for additional attenuation of scanner noise. Stimulus delivery and timing were controlled using Cogent software1 implemented in Matlab R12 (Mathworks, Inc., USA) running on a dual-core IBM Thinkpad laptop.
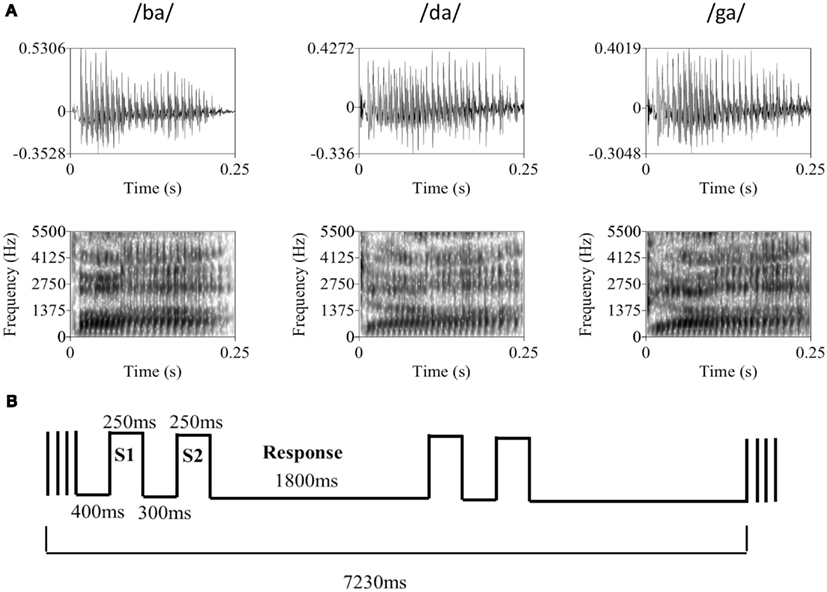
Figure 1. (A) Representative tokens from each of the three syllable categories used in the discrimination task. The top row contains the raw waveforms for each token with amplitude (y-axis) plotted against time (x-axis). The bottom row contains the associated spectrograms for each token with frequency in Hz (y-axis) plotted against time (x-axis), where darker sections indicate greater sound energy. During the experiment, auditory syllable stimuli were presented in broadband Gaussian noise at a constant level of 62 dB SPL. Syllable amplitude was held constant at the psychophysically determined threshold level (mean SNR = −13.1 dB). (B) The basic trial and block structure of the fMRI experiment. Each block consisted of two same-different discrimination trials with 250 ms auditory syllable presentations separated by a 300-ms ISI. Subjects had 1800 ms to respond. Trials occurred in the silent period between 1630 ms volume acquisitions, with a 400 ms silent period at the beginning of each block of two trials.
Trials followed a two-interval same-different discrimination procedure. Two response keys were operated by the index finger of the left hand. Trials consisted of presentation of one of the syllables, followed by a 300-ms interstimulus interval, then presentation of a second syllable. Participants pressed key 1 if the two syllables were from the same category (e.g., /ba/-/ba/) and key 2 if the syllables were from different categories (e.g., /ba/-/da/). During the period between responses, participants rested their index finger at a neutral center-point spaced equidistantly from each response key. Participants were instructed to respond as quickly and accurately as possible. Each participant took part in a behavioral practice session in a quiet room outside the scanner. During this practice session, participants were asked to perform 24 practice trials, followed by 72 trials in a “2-down, 1-up” staircase procedure that tracks the participant’s 71% threshold (Levitt, 1971). During the staircase procedure, syllable amplitude was varied with 4 dB step size. Participants then performed a second block of 72 trials following the same staircase procedure with a 2-dB step size. Threshold level was determined by eliminating the first four reversals and averaging the amplitude of the remaining reversals (four minimum). Once the threshold level was determined participants performed additional blocks of 72 trials at threshold until behavioral performance stabilized between 65 and 75% correct. Many subjects continued to improve over several runs, therefore the experimenter was instructed to make 1–2 dB adjustments to the syllable level in between runs in order to keep performance in the target range. All trials prior to scanning were presented back-to-back with a 500-ms intertrial interval and a constant same-different ratio of 1:1. Practice trials were self-paced (i.e., the next trial did not begin until a response was entered).
During the scan session, participants were placed inside the MRI scanner and, following initial survey scans, the scanner was set to “standby” in order to minimize the presence of external noise due to cooling fans and pumps in the scan room. Participants were then required to repeat the staircase procedure described above. It was necessary to set threshold performance inside the scanner because the level of ambient noise in the scan room could not be kept equivalent to our behavioral testing room. The threshold level determined inside the scanner was used throughout the remainder of the experiment (mean = 48.9 dB, SD = 5.8 dB; mean SNR = −13.1 dB). After a short rest following threshold determination, the MRI scanner was set to “start” for fMRI data collection. Volumes were acquired using a traditional sparse scanning sequence with a volume acquisition time of 1630 ms and an interscan interval of 5600 ms. Blocks of two trials, each with a 1.8 s response period, occurred in the silent period between single volume acquisitions (Figure 1B). Rest blocks (no task) were included at random at a rate of one in every six blocks. Each scanning run contained a total of 36 task blocks (72 trials) and six rest blocks. Subjects performed a total of 10 runs (720 trials). In order to manipulate response bias, subjects were cued to a particular ratio of same-different trials – 1:3, 1:2, 1:1, 2:1, or 3:1 – at the beginning of each run. Each ratio appeared twice per subject and the order of ratios was randomized across subjects. Crucially, during the practice, adaptive (pre-scan) and fMRI portions of the experiment, each run of 72 trials was designed so that the four tokens for each syllable (/ba/, /da/, /ga/) were presented 12 times. For example, in the 1:1 ratio condition (all pre-scan runs were of this type) there were 36 “same” trials (12 ba-ba, 12 da-da, 12 ga-ga) and 36 “different” trials (6 ba-da, 6 ba-ga, 6 da-ba, 6 da-ga, 6 ga-ba, 6 ga-da). So, there were 48 presentations of /ba/, 48 presentations of /da/, and 48 presentations of /ga/, where each group of 48 was divided evenly between each of the four tokens available for that speech sound. The order of trial type (same or different), speech sound identity of the stimulus pair (e.g., ba-ba, ba-da, da-ga, etc.), and token identity were drawn pseudorandomly to fit the run structure. Thus, exactly the same tokens were presented in each run, but the pairings were modified to change the same-different ratios.
Scanning Parameters
MR images were obtained in a Philips Achieva 3T (Philips Medical Systems, Andover, MA, USA) fitted with an 8-channel SENSE receiver/head coil, at the Research Imaging Center facility at the University of California, Irvine. We collected a total of 430 echo planar imaging (EPI) volumes over 10 runs using single pulse Gradient Echo EPI [matrix = 76 × 76, repetition time (TR) = 7.23 s, acquisition time (TA) = 1630 ms, echo time (TE) = 25 ms, size = 2.875 mm × 2.875 mm × 3.5 mm, flip angle = 90]. Thirty axial slices provided whole brain coverage. Slices were acquired sequentially with a 0.5-mm gap. After the functional scans, a T1-weighted structural image was acquired (140 axial slices; slice thickness = 1 mm; field of view = 240 mm; matrix 240 × 240; repetition time = 11 ms, echo time = 3.55 ms; flip angle = 18°; SENSE factor reduction 1.5 × 1.5).
