- 1 Cognitive Neuroscience Group, Department of Neurology, Brain Imaging Center, Goethe University, Frankfurt, Germany
- 2 Magnetoencephalography-Unit, Brain Imaging Center, Goethe University, Frankfurt, Germany
Speech production involves widely distributed brain regions. This MEG study focuses on the spectro-temporal dynamics that contribute to the setup of this network. In 21 participants performing a cue-target reading paradigm, we analyzed local oscillations during preparation for overt and covert reading in the time-frequency domain and localized sources using beamforming. Network dynamics were studied by comparing different dynamic causal models of beta phase coupling in and between hemispheres. While a broadband low frequency effect was found for any task preparation in bilateral prefrontal cortices, preparation for overt speech production was specifically associated with left-lateralized alpha and beta suppression in temporal cortices and beta suppression in motor-related brain regions. Beta phase coupling in the entire speech production network was modulated by anticipation of overt reading. We propose that the processes underlying the setup of the speech production network connect relevant brain regions by means of beta synchronization and prepare the network for left-lateralized information routing by suppression of inhibitory alpha and beta oscillations.
Introduction
Speech production is one of the most complex human motor acts as it bases upon linguistic processing but further requires precise sensorimotor integration. While linguistic processing involves multiple steps of abstract cognition (Indefrey and Levelt, 2004), the sensorimotor component of speech production relies on feedforward motor plans that are updated by integration of auditory and somatosensory feedback (Hickok, 2012). It is thus not surprising that a large network including prefrontal, motor, somatosensory, auditory, and associative regions has been linked with overt articulation (Price, 2010; Kell et al., 2011; Llorens et al., 2011). When studying speech, sensorimotor processing is more easily accessible than linguistic processing given the measurable physical auditory and motor signal properties. It is thus ideally suited to study spectro-temporal dynamics of large scale brain networks. Evidence is accumulating that specific spectro-temporal properties of brain regions and networks contribute to the generation of complex motor behavior (Roelfsema et al., 1997; Engel and Fries, 2010; Wang, 2010). However, little is known about the spectro-temporal dynamics and interactions underlying efficient sensorimotor mapping. During speaking, the parameters of interest are not easily accessible with standard imaging methods with high temporal resolution like EEG or MEG because of the artifacts induced by ocular and articulator movements during ongoing speech production. One approach to circumvent this problem is to study brain dynamics of preparation for speech production. As for other motor acts, motor preparation for speech production has been related to beta suppression in motor cortex (Salmelin et al., 2000; Saarinen et al., 2006). These processes could reflect covert feedforward processing prior to execution induced by a start signal and thus could serve as a model for the feedforward component of speech production.
In contrast, feedback is necessarily only produced after speech onset and thus much more difficult to study. During monkey vocalizations, suppression of activity in auditory cortices has been linked to feedback integration (Eliades and Wang, 2008). Ongoing speech production has been studied with electrocorticography, a method that is not affected by movement artifacts. By studying high gamma power and its temporal evolution during task epochs, these studies confirmed suppression of auditory cortex activity during speech production (Towle et al., 2008; Edwards et al., 2010; Flinker et al., 2010) associated with a very complex pattern of frontotemporal functional connectivity (Korzeniewska et al., 2011). Instead of studying the speech production network during active processing, another way of approaching this large scale brain network is to investigate how this network is set up. This offers the opportunity to investigate the effects of top-down implementation of task rules (Dosenbach et al., 2006; Sakai and Passingham, 2006). We hypothesize that processes termed “task set” induce recruitment of all necessary brain regions and thus increase functional connectivity within the task network. Here, we refer to these processes as cognitive planning and study them using cue-target paradigms that allow their separation from linguistic processing, motor preparation, and execution. In a cue-target reading paradigm involving covert and overt reading during fMRI, we demonstrated previously that the entire speech production network pre-activates in anticipation of linguistic stimulus material for articulation (Kell et al., 2011). Importantly, this intention to speak left-lateralizes auditory and somatosensory cortices for subsequent feedback processing. This lateralized anticipatory preactivation of auditory cortex could reflect integration of the auditory cortex into the speech network prior to a relative deactivation during ongoing feedback integration. On the one hand this supports the idea that the entire network is set up and ready for input while on the other hand these results emphasize the important contribution of sensory cortices to left-lateralization of speech production (Morillon et al., 2010; Kell et al., 2011). Taken together, these data suggest that important properties of speech production like left-lateralization can be studied by focusing on the setup of this network.
Both local oscillations and network properties of the setup of the speech production network have not been studied yet. So far, only the introspective evaluation of the intention to speak was studied with MEG (Carota et al., 2010). We expected to find task-specific changes in local oscillations in previously defined regions of the speech production network (Hickok and Poeppel, 2007; Price, 2010; Kell et al., 2011; Llorens et al., 2011). The setup of large scale brain networks has previously been related to synchronization in the beta band as demonstrated for fronto-parietal visuomotor networks (Roelfsema et al., 1997). Thus, the aim of our MEG study was twofold: first, to define the role of local oscillations contributing to the intention to speak. Second, to study the speech network’s phase dynamics in the beta band during network setup.
Materials and Methods
Participants
Twenty-six healthy right-handed (Oldfield, 1971) participants (10 female; mean age: 24.0 ± 1.7 years) took part in this MEG study, but data from five participants were discarded due to excessive movement or blink artifacts (less than 20 valid trials per condition). The study was approved by the local ethics committee (Goethe University, Frankfurt am Main, Germany) and informed consent was obtained from each participant.
