- 1 Research Group Intuitive Experts, Max Planck Institute for Research on Collective Goods, Bonn, Germany
- 2 Psychology, Duke University, Durham, NC, USA
- 3 The New School of Psychology, Interdisciplinary Center Herzliya, Herzliya, Israel
- 4 School of Social Sciences, University of Mannheim, Mannheim, Germany
Do decisions from description and from experience trigger different cognitive processes? We investigated this general question using cognitive modeling, eye-tracking, and physiological arousal measures. Three novel findings indeed suggest qualitatively different processes between the two types of decisions. First, comparative modeling indicates that evidence-accumulation models assuming averaging of all fixation-sampled outcomes predict choices best in decisions from experience, whereas Cumulative Prospect Theory predicts choices best in decisions from descriptions. Second, arousal decreased with increasing difference in expected value between gambles in description-based choices but not in experience. Third, the relation between attention and subjective weights given to outcomes was stronger for experience-based than for description-based tasks. Overall, our results indicate that processes in experience-based risky choice can be captured by sampling-and-averaging evidence-accumulation model. This model cannot be generalized to description-based decisions, in which more complex mechanisms are involved.
Introduction
According to standards of rationality, choices between risky prospects should depend on the utility of possible outcomes and their respective probabilities. Choices should thus be invariant to different formats of information presentation. Classic work, however, has shown that this invariance assumption is systematically violated: for example, framing effects (e.g., presenting information in terms of gains vs. losses) have a profound effect both on choice behavior (e.g., Tversky and Kahneman, 1981; Kühberger, 1998; Maule and Villejoubert, 2007) and judgments (e.g., Hilbig, 2009, 2012). Recently, there has been an upsurge of interest in the influence of one specific aspect of information presentation, namely whether choice-relevant information is exhaustively described or actively sampled, that is, experienced.
A growing body of research suggests a “gap” between decisions that are based on description and decisions that are based on experience (Barron and Erev, 2003; Hertwig et al., 2004; Erev and Barron, 2005; Yechiam et al., 2005a; Jessup et al., 2008). Indeed, this gap was recently corroborated on a neuronal level (FitzGerald et al., 2010). In description-based risky choice, the outcomes and their respective probabilities are fully described for both options. By contrast, in experience-based decisions, no such conclusive information is provided; rather, participants have to learn which outcomes might occur and what their approximate probabilities are through experience. For example, Barron and Erev (2003) presented the following choice problem to participants: get three points for sure vs. get four points with 0.8 probability, and zero points otherwise. Instead of receiving such a full description of the options, participants were required to make 400 selections between the two gambles by pressing one of two unmarked buttons. Each selection returned an outcome drawn from the underlying payoff structure of the corresponding option. The accumulated outcomes were converted into money and paid to the participants. According to previous findings, participants in an all gain domain should prefer the safer option due to (myopic) risk aversion (e.g., Kahneman and Tversky, 1979). By contrast, Barron and Erev (2003) found a preference for the riskier option (66%) when participants based their choices on experience. This difference between description vs. experience-based decisions concerning the preference for risky options (and other choice phenomena) is considered the descriptions-experience-“gap” (Hertwig and Erev, 2009)1.
While the description-experience “gap” has been found consistently, there still are open questions concerning the underlying cognitive mechanisms (Hertwig and Erev, 2009; Ungemach et al., 2009). In particular, it is unsolved whether choices in both formats are essentially governed by the same processes. Alternatively, and on top of obvious differences resulting from the fact that information might have to be transformed before integration, they might trigger qualitatively distinct cognitive processes. To address these questions we herein test hypotheses concerning the processes underlying one-shot experience-based decisions in comparison to decisions from descriptions.
For an in-depth process analysis we resort to measurement of information sampling using eye-tracking and analyze differences in physiological arousal in response to specific task characteristics. While previous research was limited to the analyses of information sampling for experience-based decisions only (i.e., by looking at button-press behavior), an eye-tracking approach provides insight concerning information sampling in both paradigms. To the best of our knowledge, we are the first to apply fixation-based-sampling models to both experience- and description-based risky choice. In addition, we directly test whether the degree of attention given to outcomes corresponds to their actual probability of occurrence – as is a cornerstone assumption of prominent sampling models for risky choice (Busemeyer and Townsend, 1993; Roe et al., 2001; Johnson and Busemeyer, 2005). Findings from other eye-tracking studies in the description paradigm indicate that there is at least some relation between objective probability and attention in risky choice (Fiedler and Glöckner, submitted) and in the valuations of single gambles (Ashby et al., 2012). Nevertheless, other factors such as outcomes (Ashby et al., 2012; Fiedler and Glöckner, submitted) and emerging preference (Innocenti et al., 2010; Glöckner and Herbold, 2011; Glöckner et al., 2012; Fiedler and Glöckner, submitted) have been shown to influence attention as well (see also Armel et al., 2008; Milosavljevic et al., 2010; Krajbich and Rangel, 2011).
Underweighting and Overweighting of Small Probability Outcomes
One of the main differences between decisions from experience and decisions from descriptions concerns the implications of observed choice behavior for the subjective evaluation of rare events (i.e., outcomes with small probabilities). According to Cumulative Prospect Theory (Kahneman and Tversky, 1979; Tversky and Kahneman, 1992), the most prominent model for risky choice, there should be an overweighting of rare events. By contrast, it has been argued that the choice patterns observed in decision from experience imply that rare events are underweighted (Hertwig et al., 2004; Erev and Barron, 2005; Hertwig and Erev, 2009). Specifically, analyses of choices suggest that in description-based tasks, people behave as if they overweight small probabilities, whereas they behave as if they underweight small probabilities in experience-based tasks. As described above, in description-based tasks, participants mostly (64%) prefer a certain-outcome option with an intermediate expected value (e.g., 100%, 3€) over an option with higher expected value but comprising an undesirable rare event (e.g., 80%, 4€, 20%, 0€); however, they show a reversed pattern in an experience-based task (12% choices for the certain alternative; Hertwig et al., 2004). Since the rare event is undesirable, this is in line with underweighting the probability of rare events in experience-based tasks but overweighting them in description-based tasks. Vice versa, when the rare event was desirable (e.g., 20%, €32, 80% €0), the risky alternative was preferred by the majority of participants in the description-based task, but only by the minority of the participants in the experience-based task.
Moderators and Potential Explanations
Two potential explanations of the description-experience “gap” that were previously proposed are sampling bias and recency effects2. Sampling bias refers to the tendency of individuals to draw small (and thus biased) samples. In Hertwig et al. (2004), for example, participants in the experience condition sampled only a median of 7.5 outcomes per option, even though they could have sampled endlessly without (monetary) costs. As a result, most based their final choice on a biased sample, which contained the rare event less often than its objective probability3. In view of these results and similar findings, some authors have proposed that the description-experience gap is little more than sampling error plus Prospect Theory (Fox and Hadar, 2006), suggesting that “people make equivalent choices when they use equivalent information to base their decision (on), regardless of presentation mode” (Camilleri and Newell, 2011a, p. 282). Indeed, recent studies show that the description-experience gap reduces under conditions in which more representative sampling is induced (e.g., Ungemach et al., 2009; Camilleri and Newell, 2011a) or when large representative samples can be drawn in parallel and very speedily (Hilbig and Glöckner, 2011). However, although the ubiquitous importance of sampling biases is out of question (e.g., Fiedler, 1996, 2008; Fiedler et al., 2000; Kareev and Fiedler, 2006), it has been found that even when individuals draw on large and representative samples the “gap” – though reduced – is not eliminated (Ungemach et al., 2009). Ungemach et al. (2009) argue that sampling bias alone can thus not account for the “gap.”
Recency effects refer to the tendency to focus on events more recently encountered (e.g., Hogarth and Einhorn, 1992). Particularly, only a subset of the most recent samples could be taken into account in choice. Since rare events have a lower probability to be included in these recent samples (simply because they are rare; see also text footnote 3), choices are likely to imply underweighting of these events. However, findings concerning this recency effect are equivocal. Some studies found that the second half of the samples drawn by participants predicted choices better than the first half (Hertwig et al., 2004), while others failed to find evidence for such recency effects (e.g., Ungemach et al., 2009).
