- 1Colin Powell School for Civic and Global Leadership, Department of Psychology, The City College of New York, New York, NY, United States
- 2School of Economics and Business, Department of Marketing, University of Sarajevo, Sarajevo, Bosnia and Herzegovina
The goals of this study were to identify groups of health-related behaviors among young adults (N = 314, Mage = 21.94, SD = 6.53), gauge the relation between emotional intelligence and health behaviors in this population, and assess health consciousness as mediator of said relation. Latent class analysis identified two mutually exclusive health behavior groups, which according to response patterns were labeled as Healthy and Unhealthy. The Healthy group (56%) was composed of individuals who had a healthy diet (i.e., low fat and high fiber), exercised regularly, and who frequently engaged in behaviors that prevent oral and skin-related diseases. In contrast, the Unhealthy group (44%) rarely engaged in these health-promoting behaviors. Using structural equation modeling we found a negative relation between emotional intelligence and unhealthy behaviors relative to health-promoting ones. Mediation analyses indicated that the mechanism explaining said relation was through increments in health consciousness, with large standardized indirect effects ranging between -0.52 and -0.78. As health behaviors during early adulthood are salient predictors of health outcomes in old age, the results have clear implications for the inclusion of emotional intelligence training in programs seeking to raise health awareness and cultivate health promoting behaviors in young adults, in so much as to seek to reduce the risk of chronic ailments later in life.
Introduction
Studies show that prolonged participation in poor health practices such as unhealthy eating, low physical activity, and noncompliance with disease detection and prevention guidelines are salient markers of adverse physical health conditions later in life (Rimm et al., 1995; Colditz et al., 2000; Patel et al., 2010; Chiuve et al., 2012). In particular, unhealthy eating and low physical activity promote obesity, which is a major contributor for diabetes, heart disease, and other chronic health problems in later years (Must et al., 1999; Kopelman, 2000; Bogers et al., 2007). Long-term smoking and excessive alcohol use increase the risk of developing heart and pulmonary disease as well as multiple forms of cancer (U.S. Department of Health and Human Services, 2010). Failure to participate in disease screening and prevention practices increase the risk of developing multiple illnesses and additional health complications later in life (Ajwani et al., 2003; Yach et al., 2004; Adolph et al., 2017). Therefore, research identifying the factors related to health-promoting behaviors is vital for creating interventions aimed at reducing the risk of illness, particularly among young adults whose health behaviors are susceptible to change (Frech, 2012; Johnstone and Hooper, 2016; Daw et al., 2017) and will have important health implications in late adulthood (Anderson and Horvath, 2004; National Research Council and Institute of Medicine of the National Academies, 2009; Park et al., 2014). Two psychological concepts, emotional intelligence and health consciousness, have been identified as important predictors of health behaviors.
Emotional intelligence (EI), a concept derived from principles of social intelligence (Petrides, 2011), refers to a person’s emotional competence in social interactions, particularly the perception, understanding, expression, use and regulation of feelings and emotions (Petrides and Furnham, 2001; Wong and Law, 2002; Johnson et al., 2009; Nelis et al., 2011). Substantial Meta-analytic evidence has documented EI as a positive predictor of physical and mental health (Schutte et al., 2007; Martins et al., 2010), and related studies have found EI to be correlated with health behaviors (Petrides et al., 2016). In particular, studies have shown that individuals with high EI actively participate in health-promoting behaviors, such as following a healthy diet as well as engaging in physical activity (Saklofske et al., 2007a,b; Fernández-Abascal and Martín-Díaz, 2015; Mikolajczak et al., 2015). Studies have also found that low EI is associated with the use of health-impairing substances, including alcohol, tobacco, and illicit drugs (Trinidad and Johnson, 2002; Riley and Schutte, 2003; Brackett et al., 2004; Trinidad et al., 2004). In sum, EI is a widely documented construct that relates to improved health and related behaviors.
Health consciousness corresponds to self-awareness about one’s health, and the willingness to engage in health and wellness promoting behaviors (Gould, 1988, 1990; Michaelidou and Hassan, 2008). Not surprisingly, health conscious individuals actively seek for information about how to improve their health, and adhere accordingly (Iversen and Kraft, 2006; Dutta and Feng, 2007; Basu and Dutta, 2008). Hence, individuals with high health consciousness have positive attitudes about nutrition, self-care and exercise, and accordingly have healthier lifestyles than individuals with low health consciousness (Hollis et al., 1986; Kraft and Goodell, 1993; Hoek et al., 2004; Chen, 2009). Such healthier lifestyles also include frequently visiting a primary doctor, and having a lower propensity to engage in prescription drug misuse (Mesanovic et al., 2013; Lucas et al., 2017).
Despite numerous evidence highlighting EI and health consciousness independently as important markers of behaviors that promote health and well-being, to our knowledge no research has considered the interrelation between these psychological concepts in predicting health behaviors. We posit that EI relates to health behaviors indirectly through increases in health consciousness for two primary reasons. First, high EI individuals are motivated to adjust behavior for the sake of improving their overall well-being and achieve success (Goleman, 1998). Accordingly, high-EI individuals develop high levels of self-awareness and appraisal, including awareness about behaviors that promote health (Wong and Law, 2002). Second, high EI individuals possess the capacity for emotion regulation (Dawda and Hart, 2000; Peña-Sarrionandia et al., 2015), and accordingly develop a heightened awareness of effective and healthy strategies to manage daily life stressors (Barrett et al., 2001; Peña-Sarrionandia et al., 2015). In sum, EI is a potential precursor of health consciousness, which in turn relates to actions taken to improve one’s health, thus mediating the relation between EI and health behaviors.
Current Study
In this article, we test the mediating role of health consciousness in the EI to health behaviors relation. Specifically, we hypothesized that EI and health consciousness would negatively relate to unhealthy behaviors. Moreover, we hypothesized that the relation between EI and health behaviors would be indirect through changes in health consciousness.
Materials and Methods
Procedure and Participants
Data were obtained from a large and culturally diverse public university in the Northeastern United States. Participants were prescreened for English proficiency, age, and any diagnosed physical or mental illness. A total of 314 healthy adults (18 years or older) completed self-report questionnaires in a research laboratory setting. The average participant was 21.94 (SD = 6.53) years old. Participants were mostly female (62.4%), and racial ethnic minority (85.0%), including Black (15.3%), Hispanic (33.4%), Asian (28.3%), and multi-racial (8.0%). The majority of participants (61.8%) reported household incomes of $40,000 USD or lower, which falls below the median household income of the city (United States Census Bureau, 2016). The vast majority reported their physical health between excellent and fair at time of participation (97.5%), with only eight participants reporting poor physical health. The majority of the sample had a primary doctor they visited at least every 6 months (79.0%), as well as a dentist (69.1%). The Institutional Review Board of the university approved this study, and all participants provided written consent.
Measures
Emotional Intelligence
Participants answered the Wong & Law Emotional Intelligence Scale (WLEIS; Wong and Law, 2002), which is a 16-item self-report measure of EI that is based on the four-branch ability model (Salovey and Mayer, 1990; Mayer and Salovey, 1997), and which has been identified as a theoretically supported measure of EI that correlates with personality traits (Brannick et al., 2009). Specifically, the measure gauges four dimensions of emotional intelligence including, emotional appraisal and expression of one’s emotions (SEA), emotional appraisal and recognition of other’s emotions (OEA), self-regulation of emotions (ROE) and use of emotions to enable performance (UOE). Combined, these dimensions provide a global measure of EI. Sample items include “I have a good sense of why I have certain feelings most of the time” and “I am a self-motivated person.” Items are presented using a 7-point Likert Scale (1 = Strongly Disagree … 7 = Strongly Agree). The psychometric properties of the WLEIS scale have been well-documented in multiple cultures and ethnic groups within and outside the US (Law et al., 2004; Ng et al., 2008; Li et al., 2012; Carvalho et al., 2016).
