- Department of Pedagogy, Psychology, Philosophy, Faculty of Humanities, University of Cagliari, Cagliari, Italy
This study emphasizes the importance of analyzing factors that contribute to student well-being, as a result of the multiplicity of factors that can affect their quality of life (QoL). The literature indicates that, among these factors, emotional intelligence and self-regulation play a central role in influencing adolescents’ psychological and scholastic well-being. Technology is a fundamental aspect of adolescent life but addiction to the use of smartphones is increasing, which can affect both emotional intelligence and self-regulation, and in turn individual well-being and QoL. Therefore, this study explores the role of smartphone use with respect to these aspects. Participants were 215 Italian students attending middle school. By applying partial least squares structural equation modeling (PLS-SEM), the results confirm that self-regulation affects the QoL of students, but its role varies according to the degree of smartphone addiction. In conclusion, we confirm the relevance of the relationship between self-regulation and smartphone addiction in teaching students to be aware of their time spent using smartphones. Emotional intelligence and, in general, self-regulation should be encouraged to support the well-being and QoL of students in their adolescence at school.
Introduction
The well-being of students at school is a primary concern for teachers and educators (Stefansson et al., 2018) as it is strictly related to their quality of life (QoL: Camfield and Skevington, 2008). Several studies (Shoshani et al., 2016; Navarro et al., 2017) examine factors that can positively influence student well-being and QoL in adolescence, finding it to be the result of a combination of affective, behavioral and cognitive dimensions. Some literature shows a link between emotional intelligence and well-being (Zeidner and Olnick-Shemesh, 2010), particularly at class level (Balluerka et al., 2016). Another fundamental dimension connected to these two aspects is self-regulation (Thomas et al., 2019). Self-regulation strategies facilitate students’ planning and goal-setting prior to learning by enhancing their attention-focusing and self-monitoring processes (self-reflection) during learning or task performance (Zimmerman, 2002; Cleary and Chen, 2009).
Digital society provides numerous opportunities but despite the implied advantages it also brings risks, especially for younger people (Machimbarrena et al., 2019); indeed, use of the internet can become problematic, leading to consequences for personal well-being. In particular, young people are continually increasing their smartphone use (Humphreys et al., 2013) and internet addiction has become ubiquitous (Haverlag, 2013; Yam et al., 2019). A body of research states that problematic internet use can become addictive but the issue of smartphone use is more complex; undeniably, smartphones can link to the internet and also execute various types of applications (e.g., gaming, gambling, social media use, etc.), consequently causing psychological impairment (Lin et al., 2019; Yam et al., 2019). Adolescents between 16 and 18 years old were less likely to believe in the negative impact of the internet on health than older people (Do et al., 2020). The prevalence of internet addiction is 1.2–4.9% (Mak et al., 2014) in adolescents and as high as 30% in university students (Zhang and Ho, 2017). Most studies on this issue focus on describing behaviors and consequences, including depression, anxiety, alcohol misuse, musculoskeletal discomfort, and sleep problems (Bianchi and Phillips, 2005; Ho et al., 2014; Yang et al., 2017; Zhang and Ho, 2017; Alimoradi et al., 2019; Chen et al., 2020). Generally, studies emphasize that internet addiction is inversely related to the global Life Satisfaction Index (Cheng et al., 2018) and health-related QoL (Tran et al., 2017), leading to the need to spend increasing time on internet gaming and losing interest in hobbies, relationships, and educational opportunities (Ho et al., 2014). Many studies emphasize that self-regulation constructs are adversely affected by smartphone addiction (van Deursen et al., 2015), but self-regulation may contribute to the suppression of addictive behavior (Baumeister and Vonasch, 2015). Other studies hypothesize that people who are able to express and understand emotions and regulate feelings are better adjusted psychologically and socially and have a high level of well-being (Gascó et al., 2018), therefore it is important to preserve this dimension.
What is the relationship between these variables? These premises underline the need for attention to factors that can positively or negatively affect adolescent well-being. This study considers the effects of self-regulation (hypothesis 1a, H1a) and emotional intelligence (H1b) on scholastic well-being. The innovation in this model relates to the role that smartphone dependence plays in these relationships. We assess if smartphone dependence might mediate the effects of self-regulation (H2a) and emotional intelligence (H2b) on scholastic well-being. Furthermore, it is of interest to evaluate the potential moderating effect of smartphone addiction on the relation between self-regulation and well-being (H3a) and between emotional intelligence and well-being (H3b) (Figure 1). These hypotheses are based on previous research findings in the literature (Zimmermann and Iwanski, 2014; Verzeletti et al., 2016; Chung, 2019; Xu et al., 2019).
