- 1Faculty of Sport Science, University of Extremadura, Cáceres, Spain
- 2Facultad de Educación, Universidad Autónoma de Chile, Talca, Chile
- 3Department of Physical Education, Sport and Human Movement, Autonomous University of Madrid, Madrid, Spain
- 4Faculty of Education, Psychology and Sport Sciences, University of Huelva, Huelva, Spain
- 5Faculty of Sports Sciences, Universidad Europea de Madrid, Madrid, Spain
- 6Grupo de Investigación Cultura, Educación y Sociedad, Universidad de la Costa, Barranquilla, Colombia
Background: Heart rate variability (HRV) has been considered as a measure of heart-brain interaction and autonomic modulation, and it is modified by cognitive and attentional tasks. In cognitive tasks, HRV was reduced in participants who achieved worse results. This could indicate the possibility of HRV predicting cognitive performance, but this association is still unclear in a high cognitive load sport such as chess.
Objective: To analyze modifications on HRV and subjective perception of stress, difficulty and complexity in different chess problem tasks.
Design: HRV was assessed at baseline. During the chess problems, HRV was also monitored, and immediately after chess problems the subjective stress, difficulty and complexity were also registered.
Methods: A total of 16 male chess players, age: 35.19 (13.44) and ELO: 1927.69 (167.78) were analyzed while six chess problem solving tasks with different level of difficulty were conducted (two low level, two medium level and two high level chess problems). Participants were classified according to their results into two groups: high performance or low performance.
Results: Friedman test showed a significant effect of tasks in HRV indexes and perceived difficulty, stress and complexity in both high and low performance groups. A decrease in HRV was observed in both groups when chess problems difficulty increased. In addition, HRV was significantly higher in the high performance group than in the low performance group during chess problems.
Conclusion: An increase in autonomic modulation was observed to meet the cognitive demands of the problems, being higher while the difficulty of the tasks increased. Non-linear HRV indexes seem to be more reactive to tasks difficulty, being an interesting and useful tool in chess training.
Introduction
The game of chess has been traditionally used for the study of basic cognitive processes (memory or problem solving) (Amidzic et al., 2006; Troubat et al., 2009) where the executive function plays an important role (Elkies and Stanley, 2003). Gobet and Simon (1998) suggested that expert chess players have a large database of chunks (Chase and Simon, 1973) stored in their long-term memory. This database can be used as working memory, increasing the processing capacities of chess players (Guida et al., 2012). This is supported by complex visual processing outside of conscious awareness (Kiesel et al., 2009). Moreover, skills as logic, intellectual capacity and mathematical problem-solving are required in chess players (Aciego et al., 2012; Kazemi et al., 2012; Bart, 2014; Lin et al., 2015; Mathy et al., 2016; Sala et al., 2017). Chess has been proposed as a useful tool to improve mathematical problem-solving abilities due to the cognitive processes involved in the game (Kazemi et al., 2012; Sala et al., 2015).
One of the most relevant functions of the prefrontal cortex is the decision-making. This process is extremely relevant in chess because players always have to plan and decide their next move (Koechlin and Hyafil, 2007). The prefrontal cortex is associated with the vagal function, which is easily measured by heart rate variability (HRV) (variation in the beat-to-beat interval) (Thayer et al., 2012). There is a dynamic balance between sympathetic and the parasympathetic nervous systems (autonomic modulation). Parasympathetic activity, which leads to an increment in the HRV, is frequent at rest and in relaxing situation. Sympathetic activity is related with stressful situations and leads to a reduction in the HRV (Shaffer et al., 2014).
HRV has been considered as a measure of heart-brain interaction (Shaffer et al., 2014), and it is also modified by cognitive, attentional tasks or anxiogenic response (Porges and Raskin, 1969; Goldman-Rakic, 1996; Shinba et al., 2008; Mukherjee et al., 2011). An increase in sympathetic modulation analyzed in time, frequency and nonlinear domains was observed when cognitive demand increased (Mukherjee et al., 2011; Luque-Casado et al., 2013). Furthermore, HRV was reduced in participants who achieved worse results in cognitive tasks in which the prefrontal area was involved. This could indicate the possibility of HRV predicting cognitive performance (Muthukrishnan et al., 2017), but this association is still unclear in a high cognitive load sport such as chess, where the relation between players’ psychophysiological responses and their performance is currently unknown.
This psychophysiological markers, HRV, has emerged as an interesting tool to monitor training and performance in chess (Fuentes et al., 2018), but also, it could help to determine the psychophysiological response of chess players in cognitive tasks with a different level of difficulty. Traditionally, stress assessment has been easily measured by a visual analogue scale (VAS), and it is considered a valid instrument (Lesage et al., 2012). VAS could allow determining the level of stress of a cognitive task as well as whether the perceived difficulty or complexity of chess problems corresponds with the theoretical difficulty of the problem-solving task or not. These perceptions could reinforce HRV information which may be useful in the interpretation of psychophysiological data.
