- 1Centre for Cognitive Neuroscience, Duke-NUS Medical School, Singapore, Singapore
- 2Institue of Behavioral Sciences, Medical School, University of Pécs, Pécs, Hungary
- 3Department of Psychology, Education, and Child Studies, Erasmus University, Rotterdam, Netherlands
Prolonged active engagement on cognitively demanding tasks often leads to a subjective state labeled cognitive fatigue (Meijman, 1991; Lorist et al., 2000). Although such fatigue is considered to be a complex, multifaceted state involving various causes and effects, it is widely acknowledged that reduced motivation for effort is one of its key aspects (van der Linden, 2011). Accordingly, there seems to be agreement that performance deficits in fatigue are likely to reflect a combination of reduced capacity and reduced willingness to perform (Kanfer and Ackerman, 1989; Meijman, 1991; Hockey, 1997). Despite such consensus, however, only a handful of studies have explicitly targeted the motivational factors that determine performance levels during fatigue. A potential reason for this lack of formal studies could be that motivation is particularly difficult to measure in other ways than by self-report, with the obvious drawbacks that subjects may not always be willing to report loss of motivation or may not be aware of it. In this paper, we argue that recently developed methods and insights from the field of effort-based decision making may help to elucidate how fatigue changes the motivation to perform (Chong et al., 2016; Pessiglione et al., 2017). We will discuss the parallels between theoretical models of fatigued performance and models of effort-based decision making. Further, we will discuss how methods from the effort-based decision-making field can be used to study motivational decline in fatigue-related conditions.
Fatigue, Performance and Effort: An Old Tradition
Researchers as early as Thorndike (1900) have observed that performance decline due to fatigue may depend on a reduced desire to exert further effort. A wide range of earlier theoretical models have proposed that performance critically depends on the motivated allocation of processing resources (Bartley and Chute, 1947; Kahneman, 1973; Kanfer and Ackerman, 1989; Hockey, 1997). Under fatigue, the total available resources may decline, even though that is still a matter of debate (Inzlicht et al., 2014; Christie and Schrater, 2015). More relevant here, however, is that fatigue may also act to shift performance priorities. The Motivational Control Model by Hockey (1997, 2011) describes how performance under demanding conditions (e.g., stress, fatigue) depends on mobilizing required cognitive resources. If task goals are deemed sufficiently important, allocation of such resources can be channeled through exertion of compensatory effort. Yet, this comes at the expense of increased discomfort. Alternatively, task goals could be adjusted or even abandoned. Management of effort allocation and goal selection would be arbitrated by higher-order control functions that take input from effort and goal monitoring mechanisms. A model by Boksem and Tops (2008) takes a biological perspective, and describes how effort allocation relies on a constant monitoring of the energetic costs of performance, weighted against the value of its outcomes (e.g., food or monetary reward obtained). Several brain areas are proposed to coordinate effort monitoring (e.g., anterior insula), reward (e.g., nucleus accumbens) and action outcomes (e.g., anterior cingulate cortex). Actions are only engaged when task goals are deemed sufficiently important. Both these models imply some degree of volitional regulation of resource allocation based on internal cost-benefit weighing mechanism.
This idea has been further formalized in the Integrated Resource-Allocation model by Kanfer and Ackerman (1989) and Kanfer (1990, 2011), stating that the relationships between effort, performance, and outcome value can be expressed in subjective utility functions. These functions would describe how the subjective utility of outcomes increases with better performance, and decreases with increased effort exertion. Decisions on how much effort to exert would depend on finding the optimal balance between effort, performance and utility. Under fatigue, the disutility of effort would increase, leading to less allocation of effort (Kanfer, 2011). Two more recent models similarly describe how performance levels relate to weighted decisions based on the value of the task at hand, versus the value of alternative action options (opportunity cost; Kurzban et al., 2013) (self-control depletion; Inzlicht et al., 2014).