Thus, the accumulated empirical evidence suggests that rare events are treated differently in description vs. experience-based decision making (Hau et al., 2010). However, it has been argued that biased sampling and recency cannot fully account for the description-experience gap (Ungemach et al., 2009). As such, knowledge on the mechanisms that contribute to the description-experience gap is incomplete (Hertwig and Erev, 2009; Ungemach et al., 2009; Ludvig and Spetch, 2011) which, in turn, highlights the importance of directly examining underlying processes.
Hertwig and Erev (2009) consider the possibility that the different statistical formats of information presentation (i.e., stated probabilities vs. experienced events) might trigger qualitatively different cognitive processes. The current study aims to identify such qualitative differences in processing, which stand in contrast to obvious differences that merely result from the fact that information has to be transformed in different ways before it can be integrated into a decision. For example, participants may first need to form an estimate of the outcomes’ probabilities in the experience format, but then integrate outcomes and probabilities based on the same cognitive process as participants who are provided with the exact probabilities (in the description format). Therefore, we focus on qualitative differences in terms of information integration. An example would be that in one format participants might rely on deliberately multiplying outcomes and (weighted) probabilities and adding them up whereas in the other format they may rely on automatic processes of memory retrieval in order to decide which option is better.
Theoretical Background and Methodological Approach
Herein, we investigated decisions from the perspective of evidence-accumulation models (e.g., Busemeyer and Townsend, 1993; Roe et al., 2001; Johnson and Busemeyer, 2005; Raab and Johnson, 2007; Armel et al., 2008; Milosavljevic et al., 2010; Pleskac and Busemeyer, 2010), an important class of process models for decision making (see also Rieskamp, 2008; Hilbig and Pohl, 2009; Glöckner and Herbold, 2011; Hilbig and Glöckner, 2011). To better understand the underlying processes of description-based and experience-based decisions, we used a combination of process-tracing techniques, including recording of eye-fixations (via eye-tracking), cognitive modeling, and physiological arousal measurement (indexed by skin conductance response and pupil dilation). Moreover, these measures were used on a set of decisions that were randomly generated and somewhat more complex than in previously used tasks (see also Hilbig and Glöckner, 2011). This simultaneous reliance on multiple measures in a complex set of stimuli extends the scope of previous examinations and enables direct tests of (i) whether individuals indeed treat rare events differently under experience vs. description, and (ii) which types of processing differences contribute to this “gap.”
Eye-fixation can provide important information about the weight (or importance) given to different pieces of information during the decision process (e.g., Raab and Johnson, 2007; Krajbich and Rangel, 2011; Glöckner et al., 2012). Since several evidence-accumulation models suggest that attention to outcomes should be proportional to its importance or subjective probability (Busemeyer and Townsend, 1993; Busemeyer and Johnson, 2004; Johnson and Busemeyer, 2005), eye-fixations can be used to investigate whether there are differences in the visual attention given to the rare events in both paradigms. If individuals overweight rare events, then these events are expected to receive a higher relative proportion of attention as compared to their objective probability. By contrast, if rare events are underweighted, they will receive a lower relative proportion of attention. Our data also allows testing whether overt attention is related to probability of outcomes at all. As mentioned above, some (but not all; see e.g., Armel and Rangel, 2008, for a different approach) evidence-accumulation models predict that attention to an outcome should increase with its probability and predict that “the outcome probabilities dictate where attention shifts, but only the outcome values are used in determining the momentary evaluation” (Johnson and Busemeyer, 2005, p. 843)4.
Cognitive modeling and model comparisons additionally yield insight on how (and with which properties) the underlying processes employed by decision-makers can best be described (e.g., Yechiam and Busemeyer, 2005; Yechiam et al., 2005b; Yechiam and Ert, 2007). For example, evidence-accumulation models assume that individuals repeatedly sample information about the available options, and use these samples to evaluate the options. The sampled information is automatically accumulated in a serial manner, until one option is perceived as sufficiently better than the other, and thus chosen. In the following, we rely on naïve implementations of evidence-accumulation models (i.e., averaging and summing models) to examine whether one-shot choices that are made from description vs. experience can be captured by different process models and how well the models explain behavior overall. Averaging models assume that decision-makers average the sampled outcomes for both alternative, and choose the option with the higher average. By contrast, summing models assume that decision-makers sum the sampled outcomes of each alternative, and choose the option with the higher sum.
In both paradigms “samples of information” were operationalized by the number of eye-fixations to respective outcomes. These models were contrasted with a baseline Expected Value Model and Cumulative Prospect Theory assuming objective probabilities and outcomes of gambles. In the experience condition we additionally tested a strategy assuming that participants chose the option with the highest average outcome based on the subjectively sampled outcomes. In addition, to test the recency account for decisions from experience, this averaging model was also applied using only recent subsets of samples. A previous model comparison by Erev et al. (2010) indicates that (probabilistic implementations of) Cumulative Prospect Theory predict choices best in decisions from descriptions but that the same theory performs poorly in predicting decisions from experience. Decisions from experience, in contrast, were found to be best described by an “Ensemble model” relying on the average prediction of four models including sampling models and Cumulative Prospect Theory.
Finally, potential differences in arousal between descriptions and experience were investigated. We were interested in the influence of the “difficulty” of the decision on arousal in the two paradigms, where difficulty is indicated by the similarity of options in expected values. Previous studies on probabilistic inferences (Hochman et al., 2010) and risky choice (Glöckner and Hochman, 2011) show that arousal increases with increasing conflict between the available information. Generalized to the risky choice paradigm used in the current study, arousal should be high (vs. low) if both gambles are similar concerning their expected value and/or expected utility since “pros” and “cons” for the alternatives are about equally strong in such a case (vs. one alternative being clearly better than the other). Thus, differences in the pattern of arousal for “easy” vs. “difficult” choices between the two paradigms should be an indicator for different underlying processes5. Such a comparison is critically informative concerning the question whether the “gap” is caused by relatively trivial differences in preprocessing of information only. If this were the case, a similar effect of difficulty on arousal would be expected in the experience and the description condition.
Materials and Methods
Participants and Design
Forty-four students from the University of Bonn took part in the experiment (52.3% female, mean age 23 years) and were randomly assigned to the experience or the description condition. We manipulated within-subjects whether the rare event was more or less desirable and whether there was a high or a low difference in expected value (EV-diff) between gambles resulting in a 2 (experience vs. description) × 2 (rare event more vs. less desirable) × 2 (EV-diff low vs. high) mixed design. The experiment lasted about 45 min. Participants were students recruited from the MPI Decision Lab subject pool using the database-system ORSEE (Greiner, 2004). Participants received a show up fee of 5€ plus a performance-contingent payment for the study yielding additional payoffs between 0.1 and 29.8€ (average total: 18.3€ which equals approximately 25.7 USD). The experiment was hence incentivized and there was no deception involved.
Material
Participants made 60 decisions between two gambles with two outcomes each that had an average EV of 10€. In 38 target trials an option comprising a rare event (low-probability outcome) was paired with an option comprising intermediate-probability outcomes only (i.e., between 0.33 and 0.66). The remaining 22 decisions were filler tasks with options comprising intermediate-probability outcomes only (all between 0.33 and 0.66). For 20 of the 38 target decisions, the low-probability outcome was desirable (i.e., the rare outcome was more than twice as large as the non-rare outcome), while for the other 18 target decisions it was undesirable (i.e., the rare outcome was less than half as large as the non-rare outcome). Half of the tasks were constructed so as to yield a small difference in EV between gambles (EV-diff < 0.50€), whereas the other half had a higher difference in EV (i.e., 3€ < EV-diff < 4€). All decisions were randomly generated under the above restrictions using gambles with positive outcomes only, the values of which ranged from 0.10€ to 30€. One of the target decisions had to be excluded due to a programming error leaving us with a total of 814 (22 participants × 37 decisions) choices per conditions as basis for the analyses. All decision tasks, their assignment to the within-subjects conditions, and average choices are listed in Appendix A.