The present study aimed to evaluate differences between two groups of chess players (divided according to their performance in the high and the low performance group) in the HRV and perceived subjective difficulty, stress and complexity while they were completing six chess problems with different difficulty. The initial hypotheses were that (a) HRV will be reduced and subjective difficulty, stress and complexity will be increased when the difficulty of the problem is increased and (b) players with higher performance will have higher values of HRV and less perceived difficulty, stress and complexity during the different tasks than the low performance group.
Materials and Methods
Participants
A total of 16 male chess players, age: 35.19 (13.44) were analyses (see Table 1). All the participants were classified according to the ranking system of the World Chess Federation (FIDE), which was developed by Elo (1978). In the present study, the chess players were divided into two groups, according to the results achieved solving six chess problem tasks: High performance, n: 8; ELO: 1974 (161) or low performance, n: 8; ELO: 1882 (172). The correct solution for each problem-solving task was awarded 1 point. The maximum score was 6. Players who solved more than half of the problem tasks were included in the high performance group. Participants were not on medication that could affect the autonomic nervous system. They gave written informed consent to participate in the study. All procedures were approved by the University of Extremadura research ethics committee (approval number: 85/2015) and were carried out in accordance with the Declaration of Helsinki. Exclusion criteria included: (1) inability to perform the tasks with the computer, (2) diseases that affect the autonomic nervous system, and (3) not being classified by the International Chess Federation with ELO.
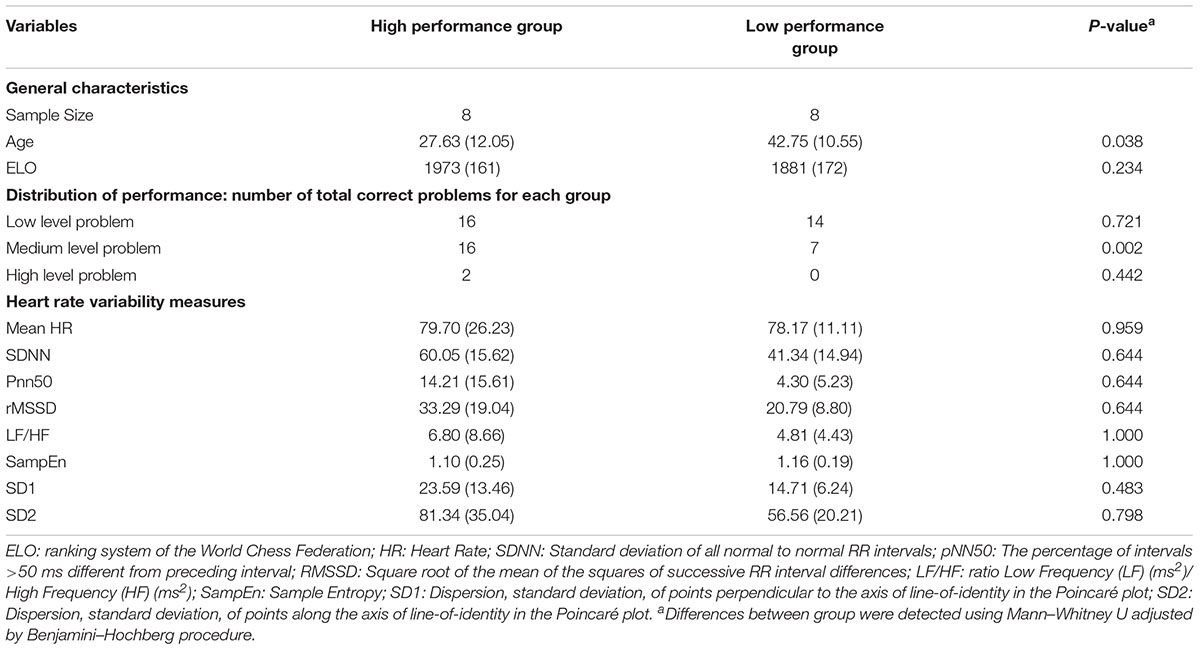
Table 1. Between group comparisons of the general characteristics, distribution of performance, and basal values of heart rate variability.
In order to calculate the sample size and power analysis of the study, a non-linear measure of HRV known as “SD1” (defined as the long-term beat-to-beat variability using Poincaré plot) was used. The sample size of the current study was 16 participants. This sample size reached 86% power to detect a difference of 14.1 (p-value < 0.05) between the null hypothesis and the alternative hypothesis using a Mann–Whitney test.
