- Charitable Medical Healthcare Foundation, Augusta, GA, United States
Pulmonary ventilation and respiration are considered to be primarily involved in oxygenation of blood for oxygen delivery to cells throughout the body for metabolic purposes. Other pulmonary physiological observations, such as respiratory sinus arrhythmia, Hering Brewer reflex, cardiorespiratory synchronization, and the heart rate variability (HRV) relationship with breathing rhythm, lack complete explanations of physiological/functional significance. The spectrum of waveforms of breathing activity correlate to anxiety, depression, anger, stress, and other positive and negative emotions. Respiratory pattern has been thought not only to be influenced by emotion but to itself influence emotion in a bi-directional relationship between the body and the mind. In order to show how filling in gaps in understanding could lead to certain future developments in mind–body medicine, biofeedback, and personal health monitoring, we review and discuss empirical work and tracings to express the vital role of bodily rhythms in influencing emotion, autonomic nervous system activity, and even general neural activity. Future developments in measurement and psychophysiological understanding of the pattern of breathing in combination with other parameters such as HRV, cardiorespiratory synchronization, and skin conductivity may allow for biometric monitoring systems to one day accurately predict affective state and even affective disorders such as anxiety. Better affective prediction based on recent research when incorporated into personal health monitoring devices could greatly improve public mental health by providing at-home biofeedback for greater understanding of one’s mental state and for mind–body affective treatments such as breathing exercises.
Introduction
William James, known as the “Father of American Psychology,” once said, “For us, emotion dissociated from all bodily feeling is inconceivable” (James, 1884). In this statement, he has identified the intimate relationship between the body and the mind. Although there is no scientific consensus on an exact definition (Celeghin et al., 2017), the concept of emotion consists of a complex range of phenomenological and physiological states, which include neurobiological components, visceral reactions, bodily responses/behaviors, and of course, often powerful feelings (Purves et al., 2018), and can be described as an adaptive, patterned neural response to external circumstances that can be found at all levels of the nervous system (Damasio and Carvalho, 2013). Emotions are “action programs,” innate physiological “programs” aimed at maintaining or restoring homeostasis by changing the body to more appropriately interact with the environment (Damasio and Carvalho, 2013). Action programs are selected based on the current information the organism has on its environment and self, evaluated so as to maximize biological fitness (Rangel et al., 2008). Emotions are similar in this sense to basic instinctual drives (also action programs) such as hunger and thirst, referred to as “primordial emotions” (Denton et al., 2009). The physiological changes of action programs may be sensed by the interoceptive system and interpreted by the cortex, further influencing emotional state (Damasio and Carvalho, 2013).
Emotions are not only a key aspect of social cognition and communication, but they also initiate homeostatic physiological and cognitive functions that allow us to survive and thrive via proper detection and response to diverse challenges and opportunities (Shariff and Tracy, 2011; LeDoux, 2012). Emotional experiences are tightly bound to bodily sensations (Pace-Schott et al., 2019) and draw attention to important events such as during physiological need, immediate threat, and social interaction (Damasio and Carvalho, 2013). They coordinate behavior and physiological states during such important events (Stemmler, 2004; Nummenmaa et al., 2014). The physical effects resulting from emotional arousal are largely mediated by the autonomic nervous system (ANS), which can include changes in heart rate, skin temperature/blood flow/sweating, gut motility, pupil size, and piloerection (Kreibig, 2010; Masaoka et al., 2012; Purves et al., 2018). Bodily responses mediated by the ANS provide the most information on emotional state (Pace-Schott et al., 2019), and the ANS acts in “sympathy” with emotion, innervating the heart, lungs, and many other bodily systems (Valderas et al., 2015). Such physiological variables associated with the variety of emotions are often effortlessly measured with the right technology and have been used to detect one’s autonomic state as in the polygraph (lie detector) (Rosenfeld, 1995). While the polygraph is often inaccurate in detecting lies, it does detect arousal accurately (Lewis and Cuppari, 2009). Such changes can occur unconsciously and in response to some stimuli much quicker than changes to conscious cognition (LeDoux, 1998). Mapping the spectra of physiological, emotional triggered responses could provide an important biomarking tool for emotional state and emotional disorders.
Emotion recognition technologies may provide a basis for monitoring emotional health and could even be used to monitor for emotion-related mental health disorders (Xiefeng et al., 2019). Because the physiological changes expressed by emotions are not voluntarily controlled, they may provide a more accurate reflection of the true emotional experiences people may be having (Wu et al., 2012). The interesting relationship between emotions and the body may shed light on undiscovered processes occurring between the body and the mind. In this article, we strive to advance the use of biometrics in the personal understanding of one’s mental state by discussing the medical potential for emotion recognition devices and applications with a user-friendly emotional spectrum that one may be placed upon in a particular moment based upon visceral cues. We approach this review from a perspective of embodied cognition, following the idea that not only does emotional state significantly influence bodily state, but bodily state also significantly influences emotional state.
Emotion, the Nervous System, and the Mind–Body Response
Emotion and bodily behaviors and sensations are linked to the point that they may be impossible to disentangle (Purves et al., 2018). Often, emotions are felt within the body (Nummenmaa et al., 2014), and these feelings occur as a result of activation of the muscular, cardiovascular, endocrine, and autonomic nervous systems (Levenson, 2006). Emotions are indeed associated with distinct bodily sensations that are culturally universal and may underlie emotional experiences, as well as corresponding to major physiological changes associated with each emotion (Ekman et al., 1983; Nummenmaa et al., 2014). We often describe our emotions in terms of bodily metaphors such as describing love as a feeling in the heart or associating fear with lowered body temperature as in the metaphor “cold feet” (Kövecses, 2000). The bodily feedback of somatic and visceral activity activated reflexively by external and internal events has been proposed to be a significant source and influencer of emotion (Barrett et al., 2007; Damasio and Carvalho, 2013; Purves et al., 2018). Voluntarily producing facial expressions or altering other bodily events such as breathing pace actually stimulates the associated emotion (Verschuuren et al., 1996) and produces the other physiological changes associated with that emotion, such as heart rate and muscle tension changes (Levenson et al., 1990).
We may understand the emotions of others by simulating them in our own minds and bodies (Niedenthal, 2007; Keysers et al., 2010; Ross and Atkinson, 2020). This simulation has been asserted to not involve conscious awareness (Wood et al., 2016). Recognizing emotional stimuli has been shown in some cases to be processed outside of conscious awareness or intent (Critchley, 2002; Philippot et al., 2002; Williams and Mattingley, 2004) and still result in the expected physiological changes (Bulut et al., 2018; