- Department of Kinesiology, Movement Control and Neuroplasticity Research Group, Group of Biomedical Sciences, KU Leuven, Leuven, Belgium
Practice of a given physical activity is known to improve the motor skills related to this activity. However, whether unrelated skills are also improved is still unclear. To test the impact of physical activity on an unpracticed motor task, 26 young adults completed the international physical activity questionnaire and performed a bimanual coordination task they had never practiced before. Results showed that higher total physical activity predicted higher performance in the bimanual task, controlling for multiple factors such as age, physical inactivity, music practice, and computer games practice. Linear mixed models allowed this effect of physical activity to be generalized to a large population of bimanual coordination conditions. This finding runs counter to the notion that generalized motor abilities do not exist and supports the existence of a “learning to learn” skill that could be improved through physical activity and that impacts performance in tasks that are not necessarily related to the practiced activity.
Introduction
Each year, physical inactivity is responsible for 13 million of disability-adjusted life-years worldwide and costs 67.5 billion of international dollars (Ding et al., 2016). In this context, the promotion of physical activity appears strongly relevant. Such promotion could be initiated through the development of motor skills that have shown to be a primary factor of engagement in physical activity (Nougier et al., 1996; Stodden et al., 2008; Barnett et al., 2009). While engagement in a certain type of physical activity is known to improve the motor skills specific to this activity (Bachman, 1961; Higbie et al., 1996), it is still unclear whether movement skills that are not directly related to this activity would also be improved. If such a generalization does occur, engagement in one type of physical activity could potentially promote engagement in other activities (Figure 1).
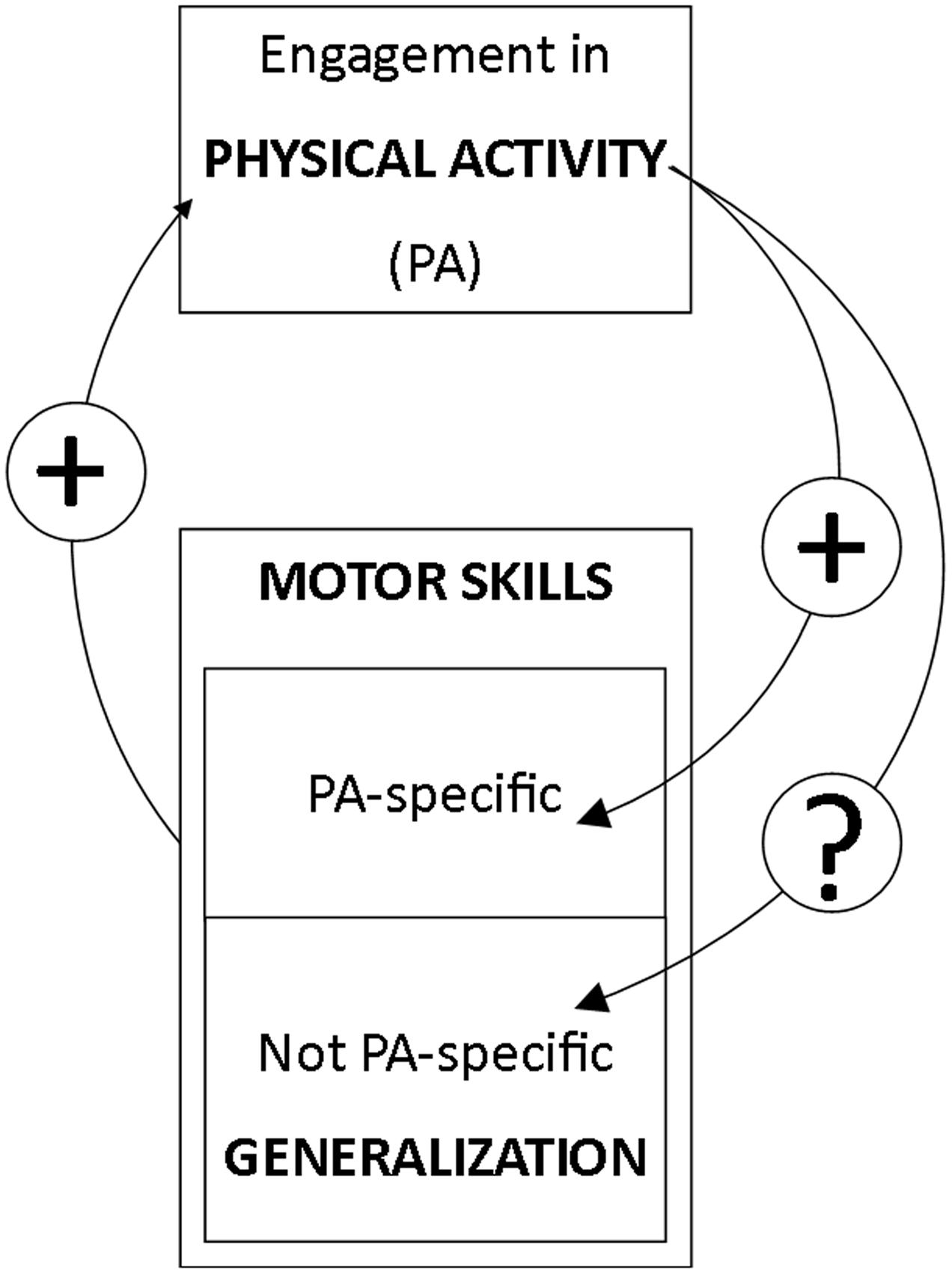
FIGURE 1. Scope of the study. Here we posit that physical activity engagement and motor skill proficiency can potentially reinforce each other via a virtuous circle. We further hypothesize that physical activity (PA) is associated with a global skill improvement that is likely related to the “learning how to learn” phenomenon (Harlow, 1949; Seidler, 2010), which is expressed in skills specific to the practiced PA, but also to other skills, not specific to the practiced PA.
