- 1Laboratório de Neuropsicologia do Desenvolvimento, Departamento de Psicologia, Universidade Federal de Minas Gerais, Belo Horizonte, Brazil
- 2Programa de Pós-Graduação em Neurociências, Universidade Federal de Minas Gerais, Belo Horizonte, Brazil
- 3Programa de Pós-Graduação em Genética, Universidade Federal de Minas Gerais, Belo Horizonte, Brazil
- 4Departamento de Biologia Geral, Universidade Federal de Minas Gerais, Belo Horizonte, Brazil
- 5Programa de Pós-graduação em Psicologia, Cognição e Comportamento, Universidade Federal de Minas Gerais, Belo Horizonte, Brazil
- 6Programa de Pós-graduação em Saúde da Criança e do Adolescente, Universidade Federal de Minas Gerais, Belo Horizonte, Brazil
- 7Instituto Nacional de Ciência e Tecnologia sobre Comportamento, Cognição e Ensino, São Carlos, Brazil
Although progress has been made, the cognitive, biological and, particularly, the genetic underpinnings of math learning difficulties (MD) remain largely unknown. This difficulty stems from the heterogeneity of MD and from the large contribution of environmental factors to its etiology. Understanding endophenotypes, e.g., the role of the Approximate Number System (ANS), may help understanding the nature of MD. MD associated with ANS impairments has been described in some genetic conditions, e.g., 22q11.2 deletion syndrome (22q11.2DS or Velocardiofacial syndrome, VCFS). Recently, a girl with MD was identified in a school population screening. She has a new syndrome resulting from a microdeletion in 22q11.2 (LCR22-4 to LCR22-5), a region adjacent to but not overlapping with region 22q11.2 (LCR22-2 to LCR22-4), typically deleted in VCFS. Here, we describe her cognitive-neuropsychological and numerical-cognitive profiles. The girl was assessed twice, at 8 and 11 years. Her numerical-cognitive performance at both times was compared to demographically similar girls with normal intelligence in a single-case, quasi-experimental study. Neuropsychological assessment was normal, except for relatively minor impairments in executive functions. She presented severe and persistent difficulties in the simplest single-digit calculations. Difficulties in commutative operations improved from the first to the second assessment. Difficulties in subtraction persisted and were severe. No difficulties were observed in Arabic number writing. Difficulties in single-digit calculation co-occurred with basic numerical processing impairments in symbolic and non-symbolic (single-digit comparison, dot sets size comparison and estimation) tasks. Her difficulties suggest ANS impairment. No difficulties were detected in visuospatial/visuoconstructional and in phonological processing tasks. The main contributions of the present study are: (a) this is the first characterization of the neuropsychological phenotype in 22q11.2DS (LCR22-4 to LCR22.5) with normal intelligence; (b) mild forms of specific genetic conditions contribute to persistent MD in otherwise typical persons; (c) heterogeneity of neurogenetic underpinnings of MD is suggested by poor performance in non-symbolic numerical processing, dissociated from visuospatial/visuoconstructional and phonological impairments; (d) similar to what happens in 22q11.2DS (LCR22-2 to LCR22-4), ANS impairments may also characterize 22q11.2DS (LCR22-4 to LCR22-5).
Introduction
Number processing abilities, such as magnitude comparison and estimation, and knowledge about the numerals, have been implicated in both typical and atypical math learning (Siegler and Braithwaite, 2017). Current discussions focus on the role of accuracy of numerical magnitude representations in the non-symbolic Approximate Number System (ANS) vs. access to these non-symbolic representations through symbolic numbers (Leibovich et al., 2017).
Accuracy in non-symbolic numerical representations has been linked to both typical (Halberda et al., 2008) and atypical (Landerl et al., 2004; Piazza et al., 2010; Mazzocco et al., 2011; Pinheiro-Chagas et al., 2014) math learning. Other studies suggest that symbolic numerical representations play a more important role (Rousselle and Noël, 2007; De Smedt and Gilmore, 2011; Szucs et al., 2013, see review in De Smedt et al., 2013). Meta-analyses indicate that correlations between number processing and math achievement are weak (r's between 0.2 and 0.3) and are slightly larger for symbolic over non-symbolic numerical processing (Chen and Li, 2014; Fazio et al., 2014; Schneider et al., 2017). It is also unknown how and when non-symbolic and symbolic processing influence math learning (Leibovich et al., 2017).
Developmental dyscalculia, math learning difficulties (MD) and number processing impairments have been described for some syndromes of environmental or genetic etiology such as fetal alcohol syndrome (Jacobson et al., 2011), fragile X syndrome in females (Mazzocco, 2001; Villalon-Reina et al., 2013), Turner's syndrome (Bruandet et al., 2004; Zougkou and Temple, 2016), Williams-Beuren syndrome (Krajcsi et al., 2009; Libertus et al., 2014) and velocardiofacial syndrome (VCFS, 22q11.2 deletion syndrome, 22q11.2DS) (Barnea-Goraly et al., 2005; De Smedt et al., 2009; Attout et al., 2017).
The presence of developmental dyscalculia among the phenotypes of many different genetic syndromes suggests that multiple specific genetic factors contribute to the emergence of dyscalculia. As the genotype-phenotype variability of genetic syndromes is large, milder forms of a given syndrome may eventually contribute to MD, particularly in individuals with normal intelligence.
One of the most investigated syndromes associated with developmental dyscalculia is 22q11.2DS, resulting from microdeletions of a specific region on chromosome 22. Chromosome 22 is the second smallest human chromosome and corresponds to approximately 1.6% of human genomic DNA (Genome Reference Consortium, 2018). Genetic alterations on chromosome 22q11.2 have been associated with numerous health conditions, including intellectual disability and schizophrenia. At least 48 genes have been identified in the region associated with 22q11.2DS, including PRODH and COMT, implicated in cognitive functions through regulation of dopamine metabolism (Karayiorgou et al., 2010; Espe, 2018).
The long arm of chromosome 22 contains interspaced low copy-repeated (LCR) sequences, which make this region susceptible to non-homologous recombination events leading to microdeletions or microduplications. Persons having typical 22q11.2DS present the microdeletion of the 22q11.2 (LCR22-2 to LCR22-4) interval.
To elucidate the genomic variations contributing to math learning difficulties, in a previous population study (n = 1,520 children), we investigated some genotypic and phenotypic characteristics of MD children, defined as standardized math achievement below the PR 25 (Ferreira et al., 2012). Among 82 MD children, we identified a 8-year-old girl presenting a microdeletion on chromosome 22q11.2 in the LCR22-4 to LCR22-5 interval (Carvalho et al., 2014).
Reviewing the literature, Carvalho and coworkers characterized a new syndrome, 22q11.2DS (LCR22-4 to LCR22-5), associated with microdeletions spanning only this interval and not extending proximally into the 22q11.2 (LCR22-2 to LCR22-4) interval (typically deleted in 22q11.2DS) or distally, into the 22q11.2 (LCR22-5 to LCR22-6) interval. Further, the authors proposed 22q11.2DS (LCR22-4 to LCR22-5) as an additional cause of dyscalculia in 22q11.2.
22q11.2DS (LCR22-4 to LCR22-5) is characterized by intellectual disability in most cases, and psychiatric symptoms and MD suggesting a heterogeneous condition (Table 1). To date, neither the neuropsychological phenotype nor the impairments in number processing have been detailed. Here, we describe a single-case, quasi-experimental study developed to characterize the cognitive-neuropsychological and numerical-cognitive endophenotypes underlying math learning difficulties in the child having the 22q11.2DS (LCR22-4 to LCR22-5) described by Carvalho et al. (2014).
22q11.2DS (LCR22-4 to LCR22-5) has already been reported in 33 persons (Saitta et al., 1999; Mikhail et al., 2007, 2014; Ben-Shachar et al., 2008; Newbern et al., 2008; Rodningen et al., 2008; Xu et al., 2008; Beaujard et al., 2009; Bruce et al., 2010; Tan et al., 2011; Verhoeven et al., 2011; Fagerberg et al., 2012; Molck et al., 2013; Carvalho et al., 2014; Lindgren et al., 2015; Spineli-Silva et al., 2017).
In general, the published studies describe in broad strokes the phenotypic and genotypic characteristics related to 22q11.2DS (LCR22-4 to LCR22-5) (Table 1), which can be summarized in five topics:
1) Distal microdeletions are a health condition independent of 22q11.2DS. Deletions and duplications in the 22q11.2 region are classified as proximal, central and distal (types I, II and III) (Burnside, 2015). 22q11.2DS (LCR22-4 to LCR22-5) is considered distal type I;
2) Although there is no consensus whether distal microdeletions cause a more subtle (Saitta et al., 1999; Mikhail et al., 2007; Carvalho et al., 2014) or more severe phenotype (Ben-Shachar et al., 2008; Xu et al., 2008; Tan et al., 2011; Lindgren et al., 2015), some characteristics have been described more frequently: (a) congenital heart diseases (most frequently of the Truncus Arteriosus type) observed in 16 of the 32 persons reported in the literature (Saitta et al., 1999; Mikhail et al., 2007, 2014; Ben-Shachar et al., 2008; Newbern et al., 2008; Xu et al., 2008; Beaujard et al., 2009; Bruce et al., 2010; Tan et al., 2011; Verhoeven et al., 2011; Fagerberg et al., 2012; Molck et al., 2013; Spineli-Silva et al., 2017); (b) prematurity and low birth weight reported in almost all patients (Saitta et al., 1999; Mikhail et al., 2007, 2014; Ben-Shachar et al., 2008; Newbern et al., 2008; Rodningen et al., 2008; Xu et al., 2008; Beaujard et al., 2009; Bruce et al., 2010; Tan et al., 2011; Verhoeven et al., 2011; Fagerberg et al., 2012; Molck et al., 2013; Lindgren et al., 2015; Spineli-Silva et al., 2017); (c) language development delay observed in six patients (Saitta et al., 1999; Ben-Shachar et al., 2008; Rodningen et al., 2008; Fagerberg et al., 2012; Lindgren et al., 2015; Spineli-Silva et al., 2017); d) bone malformations reported in 15 patients (Saitta et al., 1999; Mikhail et al., 2007, 2014; Ben-Shachar et al., 2008; Rodningen et al., 2008; Bruce et al., 2010; Tan et al., 2011; Fagerberg et al., 2012; Molck et al., 2013; Spineli-Silva et al., 2017); and, e) facial dysmorphisms marked by micrognathia, microcephaly, narrow palpebral fissures, arched eyebrows, featureless filter, hypertelorism, prominent nose, pointed chin, thin lips, etc. One or more of these phenotypes are reported in at least one patient (Saitta et al., 1999; Mikhail et al., 2007, 2014; Ben-Shachar et al., 2008; Newbern et al., 2008; Rodningen et al., 2008; Xu et al., 2008; Beaujard et al., 2009; Bruce et al., 2010; Tan et al., 2011; Verhoeven et al., 2011; Fagerberg et al., 2012; Molck et al., 2013; Lindgren et al., 2015; Spineli-Silva et al., 2017);
3) There is heterogeneity in intelligence. Most studies have qualitatively characterized intellectual disability. Normal or borderline intelligence is described for some patients (Ben-Shachar et al., 2008; Verhoeven et al., 2011; Carvalho et al., 2014);
4) Behavioral symptoms are briefly cited in most studies: (a) anxiety, social immaturity and social phobia; (b) Attention Deficit Hyperactivity Disorder (ADHD), poor impulse control, anger issues and aggressive behaviors; and, (c) Asperger disorder (Mikhail et al., 2007, 2014; Ben-Shachar et al., 2008; Carvalho et al., 2014; Lindgren et al., 2015);
5) Learning difficulties in mathematics have been reported in two cases with normal or borderline intelligence (Verhoeven et al., 2011; Carvalho et al., 2014). Additionally, Beaujard et al. (2009) described a case with family recurrence in which the mother had a history of learning difficulties.
As mentioned above, developmental dyscalculia is a heterogeneous condition, probably characterized by different subtypes and underlying cognitive mechanisms (Wilson and Dehaene, 2007; Rubinsten and Henik, 2009; Karagiannakis et al., 2014). At least five cognitive mechanisms have been implicated in typical and atypical math achievement: (a) working memory and executive processing, probably associated with ADHD; (b) phonological processing, probably associated with developmental dyslexia; (c) visuospatial and visuoconstructional processing, probably associated with nonverbal learning disability; (d) accuracy of number representations, probably underlying pure cases of developmental dyscalculia; and, eventually, (e) math anxiety, as a compound, aggravating factor.
Number processing deficits in 22q11.2DS (LCR22-4 to LCR22-5) must be contrasted to those observed in typical 22q11.2DS. In the typical 22q11.2DS, two of the most salient cognitive traits associated with developmental dyscalculia are impairments in visuospatial and visuoconstructional processing (Simon et al., 2005a,b; Antshel et al., 2008; Schoch et al., 2014; Wong et al., 2014; Attout et al., 2017), and in the accuracy of non-symbolic and symbolic numerical representations (Simon et al., 2005a,b; De Smedt et al., 2009; Oliveira et al., 2014; Attout et al., 2017; Brankaer et al., 2017). It is not known, for example, whether the numerical and visuospatial processing deficits observed in the typical 22q11.2DS reflect a common underlying impairment or may, eventually, be dissociated. Dissociation between visuospatial and numerical impairments in a case of developmental dyscalculia of genetic origin would be of theoretical interest, and would also hint at the neurobiological systems involved.