Health Consciousness
Respondents also answered questions from the Health Consciousness Scale (HCS; Gould, 1988), which is a 9-item self-report global measure of one’s health awareness. Sample items include “I reflect about my health a lot” and “I am alert to changes in my health.” Items are presented on a 7-point Likert scale (1 = Strongly Disagree … 7 = Strongly Agree). The scale has been validated in studies using international as well as US-based samples (Gould, 1990; Bearden et al., 2011; Mesanovic et al., 2013).
Health Behaviors
We assessed health behaviors via the Health Behavior Schedule II (HBS-II; Heiby et al., 2005; Frank et al., 2007), a self-report measure of acquiescence to conventional health practices that has been documented to predict compliance (Frank et al., 2007). While the original scale contains 12 items, only 9 items were used in this study, as these were most relevant for our population. Individuals were asked to state the degree to which they have succeeded (1 = Not at successful… 7 = Very successful) in eating a healthy diet, regularly exercising, flossing teeth daily, protecting skin from sun daily, refraining from smoking and drinking alcohol, taking medications as prescribed, performing a monthly breast exam, and screening for cervical/prostate cancer every 3 years. The reliability of the scale has been assessed in the literature (Frank et al., 2007).
Covariates
Respondents provided additional information including their age in years, sex (Male or Female), and family income (1 = $0 – $20,000 … 5 = $80,000 and above).
Analytical Strategy
Confirmatory Factor Analysis (CFA) with maximum likelihood estimation using the sample covariance matrix as input assessed the psychometric properties of the EI and health consciousness scales. Measures of absolute and relative fit, including the χ2 statistic, root mean square error of approximation (RMSEA), the comparative fit index (CFI), the Tucker Lewis Index (TLI), and the standardized root mean squared residual (SRMR) determined goodness of fit. In particular, a good fitting model is determined by fit measures that adhere to the following benchmarks: p-value of χ2 statistic > 0.05, RMSEA ≤ 0.06, CFI ≥ 0.95, TLI ≥ 0.95, and SRMR ≤ 0.09 (Hu and Bentler, 1999). Convergent validity of each construct was determined by comparing each construct reliability (CR) estimate against a minimum benchmark value of 0.70 and by verifying that each average variance extracted (AVE) was greater than 0.50 (Fornell and Larcker, 1981; Bagozzi and Yi, 1988). In addition, for each construct, discriminant validity was determined by comparing the AVE to the constructs’ squared correlation (Fornell and Larcker, 1981).
According to the literature, health behaviors are more likely to appear in groups or clusters than they are to appear independently (Vickers et al., 1990; Daw et al., 2017). However, the different types of health behavior groupings characterizing our population are unknown. Therefore, we used latent class analysis (LCA) to determine the patterns of health behaviors evident in our sample. Latent class analysis is a finite mixture model that probabilistically sorts respondents into several mutually exclusive groups with similar response patterns (McCutcheon, 1987; Hagenaars and McCutcheon, 2002). Thus in this setting, LCA generated a categorical outcome variable representing different types of health behavior groups. Because each health behavior question had seven possible responses (i.e., 1 = Not at successful… 7 = Very successful), we employed polytomous LCA (Linzer and Lewis, 2011). The number of groups that define the best fitting LCA model corresponds to those that minimize the Bayesian Information Criterion (BIC; Nylund et al., 2007; Tein et al., 2013) and the Consistent Akaike Information Criterion (CAIC; Akaike, 1973; McCutcheon, 1987). In addition, a good fitting model will yield an entropy index close to 1, representing clear demarcation of the classes (Ramaswamy et al., 1993).
Mediation analyses using structural equation modeling assessed the relation between emotional intelligence and health behaviors through health consciousness. Goodness of fit measures as mentioned above for CFA were adhered to. The 95% confidence interval for the indirect effect via health consciousness was obtained using 5,000 bootstrapped replications. Mediation is confirmed if such confidence interval does not contain zero (Shrout and Bolger, 2002; Hayes, 2013). All models included age, sex, and family income as covariates. We report partially and completely standardized indirect effects as measures of effect size in mediation models (Preacher and Kelley, 2011).
Pearson correlations and independent samples t-tests assessed the associations between the variables. Assumptions of normality for continuous variables and residuals in the regression models were confirmed, as all skewness and kurtosis coefficients were within the limits proposed in the literature (West et al., 1995). In addition, the homoscedasticity assumption of residuals was confirmed via non-significant White tests, which also gage nonlinear forms of heteroscedasticity (White, 1980). Multicollinearity was also ruled out as a potential confound, as the variance inflation factors were lower than 1.5. Missing cases were less than 0.01% and thus not imputed. Finally, Common Method Variance was unlikely a serious confound (Podsakoff et al., 2012), as the single factor CFA model yielded a poor fit (χ2 (434) = 2386.42, p < 0.001; RMSEA = 0.12, p < 0.001, 90% CI (0.11, 0.12); CFI = 0.49; TLI = 0.46; SRMR = 0.11). The polytomous LCA analyses were conducted via the poLCA package in R (Linzer and Lewis, 2011). All other analyses were conducted using STATA v. 15. (StataCorp, 2015).
Results
Confirmatory Factor Analysis
Confirmatory Factor Analyses (CFA) verified the psychometric properties of the EI and health consciousness scales. The CFA results appear in Table 1.
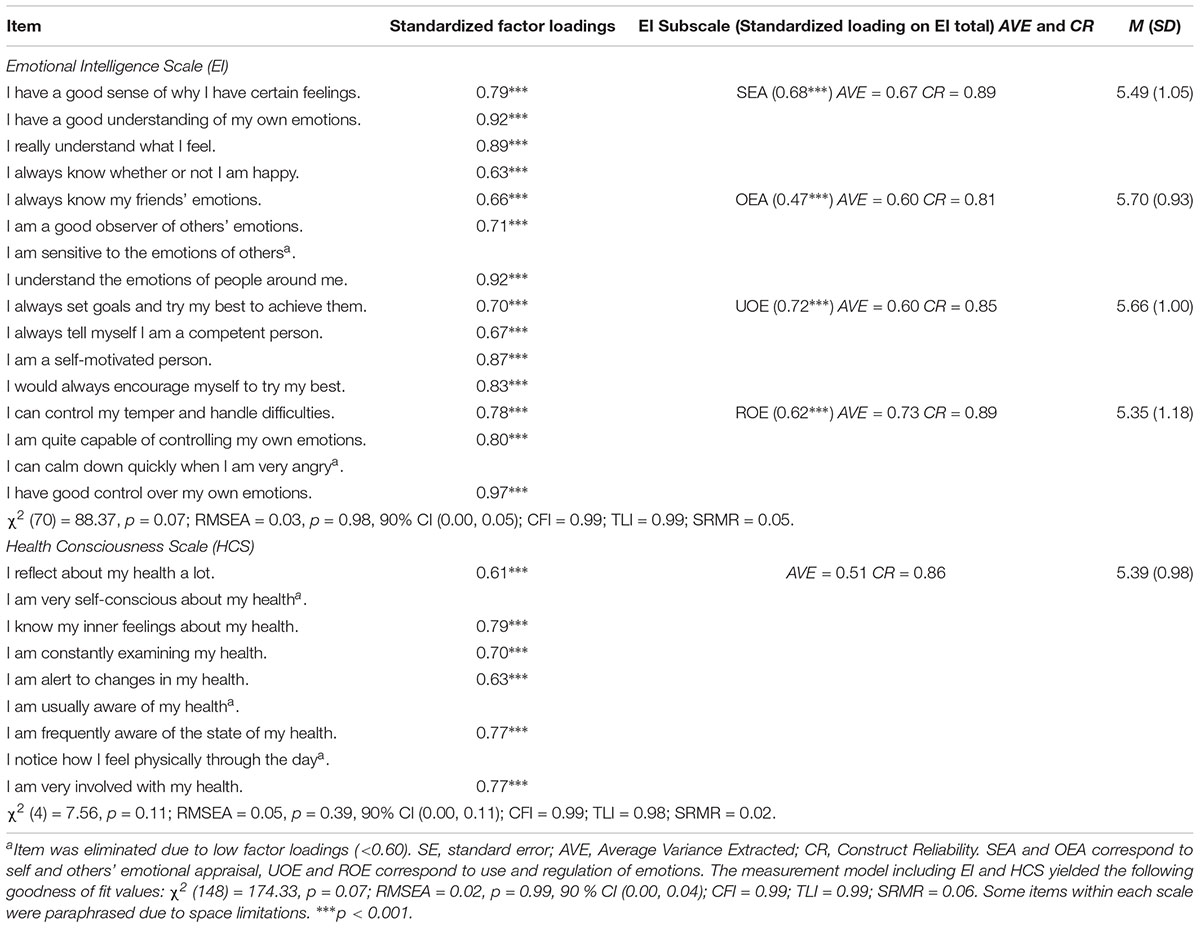
TABLE 1. Unstandardized and standardized loadings for confirmatory factor analysis of emotional intelligence and health consciousness scales.