Stimuli and Design
A cue-target reading paradigm was used to separate cognitive action planning underlying the intention to speak from linguistic processing, motor preparation, and execution (Figure 1).
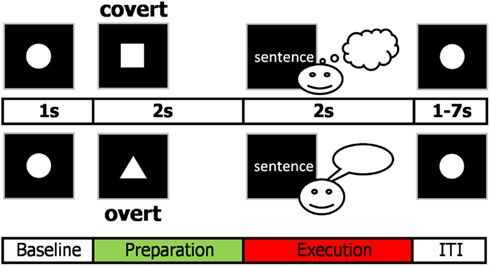
Figure 1. Cue-target paradigm used in the study. The cue indicated how to deal with the upcoming sentence. The square indexed to prepare to read the following target sentence covertly while the triangle indicated to prepare to read the target sentence overtly. The sentence was presented word by word. After each trial, participants fixated for 2–8 s (inter-trial interval, ITI, plus 1 s baseline).
Participants were informed about how to deal with a subsequently presented sentence by a visual cue, i.e., whether to overtly or covertly read the sentence. We studied preparation for overt reading and preparation for covert reading and assumed that comparing these two conditions reveals the anticipatory setup of the sensorimotor speech network. We specifically studied only the artifact-free preparation phase prior to target presentation, meaning that for the overt condition participants knew that they were about to speak but did not know yet the content of the upcoming utterance. Thus, the preparation phase was not contaminated by linguistic and motor processing.
Trials consisted of 2 s visual cue presentation indicating task rules followed by a written sentence as a target (Figure 1). A triangular cue indicated that the subsequent sentence should be read out loud while a rectangular cue designated that the sentence should be read silently. The stimuli consisted of six-word German declarative sentences (“Bunte Fahnen wehen oft im Wind”) and were presented word after word to reduce saccades. The first three words were presented for 400 ms each, the fourth and fifth word for 300 ms each, and the last one for 400 ms to allow fluent speech production. The inter-trial interval (ITI, fixation circle) ranged from 1 to 7 s (mean 4 s) with one additional second used as baseline for analysis. The white stimuli were presented on black background using a projector placed outside the magnetically shielded room. Subjects underwent a training session outside the MEG to make them familiar with the experimental design. During the experiment, participants were asked to fixate and blink little and if possible after target presentation. The whole experiment consisted of four runs (4 × 12.5 min), each containing a total of 40 covert and 40 overt reading trials.
Data Acquisition
Data were acquired using a 275-channel whole-head MEG system (Omega 2005, CTF-MEG, VSM MedTech Inc., Coquitlam, Canada) and recorded continuously at a sampling rate of 1200 Hz in a synthetic third-order axial gradiometer configuration. Participants were supine with their head stabilized by foam cushions. Head position was determined with localization coils fixed at the nasion and the preauricular points. Runs in which head movements exceeded 5 mm were excluded.
Blinks, vertical, and horizontal eye movements were detected by two pairs of electrooculography (EOG) electrodes, placed at the outer canthi of the eyes and below and above the right eye. Another pair of electrodes was placed on the upper brink of the mandible and the lower brink of the maxilla about 2 cm dorsal from the left corner of the mouth to detect mouth movement prior to speech onset (EMG).
After data acquisition, participants underwent a structural T1 MRI scan (magnetization rapid-acquisition gradient echo sequence: 144 slices, 1 slab, TR 2300 ms, voxel size 1 mm × 1 mm × 1 mm, 3 T Siemens Trio) to obtain individual head-geometry for later head-modeling. To co-register MEG and structural data the position of the localization coils was marked with a Vitamin E capsule.
Data Analysis
Data were analyzed using open source Matlab toolboxes SPM81 and Fieldtrip (version 2011-05-312). Standard preprocessing was performed with SPM8. A fifth order butterworth bandpass filter was applied between 2 and 120 Hz. Data of the two different conditions were epoched from −1500 to 2500 ms with respect to cue onset. Data of five dysfunctional channels were discarded (MRO31, MRO21, MRF22, MLT44, MRC12, MRC25). An artifact rejection based on channel thresholding of EOG- and EMG-channels was applied to the epoched data. After artifact rejection, on average 95 valid overt trials and 88 valid covert trials per participant were analyzed.
Three different analyses were performed. First, we analyzed sensor-level data. For time-frequency analyses of the entire time window from −1500 to 2500 ms, Fieldtrip multitapers implemented in SPM8 using a frequency resolution of 2.5 Hz, a time window of 800 ms resulting in three multitapers per frequency, and time steps of 50 ms were used to obtain frequency and time resolution. Data were baseline-corrected with the power in the interval from −1000 to 0 ms before averaging over trials and rescaled using the log ratio transformation. The first 1000 ms of task preparation were analyzed. We calculated statistics of the contrast between the two conditions (preparation for overt and preparation for covert reading) against baseline and of the two conditions against each other to obtain task-specific effects in Fieldtrip. A randomization test with a cluster-based threshold correction method (Maris and Oostenveld, 2007) was applied. A Monte Carlo cluster p-value below 5% (two-tailed testing) was considered significant. To dissociate oscillations from broad band effects we analyzed the frequency spectrum by calculating the powermap with fieldtrip multitapers implemented in SPM8 MEG tools with a frequency resolution of 1 Hz. Finally, to display channel-average time-frequency charts of multitaper results, anterior sensors were separately averaged from posterior sensors.