A potential explanatory mechanism of such overall improvement is the “learning to learn” phenomenon (Harlow, 1949; Seidler, 2010), whereby problem-solving skills developed for one task/context generalize to similar tasks/contexts (Bock et al., 2001; Seidler, 2004). This generalization could be explained by adaptations at the level of executive functions (e.g., attention, response planning, decision making) that have been linked to physical activity (Kraft, 2012), and/or by improved proprioception (Adamo et al., 2009; Helsen et al., 2016), which has shown to be critical for the control of movement (Sainburg et al., 1995; Nougier et al., 1996; Jackson et al., 2000). Both improved proprioception and an increased ability to reduce limb stiffness, which would allow movements with larger amplitudes, would result in larger perceived errors to learn from (Burdet et al., 2001). Finally, as suggested by Seidler (2010), this generalization could be explained by the improvement of the core skills required for the learning process, such as pattern and error detection and correction.
Here, we hypothesized that physical activity predicts motor skill proficiency in tasks that have never been practiced before such as a multi-frequency bimanual coordination task. We further posited that physical activity engagement and motor skill proficiency can potentially reinforce each other via a virtuous circle (Figure 1).
Materials and Methods
Participants
Twenty-six healthy young volunteers (age range 18–30 years; mean age 24 ± 3 years) participated in the study. All participants were right-handed according to the Edinburgh Handedness Inventory (Oldfield, 1971). The International Physical Activity Questionnaire (IPAQ; Craig et al., 2003) was used to assess total physical activity and inactivity. Participants were asked how much time per week they spend sitting (physical inactivity), play a music instrument, and play computer games. All participants had normal or corrected-to-normal vision, and none reported neurological, psychiatric, or cardiovascular disorders. This study was carried out in accordance with the recommendations of the guidelines established by the ethics committee for biomedical research at KU Leuven. All subjects gave written informed consent in accordance with the Declaration of Helsinki. The protocol was approved by the KU Leuven, Belgium.
Physical Activity
Total physical activity was assessed using the IPAQ, which assesses physical activity undertaken across a comprehensive set of domains including leisure time, domestic and gardening activities, and work-related and transport-related activities. The specific types of activity are walking, moderate-intensity activities, and vigorous-intensity activities. Frequency (days per week) and duration (time per day) are collected separately for each specific type of activity. The total score used to describe total physical activity required weighted summation of the duration (in minutes) and frequency (days) of walking, moderate-intensity, and vigorous-intensity activity. Each type of activity was weighted by its energy requirements defined in Metabolic Equivalent of Task (METs): 3.3 METs for walking, 4.0 METs for moderate physical activity, and 8.0 METs for vigorous physical activity (Ainsworth et al., 2000).