As shown, the measurement model yielded adequate absolute and relative fit statistics. In both constructs the factor loadings per item were significant (p < 0.001), but a few items were excluded as their factor loadings were below the benchmark of 0.60 suggested in the literature (Comrey and Lee, 1992). Also, as shown the values for AVE and CR were larger than the recommended benchmarks of 0.50 and 0.70, respectively. In addition, as shown on the third column (Table 1), all subscales significantly loaded onto a higher order factor representing global or total EI. Table 2 presents the correlations, shared variance and Cronbach’s alpha estimates for each construct.
All constructs were positively correlated with each other, and the correlations between all EI subscales as well as the total EI measure and health consciousness were positive and moderate to strong. In addition, the estimates for internal consistency were above 0.80 for all constructs and in each case, the AVEs were larger than the shared variance for each pair of constructs, thus confirming discriminant validity.
Latent Class Analysis on Health Behaviors
To determine the number of health behavior groups that characterize the population represented by the sample, we consecutively ran the LCA model increasing the number of groups at each iteration and retaining the aforementioned information criteria (i.e., BIC and CAIC). Figure 1 presents these information criteria plotted against the respective number of LCA groups.
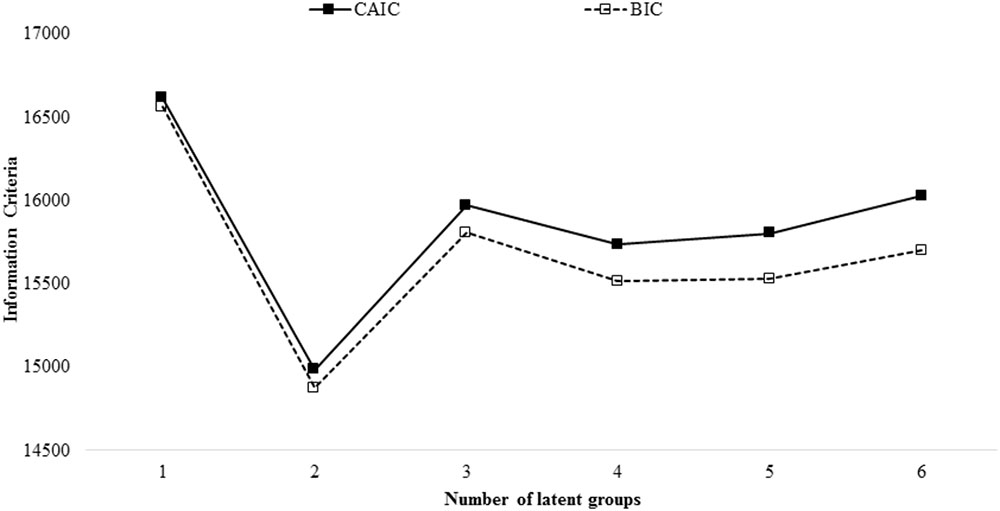
FIGURE 1. Information criteria from latent class analysis of health behaviors plotted against number of classes. BIC, Bayesian Information Criterion; CAIC, Consistent Information Criterion. The best-fitting model yields a minimum BIC and CAIC. Entropy for the two-group solution was 0.92.
As shown, the two-group model minimized the information criteria and thus yielded the best-fit overall. In addition, the entropy for the two-group model was 0.92, representing clear class delineation.
Table 3 presents the probability of response for each item, conditional on group membership, as well as group comparisons in terms of sample characteristics and relevant psychometric variables.
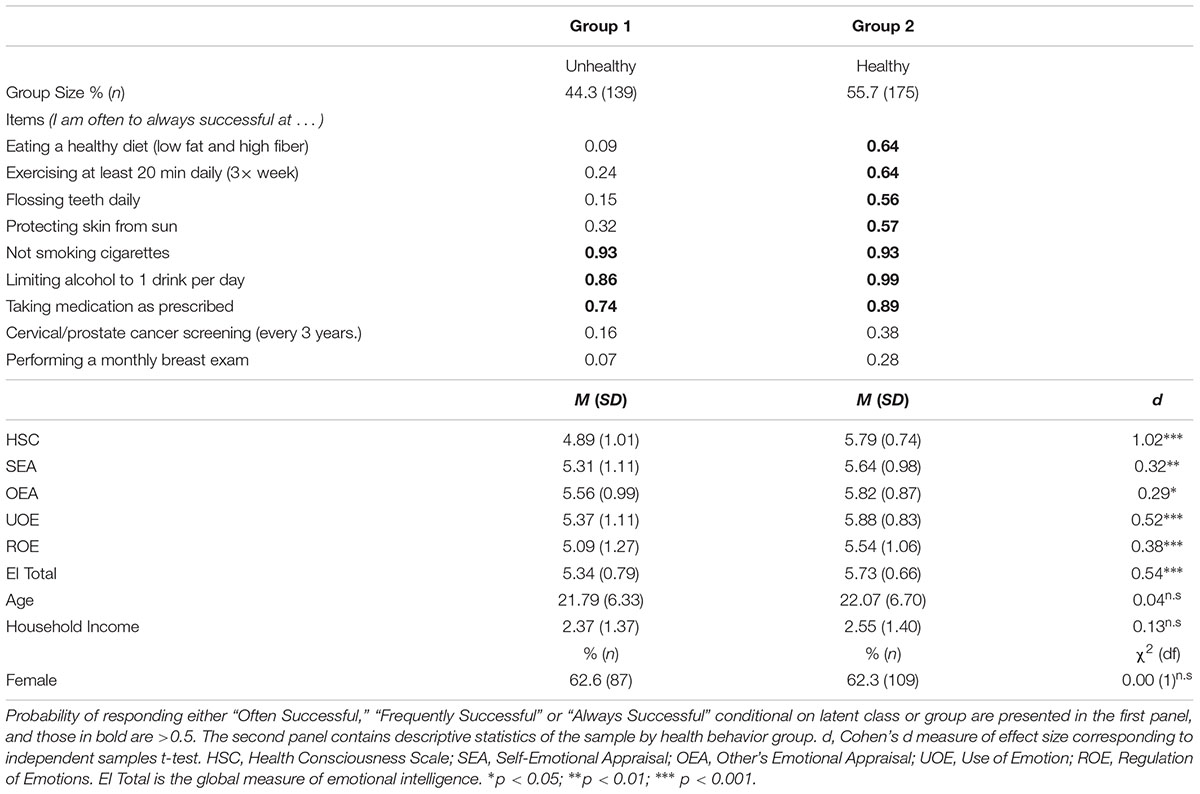
TABLE 3. Item response probabilities of health behaviors and descriptives by latent group (N = 314).