Data analysis – Behavior
We assume the participant extracts from the stimulus pair presented on a given trial a statistic (a random variable) that reflects the strength of the difference between the two substimuli in the pair. We further assume that the distribution of this difference-strength statistic is invariant with respect to the order of substimuli in a pair2. Thus, for example, the difference-strength statistic characterizing a ba-da pair is assumed to be identically distributed to the statistic characterizing a da-ba pair. Under these assumptions, there are six classes of stimuli, Sk, k = 1, 2, …, 6, three in which the two substimuli are drawn from the same category and three in which the two substimuli are drawn from different categories. We assume that the difference-strength statistic the participant extracts from a given presentation of Sk, k = 1, 2, …, 6, is a normally distributed random variable Xk with standard deviation 1 and mean μk. In a bias condition with proportion q of “different” trials, we assume the participant judges stimulus Sk to be “different” just if Xk > Cq, where Cq is the criterion adopted by the participant in the given bias condition. Under this model, the probability of a correct response in the bias condition with proportion q of “different” responses given a stimulus Sk is
where Φ is the standard normal cumulative distribution function.
This model has 11 parameters: μk, k = 1, 2, …, 6, and Cq, for q ranging across the five ratios (1:3, 1:2, 1:1, 2:1, or 3:1) of same-to-different trials. However, the model is underconstrained if all 11 parameters are free to vary as can be seen by considering Eq.1. Note, in particular, that for any real number α, the probabilities Pk,q remain the same if we substitute Cq + α for each of the Cq’s and μk + α for each of the μk’s. For current purposes it is convenient to insure that the model parameters are uniquely determined by imposing the additional constraint that the μk’s sum to 0. Thus, the model actually has only 10 degrees of freedom.
For any values μk, k = 1, 2, …, 6 and C1, C2, …, C5 (with the μk’s constrained to sum to 0), the log likelihood function is
Where Pk,q is given by Eq.1, Hk,q (Mk,q) is the number of correct (incorrect) responses given to stimulus Sk in the bias condition with proportion q of “different” trials. Data from trials for which no response was recorded were excluded from analysis (mean proportion dropped = 0.01, max = 0.057).
In short, the values μk can be thought of as six “perceptual distances” (analogous to d′) that characterize the sensory representation of the stimulus pairs, where the model estimates of these distances are assumed to be constant across bias conditions. The model is constrained such that the mean of the values μk is set to zero. The values Cq are the five criterion values – one for each bias condition – and serve as a measure of response bias where negative values indicate a bias to respond “different,” positive values indicate a bias to respond “same,” and larger values indicate a stronger bias (standard normal units). See Figure 2A for a visual representation of the parameter space based on a representative subject’s actual data.
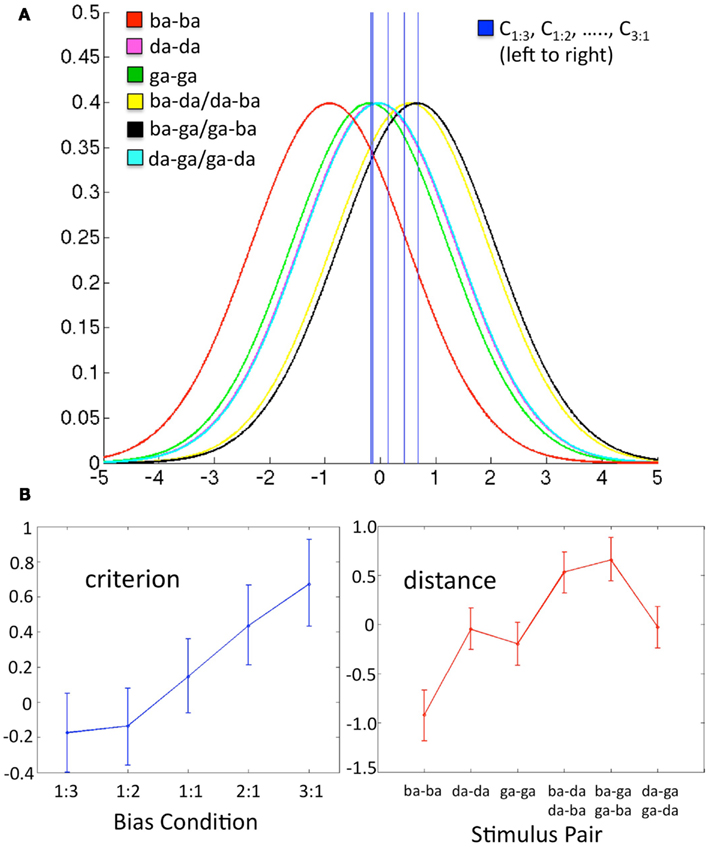
Figure 2. Data in each panel are from a representative subject. (A) Schematic of the decision space including six conditional Gaussian distributions (one for each stimulus pair) representing the likelihood of observing a given sensory response, and five criterion values (one for each bias ratio condition), where C0.333, C0.5, …., C3, fall left to right on the graph. The x-axis is the value of a difference-strength statistic representing the perceptual difference between two substimuli in a pair. The y-axis is the probability of observing a given value of the difference-strength statistic. For a given bias ratio condition, values of the difference-strength statistic that fall above (right of) the criterion line yield a “different” response, while values that fall below (left of) the criterion yield a “same” response. The positions of the six stimulus pair distributions remain fixed across conditions. Note that the magenta (da-da) and teal (da-ga/ga-da) distributions fall roughly on top of one another, as do the criteria for the 1:3 and 1:2 bias ratio conditions. (B) Model estimates (over all q = proportion of different trials, k = stimulus pair) for Cq (criterion, left) and μk (distance, right) plotted as line graphs with the Bayesian 95% credible intervals plotted as error bars. These values reflect the five criterion lines and the means of the six stimulus pair distributions plotted in (A) above, respectively.
To estimate the parameter values, we used a Bayesian modeling procedure to fit the data for each participant. This fitting procedure employs a Markov chain Monte Carlo (MCMC) algorithm that yields a sample of size 100,000 from the posterior density characterizing the joint distribution of the model parameters. The prior density for each parameter was taken to be uniform on the interval (−10, 10). Model parameters were estimated from an initial run of 100,000 with starting values of μk = 0 and Cq = 0 over all values of k and q, where the first 20,000 samples were discarded as burn-in and the remaining 80,000 were utilized for posterior estimation. A second run of 100,000 was then executed with starting values of μk and Cq equal to the mean parameter estimates from the initial run. Final parameter estimates and 95% credible intervals were derived from all 100,000 samples of the second run. A given parameter was estimated by the mean sample value for that parameter, and the 95% credible intervals were estimated by taking the 0.025 and 0.975 quantiles of the sample for that parameter. Data from a representative subject are plotted in Figure 2B: five criterion values and six distance values are displayed as line graphs with the 95% credible interval as error bars.