Given the general emphasis on effort allocation in fatigue, it is surprising that only very few empirical studies have targeted this area directly. Some studies showed that motivational incentives (e.g., monetary reward) can lead to improved performance under fatigue (Boksem et al., 2006; Hopstaken et al., 2014, 2015, 2016; but see Gergelyfi et al., 2015). A different approach was applied by Holding and colleagues (Shingledecker and Holding, 1974; Holding et al., 1983) who assessed effort allocation through a decision-making paradigm. To complete a task (detecting a fault in an electrical circuit), participants could choose between an effortful strategy (checking multiple circuits) with a higher probability of correct performance, or a less effortful strategy (checking only one circuit) with higher risk of failure. Critically, fatigued participants chose the low-effort strategy more often than well-rested participants. As these studies were the first to operationalize the assumed cost-benefit analysis explicitly as a decision process, they hold important theoretical value for the field of cognitive fatigue. Nevertheless, decision-making methodologies as used by Holding have seldom been adopted in later fatigue research.
Effort-Based Decision Making: An Emerging Field
The separate field of decision neuroscience, which is particularly involved in studying decision processes, has recently shown a surge in interest in effort-based decisions. Inspired by animal studies on motivation (Salamone et al., 1991; Walton et al., 2002; Rudebeck et al., 2006), and economic theory on expected utility (Von Neumann and Morgenstern, 1944), researchers have started to investigate how humans integrate effort and reward information in their decisions to act (Botvinick et al., 2009; Treadway et al., 2009; Kurniawan et al., 2010; Prévost et al., 2010). Similar to fatigue theory, it is proposed that the choice to engage in an action results from a weighing of action-costs (e.g., effort) against the value of its outcomes (Westbrook and Braver, 2015; Kool et al., 2017; Shenhav et al., 2017). If the required effort is high, the decision maker may assign less value to a reward compared to when effort is low. In other words, reward value is discounted based on effort costs (Westbrook et al., 2013).
A variety of paradigms has been developed to assess the influence of effort and reward on decision making (for reviews see Chong et al., 2016; Pessiglione et al., 2017). Typically, participants are given choices between performance of an effortful task, in return for a large reward, or a non-effortful task for a lower reward (Figure 1A). By sampling an individual's preference over a wide range of reward levels, a slope can be calculated that plots the willingness to accept the effort (Figure 1B). An indifference point, i.e., the reward level at which the effortful and non-effortful rewards are deemed equally attractive, can be determined over a range of effort levels, forming a discounting curve (Figure 1C). Much like the decision-making paradigms used by Holding and colleagues (Shingledecker and Holding, 1974; Holding et al., 1983), effort-discounting relies on the individual's choice of action. The particular advantage lies in the potential to estimate an integrated effort-reward value and its changes under conditions such as fatigue, as proposed by Kanfer (2011).
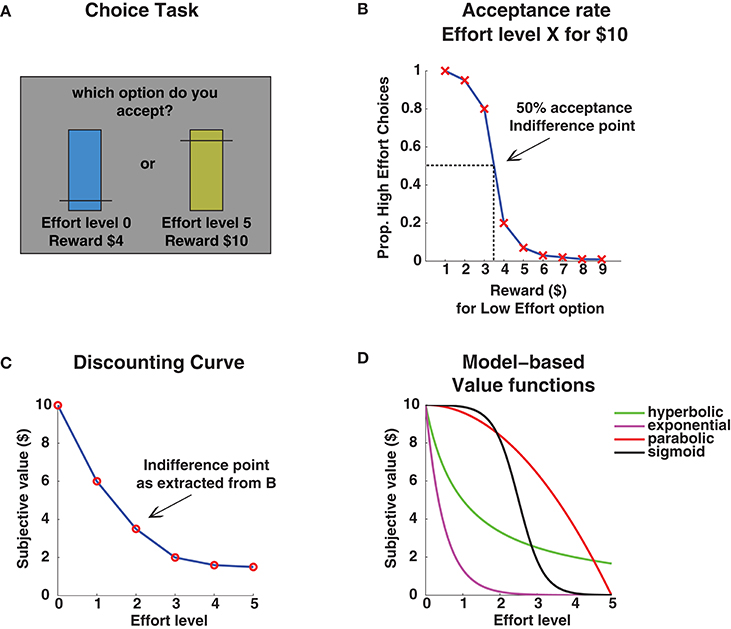
Figure 1. (A) Example choice trial, (B) Determination of indifference point, (C) Indifference points for different effort levels, (D) Theoretical discount functions.