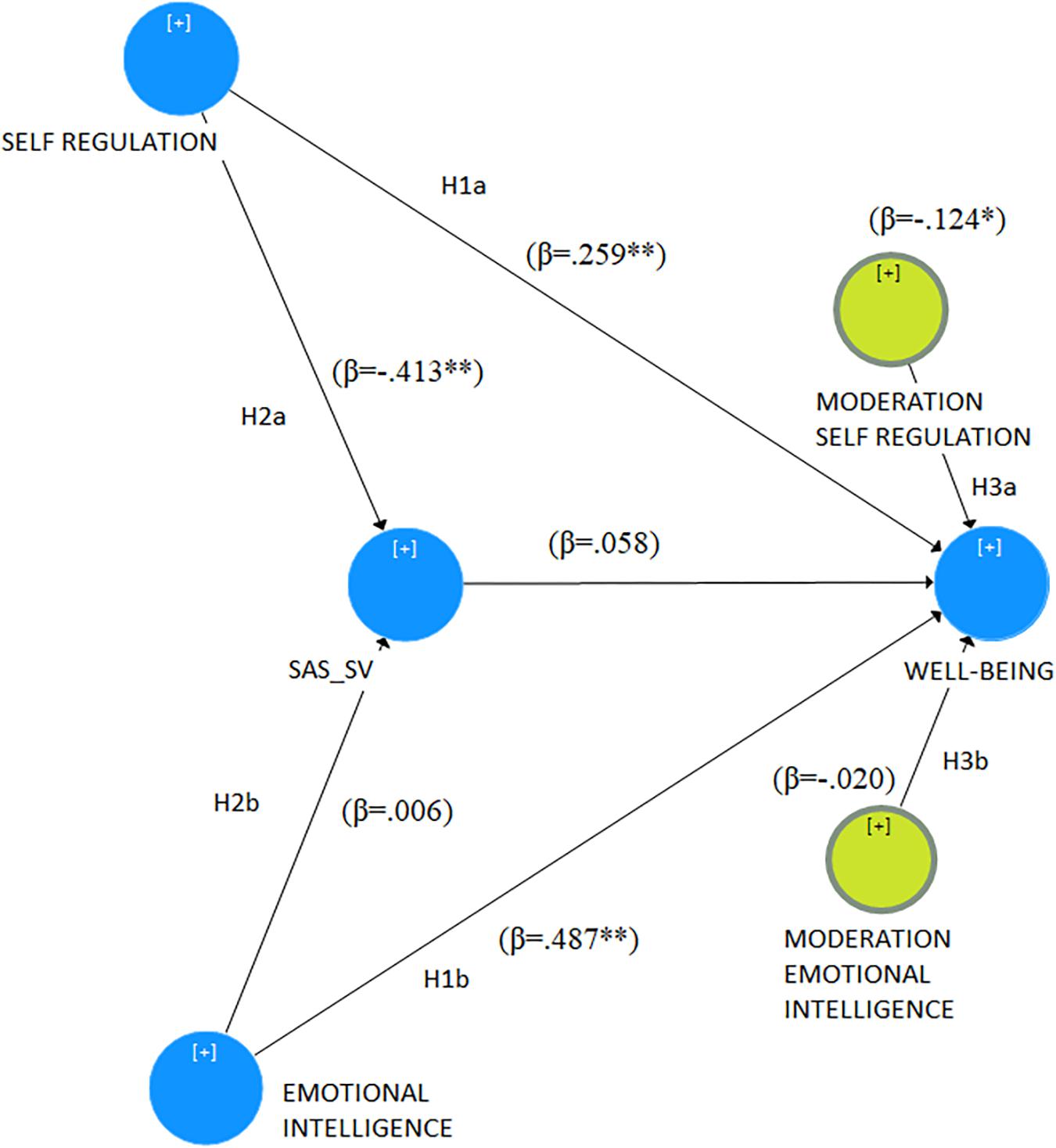
Figure 1. Conceptual framework: Results from PLS-SEM. H1a, hypothesis 1a; H1b, hypothesis 1b; H2a, hypothesis 2a; H2b, hypothesis 2b; H3a, hypothesis 3a; H3b, hypothesis 3b; SAS-SV, Smartphone Addiction Scale-Short version; β, Beta coefficient; ∗p < 0.05; ∗∗p < 0.01.