Since we were only interested in parameter differences induced by our same-different ratio manipulation, only the vector of estimated criterion values, C = (C0.333, C0.5, C1, C2, C3) were entered into second-level analyses. The mean 95% credible interval across Cq, q = 0.333, 0.5, 1, 2, 3, was calculated for each subject as a means to determine the precision of the model fit. Three subjects with a mean 95% credible interval greater than 1 (and negligible variation in the Cq’s) were excluded from further analysis. Individual subject parameter estimates for C were then entered in a multivariate analysis of variance (mANOVA) to test for differences in the group means across bias ratio conditions.
Data Analysis – MRI
Study-specific template construction and normalization of functional images
Group-level localization of function in fMRI, including identification of task-related changes in activation, can be highly dependent on accurate normalization to a group template. Surface-based (Desai et al., 2005; Argall et al., 2006) and non-linear (Klein et al., 2009, 2010) warping techniques have recently been utilized to improve normalization by accounting for individual anatomical variability. Here, we used a diffeomorphic registration method implemented within the Advanced Normalization Tools software (ANTS; Avants and Gee, 2004; Avants et al., 2008). Symmetric diffeomorphic registration (SyN) uses diffeomorphisms (differentiable and invertible maps with a differentiable inverse) to capture both large deformations and small shape changes. We constructed a study-specific group template using a diffeomorphic shape and intensity averaging technique and a cross-correlation similarity metric (Avants et al., 2007, 2010). The resulting template was then normalized using SyN to the MNI-space ICBM template3 (ICBM 2009a Non-linear Symmetric). A low-resolution (2 mm × 2 mm × 2 mm) version of the study-specific template was constructed for alignment of the functional images. Functional images for each individual subject were first motion-corrected, slice timing corrected and aligned to the individual subject anatomy in native space using AFNI software4. Following this step, the series of diffeomorphic and affine transformations mapping each individual subject’s anatomy to the MNI-space, study-specific template was applied to the aligned functional images, using the low-resolution template as a reference image. The resulting functional images were resampled to 2 mm × 2 mm × 2 mm voxels and registered to the study-specific group template in MNI-space.
fMRI analysis
Preprocessing of the data was performed using AFNI software. For each run, motion correction, slice timing correction, and coregistration of the EPI images to the high resolution anatomical were performed in a single interpolation step. Normalization of the functional images to the group template was performed as described above. Images were then high pass filtered at 0.008 Hz and spatially smoothed with an isotropic 6-mm full-width half-maximum (FWHM) Gaussian kernel. Each run was then mean scaled in the temporal domain. The global mean signal was calculated at each time point and entered as a regressor of no interest in the individual subject analysis along with motion parameter estimates.
A Generalized Least Squares Regression analysis was performed in individual subjects in AFNI (3dREMLfit). To create the regressors of interest, a stimulus-timing vector was created for each bias ratio condition by modeling each sparse image timepoint as “on” or “off” for that condition. The resulting regression coefficients for each bias ratio represented the mean PSC from rest. A linear contrast representing the average activation (versus rest) across all bias ratio conditions was also calculated for each subject.
Group analysis
First, a mask of “active” voxels was created by entering the individual subject contrast coefficients for average activation across bias ratio conditions (relative to rest) in a Mixed-Effects Meta-Analysis (AFNI 3dMEMA) at the group-level. This procedure is similar to a standard group-level t-test but also takes into account the level of intra-subject variation by accepting t-scores from each individual subject analysis. A voxel-wise threshold was applied using the false discovery rate (FDR) procedure at q < 0.05. Voxels surviving this analysis demonstrated a mean level of activity that was significantly greater than baseline across all bias conditions and all subjects at the chosen threshold. All further analyses were restricted to this set of voxels.
To evaluate whether voxels were sensitive to changes in behaviorally measured response bias, we performed an orthogonal linear regression with absolute value of the bias score as the predictor variable and PSC as the dependent variable (orthogonal regression accounts for measurement error in both the predictor and dependent variables). We chose to use the absolute value of our bias measure because the sign of Cq reflects the direction of response bias (toward “same” or “different”), and we wanted to assess the effect of overall bias magnitude on PSC, without respect to direction. As such, we will subsequently refer to the vector of bias values entered in the group fMRI analysis as |C| = (|C0.333|, |C0.5|, |C1|, |C2|, |C3|). Individual subject vectors |C| and PSC = (PSC0.333, PSC0.5, PSC1, PSC2, PSC3) were concatenated across subjects and entered in the group regression. To account for between-subject variability in |C| and PSC, measures in each individual subject vector were converted to z-scores prior to regression. Thus, the ratio of error variances in the orthogonal regression was assumed to be 1, such that the equation for the slope of the regression line (y = mx + b) took the form
where Ui = xi − mean(x) and Vi = yi − mean(y). A one-out jackknife procedure was used to estimate the standard error of the slope estimator. Jackknife t-statistics were constructed in the form
where m is the slope estimator, is the mean of the jackknife distribution of slope estimators, and is the standard deviation of the jackknife distribution of slope estimators. Hypothesis testing was performed against values from a Student’s t distribution with N−2 degrees of freedom.
In sum, our group analysis consisted of orthogonal linear regression of 75 bias scores on their corresponding 75 PSC measures (five bias ratio conditions, 15 subjects; three subjects were excluded on the basis of our criterion on the maximum allowable mean 95% Bayesian credible interval for C). Voxels were deemed to be significant at an FDR-corrected threshold of q < 0.01 with a minimum cluster size of 20 voxels.
Results
Behavioral Results
During the fMRI session, subjects performed a total of 144 same-different discrimination trials in each of five bias ratio conditions: 1:3, 1:2, 1:1, 2:1, 3:1. Consonant-vowel pairs were presented in a background of Gaussian noise at a constant SNR based on a behaviorally determined threshold performance (see Materials and Methods). Subjects were expected to make use of same-different ratio information to bias their response patterns – e.g., in the 1:3 ratio condition, subjects would be expected to respond “different” more often on uncertain trials, and in the 3:1 ratio condition subjects would be expected to respond “same” more often. As such, our behavioral measure of response bias, C, was expected to vary significantly across bias ratio conditions.The results bear out this expectation: group mean C varied significantly (Λ = 0.170, F(4, 11) = 13.461, p < 0.001) and in the expected direction (larger negative values for ratio conditions with a greater number of different trials and larger positive values for ratio conditions with a greater number of same trials; see Figure 3A). This result confirms that our treatment succeeded in manipulating response bias while holding the physical stimuli constant across conditions. Proportion correct did not vary significantly across conditions [Λ = 0.470, F(4,11) = 3.105, p = 0.061; see Figure 3B]. For completeness, we also calculated a summary d′ measure for each condition by tabulating the overall hit rate and false alarm rate across all trial types and entering these values in the standard signal detection formula for same-different designs (Independent Observation model; see Macmillan and Creelman, 2005). As expected, group mean d′ values did not vary significantly across conditions [Λ = 0.757, F(4, 14) = 1.125, p = 0.384].