A major methodological asset is that, through computational modeling, normative mathematical functions can be fit to behavioral choices (Figure 1D; Prévost et al., 2010; Klein-Flügge et al., 2015; Zénon et al., 2016; Chong et al., 2017). This helps to formalize predictions and extrapolate beyond the specific test set. Moreover, it allows to incorporate biologically plausible cost-functions, which greatly improves predictions of behavioral and neuroimaging/physiological data (Manohar et al., 2015; Klein-Flügge et al., 2016; Le Bouc et al., 2016). It is still debated whether the effort-costs can be captured by a singular value-function (particularly in the domain of cognitive effort; Białaszek et al., 2017; Chong et al., 2017; Massar et al., 2018), however, computational approaches can strongly aid to generate testable hypotheses about the distinct cognitive and neurobiological mechanisms affected (e.g., motivation versus capacity deficits; Le Bouc et al., 2016).
Fatigue and Effort-Based Decision Making: A Way Forward
We propose that fatigue research could greatly benefit from more integration of methods from decision neuroscience. Particularly, predictions from fatigue models, that have thus far remained untested could be directly examined. A starting point would be to model the effort-value function before and after a fatigue induction. A central prediction from fatigue theories would be that, under fatigue, the integrated effort-value function would be shifted toward a diminished preference for effort (Kanfer, 2011). Similar findings have been reported in related areas like sleep deprivation and physical fatigue (Libedinsky et al., 2013; Iodice et al., 2017; Massar et al., 2018), but not yet for cognitive fatigue.
Importantly, it could be tested how changes in effort-discounting relate to alterations in task performance and changes in the subjective sensation of fatigue. Several models describe subjective fatigue (or associated discomfort and effort sensation) as an internal signal that biases behavior away from non-rewarding activities (Boksem and Tops, 2008; van der Linden, 2011; Kurzban et al., 2013). Models differ slightly in the exact role they propose that subjective fatigue has in the effort-reward weighing process, but all would predict that higher felt fatigue would relate to stronger effort-avoidance. It should be noted that a recent study that looked at this relationship, did not find significant correlations between effort-discounting and subjective fatigue (Benoit et al., in review). Despite this initial negative result, we argue that more research is needed to further test the above described possibilities.
With regard to performance, effort-discounting information could be used further delineate the effects of time-on-tasks versus recovery. Studies on physical effort have already modeled how fatigue accumulates with prolonged muscle contraction, and dissipates with rest (Meyniel et al., 2013), and how this changes over different effort and reward conditions. Similarly, for cognitive performance, decline with time-on-task, and recovery with rest have been topics of investigation (Ross et al., 2014; Lim and Kwok, 2016), but have not yet been modeled in light of effort-reward tradeoffs. A similar modeling approach could be used to describe fluctuations in cognitive performance over time, formalizing the effort management process as proposed by Hockey (1997, 2011).
Furthermore, an important area where effort-based decision methods could inform fatigue research is in examining the neural mechanisms underlying motivation decline. Neuro-economic studies have revealed a particular set of brain areas and networks involved in reward valuation, effort evaluation, and subjective value computation (e.g., ventral striatum, anterior insula, anterior cingulate cortex: Prévost et al., 2010; Bartra et al., 2013; Meyniel et al., 2013; Apps et al., 2015; Massar et al., 2015; Klein-Flügge et al., 2016), many of which converge with the neural framework of fatigue as proposed by Boksem and Tops (2008). Any shifts in behavioral preference during fatigue, would likely be accompanied by alterations in the way these neural systems would interact. Studying how such changes in neural function would relate to behavioral preference and performance decrement may provide key insights into the motivational effects of fatigue.