As indicated, the first group (44.3%, n = 139) was characterized by individuals who reported not being often successful in engaging in health-promoting behaviors such as eating healthy, exercising regularly, flossing daily, and protecting their skin from the sun. The second group (55.7%, n = 175) was composed of individuals who were at least often successful in engaging in these health-promoting behaviors. Both groups were highly successful in taking medications as prescribed, limiting alcohol consumption and avoiding smoking cigarettes. Additionally, the two groups were equally unlikely to engage in regular cancer screening. Accordingly, we labeled the first group as Unhealthy and the second group as Healthy. In terms of sex, age and family income, the two groups were not statistically different from each other as presented at the bottom of Table 3. Yet, the Healthy group had higher health consciousness and EI scores than the Unhealthy group. These differences were from moderate to large as indicated by their respective Cohen’s d measure of effect size.
Mediation Analyses
Mediation analyses assessed the indirect effect of health consciousness on the relation between EI and the probability of belonging to the Unhealthy group, relative to the Healthy group. Specifically, we conducted two mediation models. The first model used the global measure of EI, and the second model used the four EI subscales (i.e., SEA, OEA, UOE, and ROE) as antecedents. Every model included sex, age, and family income as covariates, although none of them significantly related to the outcomes. The results are presented in Table 4, and depicted in Figures 2, 3.
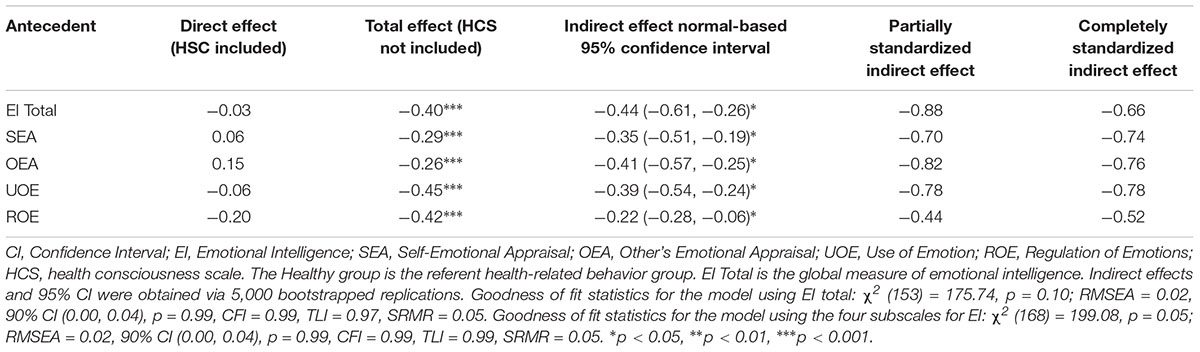
TABLE 4. Direct, total, indirect effects and effect sizes of the relation between emotional intelligence and health-related behaviors mediated by health consciousness.
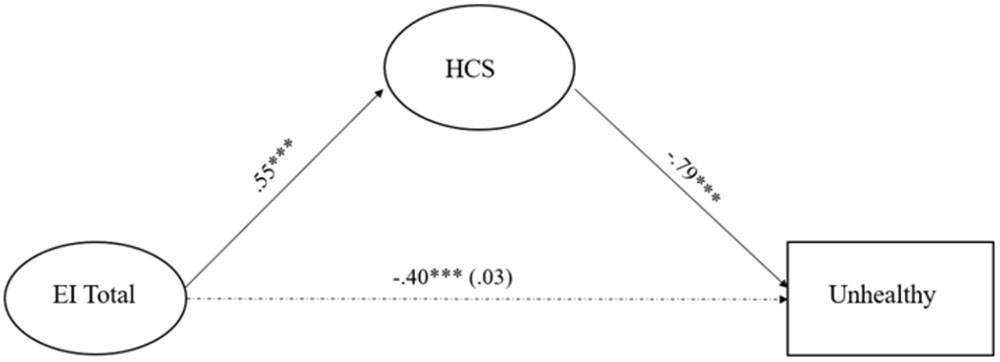
FIGURE 2. Path diagram of the relation between El global and health behaviors mediated by HCS. El Total, global measure of emotional intelligence; HCS, health consciousness scale. The model’s fit was χ2 (153) = 175.74, p = 0.10; RMSEA = 0.02, [90% CI (0.00, 0.04)], p = 0.99, CFI = 0.99, TLI = 0.97, SRMR = 0.05. The dotted line corresponds to total and direct effect of emotional intelligence. The total effect (HCS not included) is outside parenthesis, and the direct effect, computed when HCS was included in the model, is in parenthesis. ∗∗∗p < 0.001.
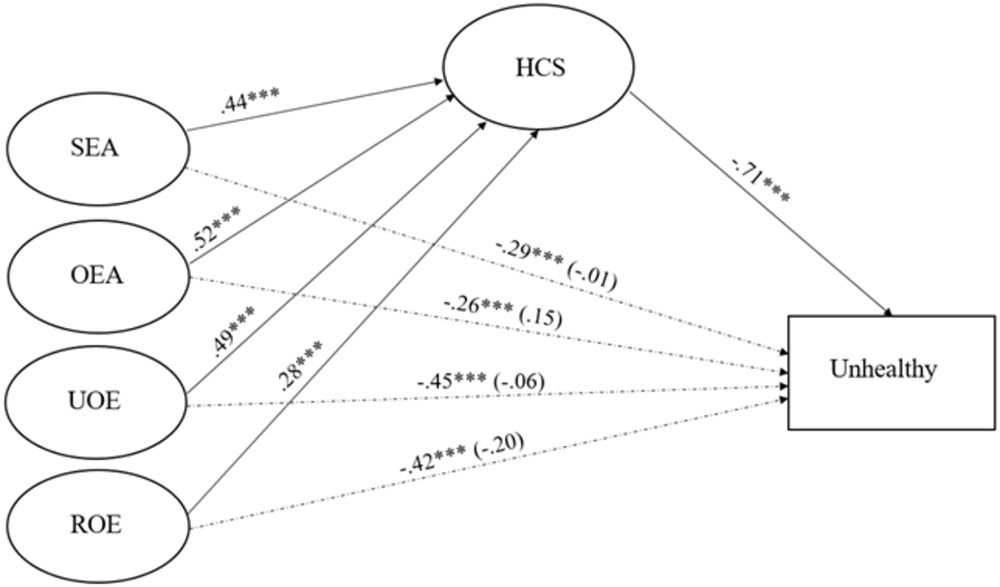
FIGURE 3. Paths for the relation between SEA, OEA; UOE and ROE and health behaviors mediated by HCS. SEA, Self-Emotional Appraisal; OEA, Other’s Emotional Appraisal; UOE, Use of Emotion; ROE, Regulation of Emotions; HCS, health consciousness scale. Model’s fit was χ2 (168) = 199.08, p = 0.05, RMSEA = 0.02, 90% CI (0.00, 0.04), p = 0.99), CFI = 0.99, TLI = 0.99, SRMR = 0.05. The dotted lines correspond to total and direct effects of the four dimensions of emotional intelligence. Direct effects, computed when HCS was in the model, are in parenthesis. ∗∗∗p < 0.001.