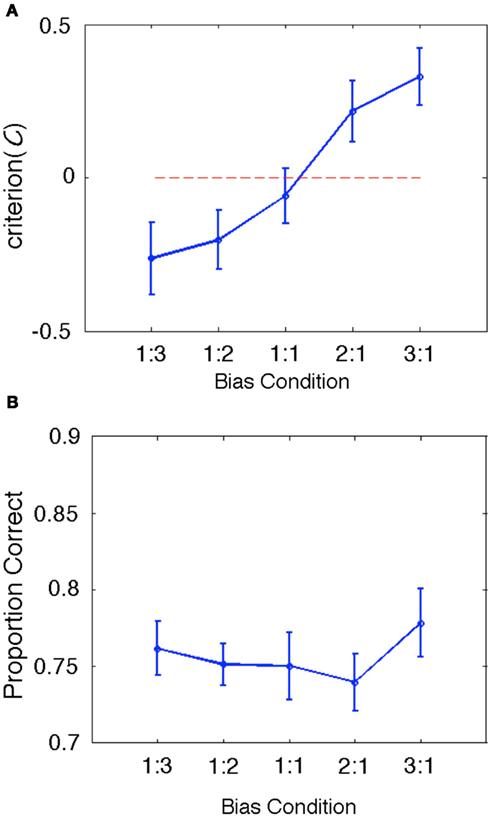
Figure 3. (A) Group behavioral results for our bias measure, C. The data are plotted as a line graph where the x-axis is the same-different ratio and the y-axis is the group mean value of C. Error bars reflect ± one standard error of the mean. The zero criterion value (no bias) is plotted as a dotted line in red. Clearly, C varies significantly in the expected direction (negative values indicate bias toward responding “different” and positive values indicate bias toward responding “same”). Also, the mean criterion value in the 1:1 bias ratio condition is closest to zero (and contains zero within ±1SE), as expected. (B) Group behavioral results for proportion correct (PC). Although PC varied slightly across conditions, this variability is likely accounted for by shifts in bias, which affect PC but not bias-corrected measures like d′.
fMRI Results
Overall activation to speech discrimination versus rest was measured on the basis of a linear contrast modeling mean activation across bias ratio conditions (see Materials and Methods). The group result for this contrast (Figure 4) reveals a typical perisylvian language network including activation in bilateral auditory cortex, anterior and posterior superior temporal gyrus (STG), posterior superior temporal sulcus (STS), and planum temporale. Activation was also observed in speech motor brain regions including left inferior frontal gyrus (IFG) and insula, bilateral motor/premotor cortex and bilateral supplementary motor area (SMA). Other active areas include bilateral parietal lobe (including somatosensory cortex), thalamus and basal ganglia, cerebellum, prefrontal cortex, and visual cortex. All activations are reported at FDR-corrected p < 0.05. Subsequent analyses were restricted to suprathreshold voxels in this task versus rest analysis.
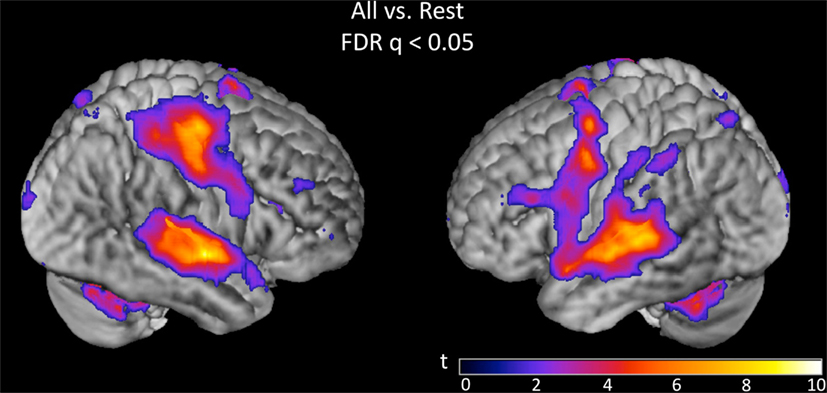
Figure 4. Group (n = 15) t-map for the contrast corresponding to mean activation in the syllable discrimination task (versus rest) across all five bias ratio conditions. The statistical image was thresholded at FDR-corrected q < 0.05. The set of voxels identified in this contrast were used as a mask for all subsequent analyses.
In order to isolate active voxels for which activity was modulated significantly by changes in response bias, we carried out a group-level regression of |C| against measured PSC. Each measure was converted to a z-score prior to regression in order to account for between-subject variability in |C| and PSC. In other words, we wanted to identify voxels for which changes in response bias (regardless of direction) were associated with changes in PSC in individual subjects. We hypothesized that voxels in speech motor brain regions would be most strongly modulated by changes in response bias. Indeed, significant voxels were almost exclusively restricted to motor and/or fronto-parietal sensory-motor brain regions. Clusters significantly modulated by response bias were identified in left ventral pre-central gyrus bordering on IFG, a more dorsal aspect of the left ventral pre-central gyrus, left insula, bilateral SMA, left ventral post-central gyrus extending into frontosylvian cortex, right superior parietal lobule, left inferior parietal lobule, and bilateral superior frontal cortex including middle frontal gyrus, superior frontal gyrus, and dorsal pre-central gyrus (see Table 1 for MNI coordinates). One additional cluster was identified in right peri-calcarine visual cortex. Each of these clusters demonstrated a strong negative relationship between |C| and PSC (i.e., signal was generally stronger when participants exhibited less response bias; see Figure 5). The significance of this relationship is discussed at length below but, briefly, we believe that signal increases were produced by more effortful processing when, (1) probabilistic information was not available to subjects (in the 1:1 condition), or (2) subjects chose to ignore available probabilistic information (low measured response bias in the 1:3, 1:2, 2:1, or 3:1 conditions), leading to increased recruitment of the sensory-motor network elaborated previously. Indeed, no clusters were found to demonstrate a positive relationship between response bias and PSC, and activity in temporal lobe structures was not correlated with response bias.