The second analysis localized the underlying sources producing the effects observed on the sensor-level. The data were co-registered with individual structural scans and the forward model (single shell) was computed using SPM8’s 3D source reconstruction. To transform the two dimensional scalp effects into a three dimensional space the linearly constrained minimum variance (LCMV) beamformer (Van Veen et al., 1997) implemented in SPM8 was used. Effects in the canonical frequency bands (delta/theta 2–6 Hz, alpha 7–13 Hz, and beta 14–30 Hz) were source-localized. Because no significant gamma effect was observed on sensor-level, this band was not further analyzed on source level. The beamformer with 5% regularization was applied to the first second of the cue period and 1 s of baseline using common filters. Conditions were contrasted against baseline or against each other in each single participant and results were analyzed at the group level using one-sample t-tests thresholded at p < 0.05, FWE cluster corrected at a voxel level of p < 0.001. To test for effects of lateralized brain activity the lateralization index (LI) toolbox was used (Wilke and Lidzba, 2007). This toolbox compares activity in anatomically defined brain regions and calculates a LI on the basis of cluster size and extent. By testing multiple statistical thresholds the consistency of lateralization is tested and considered significant if there is a threshold-independent lateralization.
The third analysis focused on the interaction of brain regions in the beta band. Given that beta synchronization has been related to the setup of large scale networks (Roelfsema et al., 1997), we studied phase relationships in this frequency range (neglecting other frequency bands) between selected bilateral regions of the speech production network from 300 to 1000 ms (Hickok and Poeppel, 2007; Price, 2010; Kell et al., 2011; Llorens et al., 2011). Because this study focuses on sensorimotor aspects of speech production the models comprised sources found previously in two fMRI studies that used a similar cue-target reading paradigm (Kell et al., 2011; Keller and Kell, in preparation): the articulatory motor cortex (M1) and two sensorimotor regions: the supplementary motor area (SMA), the lateral dorsal premotor cortex (dPMC), and the sylvian parietotemporal area (SPT) (Hickok et al., 2011). We used the posterior superior temporal sulcus (pSTS) as sensory region instead of the auditory cortex proper because of its relatively larger distance to the other sources.
To inform our analysis by biologically plausible models of oscillatory neural dynamics we used dynamic causal modeling (DCM) for phase coupling in SPM8 (Penny et al., 2009; Litvak et al., 2011). This DCM implements a network of weakly coupled oscillators, representing brain areas, in the form of differential equations that form a generative model of the observed oscillatory activity. The generative model is completed by observation equations that describe how the signal from such an oscillatory source is seen by the sensors. This observation equation is determined by the physical properties of the head model, the MEG device, and the observed signal property under investigation – which were the phases of the oscillators in our case. The system of coupled differential equations for the oscillators describes the coupling in terms of coupling constants, one constant per frequency, and for each modeled directed link between two brain areas.
For model selection, various models that differ in their coupling constants between network nodes are compared in terms of their Bayesian model evidence. The model evidence here serves as a way to assess the fit of the model to the data, penalized for the number of parameters. We performed model selection as follows: first, we tested the anatomical models for unidirectional (top-down or bottom-up coupling) and bidirectional phase coupling in the beta band within hemispheres (data from both conditions combined). Second, models with different phase coupling between hemispheres were compared. Third, we tested the model that explained the data best in step two for modulation of phase coupling in the beta band by overt speech production and allowed connections to be modulated (top-down, bottom-up, both) or un-modulated. In total, we estimated 13 biologically plausible models (Figure 2) in each single subject and compared model probabilities on the group level using a random effects Bayesian model selection procedure (Boly et al., 2011).
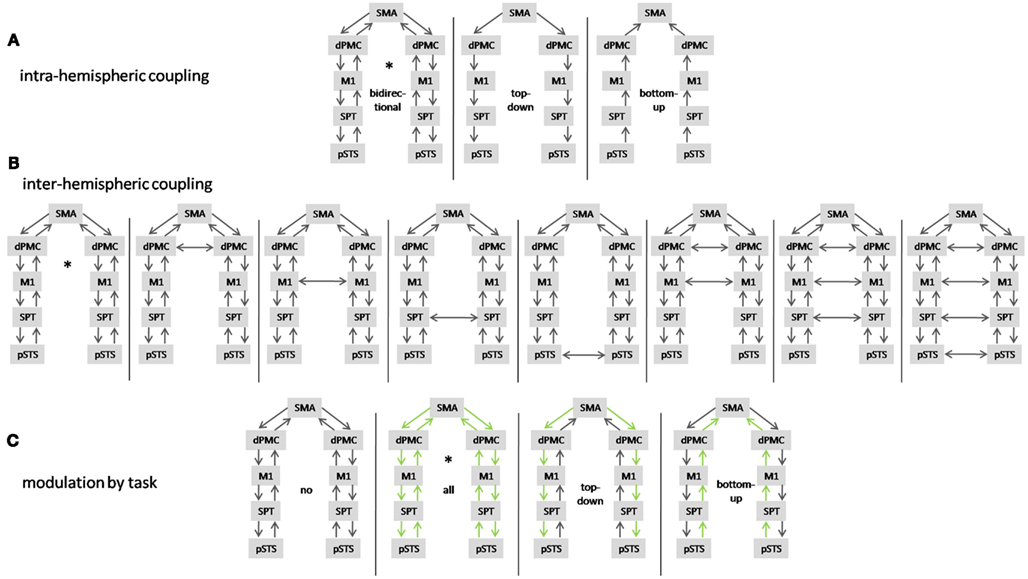
Figure 2. Summary of tested DCMs. (A) Models with unidirectional (top-down, bottom-up) and bidirectional coupling. (B) Models with different interhemispheric coupling and (C) models with different coupling modulated by preparation for overt vs. preparation for covert reading (in green). The model that explains the data best is marked by an asterisk.