Apparatus
Eye movements were recorded using the Eyegaze binocular system (LC Technologies) with remote binocular sampling rate of 120 Hz and an accuracy of about 0.45°. Images were presented on a 17″ color monitor (Samsung Syncmaster 740B, refresh rate 60 Hz, reaction time 5 ms) with a native resolution of 1280 × 1024. Fixations were identified using a 30 pixel tolerance (i.e., added max-min deviation for x and y-coordinates) and a minimum fixation time of 50 ms. Physiological arousal was measured by recording skin conductance responses using a NEXUS-8 system with a sampling rate of 32 samples per second. We used Butterworth (first order) filters to correct for high frequency and low frequency noise in the data6.
Procedure
In the description condition we relied on a procedure similar to the one used in Glöckner and Herbold (2011) which was slightly adapted by including a new decision screen to make it as similar as possible to the experience condition (Figure 1). Upon arrival, participants were familiarized with the decision task by reading a comprehensive instruction including screenshots of the paradigm. In both conditions, they were instructed to sample information as long as they liked. The decision screen was shown once participants pressed the space bar. Decisions were made by pressing buttons marked with “A” and “B” on the keyboard. In the experience condition, participants were additionally told that sampling also worked through pressing buttons “A” and “B” (see Figure 1). Individuals were calibrated and connected to the NEXUS (using the middle finger and the ring finger of the non-dominant hand). The experiment started with a test trial followed by the 60 decisions7. In both conditions, the position in which the two gambles were presented on screen (i.e., left or right) was counterbalanced between subjects.
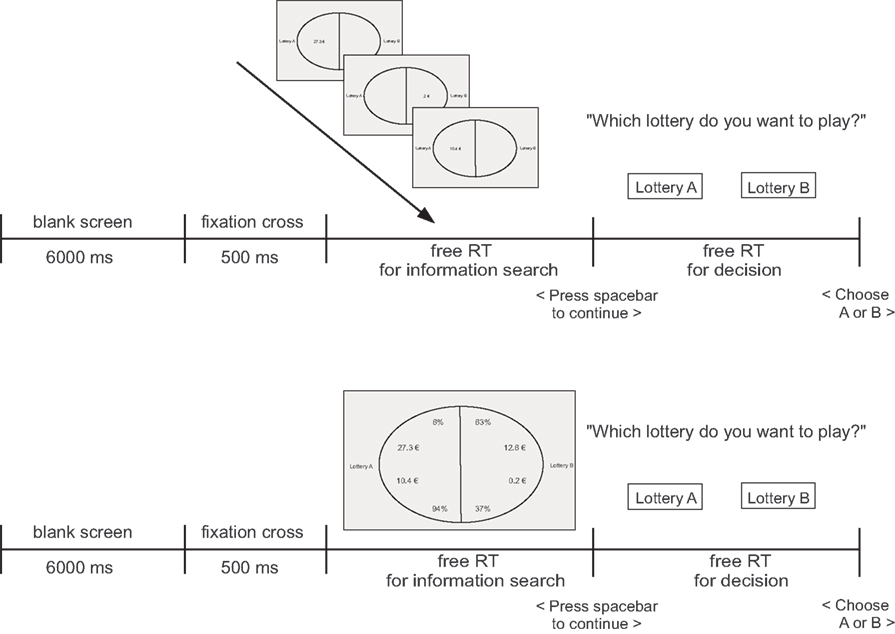
Figure 1. Procedure in the experience (top panel) and the description (bottom panel) condition. Note. RT means response time.
Each decision started with a blank screen (6 s) followed by a fixation cross (0.5 s) to center attention on the middle of the screen. Next, the gambles were presented in an ellipsoid display which ensured that information was equally distant from the initial fixation point in both conditions (Figure 1). Information for one gamble was presented on the left and for the other on the right side. After (explicit or implicit) sampling (information search phase) and pressing the space bar the decision screen appeared and individuals made their decision (decision phase). The analysis of fixations was done for the information search phase, whereas the analysis of arousal was done separately both for the information search phase and the decision phase. The decision phase was exactly identical in the two conditions.
Results
Sampling of Rare Event
In the experience condition we observed an average of 32 (Md = 30) information inspections, that is, for each decision, individuals pressed each of the two buttons about 16 times, which took them 12.8 s on average. The sampling rate is on the upper end of the spectrum observed in previous investigations which may be due to the relatively large monetary incentives (Hau et al., 2010 report median total sampling rates between 11 and 33 in a review of several previous studies). Consequently, sampling rates of low-probability outcomes (M = 0.084, SE = 0.0045) were relatively unbiased and reflected the average objective probabilities of these outcomes well (M = 0.0745).
As described above, we use fixations to outcomes as a proxy for information sampling in both paradigms. Note that the information display was much richer in the description condition containing eight pieces of information (i.e., four outcomes and four probabilities) than in the experience condition only showing one outcome at a time (see Figure 1). In the experience paradigm, there was an average of 53.5 fixations to outcomes per decision (Md = 48). Fixations also showed relatively unbiased sampling of rare events which received 0.08 of the fixations to the respective gamble. This proportion is calculated as the number of fixations to the rare event divided by the total fixations to the rare event and the alternative outcome of the respective gamble (Figure 2). As pointed out in the previous section, the presentation rate of the rare event (i.e., how often the rare event was shown in the gambles containing a rare event) was 0.084. Hence, participants did not show particularly increased or decreased fixation rates to rare events. Fixations roughly reflected the presentation (=sampling) rate and were thus also in line with the objective probability of the rare events. In the description condition, we observed 43.9 fixations on average (Md = 35) per decision with more fixations directed to outcomes (58%) than to probabilities (42%). In contrast to the experience condition, rare events were strongly oversampled in the description condition: the low-probability outcomes received 0.50 of all fixations within the respective gamble which is significantly higher than their objective probability, t(21) = 65.7, p < 0.001 (Figure 2)8. Note, that a fixation rate of 0.50 is expected if both outcomes receive equal attention. For all 22 participants, the (fixation-based) sampling percentage of rare events was higher than the objective probability of these events.
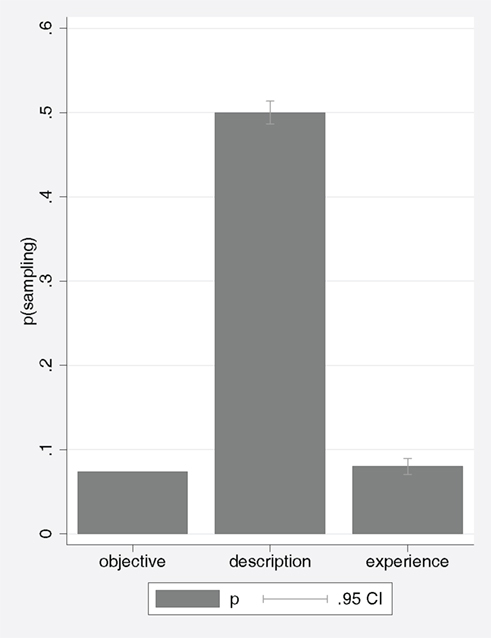
Figure 2. Proportion of fixations to the rare events compared to their objective probability. Note. Proportions of fixations are calculated using fixations to outcomes only. Proportions are calculated within gambles as the number of fixations to the rare event divided by the total fixations to the rare event and the alternative outcome of the respective gamble. Note that in the experience condition, the sampling rate of the rare event was 0.084, which is roughly reflects in the fixation rate.
In sum, there was relatively unbiased sampling of rare events in the experience condition but “oversampling” of rare events in the description condition in terms of attention. In fact, there seems to be no contingency between probability of the rare outcome and the proportion of attention it receives in the descriptions format (but, see Fiedler and Glöckner, submitted, for a more general analysis). In contrast, in the experience condition when only one piece of information is presented at a time, sampling rates measured by button-press and by fixation show a high degree of convergence. We nevertheless use both in the model comparison described below.
Choices
Overall analysis
We coded choices to indicate overweighting of small probabilities (i.e., 1 = choice for the gamble indicating overweighting; 0 = otherwise; see Appendix A) and plot this variable in Figure 3. Surprisingly, the option that – if chosen by the participants – would indicate overweighting of small probabilities was not chosen more often in the description than in the experience condition. The experience condition even shows a tendency toward stronger overweighting of small probabilities as compared to the description condition.