Method
Participants
This study involved 215 students (mean age 12.7 years; SD = 0.90) attending their last year of middle school in Sardinia (Italy).
Measures and Procedure
The survey was conducted in the third-year classes of state middle schools during the school timetable, subject to agreement from parents, the headmaster and teachers. Informed consent was given by the students’ parents after the features and aims of the study had been explained to them. We established an atmosphere of participation and trust in all classes, allowing the students to choose to participate in the research and motivating them sufficiently for the purpose of the study. To prevent teachers from interfering during the survey, they were asked to adopt a neutral stance if they were present in the classroom. All teachers were helpful and cooperative, leaving the management of the survey to the provider. Data collection was carried out during school hours from 8:30 to 13:00. Administration of the questionnaire took 25–45 min, preceded by a detailed explanation of the objectives of the survey, the structure of the questionnaire, the method of compilation and the anonymity of the test. The study was approved by the ethics committee at the University of Cagliari, Italy (the Department of Pedagogy, Psychology, Philosophy).
The study protocol comprises five sections:
(1) A questionnaire on socio-demographic characteristics collects specific information such as age and educational level.
(2) The self-regulation questionnaire (Moè and De Beni, 2000) aims to identify the components of the self-regulation approach to the study, with particular reference to three meta-cognitive dimensions: processing skills, organization and self-evaluation. Research shows how students can organize their study activities with a time-bound work programe that complies with commitments and deadlines (Ley and Young, 1998; Moè and De Beni, 2000), using schema-driven strategies (based on schematization, building diagrams, and tables, notepads, etc.) and the adoption of specific processing methods. Successful students are aware of their own study method, know how to properly assess their own preparation and are more likely to reflect on the best way to deal with their studies. The scale consists of 30 items (10 items for each dimension) to be answered on a Likert scale from 1 to 5, with higher values denoting better skills. Cronbach’s alpha coefficient for reliability is good for all dimensions: processing skills, α = 0.81; organization, α = 0.76; self-evaluation, α = 0.72.
(3) The “My Life as a Student” questionnaire (Soresi and Nota, 2003; Nota et al., 2011) allows students to explore their levels of satisfaction and well-being. This instrument consists of 26 items (on a five-point Likert scale, with higher scores indicating greater well-being) and examines seven satisfaction factors in several aspects: the school experience (α = 0.86); opportunities to make autonomous decisions (α = 0.66); relationships with classmates (α = 0.70); current living conditions (α = 0.76); family relationships (α = 0.71); praise received when due (α = 0.72); and availability of assistance (α = 0.79).
(4) The Emotional Intelligence Scale (EIS) questionnaire (Schutte et al., 1998), conducted in a validated Italian version (Ciucci et al., 2009), is designed to determine emotional intelligence and consists of 33 closed-ended statements (five-point Likert scale, with higher values representing improved emotional intelligence) such as “I am aware of my emotions as I experience them.” The three scales identified in the questionnaire measure: emotional appraisal and expression of oneself (13 items, α = 0.64), and others (10 items, α = 0.68); and regulation of emotions in oneself and others (10 items, α = 0.71).
(5) The Smartphone Addiction Scale (SAS) is a validated questionnaire designed to determine the risk level of smartphone addiction and identify high-risk groups among adolescents in Korea (Kwon et al., 2013). A short version (SAS-SV) was conducted, validated in Italy by De Pasquale et al. (2017). The questionnaire includes 10 questions (α = 0.79) describing daily disruptions in life, positive expectations, withdrawal, relationships in cyberspace, overuse and tolerance. Participants express their opinions on a six-point scale (1 = strongly disagree; 6 = strongly agree), with higher scores designating greater smartphone addiction (De Pasquale et al., 2017).
