- 1National Institute of Mental Health, Klecany, Czechia
- 2Department of Psychology, Faculty of Arts, Charles University in Prague, Prague, Czechia
- 3Third Faculty of Medicine, Charles University in Prague, Prague, Czechia
- 4Department of Psychology, Faculty of Social Studies, Masaryk University, Brno, Czechia
- 5Department of Psychology, Faculty of Arts, Masaryk University, Brno, Czechia
The character of cognitive deficit in schizophrenia is not clear due to the heterogeneity in research results. In heterogeneous conditions, the cluster solution allows the classification of individuals based on profiles. Our aim was to examine the cognitive profiles of first-episode schizophrenia spectrum disorder (FES) subjects based on cluster analysis, and to correlate these profiles with clinical variables and resting state brain connectivity, as measured with magnetic resonance imaging. A total of 67 FES subjects were assessed with a neuropsychological test battery and on clinical variables. The results of the cognitive domains were cluster analyzed. In addition, functional connectivity was calculated using ROI-to-ROI analysis with four groups: Three groups were defined based on the cluster analysis of cognitive performance and a control group with a normal cognitive performance. The connectivity was compared between the patient clusters and controls. We found different cognitive profiles based on three clusters: Cluster 1: decline in the attention, working memory/flexibility, and verbal memory domains. Cluster 2: decline in the verbal memory domain and above average performance in the attention domain. Cluster 3: generalized and severe deficit in all of the cognitive domains. FES diagnoses were distributed among all of the clusters. Cluster comparisons in neural connectivity also showed differences between the groups. Cluster 1 showed both hyperconnectivity between the cerebellum and precentral gyrus, the salience network (SN) (insula cortex), and fronto-parietal network (FPN) as well as between the PreCG and SN (insula cortex) and hypoconnectivity between the default mode network (DMN) and seeds of SN [insula and supramarginal gyrus (SMG)]; Cluster 2 showed hyperconnectivity between the DMN and cerebellum, SN (insula) and precentral gyrus, and FPN and IFG; Cluster 3 showed hypoconnectivity between the DMN and SN (insula) and SN (SMG) and pallidum. The cluster solution confirms the prevalence of a cognitive decline with different patterns of cognitive performance, and different levels of severity in FES. Moreover, separate behavioral cognitive subsets can be linked to patterns of brain functional connectivity.
Introduction
Schizophrenia is typically described as a heterogeneous disease (Tandon et al., 2009; Owen et al., 2016). A model of heterogeneity in Schizophrenia with its causes, characteristics, and course and outcome was well summarized by Seaton et al. (2001). Symptoms of schizophrenia, treatment response, and outcome vary during the course of the illness (Andreasen et al., 2005; Tandon et al., 2009). The heterogeneity has also its implication for the better understanding of its pathogenesis (Liang and Greenwood, 2015); it complicates also the search for neurobiological correlates and the character of cognitive functioning (Heilbronner et al., 2016; Owen et al., 2016).
Cognitive deficit (CD) is a well-established marker of schizophrenia (Heinrichs and Zaksanis, 1998; Mesholam-Gately et al., 2009; Fioravanti et al., 2012), observed already in the early stage of the illness (Fusar-Poli et al., 2012), and before the patient is exposed to medication (Fatouros-Bergman et al., 2014). Moreover, CD is present in ultra-high risk states (Bora and Murray, 2014), and other psychotic disorders (Bora et al., 2009, 2010) and influences daily functioning (Bowie et al., 2006). Results of research show a great variability of cognitive functioning in schizophrenia: (1) CD over time can be either stable, declining, or improving (Szöke et al., 2008); (2) CD is general or partial (Heilbronner et al., 2016); (3) different cognitive phenotypes can be considered as endophenotypes or predictors of daily functioning (Szöke et al., 2008; Wölwer et al., 2008). Factorial analyses of cognition in schizophrenia consistently show a deficit in six-to-seven cognitive factors (domains): attention, verbal, visual, and working memory (WM), speed of processing (APOP), reasoning and abstraction (ABST), and social cognition (Nuechterlein et al., 2004, 2008; Dickinson et al., 2006; Rodriguez et al., 2017). Moreover, neuroimaging studies have found a correlation between structural and functional brain analysis and cognition. One of the most important theories in this relationship is the dysconnectivity theory of schizophrenia, which implies an abnormal pattern of connections among distinct brain regions, referred as cognitive dysmetria. This disrupted connectivity results in altered functional integration since it involves either exaggerated connections or weakened pathways (Andreasen et al., 1996; Stephan et al., 2006; Fornito et al., 2011). A more recent systematic review of cognition and resting-state functional connectivity in schizophrenia found abnormalities within and between regions such as the cortico–cerebellar–striatal–thalamic loop and task-positive and task-negative cortical networks. But they did not observe unique relationships between specific functional connectivity abnormalities and distinct cognitive domains. The authors acknowledge as one of the limitations, the inter-dependency of many neuropsychological tasks on multiple cognitive processes, making it difficult to identify what cognitive functions are truly impaired in schizophrenia and the non-specific patterns of resting-state functional connectivity correlations with distinct cognitive domains (Sheffield and Barch, 2016). Therefore, while there is no doubt about the presence and impact of CD in schizophrenia, the character of the deficit remains unclear. New approaches are necessary to help us better understand the nature of cognitive functioning in schizophrenia.
In order to study the character of a heterogeneous disorder, it is useful to establish more homogenous groups inside the condition. Cluster analysis is a method that allows the classification of individuals based on their profiles, helping to reduce the heterogeneity of findings. In psychotic disorders, a cross-diagnostic cluster analysis in subjects with schizophrenia, schizoaffective disorder, and bipolar disorder with psychosis provided four different cognitive profiles. The diagnoses were distributed among all cognitive clusters and the authors concluded that specific neurocognitive profiles can underlie relevant neural abnormalities between these diagnoses (Lewandowski et al., 2014). In schizophrenia, the identification of distinct phenotypes of cognitive profiles better explains the relationship between cognitive profiles and external variables (Seaton et al., 2001) such as age, education (Goldstein et al., 1998), clinical symptomatology (Hill et al., 2002), or functional outcome (Gilbert et al., 2014). Another approach to reducing the heterogeneity of results with clusters is to examine the relationships within cognitive variables and neuroimaging procedures, for example between brain function and structure (Heinrichs and Awad, 1993; Geisler et al., 2015), or as a phenotypic feature shared with first-degree relatives (Ohi et al., 2017).
Cluster analysis of cognitive functioning in schizophrenia typically generates three clusters: mild (like-normal), moderate (partial deficit), and severe (general deficit). The number of cognitive clusters has been demonstrated to be more or less consistent, but the heterogeneity persists within the clusters. A more homogeneous sample and a combination of different external variables may help to understand the nature of the profiles.
The aim of our study was to examine cognitive profiles of first-episode schizophrenia spectrum disorders (FES) based on cluster analysis of their cognitive performance, and to correlate these profiles with clinical variables and resting state brain connectivity, as measured with magnetic resonance imaging.
Materials and Methods
The study was conducted at the National Institute of Mental Health, Czech Republic; the study protocol was approved by the Ethical Committee.
Study Population
The study subjects were diagnosed with FES according to ICD-10 criteria with F20 (55%) and F23 (45%). Patients were evaluated under a clinically stable condition, at the end of their first psychiatric hospitalization, and in a partial symptomatic remission state, according to Andreasen’s remission criteria (2005). The study subjects were diagnosed in a routine clinical process by two experienced psychiatrists. In the event of diagnostic disagreements (e.g., comorbidity), the subjects were excluded from the study. Other exclusion criteria were: neurological disorders, head injuries, comorbid psychiatric or somatic disorder, specific developmental disorders of academic skills, and other medical or cognitive conditions that may alter cognitive functioning, metal implants in the head and face, or a cardiac pacemaker. The control group consisted of healthy subjects (HC), without history of any psychiatric disorders. All of the study subjects signed an informed consent form.
Study Materials and Procedures
Neuropsychological Assessment
The study subjects were administered a battery of neuropsychological tests, consisting of the following domains (for a detailed description of the calculation of the composition and consistency of cognitive domains, see Rodriguez et al., 2017): SPOP, attention/vigilance (ATTV), WM/flexibility (FLEX), VERBM, visual memory (VISM), and ABST/executive functions (/EXEF). The composition of cognitive domains is presented in Figure 1. The mean duration of the neuropsychological assessment was 150 min. The participants were tested in two consecutive sessions with a break in between. The cognitive assessment was conducted within a maximum of 2 years after the first psychotic episode and within 1 week of imaging assessment. The cut-off on the cognitive domains for our study was SD±1.0.
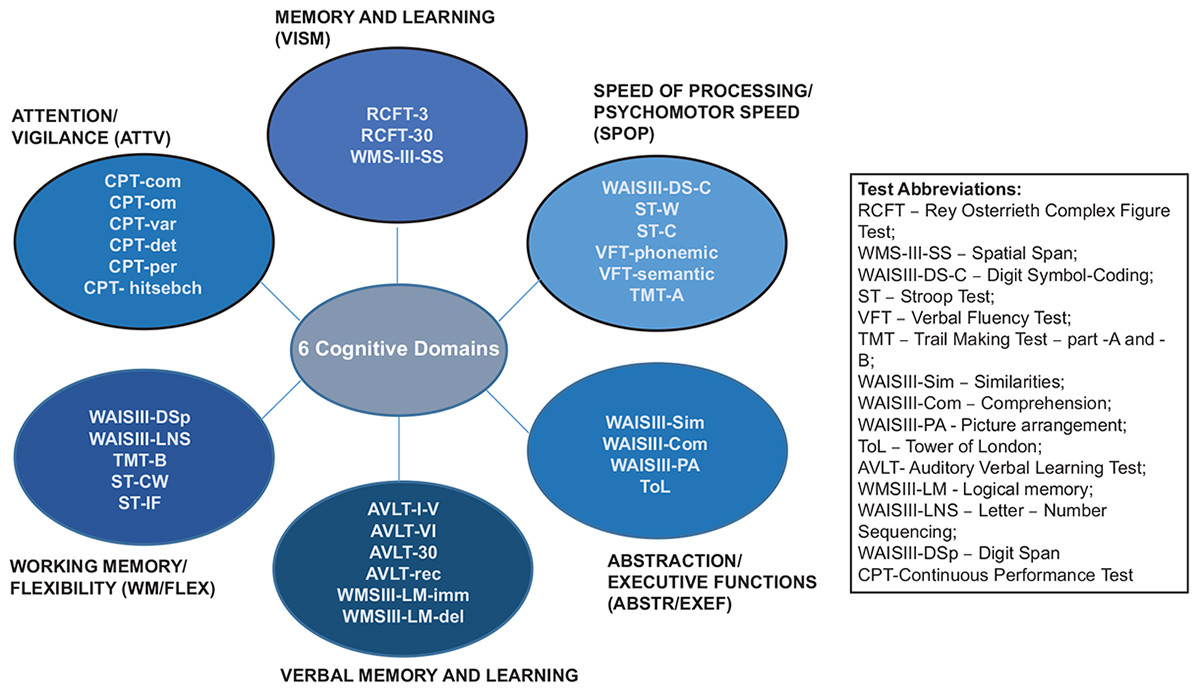
Figure 1. Composition of the cognitive domains (Rodriguez et al., 2017).
Demographic Variables of the Sample
The assessment of FES included the positive and negative syndrome scale (PANSS; Kay et al., 1987), and the short-form quality of life (QOL) questionnaire WHOQOL-BREF (The WHOQOL Group, 1998), the Czech version validated for the Czech population (Dragomirecká and Bartonová, 2006). Demographic data [age, education, gender, employment status, duration of untreated psychosis (DUP), and medication dosage in chlorpromazine equivalents (CHLPZ)] were obtained during the clinical structured interview by a trained psychiatrist.
For the subsequent analysis of the corresponding brain functional connectivity patterns in three cluster groups, we included a control group that consisted of 20 healthy individuals selected randomly from the overall control group used in behavioral analysis (z-scores).
Neuroimaging Data Acquisition and Preprocessing
The magnetic resonance images were acquired at two sites: National Mental Health Institute Klecany (NUDZ Klecany, site 1) and Institute of Clinical and Experimental Medicine Prague (IKEM Prague, site 2). The percentage of the subjects scanned at each site was 41% and 59%, respectively.
The structural and functional scans from site 1 were acquired on a Siemens MAGNETOM Prisma 3T. The T1-weighted images (T1W) with the optimized magnetization prepared by Rapid Acquisition Gradient Echo (MPRAGE) had 224 scans with a slice thickness of 0.7 mm, repetition time 2,400 ms, echo time 2,34 ms, inversion time 1,000 ms, flip angle 8°, and acquisition matrix 320 × 320 mm. The T2∗-weighted images contained 300 scans with a slice thickness of 3 mm, repetition time 2,000 ms, echo time 30 ms, acquisition matrix 64 × 64, and flip angle 70°.
The images from IKEM Prague were acquired on a Siemens Trio Tim 3T: T1W images with repetition time 2,300 ms, echo time 4.63 ms, inversion time 900 ms, slice thickness 1 mm, flip angle 10°, and acquisition matrix 256 × 256 mm; T2∗-weighted images – 300 scans with slice thickness 3 mm, repetition time 2,000 ms, echo time 30 ms, acquisition matrix 64 × 48, and flip angle 70°.
Resting state scans were acquired with eyes closed. All of the subjects were instructed to relax and keep their head still. To ensure that the subjects were comfortable and to minimize the head motion, each of them was provided with a head support and padding.
Individual resting state scans were pre-processed with SPM 8 and CONN v.17 Toolbox (Whitfield-Gabrieli and Nieto-Castanon, 2012). The data underwent standard pre-processing. The functional images were slice-timed, spatially normalized, co-registered to the respective structural scans, smoothed using a Gaussian kernel of FWHM of 8 mm, and band pass filtered (0.008–0.09 Hz). Further, for the connectivity analysis, the data underwent further steps of preprocessing in CONN: denoizing [the original distribution of the connectivity values before denoizing was at a maximum (0.48±0.23, df = 399.0)], after denoizing (0.02±0.16, df = 122.7), and CompCor algorithm discarding motion-related signal changes coming from voxels within white matter and cerebrospinal fluid in the ventricles, cardiac and respiratory fluctuations, and effects of head motion (for details, see Behzadi et al., 2007).
Statistical Analysis
Cluster Solution
For cluster calculation, the raw performance scores in the cognitive tests were converted into z-scores. The z-scores were calculated as the difference among the raw scores of the study subject and the sample mean of a larger sample of healthy controls (N = 90), divided by the standard deviation of the healthy sample. The cumulative test score of each cognitive domain was calculated as a mean of z-scores. The hierarchical cluster analysis (Ward’s cluster method with squared Euclidean distance measurements) was applied as a classification procedure in order to identify groups of study subjects with similar cognitive profiles.
Demographic Variables of the Sample
Demographic variables: gender, education, and time of cognitive assessment were tested using Fischer’s exact test. Age variable, clinical variables DUP, symptomatology, CHLPZ, and QOL were tested with the Kruskal–Wallis test.
Statistical Analysis of Resting State Functional Neuroimaging Data
Functional connectivity was computed using ROI-to-ROI analysis. The ROIs or NOIs were defined by means of the functional seeds and networks (158 ROIs) derived from standardized atlases: atlas.nii/.txt/.info, an atlas of cortical and subcortical areas from the FSL Harvard–Oxford Atlas, as well as cerebellar areas from the AAL atlas and (2) networks.nii/.txt/.info, an atlas of a few commonly used networks and areas (e.g., Default Mode MPFC/PCC/RLP/LLP areas).
In order to evaluate the average effect, a statistical analysis implied MANCOVA with four groups was performed: three groups were defined based on the behavioral cluster analysis and cognitive performance (three groups with respect to three defined clusters) and a control group of healthy individuals with a normal cognitive performance (the controls) in order to evaluate the average effect. A scanner type (1-NUDZ, 0_IKEM) was applied as a covariate. Furthermore, a post hoc analysis was performed to test between-group differences only in the brain seeds that were found to be significant in the original F-test. Multiple comparisons were thresholded at p < 0.05 FDR. Results were presented with an uncorrected p < 0.001 when no results were obtained with an FDR-corrected p < 0.05.
Results
A total of 67 FES subjects were enrolled in this study. Their ages ranged from 17 to 54 (mean, M = 29.4, SD = 7.5). The sample was composed of n = 36 men (53.7%) and n = 31 women (46.3%). The level of education was: n = 10 (14.9%) elementary school; n = 20 (29.9%) vocational school; n = 16 (23.9%) high school; n = 21 (31.3%) university. The percentage of subjects diagnosed with F20 was 55% (N = 37) and with F23 was 45% (N = 30). There was no difference between the subjects diagnosed with F20 and F23.1 in terms of their demographics (Table 1).
The majority of the sample was assessed within the first year after the onset of the illness (n = 48, 71.6%). The cognitive performance in the FES group did not significantly differ between the subjects evaluated during the first year after the onset and those evaluated during 2 years after the first psychotic episode {VISM [F(1.65) = 1.06, p > 0.05]; VERBM [F(1.65) = 0.01, p > 0.05]; SPOP [F(1.65) = 0.50, p > 0.05]; ABSTR [F(1.65) = 0.35, p > 0.05]; FLEX [F(1.65) = 0.00, p > 0.05]; ATTV [F(1.65) = 0.13, p > 0.05]}.
Cluster Solution
The hierarchical cluster analysis (Ward’s cluster method with squared Euclidean distance measurements) on the three predefined clusters of our sample confirmed the existence of various cognitive profiles with different performance levels. The first cluster was represented by a group of FES subjects with a decline in the VERBM domain and the WM/FLEX domain (z-scores below point -1.0). The second cluster was represented by a group of FES subjects with a decline in the VERBM domain only (z-scores below point -1.0) and contrarily this group performed above average in the ATTV domain (z-scores over point 1.0). The third cluster presented a generalized and severe impairment in all cognitive domains (z-scores ranging from -1.21 in the VISM domain to -3.35 in the WM/FLEX domain). The cognitive performance of these three clusters is presented in Figure 2 and the descriptive characteristics of the cognitive domains of the three clusters are presented in Table 2.