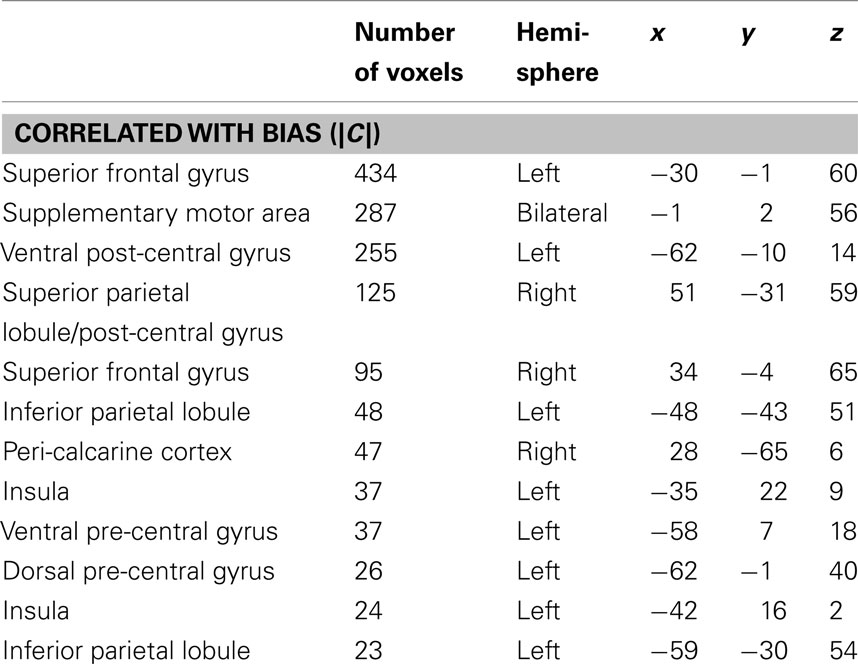
Table 1. MNI coordinates of the center of mass in activated cluster (thresholded FDR q < 0.01, minimum 20 voxels, group analysis).
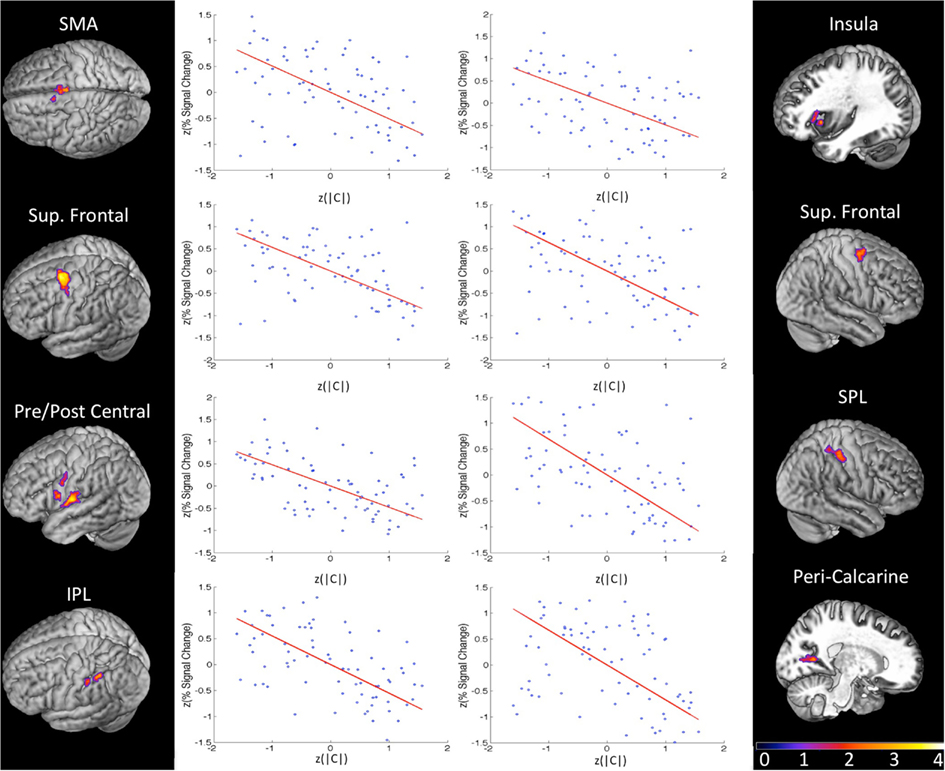
Figure 5. Group results (z-maps, where FDR q values are converted to z-scores) for the orthogonal regression of response bias (|C|) on BOLD percent signal change (PSC). Each region pictured was significant at FDR-corrected q < 0.01 with a minimum cluster size of 20 voxels. Next to each significant cluster is a scatter plot of normalized bias score (x-axis) against normalized PSC (averaged across all voxels in the region). Each plot contains five data points from each of the 15 subjects (blue) corresponding to the five same-different ratio conditions in the syllable discrimination experiment. The orthogonal least squares fit is plotted in red. There is a strong negative relationship between response bias and PSC in every region of this fronto-parietal network.
Discussion
Performance on a speech sound discrimination task involves at least two processes, perceptual analysis and response selection. In the present experiment we effectively held perceptual analysis (SNR) constant while biasing response selection (probability of same versus different trials). Our behavioral findings confirmed that this manipulation was successful, as our measure of bias, C, changed significantly across conditions as expected. This allowed us a means for identifying the brain regions involved in the response selection component of the task. When the discrimination task was compared against baseline (rest) we found a broad area of activation including superior temporal, frontal, and parietal regions bilaterally, implicating auditory as well as motor and sensory-motor regions in the performance of the task. However, when we tested for activation that was correlated with changes in response bias, only the motor and sensory-motor areas were found to be significantly modulated; no temporal lobe regions were identified. This finding is consistent with a model in which auditory-related regions in the temporal lobe are performing perceptual analysis of speech, while the motor-related regions support (some aspect of) the response selection component of the task.
A similar dissociation has been observed in monkeys performing vibrotactile frequency discrimination (VTF). In a VTF experiment, monkeys must compare the frequency of vibration of two tactile stimuli, f1 and f2, separated by a time gap. The monkey must decide whether the frequency of vibration was greater for f1 or f2, communicating its answer by pressing a button with the non-stimulated hand. Single-unit recordings taken from neurons in primary somatosensory cortex (S1) indicate that, for many S1 neurons, the average firing rate increases monotonically with increasing stimulus frequency (Hernández et al., 2000). It has been argued that this rate code serves as sensory evidence for the discrimination decision (Romo et al., 1998, 2000; Salinas et al., 2000). However, S1 firing rates do not dissociate on the basis of trial type – that is, there is no difference in the mean firing rate (across frequencies) during the comparison (f2) period for trials of type f1 > f2 versus f2 > f1, so S1 responses strictly reflect f1 frequency in the first interval and f2 frequency in the second interval, not their relation (cf., Gold and Shadlen, 2007). However, neurons in several areas exhibit activity patterns that do reflect a comparison between f1 and f2. In particular, neurons in the ventral and medial premotor cortices persist in firing during the delay period between f1 and f2, and discriminate trial type on the basis of mean firing rate during the comparison period (Romo et al., 2004). This indicates that these neurons are likely participating in maintenance and comparison of sensory representations (Hernández et al., 2002; Romo et al., 2004).
In the case of our syllable discrimination paradigm, it is unclear exactly what aspect(s) of response selection is (are) being performed by the motor and sensory-motor brain regions identified in our fronto-parietal network. Beyond perceptual analysis of the stimuli, a discrimination task involves short-term maintenance of the pair of stimuli and some evaluation and decision process. Given that our bias measure was negatively correlated with BOLD signal in the fronto-parietal network, i.e., that activation was higher with less bias, the following account is suggested. Bias can simplify a response decision by providing a viable response option in the absence of strong evidence from a perceptual analysis. In our case, knowledge of the same versus different trial ratio provides a probabilistically determined response option in the case of uncertainty. So when subjects were in doubt based on the perceptual analysis, a simple decision, go with the most likely response, was available and if used would tend to decrease activation in a neural network involved in response selection.