So far, no studies have specifically investigated the behavioral and cognitive phenotypes of distal microdeletions in 22q11.2, particularly 22q11.2 (LCR22-4 to LCR22-5). Therefore, the aim of the present study is to investigate and describe in detail the cognitive-neuropsychological characteristics of a girl presenting MD and 22q11.2DS (LCR22-4 to LCR22-5), who was assessed at ages 8 and 11. The underlying assumption is that, although this distal microdeletion is classified as a distinct syndrome, the pattern of general neuropsychological and numerical processing deficits presented by affected persons may resemble that presented by individuals with typical 22q11.2DS. This is based on the observation that some symptoms described for patients having 22q11.2DS (LCR22-4 to LCR22-5) have also been frequently described for patients having typical 22q11.2DS, e.g., conotruncal congenital heart malformations or submucous cleft palate. Therefore, there may be long range effects (Zeitz et al., 2013).
More specifically, we were interested in investigating whether the girl presents impairments in visuospatial and visuoconstructional processing and in the accuracy of numerical representations, two of the most salient phenotypic traits in the typical 22q11.2DS. We were also interested in investigating whether these two forms of impairment are dissociable. To test these hypotheses, we compared her general neuropsychological and numerical-cognitive performance at ages 8 and 11 using a single-case, quasi-experimental design (Crawford et al., 2010).
Clinical Report
A girl with 22q11.2DS (LCR22-4 to LCR22-5), was identified among children in a population screening for math learning difficulties in Belo Horizonte, Brazil (Ferreira et al., 2012; Oliveira-Ferreira et al., 2012; Carvalho et al., 2014). At the time of the screening, she was 8 years old and attending the 3rd grade of elementary school. Her intelligence was normal and her performance on a standardized arithmetic achievement test was below the PR 25. She was then referred for a comprehensive neuropsychological investigation and genotyping. Results of Multiplex Ligation-dependent Probe Amplification (MLPA) indicated the presence of an atypical distal microdeletion on chromosome 22q11.2. This microdeletion was confirmed, and its size was determined through an array CGH (947,631 bp) (Carvalho et al., 2014).
The girl underwent neuropsychological assessment twice. She was initially assessed at 8 years, by occasion of the population screening, and later at 11 years, when attending the 6th grade. She had shown learning difficulties since the beginning of elementary school. According to her mother, the difficulties had always been more severe in mathematics and in the interpretation of texts. She was retained in the 6th grade because of her math difficulties. This happened at the end of the school year, well after the second neuropsychological assessment. There was no history of difficulties in word reading and spelling or initial literacy acquisition. Her favorite subject at school was English and the girl was able to easily learn song lyrics in English.
The parents described her as a shy girl with a tendency to isolate. Additionally, according to them, the girl used to have problems expressing her needs and exposing her difficulties, especially at school. Her only friend was an 18-year-old cousin. She had difficulties initiating social interactions, especially with peers. Eventually, after becoming acquainted, she would interact normally.
At home, the girl was independent and helped with household chores, but performed at a slow pace and had difficulties concentrating in and finishing chores and homework. She was described as hyperactive, inattentive and anxious. The symptoms of hyperactivity were treated with methylphenidate for 2 months. Treatment was discontinued as the symptoms of inattention remained and anxiety symptoms were exacerbated. She had the habit of nail-biting. Parents reported some minor problems related to aggressive behavior. According to them, the girl would occasionally get into fights with her 6-year-old sister.
No information on pregnancy, delivery or initial development was available, as she was adopted at age 1 year. At that time, she was unable to sit or crawl. After 3 months with the adopted family, she began to walk and to utter her first words. Respiratory problems were constant in the first years of life. The parents also reported that occasionally the girl had nocturnal enuresis up to 7 years and a tendency to withhold urine when playing.
She lived with her adoptive parents and a younger sister, enjoying a stable home environment. The parents married 16 years ago. Both parents completed high school and had no history of learning difficulties. The adoptive father had been employed in the same company for more than 25 years. The adoptive mother was a housewife, who had serious health problems related to systemic lupus erythematosus, requiring constant treatment with corticosteroids. Her younger sister was the biological daughter of the couple. Follow-up disclosed that the biological daughter of the couple presented typical school achievement.
On clinical examination, the girl had short stature, normal weight and head circumference, narrow palpebral fissures, long nose, submucosal cleft of the palate, bifid uvula, pointed chin, long and thin fingers, short and broad nails (Carvalho et al., 2014). Her phenotypic characteristics are organized and compared to other published cases in Table 1.
Methods
The girl participated in a quasi-experimental case study. Her general neuropsychological performance was compared to that of available published Brazilian standards. Numerical-cognitive performance was compared to that of two different but demographically similar groups of typically developing children (Controls) at 8 and 11 years. Typically achieving children participating in the Control group were recruited from public schools and were assessed in the context of the same study in which she was identified. All Controls originated from the same socio-economic background as the girl. Specific statistical procedures were used to compare her performance to that of the Controls (Crawford et al., 2010). At 11 years, she also underwent a psychiatric assessment.
Participants and Procedures
All research procedures complied with the Helsinki principles and were previously approved by the local ethics in research board (Research Ethics Committee of the Federal University of Minas Gerais: CAAE: 0091.0.203.000-10). Informed parental consent was obtained for the purposes of research participation. Informed consent to participate in the study was obtained from the parents in written form and orally from the girl. A specific written consent for publication was also obtained in written form, signed by both the girl and her mother. This informed consent includes their agreement with the publication of the indirectly identifiable information such as gender and age and agreement with the publication of the case report.
All general neuropsychological tests used in the first assessment were reapplied and some tasks were added in the reassessment (Table 2). The same battery of numerical-cognitive tasks was used in the two assessments. At 8 years, the girl's performance in the numerical-cognitive evaluation battery was compared to that of a group of 35 girls (mean age = 8.32 years; SD = 0.47 years) attending the 3rd grade of public elementary schools. At 11 years, her performance in the numerical-cognitive evaluation battery was compared to the performance of a group of 24 girls (mean age = 11.38 years; SD = 0.49 years) attending the 6th grade of elementary public schools. All the individuals of both Control groups had average intelligence (PRs 50 to 75 on the Raven's Colored Progressive Matrices, Angelini et al., 1999) and did not present learning difficulties as assessed by the TDE Arithmetic and TDE Spelling (Stein, 1994; Oliveira-Ferreira et al., 2012).
Instruments
Behavioral Assessment
At 11 years, her adoptive parents responded the Child Behavior Checklist (CBCL, Rocha et al., 2012), a questionnaire that evaluates behavioral symptoms and psychosocial functioning of individuals aged 6 to 11 years. Her results in the CBCL were compared with the norms for girls of the same age group.
General Neuropsychological Assessment
In Table 2, the general neuropsychological domains evaluated when she was 8 and 11 years old, and their respective tasks and normative references, are summarized.
The Brazilian School Achievement Test (TDE), which was used as a criterion of typicality in school achievement, will be discussed in more detail. The TDE is a standardized test of school performance in children from the 2nd to 7th grades. It comprises three subtests, respectively, of Arithmetic, Reading and Spelling (Stein, 1994; Ferreira et al., 2012). The Arithmetic subtest is composed of three simple verbally presented word problems (i.e., Which is the largest, 28 or 42?) and 35 written arithmetic calculations of increasing complexity (i.e., very easy: 4-1; easy: 1230+150+1620; intermediate: 823*96; hard: 3/4+2/8). The single-word Reading subtest of the TDE consists of 70 single-word stimuli, which must be read aloud by the proband. The single-word Spelling subtest consists of dictation of 34 words of increasing syllabic complexity (i.e., toca; balanço; cristalização). The reliability coefficients (Cronbach's α) of the TDE subtests are 0.87 or higher. The TDE has been used in other studies, displaying both reliability and validity in assessing learning difficulties and their cognitive correlates (Moura et al., 2013, 2015; Haase et al., 2014; Lopes-Silva et al., 2014, 2016; Pinheiro-Chagas et al., 2014).
Numerical-Cognitive Assessment
An experimental battery for numerical-cognitive assessment in children and adolescents was used in the present, as well as in previous, studies (Costa et al., 2011; Pinheiro-Chagas et al., 2014). The numerical-cognitive battery comprises tasks of number processing and single-digit calculation. The following tasks were used:
Simple Reaction Time (SRT): The computerized RT task is a visual detection task used to control for possible differences in basic processing speed, not related to numerical tasks. In this task the picture of a wolf (height 9.31 cm; length = 11.59 cm) was displayed in the center of a black screen for a maximum time of 3,000 ms. Participants were instructed to press the spacebar on the keyboard as fast as possible whenever the wolf appeared. Each trial was terminated with the first key press. The task had 30 experimental trials, with an inter-trial interval varying between 2,000 and 8,000 ms. SRT was used to control for eventual effects of general processing speed on the numerical tasks.
Non-symbolic Magnitude Comparison Task: Participants were instructed to compare two sets of black dots, simultaneously presented in two white circles on the left and right sides of the screen. They were required to choose the larger numerosity by pressing a side-congruent key (Pinheiro-Chagas et al., 2014). On each trial, one of the two white circles contained 32 dots (reference numerosity), and the other contained 20, 23, 26, 29, 35, 38, 41, or 44 dots. Each numerosity was presented eight times, and every presentation was arranged in a different spatially pseudo-random configuration. The task comprised 64 testing trials. The maximum stimulus presentation time was 4,000 ms, and the intertrial interval was 700 ms. Between trials, a 3 cm fixation cross appeared on the screen for 500 ms. Non-numerical cues were prevented by using a MATLAB script to design and generate the sets of dots to represent the non-symbolic numerosities (Dehaene et al., 2005). This script was programmed so that, in half of the trials, dot size remained constant and total dot area covaried positively with the numerosity. In the other half of the trials, total dot area remained constant and dot size covaried negatively with numerosity. Each child's data were trimmed to exclude responses ±3 SD away from the individual mean RT. The internal Weber fraction (w) was calculated for each child as an indicator of approximate number system (ANS) or number sense acuity, based on the Log-Gaussian model of number representation (Dehaene, 2007), using the methods described by Piazza et al. (2004).
Single-digit Magnitude Comparison Task: In another task, developed by Pinheiro-Chagas et al. (2014), Arabic digits from 1 to 9 were presented on the computer screen (2.12 cm height, 2.12 cm length). The visual angle of the stimuli vertically and horizontally comprised 2.43°. The children were instructed to compare the stimuli with the reference number 5. The digits were presented in white on a black background. A predefined key on the left side of the keyboard should be pressed with the left hand, if the presented digit was less than 5. If the digit was greater than 5, a right key should be pressed with the right hand. The digit 5 was never presented on the computer screen (internal reference). Numerical distances between the stimuli and the reference digit (5) varied from 1 to 4. Each numerical distance was presented the same number of times. Between trials, a fixation point of the same size and color as the stimuli was presented on the screen. The task comprised 80 experimental trials. The maximum stimulus presentation time was 4,000 ms, and the intertrial interval was 700 ms. Dependent measures were mean accuracy and reaction times. A efficiency score P can also be used as a measure of symbolic magnitude processing efficiency, penalizing RT for inaccuracy: P = RT (1 + 2ER) according to Lyons et al. (2014). In the formula, RT means reaction time and ER stands for error rates, considering reaction time (RT) and errors rates (ER) as measures of performance for each child. ERs were multiplied by 2 because the task was a binary forced choice (ER = 0.5 indicates chance level). Higher scores indicate worse performance. If the performance were perfectly accurate, P would correspond to the individual's average RT (P = RT).
Set-size Magnitude Estimation: In the non-symbolic magnitude estimation task, participants were asked to verbally estimate the quantity of dots shown on the computer screen (Pinheiro-Chagas et al., 2014). The stimuli were black dots presented in a white circle over a black background. The numerosities were 10, 16, 24, 32, 48, 56 or 64 dots. Each numerosity was presented 5 times, each time in a different configuration. The same numerosity never appeared in consecutive trials. The task comprised 35 trials. Counting was avoided by setting the maximum stimulus presentation time to 1000 ms. The examiner, who was seated next to the child, pressed the spacebar and entered the child's response as soon as the child responded. A 3-cm wide/long fixation cross appeared on the screen between individual trials. Use of non-numerical cues was prevented by programming the stimuli in the same manner as those of the non-symbolic number comparison task, described above. Memorization effects due to the repetition of a specific stimulus were avoided in that, in each trial, the stimuli were randomly chosen from a set of 10 precomputed images with the given numerosity. For each subject, data were trimmed to exclude the responses ±3 SD from the mean chosen value across all of the trials. The mean coefficient of variation (cv) was selected as the dependent measure of ANS-accuracy.
Arabic Number Reading Task: Twenty-eight Arabic numbers printed in a booklet were presented, one at a time, and the child had to read the numbers aloud (Moura et al., 2015). The set of items consisted of numbers with up to 4 digits (3 numbers with one digit, 9 numbers with two digits, 8 numbers with three digits and 8 numbers with four digits). The internal consistency of the task is KR-20 = 0.90.
Arabic Number Writing Task: The participant was instructed to write dictated numbers using Arabic numerals (Moura et al., 2015). This task was composed of 40 items, and the numbers contained up to 4 digits (3 numbers with one digit, 9 numbers with two digits, 10 numbers with three digits and 18 numbers with four digits). The internal consistency of the task is 0.96 with the KR-20 formula.
Single-digit operations: This task consisted of single-digit addition (27 items), subtraction (27 items), and multiplication (28 items) operations for individual application, which were printed on separate sheets of paper (Costa et al., 2011). Children were instructed to answer as fast and as accurately as they could; time limit per block was 1 min. Arithmetic operations were organized in two levels of complexity and were presented to the children in separate blocks: one block consisted of simple arithmetic table facts and the other block of more complex problems. Simple additions were defined as those operations having results below 10 (e.g., 3 + 5), while complex additions were those having results between 11 and 17 (e.g., 9 + 5). Tie problems (e.g., 4 + 4) were not used for addition. Simple subtractions were defined as those operations having operands less than 10 (e.g., 9 – 6), while complex subtractions were defined as those having operands ranging from 11 to 17 (e.g., 16 – 9). No negative results were included in the subtraction problems. Simple multiplications were defined as those operations having results less than 25 and/or with the digit 5 as one of the operands (e.g., 2 × 7, 5 × 6), while complex multiplications were defined as those having products ranging from 24 to 72 (6 × 8). Tie problems were not used for multiplication. Reliability coefficients were high (Cronbach's α > 0.90).