In every instance EI was significantly related to decreases in the likelihood of belonging to the Unhealthy group relative to the Healthy group, and related to increments in health consciousness. Health consciousness was in turn related to decreases in the likelihood of belonging to the Unhealthy group relative to the Healthy group. Upon adding health consciousness to the model, the effect of EI decreased in magnitude, and was no longer significant. As indicated in the 4th column of Table 4, the indirect effect of health consciousness was significant, and according to both measures of effect size, the effect was large. Both models yielded sound goodness of fit statistics as indicated at the bottom of Table 4 and Figures 2, 3.
Discussion
This study assessed health-related behaviors within a large sample of young adults, and gaged the roles of emotional intelligence and health consciousness in predicting such. Latent class analyses identified two health-behavior groups that resemble the two broad dimensions of preventive and risk-taking behaviors observed elsewhere (Vickers et al., 1990) and thus highlight the external validity of the findings. Namely, our young adult sample was divided into two distinct health-behavior groupings: An unhealthy group (not successful at eating healthy, exercising regularly, flossing daily, and protecting their skin from the sun) and a healthy group (successful at eating healthy, exercising regularly, flossing daily, and protecting their skin from the sun). While the two groups were similar in terms of socio-demographic characteristics, the healthy group endorsed on average higher levels of health consciousness than the unhealthy group; a result that is in agreement with the literature, and our first hypothesis. More specifically, studies have shown that the degree to which individuals are concerned about their health is a strong indicator of the extent to which they will engage in health promoting behaviors, such as fitness, nutrition, and others (Kraft and Goodell, 1993; Forthofer and Bryant, 2000). Accordingly, and as observed herein, individuals who engage in health-responsible or promoting behaviors are expected to have higher levels of health consciousness than individuals who do not engage in such health-promoting behaviors.
Also, as hypothesized the healthy group had higher levels of emotional intelligence than the unhealthy group. These findings are consistent with prior evidence indicating that individuals with high emotional competencies have the propensity to engage in healthy behaviors (Johnson et al., 2009; Zeidner et al., 2012; Mikolajczak et al., 2015). Given the multitude of studies indicating that health behaviors are strongly connected to long-term physical health (e.g., Colditz et al., 2000; Chiuve et al., 2012), our findings provide additional insights building on results from meta-analytic studies documenting emotional intelligence as predictor of improved physical health (Schutte et al., 2007; Martins et al., 2010), and further highlight the importance of understanding the mechanisms that explain the emotional intelligence to health behaviors relation. Specifically, in this article we confirmed health consciousness as a conduit explaining such relation, as structural equation models yielded significant indirect paths by way of health consciousness in the relations between emotional intelligence and health behaviors, holding constant age, sex, and income. These findings expand our knowledge on the well-documented positive effect of emotional intelligence on health (Schutte et al., 2007; Martins et al., 2010) by suggesting that emotional intelligence assists in the improvement of one’s health consciousness, and consequently participation in behaviors that predict long-term physical health.
Importantly, all dimensions of emotional intelligence including appraisal (self and others’), use and regulation of emotions, were relevant for reductions in the likelihood of engaging in unhealthy behaviors compared to healthy ones by way of improvements in health consciousness. These findings add to the literature seeking to identify the unique contribution of different dimensions of emotional intelligence on health-related outcomes (Fernández-Abascal and Martín-Díaz, 2015). Specifically, our findings highlight the additive value of each dimension of emotional intelligence, as operationalized by Wong and Law (2002) toward understanding the mechanism that explains the connection between emotional capabilities and health promoting behaviors among young adults. These findings suggest that emotional intelligence, in all its dimensions, is a precursor to increases in one’s health awareness, resulting in acquiescence to healthy behaviors. In combination, our findings suggest that educational programs aiming to improve health behaviors among young adults should include emotional intelligence training in their curriculum, as such training may help develop intrinsic health awareness and the willingness to promote health. Yet, there is a need for additional research to investigate these claims more rigorously.
Particularly, a key feature of emotional intelligence is the capacity for emotion regulation (Mayer and Salovey, 1995; Peña-Sarrionandia et al., 2015), which concerns the process by which individuals regulate their emotions (Peña-Sarrionandia et al., 2015) and is an important predictor of engaging in effective behaviors for managing stress (Compas et al., 2014; Kober, 2014). Along these lines our findings, while in agreement with studies indicating a negative association between emotional intelligence and health-impairing coping behaviors (Mikolajczak et al., 2009; Espinosa et al., 2018), raise awareness about the need to investigate emotion regulation strategies as additional mechanisms explaining the connection between emotional intelligence and health behaviors, particularly as they relate to health consciousness. In the same vein, our study raises awareness about the need to identify the extent to which other salient predictors of health behaviors relate to emotional intelligence and health consciousness. In particular, researchers have highlighted genetic factors as important predictors of smoking, healthy eating, physical fitness and other behaviors (Bulik, 2004; Sutton, 2005; Chomistek et al., 2013). The interrelation between genetic predispositions, emotional intelligence and health consciousness in predicting health behaviors is unknown, yet necessary for developing effective and comprehensive approaches to improve health-related behaviors among young adults.
This study is not without limitations. First, due to our use of self-report measures we cannot rule out social desirability bias influencing the findings. Second, the study employed a cross-sectional design, which prevents us from making causal interpretations. Future studies should consider a longitudinal design that would allow for robust causal interpretations, and include measures of social desirability as covariates. Along these lines, while the measure of emotional intelligence used in this study has been identified in the literature as a reliable construct of emotional self-efficacy (Pérez et al., 2005) whose first-order factors are distinct, but correlated dimensions defining the focal construct of emotional intelligence (Walter et al., 2011), future research should also include more objective measures of emotional intelligence to address any concerns related to the use of self-report measures. Third, both health groups exhibited equally poor cancer-screening behaviors, which prevents us from generalizing the findings to these specific disease prevention approaches. On a similar vein, the two groups were highly unlikely to use substances such as nicotine, and alcohol, which is atypical of college students (Center for Behavioral Health Statistics and Quality, 2015; Skidmore et al., 2016), and thus may highlight a unique aspect of our sample that limits generalization to other young adult samples. Future studies should cast a wider net to recruit participants by perhaps selecting multiple sampling units. Finally, our study did not include an exhaustive list of health behaviors, thus limiting the findings to the health behaviors gauged herein. Future studies should include additional types of behaviors such as risky sexual behaviors and illicit drug use.
Despite these limitations, our study provides new evidence highlighting the mediating role of health consciousness in the relation between emotional intelligence and health behaviors. Given the predictive role of health behaviors during early adulthood on health outcomes in old age, the results are relevant for educators and policymakers, as they present a mechanism linking young adults’ personal characteristics to behaviors that reduce the risk of chronic illness later in life.
Conclusion
This study determined the types of health behaviors that characterize our young adult population as well as the relations between such health behaviors, health consciousness and emotional intelligence. Furthermore, this study tested health consciousness as mediator of the relation between emotional intelligence and health behaviors. The results indicate that health behaviors among young individuals can be viewed as a dichotomy of healthy and unhealthy behaviors. Individuals whose behaviors qualify as healthy tend to have higher levels of emotional intelligence than those whose behaviors are characterized as unhealthy. In our findings, health consciousness exerted an intervening pathway that explained the relation between emotional intelligence and health behaviors. Namely, individuals with low emotional intelligence also had low health consciousness, and thus a higher propensity to engage in unhealthy behaviors relative to individuals with high emotional intelligence. Accordingly, programs seeking to improve health behaviors among young adults should consider the inclusion of emotional intelligence training within the curriculum, as improvements in emotional competencies are likely to influence the development of health consciousness and accordingly improve behaviors that promote health and well-being.
Ethics Statement
This study was carried out in accordance with the recommendations of the Institutional Review Board of the City College of New York with written informed consent from all participants. All participants gave written informed consent in accordance with the Declaration of Helsinki. The protocol was approved by the Institutional Review Board of the City College of New York.