Behavioral Study
We investigated the temporal evolution of setting up a speech production task set in a behavioral study on 84 healthy young participants (13 female, mean age: 27 years). Participants were visually cued to read the upcoming sentence covertly, overtly with normal, or overtly with happy intonation. These three different tasks were necessary to create enough variability for changing task sets and to show that task set effects were stable even for different speech production tasks. The key parameter of this study was varying instruction delay between 330, 670, and 1000 ms. In total, 72 sentences were presented. The presentation of the sentence was accompanied by presentation of a tone. Speech recordings of the two overt conditions have been analyzed using Adobe Audition (San Jose, USA) by calculating the voice onset time from sentence presentation (indicated by the tone) to the first occurrence of speech. Statistical analyses (ANOVA with post hoc t-tests using Bonferroni correction resulting in an alpha of 0.00278) have been performed using SPSS (IBM, Markham, Canada).
Results
Preparation for overt or covert speaking was not associated with mouth movements. When analyzing the classical frequency bands, anterior sensors exhibited a condition-independent power increase ranging from delta to beta frequencies while posterior sensors showed suppression of alpha and beta power. No gamma effect was observed (Figure 3).
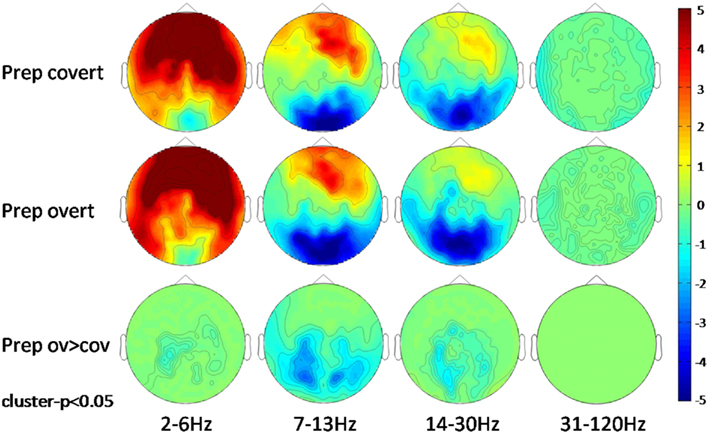
Figure 3. Scalp-frequency plots of the first second of the preparation (Prep) period for covert and overt speech production vs. baseline and for preparation for overt vs. preparation for covert speech production. Pseudo t-values are color-coded from −5 to 5. Results are cluster corrected (p < 0.05).
The power spectrum revealed an anterior broadband effect (2–15 Hz) that was only masked by the anterior alpha peak (Figure 4). In posterior sensors, alpha and beta oscillations were suppressed for both conditions. Preparation-related effects were sustained in time (Figure 5). However, differences between conditions built up only around 350 ms after cue presentation. Preparation for overt reading was associated with a pronounced alpha and beta suppression. Interestingly, this effect was particularly strong at posterior sensors while anterior sensors did not discriminate as well between conditions (Figure 5).
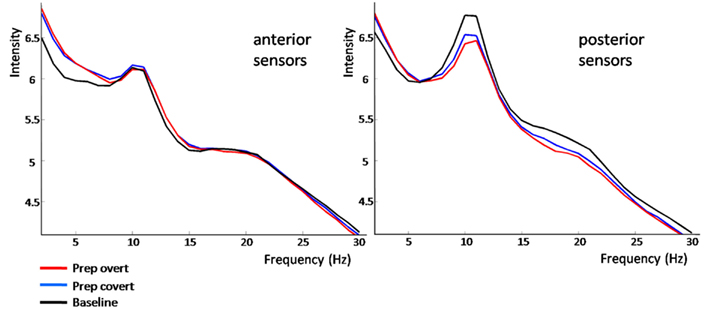
Figure 4. Frequency-power-spectrum averaged over anterior and posterior sensors, separately. Normalized power in frequencies from 2 to 30 Hz is plotted against intensity (arbitrary units) separately for baseline (black), preparation for overt (red), and covert reading (blue). For details please see Section “Results.”
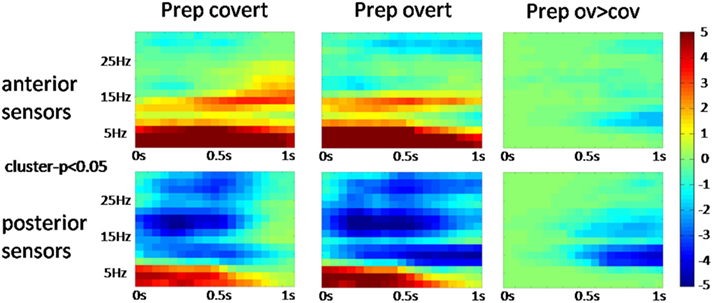
Figure 5. Time-frequency plots (cluster corrected p < 0.05) over averaged anterior and posterior sensors for the different conditions. Task preparation is associated with sustained activity changes whereas the differences between conditions emerge about 350 ms after cue presentation. Pseudo t-values are color-coded from −5 to 5.
The broadband power increase from 2 to 15 Hz observed for both conditions at anterior sensors localized to the bilateral prefrontal cortex (not shown). Even when source-localizing the broadband effect outside the alpha peak, a large overlap of prefrontal delta/theta (Table 1) and 11–15 Hz (Table 2) sources was observed (Figure 6).