Procedure
Before starting the study, participants were given instructions on procedures and protocol requirements during the problem-solving tasks. Participants underwent a familiarization period with the computer and the equipment required for testing. The research was conducted in a laboratory with automatically and continuously controlled temperature and humidity, 20.2 (1.0)°C; 56.4 (2.8)% humidity. Noise levels were kept under 30 dB during all the procedure. All the evaluation was conducted in the morning, between 10:00 and 14:00, with 1 h of no liquid and food ingestion, with no medication or caffeine ingestion in the last 24 h and with at least 24 h since the last vigorous physical activity.
Both groups (high and low performance groups) conducted a total of six chess problem-solving tasks (see Figure 1), which were selected from Total Chess Training CT-ART 3.0 by a FIDE master (ELO rating of 2300 or more). Chess problems consisted of two low-level, two medium-level and two high-level chess problems (Figure 1). Participants had two and a half minutes to solve each problem. In low-level problems, participants were encouraged to do one move in the first one and two moves in the second problem. In medium-level problems, three moves in the first and two moves in the second problem were required. Finally, in high-level problems participants were asked to do two moves in the first and four moves in the second problem (see Figure 1).
Heart rate variability was analyzed at baseline and while participants were completing the problems. Immediately after each level of difficulty (high, medium and low levels), perceived difficulty, stress and complexity of each problem were also measured (see Figure 2). The order of the problems was randomized to avoid the effects of one task on others. Problem-solving tasks were carried out using the 64-bit Fritz 15 chess engine, with Stockfish 6 for Windows. This software is one of the strongest chess engines in the world, and it is open source (GPL license). An ASUS laptop was used (Intel Core i7-6500U, 1 TB, 8 GB memory DDR3L-SDRAM). Fritz software automatically responded to moves, simulating a real chess environment, with the best move previously computed by the research group.
Heart rate variability was recorded at baseline and while performing the tasks, according to the Task Force of the European Society of Cardiology and the North American Society of Pacing and Electrophysiology (Camm et al., 1996) during a short-term 5-min period in sitting position and also following the instructions of previous studies (Beltrán-Velasco et al., 2018; Sánchez-Molina et al., 2018). HRV was measured with a reliable heart rate monitor (Polar RS800CX, Finland) (da Costa et al., 2016). Time and frequency domains, as well as non-linear measures, were extracted using Kubios HRV software (v. 2.1) (Tarvainen et al., 2014). HRV measures were merged for each level of difficulty. The duration of each level of difficulty was 5 min to comply with the short-term HRV recording recommendation (Camm et al., 1996). A medium filter was applied to correct artifacts, and the correction level identified all beat to beat intervals (RR) that were longer/shorter than 0.25 s compared to the local average. The correction was made by replacing the identified artifacts with interpolated values using a cubic spline interpolation.
For the time domain, the heart rate (HR), the standard deviation of all normal to normal RR intervals (SDNN), the percentage of intervals >50 ms different from preceding interval (pNN50) and the root of the mean of the squares of successive RR interval differences (RMSSD) were analyzed. In the frequency domain, the ratio between Low Frequency (LF) (ms2)/ High Frequency (HF) (ms2) was calculated. On the other hand, non-linear measures such as the Sample Entropy (SampEn) and the dispersion, standard deviation, of points perpendicular to the axis of line-of-identity in the Poincaré plot (SD1) and the dispersion, standard deviation, of points along the axis of line-of-identity in the Poincaré plot (SD2) were also included in the analysis. Higher values of SDNN, RMSSD, SD1, and SampEn, are associated with parasympathetic modulation as well as a reduction in these previous indexes or lower values of LF/HF and SD2 are associated with sympathetic modulation (Kamen et al., 1996; Karmakar et al., 2011; Soares-Miranda et al., 2014; Weippert et al., 2014).
A Visual Analogue Scale (VAS) (0–10) was used to measure perceived stress (during the problem-solving tasks), difficulty (perceived difficulty for each problem) and complexity (subjective perception of reaching the goal, taking into account both the limited period of time and game difficulty) after every single task. These behavioral data were collected to reinforce HRV information and to provide useful information for the interpretation.
Statistics
The SPSS statistical package (version 20.0; SPSS, Inc., Chicago, IL, United States) was used to analyze the data. Considering the sample size (n = 16) and the results in the Shapiro–Wilk and Kolmogorov–Smirnov tests, non-parametric analyses were conducted.
Friedman’s ANOVA by ranks was used to evaluate within-group differences in HRV and subjective perception in the three conditions: low, medium and high difficulty levels. Adjusted post-hoc with Dunn-Bonferroni for multiple comparisons was obtained to control Type I error (Dunn, 1961). Differences between these two groups for each level of difficulty were assessed using the Mann–Whitney U-test. The alpha-level of significant (set at 0.05) was adjusted by Benjamini–Hochberg procedure in order to control the false discovery rates (Benjamini and Hochberg, 1995).