Data Availability Statement
The raw and de-identified data supporting the conclusions of the manuscript will be made available by the authors, without undue reservation, to any qualified researcher.
Author Contributions
Both authors, AE and SK-M, contributed to the project’s design, administration, survey creation, interpretation of findings, writing, and revising of the manuscript. AE contributed to the collection and analysis of data. Both authors approved the final version of this manuscript.
Funding
This research was supported in part by a Professional Staff Congress – City University of New York (PSC-CUNY) Grant Number 68018-00-46, and by fellowship support from the Public Administration program at the City College of New York, both awarded to AE.
Conflict of Interest Statement
The authors declare that the research was conducted in the absence of any commercial or financial relationships that could be construed as a potential conflict of interest.
Acknowledgments
The authors would like to acknowledge the staff of the Spielman Research Lab, as well as volunteers of the ARHAMP Research Lab for their assistance with the collection of data. The authors would also like to thank the students who participated in this study, for without them, this study would not have been possible.
References
Adolph, M., Darnaud, C., Thomas, F., Pannier, B., Danchin, N., Batty, G. D., et al. (2017). Oral health in relation to all-cause mortality: the IPC cohort study. Sci. Rep. 7:44604. doi: 10.1038/srep44604
Ajwani, S., Mattila, K., Narhi, T. O., Tilvis, R. S., and Ainamo, A. (2003). Oral health status, C-reactive protein and mortality – a 10 year follow-up study. Gerodontology 20, 32–40. doi: 10.1111/j.1741-2358.2003.00032.x
Akaike, H. (1973). “Information theory and an extension of the maximum likelihood principle,” in Proceedings of the Second International Symposium on Information Theory, eds B. N. Petrov and F. Csàki (Budapest: Akadémiai Kiadó), 267–281.
Anderson, G., and Horvath, J. (2004). The growing burden of chronic disease in America. Public Health Rep. 119, 263–270. doi: 10.1016/j.phr.2004.04.005
Bagozzi, R. P., and Yi, Y. (1988). On the evaluation of structural equation models. J. Acad. Mark. Sci. 16, 74–94. doi: 10.1007/BF02723327
Barrett, L. F., Gross, J., Conner, T., and Benvenuto, M. (2001). Knowing what you’re feeling and knowing what to do about it: mapping the relation between emotion differentiation and emotion regulation. Cogn. Emot. 15, 713–724. doi: 10.1080/02699930143000239
Basu, A., and Dutta, M. J. (2008). The relationship between health information seeking and community participation: the roles of health information orientation and efficacy. Health Commun. 23, 70–79. doi: 10.1080/10410230701807121
Bearden, W. O., Netemeyer, R. G., and Haws, K. L. (2011). Handbook of Marketing Scales: Multi-Item Measures for Marketing and Consumer Behavior Research. Los Angeles, CA: Sage.
Bogers, R. P., Bemelmans, W. J., Hoogenveeten, R. T., Boshuizen, H. C., Woodward, M., Knekt, P., et al. (2007). Association of overweight with increased risk of coronary heart disease partly independent of blood pressure and cholesterol levels: a meta-analysis of 21 cohort studies including more than 300,000 persons. Arch. Intern. Med. 167, 1720–1728. doi: 10.1001/archinte.167.16.1720
Brackett, M. A., Mayer, J. D., and Warner, R. M. (2004). Emotional intelligence and its relation to everyday behaviour. Personal. Individ. Differ. 36, 1387–1402. doi: 10.1016/S0191-8869(03)00236-8
Brannick, M. T., Wahi, M. M., Arce, M., Johnson, H. A., Nazian, S., and Goldin, S. B. (2009). Comparison of trait and ability measures of emotional intelligence in medical students. Med. Educ. 43, 1062–1068. doi: 10.1111/j.1365-2923.2009.03430.x
Bulik, C. M. (2004). “Genetic and biological risk factors,” in Handbook of eating disorders and obesity, ed. J. K. Thompson (Hoboken, NJ: Wiley & Sons, Inc).
Carvalho, V. S., Guerrero, E., Chambel, M. J., and González-Rico, P. (2016). Psychometric properties of WLEIS as a measure of emotional intelligence in the Portuguese and Spanish medical students. Eval. Program Plann. 58, 152–159. doi: 10.1016/j.evalprogplan.2016.06.006
Center for Behavioral Health Statistics and Quality (2015). Results from the 2014 National Survey on Drug Use and Health: Detailed tables. Rockville, MD: Substance Abuse and Mental Health Services Administration.
Chen, M.-F. (2009). Attitude toward organic foods among Taiwanese as related to health consciousness, environmental attitudes, and the mediating effects of a healthy lifestyle. Br. Food J. 111, 165–178. doi: 10.1108/00070700910931986
Chiuve, S. E., Fung, T. T., Rimm, E. B., Hu, F. B., McCullough, M. L., Wang, M., et al. (2012). Alternative dietary indices both strongly predict risk of chronic disease. J. Nutr. 142, 1009–1018. doi: 10.3945/jn.111.157222
Chomistek, A. K., Chasman, D. I., Cook, N. R., Rimm, E. B., and Lee, I.-M. (2013). Physical activity, genes for physical fitness, and risk of coronary heart disease. Med. Sci. Sports Exerc. 45, 691–697. doi: 10.1249/MSS.0b013e3182784e9f
Colditz, G. A., Atwood, K. A., Emmons, K., Monson, R. R., Willett, W. C., Trichopoulous, D., et al. (2000). Harvard report on cancer prevention volume 4: harvard cancer risk index. Cancer Causes Control 11, 477–488. doi: 10.1023/A:1008984432272
Compas, B. E., Jaser, S. S., Dunbar, J. P., Watson, K. H., Bettis, A. H., Gruhn, M. A., et al. (2014). Coping and emotion regulation from childhood to early adulthood: Points of convergence and divergence. Aust. J. Psychol. 66, 71–81. doi: 10.1111/ajpy.12043
Daw, J., Margolis, R., and Wright, L. (2017). Emerging adulthood, emerging health lifestyles: sociodemographic determinants of trajectories of smoking, binge drinking, obesity and sedentary behavior. J. Health Soc. Behav. 58, 181–197. doi: 10.1177/0022146517702421
Dawda, D., and Hart, S. D. (2000). Assessing emotional intelligence: reliability and validity of the Bar-On emotional quotient-inventory (EQ-i) in university students. Personal. Individ. Differ. 28, 797–812. doi: 10.1016/S0191-8869(99)00139-7
Dutta, M., and Feng, H. (2007). Health orientation and disease state as predictors of online health support group use. Health Commun. 22, 181–189. doi: 10.1080/10410230701310323
Espinosa, A., Akinsulure-Smith, A. M., and Chu, T. (2018). Trait emotional intelligence, coping, and occupational distress among resettlement workers. Psychol. Trauma Res. Pract. Policy doi: 10.1037/tra0000377 [Epub ahead of print].