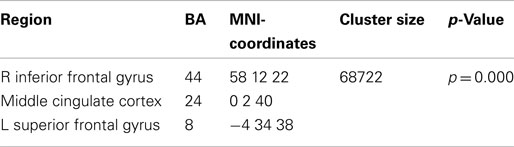
Table 1. Local maxima of sources showing a power increase (delta/theta band) for preparation for overt reading vs. baseline.
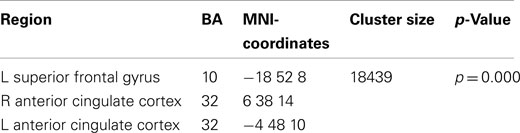
Table 2. Local maxima of sources showing a power increase (11–15 Hz) for preparation for overt reading vs. baseline.
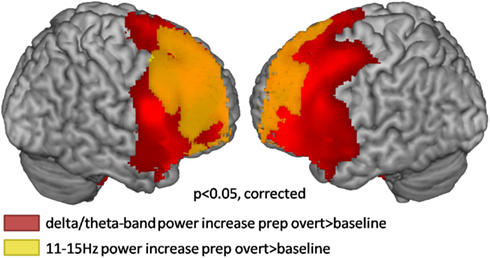
Figure 6. Source localization of broadband low frequency effects during the first second of preparation for overt reading compared to baseline (cluster corrected p < 0.05). Warm colors represent power increases.
Condition-independent decreases in alpha and beta power localized to posterior brain regions in the parietal, occipital, and temporal lobe (not shown).
Preparation for overt speech production compared to preparation for covert speech production revealed pronounced alpha suppression (Figure 7; Table 3) of the left auditory cortex (planum temporale), left superior and middle temporal cortex, the SMA, and the middle occipital gyrus.
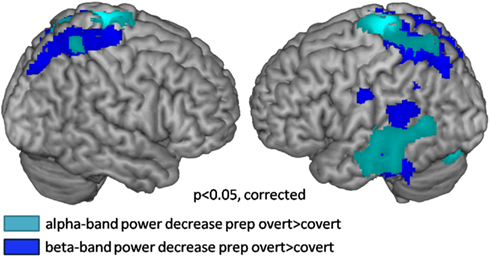
Figure 7. Source localization of oscillatory power changes in the alpha and beta bands over 1 s for the contrast preparation for overt vs. covert reading (cluster corrected p < 0.05). Cool colors represent power decreases.
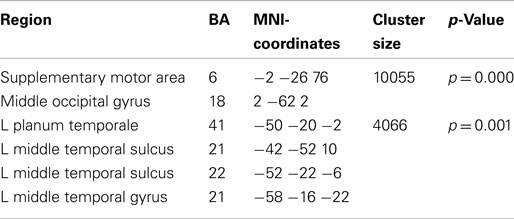
Table 3. Local maxima of sources showing a power decrease (alpha band) for preparation for overt vs. covert reading.
Lateralization of alpha suppression was most robust in the temporal lobe (LI = 0.4) followed by frontocentral cortices (LI = 0.2). The posterior beta decrease associated with the intention to speak localized (Figure 7; Table 4) to the left articulatory motor cortex, left area SPT, left superior and inferior temporal gyrus, and bilateral superior parietal cortex. The strongest left-lateralization of beta suppression was found in temporal lobe (LI = 0.5) followed by frontocentral (LI = 0.4) and parietal cortices (LI = 0.25). It is interesting to note that the decrease in alpha and beta power regionally overlapped in some, but not all regions of the speech production network. While both left mid- and inferior temporal and parietal regions showed parallel changes in alpha and beta power, the auditory cortex and the SMA showed only alpha suppression. Isolated beta suppression was observed in the articulatory motor cortex and area SPT.
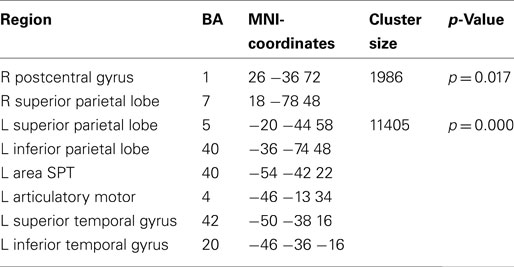
Table 4. Local maxima of sources showing a power decrease (beta band) for preparation for overt vs. covert reading.
Comparison of master-slave models against models with bidirectional coupling revealed that the speech production network is bidirectionally coupled in beta phase within hemispheres (Figure 8A). Assuming bidirectional coupling between hemispheres, the model with coupling between the SMA and bilateral dPMC, but no other interhemispheric coupling, was selected by Bayesian model comparison (Figure 8B). In all network connections, beta phase coupling was modulated by preparation for overt reading (Figure 8C).