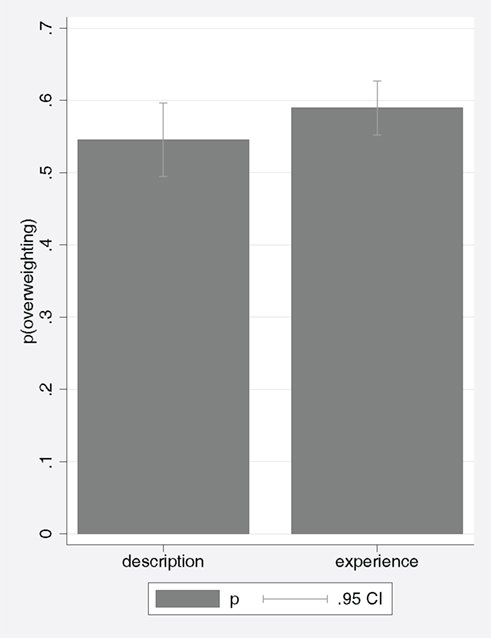
Figure 3. Choices in line with overweighting of small probabilities. Note. Higher scores in p(overweighting) indicate stronger overweighting of rare events.
Note that placing more weight to an undesirable outcome of a gamble (i.e., an outcome with a relatively low monetary value) necessarily implies placing less weight on the more desirable outcome in this gamble (holding expected value constant). Hence, if the low-probability outcome were overweighted, the gamble with the rare event should become more attractive with increasing values of the low-probability outcome (c.f. Hilbig and Glöckner, 2011). Consider, for example, a gamble paying 4€ with 80% probability and otherwise nothing. Overweighting the (undesirable) 0€ outcome would reduce the probability of choosing this option as compared to an option comprising the same expected value, but with only one sure outcome (e.g., 3.2€). Vice versa, if the 0€ outcome were replaced by, say, a 10€ (and thus desirable) outcome, overweighting this rare event would increase the probability of choosing this gamble (again compared to an option comprising the same expected value, but with one sure outcome).
We therefore analyzed whether low-probability outcomes are over- or underweighted by conducting a logistic regressions predicting choice of the option comprising the low-probability outcome by its value (i.e., desirability; values are outcomes in Euro as described in Appendix A), controlling for differences in expected value. If low probabilities are overweighted, the proportion of choices of the corresponding option should increase with increasing value of the outcome (c.f. Hilbig and Glöckner, 2011). That is, the odds-ratio coefficient for Low-Probability Outcome should be above one indicating that the probability for choosing the gamble increases with the value of the low-probability outcome9.
As expected, significant overweighting was observed in decision from description (Table 1, model 1; considering a one-sided test which is justified due to our a priori hypothesis, see Baron, 2010). Interestingly, however, we observed significant overweighting also for the experience condition (Table 1, model 2). The overall analysis indicated that there was no difference concerning overweighting between conditions as indicated by the non-significant interaction term (Table 1, model 3).
In sum, although we find oversampling of rare events in the description condition as compared to the experience condition, choice patterns in both conditions indicated overweighting of rare events. Interestingly, this result speaks against the hypothesis that decisions in both conditions are based on the same evidence-accumulation process of fixation-sampled information, since according to such models, low-probability outcomes should have had much more relative influence on choices in descriptions as compared to experience due to the strong oversampling in the former (as reported in the previous section).
Determinants of choices in the experience condition
We split tasks depending on whether the rare event was sampled (i.e., shown on the screen) (a) never, (b) once, or (c) more than once and reran the logistic regression predicting choices of the option comprising this low-probability outcome (again, with the value of the low-probability outcome and EV-difference as predictors). We found significant underweighting of low-probability outcomes for trials in which the rare event was not sampled (odds-ratio = 0.97, z = −2.33, p = 0.02), but overweighting for trials in which it was sampled once (odds-ratio = 1.08, z = 5.50, p < 0.001) or several times (odds-ratio = 1.11, z = 7.44, p < 0.001). Overweighting of rare events thus increases with the number of times they are sampled as indicated by a significant Number of Samples × Value of the Low-Probability Outcome interaction (odds-ratio = 1.05, z = 5.21, p < 0.001). The description-experience “gap” hence reduces with increasing number of samples drawn and might heavily depend on the fact that, in typical studies, many individuals do not sample the rare event at all. Thus, the high overall number of samples drawn in the current study might contribute to the fact that no evidence for underweighting of small probabilities is implied by the choice patterns in the experience condition.
Comparing Models for Risky Choices in Experience – and Description-Based Decisions
Model specification
To investigate the underlying processes more closely we calculated the predictive power of different naïve implementations of evidence-accumulation models and compared them against several competitors. Thereby, all models were implemented in a stochastic manner using a logistic choice rule (details see below) in which the probability of choosing a gamble increases with the difference in value (Vdiff) between gambles. The models only differ in the way in which values V for each gamble are calculated and therefore in Vdiff.
As basic comparison standard, we used two models that relied on objective probabilities and outcomes or transformations of these. Specifically, we considered an expected value model (EVobjective), and an implementation of Cumulative Prospect Theory (CPTobjective) with the parameters from Tversky and Kahneman, 1992 (i.e., α = 0.88, Γ = 0.61, λ = 2.25; all outcomes positive).
For the experience condition, participants might simply choose the option with the higher average of outcomes that was sampled by pressing buttons. We calculated predictions from such sampling-based models, which rely on the sampled outcomes for each gamble (or subsets of them). The first implementation takes into account all samples (SampAverAll). Note that participants have no other information than the sampled outcomes. Given this information deficit (and ignoring opportunity costs), SampAverAll is the optimal strategy to maximize chances to win money in this paradigm. As mentioned in the introduction, sampling average models might also be implemented using the most recent samples only. To test these alternative implementations, two further models that average over the last 10 (SampAverRec10) or the last five samples (SampAverRec5) per decision were calculated. These sample sizes were used since estimated average samples for recency (and also other sampling-based) models in Erev et al., 2010, e.g., see Table 3C) were between 5 and 10 (but see Discussion for limitations of this approach). To test whether summing instead of averaging of outcomes can account better for the data, we also included a model assuming summation of all sampled outcomes (SampSum). Note that for all sampling-based models introduced in this section one sample refers to one sampled outcome, independently of how often individuals looked at them.
More importantly, we considered averaging and summation models implementing evidence-accumulation based on participants actual fixations. In these model variants, valuations of gambles are based on the distribution of fixations to specific outcomes. Conceptually, fixation-based summation models (FixSummation) assume that preferences are constructed in a dynamic process in which each fixation to an outcome adds evidence for the respective gamble which is proportional to the value of the outcome. Fixation-based averaging models (FixAveraging) do the same but additionally correct for the number of fixations to each gamble so that the option with the higher average evidence is selected10. Appendix B provides a formal description of the models implemented.
For all models, we used a multi-level logistic regression model to predict individual choices of the option comprising the rare event based on difference in value between gambles (Vdiff).
Model estimation
We estimated the model fit to the choice data using multi-level (mixed-effect) logistic regressions assuming normally distributed random intercept ui according to:
and
with i indexing subjects and t indexing tasks.
All models have three estimated parameters (β0, β1, ). The best model was selected based on the Bayesian Information Criterion (BIC, Schwarz, 1978). To test the stability of the estimation we also reran the analyses using a logistic regression with cluster correction for standard errors which provides pseudo R2 values indicating how much variance can be explained by a model.
Model fitting results
In the experience conditions, the fixation-based averaging model (FixAveraging) provided the best fit to the data (Table 2). The sampling-based averaging model taking into account all samples (SampAverAll) performed nearly as well, whereas all other models turned out considerably worse. Models relying on only the most recent samples performed poorly, as did the sampling-based summation model and the fixation-based summation model.
In the description condition, by contrast, Cumulative Prospect Theory (CPTobjective) performed best, whereas both fixation-based models performed poorly. Overall, these findings indicate that attention-based evidence-accumulation models can account better for experience-based choices than for description-based choices.