Simple Word Problems: Twelve simple arithmetic problems (e.g., “Gabi has 3 reais. Debora has 6 reais. How much do they have together?”) were read aloud by the examiner and simultaneously presented in written form. The child had to solve the problem mentally and write the answer on the paper, with a time limit of 1 min per problem. The dependent variable was the number of correct responses (for more details, see Costa et al., 2011).
Statistical Analysis
All scores were z-standardized for age to facilitate comparisons. In the comparison with the published norms, a deviation of 1.5 SD from the mean was used as the cut-off score to determine whether the domain was impaired or preserved. A cut-off score of test performance was employed because diagnosis implies categorization: either the person presents or does not present some health condition. The cut-off score chosen is not overly restrictive or excessively compliant. Larger time executions in the 9-Hole Peg Test (Poole et al., 2005), Trail Making Test, and Victoria Stroop color-word interference test (Charchat-Fichman and Oliveira, 2009) indicate lower performance. Thus, in order to improve their graphic depiction, the direction of change was inverted (Figure 1). The girl's performance on the numerical-cognitive tasks was compared to that of Controls using the statistical methods for neuropsychological case studies developed by Crawford and colleagues (Crawford and Howell, 1998; Crawford and Garthwaite, 2002; Crawford et al., 2010). The analysis concerns the typicality of her performance in comparison with the Control groups. The modified t-test proposed by Crawford and Garthwaite (2002) calculated with singlims.exe was used to compare her scores on each task to that of the Control groups' means. Effect size and power analyses were also calculated (Crawford et al., 2010).
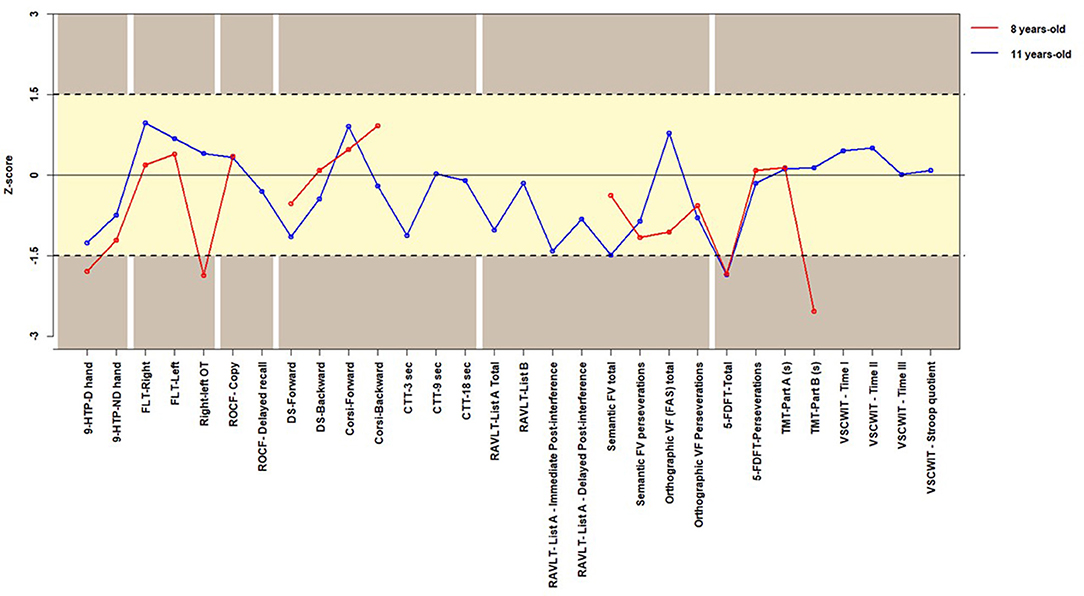
Figure 1. The girl's performance on the general neuropsychological assessment battery. 9-HPT-D.hand, 9-HPT - dominant hand; 9-HPT-ND.hand, 9-HPT - nondominant hand; FLT, finger localization task; ROCF, Rey-Osterrieth Complex Figure; Right-left OT, Right-left Orientation Task Total score; DS, Digit Span; CTT, consonant trigrams test; RAVLT, Rey Auditory-verbal Learning Test; 5-FDFT, 5-point design fluency test; Semantic VF, Semantic Verbal Fluency; Orthographic VF (FAS), Orthographic Verbal Fluency (FAS); VSCWIT, Victoria Stroop color-word interference test.
Results
The results are organized into four subsections: intelligence and school performance, behavioral assessment, general neuropsychological assessment and numerical-cognitive assessment.
Intelligence and School Performance
The girl performed normally on intelligence tests, reaching the 60th percentile on Raven's Colored Progressive Matrices (Angelini et al., 1999) in both assessments.
At 11 years, the girl obtained a WISC-III Full-Scale IQ of 98. Although her results did not show a discrepancy between Verbal and Performance IQ, she presented a heterogeneous profile in the subtests.
In the Verbal subscales, the girl obtained average scaled scores in the tasks that involved ability to synthesize and categorize verbal knowledge (Vocabulary: scaled score = 15; z-score = 1.66; Similarities: scaled score = 12; z-score = 0.66; and Comprehension: scaled score = 11; z-score = 0.33). Otherwise, she presented lower scaled scores, still in the normal range, in the tasks that evaluated word problem solving (Arithmetic: scaled score = 8; z-score = −0.66), general knowledge and intellectual curiosity (Information: scaled score = 6; z-score = −1.33), and verbal memory (Digit Span: scaled score = 6; z-score = −1.33). In the performance subscales, the girl obtained average scaled scores in the tasks that involved organization of the whole from separate elements (Object Assembly: scaled score = 12; z-score = 0.66), visual organization (Picture Completion: scaled score = 11; z-score = 0.33), visual memorization and motor coordination (Coding: scaled score = 11; z-score = 0.33). Additionally, she presented below average scaled scores in the tasks that evaluated capacity for visual attention (Symbol Search: scaled score = 6; z-score = −1.33), and analysis and interpretation (Picture Arrangement: scaled score = 4; z-score = −2.00).
The girl's performance on the Spelling and Reading subtests of the TDE (Stein, 1994) was classified as average (PRs between 25 and 75), at both ages of 8 and 11 years. In the TDE Arithmetic subtest, her performance was below the PR 25 at both 8 and 11 years. At 11 years, she was also evaluated with non-word repetition (Santos and Bueno, 2003), non-word reading and phoneme elision tasks (Lopes-Silva et al., 2014). The girl performed at the maximum level in these three phonological processing tasks.
Behavioral Assessment
During the evaluation, the girl was extremely shy and sometimes required extra incentive in order to participate. In the CBCL (Rocha et al., 2012), she attained clinical scores that identified social (T = 66), attention (T = 73), DSM-anxiety (T = 65) and DSM-ADHD problems (T = 66). Scores in the other subscales were in the typical range.
General Neuropsychological Assessment
At 8 years old, the girl had deficits in motor dexterity in the right (dominant) hand (z = −1.79) and in right-left orientation (z = −1.87). At 11 years old, her performance on both tasks did not differ from the performance of the Controls in motor dexterity and right-left orientation (Figure 1).
Visuospatial and visuoconstructional abilities were measured using the Rey-Osterrieth Complex Figure copy and delayed recall. The girl performed typically in both evaluations. At 8 years old, her performance was superior to that of the Controls (copy: z = 0.35). At 11 years old, her performance was similar to that of the Controls (copy: z = 0.32; delayed recall: z = −0.31) (Figure 1).
The girl also performed typically on short-term and working memory tasks. On the digit span, her performance was similar to that of the Controls, in both the 8-year-old (z Forward = −0.54; z Backward = 0.08) and 11-year-old (z Forward = −1.15; z Backward = −0.45) evaluations. On the Corsi Blocks, she also performed typically. Her performance was similar to the Controls in both the 8-year-old (z Forward = 0.48; z Backward = 0.92) and 11-year-old (z Forward = 0.90; z Backward = −0.21) evaluations (Figure 1).
At the 11-year-old evaluation, two tasks related to memory were added to the battery of neuropsychological tests. In the Consonantal Trigrams, which evaluate interference in short-term memory, and in the Rey auditory verbal learning test (RAVLT), a task that evaluates verbal long-term memory, the girl performed similarly to the Controls.
The girl presented evidence of impairment in some executive functions in both evaluations. At 8 years old, she. presented low productivity on the 5-point design fluency test, differing from the Controls (z = −1.84). This difference persisted in the 11-year-old assessment (z = −1.86). Productivity in the semantic word fluency task was typical at 8 years (z = −0.38) and slightly over the cut-off score at 11 years (z = −1.49). In part B of the Trail Making test, which evaluates motor skills, processing speed, attention capacity (visual search), monitoring, inhibition and set-shifting, she presented a much lower performance than the Controls at the 8-year-old assessment (z = −2.54), but no differences were found between the girl and the Controls (z = −0.14) at 11 years. At the 11-year-old assessment, one task was added to the battery with the purpose of evaluating the executive functions in more detail. In the Victoria Stroop color-word interference test, which evaluates monitoring, error detection/correction and inhibitory control, she presented satisfactory performance (Stroop quotient: z = 0.08).
Numerical-Cognitive Abilities
The results of the numerical-cognitive tasks are presented in Table 3. Although the SRT is not a numerical task, it was used to control for effects of general processing speed on numerical tasks. At the 8-year-old assessment, the girl's SRTs were slower than that of the Controls (p = 0.01, d = 2.33). At the 11-year-old assessment, her performance was similar to that of the Controls (p = 0.38, d = 0.30). An efficiency score, penalizing reaction time by error rate, was used to index the results in the single-digit magnitude comparison task. No similar compensations were used for speed-accuracy trade-offs in the non-symbolic comparison (w) and set-size magnitude estimation (cv) tasks, as the emphasis on the dependent measures in these tasks is related to accuracy.
Non-symbolic magnitude comparison: In addition to her higher reaction time on the control task, the girl presented much lower performance in reaction time on the non-symbolic magnitude comparison task (p < 0.001, d = 2.94), when compared to the Controls. At 8 years, her error rate in the non-symbolic magnitude comparison task was significatively higher (p = 0.02, d = 2.00). The log-Gaussian model did not adjust at 8 years, so it was not possible to calculate the internal Weber fraction (Table 3). At 11 years old, her reaction times on the non-symbolic magnitude comparison task were slightly above the cut-off score (p = 0.07, d = 1.51), when compared to the Controls. The internal Weber fraction was 0.28 (p = 0.06, d = 1.60).
Single-digit magnitude comparison task: At 8 years, the girl presented significantly higher RTs (p = 0.01, d = 2.63) and error rates (p = 0.03, d = 1.83) in the single-digit magnitude comparison tasks, when compared to the Controls. Her efficiency score P was significantly higher than that of the Controls (p < 0.001, d = 4.22). No significant RT (p = 0.48, d = −0.02), error rate (p = 0.22, d = 0.80) or efficiency score P (p = 0.40, d = −0.25) differences were observed at 11 years in the single-digit magnitude comparison task.
Set-size estimation: At 8 years, her performance on the set-size estimation task was random. At 11 years, she presented a significantly higher coefficient of variation when compared to the Controls on the set-size estimation task (p = < 0.001, d = 4.75).
Single-digit calculation: At 8 years, her performance was lower than that of the Controls on the single-digit operation tasks, both in simple addition (p = 0.01, d = −2.38) and in simple subtraction (p = 0.01, d = −1.06). At this age, the girl was unable to perform any slightly more complex addition or subtraction operations. Multiplication items were not applied at 8 years. At 11 years, her performance did not differ from the Controls in simple addition (p = 0.07, d = 1.54), complex addition (p = 0.14, p = −1.12), simple multiplication (p = 0.33, d = 0.43) and complex multiplication (p = 0.49, d = −0.01) (Table 3). Difficulties in simple subtraction (p < 0.001, d = −3.92) and complex subtraction (p = 0.01, d = −2.52) persisted.
Arabic number reading and writing: At 8 years, the girl presented much lower performance than the Controls on the Arabic number reading task (p = 0.05, d = −1.66). Her performance on the Arabic number writing task was normal (p = 0.48, d = −0.05). At 11 years, the girl's performance was adequate in tasks that assessed Arabic numbers reading (p = 0.28, d = 0.58) and writing (p = 0.34, d = 0.40).
Simple word problems: At 8 years, the girl's performance on simple word problems was below the cut-off score when compared to the Controls (p = 0.06, d = −1.61). At 11 years, her performance on this task was normal (p = 0.18; d = −0.93).
Discussion
This is the first study to characterize in detail the cognitive-neuropsychological phenotype, including cognitive-numerical performance, of an individual with an atypical distal microdeletion on the long arm of chromosome 22 (22q11.2DS LCR22-4 to LCR22-5).The participant is a girl identified through a school population screening for math learning difficulties (Carvalho et al., 2014). This girl was adopted in early infancy and lived in a stable family environment. She was assessed twice, at 8 and 11 years. Her intelligence was normal average at both times. Math learning difficulties persisted from 8 to 11 years, with performance below the PR 25. No difficulties were observed in word reading, word spelling and related phonological abilities. The family reported reading comprehension difficulties. Inattention and social anxiety symptoms were also observed. General neuropsychological assessment disclosed some minor alterations. Visuospatial/visuoconstructional abilities, working memory and long-term memory were average at both times. At 8 years, she exhibited impairments in motor dexterity, right-left orientation and alertness. These impairments were not observed at the 11 years assessment. Difficulties with some executive function tasks were detected at 8 years, such as in the productivity of the 5-point-design fluency task and the set-shifting dimension of the trail-making test. These difficulties had largely disappeared by 11 years.