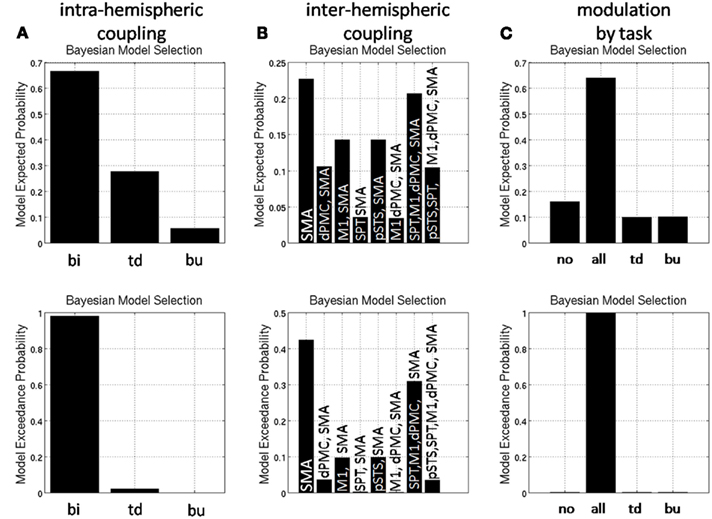
Figure 8. Random effects Bayesian model selection of (A) models with unidirectional [top-down (td), bottom-up (bu)] and bidirectional (bi) coupling and (B) models comparing different interhemispheric coupling (for abbreviations please see text) and (C) models with different modulatory task effects [no modulation by task (no), modulation of top-down and bottom-up coupling (all)].
Discussion
The setup of the speech production network was associated with a sustained reduction in oscillatory alpha and beta power that occurred about 350 ms after cue presentation. These changes were primarily left-lateralized, particularly in temporal cortex. While auditory regions showed stronger suppression and lateralization of alpha power, the auditory-motor region area SPT, and the articulatory motor cortex showed primarily suppressed beta power when speaking was anticipated. Note that alpha suppression extended beyond sensory cortices: we observed this sustained power decrease also in mid-temporal regions related to phonology like the superior temporal sulcus (Hickok and Poeppel, 2007) and also in the SMA. Changes in both alpha and beta oscillations have previously been shown to be behaviorally relevant (Hammond et al., 2007; Dugue et al., 2011). Our data indicate that cognitive planning prior to actual sensory or motor processing could contribute to such changes in behavior. Those findings suggest that preparation of the speech production network extends beyond parietal regions that have previously been related to the conscious introspection of the intention to speak (Carota et al., 2010).
Alpha oscillations are thought to rhythmically inhibit sensory cortices (Jensen and Mazaheri, 2010; Klimesch et al., 2011). Auditory cortex activation has been related to alpha suppression (Hartmann et al., 2012). The observed alpha suppression in sensory, associative, and premotor regions in this study could tentatively be interpreted as an active disinhibition of task-relevant regions. This parallels findings of increased preparatory BOLD activity in auditory cortex during the intention to speak (Kell et al., 2011) given that alpha oscillation amplitude and BOLD fMRI activity are typically anti-correlated (Brookes et al., 2005).
Sustained beta power increase has been related to maintenance of a network in its status quo (Engel and Fries, 2010). The observation of beta suppression in motor-related regions could indicate a preparation for a change in the motor system (Saleh et al., 2010). Importantly, this already occurs before movement or even specific motor preparation is possible. Thus, beta suppression very likely plays an additional role to the one previously suggested in the framework of motor preparation. Whether beta oscillations play an equivalent inhibitory role in the motor system (Hammond et al., 2007; Engel and Fries, 2010) compared to alpha oscillations in more sensory regions, remains open to further research.
An important limitation of our study is the use of an explicit (overt) and an implicit (covert) condition. We thus cannot exclude that condition-specific effects are partly related to different levels of difficulty and attention. A study investigating the interaction of attentional and task factors could not reveal a modulation of speech network activity by task difficulty or attentional demand (Keller and Kell, in preparation).
Although beta power is suppressed in several nodes of the speech production network, these brain regions mutually increase their beta phase coupling in anticipation of overt reading. Beta band synchronicity has previously been related to coupling in large scale networks like the frontoparieto-occipital visuomotor network (Roelfsema et al., 1997). We extend this finding to the speech production network. Interestingly, we did not detect hierarchical asymmetry in coupling strength between top-down and bottom-up connections, suggesting that beta synchronicity may indeed play an important role for the setup of the network prior to stimulus related processing. It is conceivable that this pattern changes when ongoing top-down and bottom-up processes take place during execution. Note that our DCMs explain data only after the visual cue was decoded. This is a trial phase after bottom-up processing of the cue has taken place. In our study, the premotor cortex including the dPMC and the SMA connects the left with the right sensorimotor speech system during network setup. The premotor cortex is thus ideally suited to integrate information from both hemispheres. This region has previously been associated with auditory-motor integration (Neef et al., 2011), suggesting that the bilateral premotor cortex integrates sensory feedback into a motor program (Hickok et al., 2011).
The effects specifically related to speech preparation were preceded by condition-independent preparatory effects. An early posterior alpha and beta suppression which likely originated from parietal sources was accompanied by a prefrontal increase in low frequencies ranging from 2 to 15 Hz. Importantly, this low frequency effect was not modulated by specific task content and was thus observed during task preparation both for overt and for covert reading. Given that there is considerable debate on what broad band effects signify, the mechanisms behind such an effect have to be studied in more detail. Such fronto-parietal activity – although unlikely oscillatory in nature – could reflect the executive control of implementing a “task set” (Dosenbach et al., 2006; Sakai and Passingham, 2006). Given that this activity did not differ between conditions, the tasks seem to have required similar amounts of executive control. The observed time courses of this activity suggest that the first 350 ms after cue presentation are used to decode the instruction and to generate the appropriate rules for behavioral control. Changes specific to the set up of the speech production network (sensorimotor alpha and beta suppression) were only detected after 350 ms. This indicates that it takes at least this time to set up this large scale network. To test this claim, we performed an additional behavioral study under the assumption that short instruction delays of less than 350 ms do not allow a proper setup of the speech production network resulting in increased reaction times measured as speech onset after target presentation. Indeed, shortening instruction delays to 330 ms increased reaction time in overt reading tasks (Figure 9). This suggests that this duration does not allow for a proper setup of the speech production network, extending network setup well into the execution phase which in turn delays speech onset. We thus believe that sensorimotor alpha and beta suppression is essential to set up the speech production network.