Although our experiment cannot determine which aspects of the response selection process are driving activation in the fronto-parietal network, the location of some of the activations suggest some likely possibilities. For example, the involvement of lower-level motor speech areas such as ventral and dorsal premotor cortex, regions previously implicated in phonological working memory (Hickok et al., 2003; Buchsbaum et al., 2005a,b, 2011), suggest that these regions support response selection via short-term maintenance of phonological information. One possibility, therefore, is that in the absence of either decisive perceptual information or a strong decision bias, subjects will work harder to come to a decision and as part of this effort will maintain short-term activation of the stimuli in working memory for a longer period of time resulting in more activation in regions supporting articulatory rehearsal.
An alternative view of the role of the motor system is that it is particularly involved in speech perception during degraded listening conditions, such as in the current experiment in which stimuli were presented in noise and near psychophysical threshold (Binder et al., 2004; Callan et al., 2004; Zekveld et al., 2006; Shahin et al., 2009; D’Ausilio et al., 2011). This view is not inconsistent with the present findings, at least broadly. For example, the motor system may assist in perception via its role in phonological working memory. In this case, the explanation of our findings proposed above would hold equally well as this “alternative.” However, most motor-oriented theorists promote a more powerful role for the motor system in speech perception, holding that it contributes substantively to the perceptual analysis. Some of these authors have argued for a strong version of the motor theory of speech perception by which motor representations themselves must be activated in order for perception to occur (Gallese et al., 1996; Fadiga and Craighero, 2006). Others hold a more moderate view in which the motor system is able to modulate sensory analysis of speech, for example, via predictive coding (Callan et al., 2004; Wilson and Iacoboni, 2006; Skipper et al., 2007; Bever and Poeppel, 2010). The present data do not provide strong support for either of these possibilities because if the motor system were contributing to perceptual analysis, then modulating motor activity, as we successfully achieved in our study would have been expected to result in a modulation of perceptual discrimination, which it did not.
The above argument, that modulation of the motor system did not result in a corresponding modulation of perceptual discrimination and therefore the motor system is not contributing to perception, is dependent on whether we modulated the relevant components of the motor system. To assess this, we examined the relation between our biased-induced modulation and two prominent previous TMS studies that have targeted the motor speech system and found modulatory effects on (potentially biased) measures of performance. Figure 6 shows that the regions that are correlated with our measure of bias overlap those regions that were stimulated in previous TMS studies of motor involvement in speech perception. This suggests that we were successfully able to modulate activity in these same regions and yet still failed to observe an effect on perceptual discrimination.
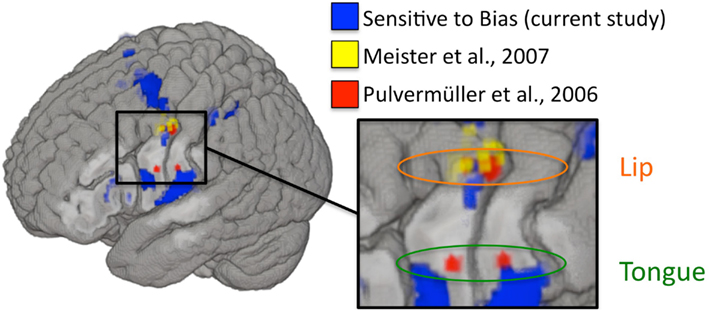
Figure 6. Left hemisphere activations that were sensitive to changes in response bias are plotted in blue (current study). MNI coordinates used to target rTMS in Meister et al. (2007; listed in their Table 1) are plotted as 2 mm radius spheres in yellow. Motor ROIs from Pulvermüller et al. (2006) are plotted in red as 3 mm radius spheres around the MNI peak activation foci (8 mm radius ROIs were used in the original study). Depth is represented faithfully – activations nearest to the displayed cortical surface are increasingly bold in color, while activations farthest from the displayed surface are increasingly transparent. Targets in Meister et al. were peak activations from an fMRI localizer experiment involving perception of auditory speech. Disruptive stimulation of these targets resulted in a slight decrement in syllable identification performance. The motor ROIs from Pulvermüller et al. were identified on the basis of activation to lip and tongue movements in a motor localizer experiment. The motor somatotopy established therein is also shown (lip foci are circled in orange and tongue foci are circled in green). The two posterior foci (one in the lip region and one in the tongue region) were chosen to target TMS stimulation in D’Ausilio et al. (2009), which demonstrated that excitatory stimulation to lip and tongue motor cortex selectively facilitated identification of phonemes relying on those articulators.
What might explain the discrepancy between our finding, that the motor speech system is modulated by manipulations of bias (and not perceptual discrimination), and TMS findings, which show that stimulation of portions of the motor speech system affect measures of speech perception? Given that none of the previous TMS studies used an unbiased dependent measure, it is a strong possibility that what is being affected by TMS to motor speech areas is not speech perception ability but response bias. An alternative possibility is consistent with our proposed interpretation of what is driving the bias correlation in our experiment, namely that motor speech regions support speech perception tasks only indirectly via articulatory rehearsal. This could explain Meister et al.’s (2007) result that stimulation to premotor cortex caused a decline in performance on the assumption that active maintenance of the stimulus provides some benefit to performance. It could also explain the somatotopic-specificity result of D’Ausilio et al. (2009) on the assumption that stimulating lip or tongue areas biased the contents of phonological working memory. Further TMS studies using unbiased measures will be needed to sort out these possibilities.
To review, we specifically modulated response bias in a same-different syllable discrimination task by cueing participants to the same-different ratio, which was varied over five values (1:3, 1:2, 1:1, 2:1, 3:1). We used the measure C from our modified signal detection analysis to evaluate performance, where this measure is taken to represent behavioral response bias. Our experimental manipulation was successful in that response bias varied significantly across conditions while the physical stimuli remained constant, and we demonstrated that overall magnitude of response bias (|C|) correlated significantly with BOLD PSC in a fronto-parietal network of motor and sensory-motor brain regions. We had predicted that we would observe a significant relationship between response bias and BOLD activity in frontal but not temporal brain structures. Indeed, 8 of 12 clusters demonstrating a significant relationship between bias and PSC were entirely confined to frontal cortex or contained voxels in frontal cortex. None of the clusters contained voxels in temporal cortex. In each region there was a strong negative relationship between response bias and BOLD activation level, which we argued was due to our sensory-motor network participating in response selection components of the syllable discrimination task. In particular, we argued that the load on response selection was reduced when a probabilistically determined response option was available, resulting in lower activation levels. We also demonstrated that several of our clusters in the vicinity of the left premotor cortex overlap quite well with premotor foci previously implicated in modulation of speech perception ability. However, our results undermine claims that speech perception is supported directly by premotor cortex since our manipulation of response bias successfully modulated activity in these regions without modulating speech discrimination performance.
Conflict of Interest Statement
The authors declare that the research was conducted in the absence of any commercial or financial relationships that could be construed as a potential conflict of interest.