Experimental Setup
Skilled movement proficiency was assessed using a bimanual tracking task (Sisti et al., 2011; Beets et al., 2015; Serbruyns et al., 2015; Maes et al., 2017). Participants were seated in front of a computer monitor with the forearms resting on a custom-made adjustable ramp. A 5-cm diameter dial was mounted at the end of each ramp. The task consisted in turning the handle of the dials with the thumb and index according to specific patterns without visual feedback of the upper limbs. Angular displacements were registered by means of non-ferromagnetic optical shaft encoders (Avago Technologies, sampling frequency = 100 Hz, accuracy = 0.089°) fixed to the rotation axes of the dials. The gain was set to 10 arbitrary units (au) per rotation indicating that drawing a vertical or horizontal line on a screen consisting of 162 au, required 16.2 complete rotations of the left or right dial, respectively. Rotating the dials moved a red cursor on the screen serving as online visual feedback.
Bimanual Tracking Task
Participants were instructed to track a white target dot moving along a target line by rotating both dials simultaneously. Four coordination patterns imposed by the target line direction were tested: both hands rotating inward, outward, clockwise, or counter-clockwise. The left and right hands, respectively, controlled movements on the vertical and horizontal axis. Each pattern was performed according to five frequency ratios: 1:1, 1:2, 1:3, 2:1, and 3:1 (left hand:right hand) resulting in 20 different target line directions (Figure 2A).
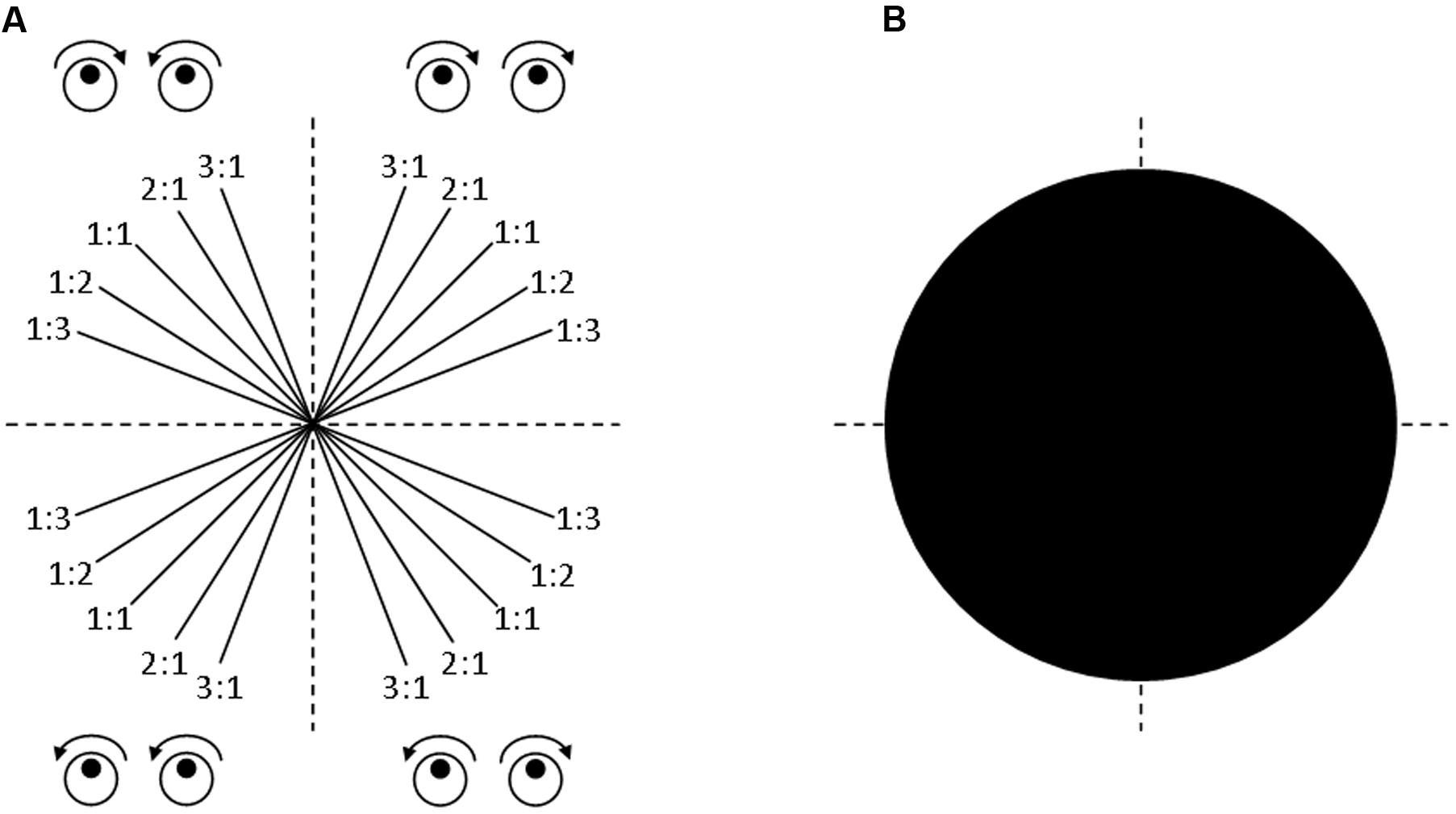
FIGURE 2. Bimanual coordination task. Illustration of the 20 bimanual coordination conditions (A). Considering these conditions as random in a linear mixed model allows the results to be generalized to all the possible conditions (B).