Results
Participants were classified according to their performance on the tasks into two groups: high and low performance groups. Mann–Whitney U at baseline revealed significant differences between groups at age (U = 12.50, p = 0.038, r = 0.51), with high performance group aged 27.63 (12.05) and low performance group aged 42.75 (10.55). However, non-significant differences between groups were observed at baseline in any of the HRV measures or ELO (U = 20, p = 0.234, r = 0.31). The high performance group achieved a significantly higher number of correct solved problems in the medium level problem [χ2 (1, N = 16) = 12.44, p < 0.01] (see Table 1).
Within-Group Differences
In the high performance group, the task difficulty increment led to significant changes in the autonomic modulation and subjective difficulty, stress and complexity. Comparing medium vs. high-level problems, the HRV (RMSSD and SD1) significantly decreased when difficulty increased. In the same line, when compared low vs. high-level problems, subjective difficulty, stress and complexity increased (see Table 2). In the low performance group, significant effects of the difficulty increments were observed in mean heart rate and perceived difficulty and stress when low vs. high-level problems were compared (see Table 2).
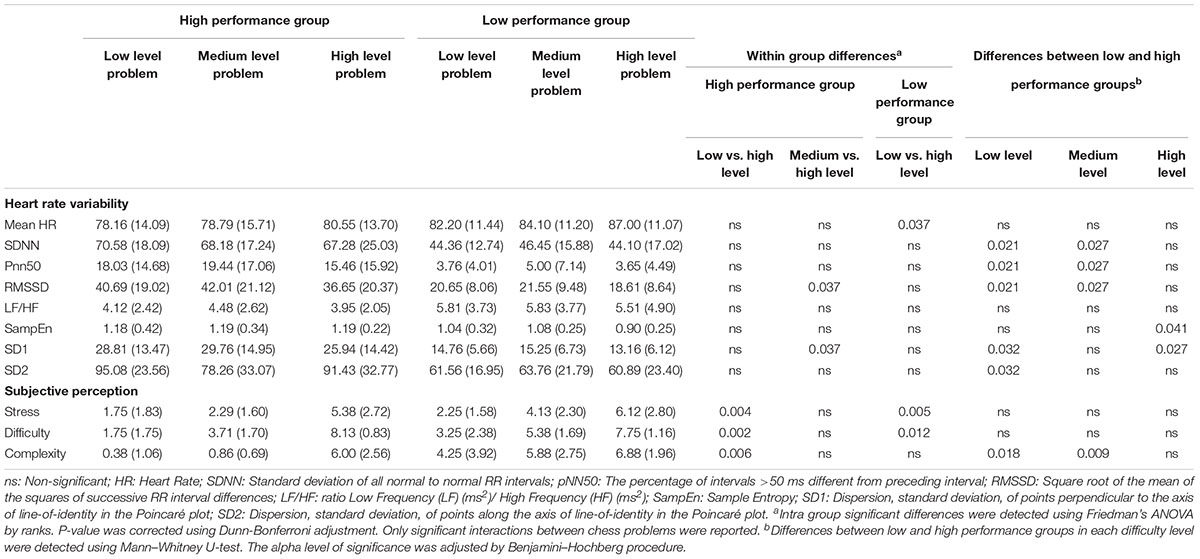
Table 2. Mean (SD) of the different chess problem for each group and intra and inter groups comparisons for heart rate variability and subjective perception.
Between-Group Differences
During low-difficulty level problems, the high performance group obtained an increased HRV (higher values of SDNN, Pnn50, rMSSD, and SD1) and a reduction in the perceived complexity compared to the low performance group (see Table 2). In the same line, in the medium-difficulty level problem, the high performance group obtained higher values of HRV (higher values of SDNN, Pnn50, and rMSSD) and a reduction in the perceived complexity compared to the low performance group (see Table 2). Moreover, during the high-level problems, higher parasympathetic activity (higher values of HRV variables such as SampEn and SD1) were found in the high performance group compared with the low performance group while the perceived complexity was not different (see Table 2).
Discussion
The present study examined differences in HRV and perceived subjective difficulty, stress and complexity during chess tasks of varying difficulty in different chess performance players. Overall, results showed that when task difficulty increased, HRV decreased in both the high and the low performance groups. Regarding subjective measures, perceived difficulty, stress and complexity increased when the difficulty of the task increased in the high performance group, whereas in the low performance group, only perceived difficulty and stress increased when the task difficulty increased. Therefore, results were in line with the initial hypotheses, where we stated that highly difficult tasks would produce a decrease in HRV. That hypothesis assumed that higher-performing players would reach higher parasympathetic modulation (higher HRV), as well as lower perceived stress, difficulty and complexity during the different tasks than the low performance group.