In order to verify the research hypotheses, this work proposed a conceptual model including those dimensions that might potentially affect student well-being. The model was devised on the basis of previous relevant works in the literature (Zimmermann and Iwanski, 2014; Gascó et al., 2018; Machimbarrena et al., 2019; Do et al., 2020). The conceptual model was assessed by applying component-based partial least squares structural equation modeling (PLS-SEM), designed to determine the values of the variables in relation to the predicted purpose (Chin, 1998). In this work PLS-SEM was used as the main statistical technique to evaluate our model due to the multiplicity of constructs and relationships to be assessed (Hair et al., 2011).
This statistical approach is particularly suitable for small samples, demonstrates robustness of non-normal data and has fewer restrictive assumptions than factor-based SEM. PLS-SEM analyses both the outer measurement model (referring to the quality, reliability and validity of the construct under study) and the inner model (where paths between latent variables are estimated) (Hair et al., 2012; Sarstedt et al., 2017). Statistical analyses are performed using the software R 3.6.1 (R Core Team, 2019) and Smart-PLS (V.3.2.8) (Ringle et al., 2015). In the model assessed, the subscales related to the constructs of self-regulation (processing skills; organization; self-evaluation) (Moè and De Beni, 2000) and emotional intelligence (emotions related to others and themselves; regulation and use of emotions) (Ciucci et al., 2009) were used as observed variables. For smartphone addiction on the SAS-SV (De Pasquale et al., 2017), all 10 items that explore the construct were used as observed variables. Well-being was measured by the seven subscales identified on the “My Life as a Student” questionnaire (Soresi and Nota, 2003).
Results
Descriptive statistics were performed on each variable to evaluate the distribution (Table 1). PLS-SEM was then performed with a reflective measurement model (Hair et al., 2019). Table 2 illustrates the indicators used for the outer measurement model. The factor loadings obtained vary from to 0 458 to 0.862 for all constructs; the consistent reliability coefficient Rho_A was consistently greater than 0.7, which indicates an acceptable internal reliability for the dimensions (Dijkstra and Henseler, 2015). The constructs indicated an average variance extracted (AVE) value higher than 0.5, indicating convergent validity (Fornell and Larcker, 1981). The adjusted R2 value was 0.161 for smartphone addiction and 0.390 for well-being, highlighting weak and moderate effects, respectively (Hair et al., 2011).
Concerning the inner model, each path is calculated and assessed by applying the bootstrapping routine (5000 subsamples from the original data), calculating standard errors, T values and p values. This procedure identifies the significance of each relationship and effect (Hair et al., 2019; Table 3). Specifically, the positive effects of self-regulation (H1a) (β = 0.259∗∗) and emotional intelligence (H1b) (β = 0.487∗∗) on well-being are confirmed (Table 3). Although the negative effect of self-regulation on smartphone addiction is confirmed (β = −0.413∗∗), the influence of smartphone addiction on well-being has not been established as an indirect overall effect, which does not support H2a. H2b has not been confirmed, highlighting that there is no indirect overall effect between emotional intelligence, smartphone addiction, and well-being. Furthermore, the findings emphasize a significant negative moderation effect of smartphone addiction on the relationship between self-regulation and well-being (H3a) (β = −0.124∗). The moderation effect of smartphone addiction on the relationship between emotional intelligence and well-being (H3b) has not been confirmed (Table 3).
Discussion and Conclusion
The findings of this work highlight the multivariate relationships affecting adolescent well-being, including the role played by their dependence on smartphones. To the best of our knowledge, few works in the literature have referred to the relation between smartphone addiction, emotional intelligence, self-regulation and well-being. The literature features a series of studies showing that many factors influence well-being and QoL in adolescence (Jovanović, 2016). QoL and satisfaction are defined as cognitive components of subjective well-being (Diener et al., 1999). Many scholars emphasize the multiplicity of factors concerning the emotional and self-regulation processes of young adolescents (e.g., Abe, 2011). Furthermore, recently it has been highlighted that in our daily lives (work, school, leisure) attention is often directed to smartphones. There are many advantages to using technology but the excessive use of smartphones for continuous connectivity can lead to internet addiction (Tonioni and Corvino, 2011) and to the alarming phenomenon of hikikomori (Suwa and Suzuki, 2013).
These relevant facts support the necessity to deepen our knowledge of the relationship between smartphone addiction and well-being, specifically in adolescents. The current model assessed relationships that have seldom been tested empirically before (e.g., mediation and moderation effects of smartphone addiction in the relationships between self-regulation and well-being and between emotional intelligence and well-being). This study attempted to identify the dimensions affecting adolescent well-being and has highlighted some interesting insights. In a closer look at the relationships between the variables that underlie scholastic QoL, our findings confirm the positive effect of self-regulation and emotional intelligence on well-being. The negative effect of self-regulation on smartphone addiction was also highlighted. However, the indirect effects of self-regulation, smartphone addiction, and well-being have not been established. Moreover, the indirect overall effects of emotional intelligence, smartphone addiction, and well-being have not been confirmed. It is of interest that the results emphasize significant negative moderation effects of smartphone addiction on the relationship between self-regulation and well-being, highlighting that the effect of self-regulation on well-being can vary depending on the level of smartphone addiction. Specifically, this last significant moderation effect implies that a low level of smartphone addiction enhances the positive relation between self-regulation and well-being; on the other hand, when smartphone addiction is high, the positive relationship between self-regulation and well-being is weakened. These findings shed light on issues that should be taken into consideration to improve adolescent well-being.
Moreover, it should be pointed out that some limitations of these findings might derive from the cross-sectional research design, the non-probabilistic sampling method in the Italian context and the presentation of self-report questionnaires.
New technologies offer endless possibilities for students and schools, but we must find ways to benefit, depending on the level of smartphone addiction. For example, smartphone applications can be used to deliver immersive virtual reality therapy for treating internet addiction in adolescents (Zhang et al., 2017). Education must play an active role in helping digital natives learn about and use these new tools. Emphasis should be placed on education concerning emotional intelligence and self-regulation in order to achieve psychological and social well-being, and in turn global life satisfaction (Huebner et al., 2005).
Data Availability Statement
The datasets for this study are available from corresponding author on reasonable request.
Ethics Statement
The studies involving human participants were reviewed and approved by the Department of Pedagogy, Psychology, Philosophy, Faculty of Humanities, University of Cagliari, Cagliari, Italy. Written informed consent to participate in this study was provided by the participants’ legal guardian/next of kin.
Author Contributions
MM and MP contributed to the design of the study. MA analyzed the data. All authors wrote, read, revised and approved the final manuscript.
Conflict of Interest
The authors declare that the research was conducted in the absence of any commercial or financial relationships that could be construed as a potential conflict of interest.
References
Abe, J. A. A. (2011). Positive emotions, emotional intelligence, and successful experiential learning. Pers. Individ. Differ. 51, 817–822. doi: 10.1016/j.paid.2011.07.004
Alimoradi, Z., Lin, C.-Y., Broström, A., Bülow, P. H., Bajalan, Z., Griffiths, M. D., et al. (2019). Internet addiction and sleep problems: a systematic review and meta-analysis. Sleep Med. Rev. 47, 51–61. doi: 10.1016/j.smrv.2019.06.004
Balluerka, N., Gorostiaga, A., Alonso-Arbiol, I., and Aritzeta, A. (2016). Peer attachment and class emotional intelligence as predictors of adolescents’ psychological well-being: a multilevel approach. J. Adolesc. 53, 1–9. doi: 10.1016/j.adolescence.2016.08.009
Baumeister, R. F., and Vonasch, A. J. (2015). Uses of self-regulation to facilitate and restrain addictive behavior. Addict. Behav. 44, 3–8. doi: 10.1016/j.addbeh.2014.09.011
Bianchi, A., and Phillips, J. G. (2005). Psychological predictors of problem mobile phone use. Cyber. Psychol. Behav. 8, 39–51. doi: 10.1089/cpb.2005.8.39
Camfield, L., and Skevington, S. M. (2008). On subjective well-being and quality of life. J. Health Psychol. 13, 764–775. doi: 10.1177/1359105308093860
Chen, I.-H., Strong, C., Lin, Y.-C., Tsai, M.-C., Leung, H., Lin, C.-Y., et al. (2020). Time invariance of three ultra-brief internet-related instruments: smartphone Application-Based Addiction Scale (SABAS), Bergen Social Media Addiction Scale (BSMAS), and the nine-item Internet Gaming Disorder Scale- Short Form (IGDS-SF9) (Study Part B). Addict. Behav. 101:105960. doi: 10.1016/j.addbeh.2019.04.018
Cheng, C., Cheung, M. W. L., and Wang, H. (2018). Multinational comparison of internet gaming disorder and psychosocial problems versus well-being: Meta-analysis of 20 countries. Comput. Hum. Behav. 88, 153–167. doi: 10.1016/j.chb.2018.06.033
Chin, W. W. (1998). “The partial least squares approach to structural equation modeling,” in Modern Methods for Business Research, ed. G. A. Marcoulides, (London: Lawrence Erlbaum), 295–336.
Chung, M. S. (2019). The effect of internet addiction prevention program on adolescents’ self-regulation-a systematic review and meta-analysis. J. Digit. Converg. 17, 347–355. doi: 10.14400/JDC.2019.17.8.347
Ciucci, E., Menesini, E., Primi, C., Grazzani Gavazzi, I., and Antoniotti, C. (2009). Psychometric properties of the Italian version of the Emotional Intelligence Scale (EIS) with preadolescents counseling. Ital. J. Res. Interv. 2, 85–100.
Cleary, T. J., and Chen, P. P. (2009). Self-regulation, motivation, and math achievement in middle school: variations across grade level and math context. J. Sch. Psychol. 47, 291–314. doi: 10.1016/j.jsp.2009.04.002
De Pasquale, C., Sciacca, F., and Hichy, Z. (2017). Italian validation of smartphone addiction scale short version for adolescents and young adults (SAS-SV). Psychology 08, 1513–1518. doi: 10.4236/psych.2017.810100
Diener, E., Suh, E. M., Lucas, R. E., and Smith, H. L. (1999). Subjective well-being: three decades of progress. Psychol. Bull. 125:276. doi: 10.1037/0033-2909.125.2.276
Dijkstra, T. K., and Henseler, J. (2015). Consistent partial least squares path modeling. MIS Q. 39, 397–316.
Do, H. N., Onyango, B., Prakash, R., Tran, B. X., Nguyen, Q. N., Nguyen, L. H., et al. (2020). Susceptibility and perceptions of excessive internet use impact on health among Vietnamese youths. Addict. Behav. 101:105898. doi: 10.1016/j.addbeh.2019.01.043
Fornell, C., and Larcker, D. F. (1981). Evaluating structural equation models with unobservable variables and measurement error. J. Mark. Res. 18:39. doi: 10.2307/3151312
Gascó, V. P., Badenes, L. V., and Plumed, A. G. (2018). Trait emotional intelligence and subjective well-being in adolescents: the moderating role of feelings. Psicothema 30, 310–315. doi: 10.7334/psicothema2017.232
Hair, J. F., Ringle, C. M., and Sarstedt, M. (2011). PLS-SEM: indeed a silver bullet. J. Mark. Theory Pract. 19, 139–152. doi: 10.2753/MTP1069-6679190202
Hair, J. F., Risher, J. J., Sarstedt, M., and Ringle, C. M. (2019). When to use and how to report the results of PLS-SEM. Eur. Bus. Rev. 31, 2–24. doi: 10.1108/EBR-11-2018-0203
Hair, J. F., Sarstedt, M., Pieper, T. M., and Ringle, C. M. (2012). The use of partial least squares structural equation modeling in strategic management research: a review of past practices and recommendations for future applications. Long Range Plan. 45, 320–340. doi: 10.1016/j.lrp.2012.09.008
Haverlag, D. (2013). Compulsief Gebruik Van Sociale Media op de Smartphone. Available at: https://dspace.library.uu.nl/handle/1874/280913 (accessed September 27, 2019).