Persistent math learning difficulties were associated with impairments in both non-symbolic and symbolic numerical magnitude processing and in single-digit calculation. Statistically significant slower reaction times and higher error rates were observed in all non-symbolic and symbolic numerical magnitude processing tasks at 8 years. At 11 years, single-digit magnitude comparison was average, however, she exhibited difficulties with the accuracy of non-symbolic numerical representations (d = 1.60) and set-size estimation (d = 4.75). Single-digit calculation was consistently impaired at both times. At 11 years, the girl had mastered single-digit addition and multiplication calculations, but she was still struggling with even the most simple subtraction problems. She did not present difficulties with very simple word problems involving single-digit addition and subtraction, at either time. Symbolic numerical transcoding was also typically acquired.
We will discuss the main theoretical and clinical/educational issues raised by the present study in four sections: (a) neuropsychological functioning; (b) cognitive-numerical abilities; (c) mechanisms of math learning difficulties; and (d) clinical and educational implications.
Neuropsychological Functioning
Atypical 22q11.2DS (LCR22-4 a LCR22-5) is a new genetic entity, related but different from typical 22q11.2DS (LCR22-2 a LCR22-4) (Carvalho et al., 2014). Previous research consists exclusively of case (series) reports. The behavioral and cognitive profile of affected individuals was characterized only qualitatively, through clinical description. In this study, we move a step forward, reporting data from a detailed neuropsychological investigation and testing hypothesis regarding the nature of observed cognitive-numerical impairments. We first discuss the results of the general neuropsychologicalassessment.
Intelligence: In general, most cases of 22q11.2DS (LCR22-4 to LCR22-5) have been described as having intellectual disability and receiving special education (Ben-Shachar et al., 2008; Xu et al., 2008; Mikhail et al., 2014; Lindgren et al., 2015). Only one study reported the IQ of a girl with microdeletion in LCR22-4 to LCR22-5 region. In this study, Verhoeven et al. (2011) described a 17-year-old female and her level of intelligence was found to be borderline (total WISC-R IQ = 73). Two cases presenting presumably normal intelligence without detailed description were reported by Ben-Shachar et al. (2008) and Fagerberg et al. (2012).
In children with typical 22q11.2DS, intellectual disability is present in 40% to 45% of affected individuals. When intelligence is normal, usually the IQ is in the borderline range (IQ = 70 to 85, Swillen et al., 1997; Woodin et al., 2001; Green et al., 2009). In children, lower scores are observed in the Performance IQ. This discrepancy tends to decrease in adults (Moberg et al., 2018). One hypothesis is that concomitant lowering of Verbal IQ tends to reduce the discrepance. A reduction of Verbal IQ from childhood to adolescence has been reported in some individuals with typical 22q11.2DS, and it is considered a risk factor for psychosis (Gothelf et al., 2005, 2009).
General intelligence scores remained stable in this girl for three years. Further follow-up is required. Normal intelligence in our participant indicates that intellectual disability is not an necessary phenotypic trait in 22q11.2 (LCR22-4 to LCR22-5). Research on intellectual abilities of individuals with genetic syndromes is biased by the fact that most severe cases have a higher probability of being recognized by families, clinicians and educators.
Visuospatial and motor abilities: Previous reports have underscored the severity of impairments in motor dexterity and visuospatial/visuoconstructional processing in cases of 22q11.2DS (LCR22-4 to LCR22-5). Lindgren et al. (2015) described a 4-year-old patient with 22q11.2DS (LCR22-4 to LCR22-5), that presented deficits in visual perception and motor integration, and mildly delayed gross motor milestones. In 2008, Rodningen and coworkers briefly described a 7-year-old patient with 22q11.2 (LCR22-4 to LCR22-5), presenting the same profile. Additional cases of 22q11.2DS (LCR22-4 to LCR22-5) showing motor deficits have been reported in the literature (Ben-Shachar et al., 2008; Beaujard et al., 2009; Verhoeven et al., 2011; Fagerberg et al., 2012; Mikhail et al., 2014; Spineli-Silva et al., 2017). Impairments in visuomotor integration were reported in two additional articles (Mikhail et al., 2007; Verhoeven et al., 2011).
Individuals with typical 22q11.2DS also present motor delays and difficulties with motor coordination from infancy on (Swillen et al., 1999; Bearden et al., 2001; Gerdes et al., 2001; Vicari et al., 2011). Large and consistent deficits were found for motor skills (d = −1.17) (Moberg et al., 2018). Additionally, occurrence of visuospatial and visuoconstructional impairments is frequent although variable in typical 22q11.2DS (Antshel et al., 2008; Jacobson et al., 2010; Schoch et al., 2014).
Most individuals previously reported with atypical 22q11.2DS were observed in infancy and at preschool age. Unfortunately, as our participant was adopted, there is no information regarding her obstetric and early infancy developmental background. The family reports motor delay at the end of the first year, when she was adopted. This improved in the following 3 months. Minor impairments in motor dexterity, body representation and alertness were observed at 8 years and improved with time (Figure 1). Additionally, and importantly, she did not present visuospatial/visuoconstructional impairments at either time (Figure 1). Anyway, the severity of visuospatial and motor impairments in previous reports of both atypical and typical 22q11.2DS contrast with the mildness of impairments in our participant.
Memory: Memory functions were not investigated in previous reports of atypical 22q11.2DS.
In general, individuals with typical 22q11.2DS present better performance on tasks of verbal rather than visuospatial memory (Woodin et al., 2001; Wong et al., 2014). However, both kinds of memory are impaired compared to controls. Moderate to large effect sizes were found for verbal memory (d = −0.70) and visual memory (d = −1.0) (Moberg et al., 2018). Individuals with typical 22q11.DS present similar performance as controls in tasks of information acquisition and retrieval (Lajiness-O'Neill et al., 2006; Debbané et al., 2008). Difficulties are more apparent in tasks in which the participant needs to discriminate stimulus relevance. These memory alterations may constitute a trait vulnerability marker signaling increased risk for schizophrenia in the typical 22q11.2DS population (Debbané et al., 2008).
Working and episodic visuospatial memories were intact in our participant (Figure 1). A discrepancy between higher digit span scores and lower but still normal total WISC Digit scores was observed and may be ascribed to attentional fluctuation. Difficulties with attention were also qualitatively observed in the RAVLT performance.
Executive functions: Deficits in executive functions were described in the case of 22q11.2DS (LCR22-4 to LCR22-5) reported by Verhoeven et al. (2011). They described an 18-year-old girl with borderline intelligence and deficits related to planning and concentration. Other reported cases have presented more severe cognitive impairments related to intellectual disability.
Impairments in executive functions are frequent, severe and persistent in individuals with typical 22q11.2DS (Woodin et al., 2001; Robin and Shprintzen, 2005). Moberg et al. (2018) observed moderate to large impairments in basic executive functions (up to d = −0.90). Executive function impairments, together with progressive verbal IQ decline, may play a role in the vulnerability to psychiatric disorders, such as psychoses (Gothelf et al., 2005, 2009).
The girl presented difficulties with some executive function tasks. We feel that her deficits in executive functions were slight and tended to improve. In the 3-year period of observation, no deterioration in her cognitive status was observed.
Psychosocial functioning: The girl is the eighth case with 22q11.2DS (LCR22-4 to LCR22-5) reported in the literature presenting symptoms of impulsivity and inattentiveness (Mikhail et al., 2007; Fagerberg et al., 2012). Her psychosocial functioning profile, including attention, social and anxiety problems, had some similarities and differences with those reported previously. Mikhail et al. (2014) described 4 cases with social immaturity, poor impulse control and anger issues, ADHD, anxiety and Asperger Disorder. The girl did not present symptoms of autism, but she presented characteristics of social phobia. Aggressive behaviors also seem to be common in patients with 22q11.2DS (LCR22-4 to LCR22-5) (Ben-Shachar et al., 2008; Verhoeven et al., 2011; Mikhail et al., 2014; Lindgren et al., 2015). Aggressive behavior was not a major issue in the participant.
The relatively mild psychosocial impairment observed in our participant contrasts with the more severe difficulties encountered by individuals with both atypical and typical 22q11.2DS, including the risk of psychosis (Bassett and Chow, 2008). In typical 22q11.2DS, psychosis is estimated to occur in up to 22.6% of patients after adolescence (Bassett and Chow, 2008).
School learning difficulties: Normal intelligence and math learning difficulties have been described in two cases of 22q11.2DS (LCR22-4 to LCR22-5) (Verhoeven et al., 2011; Carvalho et al., 2014). The most salient phenotypic features presented by this participant were the difficulties with number processing and arithmetic calculation. This is the first study to report a detailed neuropsychological investigation of an individual with 22q11.2DS (LCR22-4 to LCR22-5) with normal intelligence and specific learning difficulties.
In summary, the present study suggests a huge variability in the cognitive and behavioral phenotype of 22q11.2DS (LCR22-4 to LCR22-5). Less severely affected individuals may have normal intelligence associated with milder behavioral issues and specific school learning problems. Next, we compare these math learning difficulties with those observed in typical 22q11.2DS (LCR22-2 to LCR22-4). Math learning difficulties will be emphasized, as they are a prominent feature of the present participant as well as in typical 22q11.2DS.
Cognitive-Numerical Abilities
It is interesting to compare the profile of cognitive-numerical and arithmetic performance observed in the girl with that of typical 22q11.2DS. Math learning difficulties are a hallmark of the 22q11.2DS phenotype in individuals with normal intelligence (De Smedt et al., 2009). Math learning difficulties in typical 22q11.2DS seem to be unrelated to phonological processing impairments and probably reflect difficulties in more basic numerical and/or visuospatial processing (De Smedt et al., 2008).
De Smedt et al. (2009) observed that 22q11.2DS children's performance did not differ from that of controls in the tasks of reading numbers and single digit calculation. However, 22q11.2DS children were slower than controls in number comparison and in addition/subtraction calculations with larger numbers.
Oliveira et al. (2014) were the first to report inaccuracy of non-symbolic numerical magnitude representations (indexed by the internal Weber fraction, w) in typical 22q11.2DS. However, performance was variable, as not all individuals with 22q11.2DS presented impairments in ANS accuracy. Impairment in ANS, indexed by w in the non-symbolic numerical comparison task, was later confirmed by Attout et al. (2017). Additionally, these authors observed that ANS accuracy was impaired in the visuospatial but not in the auditory version of the non-symbolic comparison task. This suggests a connection between non-symbolic numerical and visuospatial representations. As mentioned before, visuospatial impairments are an important feature of typical 22q11.2DS.
A connection between numerical and spatial representations is suggested by the mental number line model of approximate numerical representations (Dehaene, 1997, 2007; Nieder and Dehaene, 2009). According to this model, the psychophysical signature of numerical magnitude representations suggests a spatialization of approximate numerical representations: (a) numerical magnitude discriminations are increasingly (ratio variability) and proportionally (scalar variability) more difficult as the distance between the numerical stimuli decreases; (b) accuracy in numerical representations also decreases as the numerical magnitude increases in a logarithmically compressed way; finally, (c) smaller digits are processed preferentially by the right and larger digits by the left hemispheres, suggesting a spatial orientation of the mental number line. According to Dehaene (2007) and Nieder and Dehaene (2009), these characteristics indicate that non-symbolic numbers may be represented approximately as a log-Gaussian distribution of the neuronal discharges ordered by numerical magnitudes.
The spatial nature of numerical representations and their impairments in typical 22q11.2DS have been explored in several studies by Simon et al. (2005a,b) and Simon (2008). In these studies, impaired performance of children with 22q11.2DS in a non-symbolic comparison task was associated with visuospatial manipulations reducing stimuli discriminability. According to the granularity hypothesis, Simon (2008) attributed the numerical processing deficits of individuals with 22q11.2DS to a more basic spatial representation inaccuracy or lack of spatial resolution.
Our participant presented persistent math difficulties, investigated from 8 to 11 years. Four possible cognitive-numerical sources for these difficulties may be considered: (a) visuospatial and visuoconstructional impairments; (b) phonological processing impairment; (c) basic numerical impairment; (d) executive dysfunction. The first two are discarded because there was no evidence of impairment in visuospatial/visuoconstructional and phonological processing abilities. Transcoding abilities of more complex numerals is indicative of good spatial and phonological processing abilities. Moreover, improving ability with commutative single-digit operations and persisting difficulties with subtraction suggest an impairment in the ANS. This hypothesis will be considered next.
In the present participant, the agreement among impairments of numerical processing in different modalities and tasks and their persistence is remarkable. Some evidence indicates that experimental tasks of numerical processing lack concurrent validity (Maloney et al., 2010; Price et al., 2012; Pinheiro-Chagas et al., 2014; Smets et al., 2015) and their test-retest reliability has not been explored extensively (Haase et al., 2014). The results indicate that, at least in some cases, basic numerical impairments may be consistent and persistent.
The most remarkable feature of numerical-cognitive impairments in the girl is related to severe impairments in basic numerical magnitude processing. The available data do not allow us to definitely decide if her impairments are related to non-symbolic numerical magnitude representational inaccuracy (Landerl et al., 2004) or to access to non-symbolic representations from symbolic ones (Rousselle and Noël, 2007). Accordingly, an individual could have difficulties learning math owing to some basic numerical magnitude representational deficit or to difficulties with accessing, storing and manipulating numerical information in working memory. These hypotheses will be addressed in the next section, in the context of the mechanisms putatively involved in MD.