Procedure
Prior to data recording, participants performed 12 trials with different target lines for familiarization. Before each recorded trial, the target line appeared for 2 s. Then the target dot moved over the line at a constant speed from start (center of the screen) to end for 10 s. The goal was to match the white target dot movement with the red dot as accurately as possible in both space and time. The inter-trial interval was 3 s. Four 6-min blocks with 3 min rest in between were administered, each consisting of 24 trials, presented in a pseudorandom order. Therefore, all participants performed 96 recorded trials. Each block included all 20 distinctive target lines and an additional 1:1 trial for each coordination pattern.
Kinematic Data Analysis
Accuracy was assessed using the target deviation of the time series and was computed as follows for each trial:
where n is the number of data samples over a trial of 10 s (10 × 102), x2 and y2 are the respective position of the red cursor on the x- and y axis, and x1 and y1 are the respective position of the white target dot on the x- and y axis. Larger target deviation scores reflected poorer performance.
Statistical Analysis
The extent to which total physical activity, music practice, and computer gaming were predictive of target deviation was analyzed using a linear mixed model. Unlike traditional analyses of variance, linear mixed models take into account the sampling variability of both the participants and conditions, thereby limiting a large inflation of false positives (Boisgontier and Cheval, 2016). Moreover, as illustrated in Figure 2B, treating both participants and conditions as random effects allows generalizing the results to the population of participants, but also