A related question is whether effects of fatigue would transfer across tasks, or alternatively be more task-specific. Different tasks have been used in effort-based decision studies (e.g., working memory, task-switching, sustained attention; Kool et al., 2010; Westbrook et al., 2013; Apps et al., 2015; Massar et al., 2016), and different tasks have resulted in distinct carry-over effects after fatigue induction (Massar et al., 2010). It is therefore possible that any changes in effort-preference would depend on the overlap in brain circuitry that is being taxed during fatigue induction (Blain et al., 2016).
Conclusion
In this paper, we have outlined how motivation and effort considerations have long been influential in theoretical models of fatigue, and how these ideas hold strong parallels with more recent theories of effort-based decision making. Although some researchers have started to explore the overlap, both fields still largely exist as separate areas. We would urge for a much stronger integration of these fields, and the adoption of decision methods to inform fatigue research. We are not the first to advocate the theoretical link between these fields, but we argue that the methodological development of effort-based decision making has now advanced to such extent that it can strongly accelerate insights in fatigue research.
Author Contributions
SM drafted the first version of the manuscript. DvdL and ÁC participated in writing and critical revision of the manuscript. All authors approved the final version.
Conflict of Interest Statement
The authors declare that the research was conducted in the absence of any commercial or financial relationships that could be construed as a potential conflict of interest.
The reviewer JJ and handling editor declared their shared affiliation.
Acknowledgments
ÁC was supported by National Research, Development and Innovation Office (NKFIH K120012).
References
Apps, M., Grima, L. L., Manohar, S., and Husain, M. A. (2015). The role of cognitive effort in subjective reward devaluation and risky decision-making. Sci. Rep. 5:16880. doi: 10.1038/srep16880
Bartra, O., Mcguire, J. T., and Kable, J. W. (2013). The valuation system: a coordinate-based meta-analysis of BOLD fMRI experiments examining neural correlates of subjective value. NeuroImage 76, 412–427. doi: 10.1016/j.neuroimage.2013.02.063
Białaszek, W., Marcowski, P., and Ostaszewski, P. (2017). Physical and cognitive effort discounting across different reward magnitudes: tests of discounting models. PLoS ONE 12:e0182353. doi: 10.1371/journal.pone.0182353
Blain, B., Hollard, G., and Pessiglione, M. (2016). Neural mechanisms underlying the impact of daylong cognitive work on economic decisions. Proc. Natl. Acad. Sci. U.S.A. 113, 6967–6972. doi: 10.1073/pnas.1520527113
Boksem, M. A., Meijman, T. F., and Lorist, M. M. (2006). Mental fatigue, motivation and action monitoring. Biol. Psychol. 72, 123–132. doi: 10.1016/j.biopsycho.2005.08.007
Boksem, M. A., and Tops, M. (2008). Mental fatigue: costs and benefits. Brain Res. Rev. 59, 125–139. doi: 10.1016/j.brainresrev.2008.07.001
Botvinick, M. M., Huffstetler, S., and Mcguire, J. T. (2009). Effort discounting in human nucleus accumbens. Cogn. Affect. Behav. Neurosci. 9, 16–27. doi: 10.3758/CABN.9.1.16
Chong, T. T., Apps, M., Giehl, K., Sillence, A., Grima, L. L., and Husain, M. (2017). Neurocomputational mechanisms underlying subjective valuation of effort costs. PLoS Biol. 15:e1002598. doi: 10.1371/journal.pbio.1002598
Chong, T. T., Bonnelle, V., and Husain, M. (2016). Quantifying motivation with effort-based decision-making paradigms in health and disease. Prog. Brain Res. 229, 71–100. doi: 10.1016/bs.pbr.2016.05.002
Christie, S. T., and Schrater, P. (2015). Cognitive cost as dynamic allocation of energetic resources. Front. Neurosci. 9:289. doi: 10.3389/fnins.2015.00289
Gergelyfi, M., Jacob, B., Olivier, E., and Zénon, A. (2015). Dissociation between mental fatigue and motivational state during prolonged mental activity. Front. Behav. Neurosci. 9:176. doi: 10.3389/fnbeh.2015.00176
Hockey, G. R. (1997). Compensatory control in the regulation of human performance under stress and high workload; a cognitive-energetical framework. Biol. Psychol. 45, 73–93. doi: 10.1016/S0301-0511(96)05223-4
Hockey, G. R. (2011). “A motivational control theory of cognitive fatigue,” in Cognitive fatigue: Multidisciplinary Perspectives on Current Research and Future Applications, ed P. L. Ackerman (Washington, DC: American Psychological Association), 167–187.