Robustness checks and further analyses
In the experience condition, sampling of outcomes and fixations to the respective outcomes are necessarily highly correlated. As one would expect, the predictions of the two best models in the experience paradigm SampAverAll and FixAveraging were therefore also highly correlated (b = 0.93, t = 93.60, p < 0.001). We tested whether, despite this high degree of overlap, both models make unique contributions in predicting choices by including both predictors simultaneously in a logistic regression (clustering at the participant level and correcting for individual differences using dummies). The predictors of both models remained significant at p < 0.05 indicating that both models have unique predictive power.
We conducted further tests of whether modified implementations of the models mentioned above improve model fit. First, one might suspect that our implementations of fixation-based models might be suboptimal since they take into account frequency of fixations only and ignore the duration of these fixations. To test this hypothesis, we calculated model implementations for the FixAveraging and the FixSummation models in which each fixated outcome is weighted by the duration of the respective fixation. In both conditions, model fit decreased when weighting fixation by duration compared to using frequency of fixations. Second, in the description condition, fixations to outcomes and their probabilities might both be considered to provide evidence of attention to the respective gamble. We therefore implemented fixation-based models in which each fixation to a probability was also counted as evidence for the outcome connected with this probability. In both implementations, the model fit improved slightly (by about three BIC points), which, however, does not change any of above conclusions.
Arousal Measures: Pupil Dilation and Skin Conductance Response
Finally, we analyzed increases in physiological arousal between conditions and tasks as measured by (a) pupil dilation and (b) skin conductance response. A focus was placed on differences in affective responses to our manipulation of EV-difference between conditions, indicating differences in processing. As dependent measures we calculated peak arousal scores, that is, the maximum increase of arousal as measured by pupil dilation and skin conductance from baseline (i.e., measured at fixation cross presentation) in the respective part of the decision process. We thereby conducted analyses separately for the information search phase (i.e., in which the information about the options was presented and sampled) and the decision phase (i.e., in which the decision screen was presented). Due to unsystematic breakdowns of the NEXUS system, we lost parts of the data for several participants, leaving us with 33 (out of the 44) complete sets for the analysis of skin conductance (15 experience, 18 description). Peak arousal scores for pupil dilation and skin conductance response showed a medium correlation [r = 0.34, t(35) = 2.13, p < 0.05; scores aggregated at the task level].
Pupil dilation
For both conditions, we regressed pupil dilation scores on absolute EV-difference (based on objective probabilities and outcomes), controlling for effects of trial order by including trial number as predictor, and differences between subjects by including subject dummies. In the description condition, pupil dilation decreased with increasing EV-difference, which was not the case in the experience condition (Figure 4, left). The effect in the description condition turned out significant both in the information search phase [b = −0.014, t(21) = −3.03, p = 0.006] and the decision phase [b = −0.010, t(21) = −2.11, p = 0.047]. In the experience condition, the effect was not significant [information search phase: b = −0.00097, t(21) = 0.29, p = 0.77; decision phase: b = −0.0029, t(21) = −0.66, p = 0.518]11 which also holds when using experienced (i.e., subjectively sampled) probabilities to calculate EV-difference instead of objective probabilities (both p > 0.25).
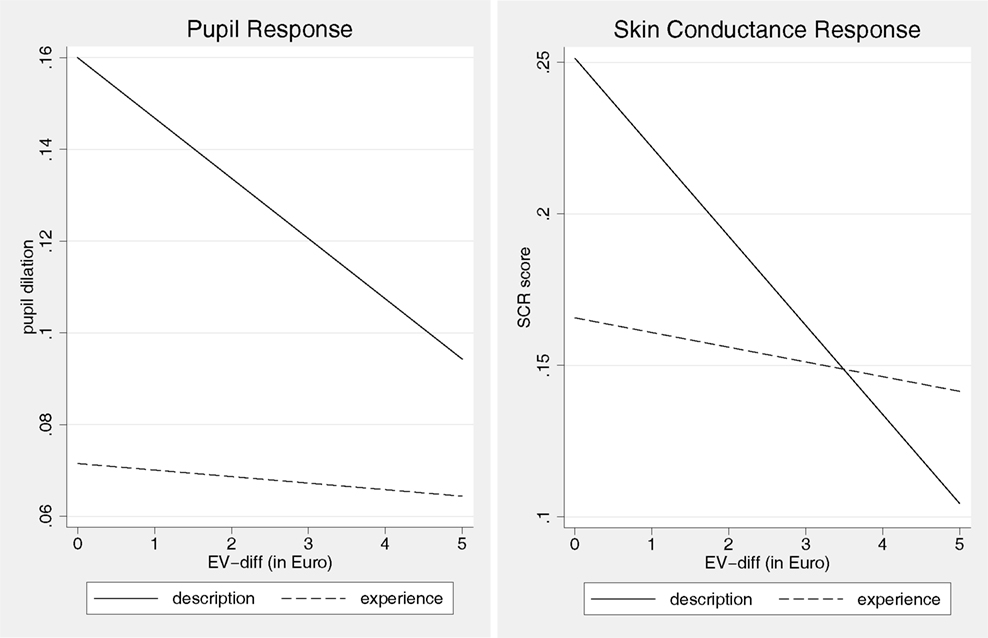
Figure 4. Physiological arousal. Note. The graphs show predicted peak arousal scores for Pupil Dilation (left panel) and Skin Conductance Response (right panel) with high numbers indicating high arousal. Both scores are differences from baseline measured at presentation of the fixation cross preceding the respective trial. Pupil dilation scores are in millimeters and refer to changes in radius. Graphs are based on a joint analysis over information search phase and decision phase.
Skin conductance response
We regressed skin conductance response scores on absolute EV-difference, controlling for effects of trial order, and differences between subjects by including subject dummies. The results nicely converge with the findings concerning pupil dilation: skin conductance response decreased with increasing EV-difference in the description condition but not (or much less so) in the experience condition (Figure 4, right). EV-difference did not predict arousal for the experience condition, neither in the information search phase nor in the phase in which the decision screen was shown (both p > 0.23). In the description condition, by contrast, we found strong corresponding effects in both the search phase [b = −0.034, t(17) = −2.31, p = 0.034] and the decision phase [b = −0.022, t(17) = −3.04, p = 0.007]. Since the decision phase was exactly identical in both conditions, the difference between conditions indicates that there might be qualitative differences in processing between both conditions that do not only concern trivial differences in information search but also the way in which information is integrated. We will discuss this issue in more detail the Section “Discussion.”
Note, however, that the general level of arousal did not differ significantly between conditions, neither for pupil dilation [b = −0.009, t(43) = 0.5, p = 0.62] nor for skin conductance response [b = 0.023, t(32) = 0.87, p = 0.40], and coefficients even pointed in opposite directions (condition dummy coded with Experience = 1).
Discussion
In the current work, we examined processing differences between one-shot decisions from description vs. experience using eye-tracking, cognitive modeling, and physiological arousal. Concerning choices, we did not find underweighting of low-probability outcomes in experience-based decisions and therefore our results do not replicate the description-experience gap in choices. It is noteworthy that this also holds when considering the 37 decisions independently (see Appendix A). Although we did not expect this result, it is interesting since it is in line with recent evidence pointing at important moderators for observing the descriptions-experience “gap12.”
First, our findings are in line with Camilleri and Newell (2011b) who find that behavior in one-shot experience-based decisions (i.e., sampling information and then making one decision that is incentivized) leads to behavior more in line with decisions from description compared to repeated experience-based decision (i.e., each sample is incentivized and individuals receive immediate feedback; see also text footnote 1, above). Second, in our study, participants sampled more than twice as often as participants in the original study on one-shot experience-based decisions by Hertwig et al. (2004). As a consequence of this low sampling rate, 78% of their participants sampled the rare event less often than expected (Camilleri and Newell, 2011b). We did not observe such a bias in sampling. Therefore, our findings are in line with Ungemach et al. (2009) in showing that the gap reduces with increasing sample size and the argument that the effect is largely driven by biased samples (Fox and Hadar, 2006; Camilleri and Newell, 2011b).
Most importantly, even though choices did not reveal a “gap” between descriptions and experience, a more in-depth model comparison based on choices as well as an analysis of process measures suggest that the underlying cognitive processes in the two types of paradigms are markedly different.