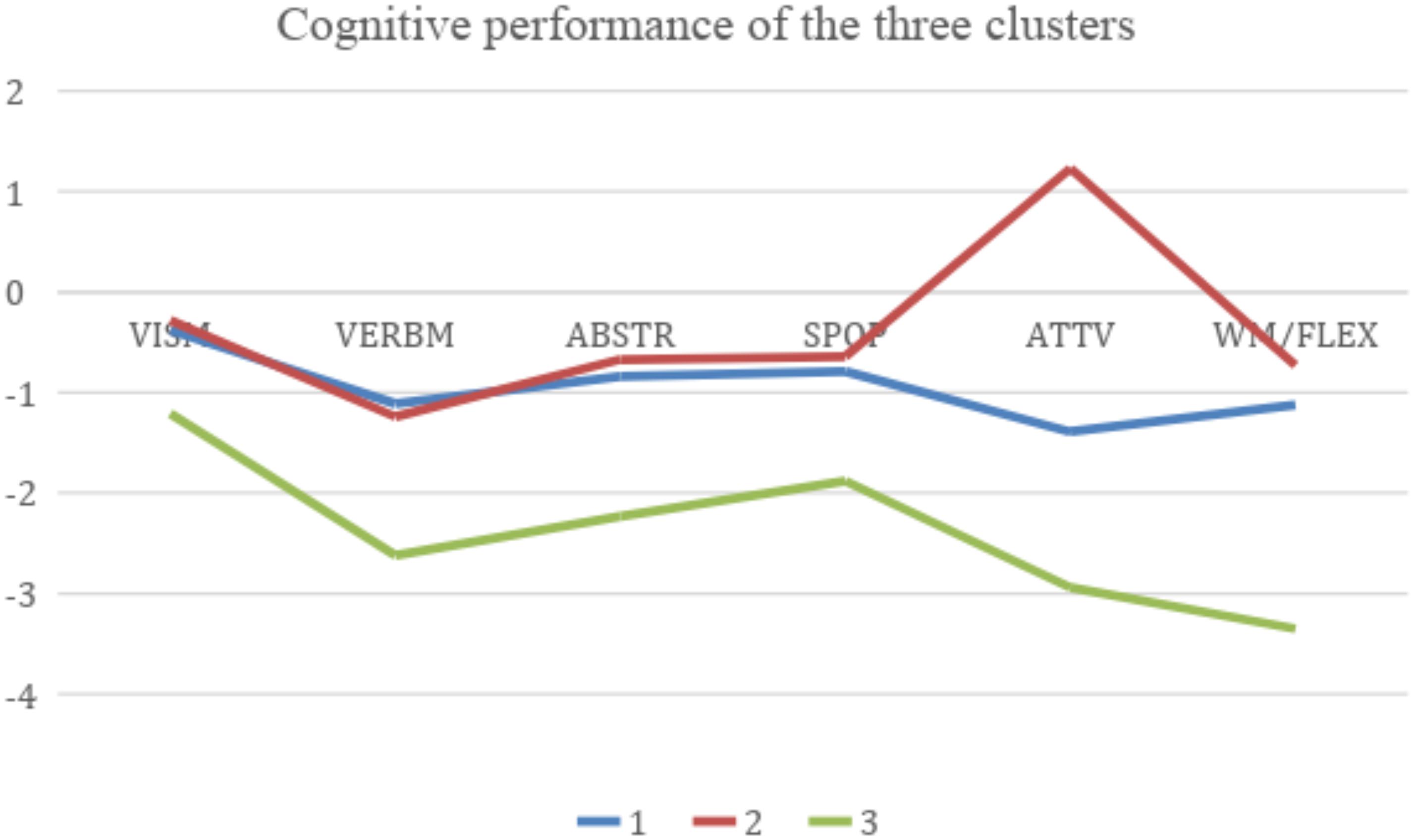
Figure 2. Cognitive performance of the three clusters. Blue line Cluster 1: decline in the VERBM and WM/FLEX domains (z-scores below point -1.0). Red line Cluster 2: decline in the VERBM domain only (z-scores below point -1.0) and above average performance in the ATTV domain (z-scores over point 1.0). Green line: Cluster 3: generalized and severe impairment in all cognitive domains (z-scores ranging from -1.21 in the VISM domain to -3.35 in the WM/FLEX domain). Domains: VISM, visual memory and learning; VERBM, verbal memory and learning; ABSTR/EXEF, abstraction/executive functions; SPOP, speed of processing/psychomotoric speed; ATTV, attention/vigilance; WM/FLEX, working memory/flexibility.
The clusters did not differ in terms of their demographic variables (gender, education, time of cognitive assessment tested using Fischer’s exact test, and age variable tested using the Kruskal–Wallis test), clinical variables (DUP, PANSS, CHLPZ), and QOL (WHOQOL-BREF). The results are presented in Table 3. In addition, there was no difference between the subjects diagnosed with F20 and F23.1 in terms of their cognitive median (Table 4) or cluster distribution (Table 5).
Resting State Connectivity Results
For the subsequent analysis of the corresponding brain functional connectivity patterns in the three cluster groups, we included a control group that consisted of 20 healthy individuals randomly selected from the larger healthy control sample included in the behavioral analysis (z-scores). The groups did not differ in terms of their demographic (Table 6), but education (Table 7) characteristics. There were no differences between groups on cognitive domain characteristics (Table 8). The distribution of the subjects scanned at each site (site 1 and site 2) was as follows: 40% and 60% for Cluster 1; 41% and 59% for Cluster 2; 50% and 50% for Cluster 3; and 50% and 50% for Cluster 4 (control group).
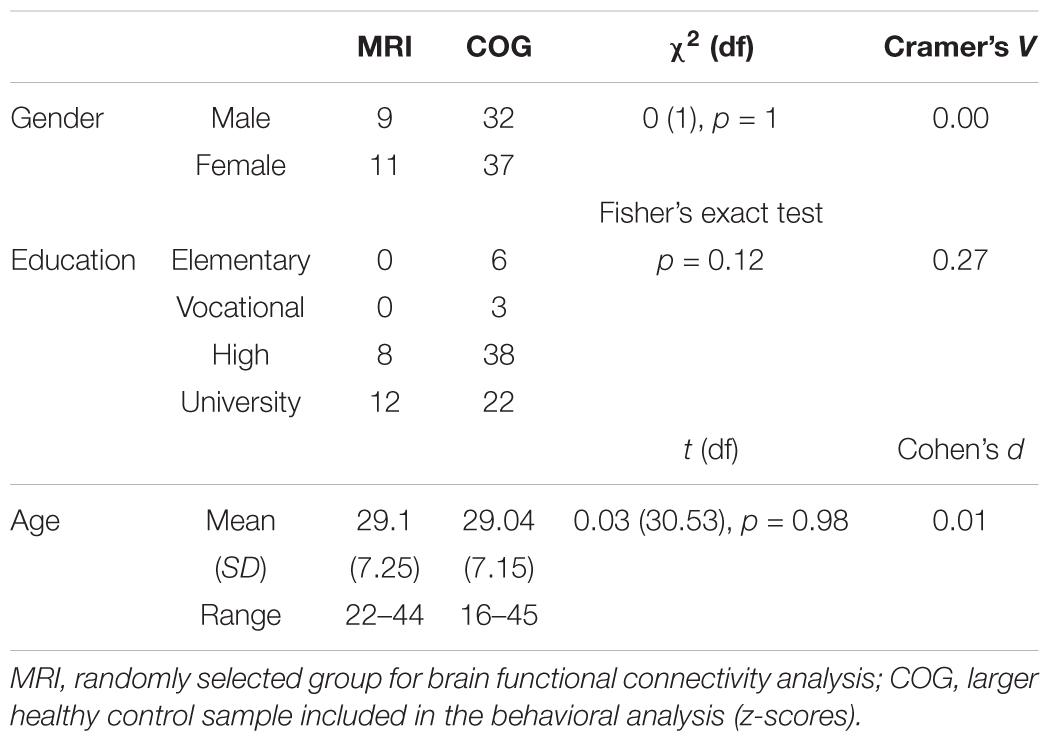
Table 6. Demographic comparison between the selected group for brain functional connectivity analysis and the larger healthy control sample.
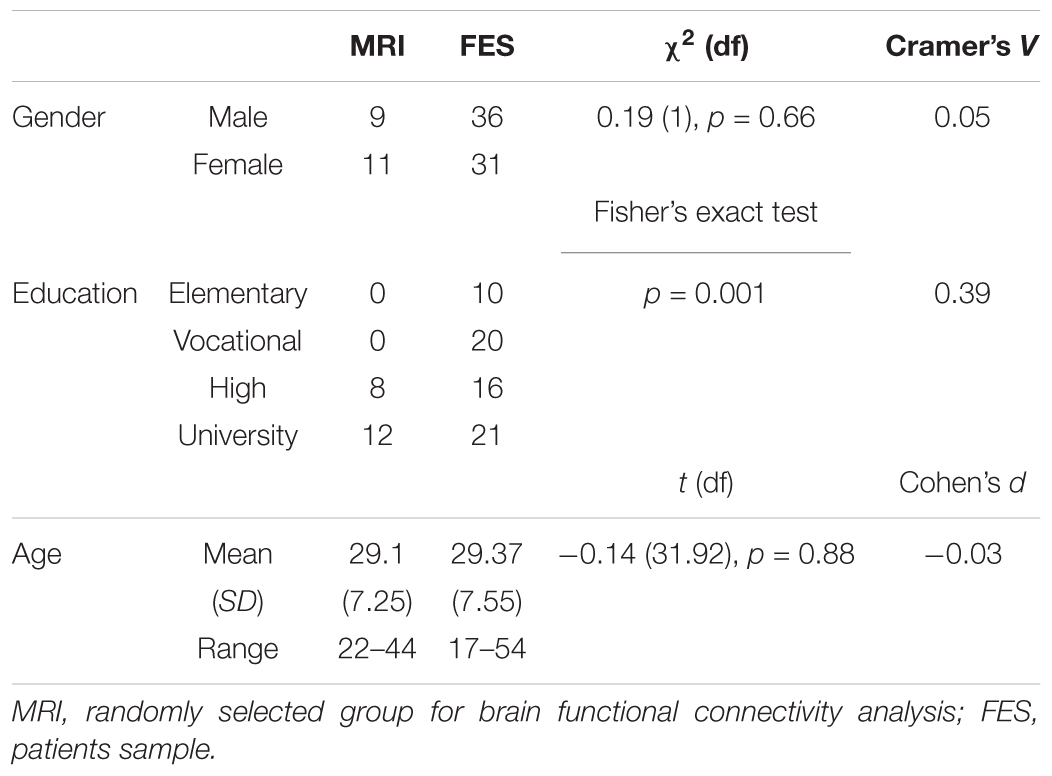
Table 7. Demographic comparison between the selected group of HC for brain functional connectivity analysis and the patients sample.
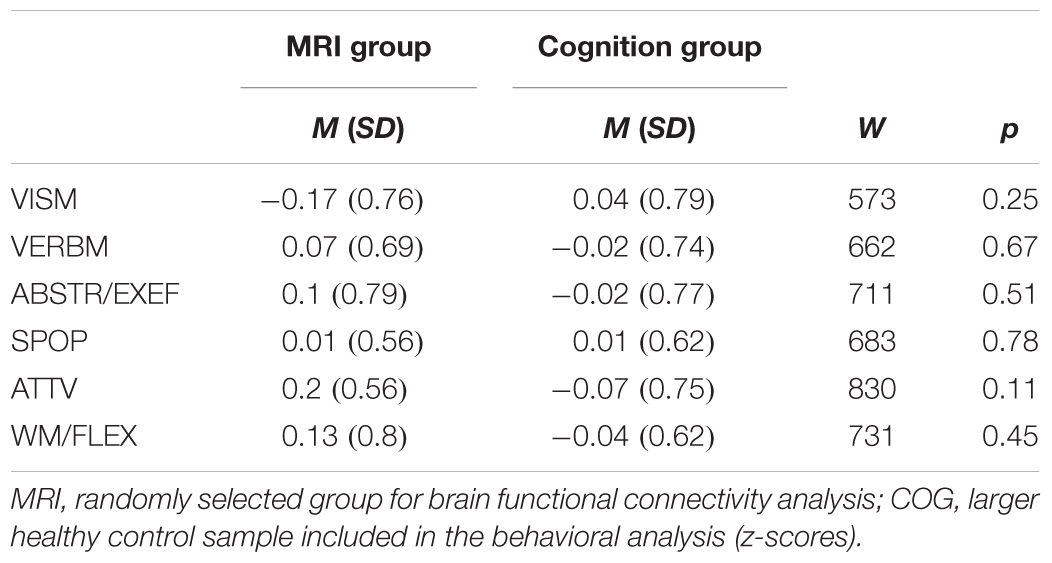
Table 8. Cognitive domain comparison between the selected group for brain functional connectivity analysis and the larger healthy control sample.
The main effect of the group was found between the seeds of the large-scale networks (LSNs) such as the default mode network (DMN); salience network (SN); fronto–parietal network (FPN); and the seeds in the cerebellum, thalamus, somato-motor, and temporal cortices (Figure 3 and Table 9).
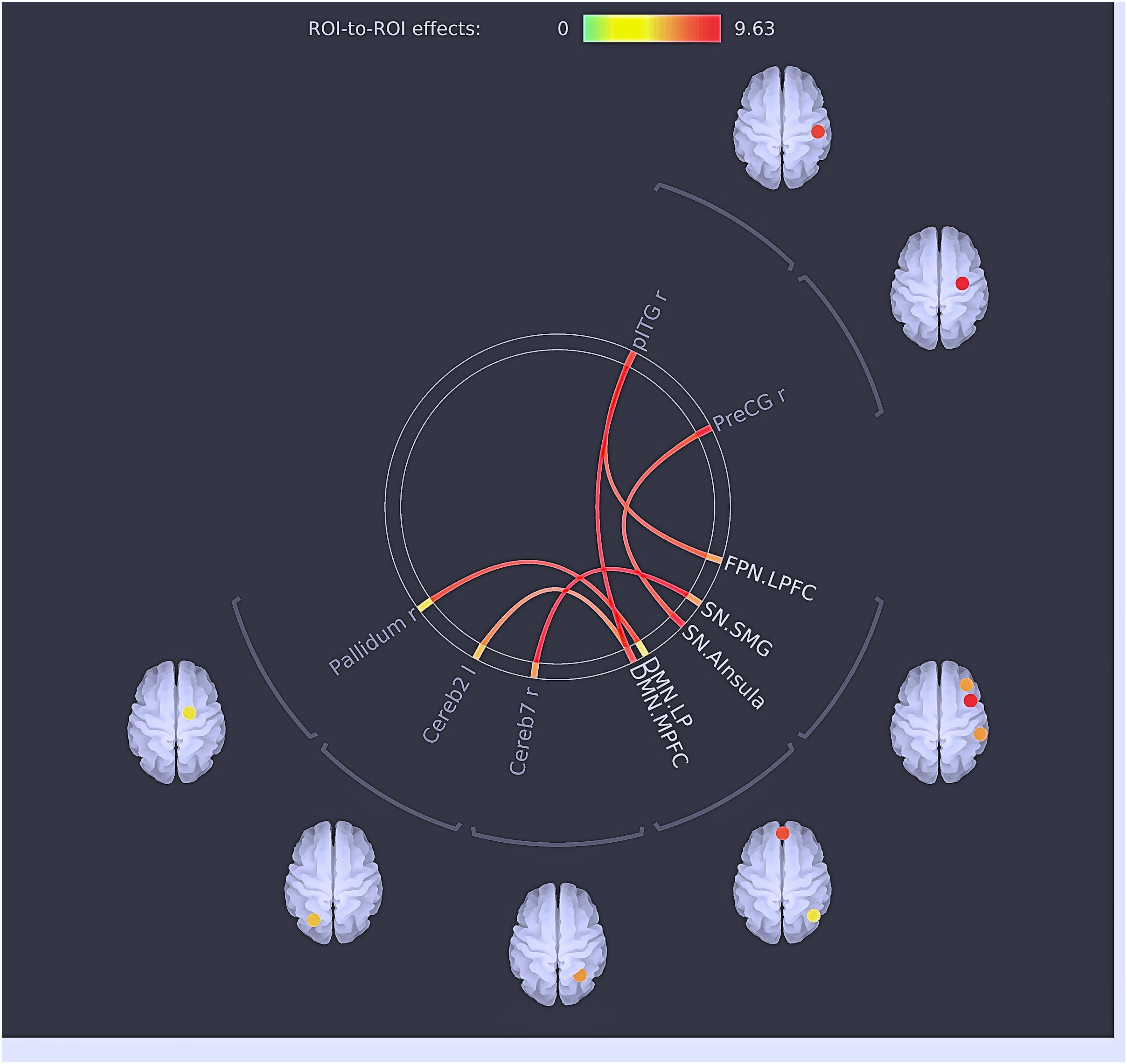
Figure 3. Results of multivariate analysis: the main effects of ROIs. The red lines represent positive connections/effects between ROI.
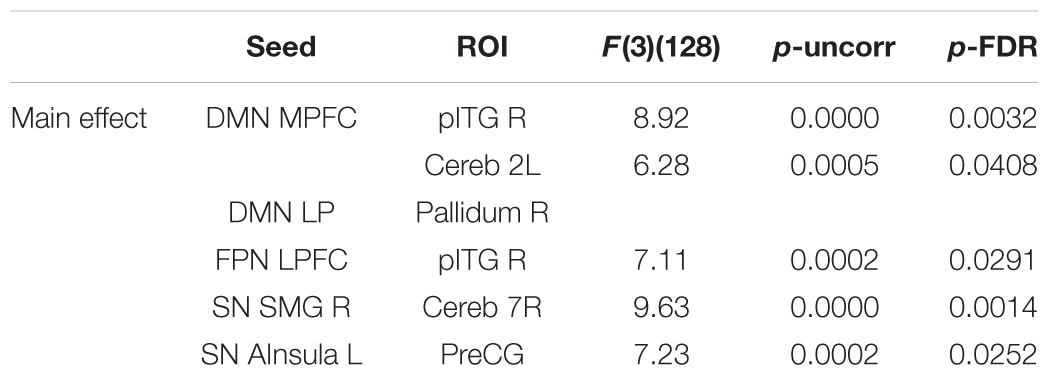
Table 9. Results of the multivariate analysis (MANCOVA) with the cognitive cluster groups and healthy individuals and the scanner type as a covariate.
Post hoc tests revealed significant between-group (clusters) differences, resulting in both hyper- and hypoconnectivity between the subcortical–cortical and cortical–cortical seeds (Figure 4 and Table 10). Clusters 1, 2, and 3 (patient groups) showed a similar pattern of strong positive connectivity between the MPFC and inferior temporal region compared to the HC. Cluster 1 and Cluster 2 differed from the HC in connectivity between the medial prefrontal cortex (MPFC, anterior DMN) and cerebellum; lateral prefrontal cortex (LPFC, FPN) and inferior temporal gyrus (IFG); and anterior insula cortex (part of the SN) and precentral gyrus (PreCG), being higher in the patient groups. In addition, as with Cluster 1, the differences between Cluster 3 and the HC were observed in the hypoconnectivity between the MPFC and anterior insula (FDR uncorr). Also, Cluster 1 demonstrated hyperconnectivity between the supramarginal gyrus (SMG, SN) and seeds in the precentral gyrus and cerebellum, as well as in the anterior insular part and the right pallidum and cerebellum compared to the HC. At the same time, hypoconnectivity between the MPFC and SMG and anterior insula (parts of the SN) was found. In contrast to the HC, Cluster 3 showed hypoconnectivity between the right SMG and the pallidum (FDR uncorr).