Fernández-Abascal, E. G., and Martín-Díaz, M. D. (2015). Dimensions of emotional intelligence related to physical and mental health and to health behaviors. Front. Psychol. 6:317. doi: 10.3389/fpsyg.2015.00317
Fornell, C., and Larcker, D. F. (1981). Evaluating structural equation models with unobservable variables and measurement error. J. Mark. Res. 18, 39–50. doi: 10.2307/3151312
Forthofer, M. S., and Bryant, C. A. (2000). Using audience-segmentation techniques to tailor health behavior change strategies. Am. J. Health Behav. 24, 36–43. doi: 10.5993/AJHB.24.1.6
Frank, M. T., Heiby, E. M., and Lee, J. H. (2007). Assessment of determinants of compliance to twelve health behaviors: psychometric evaluation of the Health Behavior Schedule – II. Psychol. Rep. 100, 1281–1297. doi: 10.2466/pr0.100.4.1281-1297
Frech, A. (2012). Healthy behavior trajectories between adolescence and young adulthood. Adv. Life Course Res. 17, 59–68. doi: 10.1016/j.alcr.2012.01.003
Gould, S. J. (1988). Consumer attitudes toward health and health care: a differential perspective. J. Consum. Aff. 22, 96–118. doi: 10.1111/j.1745-6606.1988.tb00215.x
Gould, S. J. (1990). Health consciousness and health behavior: The application of a new health consciousness scale. Am. J. Prev. Med. 6, 228–237. doi: 10.1016/S0749-3797(18)31009-2
Hagenaars, J. A., and McCutcheon. (2002). A. Applied Latent Class Analysis. Cambridge: Cambridge University Press.
Hayes, A. F. (2013). Introduction to Mediation, Moderation, and Conditional Process Analysis: A Regression-Based Approach. New York, NY: Guilford Press.
Heiby, E. M., Lukens, C. L., and Frank, M. R. (2005). The health compliance model-II. Behav. Anal. Today 6, 24–42. doi: 10.1037/h0100050
Hoek, A. C., Luning, P. A., Stafleu, A., and de Graaf, C. (2004). Food-related lifestyle and health attitudes of Dutch vegetarians, non-vegetarian consumers of meat substitutes, and meat consumers. Appetite 42, 265–272. doi: 10.1016/j.appet.2003.12.003
Hollis, J. F., Carmody, T. P., Connor, S. L., Fey, S. G., and Matarazzo, J. D. (1986). The nutrition attitude survey: associations with dietary habits, psychological and physical well-being, and coronary risk factors. Health Psychol. 5, 359–374. doi: 10.1037/0278-6133.5.4.359
Hu, L., and Bentler, P. M. (1999). Cutoff criteria for fit indexes in covariance structure analysis: conventional criteria versus new alternatives. Struct. Equ. Modeling 6, 1–55. doi: 10.1080/10705519909540118
Iversen, A. C., and Kraft, P. (2006). Does socio-economic status and health consciousness influence how women respond to health related messages in the media? Health Educ. Res. 21, 601–610. doi: 10.1093/her/cyl014
Johnson, S. J., Batey, M., and Holdsworth, L. (2009). Personality and health: The mediating role of trait emotional intelligence and work locus of control. Personal. Individ. Differ. 47, 470–475. doi: 10.1016/j.paid.2009.04.025
Johnstone, M.-L., and Hooper, S. (2016). Social influence and green consumption behaviour: a need for greater government involvement. J. Mark. Manag. 32, 827–855. doi: 10.1080/0267257X.2016.1189955
Kober, H. (2014). “Emotion regulation and substance use disorders,” in Handbook of emotion regulation, ed. J. J. Gross (New York, NY: Guildford Press), 428–446.
Kraft, F. B., and Goodell, P. W. (1993). Identifying the health conscious consumer. J. Health Care Mark. 13, 18–25.
Law, K. S., Wong, C. S., and Song, L. J. (2004). The construct and criterion validity of emotional intelligence and its potential utility for management studies. J. Appl. Psychol. 89, 483–496. doi: 10.1037/0021-9010.89.3.483
Li, T., Saklofske, D. H., Bowden, S. C., Yan, G., and Fung, T. S. (2012). The measurement invariance of the Wong and Law emotional intelligence scale (WLEIS) across three Chinese university student groups from Canada and China. J. Psychoeduc. Assess. 30, 439–452. doi: 10.1177/0734282912449449
Linzer, D. A., and Lewis, J. (2011). poLCA: an R package for polytomous variable latent class analysis. J. Stat. Software 42, 1–29. doi: 10.18637/jss.v042.i10
Lucas, G., Neeper, M., Linde, B., and Bennett, J. (2017). Preventing prescription drug misuse in work settings: efficacy of a brief intervention in health consciousness. J. Med. Internet Res. 19:e242. doi: 10.2196/jmir.7828
Martins, A., Ramalho, N., and Morin, E. (2010). A comprehensive meta-analysis of the relationship between emotional intelligence and health. Personal. Individ. Differ. 49, 554–564. doi: 10.1016/j.paid.2010.05.029
Mayer, J. D., and Salovey, P. (1995). Emotional intelligence and the construction and regulation of feelings. Appl. Preventive Psychol. 4, 197–208. doi: 10.1016/S0962-1849(05)80058-7
Mayer, J. D., and Salovey, P. (1997). “What is emotional intelligence?,” in Emotional development and emotional intelligence: Educational implications, eds P. Salovey and D. J. Sluyter (New York, NY: Basic Books), 3–34.
Mesanovic, E., Kadic-Maglajlic, S., and Cicic, M. (2013). Insights into health consciousness in Bosnia and Herzegovina. Proc. Soc. Behav. Sci. 81, 570–575. doi: 10.1016/j.sbspro.2013.06.478
Michaelidou, N., and Hassan, L. M. (2008). The role of health consciousness, food safety concern and ethical identity on attitudes and intentions towards organic food. Int. J. Consum. Stud. 32, 163–170. doi: 10.1111/j.1470-6431.2007.00619.x
Mikolajczak, M., Avalosse, H., Vancorenland, S., Verniest, R., Callens, M., van Borack, N., et al. (2015). A nationally representative study of emotional competence and health. Emotion 15, 653–567. doi: 10.1037/emo0000034
Mikolajczak, M., Petrides, K. V., and Hurry, J. (2009). Adolescents choosing self-harm as an emotion regulation strategy: the protective role of trait emotional intelligence. Br. J. Clin. Psychol. 48, 181–193. doi: 10.1348/014466508X386027
Must, A., Spandano, J., Coakely, E. H., Field, A. E., Colditz, G., and Dietz, W. H. (1999). The disease burden associated with overweight and obesity. J. Am. Med. Assoc. 282, 1523–1529. doi: 10.1001/jama.282.16.1523
National Research Council and Institute of Medicine of the National Academies. (2009). Adolescent Health Services: Missing Opportunities. Washington DC: National Academies Press.
Nelis, D., Kotsou, I., Quoidbach, J., Hansenne, M., Weytens, F., Dupuis, P., et al. (2011). Increasing emotional competence improves psychological and physical well-being, social relationships, and employability. Emotion 11, 354–366. doi: 10.1037/a0021554
Ng, K.-M., Wang, C., Zalaquett, C. P., and Bodenhorn, N. (2008). A confirmatory factor analysis of the Wong and Law emotional intelligence scale in a sample of international college students. Int. J. Adv. Couns. 30, 131–144. doi: 10.1007/s10447-008-9051-3
Nylund, K., Asparouhov, T., and Muthén, B. O. (2007). Deciding on the number of classes in latent class analysis and growth mixture modeling: a monte carlo simulation study. Struct. Equ. Modeling 14, 535–569. doi: 10.1080/10705510701575396
Park, M. J., Scott, J. T., Adams, S. H., Brindis, C. D., and Irwin, C. E. (2014). Adolescent and young adult health in the United States in the past decade: little improvement and young adults remain worse off than adolescents. J. Adolescent Health 55, 3–16. doi: 10.1016/j.jadohealth.2014.04.003
Patel, A. V., Bernstein, L., Deka, A., Feigelson, H. S., Campbell, P. T., Gapstur, S. M., et al. (2010). Leisure time spent sitting in relation to total mortality in a prospective cohort of US adults. Am. J. Epidemiol. 172, 419–429. doi: 10.1093/aje/kwq155
Peña-Sarrionandia, A., Mikolajczak, M., and Gross, J. (2015). Integrating emotion regulation and emotional intelligence traditions: a meta-analysis. Front. Psychol. 6:160. doi: 10.3389/fpsyq.2015.00160
Pérez, J. C., Petrides, K. V., and Furnham, A. (2005). “Measuring trait emotional intelligence,” in Emotional Intelligence: An International Handbook, eds R. Schulze and R. D. Roberts (Ashland, OH: Hogrefe & Huber Publishers), 181–201.