to the population of conditions (Barr et al., 2013). Finally, linear mixed models take each single observation into account, thereby avoiding information loss due to averaging. The linear mixed model specified participants (n = 26) and target lines (n = 20) as random factors and was built using the R language lmerTest package, version 2.0-301. Target deviation was normalized using the Box-Cox method. The predictive variable was total physical activity as measured by the IPAQ. The control variables were age, gender, session, trial order, physical inactivity, computer game practice, and music practice. The continuous variables were scaled and centered on zero.
Results
The distribution of physical activity, music practice, computer game practice, and physical inactivity are illustrated as a function of age in Figure 3.
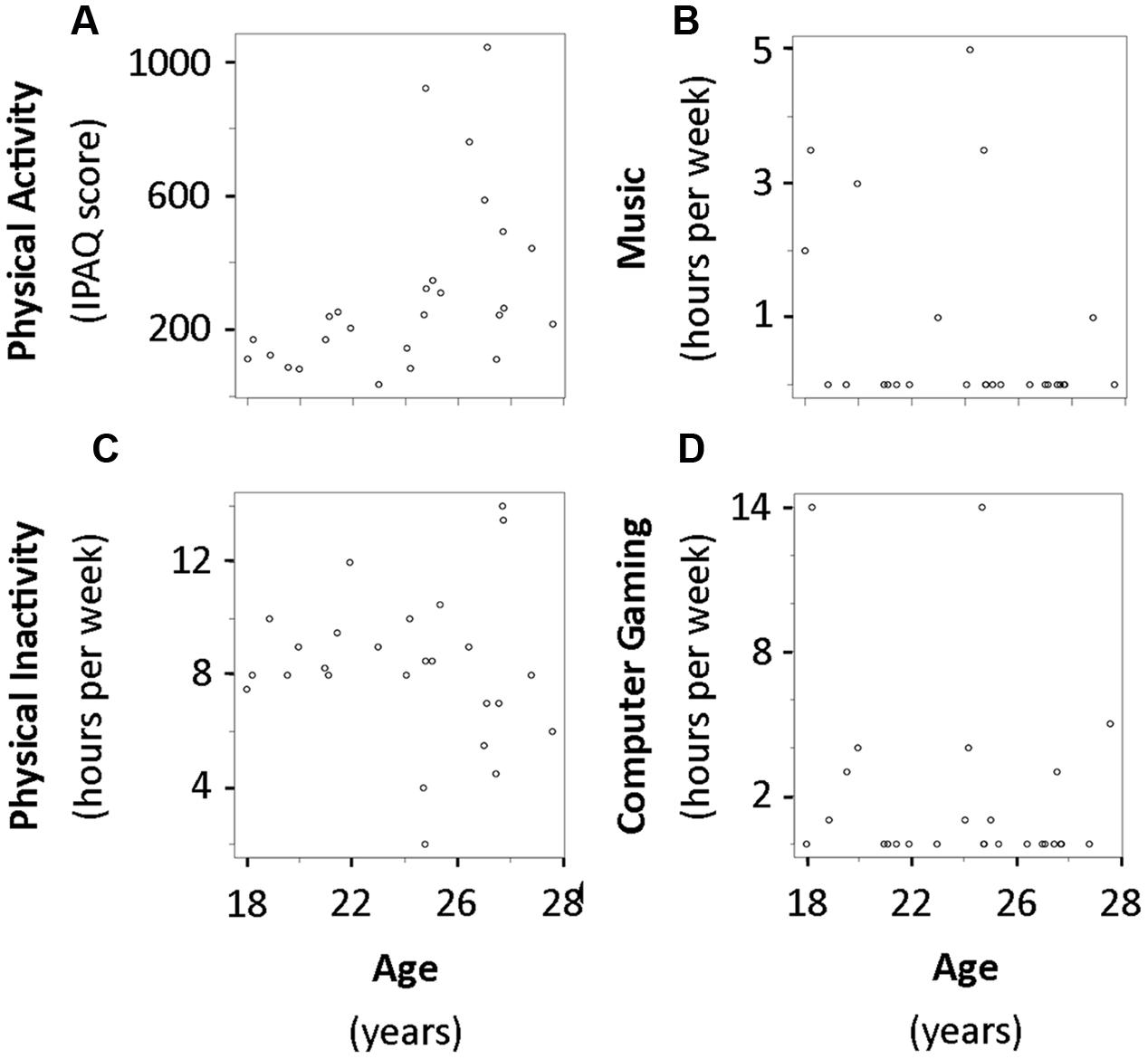
FIGURE 3. Raw data of PA (A), music practice (B), physical inactivity (C), and computer game practice (D) as a function of age. IPAQ, International Physical Activity Questionnaire.
Results of the linear mixed model (Table 1) showed a significant effect of physical activity (b = -0.335, p = 0.005) on target deviation with higher total physical activity predicting lower target deviation and thus better performance (Figure 4). This effect was observed while controlling for age (b = 0.142, p = 0.101), gender (b = -0.241, p = 0.178), session (b = -0.160, p < 0.001), trial order (b = -0.001, p = 0.660), physical inactivity (b = -0.140, p = 0.137), computer game practice (b = -0.021, p = 0.863), and music practice (b = -0.286, p = 0.009). These latter results showed that the number of hours of music practice per week, but not computer games practice, predicted lower target deviation.