Holding, D. H., Loeb, M., and Baker, M. A. (1983). Effects and aftereffects of continuous noise and computation work on risk and effort choices. Motiv. Emot. 7, 331–344. doi: 10.1007/BF00991643
Hopstaken, J. F., van der Linden, D., Bakker, A. B., and Kompier, M. A. J. (2014). A multifaceted investigation of the link between mental fatigue and task disengagement. Psychophysiology 52, 305–315. doi: 10.1111/psyp.12339
Hopstaken, J. F., van der Linden, D., Bakker, A. B., and Kompier, M. A. J. (2015). The window of my eyes: task disengagement and mental fatigue covary with pupil dynamics. Biol. Psychol. 110, 100–106. doi: 10.1016/j.biopsycho.2015.06.013
Hopstaken, J. F., van der Linden, D., Bakker, A. B., Kompier, M. A. J., and Leung, Y. K. (2016). Shifts in attention during mental fatigue: evidence from subjective, behavioral, physiological, and eye-tracking data. J. Exp. Psychol. Hum. Percept. Perform. 42, 878–889. doi: 10.1037/xhp0000189
Inzlicht, M., Schmeichel, B. J., and Macrae, C. N. (2014). Why self-control seems (but may not be) limited. Trends Cogn. Sci. 18, 127–133. doi: 10.1016/j.tics.2013.12.009
Iodice, P., Calluso, C., Barca, L., Bertollo, M., Ripari, P., and Pezzulo, G. (2017). Fatigue increases the perception of future effort during decision making. Psychol. Sport Exercise 33, 150–160. doi: 10.1016/j.psychsport.2017.08.013
Kanfer, R. (1990). “Motivation theory and Industrial/Organizational psychology,” in Handbook of Industrial and Organizational Psychology, Theory in Industrial and Organizational Psychology, Vol. 1, eds M. D. Dunnette and L. Hough (Palo Alto, CA: Consulting Psychologists Press), 75–170.
Kanfer, R. (2011). “Determinants and consequences of subjective cognitive fatigue,” in Cognitive Fatigue: Multidisciplinary Perspectives on Current Research and Future Applications, ed P. L. Ackerman (Washington, DC: American Psychological Association), 189–207.
Kanfer, R., and Ackerman, P. L. (1989). Motivation and cognitive abilities: an integrative/aptitude-treatment interaction approach to skill acquisition. J. Appl. Psychol. 74, 657–690. doi: 10.1037/0021-9010.74.4.657
Klein-Flügge, M. C., Kennerley, S. W., Friston, K., and Bestmann, S. (2016). Neural signatures of value comparison in human cingulate cortex during decisions requiring an effort-reward trade-off. J. Neurosci. 36, 10002–10015. doi: 10.1523/JNEUROSCI.0292-16.2016
Klein-Flügge, M. C., Kennerley, S. W., Saraiva, A. C., Penny, W. D., and Bestmann, S. (2015). Behavioral modeling of human choices reveals dissociable effects of physical effort and temporal delay on reward devaluation. Plos Comput. Biol. 11:e1004116. doi: 10.1371/journal.pcbi.1004116
Kool, W., Mcguire, J. T., Rosen, Z. B., and Botvinick, M. M. (2010). Decision making and the avoidance of cognitive demand. J. Exp. Psychol. 139, 665–682. doi: 10.1037/a0020198
Kool, W., Shenhav, A., and Botvinick, M. M. (2017). Cognitive Control as Cost-Benefit Decision Making, Vol. 35. Chichester: John Wiley & Sons, Ltd.