Within-group results in terms of HRV indicated that SD1 and rMSSD were higher in the medium level problems compared with the high level problems in the high performance group. This could indicate that the sympathetic responses were higher when the problem difficulty increased, which could be aimed to deal with the problem’s cognitive demands (Wickens et al., 2015). In line with these results, previous researchers have shown that the greater the cognitive load, the lower the HRV (Hjortskov et al., 2004; Mukherjee et al., 2011), supporting the idea of HRV as a reliable and sensitive index to mental effort (Mukherjee et al., 2011). In addition, an increased in the subjective perception of stress, difficulty and complexity was observed between the low and the high level problems. However, the low performance group did not show these patterns in HRV when the task difficulty was increased. This could indicate that the cognitive requirements of the three problems were too high for them. This hypothesis could be supported by the differences in the perception of complexity showed in the between group comparisons. In this regard, the low performance group perceived both the low and the medium difficulty levels as more complex compared with the high performance group. Therefore, HRV seems to be sensitive enough to be used as a training tool for monitoring and controlling the cognitive load.
In addition, between-group analysis revealed that HRV – specifically SDNN, rMSSD and Pnn50 – was significantly higher in the high performance group than in the low performance group during chess problems of varying difficulty. The unsuccessful performance of the low performance group could be related to the higher anxiogenic response, associated to the significantly lower HRV values, in comparison with the high performance group (Gur et al., 1988). In line with this result, a previous study reported that HRV was reduced in a group who achieved worse results in a cognitive task (Muthukrishnan et al., 2017). Findings from the current study could support the possibility of HRV predicting cognitive performance in chess players.
Chess has been used for the study of cognitive processes such as memory, problem solving or decision making (Chase and Simon, 1973; Kiesel et al., 2009; Villafaina et al., 2018). In our study, different chess problem tasks where abilities like logic and mathematics have a great influence were proposed. Trinchero and Sala (2016) hypothesized that the heuristic method, i.e., a method for arriving at satisfactory solutions with the modest amount of computations (Simon, 1990), could help to reduce the potential solutions of the task and lead to a reduction in the cognitive load (Shah and Oppenheimer, 2008). In the current study, higher values of HRV were detected in the high performance group compared with the low performance group, which could indicate that high performance group experimented lower cognitive load. Future studies are encouraged to explore the potential association of cognitive load and HRV following the basis of the heuristic method.
Chess has been used for the study of cognitive processes such as memory, problem-solving or decision making (Chase and Simon, 1973; Kiesel et al., 2009; Villafaina et al., 2018). In our study different chess problem tasks, where abilities like logic and mathematics have a great influence, were proposed. Trinchero and Sala (2016) hypothesized that the heuristic method, i.e., a method for arriving at satisfactory solutions with the modest amount of computations (Simon, 1990), could help to reduce the potential solutions of the task and lead to a reduction in the cognitive load (Shah and Oppenheimer, 2008). This hypothesized reduction in the cognitive load could lead to a reduction in the sympathetic modulation, decreasing the HRV indexes. We reported in our study higher values of HRV in the high performance group compared with the low performance group while performing different levels of problem difficulty. Probably, this could indicate that the cognitive load of the high performance group was lower. Therefore, it is possible that this group had more problem-solving heuristic resources than the low performance group. However, future studies should evaluate this hypothesis.
Results of within and between group analyses revealed significant differences in SD1 (an HRV non-linear measure). This could indicate that this index may be more sensitive to changes caused by cognitive processing than others. In line with our results, time measures and SD1 have emerged as reliable and sensitive indexes of mental effort (Mukherjee et al., 2011). This may support the idea of non-linear analysis as an adequate measure in complex dynamic systems (Goldberger, 1996) and reinforce the suitability of HRV to be used to monitor and control the mental effort.
One limitation of the current study was the significant between-group differences that were observed in the participants’ age. However, the two groups did not statistically differ in HRV at baseline in any of the studied variables. Nevertheless, since HRV could be influenced by age (Reardon and Malik, 1996) and there is a lack of studies focused on the influence of age on HRV during cognitive tasks, the obtained results should be taken with caution. Also, the sample size was relatively small. Another potential limitation may be the fact that problem-solving tasks were conducted with the computer. This could affect motivation, and therefore the cognitive engagement of our participants in the problem-solving tasks. Although all participants had played chess with the computer previously, results might be different to those obtained in “real” chess. Furthermore, the sample size (n = 16) was relatively small, which could also affect the HRV parameter, making harder the comparisons according to body mass index or age. This study was only developed with men, so future studies should determine if these results could be extrapolated to women.
Practical Applications
Results of the present study showed how increasing the difficulty level of chess problems could modify the HRV according to cognitive performance. Thus, HRV is sensitive enough to detect changes in HRV as a consequence of the increase of the cognitive demands.