Acknowledgments
During this investigation, Jonathan Henry Venezia was supported by the National Institute on Deafness and Other Communication Disorders Award DC010775 from the University of California, Irvine. The investigation was supported by the National Institute on Deafness and Other Communication Disorders Award DC03681.
Footnotes
- ^http://www.vislab.ucl.ac.uk/cogent 2000.php
- ^This assumption may not be valid. An alternative is that the order of presentation should be reflected in the sign of the difference-strength statistic. To ensure that the brain-bias relationship in motor-related regions was not dependent on our model assumptions, we reran the analysis using an alternative model which assumed that the difference-strength extracted from a given stimulus pair is the absolute value of a normal random variable. We found that the same motor-related regions were strongly correlated with bias calculated using the alternative model.
- ^http://www.bic.mni.mcgill.ca/ServicesAtlases/ICBM152NLin2009
- ^http://afni.nimh.nih.gov/afni
References
Argall, B. D., Saad, Z. S., and Beauchamp, M. S. (2006). Simplified intersubject averaging on the cortical surface using SUMA. Hum. Brain Mapp. 27, 14–27.
Avants, B., Anderson, C., Grossman, M., and Gee, J. C. (2007). Spatiotemporal normalization for longitudinal analysis of gray matter atrophy in frontotemporal dementia. Med. Image Comput. Comput. Assist. Interv. 10(Pt 2), 303–310.
Avants, B., Duda, J. T., Kim, J., Zhang, H., Pluta, J., Gee, J. C., and Whyte, J. (2008). Multivariate analysis of structural and diffusion imaging in traumatic brain injury. Acad. Radiol. 15, 1360–1375.
Avants, B., and Gee, J. C. (2004). Geodesic estimation for large deformation anatomical shape averaging and interpolation. Neuroimage 23, 139–150.
Avants, B. B., Yushkevich, P., Pluta, J., Minkoff, D., Korczykowski, M., Detre, J., and Gee, J. C. (2010). The optimal template effect in hippocampus studies of diseased populations. Neuroimage 49, 2457–2466.
Baker, E., Blumstein, S. E., and Goodglass, H. (1981). Interaction between phonological and semantic factors in auditory comprehension. Neuropsychologia 19, 1–15.
Bever, T. G., and Poeppel, D. (2010). Analysis by synthesis: a (re-) emerging program of research for language and vision. Biolinguistics 4, 174–200.
Binder, J. R., Liebenthal, E., Possing, E. T., Medler, D. A., and Ward, B. D. (2004). Neural correlates of sensory and decision processes in auditory object identification. Nat. Neurosci. 7, 295–301.
Bishop, D. V., Brown, B. B., and Robson, J. (1990). The relationship between phoneme discrimination, speech production, and language comprehension in cerebral-palsied individuals. J. Speech Hear. Res. 33, 210–219.
Blumstein, S. E. (1995). “The neurobiology of the sound structure of language,” in The Cognitive Neurosciences, ed. M. S. Gazzaniga (Cambridge, MA: The MIT Press), 915–929.
Buchsbaum, B. R., Baldo, J., Okada, K., Berman, K. F., Dronkers, N., D’Esposito, M., and Hickok, G. (2011). Conduction aphasia, sensory-motor integration, and phonological short-term memory – an aggregate analysis of lesion and fMRI data. Brain Lang. 119, 119128.
Buchsbaum, B. R., Olsen, R. K., Koch, P., and Berman, K. F. (2005a). Human dorsal and ventral auditory streams subserve rehearsal-based and echoic processes during verbal working memory. Neuron 48, 687–697.
Buchsbaum, B. R., Olsen, R. K., Koch, P. F., Kohn, P., Kippenhan, J. S., and Berman, K. F. (2005b). Reading, hearing, and the planum temporale. Neuroimage 24, 444–454.
Callan, D. E., Jones, J. A., Callan, A. M., and Akahane-Yamada, R. (2004). Phonetic perceptual identification by native- and second-language speakers differentially activates brain regions involved with acoustic phonetic processing and those involved with articulatory-auditory/orosensory internal models. Neuroimage 22, 1182–1194.
Christen, H. J., Hanefeld, F., Kruse, E., Imhäuser, S., Ernst, J. P., and Finkenstaedt, M. (2000). Foix-Chavany-Marie (anterior operculum) syndrome in childhood: a reappraisal of Worster-Drought syndrome. Dev. Med. Child Neurol. 42, 122–132.
D’Ausilio, A., Bufalari, I., Salmas, P., and Fadiga, L. (2011). The role of the motor system in discriminating normal and degraded speech sounds. Cortex.
D’Ausilio, A., Pulvermuller, F., Salmas, P., Bufalari, I., Begliomini, C., and Fadiga, L. (2009). The motor somatotopy of speech perception. Curr. Biol. 19, 381–385.
Davis, M. H., and Johnsrude, I. S. (2003). Hierarchical processing in spoken language comprehension. J. Neurosci. 23, 3423–3431.
Desai, R., Liebenthal, E., Possing, E. T., Waldron, E., and Binder, J. R. (2005). Volumetric vs. surface-based alignment for localization of auditory cortex activation. Neuroimage 26, 1019–1029.
Fadiga, L., and Craighero, L. (2006). Hand actions and speech representation in Broca’s area. Cortex 42, 486–490.
Fadiga, L., Craighero, L., Buccino, G., and Rizzolatti, G. (2002). Speech listening specifically modulates the excitability of tongue muscles: a TMS study. Eur. J. Neurosci. 15, 399–402.
Galantucci, B., Fowler, C. A., and Turvey, M. T. (2006). The motor theory of speech perception reviewed. Psychon. B. Rev. 13, 361–377.
Gallese, V., Fadiga, L., Fogassi, L., and Rizzolatti, G. (1996). Action recognition in the premotor cortex. Brain 119, 593–609.
Gold, J. I., and Shadlen, M. N. (2007). The neural basis of decision making. Annu. Rev. Neurosci. 30, 535–574.
Goodglass, H., Kaplan, E., and Barresi, B. (2001). The Assessment of Aphasia and Related Disorders. Philadelphia: Lippincott Williams & Wilkins.
Hasson, U., Skipper, J. I., Nusbaum, H. C., and Small, S. L. (2007). Abstract coding of audiovisual speech: beyond sensory representation. Neuron 56, 1116–1126.
Hernández, A., Zainos, A., and Romo, R. (2000). Neuronal correlates of sensory discrimination in the somatosensory cortex. Proc. Natl. Acad. Sci. U.S.A. 97, 6191–6196.
Hernández, A., Zainos, A., and Romo, R. (2002). Temporal evolution of a decision-making process in medial premotor cortex. Neuron 33, 959–972.
Hickok, G. (2009). Eight problems for the mirror neuron theory of action understanding in monkeys and humans. J. Cogn. Neurosci. 21, 1229–1243.
Hickok, G., Buchsbaum, B., Humphries, C., and Muftuler, T. (2003). Auditory–motor interaction revealed by fMRI: speech, music, and working memory in area spt. J. Cogn. Neurosci. 15, 673–682.