Kurniawan, I. T., Seymour, B., Talmi, D., Yoshida, W., Chater, N., and Dolan, R. J. (2010). Choosing to make an effort: the role of striatum in signaling physical effort of a chosen action. J. Neurophysiol. 104, 313–321. doi: 10.1152/jn.00027.2010
Kurzban, R., Duckworth, A., Kable, J. W., and Myers, J. (2013). An opportunity cost model of subjective effort and task performance. Behav. Brain Sci. 36, 661–679. doi: 10.1017/S0140525X12003196
Le Bouc, R., Rigoux, L., Schmidt, L., Degos, B., Welter, M.-L., Vidailhet, M., et al. (2016). Computational dissection of dopamine motor and motivational functions in humans. J. Neurosci. 36, 6623–6633. doi: 10.1523/JNEUROSCI.3078-15.2016
Libedinsky, C., Massar, S. A. A., Ling, A., Chee, W. Y., Huettel, S. A., and Chee, M. W. L. (2013). Sleep deprivation alters effort discounting but not delay discounting of monetary rewards. Sleep 36, 899–904. doi: 10.5665/sleep.2720
Lim, J., and Kwok, K. (2016). The effects of varying break length on attention and time on task. Hum. Fact. 58, 472–481. doi: 10.1177/0018720815617395
Lorist, M. M., Klein, M., Nieuwenhuis, S., De Jong, R., Mulder, G., and Meijman, T. F. (2000). Mental fatigue and task control: planning and preparation. Psychophysiology 37, 614–625. doi: 10.1111/1469-8986.3750614
Manohar, S. G., Chong, T. T.-J., Apps, M. A. J., Batla, A., Stamelou, M., Jarman, P. R., et al. (2015). Reward pays the cost of noise reduction in motor and cognitive control. Curr. Biol. 25, 1707–1716. doi: 10.1016/j.cub.2015.05.038
Massar, S. A., Libedinsky, C., Weiyan, C., Huettel, S. A., and Chee, M. W. (2015). Separate and overlapping brain areas encode subjective value during delay and effort discounting. NeuroImage 120, 104–113. doi: 10.1016/j.neuroimage.2015.06.080
Massar, S. A. A., Lim, J., Sasmita, K., and Chee, M. W. L. (2018). Sleep deprivation increases the costs of attentional effort: performance, preference and pupil size. Neuropsychologia. doi: 10.1016/j.neuropsychologia.2018.03.032. [Epub ahead of print].
Massar, S. A., Lim, J., Sasmita, K. S., and Chee, M. (2016). Rewards boost sustained attention through higher effort: a value-based decision making Approach. Biol. Psychol. 120, 21–27. doi: 10.1016/j.biopsycho.2016.07.019
Massar, S. A., Wester, A. E., Volkerts, E. R., and Kenemans, J. L. (2010). Manipulation specific effects of mental fatigue: evidence from novelty processing and simulated driving. Psychophysiology 47, 1119–1126. doi: 10.1111/j.1469-8986.2010.01028.x
Meijman, T. (1991). Over Vermoeidheid: Arbeidspsychologische Stud ies Naar Beleving Van Belastingseffecten (Fatigue: Studies on the Perception of Workload Effects). Dissertation, Amsterdam: University of Amsterdam.