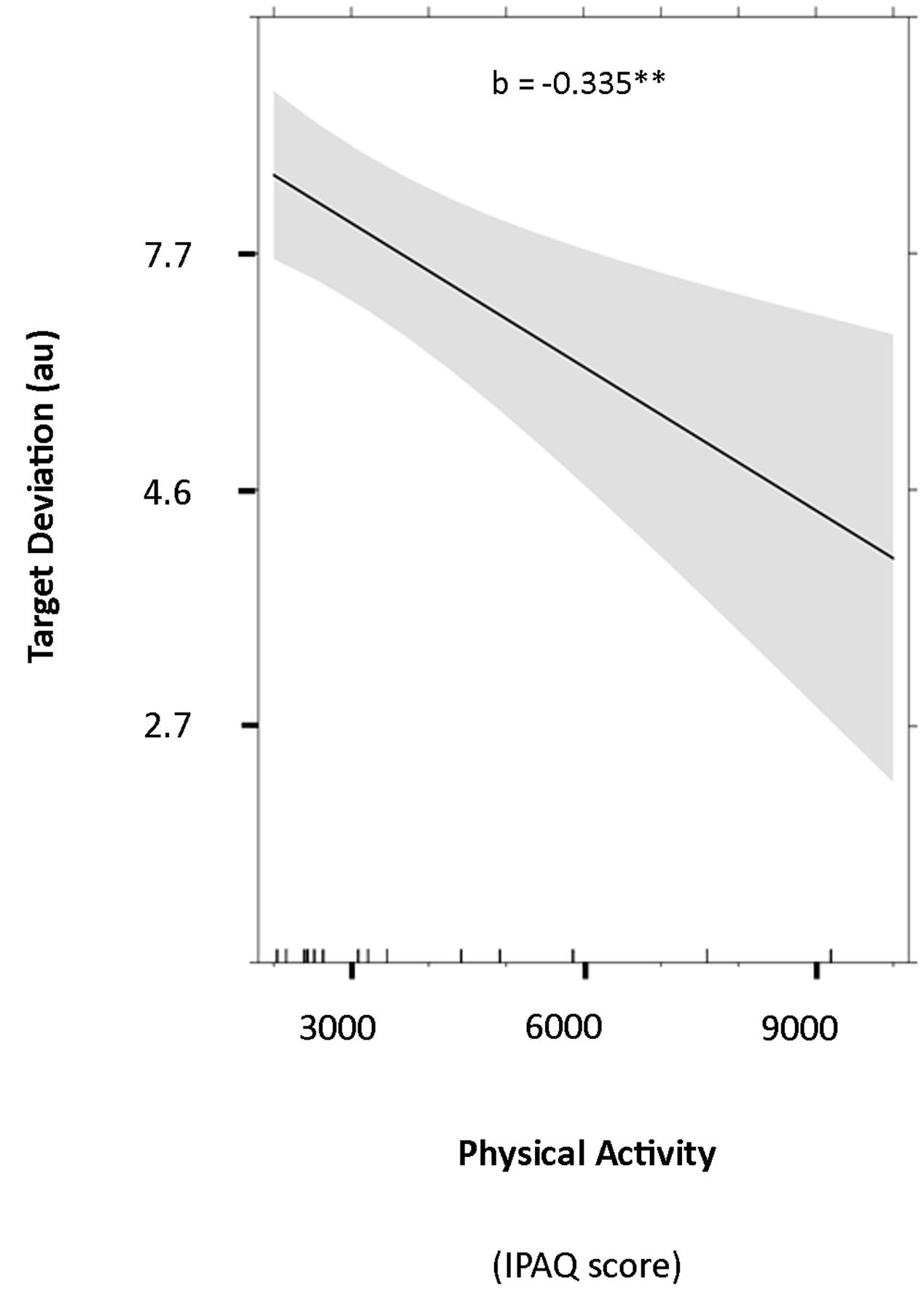
FIGURE 4. Fixed effect and the 95% confidence interval of total PA on target deviation. This effect was significant while controlling for all the other factors reported in Table 1. The scale of target deviation was back transformed. IPAQ, International Physical Activity Questionnaire. ∗∗p < 0.01.
Discussion
Here we investigated whether engagement in physical activity improves overall motor skill proficiency by means of an unpracticed bimanual coordination task. Results revealed that usual physical activity was predictive of performance in multiple conditions of this new and complex bimanual coordination task. This result is consistent with studies showing a relationship between the level of physical activity and motor speed. Specifically, in a discrete unimanual aiming task young active adults showed faster reaction times and movement times than sedentary young adults, while accuracy was similar (Van Halewyck et al., 2014; Helsen et al., 2016). Reaction times were also faster in active young and older adults than their sedentary peers (Lupinacci et al., 1993).
Our results demonstrate that higher total physical activity enhances performance on an unpracticed task. This result supports previous findings showing transfer between unrelated skills of a unimanual joystick tracking (Bock et al., 2001) or aiming task (Seidler, 2004) and reveals that such transfer can be demonstrated using a metric encompassing multiple skills (i.e., total physical activity). These findings run counter to the notion that motor abilities are highly specific and that practice effects are skill and context specific (e.g., Higbie et al., 1996). Conversely, our findings support the existence of a “learning to learn” skill (Harlow, 1949; Seidler, 2010) that could be improved through physical activity and that would impact performance in tasks that are not necessarily related to the practiced activity. Future studies should investigate, whether this impact of physical activity is related to the participants’ experience with a wide range of activities or to their overall level of fitness. Here, we showed that this impact was significant, irrespective of music practice, and computer gaming. Future research should also follow up on the significant relation between music practice and bimanual coordination to see if this relation is mediated by different processes or mechanisms other than those that underlie the relation between total physical activity and bimanual coordination.