Cognitive Mechanisms of Math Learning Difficulties
No substantive qualitative differences were observed in the cognitive mechanisms putatively underlying the present participant's math difficulties and those observed multifactorial developmental math learning difficulties (Wilson and Dehaene, 2007; Karagiannakis et al., 2014). The mathematical behavioral genetic approach partitions variance at the population level and does not allow identification of specific mechanisms implicated in single individuals. This can be accomplished only by molecular-genetic and neuropsychological investigations of specific genetic etiologies.
Current multiple deficit models of developmental disabilities consider that the phenotypic expression is dependent on complex genetic-environmental interactive mechanisms (Pennington, 2006; Johnson, 2012). Relationships between the genetic-environmental etiologic level and the phenotypic expression are not simple, one-to-one, and are subject to environmental sources of regulation at different times. The construct endophenotype was suggested to characterize intermediate steps in this complex, epigenetic path from the genotype to the phenotype (Rutter et al., 2006; Bishop, 2009).
Several endophenotypes were identified in the present study as potentially relevant for the girl's math difficulties as well as for math difficulties in general. In addition to basic numerical processing, discussed in the last section, the following potentially relevant mechanisms were identified in the present participant:
Motor ability: Basic perceptual and motor impairments are a frequent observation in several developmental disorders (Denckla, 1997, 2003), and are predictive of cognitive and behavioral problems at school age (Batstra et al., 2003). Deficits in finger gnosias (Costa et al., 2011) and motor incoordination (Lonnemann et al., 2011) have been described in children with MD. The meaning of these perceptual and motor impairments is uncertain. Bottom-up theories interpret cognitive deficits as a consequence of a disordered developmental process, encompassing the most basic perceptual motor abilities from infancy on (Nicolson and Fawcett, 2010; Elliott and Grigorenko, 2014). According to the procedural deficit hypothesis, MD could be related to difficulties in automatizing the implicit associations underlying numerical concepts and operations (Vandervert, 2017; Prado, 2018). An alternative explanation is that perceptual and motor impairments constitute markers of severity or co-localizares, indicating the presence and anatomic location of brain dysfunction (Denckla, 1997, 2003).
Working memory and executive functions: Impairments in working memory (Raghubar et al., 2010) and executive functions (Bull and Lee, 2014) are an important trait identified in individuals with MD. The ability to store and manipulate information temporarily in working memory is an important requirement at every step in the acquisition of arithmetics, such as counting (Geary et al., 2004), single-digit calculation (Menon et al., 2000; De Smedt et al., 2009), multi-digit calculation (Klein et al., 2009), numerical transcoding, (Barrouillet et al., 2004; Camos, 2008) and word problem solving (Swanson and Sachse-Lee, 2001). Attentional and executive functions have been implied, even in basic quantitative-numerical decisions (Clayton and Gilmore, 2015; Merkley et al., 2016). For example, inhibition of irrelevant perceptual dimensions may play a role in non-symbolic numerical magnitude comparisons. It is notoriously difficult to experimentally control covariation between the discrete numerical and continuous dimensions of stimuli in these tasks (Leibovich and Henik, 2014). The difficulty of the task could then be related to the need to inhibit the irrelevant continuous dimensions, such as surface and luminance, in order to decide based on the relevant discrete magnitude dimension. Other research indicates, however, that in the range of numerosities usually investigated, discrete numerosity is more perceptually salient and associated with math achievement than continuous dimensions such as texture (Anobile et al., 2016).
Math anxiety: Math anxiety is weakly and negatively associated with math achievement, with correlations on the order of −0.25 to −0.40 (Hembree, 1990). Math anxiety is both a risk factor and a consequence of MD (Ma, 1999). However, math anxiety and achievement are dissociable phenomena (Lee, 2009; Stankov et al., 2012), with both highperforming individuals being anxious and lowperforming individuals not being anxious. Usually, math anxiety is not considered a sort of learning disability (Ashcraft and Krause, 2007). It is considered an important concomitant or aggravating factor of existing difficulties.
In summary, several mechanisms were identified as potentially relevant for the MD in the present participant. It is important to balance and to integrate the evidence, connecting it to the big picture of MD in general. It is unfortunate that the genetic and psychosocial background of the participant before adoption is unknown. Data indicates that adopted children have been previously subject to both genetic and environmental risks for poor school achievement (Van Ijzendoorn et al., 2005).
Perceptual and motor impairments and anxiety may also have played a role in the genesis of the girl's math difficulties. Right-left orientation difficulties and motor dexterity improved with time but could have played a role at a crucial moment in learning arithmetics. Math anxiety may have competed for cognitive resources required for math learning at several moments.
The most interesting question is the relative role played by basic numerical processing and executive functioning. The possibility that executive dysfunction may have played a role cannot be excluded. First, her difficulties with executive functions were relatively mild, at least at the times of assessment; and, the clinical history does not suggest severe impairments in self-regulation. Second, her basic numerical processing difficulties were severe, persistent and concordant across modalities and tasks.
The numerical processing abilities of the participant can be interpreted in terms of the criteria proposed by Rousselle and Noël (2007). According to these authors, an access disorder, probably related to executive dysfunction, is characterized by variable and discrepant performance, with sparing of non-symbolic over symbolic numerical processing. The representational deficit is otherwise characterized by modality-independent and comprehensive difficulties with numerical processing. The pervasiveness of the girl's numerical processing difficulties and the mildness of her executive function difficulties suggest a representational deficit.
Investigations at the population and single individual level play complementary roles in partitioning variance and identifying specific sources of difficulties in math achievement. Since working memory and executive function impairments are frequent in all developmental disorders, one important question is related to the specificity of the problem. Why should one kid develop difficulties only in math and the other only in reading?
Multiple deficit models help to understand the complex interplay between specific and general cognitive factors in the origin of MD. According to a model proposed by Johnson (2012), a kid with a basic numerical processing impairment could compensate for the resulting difficulties, if executive processing resources are available. Otherwise, when general processing resources are insufficient, the difficulties are not compensated and may call attention of parents, educators and clinicians, leading to a diagnosis. In the present participant, multiple sources of cognitive and psychosocial variability were identified that could interact with the genetic condition, leading to math learning difficulties.
Clinical and Educational Implications
The main results of our study are that math learning difficulties may be associated with a specific genetic etiology (22q11.2DS; LCR22-4 to LCR22-5) and with more or less specific cognitive mechanisms (ANS and/or executive function impairments). Obviously, identifying a potential specific genetic etiology in a case of MD does not ensure that it plays a causal role in the difficulties of that single individual. It also does not exclude a role for other genetic or environmental factors. It is especially important to consider this in the present individual, as the girl was adopted and little information is available on her background before adoption. What are the implications of these findings for neuropsychological and educational practice?
Etiology of developmental and learning disorders is considered to be multifactorial; i.e., resulting from the interaction of several polygenic and environmental influences (Asbury and Plomin, 2013). It is, however, increasingly being recognized that, at the individual level, specific causes may play a role (Carvalho et al., 2014). For example, chromosomal aneuploidies have been recognized as a cause of language development and reading learning difficulties (Simpson et al., 2014). Specific genetic causes also contribute to autism (Cohen et al., 2005). The extreme variability of clinical presentation makes diagnosis difficult in milder cases.
Other research indicates that individuals with learning difficulties present higher rates of medical, especially neurological and psychiatric, comorbidities. This may occur in math (Shalev and Gross-Tsur, 1993) although not in reading learning difficulties (Cuvellier et al., 2004; Billard et al., 2008). Focal cerebral damage has been reported in cases of developmental dyscalculia and dyslexia (Levin et al., 1996; Daigneault and Braun, 2002). Rolandic epilepsy is commonly associated with learning difficulties in children of normal intelligence (Canavese et al., 2007). Common diseases, such as diabetes and asthma, are also more common in children with learning difficulties than in the general population (Blackman and Gurka, 2007; Hannonen et al., 2010).
Specific etiologies might be more common than usually thought. They are not identified because they are not looked for. Polygenes play a causative role at the population but not at the single individual level. The same holds for psychosocial factors. Deprivation, neglect or maltreatment are the most important risk factors for psychopathology and learning difficulties at the population level (Altarac and Saroha, 2007; Belsky, 2007). In a single individual, it is often difficult to establish a causative role for these psychosocial influences, as not all individuals subject to a risk present the outcome (Caspi et al., 2003; Nobile et al., 2010).
Even if the occurrence of a specific etiology were an infrequent event, underdiagnosis has important consequences, as the individual is deprived of proper health and educational counseling. This is especially important in the era of response to intervention (RTI). Learning difficulties are increasingly being handled by teachers in the schools, using the RTI approach, without referral to specialists (Hale et al., 2010). In the RTI approach, it may take several semesters until teachers recognize that a kid presents more severe and stable difficulties that do not respond to the interventions. Furthermore, they may be associated with a higher probability of a genetic etiology. Referrals for specialized diagnosis and care may be delayed for these individuals.
We argue that teachers must be aware of the possibility that children with learning difficulties are a group at risk for several medical, neurological and psychiatric conditions. Our results suggest that math learning difficulties may function as a kind of red-flag, pointing to possible genetic etiologies. Some red-flags for genetic syndromes may be minor, albeit observable by teachers: short or tall stature, congenital malformations, hypotonia, poor motor coordination, anomalous handedness, history of developmental delay, etc. “Funny face” is an important red-flag. These children have no facial malformations but, rather, small, subtle dysmorphisms such as a low nasal bridge, markedly upslanting or downslanting palpebral fissures, small or prominent chin, low set ears, etc. (Huang et al., 2010). Normal people may have one or two such dysmorphisms, but they are not enough to characterize a “funny face.” Minor motor impairments may also hint at a neurological etiology (Daigneault and Braun, 2002; Batstra et al., 2003). Adoption is another important risk factor for developmental disorders of genetic or environmental etiology (Altarac and Saroha, 2007; Tenenbaum et al., 2011). However, it is important not to forget that most children with math learning difficulties will have a perfectly normal constitution and no genetic syndrome.
Finally, our research design has no power to establish a definite role for ANS over executive function impairments in the etiology of the girl's math learning difficulties. Results indicate however, that specific mechanisms, such as ANS and/or executive function impairments vs. phonological and/or visuospatial/visuoconstructional processing, may play a role in specific individuals.
Again, in a given individual, it may difficult to reliably identify which cognitive mechanisms underlie the difficulties. Our own experience has been that, in accordance with the multiple deficits hypothesis, specific and general cognitive impairments interact in complex ways (Haase et al., 2014; Júlio-Costa et al., 2015; Gomides et al., 2018). Identification of the putative mechanisms is relevant for the planning of more efficient interventions (Gomides et al., 2018). Anyway, alone or interacting with general cognitive impairments, ANS may play a role in math learning difficulties. Future research should address the specific mechanisms and crucial developmental period(s) of the ANS involvement with math learning, as well as intervention strategies.
This investigation of a girl with 22q11.2DS (LCR22-4 to LCR22-5), allows us to raise the following points: (a) specific genetic alterations, such as atypical 22q11.2DS, may be related to math learning difficulties in individuals with normal intelligence and slight phenotypic traits that would remain otherwise unrecognized; (b) math learning difficulties may be severe and persistent in these cases, involving both non-symbolic and symbolic numerical magnitude processing, and eventually be associated with executive dysfunctions; (c) although the microdeleted regions in typical and atypical cases of 22q11.2 are non-overlapping, their phenotypic traits may be broadly shared, suggesting long-range interactions and complexity of genotype-phenotype associations (Zeitz et al., 2013); (d) numerical-cognitive impairments were dissociated from spared visuospatial abilities, suggesting heterogeneity of neurogenetic underpinnings. Further studies have the challenge of showing more evidence for these issues.
Author Contributions
VH and MC delineated the study; LO, AJ-C, and VH conducted the neuropsychological evaluation; FS and MC conducted the genetic analyses. All authors contributed in analysing the results and writing the paper. All authors read the final version of the paper and agree with the content of the manuscript.
Funding
This study was supported by grants from the Fundação de Amparo à Pesquisa do Estado de Minas Gerais (FAPEMIG, APQ-02755-SHA, APQ-03289-10, APQ-02953-14, APQ-03642-12). VH is supported by a CNPq fellowship (409624/2006-3, 308157/2011-7, 308267/2014-1) and Programa de Capacitação em Neuropsicologia do Desenvolvimento (FEAPAEs-MG, APAE-BH, PRONAS-Ministério da Saúde, Brasil). MC is supported by a CNPq fellowship (312068/2015-8). LO, AJ-C, and FS are supported by PhD fellowships from the Coordenação de Aperfeiçoamento de Pessoal de Ensino Superior (CAPES).
Conflict of Interest Statement
The authors declare that the research was conducted in the absence of any commercial or financial relationships that could be construed as a potential conflict of interest.
Acknowledgments
The authors thank to the children, their parents, and also to the principals of the schools for taking part in this research. We thank to Mr. Peter Laspina, from ViaMundi Idiomas e Traduções for reviewing this manuscript.
References
Altarac, M., and Saroha, E. (2007). Lifetime prevalence of learning disability among US children. Pediatrics 119(Suppl. 1), S77–S83. doi: 10.1542/peds.2006-2089L
Angelini, A. L., Alves, I. C. B., Custódio, E. M., Duarte, W. F., and Duarte, J. L. M. (1999). Matrizes Progressivas Coloridas de Raven: Escala Especial. Manual. São Paulo: CETEPP.