Evidence for Qualitative Processing Differences between Decisions from Description vs. Decisions from Experience
Our findings indicate qualitative processing differences between decisions from description and decisions from experience that go beyond trivial differences concerning preprocessing of information. As such, the current results speak against the hypothesis that individuals merely transform information in an initial preprocessing stage, but later rely on the same integration process in both paradigms. This conclusion is based on three novel findings which we briefly summarize in what follows.
First, the model comparison for choices indicates that notably different models explain choices best in the two conditions, which replicates and extends findings from Erev et al. (2010). Evidence-accumulations models assuming sampling of outcomes by fixation and linear integration of these outcomes, which have been suggested as models for risky choices in general (Busemeyer and Townsend, 1993), can account well for decisions in the experience paradigm but not in the description paradigm. In the description paradigm, by contrast, Cumulative Prospect Theory was the best model, which converges with other recent findings from comprehensive model comparisons (Erev et al., 2010; Glöckner and Pachur, 2012).
Second, we find that an effect of EV-difference on arousal, as measured by pupil dilation and skin conductance response, can be found in description-based but not in experience-based choices. If the same cognitive processes had been at work for information integration in both conditions, the effect of EV-difference on arousal should have been comparable. Together with the modeling results, the physiological data suggests that decisions from descriptions involve more complex mathematical processes of computation such as coherence construction (Glöckner and Betsch, 2008; see Models for Decision from Description, for details) or other ways of subjectively weighting outcomes (Ayal and Hochman, 2009), which are highly affected by expected value differences (see Ayal and Hochman, 2009; Glöckner and Hochman, 2011). By contrast, experience-based decisions might involve simpler processes based on linear integration and a comparison of the averages of experienced outcomes13. These processes appear to be more similar to accumulation of fixation-sampled evidence until a certain threshold is reached (Busemeyer and Townsend, 1993; Raab and Johnson, 2007; Krajbich and Rangel, 2011). Alternatively, they might also be similar to memory prompting (Dougherty et al., 1999; Thomas et al., 2008) or instance-based learning (Lejarraga et al., 2012)14. Presumably, these types of processes essentially require little more than remembering what was previously seen. Since these types of decisions are more easily constructed, they are arguably less sensitive to task-difficulty manipulations (e.g., differences in expected values). Of course, this interpretation of the arousal results will require further tests in future research.
Third, the link between attention measured by overt fixations and weight placed on specific outcomes in the decision tasks seems to be much stronger in decisions from experience than in decisions from descriptions. In the latter, our findings indicate that despite substantial fixation-based oversampling of rare events, there was relatively little overweighting and thus, fixation-based models perform relatively poorly (despite predictive power well above chance-level). In the experience condition, by contrast, the good model fit for fixation-based models indicates that the relation between attention and weight is quite strong.
Models for Decisions from Experience
The current findings speak to several important questions concerning the specific processes underlying decisions from experience in the sampling paradigm. Specifically, they support the idea that certain implementations of evidence-accumulation models (Busemeyer and Townsend, 1993; see also Roe et al., 2001; Raab and Johnson, 2007; Jessup et al., 2008; Krajbich and Rangel, 2011) can account well for processes in decisions from experience. In addition, our data provide relatively clear hints on which implementations should be preferred: first, models assuming averaging of outcomes are superior to models assuming summing. This speaks against models assuming evidence-accumulation without standardization for the number of samples. Prominent evidence-accumulation models for decision making are conceptually based on the idea that there is a mere process of accumulation which does not include standardization for number of samples (e.g., Busemeyer and Townsend, 1993; Johnson and Busemeyer, 2005; Raab and Johnson, 2007; Krajbich and Rangel, 2011). Second, models taking into account all samples account for behavior better than recency-based models which rely only on a subset of samples. Note, however, that our investigation did not address models which assume that the number of recently sampled outcomes is a free parameter that reflect individual differences in sampling size. Thus, we cannot rule out the possibility that such more complex models, as well as models which assume decreasing weights for outcomes that are less recent may further improve the predictive power for participants’ behavior in decisions from sampling.
Models for Decisions from Description
In line with prior evidence, our results indicate that choices in risky decisions from descriptions can be described adequately by Cumulative Process Theory (e.g., Tversky and Kahneman, 1992; Glöckner and Pachur, 2012)15. Nevertheless, process implementations of this theory assuming serial stepwise calculations of weighted sums have been rejected (Glöckner and Herbold, 2011). Instead, processes that rely at least partially on more complex automatic-intuitive mechanisms have received support. Process measures in Glöckner and Herbold were most in line with implementations of coherence construction models. The suggested adaptation of the Parallel Constraint Satisfaction Model (Thagard, 1989; Holyoak and Simon, 1999; Simon et al., 2004; Betsch and Glöckner, 2010) to risky choice assumes that probability weighted outcomes are used as competing pros and cons (i.e., cues) speaking for one or the other option and that initial advantages of one option are accentuated by partially relying on automatic-intuitive processes. The effect of conflict manipulated by decreasing EV-difference (as opposed to coherence) on arousal observed in the current study provides further support for this approach, and is in line with previous findings demonstrating a link between coherence and arousal (Hochman et al., 2010; Glöckner and Hochman, 2011). As noted above, the arousal findings might, however, also be explained by other mechanisms and further research is needed to investigate the processes underlying risky choice from description.
Summary
The current results demonstrate that there are considerable differences in the cognitive processes underlying one-shot decisions from experience vs. description. In experience-based decisions, individuals are not explicitly provided with probability information and therefore evaluate options in a way that can be well-captured by naïve evidence-accumulation models assuming averaging of all fixation-sampled outcomes. The process seems to be based on a linear integration and a comparison of the averages. Thus, the difference between expected values of options does not influence arousal. Decisions from descriptions, by contrast, cannot be described well by fixation-based evidence-accumulation. Choices are more in line with Cumulative Prospect Theory, which, however, does not claim to describe processes. The findings that (i) different models account for choices best in the two paradigms, (ii) arousal increases with difference in expected value between options only in descriptions but not in experience, and (iii) the link between attention and weight given to certain outcomes is much stronger in experience indicate that qualitatively different kinds of processes are at work.
Conflict of Interest Statement
The authors declare that the research was conducted in the absence of any commercial or financial relationships that could be construed as a potential conflict of interest.
Footnotes
- ^Note, however, that the choice problem in Barron and Erev (2003) differed from a description-based task not only by in terms of how information was acquired. Rather, whereas description-based tasks usually require a one-shot decision, the feedback task used in Barron and Erev required participants to make repeated choices with feedback, all of which had monetary consequences. To rule out that specifically this feedback aspect may have driven the “gap,” subsequent research replicated the “gap” in a one-shot experience-based task (i.e., sampling task): Participants again sample single outcomes drawn from each of the choice options. However, none of these samples is consequential. Instead, after the sampling phase, participants make a single consequential choice (e.g., Hertwig et al., 2004). Although both experience-based paradigms reveal choice patterns that differ from those typically found in the description-based paradigm, recent research also indicates considerable differences between the two experience-based tasks. Specifically, the description-experience “gap” is much stronger in the feedback task as compared to the sampling task (Camilleri and Newell, 2011b). In addition, the differences between the two experience-based paradigms are actually larger than between sampling and description.
- ^Two explanations that have also been suggested but will not be considered further herein are that individuals might underestimate the probabilities of rare events (i.e., estimation error; Hertwig and Erev, 2009) and that individuals might use different decision policies (Hills and Hertwig, 2010) reflected in either often switching between options (i.e., piece-wise sampling) or continuous sampling within one option (i.e., comprehensive sampling).
- ^In Hertwig et al. (2004) 78% of the participants made choices based on a sample of information which contained the rare event less often than its objective probability. Note that this is not caused by unequal (biased) sampling between options but due to a mere statistical effect that in small samples the majority of individuals often do not get to see the rare event at all. More precisely, the mean of the relative sampling frequency of the rare events equals their objective probability but due to the skewness in the distribution the median falls below the mean.