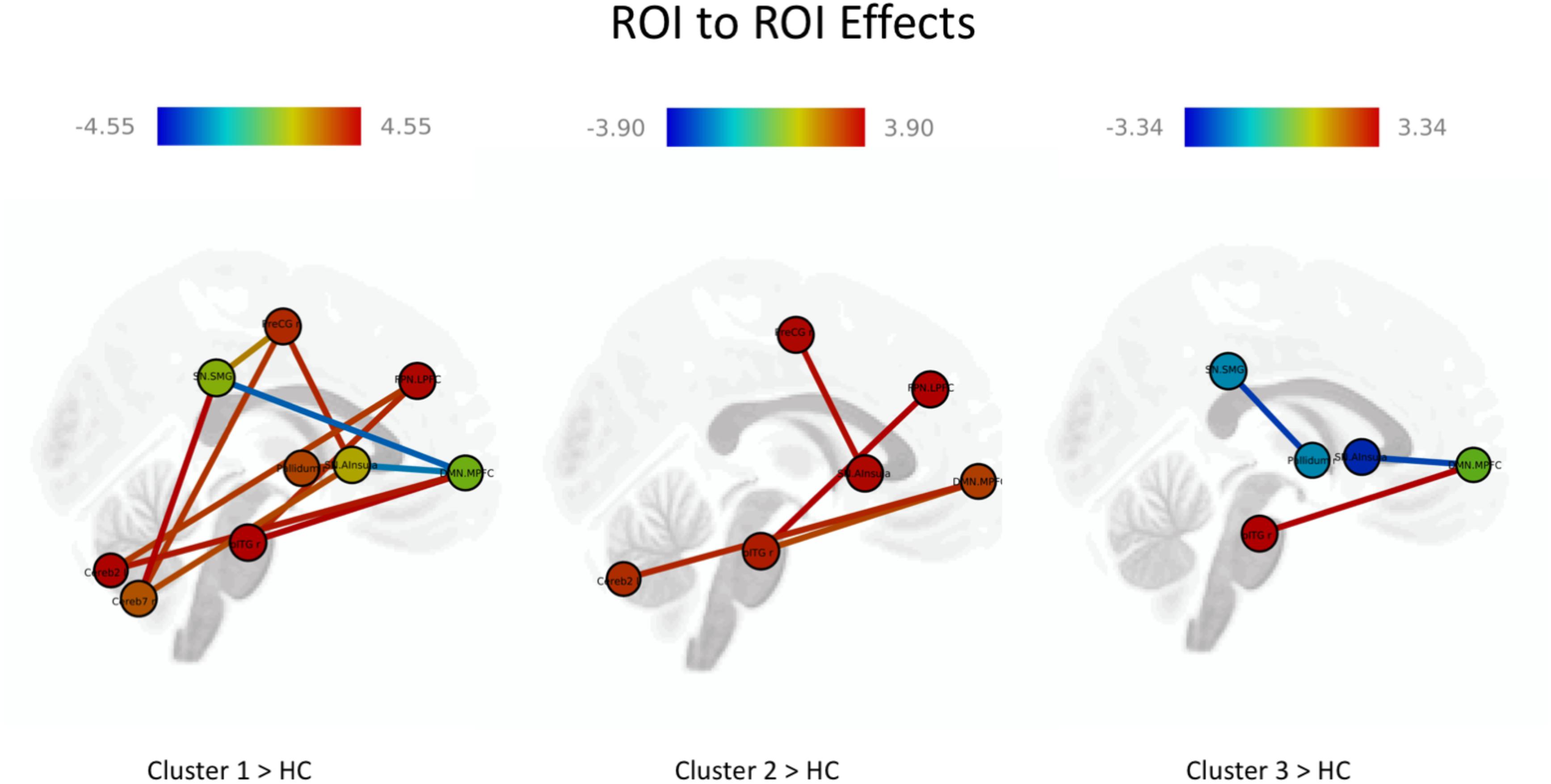
Figure 4. Results of the comparison between the cognitive cluster groups and healthy individuals. The red lines represent positive and blue line negative ROI-to-ROI significant effects. The darker red means a higher number of positive than negative effects and the darker blue a higher number of negative than positive effects.
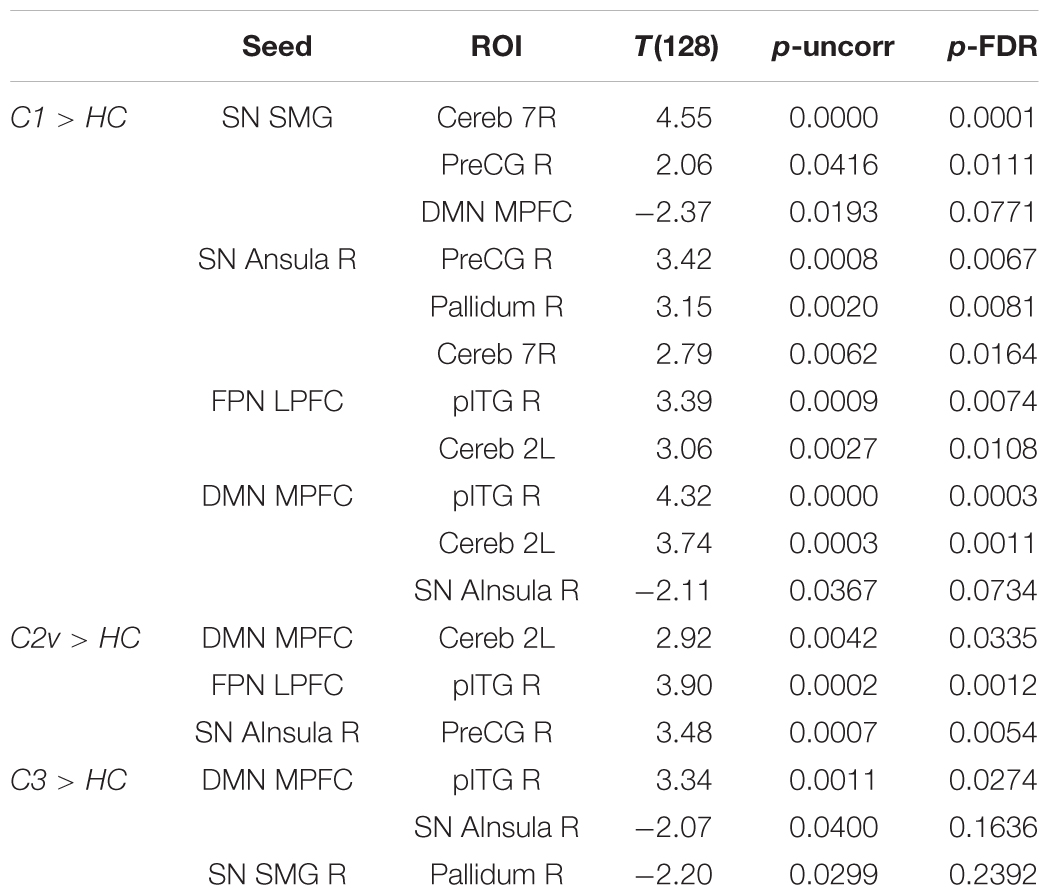
Table 10. Post hoc tests: between-group comparison of cluster groups vs. the group of healthy individuals.
Discussion
Based on the cluster analysis of their cognitive performance, we examined the cognitive profiles of FES subjects, and correlated these profiles with clinical variables and resting state brain connectivity measured with magnetic resonance images.
Our sample consisted of patients with first-episode early stage schizophrenia spectrum disorders, homogeneous in age, education, and gender. In contrast to other studies with chronic patients (Goldstein et al., 1998), we did not find any significant differences between clusters in external variables, such as demographic characteristics, DUP, dosage of medication, or subjective evaluation of their QOL. Moreover, we did not find differences in the sample between individuals with F20 and F23 diagnoses. We find these results consistent with more recent studies that also assessed a sample at first disease onset (e.g., Gilbert et al., 2014) or a sample of mixed psychotic disorders (Lewandowski et al., 2014).
The findings of this study confirmed in three predefined subgroups different cognitive patterns and levels of performance. The presence of various cognitive performance subgroups has been replicated in other schizophrenia studies, regardless of the methodological differences such as the composition of neuropsychological measures and study samples (Seaton et al., 1999; Gilbert et al., 2014). Subgroups of cognitive performance have also been found in first-degree relatives (Quee et al., 2014; Ohi et al., 2017; Rodriguez et al., 2018) and in other psychotic disorders (Lewandowski et al., 2014).
In our study all clusters scored at least 1SD below the average on Verbal Memory domain, and Cluster 2 scored above average in the attention domain. Despite the fact that the cluster analysis of the composition of affected cognitive domains within the clusters and the sample size in our study differed from other studies (e.g., Goldstein et al., 1998; Hill et al., 2002; Gilbert et al., 2014; Ohi et al., 2017), including our previous research (Rodriguez et al., 2017), we identified a subgroup with moderate deficit (Cluster 1), a mild (like-normal) cognitive subgroup (Cluster 2), and a subgroup with a generalized CD (Cluster 3) similarly to previously published findings (Seaton et al., 2001; Gilbert et al., 2014; Rodriguez et al., 2017). Gilbert et al. (2014) also found three clusters (in four domains) based on a defined cognitive decline under the 16th-1SD percentile in the cognitive domains. As in our work, they found a “near-normal functioning cluster,” but not a “normal cluster.” The mild (like-normal) group in our sample yielded a cognitive performance above the average in one domain (the attention domain in Cluster 2). There is a body of evidence suggesting that a highly functioning cognitive subgroup in schizophrenia may perform above average in at least one cognitive domain (Goldstein and Shemansky, 1995; Geisler et al., 2015). In the cluster with generalized and severe deficit (Cluster 3) one of the most impaired domains was verbal memory, and as expected in first episodes, this group was the least represented in the whole sample. The small representation of the sample in this cluster was expected, since the subjects are at the early stages of their illness, and are not affected by the collateral effects of the course of the illness (Volavka and Vevera, 2018). The longitudinal stability of cognitive cluster subtypes becomes later, with the trajectory of the disorder (Heinrichs et al., 1997).
Due the sample size, the number of domains (six), the variables to be analyzed, and in concordance with other previous studies (for e.g., Gilbert et al., 2014; Ohi et al., 2017; Rodriguez et al., 2017; Uren et al., 2017), we pre-defined only three clusters. An increase in the number of subjects analyzed could yield different cluster solutions in future research. Nevertheless, the number of cognitive clusters is more or less consistent across the different studies.
Cluster analyses based on cognitive performance determined the level of performance in our sample. In order to better reflect the external validity of the three subgroups, we analyzed them with neuroimaging variables. Several studies have attempted to do this, uncovering a consistent relationship within the cognitive profiles and brain structure (Heinrichs and Awad, 1993; Ohi et al., 2017), functional connectivity (He et al., 2013; Du et al., 2018), or both (Geisler et al., 2015). However, the majority of these studies did not select a homogenous sample.