Anobile, G., Castaldi, E., Turi, M., Tinelli, F., and Burr, D. C. (2016). Numerosity but not texture-density discrimination correlates with math ability in children. Dev. Psychol. 52:1206. doi: 10.1037/dev0000155
Antshel, K. M., Fremont, W., and Kates, W. R. (2008). The neurocognitive phenotype in velo-cardio-facial syndrome: a developmental perspective. Dev. Disabil. Res. Rev. 14, 43–51. doi: 10.1002/ddrr.7
Asbury, K., and Plomin, R. (2013). G is for Genes: The Impact of Genetics on Education and Achievement. Chichester, UK: Wiley Blackwell. doi: 10.1002/9781118482766
Ashcraft, M. H., and Krause, J. A. (2007). Working memory, math performance, and math anxiety. Psychon. Bull. Rev. 14, 243–248. doi: 10.3758/BF03194059
Attout, L., Noël, M. P., Vossius, L., and Rousselle, L. (2017). Evidence of the impact of visuo-spatial processing on magnitude representation in 22q11. 2 microdeletion syndrome. Neuropsychologia 99, 296–305. doi: 10.1016/j.neuropsychologia.2017.03.023
Barnea-Goraly, N., Eliez, S., Menon, V., Bammer, R., and Reiss, A. L. (2005). Arithmetic ability and parietal alterations: a diffusion tensor imaging study in velocardiofacial syndrome. Cogn. Brain Res. 25, 735–740. doi: 10.1016/j.cogbrainres.2005.09.013
Barrouillet, P., Camos, V., Perruchet, P., and Seron, X. (2004). ADAPT: A developmental, asemantic, and procedural model for transcoding from verbal to Arabic numerals. Psychol. Rev. 111, 368–394. doi: 10.1037/0033-295X.111.2.368
Bassett, A. S., and Chow, E. W. (2008). Schizophrenia and 22q11.2 deletion syndrome. Curr. Psychiatry Re. 10:148. doi: 10.1007/s11920-008-0026-1
Batstra, L., Neeleman, J., and Hadders-Algra, M. (2003). The neurology of learning and behavioural problems in pre-adolescent children. Acta Psychiatr. Scand. 108, 92–100. doi: 10.1034/j.1600-0447.2003.00127.x
Bearden, C. E., Woodin, M. F., Wang, P. P., Moss, E., McDonald-McGinn, D., Zackai, E., et al. (2001). The neurocognitive phenotype of the 22q11. 2 deletion syndrome: selective deficit in visual-spatial memory. J. Clini. Exp. Neuropsychol. 23, 447–464. doi: 10.1076/jcen.23.4.447.1228
Beaujard, M. P., Chantot, S., Dubois, M., Keren, B., Carpentier, W., Mabboux, P., et al. (2009). Atypical deletion of 22q11.2: detection using the FISH TBX1 probe and molecular characterization with high-density SNP arrays. Eur. J. Med. Genet. Year 52, 321–327. doi: 10.1016/j.ejmg.2009.05.010
Belsky, J. (2007). Experience in childhood and the development of reproductive strategies. Acta Psychol. Sin. 39, 454–468.
Ben-Shachar, S., Ou, Z., Shaw, C. A., Belmont, J. W., Patel, M. S., Hummel, M., Amato, S., et al. (2008). 22q11.2 distal deletion: a recurrent genomic disorder distinct from DiGeorge syndrome and velocardiofacial syndrome. Am. J. Hum. Genet. 82, 214–221. doi: 10.1016/j.ajhg.2007.09.014
Billard, C., Fluss, J., Ducot, B., Warszawski, J., Ecalle, J., Magnan, A., et al. (2008). Study of causal factors of reading impairment in a sample of 1062 7 to 8-year-old children. Arch. Pediatrie 15, 1058–1067. doi: 10.1016/j.arcped.2008.02.020
Bishop, D. V. (2009). Genes, cognition, and communication. Ann. N. Y. Acad. Sci. 1156, 1–18. doi: 10.1111/j.1749-6632.2009.04419.x
Blackman, J. A., and Gurka, M. J. (2007). Developmental and behavioral comorbidities of asthma in children. J. Dev. Behav. Pediatr. 28, 92–99. doi: 10.1097/01.DBP.0000267557.80834.e5
Brankaer, C., Ghesquière, P., De Wel, A., Swillen, A., and De Smedt, B. (2017). Numerical magnitude processing impairments in genetic syndromes: a cross-syndrome comparison of Turner and 22q11. 2 deletion syndromes. Dev. Sci. 20:e12458. doi: 10.1111/desc.12458
Bruandet, M., Molko, N., Cohen, L., and Dehaene, S. (2004). A cognitive characterization of dyscalculia in Turner syndrome. Neuropsychologia 42, 288–298. doi: 10.1016/j.neuropsychologia.2003.08.007
Bruce, S., Hannula-Jouppi, K., Puoskari, M., Fransson, I., Simola, K. O., Lipsanen-Nyman, M., et al. (2010). Submicroscopic genomic alterations in Silver–Russell syndrome and Silver–Russell-like patients. J. Med. Genet. 47, 816–822. doi: 10.1136/jmg.2009.069427
Bull, R., and Lee, K. (2014). Executive functioning and mathematics achievement. Child Dev. Perspect. 8, 36–41. doi: 10.1111/cdep.12059
Burnside, R. D. (2015). 22q11. 21 deletion syndromes: a review of proximal, central, and distal deletions and their associated features. Cytogenet. Genome Res. 146, 89–99. doi: 10.1159/000438708
Camos, V. (2008). Low working memory capacity impedes both efficiency and learning of number transcoding in children. J. Exp. Child Psychol. 99, 37–57. doi: 10.1016/j.jecp.2007.06.006
Canavese, C., Rigardetto, R., Viano, V., Vittorini, R., Bassi, B., Pieri, I., et al. (2007). Are dyslexia and dyscalculia associated with Rolandic epilepsy? A short report on ten Italian patients. Epileptic Disord. 9, 432–436. doi: 10.1684/epd.2007.0138
Carvalho, M. R. S., Vianna, G., Oliveira, L. F. S., Aguiar, M. J. B., Zen, P., and Haase, V. G. (2014). Are 22q11.2 distal deletions associated with math difficulties? Am. J. Med. Genet. A 164, 2256–2262. doi: 10.1002/ajmg.a.36649
Caspi, A., Sugden, K., Moffitt, T. E., Taylor, A., Craig, I. W., Harrington, H., et al. (2003). Influence of life stress on depression: moderation by a polymorphism in the 5-HTT gene. Science 301, 386–389. doi: 10.1126/science.1083968
Charchat-Fichman, H., and Oliveira, R. M. (2009). Performance of 119 Brazilian children on Stroop paradigm: victoria version. Arq. Neuropsiquiatr. 67, 445–449. doi: 10.1590/S0004-282X2009000300014
Chen, Q., and Li, J. (2014). Association between individual differences in non-symbolic number acuity and math performance: a meta-analysis. Acta Psychol. 148, 163–172. doi: 10.1016/j.actpsy.2014.01.016
Clayton, S., and Gilmore, C. (2015). Inhibition in dot comparison tasks. ZDM 47, 759–770. doi: 10.1007/s11858-014-0655-2
Cohen, D., Pichard, N., Tordjman, S., Baumann, C., Burglen, L., Excoffier, E., et al. (2005). Specific genetic disorders and autism: clinical contribution towards their identification. J. Autism Dev. Disord. 35, 103–116. doi: 10.1007/s10803-004-1038-2
Costa, A. J., Lopes-Silva, J. G., Pinheiro-Chagas, P., Krinzinger, H., Lonnemann, J., Willmes, K., et al. (2011). A hand full of numbers: a role for offloading in arithmetics learning. Front. Psychol. 2:368. doi: 10.3389/fpsyg.2011.00368
Crawford, J. R., and Garthwaite, P. H. (2002). Investigation of the single case in neuropsychology: Confidence limits on the abnormality of test scores and test score differences. Neuropsychologia 40, 1196–1208. doi: 10.1016/S0028-3932(01)00224-X
Crawford, J. R., Garthwaite, P. H., and Porter, S. (2010). Point and interval estimates of effect sizes for the case controls design in neuropsychology: Rationale, methods, implementations, and proposed reporting standards. Cogn. Neuropsychol. 27, 245–260. doi: 10.1080/02643294.2010.513967
Crawford, J. R., and Howell, D. C. (1998). Comparing an individual's test score against norms derived from small samples. Clin. Neuropsychol. 12, 482–486.
Cuvellier, J. C., Pandit, F., Casalis, S., Lemaître, M. P., Cuisset, J. M., Platof, A., et al. (2004). Analyse d'une population de 100 enfants adressés pour troubles d'apprentissage scolaire. Archives de Pédiatrie 11, 201–206. doi: 10.1016/j.arcped.2003.12.004
Daigneault, S., and Braun, C. M. (2002). Pure severe dyslexia after a perinatal focal lesion: evidence of a specific module for acquisition of reading. J. Dev. & Behav. Pediatr. 23, 256–265. doi: 10.1097/00004703-200208000-00011
De Smedt, B., and Gilmore, C. K. (2011). Defective number module or impaired access? Numerical magnitude processing in first graders with mathematical difficulties. J. Exp. Child Psychol. 108, 278–292. doi: 10.1016/j.jecp.2010.09.003
De Smedt, B., Noël, M. P., Gilmore, C., and Ansari, D. (2013). How do symbolic and non-symbolic numerical magnitude processing skills relate to individual differences in children's mathematical skills? A review of evidence from brain and behavior. Trends Neurosci. Educ. 2, 48–55. doi: 10.1016/j.tine.2013.06.001
De Smedt, B., Reynvoet, B., Swillen, A., Verschaffel, L., Boets, B., and Ghesquière, P. (2009). Basic number processing and difficulties in single-digit arithmetic: evidence from velo-cardio-facial syndrome. Cortex 45, 177–188. doi: 10.1016/j.cortex.2007.06.003
De Smedt, B., Swillen, A., Devriendt, K., Fryns, J. P., Verschaffel, L., Boets, B., et al. (2008). Cognitive correlates of mathematical disabilities in children with velo-cardio-facial syndrome. Genet. Couns. 19, 71–94.
Debbané, M., Glaser, B., and Eliez, S. (2008). Encoding and retrieval processes in velo-cardio-facial syndrome (VCFS). Neuropsychology 22:226. doi: 10.1037/0894-4105.22.2.226
Dehaene, S. (1997). The Number Sense: How the Mind Creates Mathematics. New York, NY: Oxford University Press.
Dehaene, S. (2007). “Symbols and quantities in parietal cortex: elements of a mathematical theory of number representation and manipulation,” in Sensoriomotor Foundations of Higher Cognition - Attention and Performance XXII, eds P. Haggard, Y. Rossetti, and M. Kawato (Cambridge: Harvard University Press), 527–574.
Dehaene, S., Izard, I., and Piazza, M. (2005). Control Over Non-Numerical Parameters in Numerosity Experiments. Available online at: www.unicog.org/docs/DocumentationDotsGeneration.doc
Denckla, M. B. (1997). “The neurobehavioral examination in children,” in Behavioral Neurology and Neuropsychology, eds T. E. Feinberg and M. J. Farah (New York, NY: McGraw-Hill), 721–728.
Denckla, M. B. (2003). ADHD: topic update. Brain Dev. 25, 383–389. doi: 10.1016/S0387-7604(03)00057-3
Elliott, J. G., and Grigorenko, E. L. (2014). The Dyslexia Debate (No. 14). London: Cambridge University Press.
Espe, S. (2018). MalaCards: the human disease database. J. Med. Library Assoc. 106, 140. doi: 10.5195/JMLA.2018.253
Fagerberg, C. R., Graakjaer, J., Heinl, U. D., Ousager, L. B., Dreyer, I., Kirchhoff, M., et al. (2012). Heart defects and other features of the 22q11 distal deletion syndrome. Eur. J. Med. Genet. 56, 98–107. doi: 10.1016/j.ejmg.2012.09.009
Fazio, L. K., Bailey, D. H., Thompson, C. A., and Siegler, R. S. (2014). Relations of different types of numerical magnitude representations to each other and to mathematics achievement. J. Exp. Child Psychol. 123, 53–72. doi: 10.1016/j.jecp.2014.01.013
Ferreira, F. O., Wood, G., Pinheiro-Chagas, P., Lonnemann, J., Krinzinger, H., Willmes, K., et al. (2012). Explaining school mathematics performance from symbolic and nonsymbolic magnitude processing: similarities and differences between typical and low-achieving children. Psychol. Neurosci. 5, 37–46. doi: 10.3922/j.psns.2012.1.06
Figueiredo, V. L. M. (2002). WISC-III: Escala de Inteligência Wechsler para Crianças. Manual Adaptação e Padronização Brasileira. São Paulo: Casa do Psicólogo.
Figueiredo, V. L. M., and Nascimento, E. (2007). Desempenhos nas duas tarefas do subteste dígitos do WISC-III e do WAIS-III. Psicologia 23, 313–318. doi: 10.1590/S0102-37722007000300010
Geary, D. C., Hoard, M. K., Byrd-Craven, J., and DeSoto, M. C. (2004). Strategy choices in simple and complex addition: Contributions of working memory and counting knowledge for children with mathematical disability. J. Exp. Child Psychol. 88, 121–151. doi: 10.1016/j.jecp.2004.03.002
Genome Reference Consortium (2018). Available online at: https://www.ncbi.nlm.nih.gov/grc/human/data.