Ho, R. C., Zhang, M. W., Tsang, T. Y., Toh, A. H., Pan, F., Lu, Y., et al. (2014). The association between internet addiction and psychiatric co-morbidity: a meta-analysis. BMC Psychiatry 14:183. doi: 10.1186/1471-244X-14-183
Huebner, E. S., Valois, R. F., Paxton, R. J., and Drane, J. W. (2005). Middle school students’ perceptions of quality of life. J. Happiness Stud. 6, 15–24. doi: 10.1007/s10902-004-1170-x
Humphreys, L., Von Pape, T., and Karnowski, V. (2013). Evolving mobile media: uses and conceptualizations of the mobile internet. J. Comput. Commun. 18, 491–507. doi: 10.1111/jcc4.12019
Jovanović, V. (2016). The validity of the Satisfaction with Life Scale in adolescents and a comparison with single-item life satisfaction measures: a preliminary study. Qual. Life Res. 25, 3173–3180. doi: 10.1007/s11136-016-1331-5
Kwon, M., Lee, J.-Y., Won, W.-Y., Park, J.-W., Min, J.-A., Hahn, C., et al. (2013). Development and validation of a Smartphone Addiction Scale (SAS). PLoS One 8:e56936. doi: 10.1371/journal.pone.0056936
Ley, K., and Young, D. B. (1998). Self-regulation behaviors in underprepared (Developmental) and regular admission college students. Contemp. Educ. Psychol. 23, 42–64. doi: 10.1006/ceps.1997.0956
Lin, C.-Y., Imani, V., Broström, A., Nilsen, P., Fung, X. C. C., Griffiths, M. D., et al. (2019). Smartphone application-based addiction among iranian adolescents: a psychometric study. Int. J. Ment. Health Addict. 17, 765–780. doi: 10.1007/s11469-018-0026-2
Machimbarrena, J., González-Cabrera, J., Ortega-Barón, J., Beranuy-Fargues, M., Álvarez-Bardón, A., and Tejero, B. (2019). Profiles of problematic internet use and its impact on Adolescents’ health-related quality of life. Int. J. Environ. Res. Public Health 16, 3877. doi: 10.3390/ijerph16203877
Mak, K.-K., Lai, C.-M., Watanabe, H., Kim, D.-I., Bahar, N., Ramos, M., et al. (2014). Epidemiology of internet behaviors and addiction among adolescents in six asian countries. Cyberpsychol. Behav. Soc. Netw. 17, 720–728. doi: 10.1089/cyber.2014.0139
Moè, A., and De Beni, R. (2000). Strategie di autoregolazione e successo scolastico: Uno studio con ragazzi di scuola superiore e universitari. Psicol. dell’Educazione e della Form. 2, 31–44.
Navarro, D., Montserrat, C., Malo, S., González, M., Casas, F., and Crous, G. (2017). Subjective well-being: what do adolescents say? Child Fam. Soc. Work 22, 175–184. doi: 10.1111/cfs.12215
Nota, L., Soresi, S., Ferrari, L., and Wehmeyer, M. L. (2011). A multivariate analysis of the self-determination of adolescents. J. Happiness Stud. 12, 245–266. doi: 10.1007/s10902-010-9191-0
Ringle, C. M., Wende, S., and Becker, J.-M. (2015). SmartPLS 3. Available at: http://www.smartpls.com (accessed September 09, 2019).
Sarstedt, M., Ringle, C. M., and Hair, J. F. (2017). “Partial least squares structural equation modeling,” in Handbook of Market Research, eds H. Christian, K. Martin, and V. Arnd, (Cham: Springer International Publishing), 1–40. doi: 10.1007/978-3-319-05542-8_15-1
Schutte, N. S., Malouff, J. M., Hall, L. E., Haggerty, D. J., Cooper, J. T., Golden, C. J., et al. (1998). Development and validation of a measure of emotional intelligence. Pers. Individ. Differ. 25, 167–177.
Shoshani, A., Steinmetz, S., and Kanat-Maymon, Y. (2016). Effects of the Maytiv positive psychology school program on early adolescents’ well-being, engagement, and achievement. J. Sch. Psychol. 57, 73–92. doi: 10.1016/j.jsp.2016.05.003
Soresi, S., and Nota, L. (2003). La mia vita da Studente. Firenze: ITER–Institute of Training Education and Research.
Stefansson, K. K., Gestsdottir, S., Birgisdottir, F., and Lerner, R. M. (2018). School engagement and intentional self-regulation: a reciprocal relation in adolescence. J. Adolesc. 64, 23–33. doi: 10.1016/j.adolescence.2018.01.005
Suwa, M., and Suzuki, K. (2013). The phenomenon of “hikikomori” (social withdrawal) and the socio-cultural situation in Japan today. J. Psychopathol. 19, 191–198.