In patients, the overall and substantial differences in whole brain connectivity in our study were found between LSNs such as the DMN, SN, FPN and cortical (somato-motor and temporal), subcortical structures (thalamus), and cerebellum. The dysconnectivity in LSNs and its relation to cognitive dysfunction in patients with schizophrenia was initially suggested by Bressler (2002) and later developed by Bressler and Menon (2010). Using multimodal structural and functional imaging approaches, they supported the view that cognition resulted from a dynamic interaction of distributed brain areas operating in LSNs. The patterns of dysconnectivity in three clusters encompass both the hyper- and hypoconnectvity of brain networks. Recent studies have frequently reported either hypoconnectivity or hyperconnectivity of brain networks in patients with schizophrenia and schizophrenia spectrum disorders (for a review see Dong et al., 2018). It seems that both decreased and increased connectivity result in the worse cognitive performance. As Skudlarski et al. (2010) suggest, increased connectivity may indicate an increased neural effort due to the presence of structural damage in certain brain structures, while a decrease in the connectivity would point to a different pattern or the decoupling of structural and functional connections (Skudlarski et al., 2010). In this regard, the increased connection between the medial frontal gyrus (DMN) and the IFG may be explained by potential structural changes in the temporal gyrus, a finding that is repeatedly described in schizophrenia patients (Onitsuka et al., 2004; Kuroki et al., 2007). At the same time, a hyperconnectivity pattern between the IFG and the medial frontal cortex (anterior seed of DMN) presumably indicates a lack of suppression (Seidman et al., 2014) exclusively pronounced in patients with CDs (Zhou et al., 2016). This assumption could be tested in a future study.
Cluster 1 and Cluster 2 shared a common hyperconnectivity between the MPFC (DMN) and the cerebellum. While examining the functional connectivity between the task-responsive parts of the cerebellum, Brissenden et al. (2016) concluded that cerebellum-to-cortex functional connectivity strongly predicted the pattern of cortical activation during attentional task performance. Increased connectivity between the DMN and cerebellar seeds found by Guo et al. (2015, 2018) in patients with schizophrenia and their siblings was suggested to be a potential endophenotype for schizophrenia. Another commonality that was shared by the relatively similar cognitive Clusters 1 and 2 is hyperconnectivity between the anterior insula cortex (SN) and the IFG. This particular connection was hypothesized by Tops and Boksem (2011) for the elaboration of attentional and WM processing. As they assume, the SN–IFG connection aims to facilitate fast and accurate responses, but it may cause slow responses, or it may interfere with the accuracy and speed of performance in the next trial when processing of the next stimulus follows prolonged elaborate processing. Altered connectivity between the anterior insula and auditory cortices has been shown to be significantly associated with cognitive impairment (Tian et al., 2018). Another pattern of increased connections between the anterior insula and the somatosensory cortex, and specifically the precentral gyrus, was reported by Tian et al. (2018). Although the authors did not draw parallels with symptoms or cognitive functioning, such a pattern may reflect talk-level control deficits and/or difficulties in focal attention processing (Nelson et al., 2010).
Frontal–parietal network connectivity, altered in both Cluster 1 and Cluster 2, is considered to be a flexible hub adapting to old and novel tasks (Fassbender et al., 2006; Zanto and Gazzaley, 2013) and is associated with cognitive control. Altered connectivity in schizophrenia patients was shown to be linked to WM deficits and notably to a failure of context-sensitive coupling (Nielsen et al., 2017). At the same time, fronto-temporal connections, as suggested by Kobayashi (2009), are associated with visual recognition and memory. Specifically, the ITG plays an important role in linking visual stimuli with a reward outcome.
In Clusters 1 and 3, we identified similar patterns of hypoconnectivity between the MPFC (DMN) and the anterior insula (SN). A similar finding was reported in the study of Manoliu et al. (2013) demonstrating a decreased temporal dependence of DMN activity on SN activity in patients with schizophrenia during acute psychosis. However, the study of Wang et al. (2015) reports the opposite results. With respect to cognitive performance, the degree of disruption of the SN that modulates the DMN and central executive networks correlates with lower cognitive performance in an aging population (Chand et al., 2017), although no evidence has been provided in schizophrenia.
Exclusively for Cluster 1, we identified hyperconnectivity between the FPN (LPFC) and the cerebellum. This is in line with the findings of Kim et al. (2017) who demonstrated an increased fronto-cerebellar connectivity (r = 0.57, p < 0.001). With respect to cognitive performance, together with the FPN, the cerebellum and specifically its posterior part play a role in high cognitive processing, i.e., motor control, subsequently updating actual and mental motor performance (Bonzano et al., 2016). Another finding that was prominent only in the first cluster is hypoconnectivity between the SMG and the DMN. The SMG supports the role in multiple cognitive domains such as visual word recognition in memory tasks (Stoeckel et al., 2009), behavioral switching (Jubault et al., 2007), or decision-making (Boorman and Rushworth, 2009). In schizophrenia, the SMG shows a significant gray matter reduction (Palaniyappan and Liddle, 2012), thereby affecting cognitive processing.
Hypoconnectivity of the SMN and basal ganglia (pallidum) was found exclusively in Cluster 3. Decreased activity of the pallidum has been reported to be associated with processing speed alterations in patients with schizophrenia (Mwansisya et al., 2013); however, in such an association it may impact VISM processing.
Taken together, using explorative correlation analysis of the whole brain connectome, we were able to identify three patterns of connectivity, specifically in the brain networks and seeds that are related to the interactions of the attentional and memory systems. These three clusters were associated with different constellations of CDs that differed mostly in attention and FLEX.
Limitations
The main limitations in our study include the sample size for the cluster analysis and the unclear stability of the clusters over time. In a larger sample we could predefine more clusters, which could reveal better differences in cognitive performance. We analyzed a sample of patients with FES. Since the subjects are in their early stage, we do not know the course of their illness yet. The longitudinal stability of cognitive cluster subtypes becomes apparent later, with the trajectory of the disorder (Heinrichs et al., 1997). Our next study will attempt to cover these limitations. We will increase the sample size, and will assess the sample after 1 year in order to follow-up the course. Lastly, given that the clusters could not be directly matched to the whole brain connectivity patterns and were only investigated by comparing the groups in the form of an explorative analysis, the results should be interpreted with caution. Our limited sample size may have prevented us from finding smaller differences between the cluster groups, though by adding uncorrected results we highlighted the directionality (positive or negative) of the connectivity patterns in three cluster groups that impacted the cognitive performance (discussed above). Besides, the control group was matched only by age and gender, the education level was lower in patients. Indeed, the education level was shown to increase brain efficacy in healthy individuals (Marques et al., 2015). The effect of education will be considered in the future research.
In conclusion, the cluster solution confirms the prevalence of a cognitive decline in FESs with different patterns of cognitive performance, and different levels of severity. Moreover, separate behavioral cognitive subsets can be linked to patterns of brain functional connectivity.
Data Availability
The datasets generated for this study are available on request to the corresponding author.
Ethics Statement
The study was conducted at the National Institute of Mental Health, Czech Republic; the study protocol was approved by the Ethical Committee of the institution: Etická Komise NÚDZ. All of the study subjects signed an informed consent form before the enrolment.
Author Contributions
MR designed the study and wrote the original protocol together with ZK, FS, and YZ. AD, JJ, PS, VV, and MH recruited the participants, and performed the neuropsychological and fMRI assessment. AC, BD, and YZ pre-processed the data and performed the statistical analysis. MR supervised the study. MR, YZ, and PM wrote the first draft of the manuscript and contributed to the data interpretation. All of the authors discussed the results and contributed to the final version of the manuscript and have approved it.
Funding
This study is a result of the research funded by the project No. 16-13093S “Cognitive profiles in patients with first-episodes of schizophrenia spectrum disorders and their relationship with daily functioning” with the financial support of the Grant Agency of the Czech Republic (GACR), the project No. 15-28998A “Endophenotypes of psychotic disorders” with the financial support of the “Ministry of Health of the Czech Republic” and and by the project “Sustainability for the National Institute of Mental Health”, under grant number LO1611, with a financial support from the Ministry of Education, Youth and Sports of the Czech Republic under the NPU I program.
Conflict of Interest Statement
The authors declare that the research was conducted in the absence of any commercial or financial relationships that could be construed as a potential conflict of interest.
Acknowledgments
We are grateful to the ESO team members for their assistance in the collection of parts of the clinical data.
References
Andreasen, N. C., Carpenter, W. T., and Kane, J. M. (2005). Remission in schizophrenia: proposed criteria and rationale for consensus. Am. J. Psychiatry 162, 441–449. doi: 10.1176/appi.ajp.162.3.441
Andreasen, N. C., O’Leary, D. S., Cizadlo, T., Arndt, S., Rezai, K., Ponto, L. L., et al. (1996). Schizophrenia and cognitive dysmetria: a positron-emission tomography study of dysfunctional prefrontal-thalamic-cerebellar circuitry. Proc. Natl. Acad. Sci. U.S.A. 93, 9985–9990. doi: 10.1073/pnas.93.18.9985
Behzadi, Y., Restom, K., Liau, J., and Liu, T. T. (2007). A component based noise correction method (CompCor) for BOLD and perfusion based fMRI. Neuroimage 37, 90–101. doi: 10.1016/j.neuroimage.2007.04.042
Bonzano, L., Roccatagliata, L., Ruggeri, P., Papaxanthis, C., and Bove, M. (2016). Frontoparietal cortex and cerebellum contribution to the update of actual and mental motor performance during the day. Sci. Rep. 6:30126. doi: 10.1038/srep30126
Boorman, E. D., and Rushworth, M. F. (2009). Conceptual representation and the making of new decisions. Neuron 63, 721–723. doi: 10.1016/j.neuron.2009.09.014
Bora, E., and Murray, R. M. (2014). Meta-analysis of cognitive deficits in ultra-high risk to psychosis and first-episode psychosis: do the cognitive deficits progress over, or after, the onset of psychosis? Schizophr. Bull. 40, 744–755. doi: 10.1093/schbul/sbt085
Bora, E., Yücel, M., and Pantelis, C. H. (2009). Cognitive functioning in schizophrenia, schizoaffective disorder and affective psychoses: meta-analytic study. Br. J. Psychiatry 195, 475–482. doi: 10.1192/bjp.bp.108.055731
Bora, E., Yücel, M., and Pantelis, C. H. (2010). Cognitive impairment in schizophrenia and affective psychoses: implications for DSM-V criteria. Schizophr. Bull. 36, 36–42. doi: 10.1093/schbul/sbp094
Bowie, C. R., Reichenberg, A., Patterson, T. L., Heaton, R. K., and Harvey, P. D. (2006). Determinants of real-world functional performance in schizophrenia subjects: correlations with cognition, functional capacity, and symptoms. Am. J. Psychiatry 163, 418–425. doi: 10.1176/appi.ajp.163.3.418
Bressler, S. L. (2002). Understanding cognition through large-scale cortical networks. Curr. Dir. Psychol. Sci. 11, 58–61. doi: 10.1111/1467-8721.00168
Bressler, S. L., and Menon, V. (2010). Large-scale brain networks in cognition: emerging methods and principles. Trends Cogn. Sci. 14, 277–290. doi: 10.1016/j.tics.2010.04.004
Brissenden, J. A., Levin, E. J., Osher, D. E., Halko, M. A., and Somers, D. (2016). Functional evidence for a cerebellar node of the dorsal attention network. J. Neurosci. 36, 6083–6096. doi: 10.1523/JNEUROSCI.0344-16.2016
Chand, G. B., Wu, J., Hajjar, I., and Qui, D. (2017). Interactions of the salience network and its subsystems with the default mode and the central executive networks in normal aging and mind cognitive impairment. Brain Connect. 7, 401–412. doi: 10.1089/brain.2017.0509
Dickinson, D., Ragland, J. D., Calkins, M. E., Gold, J. M., and Gur, R. C. (2006). A comparison of cognitive structure in schizophrenia patients and healthy controls using confirmatory factor analysis. Schizophr. Res. 85, 20–29. doi: 10.1016/j.schres.2006.03.003
Dong, D., Wang, Y., Chang, X., Luo, C., and Yao, D. (2018). Dysfunction of large scale networks in schizophrenia: a meta-analysis of resting state functional connectivity. Schizophr. Bull. 44, 168–181. doi: 10.1093/schbul/sbx034
Dragomirecká, E., and Bartonová, J. (2006). WHOQOL-BREF. WHOQOL-100. Příručka pro Uživatele České Verze Dotazníku Kvality Života Světové Zdravotnické Organizace. Praha: Psychiatrické centrum Praha.