Gerdes, M., Solot, C., Wang, P. P., McDonald-McGinn, D. M., and Zackai, E. H. (2001). Taking advantage of early diagnosis: preschool children with the 22q11. 2 deletion. Genet. Med. 3:40. doi: 10.1097/00125817-200101000-00009
Gomides, M. R. A., Martins, G. Z., Starling-Alves, I., Júlio-Costa, A., Jaeger, A., and Haase, V. G. (2018). Heterogeneity of math difficulties and its implications for interventions in multiplication skills. Dement. Neuropsychol. 12, 256–263. doi: 10.1590/1980-57642018dn12-030006
Gothelf, D., Eliez, S., Thompson, T., Hinard, C., Penniman, L., Feinstein, C., et al. (2005). COMT genotype predicts longitudinal cognitive decline and psychosis in 22q11. 2 deletion syndrome. Nat. Neurosci. 8:1500. doi: 10.1038/nn1572
Gothelf, D., Frisch, A., Michaelovsky, E., Weizman, A., and Shprintzen, R. J. (2009). Velocardiofacial syndrome. J. Ment. Health Res. Intellect. Disabil. 2, 149–167. doi: 10.1080/19315860902756136
Green, T., Gothelf, D., Glaser, B., Debbane, M., Frisch, A., Kotler, M., et al. (2009). Psychiatric disorders and intellectual functioning throughout development in velocardiofacial (22q11. 2 deletion) syndrome. J. Am. Acad. Child Adolesc. Psychiatry 48, 1060–1068. doi: 10.1097/CHI.0b013e3181b76683
Haase, V. G., Júlio-Costa, A., Lopes-Silva, J. B., Starling-Alves, I., Antunes, A., Pinheiro-Chagas, P., et al. (2014). Contributions from specific and general factors to unique deficits: two cases of mathematics learning difficulties. Front. Psychol. 5:102. doi: 10.3389/fpsyg.2014.00102
Halberda, J., Mazzocco, M. M. M., and Feigenson, L. (2008). Individual differences in non-verbal number acuity correlate with maths achievement. Nature 455, 665–668. doi: 10.1038/nature07246
Hale, J., Alfonso, V., Berninger, V., Bracken, B., Christo, C., Clark, E., et al. (2010). Critical issues in response-to-intervention, comprehensive evaluation, and specific learning disabilities identification and intervention: an expert white paper consensus. Learn. Disabil. Q. 33, 223–236. doi: 10.1177/073194871003300310
Hannonen, R., Komulainen, J., Eklund, K., Tolvanen, A., Riikonen, R., and Ahonen, T. (2010). Verbal and academic skills in children with early-onset type 1 diabetes. Dev. Med. Child Neurol. 52, e143–e147. doi: 10.1111/j.1469-8749.2010.03648.x
Hembree, R. (1990). The nature, effects, and relief of mathematics anxiety. J. Res. Math. Educ. 21, 33–46.
Huang, C. J., Chiu, H. J., Lan, T. H., Wang, H. F., Kuo, S. W., Chen, S. F., et al. (2010). Significance of morphological features in schizophrenia of a Chinese population. J. Psychiatr. Res. 44, 63–68. doi: 10.1016/j.jpsychires.2009.06.004
Jacobson, C., Shearer, J., Habel, A., Kane, F., Tsakanikos, E., and Kravariti, E. (2010). Core neuropsychological characteristics of children and adolescents with 22q11. 2 deletion. J. Intell. Disabil. Res. 54, 701–713. doi: 10.1111/j.1365-2788.2010.01298.x
Jacobson, J. L., Dodge, N. C., Burden, M. J., Klorman, R., and Jacobson, S. W. (2011). Number processing in adolescents with prenatal alcohol exposure and ADHD: differences in the neurobehavioral phenotype. Alcoholism 35, 431–442. doi: 10.1111/j.1530-0277.2010.01360.x
Johnson, M. H. (2012). Executive function and developmental disorders: the flip side of the coins. Trends Cogn. Sci. 16, 454–457. doi: 10.1016/j.tics.2012.07.001
Júlio-Costa, A., Starling-Alves, I., Lopes-Silva, J. B., Wood, G., and Haase, V. G. (2015). Stable measures of number sense accuracy in math learning disability: Is it time to proceed from basic science to clinical application? PsyCh J. 4, 218–225. doi: 10.1002/pchj.114
Karagiannakis, G., Baccaglini-Frank, A., and Papadatos, Y. (2014). Mathematical learning difficulties subtypes classification. Front. Hum. Neurosci. 8:57. doi: 10.3389/fnhum.2014.00057
Karayiorgou, M., Simon, T. J., and Gogos, J. A. (2010). 22q11. 2 microdeletions: linking DNA structural variation to brain dysfunction and schizophrenia. Nat. Rev. Neurosci. 11:402. doi: 10.1038/nrn2841
Klein, E., Nuerk, H. C., Wood, G., Knops, A., and Willmes, K. (2009). The exact vs. approximate distinction in numerical cognition may not be exact, but only approximate: How different processes work together in multi-digit addition. Brain Cogn. 69, 369–381. doi: 10.1016/j.bandc.2008.08.031
Krajcsi, A., Lukács, A., Ignács, J., Racsmány, M., and Pléh, C. (2009). Numerical abilities in Williams syndrome: dissociating the analogue magnitude system and verbal retrieval. J. Clin. Exp. Neuropsychol. 31, 439–446. doi: 10.1080/13803390802244126
Lacerda, S. S. (2012). Características Psicométricas do Teste de Aprendizagem Auditivo-Verbal de Rey e do Teste de Aprendizagem Visual de Desenhos de Rey para a população brasileira, Unpublished doctoral thesis. Universidade de São Paulo, São Paulo.
Lajiness-O'Neill, R., Beaulieu, I., Asamoah, A., Titus, J. B., Bawle, E., Ahmad, S., et al. (2006). The neuropsychological phenotype of velocardiofacial syndrome (VCFS): Relationship to psychopathology. Arch. Clin. Neuropsychol. 21, 175–184.
Landerl, K., Bevan, A., and Butterworth, B. (2004). Developmental dyscalculia and basic numerical capacities: a study of 8–9-year-old students. Cognition 93, 99–125. doi: 10.1016/j.cognition.2003.11.004
Lee, J. (2009). Universals and specifics of math self-concept, math self-efficacy, and math anxiety across 41 PISA 2003 participating countries. Learn. Individ. Differ. 19, 355–365. doi: 10.1016/j.lindif.2008.10.009
Leibovich, T., and Henik, A. (2014). Comparing performance in discrete and continuous comparison tasks. Q. J. Exp. Psychol. 67, 899–917. doi: 10.1080/17470218.2013.837940
Leibovich, T., Katzin, N., Harel, M., and Henik, A. (2017). From “sense of number” to “sense of magnitude”: The role of continuous magnitudes in numerical cognition. Behav. Brain Sci. 40:e164. doi: 10.1017/S0140525X16000960
Levin, H. S., Scheller, J., Rickard, T., Grafman, J., Martinkowski, K., Winslow, M., et al. (1996). Dyscalculia and dyslexia after right hemisphere injury in infancy. Arch. Neurol. 53, 88–96. doi: 10.1001/archneur.1996.00550010108024
Libertus, M. E., Feigenson, L., Halberda, J., and Landau, B. (2014). Understanding the mapping between numerical approximation and number words: Evidence from Williams syndrome and typical development. Dev. Sci. 17, 905–919. doi: 10.1111/desc.12154
Lindgren, V., McRae, A., Dineen, R., Saulsberry, A., Hoganson, G., and Schrift, M. (2015). Behavioral abnormalities are common and severe in patients with distal 22q11. 2 microdeletions and microduplications. Mol. Genet. Genom. Med. 3, 346–353. doi: 10.1002/mgg3.146
Lonnemann, J., Linkersdörfer, J., Heselhaus, V., Hasselhorn, M., and Lindberg, S. (2011). Relations between balancing and arithmetic skills in children–Evidence of cerebellar involvement? J. Neurolinguistics 24, 592–601. doi: 10.1016/j.jneuroling.2011.02.005
Lopes-Silva, J. B., Moura, R., Júlio-Costa, A., Geraldi Haase, V., and Wood, G. (2014). Phonemic awareness as a pathway to number transcoding. Front. Psychol. 5:13. doi: 10.3389/fpsyg.2014.00013
Lopes-Silva, J. B., Moura, R., Júlio-Costa, A., Wood, G., Salles, J. F., and Haase, V. G. (2016). What is specific and what is shared between numbers and words? Front. Psychol. 7:22. doi: 10.3389/fpsyg.2016.00022
Lyons, I. M., Price, G. R., Vaessen, A., Blomert, L., and Ansari, D. (2014). Numerical predictors of arithmetic success in grades 1–6. Dev. Sci. 17, 714–726. doi: 10.1111/desc.12152
Ma, X. (1999). A meta-analysis of the relationship between anxiety toward mathematics and achievement in mathematics. J. Res. Math. Educ. 30, 520–540.
Maloney, E. A., Risko, E. F., Preston, F., Ansari, D., and Fugelsang, J. (2010). Challenging the reliability and validity of cognitive measures: the case of the numerical distance effect. Acta Psychol. 134, 154–161. doi: 10.1016/j.actpsy.2010.01.006
Mazzocco, M. M. (2001). Math learning disability and math LD subtypes: evidence from studies of Turner syndrome, fragile X syndrome, and neurofibromatosis type 1. J. Learn. Disabil. 34, 520–533. doi: 10.1177/002221940103400605
Mazzocco, M. M., Feigenson, L., and Halberda, J. (2011). Impaired acuity of the approximate number system underlies mathematical learning disability (dyscalculia). Child Dev. 82, 1224–1237. doi: 10.1111/j.1467-8624.2011.01608.x
Menon, V., Rivera, S. M., White, C. D., Glover, G. H., and Reiss, A. L. (2000). Dissociating prefrontal and parietal cortex activation during arithmetic processing. Neuroimage 12, 357–365. doi: 10.1006/nimg.2000.0613
Merkley, R., Thompson, J., and Scerif, G. (2016). Of huge mice and tiny elephants: exploring the relationship between inhibitory processes and preschool math skills. Front. Psychol. 6:1903. doi: 10.3389/fpsyg.2015.01903
Mikhail, F. M., Burnside, R. D., Rush, B., Ibrahim, J., Godshalk, R., Rutledge, S. L., et al. (2014). The recurrent distal 22q11. 2 microdeletions are often de novo and do not represent a single clinical entity: a proposed categorization system. Genet. Med. 16:92. doi: 10.1038/gim.2013.79
Mikhail, F. M., Descartes, M., Piotrowski, A., Andersson, R., Diaz de Ståhl, T., Komorowski, J., et al. (2007). A previously unrecognized microdeletion syndrome on chromosome 22 band q11.2 encompassing the BCR gene. Am. J. Med. Genet. 143A, 2178–2184. doi: 10.1002/ajmg.a.31882
Moberg, P. J., Richman, M. J., Roalf, D. R., Morse, C. L., Graefe, A. C., Brennan, L., et al. (2018). Neurocognitive functioning in patients with 22q11. 2 deletion syndrome: a meta-analytic review. Behav. Genet. 48, 1–12. doi: 10.1007/s10519-018-9903-5
Molck, M. C., Vieira, T. P., Sgardioli, I. C., Simioni, M., Dos Santos, A. P., Souza, J., et al. (2013). Atypical copy number abnormalities in 22q11. 2 region: Report of three cases. Eur. J. Med. Genet. 56, 515–520. doi: 10.1016/j.ejmg.2013.07.002
Moura, R., Lopes-Silva, J. B., Vieira, L. R., Paiva, G. M., de Almeida Prado, A. C., Wood, G., et al. (2015). From “Five” to 5 for 5 minutes: arabic number transcoding as a short, specific, and sensitive screening tool for mathematics learning difficulties. Arch. Clin. Neuropsychol. 30, 88–98. doi: 10.1093/arclin/acu071
Moura, R. J., Wood, G., Pinheiro-Chagas, P., Lonnemann, J., Krinzinger, H., Willmes, K., et al. (2013). Transcoding abilities in typical and atypical mathematics achievers: the role of working memory, procedural and lexical competencies. J. Exp. Child Psychol. 116, 707–727. doi: 10.1016/j.jecp.2013.07.008
Newbern, J., Zhong, J., Wickramasinghe, R. S., Li, X., Wu, Y., Samuels, I., et al. (2008). Mouse and human phenotypes indicate a critical conserved role for ERK2 signaling in neural crest development. Proc. Natl. Acad. Sci. U.S.A. 105, 17115–17120. doi: 10.1073/pnas.0805239105
Nieder, A., and Dehaene, S. (2009). Representation of number in the brain. Annu. Rev. Neurosci. 32, 185–208. doi: 10.1146/annurev.neuro.051508.135550
Nobile, M., Rusconi, M., Bellina, M., Marino, C., Giorda, R., Carlet, O., et al. (2010). COMT Val158Met polymorphism and socioeconomic status interact to predict attention deficit/hyperactivity problems in children aged 10–14. Eur. Child Adolesc. Psychiatry 19, 549–557. doi: 10.1007/s00787-009-0080-1
Oliveira, L. F., Santos, A. O., Vianna, G. S., Di Ninno, C. Q., Giacheti, C. M., Carvalho, M. R., et al. (2014). Impaired acuity of the approximate number system in 22q11. 2 microdeletion syndrome. Psychol. Neurosci. 7:151. doi: 10.3922/j.psns.2014.02.04
Oliveira, M., Rigoni, M., Andretta, I., and Moraes, J. F. (2004). Validação do teste figuras complexas de Rey para a população brasileira. Avaliação Psicológica 3, 33–38.