Therefore, monitoring the HRV of chess players could be useful to control the cognitive load of the proposed tasks. Although further research is needed, when the HRV of the chess player is decreased, the cognitive stimulus may be leading to a relevant cognitive load. However, when the cognitive load does not change the HRV of the player, probably the cognitive load may be too high or too low (as could happen in the low performance group). Thus HRV is presented as an easy and highly applicable training tool for chess players that may be considered by trainers to control the cognitive load in chess-related tasks.
Conclusion
To conclude, this is the first study reporting a decrease of HRV to meet the cognitive demands of the problems in chess players. HRV was significantly higher in the high performance group than in the low performance group when solving chess problems of varying difficulty. In addition, the low performance group perceived the problem solving tasks as more complex than the high performance group. These results open a new field where HRV could be an interesting and useful tool in chess training to assess the cognitive demands and capacities of chess players. However, the relatively small sample size and the difference in age between groups makes that these findings should be taken with caution.
Author Contributions
JF-G, SV, and VC-S conceived the study. JF-G, SV, and DC-M collected the data. PO, VC-S, RdV, DC-M, JF-G, and SV analyzed the data. PO, VC-S, RdV, and DC-M designed the figures and tables. SV, JF-G, and DC-M wrote the manuscript. VC-S, SV, PO, and RdV provided critical revisions on the successive drafts. All authors approved the manuscript in its final form.
Funding
DC-M was supported by a grant from the Spanish Ministry of Education, Culture and Sport (FPU14/01283). SV was supported by a grant from the regional department of economy and infrastructure of the Government of Extremadura and European Social Fund (PD16008). The funders had no role in study design, data collection and analysis, decision to publish, or preparation of the manuscript.
Conflict of Interest Statement
The authors declare that the research was conducted in the absence of any commercial or financial relationships that could be construed as a potential conflict of interest.
References
Aciego, R., Garcia, L., and Betancort, M. (2012). The benefits of chess for the intellectual and social-emotional enrichment in schoolchildren. Span. J. Psychol. 15, 551–559. doi: 10.5209/rev_SJOP.2012.v15.n2.38866
Amidzic, O., Riehle, H. J., and Elbert, T. (2006). Toward a psychophysiology of expertise - Focal magnetic gamma bursts as a signature of memory chunks and the aptitude of chess players. J. Psychophysiol. 20, 253–258. doi: 10.1027/0269-8803.20.4.253
Bart, W. M. (2014). On the effect of chess training on scholastic achievement. Front. Psychol. 5:762. doi: 10.3389/fpsyg.2014.00762
Beltrán-Velasco, A. I., Bellido-Esteban, A., Ruisoto-Palomera, P., and Clemente-Suárez, V. J. (2018). Use of portable digital devices to analyze autonomic stress response in psychology objective structured clinical examination. J. Med. Syst. 42:35. doi: 10.1007/s10916-018-0893-x
Benjamini, Y., and Hochberg, Y. (1995). Controlling the false discovery rate: a practical and powerful approach to multiple testing. J. R. Stat. Soc. Ser. B 57, 289–300. doi: 10.1111/j.2517-6161.1995.tb02031.x
Camm, A. J., Malik, M., Bigger, J. T., Breithardt, G., Cerutti, S., Cohen, R. J., et al. (1996). Heart rate variability. Standards of measurement, physiological interpretation, and clinical use. Eur. Heart J. 17, 354–381. doi: 10.1093/oxfordjournals.eurheartj.a014868
Chase, W. G., and Simon, H. A. (1973). Perception in chess. Cognit. Psychol. 4, 55–81. doi: 10.1016/0010-0285(73)90004-2
da Costa, M. P., Da Silva, N. T., De Azevedo, F. M., Pastre, C. M., and Marques Vanderlei, L. C. (2016). Comparison of Polar((R)) RS800G3 heart rate monitor with Polar((R)) S810i and electrocardiogram to obtain the series of RR intervals and analysis of heart rate variability at rest. Clin. Physiol. Funct. Imag. 36, 112–117. doi: 10.1111/cpf.12203