Petrides, K. V. (2011). “Ability and trait emotional intelligence,” in The Wiley-Blackwell Handbook of Individual Differences, eds T. Chamorro-Premuzic, S. von Stumm, and A. Furnham (Malden, MA: Blackwell Publishing), 656–678. doi: 10.1002/9781444343120.ch25
Petrides, K. V., and Furnham, A. (2001). Trait emotional intelligence: Psychometric investigation with reference to established taxonomies. Eur. J. Personal. 15, 425–448. doi: 10.1002/per/416
Petrides, K. V., Mikolajczak, M., Mavroveli, S., Sanchez-Ruiz, M. J., Furnham, A., and Pérez, J. C. (2016). Developments in trait emotional intelligence research. Emot. Rev. 8, 1–7. doi: 10.1177/1754073916650493
Podsakoff, P. M., MacKenzie, S. B., and Podsakoff, N. P. (2012). Sources of method bias in social science research and recommendations on how to control it. Annu. Rev. Psychol. 63, 539–569. doi: 10.1146/annurev-psych-120710-100452
Preacher, K. J., and Kelley, K. (2011). Effect size measures for mediation models: quantitative strategies for communicating indirect effects. Psychol. Methods 16, 93–115. doi: 10.1037/a0022658
Ramaswamy, V., DeSarbo, W. S., Reibstein, D. J., and Robinson, W. T. (1993). An empirical pooling approach for estimating marketing mix elasticities with PIMS data. Mark. Sci. 12, 103–124. doi: 10.1287/mksc.12.1.103
Riley, H., and Schutte, N. S. (2003). Low emotional intelligence as predictor of substance-use problems. J. Drug Educ. 33, 391–398. doi: 10.2190/6DH9-YT0M-FT99-2X05
Rimm, E. B., Stampfer, M. J., Giovannucci, E., Ascherio, A., Spiegelman, D., Colditz, G. A., et al. (1995). Body size and fat distribution as predictors of coronary heart disease among middle-aged and older US men. Am. J. Epidemiol. 141, 1117–11127. doi: 10.1093/oxfordjournals.aje.a117385
Saklofske, D. H., Austin, E. J., Galloway, J., and Davidson, K. (2007a). Individual difference correlates of health related behaviours: preliminary evidence for links between emotional intelligence and coping. Personal. Individ. Diff. 42, 491–502. doi: 10.1016/j.paid.2006.08.006
Saklofske, D. H., Austin, E. J., Rohr, B. A., and Andrews, J. J. W. (2007b). Personality, emotional intelligence and exercise. J. Health Psychol. 12, 937–948. doi: 10.1177/1359105307082458
Salovey, P., and Mayer, J. D. (1990). Emotional intelligence. Imagin. Cogn. Personal. 9, 185–211. doi: 10.2190/DUGG-P24E-52WK-6CDG
Schutte, N. S., Malouff, J. M., Thorsteinsson, E. B., Bhullar, N., and Rooke, S. E. (2007). A meta-analytic investigation of the relationship between emotional intelligence and health. Personal. Individ. Diff. 42, 921–933. doi: 10.1016/j.paid.2006.09.003
Shrout, P. E., and Bolger, N. (2002). Mediation in experimental and nonexperimental studies: new procedures and recommendations. Psychol. Methods 7, 422–445. doi: 10.1037/1082-989X.7.4.422
Skidmore, C. R., Kaufman, E. A., and Crowell, S. E. (2016). Substance use among college students. Child Adolescent Psychiatric Clin. North Am. 25, 735–753. doi: 10.1016/j.chc.2016.06.004
Sutton, S. R. (2005). “Determinants of health-related behaviors: Theoretical and methodological issues,” in The Sage Handbook of Health Psychology, eds S. Sutton, A. Baum, and M. Johnston (London: Sage), 94–126.
Tein, J.-Y., Coxe, S., and Cham, H. C. (2013). Statistical power to detect the correct number of classes in latent profile analysis. Struct. Equat. Modeling 20, 640–657. doi: 10.1080/10705511.2013.824781
Trinidad, D. R., and Johnson, C. A. (2002). The association between emotional intelligence and early adolescent tobacco and alcohol use. Personal. Individ. Differ. 32, 95–105. doi: 10.1016/S0191-8869(01)00008-3
Trinidad, D. R., Unger, J. B., Chou, C. P., and Johnson, C. A. (2004). The protective association of emotional intelligence with psychosocial smoking risk factors for adolescents. Personal. Individ. Differ. 36, 945–954. doi: 10.1016/S0191-8869(03)00163-6
U.S. Department of Health and Human Services (2010). Healthy People 2010: Understanding and Improving Health. Washington, DC: Department of Health and Human Services.
United States Census Bureau (2016). 2012-2016 American Community Survey 5-Year Estimates. Median Household Income in the Past 12 Months (Table B19013). Available at: https://factfinder.census.gov/faces/tableservices/jsf/pages/productview.xhtml?src=bkmk
Vickers, R. R., Conway, T. L., and Hervig, L. K. (1990). Demonstration of replicable dimensions of health behaviors. Prev. Med. 19, 377–401. doi: 10.1016/0091-7435(90)90037-K
Walter, F., Cole, M. S., and Humphrey, R. H. (2011). Emotional Intelligence: Sine qua non of Leadership or Folderol?. Briarcliff Manor, N Y: Academy of Management, doi: 10.5465/amp.25.1.45
West, S. G., Finch, J. F., and Curran, P. I. (1995). “Structural equation models with non-normal variables,” in Structural Equation Modeling, ed. R. Hoyle (Thousand Oaks, CA: Sage), 56–75.
White, H. (1980). A heteroscedasticity-consistent covariance matrix estimator and a direct test for heteroscedasticity. Econometrica 48, 817–838. doi: 10.2307/1912934
Wong, C. S., and Law, K. S. (2002). The effects of leader and follower emotional intelligence on performance and attitude: an exploratory study. Leadersh. Q. 13, 243–274. doi: 10.1016/S1048-9843(02)00099-1
Yach, D., Hawkes, C., Gould, C. L., and Hofman, K. J. (2004). The global burden of chronic diseases: overcoming impediments to prevention and control. JAMA 291, 2616–2622. doi: 10.1001/jama.291.21.2616
Keywords: health consciousness, emotional intelligence, health behaviors, latent class analysis, mediation
Citation: Espinosa A and Kadić-Maglajlić S (2018) The Mediating Role of Health Consciousness in the Relation Between Emotional Intelligence and Health Behaviors. Front. Psychol. 9:2161. doi: 10.3389/fpsyg.2018.02161
Received: 06 July 2018; Accepted: 22 October 2018;
Published: 08 November 2018.
Edited by:
Stella Mavroveli, Imperial College London, United KingdomReviewed by:
Kyung Mook Choi, Korea University, South KoreaHsien-Yuan Lane, China Medical University, Taiwan
Copyright © 2018 Espinosa and Kadić-Maglajlić. This is an open-access article distributed under the terms of the Creative Commons Attribution License (CC BY). The use, distribution or reproduction in other forums is permitted, provided the original author(s) and the copyright owner(s) are credited and that the original publication in this journal is cited, in accordance with accepted academic practice. No use, distribution or reproduction is permitted which does not comply with these terms.
*Correspondence: Adriana Espinosa, aespinosa@ccny.cuny.edu