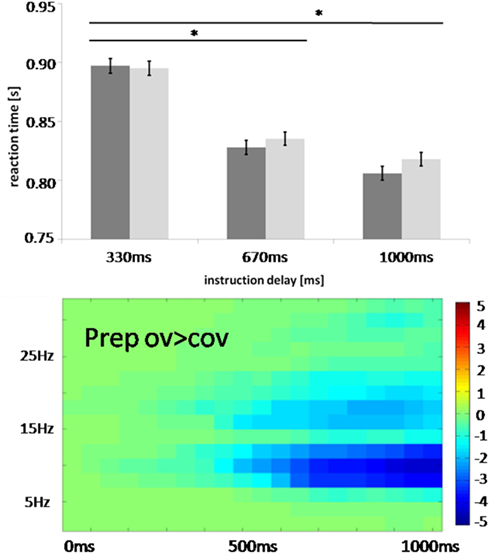
Figure 9. Comparison of behavioral task set effects from 84 participants with the temporal evolution of oscillations in the actual study. Instruction delay between cue and target presentation varied between 330, 670, and 1000 ms. Reaction time was measured as speech onset for neutral (dark gray) and happy intonation (light gray) after target presentation. Error bars indicate standard error, *significance at p < 0.05 corrected. Note that the set up of the speech production network coincides with occurrence of alpha and beta suppression.
Taken together, our findings suggest that the brain sets up the speech production network by means of beta synchronization and prepares the network for left-lateralized information processing by suppression of frontotemporal alpha and beta oscillations.
Conflict of Interest Statement
The authors declare that the research was conducted in the absence of any commercial or financial relationships that could be construed as a potential conflict of interest.
Acknowledgments
We want to thank Christian Keller for analyzing lateralization indices, Lucia Melloni, Cerisa Stawowsky, and Charles Schroeder for their creative comments and help.
Footnotes
References
Boly, M., Garrido, M. I., Gosseries, O., Bruno, M. A., Boveroux, P., Schnakers, C., Massimini, M., Litvak, V., Laureys, S., and Friston, K. (2011). Preserved feedforward but impaired top-down processes in the vegetative state. Science 332, 858–862.
Brookes, M. J., Gibson, A. M., Hall, S. D., Furlong, P. L., Barnes, G. R., Hillebrand, A., Singh, K. D., Holliday, I. E., Francis, S. T., and Morris, P. G. (2005). GLM-beamformer method demonstrates stationary field, alpha ERD and gamma ERS co-localisation with fMRI BOLD response in visual cortex. Neuroimage 26, 302–308.
Carota, F., Posada, A., Harquel, S., Delpuech, C., Bertrand, O., and Sirigu, A. (2010). Neural dynamics of the intention to speak. Cereb. Cortex 20, 1891–1897.
Dosenbach, N. U., Visscher, K. M., Palmer, E. D., Miezin, F. M., Wenger, K. K., Kang, H. C., Burgund, E. D., Grimes, A. L., Schlaggar, B. L., and Petersen, S. E. (2006). A core system for the implementation of task sets. Neuron 50, 799–812.
Dugue, L., Marque, P., and Vanrullen, R. (2011). The phase of ongoing oscillations mediates the causal relation between brain excitation and visual perception. J. Neurosci. 31, 11889–11893.
Edwards, E., Nagarajan, S. S., Dalal, S. S., Canolty, R. T., Kirsch, H. E., Barbaro, N. M., and Knight, R. T. (2010). Spatiotemporal imaging of cortical activation during verb generation and picture naming. Neuroimage 50, 291–301.
Eliades, S. J., and Wang, X. (2008). Neural substrates of vocalization feedback monitoring in primate auditory cortex. Nature 453, 1102–1106.
Engel, A. K., and Fries, P. (2010). Beta-band oscillations – signalling the status quo? Curr. Opin. Neurobiol. 20, 156–165.
Flinker, A., Chang, E. F., Kirsch, H. E., Barbaro, N. M., Crone, N. E., and Knight, R. T. (2010). Single-trial speech suppression of auditory cortex activity in humans. J. Neurosci. 30, 16643–16650.
Hammond, C., Bergman, H., and Brown, P. (2007). Pathological synchronization in Parkinson’s disease: networks, models and treatments. Trends Neurosci. 30, 357–364.
Hartmann, T., Schlee, W., and Weisz, N. (2012). It’s only in your head: expectancy of aversive auditory stimulation modulates stimulus-induced auditory cortical alpha desynchronization. Neuroimage 60, 170–178.
Hickok, G. (2012). Computational neuroanatomy of speech production. Nat. Rev. Neurosci. 13, 135–145.
Hickok, G., Houde, J., and Rong, F. (2011). Sensorimotor integration in speech processing: computational basis and neural organization. Neuron 69, 407–422.
Hickok, G., and Poeppel, D. (2007). The cortical organization of speech processing. Nat. Rev. Neurosci. 8, 393–402.
Indefrey, P., and Levelt, W. J. (2004). The spatial and temporal signatures of word production components. Cognition 92, 101–144.