Dunn, O. J. (1961). Multiple comparisons among means. J. Am. Stat. Assoc. 56, 52–64. doi: 10.1080/01621459.1961.10482090
Elkies, N. D., and Stanley, R. P. (2003). The mathematical knight. Math. Intell. 25, 22–34. doi: 10.1007/BF02985635
Fuentes, J. P., Villafaina, S., Collado-Mateo, D., De La Vega, R., Gusi, N., and Clemente-Suarez, V. J. (2018). Use of biotechnological devices in the quantification of psychophysiological workload of professional chess players. J. Med. Syst. 42, 40–40. doi: 10.1007/s10916-018-0890-0
Gobet, F., and Simon, H. A. (1998). Expert chess memory: revisiting the chunking hypothesis. Memory 6, 225–255. doi: 10.1080/741942359
Goldberger, A. L. (1996). Non-linear dynamics for clinicians: chaos theory, fractals, and complexity at the bedside. Lancet 347, 1312–1314. doi: 10.1016/S0140-6736(96)90948-4
Goldman-Rakic, P. S. (1996). The prefrontal landscape: implications of functional architecture for understanding human mentation and the central executive. Philos. Trans. R. Soc. Lond. Ser. B Biol. Sci. 351, 1445–1453. doi: 10.1098/rstb.1996.0129
Guida, A., Gobet, F., Tardieu, H., and Nicolas, S. (2012). How chunks, long-term working memory and templates offer a cognitive explanation for neuroimaging data on expertise acquisition: a two-stage framework. Brain Cognit. 79, 221–244. doi: 10.1016/j.bandc.2012.01.010
Gur, R. C., Gur, R. E., Skolnick, B. E., Resnick, S. M., Silver, F. L., Chawluk, J., et al. (1988). Effects of task-difficulty on regional cerebral blood flow - relationships with anxiety and performance. Psychophysiology 25, 392–399. doi: 10.1111/j.1469-8986.1988.tb01874.x
Hjortskov, N., Rissén, D., Blangsted, A. K., Fallentin, N., Lundberg, U., and Søgaard, K. (2004). The effect of mental stress on heart rate variability and blood pressure during computer work. Eur. J. Appl. Physiol. 92, 84–89. doi: 10.1007/s00421-004-1055-z
Kamen, P. W., Krum, H., and Tonkin, A. M. (1996). Poincare plot of heart rate variability allows quantitative display of parasympathetic nervous activity in humans. Clin. Sci. 91, 201–208. doi: 10.1042/cs0910201
Karmakar, C. K., Khandoker, A. H., Voss, A., and Palaniswami, M. (2011). Sensitivity of temporal heart rate variability in Poincaré plot to changes in parasympathetic nervous system activity. Biomed. Eng. Online 10:17. doi: 10.1186/1475-925X-10-17
Kazemi, F., Yektayar, M., and Abad, A. M. B. (2012). Investigation the impact of chess play on developing meta-cognitive ability and math problem-solving power of students at different levels of education. Proc. Soc. Behav. Sci. 32, 372–379. doi: 10.1016/j.sbspro.2012.01.056
Kiesel, A., Kunde, W., Pohl, C., Berner, M. P., and Hoffmann, J. (2009). Playing chess unconsciously. J. Exp. Psychol. Learn. Mem. Cogn. 35, 292–298. doi: 10.1037/a0014499
Koechlin, E., and Hyafil, A. (2007). Anterior prefrontal function and the limits of human decision-making. Science 318, 594–598. doi: 10.1126/science.1142995
Lesage, F.-X., Berjot, S., and Deschamps, F. (2012). Clinical stress assessment using a visual analogue scale. Occupat. Med. 62, 600–605. doi: 10.1093/occmed/kqs140
Lin, Q., Cao, Y., and Gao, J. (2015). The impacts of a GO-game (Chinese chess) intervention on Alzheimer disease in a Northeast Chinese population. Front. Aging Neurosci. 7:163. doi: 10.3389/fnagi.2015.00163
Luque-Casado, A., Zabala, M., Morales, E., Mateo-March, M., and Sanabria, D. (2013). Cognitive performance and heart rate variability: the influence of fitness level. PLoS One 8:e56935. doi: 10.1371/journal.pone.0056935
Mathy, F., Fartoukh, M., Gauvrit, N., and Guida, A. (2016). Developmental abilities to form chunks in immediate memory and its non-relationship to span development. Front. Psychol. 7:201. doi: 10.3389/fpsyg.2016.00201
Mukherjee, S., Yadav, R., Yung, I., Zajdel, D. P., and Oken, B. S. (2011). Sensitivity to mental effort and test-retest reliability of heart rate variability measures in healthy seniors. Clin. Neurophysiol. 122, 2059–2066. doi: 10.1016/j.clinph.2011.02.032
Muthukrishnan, S. P., Gurja, J. P., and Sharma, R. (2017). Does heart rate variability predict human cognitive performance at higher memory loads? Indian J. Physiol. Pharmacol. 61, 14–22.