Finally, as motor skills are known to be a primary factor for engagement in physical activity, these results are encouraging for the promotion of physical activity. Indeed, they reveal that once engaged in physical activity, this engagement could potentially be auto-reinforced through an overall improvement of motor skill proficiency.
Author Contributions
Experimental design: SS and LS. Experimental conduct: LS. Data analysis: MB. First draft preparation: MB. Manuscript preparation: MB and SS.
Conflict of Interest Statement
The authors declare that the research was conducted in the absence of any commercial or financial relationships that could be construed as a potential conflict of interest.
Acknowledgments
MB is supported by a research grant (1504015N) and a post-doctoral fellowship of the Research Foundation–Flanders (FWO). This study was supported by the FWO (G0721.12; G0708.14), the Interuniversity Attraction Poles Program initiated by the Belgian Science Policy Office (P7/11), and the KU Leuven Research Fund (C16/15/070).
Footnotes
References
Adamo, D. E., Alexander, N. B., and Brown, S. H. (2009). The influence of age and physical activity on upper limb proprioceptive ability. J. Aging Phys. Act. 17, 272–293. doi: 10.1123/japa.17.3.272
Ainsworth, B. E., Haskell, W. L., Whitt, M. C., Irwin, M. L., Swartz, A. M., and Strath, S. J. (2000). Compendium of physical activities: an update of activity codes and MET intensities. Med. Sci. Sports Exerc. 32, S498–S504. doi: 10.1097/00005768-200009001-00009
Bachman, J. C. (1961). Specificity vs. generality in learning and performing two large muscle motor tasks. Res. Q. 32, 3–31. doi: 10.1080/10671188.1961.10762064
Barnett, L. M., van Beurden, E., Morgan, P. J., Brooks, L. O., and Beard, J. R. (2009). Childhood motor skill proficiency as a predictor of adolescent physical activity. J. Adolesc. Health 44, 252–259. doi: 10.1016/j.jadohealth.2008.07.004
Barr, D. J., Levy, R., Scheepersm, C., and Tily, H. J. (2013). Random effects structure for confirmatory hypothesis testing: keep it maximal. J. Mem. Lang. 68, 255–278. doi: 10.1016/j.jml.2012.11.001
Beets, I. A., Gooijers, J., Boisgontier, M. P., Pauwels, L., Coxon, J. P., Wittenberg, G., et al. (2015). Reduced neural differentiation between feedback conditions after bimanual coordination training with and without augmented visual feedback. Cereb. Cortex 25, 1958–1969. doi: 10.1093/cercor/bhu005
Bock, O., Schneider, S., and Bloomberg, J. (2001). Conditions for interference versus facilitation during sequential sensorimotor adaptation. Exp. Brain Res. 138, 359–365. doi: 10.1007/s002210100704
Boisgontier, M. P., and Cheval, B. (2016). The anova to mixed model transition. Neurosci. Biobehav. Rev. 68, 1004–1005. doi: 10.1016/j.neubiorev.2016.05.034
Burdet, E., Osu, R., Franklin, D. W., Milner, T. E., and Kawato, M. (2001). The central nervous system stabilizes unstable dynamics by learning optimal impedance. Nature 414, 446–449. doi: 10.1038/35106566
Craig, C. L., Marshall, A. L., Sjostrom, M., Bauman, A. E., Booth, M. L., Ainsworth, B. E., et al. (2003). International physical activity questionnaire: 12-country reliability and validity. Med. Sci. Sports Exerc. 35, 1381–1395. doi: 10.1249/01.MSS.0000078924.61453.FB
Ding, D., Lawson, K. D., Kolbe-Alexander, T. L., Finkelstein, E. A., Katzmarzyk, P. T., van Mechelen, W., et al. (2016). The economic burden of physical inactivity: a global analysis of major non-communicable diseases. Lancet 388, 1311–1324. doi: 10.1016/S0140-6736(16)30383-X
Harlow, H. F. (1949). The formation of learning sets. Psychol. Rev. 56, 51–65. doi: 10.1037/h0062474
Helsen, W. F., Van Halewyck, F., Levin, O., Boisgontier, M. P., Lavrysen, A., and Elliott, D. (2016). Manual aiming in healthy aging: does proprioceptive acuity make the difference? Age 38:45. doi: 10.1007/s11357-016-9908-z
Higbie, E. J., Cureton, K. J., Warren, G. L. III, and Prior, B. M. (1996). Effects of concentric and eccentric training on muscle strength, cross-sectional area, and neural activation. J. Appl. Physiol. 81, 2173–2181.