Hickok, G., Costanzo, M., Capasso, R., and Miceli, G. (2011). The role of Broca’s area in speech perception: evidence from aphasia revisited. Brain Lang. 119, 214–220.
Hickok, G., Okada, K., Barr, W., Pa, J., Rogalsky, C., Donnelly, K., Barde, L., and Grant, A. (2008). Bilateral capacity for speech sound processing in auditory comprehension: evidence from Wada procedures. Brain Lang. 107, 179–184.
Klein, A., Andersson, J., Ardekani, B. A., Ashburner, J., Avants, B., Chiang, M. C., Christensen, G. E., Collins, D. L., Gee, J., Hellier, P., Song, J. H., Jenkinson, M., Lepage, C., Rueckert, D., Thompson, P., Vercauteren, T., Woods, R. P., Mann, J. J., and Parsey, R. V. (2009). Evaluation of 14 nonlinear deformation algorithms applied to human brain MRI registration. Neuroimage 46, 786–802.
Klein, A., Ghosh, S. S., Avants, B., Yeo, B. T. T., Fischl, B., Ardekani, B., Gee, J. C., Mann, J. J., and Parsey, R. V. (2010). Evaluation of volume-based and surface-based brain image registration methods. Neuroimage 51, 214–220.
Lenneberg, E. H. (1962). Understanding language without ability to speak: a case report. J. Abnorm. Soc. Psychol. 65, 419–425.
Levine, D. N., and Mohr, J. P. (1979). Language after bilateral cerebral infarctions: role of the minor hemisphere in speech. Neurology 29, 927–938.
Macmillan, N. A., and Creelman, C. D. (2005). Detection Theory: A User’s Guide. Mahwah, NJ: Erlbaum.
Meister, I. G., Wilson, S. M., Deblieck, C., Wu, A. D., and Iacoboni, M. (2007). The essential role of premotor cortex in speech perception. Curr. Biol. 17, 16921696.
Miceli, G., Gainotti, G., Caltagirone, C., and Masullo, C. (1980). Some aspects of phonological impairment in aphasia. Brain Lang. 11, 159–169.
Moineau, S., Dronkers, N. F., and Bates, E. (2005). Exploring the processing continuum of single-word comprehension in aphasia. J. Speech Lang. Hear. Res. 48, 884–896.
Möttönen, R., and Watkins, K. E. (2009). Motor representations of articulators contribute to categorical perception of speech sounds. J. Neurosci. 29, 9819–9825.
Pulvermüller, F., and Fadiga, L. (2010). Active perception: sensorimotor circuits as a cortical basis for language. Nat. Rev. Neurosci. 11, 351–360.
Pulvermüller, F., Huss, M., Kherif, F., Moscoso del Prado Martin, F., Hauk, O., and Shtyrov, Y. (2006). Motor cortex features maps articulatory of speech sounds. Proc. Natl. Acad. Sci. U.S.A. 103, 7865–7870.
Rizzolatti, G., and Craighero, L. (2004). The mirror-neuron system. Annu. Rev. Neurosci. 27, 169–192.
Rogalsky, C., Love, T., Driscoll, D., Anderson, S. W., and Hickok, G. (2011). Are mirror neurons the basis of speech perception? Evidence from five cases with damage to the purported human mirror system. Neurocase 17, 178–187.
Romo, R., Hernández, A., Zainos, A., and Salinas, E. (1998). Somatosensory discrimination based on cortical microstimulation. Nature 392, 387.
Romo, R., Hernández, A., and Zainos, A. (2004). Neuronal correlates of a perceptual decision in ventral premotor cortex. Neuron 41, 165–173.
Romo, R., Hernández, A., Zainos, A., Brody, C. D., and Lemus, L. (2000). Sensing without touching: psychophysical performance based on cortical microstimulation. Neuron 26, 273–278.
Salinas, E., Hernández, A., Zainos, A., and Romo, R. (2000). Periodicity and firing rate as candidate neural codes for the frequency of vibrotactile stimuli. J. Neurosci. 20, 5503–5515.
Sato, M., Grabski, K., Glenberg, A. M., Brisebois, A., Basirat, A., Ménard, L., and Cattaneo, L. (2011). Articulatory bias in speech categorization: evidence from use-induced motor plasticity. Cortex 47, 1001–1003.
Sato, M., Tremblay, P., and Gracco, V. L. (2009). A mediating role of the premotor cortex in phoneme segmentation. Brain Lang. 111, 1–7.
Shahin, A. J., Bishop, C. W., and Miller, L. M. (2009). Neural mechanisms for illusory filling-in of degraded speech. Neuroimage 44, 1133–1143.
Skipper, J. I., Nusbaum, H. C., and Small, S. L. (2005). Listening to talking faces: motor cortical activation during speech perception. Neuroimage 25, 76–89.
Skipper, J. I., van Wassenhove, V., Nusbaum, H. C., and Small, S. L. (2007). Hearing lips and seeing voices: how cortical areas supporting speech production mediate audiovisual speech perception. Cereb. Cortex 17, 2387–2399.
Venezia, J. H., and Hickok, G. (2009). Mirror neurons, the motor system and language: from the motor theory to embodied cognition and beyond. Lang. Linguist. Compass 3, 1403–1416.
Watkins, K. E., Strafella, A. P., and Paus, T. (2003). Seeing and hearing speech excites the motor system involved in speech production. Neuropsychologia 41, 989–994.
Weller, M. (1993). Anterior opercular cortex lesions cause dissociated lower cranial nerve palsies and anarthria but no aphasia: Foix-Chavany-Marie syndrome and “automatic voluntary dissociation” revisited. J. Neurol. 240, 199–208.
Wilson, S. M., and Iacoboni, M. (2006). Neural responses to non-native phonemes varying in producibility: evidence for the sensorimotor nature of speech perception. Neuroimage 33, 316–325.
Wilson, S. M., Saygin, A. P., Sereno, M. I., and Iacoboni, M. (2004). Listening to speech activates motor areas involved in speech production. Nat. Neurosci. 7, 701–702.
Keywords: signal detection, response bias, speech perception, fMRI
Citation: Venezia JH, Saberi K, Chubb C and Hickok G (2012) Response bias modulates the speech motor system during syllable discrimination. Front. Psychology 3:157. doi: 10.3389/fpsyg.2012.00157
Received: 22 November 2011; Accepted: 30 April 2012;
Published online: 28 May 2012.
Edited by:
Claude Alain, Rotman Research Institute, CanadaReviewed by:
Jochen Kaiser, Johann Wolfgang Goethe University, GermanyChristophe Micheyl, University of Minnesota, USA
Copyright: © 2012 Venezia, Saberi, Chubb and Hickok. This is an open-access article distributed under the terms of the Creative Commons Attribution Non Commercial License, which permits non-commercial use, distribution, and reproduction in other forums, provided the original authors and source are credited.
*Correspondence: Gregory Hickok, Department of Cognitive Sciences, University of California Irvine, 3151 Social Sciences Plaza, Irvine, CA 92697, USA. e-mail:Z3JlZy5oaWNrb2tAdWNpLmVkdQ==