Porges, S. W., and Raskin, D. C. (1969). Respiratory and heart rate components of attention. J. Exp. Psychol. 81, 497–503. doi: 10.1037/h0027921
Reardon, M., and Malik, M. (1996). Changes in heart rate variability with age. Pacing Clin. Electrophysiol. 19, 1863–1866. doi: 10.1111/j.1540-8159.1996.tb03241.x
Sala, G., Foley, J. P., and Gobet, F. (2017). The effects of chess instruction on pupils’ cognitive and academic skills: state of the art and theoretical challenges. Front. Psychol. 8:238. doi: 10.3389/fpsyg.2017.00238
Sala, G., Gorini, A., and Pravettoni, G. (2015). Mathematical problem-solving abilities and chess: an experimental study on young pupils. Sage Open 5:2158244015596050. doi: 10.1177/2158244015596050
Sánchez-Molina, J., Robles-Pérez, J. J., and Clemente-Suárez, V. J. (2018). Assessment of psychophysiological response and specific fine motor skills in combat units. J. Med. Syst. 42:67. doi: 10.1007/s10916-018-0922-9
Shaffer, F., Mccraty, R., and Zerr, C. L. (2014). A healthy heart is not a metronome: an integrative review of the heart’s anatomy and heart rate variability. Front. Psychol. 5:1040. doi: 10.3389/fpsyg.2014.01040
Shah, A. K., and Oppenheimer, D. M. (2008). Heuristics made easy: an effort-reduction framework. Psychol. Bull. 134:207. doi: 10.1037/0033-2909.134.2.207
Shinba, T., Kariya, N., Matsui, Y., Ozawa, N., Matsuda, Y., and Yamamoto, K. (2008). Decrease in heart rate variability response to task is related to anxiety and depressiveness in normal subjects. Psychiatry Clin. Neurosci. 62, 603–609. doi: 10.1111/j.1440-1819.2008.01855.x
Simon, H. A. (1990). Invariants of human behavior. Annu. Rev. Psychol. 41, 1–20. doi: 10.1146/annurev.ps.41.020190.000245
Soares-Miranda, L., Sattelmair, J., Chaves, P., Duncan, G., Siscovick, D. S., Stein, P. K., et al. (2014). Physical activity and heart rate variability in older adults: the Cardiovascular Health Study. Circulation 129, 2100–2110. doi: 10.1161/CIRCULATIONAHA.113.005361
Tarvainen, M. P., Niskanen, J.-P., Lipponen, J. A., Ranta-Aho, P. O., and Karjalainen, P. A. (2014). Kubios HRV - Heart rate variability analysis software. Comput. Methods Progr. Biomed. 113, 210–220. doi: 10.1016/j.cmpb.2013.07.024
Thayer, J. F., Ahs, F., Fredrikson, M., Sollers, J. J., and Wager, T. D. (2012). A meta-analysis of heart rate variability and neuroimaging studies: implications for heart rate variability as a marker of stress and health. Neurosci. Biobehav. Rev. 36, 747–756. doi: 10.1016/j.neubiorev.2011.11.009
Trinchero, R., and Sala, G. (2016). Chess training and mathematical problem-solving: the role of teaching heuristics in transfer of learning. Eurasia J. Math. Sci. Technol. Educ. 12, 655–668. doi: 10.12973/eurasia.2016.1255a
Troubat, N., Fargeas-Gluck, M.-A., Tulppo, M., and Dugue, B. (2009). The stress of chess players as a model to study the effects of psychological stimuli on physiological responses: an example of substrate oxidation and heart rate variability in man. Eur. J. Appl. Physiol. 105, 343–349. doi: 10.1007/s00421-008-0908-2
Villafaina, S., Collado-Mateo, D., Cano-Plasencia, R., Gusi, N., and Fuentes, J. P. (2018). Electroencephalographic response of chess players in decision-making processes under time pressure. Physiol. Behav. 198, 140–143. doi: 10.1016/j.physbeh.2018.10.017
Weippert, M., Behrens, M., Rieger, A., and Behrens, K. (2014). Sample entropy and traditional measures of heart rate dynamics reveal different modes of cardiovascular control during low intensity exercise. Entropy 16, 5698–5711. doi: 10.3390/e16115698
Keywords: autonomic modulation, chess, cognition, heart rate variability, cognitive load
Citation: Fuentes-García JP, Villafaina S, Collado-Mateo D, de la Vega R, Olivares PR and Clemente-Suárez VJ (2019) Differences Between High vs. Low Performance Chess Players in Heart Rate Variability During Chess Problems. Front. Psychol. 10:409. doi: 10.3389/fpsyg.2019.00409
Received: 15 July 2018; Accepted: 11 February 2019;
Published: 26 February 2019.
Edited by:
Timothy L. Hubbard, Arizona State University, United StatesReviewed by:
Dirk Koester, Bielefeld University, GermanyFrancisco J. Ruiz, Fundación Universitaria Konrad Lorenz, Colombia
Fleur Margaret Howells, University of Cape Town, South Africa
Copyright © 2019 Fuentes-García, Villafaina, Collado-Mateo, de la Vega, Olivares and Clemente-Suárez. This is an open-access article distributed under the terms of the Creative Commons Attribution License (CC BY). The use, distribution or reproduction in other forums is permitted, provided the original author(s) and the copyright owner(s) are credited and that the original publication in this journal is cited, in accordance with accepted academic practice. No use, distribution or reproduction is permitted which does not comply with these terms.
*Correspondence: Santos Villafaina, c3ZpbGxhZmFpbmFAdW5leC5lcw==