Jensen, O., and Mazaheri, A. (2010). Shaping functional architecture by oscillatory alpha activity: gating by inhibition. Front. Hum. Neurosci. 4:186. doi:10.3389/fnhum.2010.00186
Kell, C. A., Morillon, B., Kouneiher, F., and Giraud, A. L. (2011). Lateralization of speech production starts in sensory cortices – a possible sensory origin of cerebral left dominance for speech. Cereb. Cortex 21, 932–937.
Klimesch, W., Fellinger, R., and Freunberger, R. (2011). Alpha oscillations and early stages of visual encoding. Front. Psychol. 2:118. doi:10.3389/fpsyg.2011.00118
Korzeniewska, A., Franaszczuk, P. J., Crainiceanu, C. M., Kus, R., and Crone, N. E. (2011). Dynamics of large-scale cortical interactions at high gamma frequencies during word production: event related causality (ERC) analysis of human electrocorticography (ECoG). Neuroimage 56, 2218–2237.
Litvak, V., Mattout, J., Kiebel, S., Phillips, C., Henson, R., Kilner, J., Barnes, G., Oostenveld, R., Daunizeau, J., Flandin, G., Penny, W., and Friston, K. (2011). EEG and MEG data analysis in SPM8. Comput. Intell. Neurosci. 2011, 852961.
Llorens, A., Trebuchon, A., Liegeois-Chauvel, C., and Alario, F. X. (2011). Intra-cranial recordings of brain activity during language production. Front. Psychol. 2:375. doi:10.3389/fpsyg.2011.00375
Maris, E., and Oostenveld, R. (2007). Nonparametric statistical testing of EEG- and MEG-data. J. Neurosci. Methods 164, 177–190.
Morillon, B., Lehongre, K., Frackowiak, R. S., Ducorps, A., Kleinschmidt, A., Poeppel, D., and Giraud, A. L. (2010). Neurophysiological origin of human brain asymmetry for speech and language. Proc. Natl. Acad. Sci. U.S.A. 107, 18688–18693.
Neef, N. E., Jung, K., Rothkegel, H., Pollok, B., Von Gudenberg, A. W., Paulus, W., and Sommer, M. (2011). Right-shift for non-speech motor processing in adults who stutter. Cortex 47, 945–954.
Oldfield, R. C. (1971). The assessment and analysis of handedness: the Edinburgh inventory. Neuropsychologia 9, 97–113.
Penny, W. D., Litvak, V., Fuentemilla, L., Duzel, E., and Friston, K. (2009). Dynamic causal models for phase coupling. J. Neurosci. Methods 183, 19–30.
Price, C. J. (2010). The anatomy of language: a review of 100 fMRI studies published in 2009. Ann. N. Y. Acad. Sci. 1191, 62–88.
Roelfsema, P. R., Engel, A. K., Konig, P., and Singer, W. (1997). Visuomotor integration is associated with zero time-lag synchronization among cortical areas. Nature 385, 157–161.
Saarinen, T., Laaksonen, H., Parviainen, T., and Salmelin, R. (2006). Motor cortex dynamics in visuomotor production of speech and non-speech mouth movements. Cereb. Cortex 16, 212–222.
Sakai, K., and Passingham, R. E. (2006). Prefrontal set activity predicts rule-specific neural processing during subsequent cognitive performance. J. Neurosci. 26, 1211–1218.
Saleh, M., Reimer, J., Penn, R., Ojakangas, C. L., and Hatsopoulos, N. G. (2010). Fast and slow oscillations in human primary motor cortex predict oncoming behaviorally relevant cues. Neuron 65, 461–471.
Salmelin, R., Schnitzler, A., Schmitz, F., and Freund, H. J. (2000). Single word reading in developmental stutterers and fluent speakers. Brain 123(Pt 6), 1184–1202.
Towle, V. L., Yoon, H. A., Castelle, M., Edgar, J. C., Biassou, N. M., Frim, D. M., Spire, J. P., and Kohrman, M. H. (2008). ECoG gamma activity during a language task: differentiating expressive and receptive speech areas. Brain 131, 2013–2027.
Van Veen, B. D., Van Drongelen, W., Yuchtman, M., and Suzuki, A. (1997). Localization of brain electrical activity via linearly constrained minimum variance spatial filtering. IEEE Trans. Biomed. Eng. 44, 867–880.
Wang, X. J. (2010). Neurophysiological and computational principles of cortical rhythms in cognition. Physiol. Rev. 90, 1195–1268.
Keywords: preparation, task set, alpha band, beta band, phase coupling, auditory cortex, MEG, DCM
Citation: Gehrig J, Wibral M, Arnold C and Kell CA (2012) Setting up the speech production network: how oscillations contribute to lateralized information routing. Front. Psychology 3:169. doi: 10.3389/fpsyg.2012.00169
Received: 27 January 2012; Accepted: 10 May 2012;
Published online: 06 June 2012.
Edited by:
Marcela Pena, Catholic University of Chile, ChileReviewed by:
Tamara Swaab, University of California Davis, USAAntonino Vallesi, La Scuola Internazionale Superiore di Studi Avanzati, Italy
Copyright: © 2012 Gehrig, Wibral, Arnold and Kell. This is an open-access article distributed under the terms of the Creative Commons Attribution Non Commercial License, which permits non-commercial use, distribution, and reproduction in other forums, provided the original authors and source are credited.
*Correspondence: Christian A. Kell, Cognitive Neuroscience Group, Brain Imaging Center, Goethe University, Schleusenweg 2-16, 60528 Frankfurt, Germany. e-mail:Yy5rZWxsQGVtLnVuaS1mcmFua2Z1cnQuZGU=