- ^Note that this model statement is concerned with mental sampling. Therefore our test necessitates accepting the empirically well supported eye-mind hypothesis (Just and Carpenter, 1976) stating that individuals fixate the information they process. Also note that Prospect Theory (Kahneman and Tversky, 1979) is an as-if model, which does not necessarily imply a relation between decision weights and attention.
- ^These differences may thereby be explained by multiple process accounts. We do not aim to distinguish between them and they have to be further dissected in future research.
- ^We used a filter allowing a band from 0.1 to 1 Hz. To test the robustness of our findings we also conducted the analysis using a FIR Bandpass 128 filter [Parks–McClellan (optimal)] with the same band which essentially led to the same results.
- ^Due to a programming error, order was randomized only in the experience condition but it was fixed in the description condition (using the order presented in Appendix A). Note, however, that gambles were randomly generated, half of them were side-reversed which was counterbalanced between subjects, and they were intermixed with blocks of distractors. We therefore consider it very unlikely that this difference could have influenced our results. We nevertheless cannot completely rule out this possibility.
- ^Analyzing total fixation durations instead of number of fixations led to the same conclusions.
- ^Here and in all following regressions we used cluster correction on the level of subjects to correct for dependencies in errors caused by the repeated measurement design (Rogers, 1993). We also conducted the analyses using multi-level random effects models (i.e., random intercept), which leads to the same conclusions.
- ^Note that – in contrast to averaging models – summation models take into account biased sampling toward one of the options. For decisions with all non-negative outcomes increased attention toward one option should lead to a choice bias in favor of this option. This implementation of fixation-based summation models is similar to evidence-accumulation models suggested by Rangel, Krajbich and colleagues (Armel et al., 2008; Krajbich and Rangel, 2011).
- ^In the description condition trial order (jointly calculated over search and decision phase) turned out significant as well, b = −0.0022, t(21) = −4.16, p < 0.001, which was not the case in the experience condition, b = 0.0002, t(21) = 0.47, p = 0.644. A further regression analysis was conducted including two-way interaction terms for condition by EV-diff and condition by trial number (all variables centered) in the model (but excluding subject dummies). As indicated by the trial order coefficients reported above, participants in the two conditions reacted differently on trial order [IE: b = 0.0023, t(21) = 3.83, p < 0.001]. Most importantly, however, the interaction effect of EV-diff and condition was significant even when controlling for this effect [IE: b = 0.0117, t(21) = 2.58, p = 0.013].
- ^Of course, it is also generally important to report non-replications to avoid the problem of publication bias (Renkewitz et al., 2011).
- ^One factor contributing to this might be the simpler information display in the experience condition (see Figure 1).
- ^For a more general classification of these kinds of processes and their role in decision making, see also Glöckner and Witteman (2010).
- ^It should be noted that CPT has been rejected in favor of competing models in complex multi-outcome risky choices (Birnbaum, 2006, 2008a,b) but it is nonetheless considered a good paramorphic model for risky choices between two options with two outcomes each.
References
Armel, K. C., Beaumel, A., and Rangel, A. (2008). Biasing simple choices by manipulating relative visual attention. Judgm. Decis. Mak. 3, 396–403.
Armel, K. C., and Rangel, A. (2008). The impact of computation time and experience on decision values. Am. Econ. Rev. 98, 163–168.
Ashby, N. J. S., Dickert, S., and Glöckner, A. (2012). Focusing on what you own: biased information uptake due to ownership. Judgm. Decis. Mak. 7, 254–267.
Ayal, S., and Hochman, G. (2009). Ignorance or integration: the cognitive processes underlying choice behavior. J. Behav. Decis. Mak. 22, 455–474.
Baron, J. (2010). Looking at individual subjects in research on judgment and decision making (or anything). Acta Psychologica Psychol. Sin. 42, 1–11.
Barron, G., and Erev, I. (2003). Small feedback-based decisions and their limited correspondence to description-based decisions. J. Behav. Decis. Mak. 16, 215–233.
Betsch, T., and Glöckner, A. (2010). Intuition in judgment and decision making: extensive thinking without effort. Psychol. Inq. 21, 279–294.
Birnbaum, M. H. (2006). Evidence against prospect theories in gambles with positive, negative, and mixed consequences. J. Econ. Psychol. 27, 737–761.
Birnbaum, M. H. (2008b). New tests of cumulative prospect theory and the priority heuristic: probability-outcome tradeoff with branch splitting. Judgm. Decis. Mak. 3, 304–316.
Busemeyer, J. R., and Johnson, J. G. (2004). “Computational models of decision making,” in Blackwell Handbook of Judgment and Decision Making, eds D. J. Koehler and N. Harvey (Malden, MA: Blackwell Publishing), 133–154.
Busemeyer, J. R., and Townsend, J. T. (1993). Decision field theory: a dynamic-cognitive approach to decision making in an uncertain environment. Psychol. Rev. 100, 432–459.
Camilleri, A. R., and Newell, B. R. (2011a). Description- and experience-based choice: does equivalent information equal equivalent choice? Acta Psychol. (Amst.) 136, 276–284.
Camilleri, A. R., and Newell, B. R. (2011b). When and why rare events are underweighted: a direct comparison of the sampling, partial feedback, full feedback and description choice paradigms. Psychon. Bull. Rev. 18, 377–384.
Dougherty, M. R. P., Gettys, C. F., and Ogden, E. E. (1999). MINERVA-DM: a memory processes model for judgments of likelihood. Psychol. Rev. 106, 180–209.
Erev, I., and Barron, G. (2005). On adaptation, maximization and reinforcement learning among cognitive strategies. Psychol. Rev. 112, 912–931.
Erev, I., Ert, E., Roth, A. E., Haruvy, E., Herzog, S. M., Hau, R., Hertwig, R., Stewart, T., West, R., and Lebiere, C. (2010). A choice prediction competition: choices from experience and from description. J. Behav. Decis. Mak. 23, 15–47.
Fiedler, K. (1996). Explaining and simulating judgment biases as an aggregation phenomenon in probabilistic, multiple-cue environments. Psychol. Rev. 103, 193–214.
Fiedler, K. (2008). The ultimate sampling dilemma in experience-based decision making. J. Exp. Psychol. Learn. Mem. Cogn. 34, 186–203.
Fiedler, K., Brinkmann, B., Betsch, T., and Wild, B. (2000). A sampling approach to biases in conditional probability judgments: beyond base rate neglect and statistical format. J. Exp. Psychol. Gen. 129, 399–418.
FitzGerald, T. H. B., Seymour, B., Bach, D. R., and Dolan, R. J. (2010). Differentiable neural substrates for learned and described value and risk. Curr. Biol. 20, 1823–1829.
Fox, C. R., and Hadar, L. (2006). “Decisions from experience” =sampling error+prospect theory: reconsidering Hertwig, Barron, Weber and Erev (2004). Judgm. Decis. Mak. 1, 159–161.
Glöckner, A., and Betsch, T. (2008). Modeling option and strategy choices with connectionist networks: towards an integrative model of automatic and deliberate decision making. Judgm. Decis. Mak. 3, 215–228.
Glöckner, A., Heinen, T., Johnson, J., and Raab, M. (2012). Network approaches for expert decisions in sports. Hum. Mov. Sci. 31, 318–333.
Glöckner, A., and Herbold, A.-K. (2011). An eye-tracking study on information processing in risky decisions: evidence for compensatory strategies based on automatic processes. J. Behav. Decis. Mak. 24, 71–98.
Glöckner, A., and Hochman, G. (2011). The interplay of experience-based affective and probabilistic cues in decision making: arousal increases when experience and additional cues conflict. Exp. Psychol. 58, 132–141.
Glöckner, A., and Pachur, T. (2012). Cognitive models of risky choice: parameter stability and predictive accuracy of prospect theory. Cognition 123, 21–32.
Glöckner, A., and Witteman, C. L. M. (2010). Beyond dual-process models: a categorization of processes underlying intuitive judgment and decision making. Think. Reas. 16, 1 –25.
Greiner, B. (2004). “An online recruitment system for economic experiments,” in Forschung und wissenschaftliches Rechnen 2003. GWDG Bericht 63, eds K. Kremers and V. Macho (Göttingen: Ges. für Wiss. Datenverarbeitung), 79–93.