Meyniel, F., Sergent, C., Rigoux, L., Daunizeau, J., and Pessiglione, M. (2013). Neurocomputational account of how the human brain decides when to have a break. Proc. Natl. Acad. Sci. U.S.A. 110, 2641–2646. doi: 10.1073/pnas.1211925110
Pessiglione, M., Vinckier, F., Bouret, S., Daunizeau, J., and Le Bouc, R. (2017). Why not try harder? Computational approach to motivation deficits in neuro-psychiatric diseases. Brain 14, 629–650. doi: 10.1093/brain/awx278
Prévost, C., Pessiglione, M., Météreau, E., Cléry-Melin, M.-L., and Dreher, J.-C. (2010). Separate valuation subsystems for delay and effort decision costs. J. Neurosci. 30, 14080–14090. doi: 10.1523/JNEUROSCI.2752-10.2010
Ross, H. A., Russell, P. N., and Helton, W. S. (2014). Effects of breaks and goal switches on the vigilance decrement. Exp. Brain Res. 232, 1729–1737. doi: 10.1007/s00221-014-3865-5
Rudebeck, P. H., Walton, M. E., Smyth, A. N., Bannerman, D. M., and Rushworth, M. F. S. (2006). Separate neural pathways process different decision costs. Nat. Neurosci. 9, 1161–1168. doi: 10.1038/nn1756
Salamone, J. D., Steinpreis, R. E., McCullough, L. D., Smith, P., Grebel, D., and Mahan, K. (1991). Haloperidol and nucleus accumbens dopamine depletion suppress lever pressing for food but increase free food consumption in a novel food choice procedure. Psychopharmacology 104, 515–521. doi: 10.1007/BF02245659
Shenhav, A., Musslick, S., Lieder, F., Kool, W., Griffiths, T. L., Cohen, J. D., et al. (2017). Toward a rational and mechanistic account of mental effort. Annu. Rev. Neurosci. 40, 99–124. doi: 10.1146/annurev-neuro-072116-031526
Shingledecker, C. A., and Holding, D. H. (1974). Risk and effort measures of fatigue. J. Motor Behav. 6, 17–25. doi: 10.1080/00222895.1974.10734975
Treadway, M. T., Buckholtz, J. W., Schwartzman, A. N., Lambert, W. E., and Zald, D. H. (2009). Worth the “EEfRT”? The effort expenditure for rewards task as an objective measure of motivation and anhedonia. PLoS ONE 4:e6598. doi: 10.1371/journal.pone.0006598
van der Linden, D. (2011). “The urge to stop: the cognitive and biological nature of acute mental fatigue,” in Cognitive Fatigue: Multidisciplinary Perspectives on Current Research and Future Applications, 1st Edn, ed P. L. Ackerman (Washington, DC: American Psychological Association), 149–164.
Von Neumann, J., and Morgenstern, O. (1944). The Theory of Games And Economic Behavior. Princeton, NJ: Princeton University Press.
Walton, M. E., Bannerman, D. M., and Rushworth, M. F. S. (2002). The role of rat medial frontal cortex in effort-based decision making. J. Neurosci. 22, 10996–11003. doi: 10.1523/JNEUROSCI.22-24-10996.2002
Westbrook, A., and Braver, T. S. (2015). Cognitive effort: a neuroeconomic approach. Cogn. Affect. Behav. Neurosci. 15, 395–415. doi: 10.3758/s13415-015-0334-y
Westbrook, A., Kester, D., and Braver, T. S. (2013). What is the subjective cost of cognitive effort? Load, trait, and aging effects revealed by economic preference. PLoS ONE 8:e68210. doi: 10.1371/journal.pone.0068210
Keywords: fatigue, motivation, performance, cognitive effort, effort-based decision-making
Citation: Massar SAA, Csathó Á and Van der Linden D (2018) Quantifying the Motivational Effects of Cognitive Fatigue Through Effort-Based Decision Making. Front. Psychol. 9:843. doi: 10.3389/fpsyg.2018.00843
Received: 22 December 2017; Accepted: 11 May 2018;
Published: 30 May 2018.
Edited by:
Monicque Lorist, University of Groningen, NetherlandsReviewed by:
Erik Bijleveld, Radboud University Nijmegen, NetherlandsJacob Jolij, University of Groningen, Netherlands (M. de Jong contributed to the review of Jacob Jolij)
Copyright © 2018 Massar, Csathó and Van der Linden. This is an open-access article distributed under the terms of the Creative Commons Attribution License (CC BY). The use, distribution or reproduction in other forums is permitted, provided the original author(s) and the copyright owner are credited and that the original publication in this journal is cited, in accordance with accepted academic practice. No use, distribution or reproduction is permitted which does not comply with these terms.
*Correspondence: Stijn A. A. Massar, c3Rpam5tYXNzYXJAZ21haWwuY29t