Du, Y., Fu, Z., and Calhoun, V. D. (2018). Classification and prediction of brain disorders using functional connectivity: promising but challenging. Front. Neurosci. 12:525. doi: 10.3389/fnins.2018.00525
Fassbender, C., Simoes-Franklin, C., Murphy, K., Hester, R., Meaney, J., Robertson, I. H., et al. (2006). The role of the right fronto-parietal network in cognitive control: common activations for “cues-to-attend” and response inhibition. J. Psychophys. 20, 286–296. doi: 10.1027/0269-8803.20.4.286
Fatouros-Bergman, H., Cervenka, S., Flyckt, L., Edman, G., and Farde, L. (2014). Meta-analysis of cognitive performance in drug-naive patients with schizophrenia. Schizophr. Res. 158, 156–162. doi: 10.1016/j.schres.2014.06.034
Fioravanti, M., Bianchi, V., and Cinti, M. E. (2012). Cognitive deficits in schizophrenia: an updated metanalysis of the scientific evidence. BMC Psychiatry 12:64. doi: 10.1186/1471-244X-12-64
Fornito, A., Yoon, J., Zalesky, A., Bullmore, E. T., and Carter, C. S. (2011). General and specific functional connectivity disturbances in first-episode schizophrenia during cognitive control performance. Biol. Psychiatry 70, 64–72. doi: 10.1016/j.biopsych.2011.02.019
Fusar-Poli, P., Deste, G., Smieskova, R., Barlati, S., Yung, A., Howes, A., et al. (2012). Cognitive functioning in prodromal psychosis: a meta-analysis. Arch. Gen. Psychiatry 69, 562–571. doi: 10.1001/archgenpsychiatry.2011.1592
Geisler, D., Walton, E., Naylor, M., Roessner, V., Lim, K. O., Schulz, S. C., et al. (2015). Brain structure and function correlates of cognitive subtypes in schizophrenia. Psychiatry Res. 234, 74–83. doi: 10.1016/j.pscychresns.2015.08.008
Gilbert, E., Merette, C., Jomphe, V., Emond, C., Rouleau, N., Bouchard, R. H., et al. (2014). Cluster analysis of cognitive deficits may mark heterogeneity in schizophrenia in terms of outcome and response to treatment. Eur. Arch. Psychiatry Clin. Neurosci. 264, 333–343. doi: 10.1007/s00406-013-0463-7
Goldstein, G., Allen, D. N., and Seaton, B. E. (1998). A comparison of clustering solutions for cognitive heterogeneity in schizophrenia. J. Int. Neuropsychol. Soc. 4, 353–362.
Goldstein, G., and Shemansky, W. J. (1995). Influences on cognitive heterogeneity in schizophrenia. Schizophr. Res. 18, 59–69. doi: 10.1016/0920-9964(95)00040-2
Guo, W., Liu, F., Chen, J., Wu, R., Zhan, Z., Yu, M., et al. (2015). Resting state cerebellar-cerebral networks are differently affected in first episode, drug-naïve schizophrenia patients and unaffected siblings. Sci. Rep. 5:17275. doi: 10.1038/srep17275
Guo, W., Zhang, F., Liu, F., Chen, J., Wu, R., Chen, D. Q., et al. (2018). Cerebellar abnormalities in first episode, drug-naive schizophrenia at rest. Psychiatry Res. Neuroimaging 276, 73–79. doi: 10.1016/j.pscychresns.2018.03.010
He, Z., Deng, W., Li, M., Chen, Z., Jiang, L., Wang, Q., et al. (2013). Aberrant intrinsic brain activity and cognitive deficit in first episode treatment-naive patients with schizophrenia. Psychol. Med. 43, 769–780. doi: 10.1017/S0033291712001638
Heilbronner, U., Samara, M., Leucht, S., Falkai, P., and Schulze, T. G. (2016). The longitudinal course of schizophrenia across the lifespan: clinical, cognitive, and neurobiological aspects. Harv. Rev. Psychiatry 24, 118–128. doi: 10.1097/HRP.0000000000000092
Heinrichs, R., and Zaksanis, K. (1998). Neurocognitive deficit in schizophrenia: a quantitative review of the evidence. Neuropsychology 12, 426–445. doi: 10.1037/0894-4105.12.3.426
Heinrichs, R. W., and Awad, A. G. (1993). Neurocognitive subtypes of chronic schizophrenia. Schizophr. Res. 9, 49–58. doi: 10.1016/0920-9964(93)90009-8
Heinrichs, R. W., Ruttan, L., Zakzanis, K. K., and Case, D. (1997). Parsing schizophrenia with neurocognitive tests: evidence of stability and validity. Brain Cogn. 35, 207–224. doi: 10.1006/brcg.1997.0938
Hill, K. S., Ragland, J. D., Gur, R. C., and Gur, R. E. (2002). Neuropsychological profiles delineate distinct profiles of schizophrenia, and interaction between memory and executive function, and uneven distribution of clinical subtypes. J. Clin. Exp. Neuropsychol. 24, 765–780. doi: 10.1076/jcen.24.6.765.8402
Jubault, T., Ody, C., and Koechlin, E. (2007). Serial organization of human behaviour in the inferior parietal cortex. J. Neurosci. 27, 11028–11036. doi: 10.1523/JNEUROSCI.1986-07.2007
Kay, S. R., Fiszbein, A., and Opler, L. A. (1987). The positive and negative syndrome scale (PANSS) for schizophrenia. Schizophr. Bull. 1987, 261–276. doi: 10.1093/schbul/13.2.261
Kim, D. J., Bartolomeo, L., Bolbecker, A., Lundin, N., Purcell, J., Moussa-Tooks, A., et al. (2017). Abnormal cortico-cerebellar resting state connectivity in sensory-motor networks in schizophrenia. Proc. Int. Congr. Schizophr. Res. 43(Suppl. 1):S238. doi: 10.1093/schbul/sbx022.071
Kobayashi, S. (2009). Reward Neurophysiology and Primate Cerebral Cortex/Temporal Cortex in Encyclopedia of Neuroscience, eds J. R. Davis, A. C. Giles, C. H. Rankin, J. Bell, H. Kimura, T. Uemura, et al. (Berlin: Springer). doi: 10.1007/978-3-540-29678-2_3
Kuroki, N., Shenton, M., Salisbury, D. F., Hirayasu, Y., Onitsuka, T., Hershfield, H., et al. (2007). Middle and inferior temporal gyrus gray matter volume abnormalities in first-episode schizophrenia: an MRI study. Am. J. Psychiatry 163, 2103–2110. doi: 10.1176/ajp.2006.163.12.2103
Lewandowski, K. E., Sperry, S. H., Cohen, B. M., and Öngür, D. (2014). Cognitive variability in psychotic disorders: a cross-diagnostic cluster analysis. Psychol. Med. 44, 3239–3248. doi: 10.1017/S0033291714000774
Liang, S. G., and Greenwood, T. A. (2015). The impact of clinical heterogeneity in schizophrenia on genomic analyses. Schizophr. Res. 161, 490–495. doi: 10.1016/j.schres.2014.11.019
Manoliu, A., Meng, C., Brandl, F., Doll, A., Tahmasian, M., Scherr, M., et al. (2013). Insular dysfunction reflects altered between-network connectivity and severity of negative symptoms in schizophrenia during psychotic remission. Front. Hum. Neurosci. 7:216. doi: 10.3389/fnhum.2013.00216
Marques, P., Soares, J. M., Magalhaes, R., Santos, N. C., and Sousa, N. (2015). The bounds of education in the human brain connectome. Sci. Rep. 5:12812. doi: 10.1038/srep12812
Mesholam-Gately, R. I., Giuliano, A. J., Goff, K. P., Faraone, S. V., and Seidman, L. J. (2009). Neurocognition in first-episode schizophrenia: a meta-analytic review. Neuropsychology 23, 315–336. doi: 10.1037/a0014708
Mwansisya, T. E., Wang, Z., Tao, H., Zhang, H., Hu, A., Guo, S., et al. (2013). The diminished interhemispheric connectivity correlates with negative symptoms and cognitive impairment in first-episode schizophrenia. Schizophr. Res. 150, 144–150. doi: 10.1016/j.schres.2013.07.018
Nelson, S., Dosenbach, N. U. F., Cohen, A. L., Wheeler, M. E., and Schlaggar, B. L. (2010). Role of the anterior insula in task-level control and focal attention. Brain Struct. Funct. 2014, 669–680. doi: 10.1007/s00429-010-0260-2
Nielsen, J. D., Madsen, K. H., Wang, Z., Liu, Z., Friston, K. J., and Zhou, Y. (2017). Working memory modulation in frontoparietal network connectivity in first episode of schizophrenia. Cereb. Cortex 27, 3832–3841. doi: 10.1093/cercor/bhx050
Nuechterlein, K. H., Barch, D. M., Gold, J. M., Goldberg, T. E., Green, M. F., and Heaton, R. K. (2004). Identification of separable cognitive factors in schizophrenia. Schizophr. Res. 72, 29–39. doi: 10.1016/j.schres.2004.09.007