Jackson, G. M., Jackson, S. R., Husain, M., Harvey, M., Kramer, T., and Dow, L. (2000). The coordination of bimanual prehension movements in a centrally deafferented patient. Brain 123, 380–393. doi: 10.1093/brain/123.2.380
Kraft, E. (2012). Cognitive function, physical activity, and aging: possible biological links and implications for multimodal interventions. Neuropsychol. Dev. Cogn. B Aging Neuropsychol. Cogn. 19, 248–263. doi: 10.1080/13825585.2011.645010
Lupinacci, N. S., Rikli, R. E., Jones, C. J., and Ross, D. (1993). Age and physical activity effects on reaction time and digit symbol substitution performance in cognitively active adults. Res. Q. Exerc. Sport 64, 144–150. doi: 10.1080/02701367.1993.10608791
Maes, C., Gooijers, J., Orban de Xivry, J. J., Swinnen, S. P., and Boisgontier, M. P. (2017). Two hands, one brain, and aging. Neurosci. Biobehav. Rev. 75, 234–256. doi: 10.1016/j.neubiorev.2017.01.052
Nougier, V., Bard, C., Fleury, M., Teasdale, N., Cole, J., Forget, R., et al. (1996). Control of single-joint movements in deafferented patients: evidence for amplitude coding rather than position control. Exp. Brain Res. 109, 473–482. doi: 10.1007/BF00229632
Oldfield, R. C. (1971). The assessment and analysis of handedness: the Edinburgh inventory. Neuropsychologia 9, 97–113. doi: 10.1016/0028-3932(71)90067-4
Sainburg, R. L., Ghilardi, M. F., Poizner, H., and Ghez, C. (1995). Control of limb dynamics in normal subjects and patients without proprioception. J. Neurophysiol. 73, 820–835.
Seidler, R. D. (2004). Multiple motor learning experiences enhance motor adaptability. J. Cogn. Neurosci. 16, 65–73. doi: 10.1162/089892904322755566
Seidler, R. D. (2010). Neural correlates of motor learning, transfer of learning, and learning to learn. Exerc. Sport Sci. Rev. 38, 3–9. doi: 10.1097/JES.0b013e3181c5cce7
Serbruyns, L., Gooijers, J., Caeyenberghs, K., Meesen, R. L., Cuypers, K., Sisti, H. M., et al. (2015). Bimanual motor deficits in older adults predicted by diffusion tensor imaging metrics of corpus callosum subregions. Brain Struct. Funct. 220, 273–290. doi: 10.1007/s00429-013-0654-z
Sisti, H. M., Geurts, M., Clerckx, R., Gooijers, J., Coxon, J. P., Heitger, M. H., et al. (2011). Testing multiple coordination constraints with a novel bimanual visuomotor task. PLoS ONE 6:e23619. doi: 10.1371/journal.pone.0023619
Stodden, D. F., Goodway, J. D., Langendorfer, S. J., Roberton, M. A., Rudisill, M. E., Garcia, C., et al. (2008). A developmental perspective on the role of motor skill competence in physical activity: an emergent relationship. Quest. 60, 290–306. doi: 10.1080/00336297.2008.10483582
Keywords: bimanual coordination, computer games, health, music, physical activity
Citation: Boisgontier MP, Serbruyns L and Swinnen SP (2017) Physical Activity Predicts Performance in an Unpracticed Bimanual Coordination Task. Front. Psychol. 8:249. doi: 10.3389/fpsyg.2017.00249
Received: 09 November 2016; Accepted: 08 February 2017;
Published: 20 February 2017.
Edited by:
Maarten A. Immink, University of South Australia, AustraliaReviewed by:
David Ian Anderson, San Francisco State University, USAJason B. Boyle, The University of Texas at El Paso, USA
Copyright © 2017 Boisgontier, Serbruyns and Swinnen. This is an open-access article distributed under the terms of the Creative Commons Attribution License (CC BY). The use, distribution or reproduction in other forums is permitted, provided the original author(s) or licensor are credited and that the original publication in this journal is cited, in accordance with accepted academic practice. No use, distribution or reproduction is permitted which does not comply with these terms.
*Correspondence: Matthieu P. Boisgontier, bWF0dGhpZXUuYm9pc2dvbnRpZXJAa3VsZXV2ZW4uYmU=