Oliveira-Ferreira, F., Costa, D. S., Micheli, L. R., Oliveira, L. F. S., Pinheiro-Chagas, P., and Haase, V. G. (2012). School Achievement Test: normative data for a representatitive sample of elementar school children. Psychol. Neurosci. 5, 157–164. doi: 10.3922/j.psns.2012.2.05
Pennington, B. F. (2006). From single to multiple deficit models of developmental disorders. Cognition 101, 385–413. doi: 10.1016/j.cognition.2006.04.008
Piazza, M., Facoetti, A., Trussardi, A. N., Berteletti, I., Conte, S., Lucangeli, D., et al. (2010). Developmental trajectory of number acuity reveals a severe impairment in developmental dyscalculia. Cognition 116, 33–41. doi: 10.1016/j.cognition.2010.03.012
Piazza, M., Izard, V., Pinel, P., LeBihan, D., and Dehaene, S. (2004). Tuning curves for approximate numerosity in the human parietal cortex. Neuron 44, 547–555. doi: 10.1016/j.neuron.2004.10.014
Pinheiro-Chagas, P., Wood, G., Knops, A., Krinzinger, H., Lonnemann, J., Starling-Alves, I., et al. (2014). In how many ways is the approximate number system associated with exact calculation? PLoS ONE 9:e111155. doi: 10.1371/journal.pone.0111155
Poole, J. L., Burtner, P. A., Torres, T. A., McMullen, C. K., Markham, A., Marcum, M. L., et al. (2005). Measuring dexterity in children using the Nine-hole Peg Test. J. Hand Ther. 18, 348–351. doi: 10.1197/j.jht.2005.04.003
Prado, J. (2018). “The interplay between learning arithmetic and learning to read: insights from developmental cognitive neuroscience,” in Heterogeneity of Function in Numerical Cognition, eds A. Henik and W. Fias (Cambridge: Academic Press), 27–49.
Price, G. R., Palmer, D., Battista, C., and Ansari, D. (2012). Nonsymbolic numerical magnitude comparison: Reliability and validity of different task variants and outcome measures, and their relationship to arithmetic achievement in adults. Acta Psychol. 140, 50–57. doi: 10.1016/j.actpsy.2012.02.008
Raghubar, K. P., Barnes, M. A., and Hecht, S. A. (2010). Working memory and mathematics: a review of developmental, individual difference, and cognitive approaches. Learn. Individ. Differ. 20, 110–122. doi: 10.1016/j.lindif.2009.10.005
Robin, N. H., and Shprintzen, R. J. (2005). Defining the clinical spectrum of deletion 22q11.2. J. Pediatr. 147, 90–96. doi: 10.1016/j.jpeds.2005.03.007
Rocha, M. M., Rescorla, L. A., Emerich, D. R., Silvares, E. F. M., Borsa, J. C., Araújo, L. G. S., et al. (2012). Behavioural/emotional problems in Brazilian children: findings from parents' reports on the child behavior checklist. Epidemiol. Psychiatr. Sci. 22, 329–338. doi: 10.1017/S2045796012000637
Rodningen, O. K., Prescott, T., Eriksson, A. S., and Rosby, O. (2008). 1.4 Mb recurrent 22q11.2 distal deletion syndrome, two new cases expand the phenotype. Eur. J. Med. Genet. 51, 646–650. doi: 10.1016/j.ejmg.2008.07.007
Rousselle, L., and Noël, M. P. (2007). Basic numerical skills in children with mathematics learning disabilities: a comparison of symbolic vs non-symbolic number magnitude processing. Cognition 102, 361–395. doi: 10.1016/j.cognition.2006.01.005
Rubinsten, O., and Henik, A. (2009). Developmental dyscalculia: heterogeneity might not mean different mechanisms. Trends Cogn. Sci. 13, 92–99. doi: 10.1016/j.tics.2008.11.002
Rutter, M., Moffitt, T. E., and Caspi, A. (2006). Gene–environment interplay and psychopathology: multiple varieties but real effects. J. Child Psychol. Psychiatry 47, 226–261. doi: 10.1111/j.1469-7610.2005.01557.x
Saitta, S. C., McGrath, J. M., Mensch, H., Shaikh, T. H., Zackai, E. H., and Emanuel, B. S. (1999). A 22q11.2 deletion that excludes UFD1L and CDC45L in a patient with conotruncal and craniofacial defects. Am. J. Hum. Genet. 65, 562–566. doi: 10.1086/302514
Santos, F. H., and Bueno, O. F. A. (2003). Validation of the Brazilian children's test of pseudoword repetition in Portuguese speakers aged 4 to 10 years. Braz. J. Med. Biol. Res. 36, 1633–1547. doi: 10.1590/S0100-879X2003001100012
Santos, F. H., Mello, C. B., Bueno, O. F. A., and Dellatolas, G. (2005). Cross-cultural differences for three visual memory tasks in Brazilian children. Percept. Mot. Skills 101, 421–433. doi: 10.2466/pms.101.2.421-433
Schneider, M., Beeres, K., Coban, L., Merz, S., Susan Schmidt, S., Stricker, J., et al. (2017). Associations of non-symbolic and symbolic numerical magnitude processing with mathematical competence: a meta-analysis. Dev. Sci. 20:e12372. doi: 10.1111/desc.12372
Schoch, K., Harrell, W., Hooper, S. R., Ip, E. H., Saldana, S., Kwapil, T. R., et al. (2014). Applicability of the nonverbal learning disability paradigm for children with 22q11. 2 deletion syndrome. J. Learn. Disabil. 47, 153–166. doi: 10.1177/0022219412443556
Shalev, R. S., and Gross-Tsur, V. (1993). Developmental dyscalculia and medical assessment. J. Learn. Disabil. 26, 134–137. doi: 10.1177/002221949302600206
Siegler, R. S., and Braithwaite, D. W. (2017). Numerical development. Annu. Rev. Psychol. 68, 187–213. doi: 10.1146/annurev-psych-010416-044101
Simon, T. J. (2008). A new account of the neurocognitive foundations of impairments in space, time, and number processing in children with chromosome 22q11. 2 deletion syndrome. Dev. Disabil. Res. Rev. 14, 52–58. doi: 10.1002/ddrr.8
Simon, T. J., Bearden, C. E., Mc-Ginn, D. M., and Zackai, E. (2005a). Visuospatial and numerical cognitive deficits in children with chromosome 22q11. 2 deletion syndrome. Cortex 41, 145–155. doi: 10.1016/S0010-9452(08)70889-X
Simon, T. J., Ding, L., Bish, J. P., McDonald-McGinn, D. M., Zackai, E. H., and Gee, J. (2005b). Volumetric, connective, and morphologic changes in the brains of children with chromosome 22q11. 2 deletion syndrome: an integrative study. Neuroimage 25, 169–180. doi: 10.1016/j.neuroimage.2004.11.018
Simpson, N. H., Addis, L., Brandler, W. M., Slonims, V., Clark, A., Watson, J., et al. (2014). Increased prevalence of sex chromosome aneuploidies in specific language impairment and dyslexia. Dev. Med. Child Neurol. 56, 346–353. doi: 10.1111/dmcn.12294
Smets, K., Sasanguie, D., Szücs, D., and Reynvoet, B. (2015). The effect of different methods to construct non-symbolic stimuli in numerosity estimation and comparison. J. Cogn. Psychol. 27, 310–325. doi: 10.1080/20445911.2014.996568
Spineli-Silva, S., Bispo, L. M., Gil-da-Silva-Lopes, V. L., and Vieira, T. P. (2017). Distal deletion at 22q11. 2 as differential diagnosis in Craniofacial Microsomia: case report and literature review. Eur. J. Med. Genet. 61, 262–268. doi: 10.1016/j.ejmg.2017.12.013
Stankov, L., Lee, J., Luo, W., and Hogan, D. J. (2012). Confidence: A better predictor of academic achievement than self-efficacy, self-concept and anxiety? Learn. Individ. Differ. 22, 747–758. doi: 10.1016/j.lindif.2012.05.013
Stein, L. M. (1994). Teste de Desempenho Escolar: Manual para Aplicação e Interpretação. São Paulo: Casa do Psicólogo.
Swanson, H. L., and Sachse-Lee, C. (2001). Mathematical problem solving and working memory in children with learning disabilities: both executive and phonological processes are important. J. Exp. Child Psychol. 79, 294–321. doi: 10.1006/jecp.2000.2587
Swillen, A., Devriendt, K., Legius, E., Eyskens, B., Dumoulin, M., Gewillig, M., et al. (1997). Intelligence and psychosocial adjustment in velocardiofacial syndrome: a study of 37 children and adolescents with VCFS. J. Med. Genet. 34, 453–458. doi: 10.1136/jmg.34.6.453
Swillen, A., Vandeputte, L., Cracco, J., Maes, B., Ghesquière, P., Devriendt, K., et al. (1999). Neuropsychological, learning and psychosocial profile of primary school aged children with the velo-cardio-facial syndrome (22q11 deletion): evidence for a nonverbal learning disability? Child Neuropsychol. 5, 230–241. doi: 10.1076/0929-7049(199912)05:04;1-R;FT230
Szucs, D., Devine, A., Soltesz, F., Nobes, A., and Gabriel, F. (2013). Developmental dyscalculia is related to visuo-spatial memory and inhibition impairment. Cortex 49, 2674–2688. doi: 10.1016/j.cortex.2013.06.007
Tan, T. Y., Collins, A., James, P. A., McGillivray, G., Stark, Z., Gordon, C. T., et al. (2011). Phenotypic variability of distal 22q11.2 copy number abnormalities. Am. J. Med. Genet. Part A 155, 1623–1633. doi: 10.1002/ajmg.a.34051
Tenenbaum, A., Talia Dor, M. D., Yael Castiel, R. N., and Sapir, A. (2011). Fetal alcohol spectrum disorder in Israel: increased prevalence in an at-risk population. Religion 59:9.
Van Ijzendoorn, M. H., Juffer, F., and Poelhuis, C. W. K. (2005). Adoption and cognitive development: a meta-analytic comparison of adopted and nonadopted children's IQ and school performance. Psychol. Bull. 131:301. doi: 10.1037/0033-2909.131.2.301
Vandervert, L. (2017). The origin of mathematics and number sense in the cerebellum: with implications for finger counting and dyscalculia. Cerebell. Ataxias 4:12. doi: 10.1186/s40673-017-0070-x
Vaz, I. A., Cordeiro, P. M., de Macedo, E. C., and Lukasova, K. (2010). Memória de trabalho em crianças avaliada pela tarefa de Brown-Peterson. Pró-Fono 22, 95–100. doi: 10.1590/S0104-56872010000200005
Verhoeven, W., Egger, J., Brunner, H., and De Leeuw, N. (2011). A patient with a de novo distal 22q11.2 microdeletion and anxiety disorder. Am. J. Med. Genet A 155, 392–397. doi: 10.1002/ajmg.a.33802
Vicari, S., Mantovan, M., Addona, F., Costanzo, F., Verucci, L., and Menghini, D. (2011). Neuropsychological profile of Italian children and adolescents with 22q11.2 deletion syndrome with and without intellectual disability. Behav. Genet. 42, 287–298. doi: 10.1007/s10519-011-9499-5
Villalon-Reina, J., Jahanshad, N., Beaton, E., Toga, A. W., Thompson, P. M., and Simon, T. J. (2013). White matter microstructural abnormalities in girls with chromosome 22q11. 2 deletion syndrome, Fragile X or Turner syndrome as evidenced by diffusion tensor imaging. Neuroimage 81, 441–454. doi: 10.1016/j.neuroimage.2013.04.028
Wilson, A. J., and Dehaene, S. (2007). Number sense and developmental dyscalculia. Hum. Behav. Learn. Dev. Brain Atypical Dev. 2, 212–237.
Wong, T. T. Y., Ho, C. S. H., and Tang, J. (2014). Identification of children with mathematics learning disabilities (MLDs) using latent class growth analysis. Res. Dev. Disabil. 35, 2906–2920. doi: 10.1016/j.ridd.2014.07.015
Woodin, M., Wang, P. P., Aleman, D., Donald-McGinn, D., Zackai, E., and Moss, E. (2001). Neuropsychological profile of children and adolescents with the 22q11.2 microdeletion. Genet. Med. 3, 34–39. doi: 10.1097/00125817-200101000-00008
Xu, J., Fan, Y. S., and Siu, V. M. (2008). A child with features of Goldenhar syndrome and a novel 1.12 Mb deletion in 22q11.2 by cytogenetics and oligonucleotide array CGH: Is this a candidate region for the syndrome? Am. J. Med. Genet. A 146A, 1886–1889. doi: 10.1002/ajmg.a.32359
Zeitz, M. J., Lerner, P. P., Ay, F., Van Nostrand, E., Heidmann, J. D., Noble, W. S., et al. (2013). Implications of COMT long-range interactions on the phenotypic variability of 22q11. 2 deletion syndrome. Nucleus 4, 487–493. doi: 10.4161/nucl.27364
Keywords: math learning difficulties, developmental dyscalculia, 22q11.2DS (LCR22-4 to LCR22-5), cognitive phenotype, Weber fraction, approximate number system
Citation: Oliveira LFS, Júlio-Costa A, Santos FC, Carvalho MRS and Haase VG (2018) Numerical Processing Impairment in 22q11.2 (LCR22-4 to LCR22-5) Microdeletion: A Cognitive-Neuropsychological Case Study. Front. Psychol. 9:2193. doi: 10.3389/fpsyg.2018.02193
Received: 01 May 2018; Accepted: 23 October 2018;
Published: 21 November 2018.
Edited by:
Marcus Lindskog, Uppsala University, SwedenReviewed by:
Kevin Antshel, Syracuse University, United StatesAnn Dowker, University of Oxford, United Kingdom
Copyright © 2018 Oliveira, Júlio-Costa, Santos, Carvalho and Haase. This is an open-access article distributed under the terms of the Creative Commons Attribution License (CC BY). The use, distribution or reproduction in other forums is permitted, provided the original author(s) and the copyright owner(s) are credited and that the original publication in this journal is cited, in accordance with accepted academic practice. No use, distribution or reproduction is permitted which does not comply with these terms.
*Correspondence: Maria Raquel Santos Carvalho, mraquel@icb.ufmg.br