Nuechterlein, K. H., Green, M. F., Kern, R. S., Baade, L. E., Barch, D. M., Cohen, J. D., et al. (2008). The MATRICS Consensus Cognitive Battery, part 1: test selection, reliability, and validity. Am. J. Psychiatry 165, 203–213. doi: 10.1176/appi.ajp.2007.07010042
Ohi, K., Shimada, T., Nemoto, K., Kataoka, Y., Yasuyama, T., Kimura, K., et al. (2017). Cognitive clustering in schizophrenia patients, their first-degree relatives and healthy subjects is associated with anterior cingulate cortex volume. Neuroimage Clin. 16, 248–256. doi: 10.1016/j.nicl.2017.08.008
Onitsuka, T., Shenton, M., Salsbery, D. F., Dickey, C. C., Kasai, K., Toner, S. K., et al. (2004). Middle and inferior temporal gyrus gray matter volume abnormalities in chronic schizophrenia: an fMRI study. Am. J. Psychiatry 161, 1603–1611. doi: 10.1176/appi.ajp.161.9.1603
Owen, M. J., Sawa, A., and Mortensen, P. B. (2016). Schizophrenia. Lancet 388, 86–97. doi: 10.1016/S0140-6736(15)01121-6
Palaniyappan, L., and Liddle, P. F. (2012). Dissociable morphometric differences of the inferior parietal lobule in schizophrenia. Eur. Arch. Psychiatry Clin. Neurosci. 262, 579–587. doi: 10.1007/s00406-012-0314-y
Quee, P. J., Alizadeh, B. Z., Aleman, A., van den Heuvel, E. R., and Group Investigators (2014). Cognitive subtypes in non-affected siblings of schizophrenia patients: characteristics and profile congruency with affected family members. Psychol. Med. 44, 395–405. doi: 10.1017/S0033291713000809
Rodriguez, M., Fajnerová, I., Sedláková, K., Dorazilová, A., Vorácková, V., Paštrnák, M., et al. (2017). Cluster analysis and correlations between cognitive domains: cognitive performance in a Czech sample of first episodes schizophrenia spectrum disorders - Preliminary results. Psychiatrie 21, 4–11.
Rodriguez, M., Vorácková, V., Knytl, P., Šustová, P., Dorazilová, A., Cvrčková, A., et al. (2018). Cognitive profile of healthy siblings of patients with first-episode psychosis as a candidate endophenotype. Nice, France, 03.03.2018 - 06.03.2018. Eur. Psychiatry 48:S129.
Seaton, B. E., Allen, D. N., Goldstein, G., Kelley, M. E., and van Kammen, D. P. (1999). Relations between cognitive and symptom profile heterogeneity in schizophrenia. J. Nerv. Ment. Dis. 187, 414–419. doi: 10.1097/00005053-199907000-00004
Seaton, B. E., Goldstein, G., and Allen, D. N. (2001). Sources of heterogeneity in schizophrenia: the role of neuropsychological functioning. Neuropsychol. Rev. 11, 45–67. doi: 10.1023/A:1009013718684
Seidman, L. J., Rosso, I. M., Thermenos, H. W., Markis, N., Juelish, R., and Gabrieli, J. D. (2014). Medial temporal lobe default mode functioning and hippocampal structure as vulnerability indicators for schizophrenia: a MRI study of non-psychotic adolescent first-degree relatives. Schizophr. Res. 159, 426–434. doi: 10.1016/j.schres.2014.09.011
Sheffield, J. M., and Barch, D. M. (2016). Cognition and resting-state functional connectivity in schizophrenia. Neurosci. Biobehav. Rev. 61, 108–120. doi: 10.1016/neubiorev.2015.12.007
Skudlarski, P., Jagannathan, K., Anderson, K., Stevens, M. C., Calhoun, V. D., Skudlarska, B. A., et al. (2010). Brain connectivity is not only lower but different in schizophrenia: a combined anatomical and functional approach. Biol. Psychiatry 68, 61–69. doi: 10.1016/j.biopsych.2010.03.035
Stephan, K. E., Mattout, J., David, O., and Friston, K. J. (2006). Models of functional neuroimaging data. Curr. Med. Imaging Rev. 2, 15–34. doi: 10.2174/157340506775541659
Stoeckel, C., Gough, P. M., Watkins, K. E., and Delvin, J. T. (2009). Supramarginal gyrus involvement in visual word recognition. Cortex 45, 1091–1096. doi: 10.1016/j.cortex.2008.12.004
Szöke, A., Trandafir, A., Dupont, M., Méary, A., Schürhoff, F., and Leboyer, M. (2008). Longitudinal studies of cognition in schizophrenia: meta-analysis. Br. J. Psychiatry 192, 248–257. doi: 10.1192/bjp.bp.106.029009
Tandon, R., Nasrallah, H. A., and Keshavan, M. S. (2009). Schizophrenia, “just the facts” 4. Clinical features and conceptualization. Schizophr. Res. 110, 1–23. doi: 10.1016/j.schres.2009.03.005
Tian, Y., Zalesky, A., Bousman, C., Everall, I., and Pantelis, C. (2018). Insula functional connectivity in schizophrenia: subregions, gradients and symptoms. Biol. Psychiatry doi: 10.1016/j.bpsc.2018.12.003 [Epub ahead of print].
Tops, M., and Boksem, M. A. S. (2011). A potential role of the inferior frontal gyrus and anterior insula in cognitive control, brain rhythms, and event-related potentials. Front. Psychol. 2:330. doi: 10.3389/fpsyg.2011.00330
Uren, J., Cotton, S. M., Killackey, E., Saling, M. M., and Allott, K. (2017). Cognitive clusters in first-episode psychosis: overlap with healthy controls and relationship to concurrent and prospective symptoms and functioning. Neuropsychology 31, 787–797. doi: 10.1037/neu0000367
Volavka, J., and Vevera, J. (2018). Very long-term outcome of schizophrenia. Int. J. Clin. Pract. 72:e13094. doi: 10.1111/ijcp.13094
Wang, H. L., Huang, H., Chen, C., Li, P. F., Zhou, Y., and Jiang, T. Z. (2015). Aberrant functional connectivity within and across the default mode, central-executive and salience network in patients with schizophrenia: a resting state fMRI study. Eur. Psychiatry 30(Suppl. 1):253. doi: 10.1016/j.pnpbp.2017.07.007
Whitfield-Gabrieli, S., and Nieto-Castanon, A. (2012). Conn: a functional connectivity toolbox for correlated and anticorrelated brain networks. Brain Connect. 2, 125–141. doi: 10.1089/brain.2012.0073
The WHOQOL Group (1998). Development of the World Health Organization WHOQOL-BREF quality of life assessment. Psychol. Med. 28, 551–558.
Wölwer, W., Brinkmeyer, J., Riesbeck, M., Freimüller, L., Klimke, A., Wagner, A., et al. (2008). Neuropsychological impairments predict the clinical course in schizophrenia. Eur. Arch. Psychiatry Clin. Neurosci. 258, 28–34. doi: 10.1007/s00406-008-5006-2
Zanto, T. P., and Gazzaley, A. (2013). Fronto-parietal network: flexible hub of cognitive control. Trends Cogn. Sci. 33, 16268–16274. doi: 10.1016/j.tics.2013.10.001
Keywords: cognitive deficit, schizophrenia, first episodes, cognitive profiles, heterogeneity, cluster analysis, resting state functional connectivity
Citation: Rodriguez M, Zaytseva Y, Cvrčková A, Dvořaček B, Dorazilová A, Jonáš J, Šustová P, Voráčková V, Hájková M, Kratochvílová Z, Španiel F and Mohr P (2019) Cognitive Profiles and Functional Connectivity in First-Episode Schizophrenia Spectrum Disorders – Linking Behavioral and Neuronal Data. Front. Psychol. 10:689. doi: 10.3389/fpsyg.2019.00689
Received: 23 October 2018; Accepted: 12 March 2019;
Published: 02 April 2019.
Edited by:
Drozdstoy Stoyanov Stoyanov, Plovdiv Medical University, BulgariaReviewed by:
Sevdalina Kandilarova, Plovdiv Medical University, BulgariaPaola Valsasina, San Raffaele Scientific Institute (IRCCS), Italy
Copyright © 2019 Rodriguez, Zaytseva, Cvrčková, Dvořaček, Dorazilová, Jonáš, Šustová, Voráčková, Hájková, Kratochvílová, Španiel and Mohr. This is an open-access article distributed under the terms of the Creative Commons Attribution License (CC BY). The use, distribution or reproduction in other forums is permitted, provided the original author(s) and the copyright owner(s) are credited and that the original publication in this journal is cited, in accordance with accepted academic practice. No use, distribution or reproduction is permitted which does not comply with these terms.
*Correspondence: Mabel Rodriguez, bWFiZWwucm9kcmlndWV6QG51ZHouY3o=