Thomas, V., Muls, J., De Backer, F., and Lombaerts, K. (2019). Exploring self-regulated learning during middle school: views of parents and students on parents’ educational support at home. J. Fam. Stud. 1–19. doi: 10.1080/13229400.2018.1562359
Tonioni, F., and Corvino, S. (2011). Dipendenza da internet e psicopatologia web-mediata. Recent. Prog. Med. 102, 417–420. doi: 10.1701/975.10605
Tran, B. X., Huong, L. T., Hinh, N. D., Nguyen, L. H., Le, B. N., Nong, V. M., et al. (2017). A study on the influence of internet addiction and online interpersonal influences on health-related quality of life in young Vietnamese. BMC Public Health 17:138. doi: 10.1186/s12889-016-3983-z
van Deursen, A. J. A. M., Bolle, C. L., Hegner, S. M., and Kommers, P. A. M. (2015). Modeling habitual and addictive smartphone behavior. Comput. Human Behav. 45, 411–420. doi: 10.1016/j.chb.2014.12.039
Verzeletti, C., Zammuner, V. L., Galli, C., and Agnoli, S. (2016). Emotion regulation strategies and psychosocial well-being in adolescence. Cogent. Psychol. 3:1199294. doi: 10.1080/23311908.2016.1199294
Xu, T.-T., Wang, H.-Z., Fonseca, W., Zimmerman, M. A., Rost, D. H., Gaskin, J., et al. (2019). The relationship between academic stress and adolescents’ problematic smartphone usage. Addict. Res. Theory 27, 162–169. doi: 10.1080/16066359.2018.1488967
Yam, C.-W., Pakpour, A. H., Griffiths, M. D., Yau, W.-Y., Lo, C.-L. M., Ng, J. M. T., et al. (2019). Psychometric testing of three chinese online-related addictive behavior instruments among hong kong university students. Psychiatr. Q. 90, 117–128. doi: 10.1007/s11126-018-9610-7
Yang, S.-Y., Chen, M.-D., Huang, Y.-C., Lin, C.-Y., and Chang, J.-H. (2017). Association between smartphone use and musculoskeletal discomfort in adolescent students. J. Commun. Health 42, 423–430. doi: 10.1007/s10900-016-0271-x
Zeidner, M., and Olnick-Shemesh, D. (2010). Emotional intelligence and subjective well-being revisited. Pers. Individ. Differ. 48, 431–435. doi: 10.1016/j.paid.2009.11.011
Zhang, M. W. B., and Ho, R. C. M. (2017). Smartphone applications for immersive virtual reality therapy for internet addiction and internet gaming disorder. Technol. Heal. Care 25, 367–372. doi: 10.3233/THC-161282
Zhang, M. W. B., Tran, B. X., Huong, L. T., Hinh, N. D., Nguyen, H. L. T., Tho, T. D., et al. (2017). Internet addiction and sleep quality among Vietnamese youths. Asian J. Psychiatr. 28, 15–20. doi: 10.1016/j.ajp.2017.03.025
Zimmerman, B. J. (2002). Achieving Self-Regulation: The Trial and Triumph of Adolescence. California: ERIC.
Keywords: adolescence, smartphone addiction, self-regulation, emotional intelligence, well-being, quality of life
Citation: Mascia ML, Agus M and Penna MP (2020) Emotional Intelligence, Self-Regulation, Smartphone Addiction: Which Relationship With Student Well-Being and Quality of Life? Front. Psychol. 11:375. doi: 10.3389/fpsyg.2020.00375
Received: 31 October 2019; Accepted: 18 February 2020;
Published: 06 March 2020.
Edited by:
Daniela Traficante, Catholic University of the Sacred Heart, ItalyReviewed by:
Roger C. Ho, National University of Singapore, SingaporeChung-Ying Lin, The Hong Kong Polytechnic University, Hong Kong
Copyright © 2020Mascia, Agus and Penna. This is an open-access article distributed under the terms of the Creative Commons Attribution License (CC BY). The use, distribution or reproduction in other forums is permitted, provided the original author(s) and the copyright owner(s) are credited and that the original publication in this journal is cited, in accordance with accepted academic practice. No use, distribution or reproduction is permitted which does not comply with these terms.
*Correspondence: Maria Lidia Mascia, bWFyaWFsaWRpYS5tYXNjaWFAdW5pY2EuaXQ=; YXJpYWxpZGlhbWFzY2lhQGdtYWlsLmNvbQ==