Hau, R., Pleskac, T. J., and Hertwig, R. (2010). Decisions from experience and statistical probabilities: why they trigger different choices than a priori probabilities. J. Behav. Decis. Mak. 23, 48–68.
Hertwig, R., Barron, G., Weber, E. U., and Erev, I. (2004). Decisions from experience and the effect of rare events in risky choice. Psychol. Sci. 15, 534–539.
Hertwig, R., and Erev, I. (2009). The description-experience gap in risky choice. Trends Cogn. Sci. (Regul. Ed.) 13, 517–523.
Hilbig, B. E. (2009). Sad, thus true: negativity bias in judgments of truth. J. Exp. Soc. Psychol. 45, 983–986.
Hilbig, B. E. (2012). Good things don’t come easy (to mind): explaining framing effects in judgments of truth. Exp. Psychol. 59, 38–46.
Hilbig, B. E., and Glöckner, A. (2011). Yes, they can! Appropriate weighting of small probabilities as a function of information acquisition. Acta Psychol. (Amst.) 138, 390–396.
Hilbig, B. E., and Pohl, R. F. (2009). Ignorance- versus evidence-based decision making: a decision time analysis of the recognition heuristic. J. Exp. Psychol. Learn. Mem. Cogn. 35, 1296–1305.
Hills, T. T., and Hertwig, R. (2010). Information search in decisions from experience. Psychol. Sci. 21, 1787–1792.
Hochman, G., Ayal, S., and Glöckner, A. (2010). Physiological arousal in processing recognition information: ignoring or integrating cognitive cues? Judgm. Decis. Mak. 5, 285–299.
Hogarth, R., and Einhorn, H. (1992). Order effects in belief updating: the belief-adjustment model. Cogn. Psychol. 24, 1–55.
Holyoak, K. J., and Simon, D. (1999). Bidirectional reasoning in decision making by constraint satisfaction. J. Exp. Psychol. Gen. 128, 3–31.
Innocenti, A., Rufa, A., and Semmoloni, J. (2010). Overconfident behavior in informational cascades: an eye-tracking study. J. Neurosci. Psychol. Econ. 3, 74–82.
Jessup, R. K., Bishara, A. J., and Busemeyer, J. R. (2008). Feedback produces divergence from prospect theory in descriptive choice. Psychol. Sci. 19, 1015–1022.
Johnson, J. G., and Busemeyer, J. R. (2005). A dynamic, stochastic, computational model of preference reversal phenomena. Psychol. Rev. 112, 841–861.
Just, M. A., and Carpenter, P. A. (1976). Eye fixations and cognitive-processes. Cogn. Psychol. 8, 441–480.
Kahneman, D., and Tversky, A. (1979). Prospect theory: an analysis of decision under risk. Econometrica 47, 263–292.
Kareev, Y., and Fiedler, K. (2006). Nonproportional sampling and the amplification of correlations. Psychol. Sci. 17, 715–720.
Krajbich, I., and Rangel, A. (2011). Multialternative drift-diffusion model predicts the relationship between visual fixations and choice in value-based decisions. Proc. Natl. Acad. Sci. U.S.A. 108, 13852–13857.
Kühberger, A. (1998). The influence of framing on risky decisions: a meta-analysis. Organ. Behav. Hum. Decis. Process 75, 23–55.
Lejarraga, T., Dutt, V., and Gonzalez, C. (2012). Instance-based learning: a general model of repeated binary choice. J. Behav. Decis. Mak. 25, 143–153.
Ludvig, E. A., and Spetch, M. L. (2011). Of black swans and tossed coins: is the description-experience gap in risky choice limited to rare events? PLoS ONE 6, e20262. doi:10.1371/journal.pone.0020262
Maule, J., and Villejoubert, G. (2007). What lies beneath: reframing framing effects. Think. Reas. 13, 25–44.
Milosavljevic, M., Malmaud, J., Huth, A., Koch, C., and Rangel, A. (2010). The drift diffusion model can account for the accuracy and reaction time of value-based choices under high and low time pressure. Judgm. Decis. Mak. 5, 437–449.
Pleskac, T. J., and Busemeyer, J. R. (2010). Two-stage dynamic signal detection: a theory of choice, decision time, and confidence. Psychol. Rev. 117, 864–901.
Raab, M., and Johnson, J. G. (2007). Expertise-based differences in search and option-generation strategies. J. Exp. Psychol. Appl. 13, 158–170.
Renkewitz, F., Fuchs, H. M., and Fiedler, S. (2011). Is there evidence of publication biases in JDM research? Judgm. Decis. Mak. 6, 870–881.
Rieskamp, J. (2008). Probabilistic nature of preferential choice. J. Exp. Psychol. Learn. Mem. Cogn. 34, 1446–1465.
Roe, R., Busemeyer, J. R., and Townsend, J. (2001). Multiattribute decision field theory: a dynamic, connectionist model of decision making. Psychol. Rev. 108, 370–392.
Simon, D., Krawczyk, D. C., and Holyoak, K. J. (2004). Construction of preferences by constraint satisfaction. Psychol. Sci. 15, 331–336.
Thomas, R. P., Dougherty, M. R., Sprenger, A. M., and Harbison, J. I. (2008). Diagnostic hypothesis generation and human judgment. Psychol. Rev. 115, 155–185.
Tversky, A., and Kahneman, D. (1981). The framing of decisions and the psychology of choice. Science 211, 453–458.
Tversky, A., and Kahneman, D. (1992). Advances in prospect theory: cumulative representation of uncertainty. J. Risk Uncertain 5, 297–323.
Ungemach, C., Chater, N., and Stewart, N. (2009). Are probabilities overweighted or underweighted when rare outcomes are experienced (rarely)? Psychol. Sci. 20, 473–479.
Yechiam, E., Barron, G., and Erev, I. (2005a). The role of personal experience in contributing to different patterns of response to rare terrorist attacks. J. Conflict Resolut. 49, 430–439.
Yechiam, E., Busemeyer, J. R., Stout, J. C., and Bechara, A. (2005b). Using cognitive models to map relations between neuropsychological disorders and human decision-making deficits. Psychol. Sci. 16, 973–978.
Yechiam, E., and Busemeyer, J. R. (2005). Comparison of basic assumptions embedded in learning models for experience-based decision making. Psychon. Bull. Rev. 12, 387–402.
Yechiam, E., and Ert, E. (2007). Evaluating the reliance on past choices in adaptive learning models. J. Math. Psychol. 51, 75–84.
Appendices
Appendix A
Appendix B
Summation models
To implement summation models we calculate Vdiff as follows:
Where A and B represent the two choice options, O represent the outcome, 1 and 2 the first and second outcome of each option, and indicate the number of fixations to the outcomes X.
Averaging models
For averaging models, the sums of sampled outcomes for each option were additionally standardized by the total number of fixations to the respective option according to:
Keywords: risky choices, description vs. experience gap, sampling, eye-tracking, evidence-accumulation, prospect theory
Citation: Glöckner A, Fiedler S, Hochman G, Ayal S and Hilbig BE (2012) Processing differences between descriptions and experience: a comparative analysis using eye-tracking and physiological measures. Front. Psychology 3:173. doi: 10.3389/fpsyg.2012.00173
Received: 15 December 2011; Paper pending published: 02 January 2012;
Accepted: 15 May 2012; Published online: 13 June 2012.
Edited by:
Eldad Yechiam, Technion – Israel Institute of Technology, IsraelReviewed by:
Thomas Hills, University of Basel, SwitzerlandEyal Ert, The Hebrew University of Jerusalem, Israel
Copyright: © 2012 Glöckner, Fiedler, Hochman, Ayal and Hilbig. This is an open-access article distributed under the terms of the Creative Commons Attribution Non Commercial License, which permits non-commercial use, distribution, and reproduction in other forums, provided the original authors and source are credited.
*Correspondence: Andreas Glöckner, Research Group Intuitive Experts, Max Planck Institute for Research on Collective Goods, Kurt-Schumacher-Str. 10, D-53113 Bonn, Germany. e-mail:Z2xvZWNrbmVyQGNvbGwubXBnLmRl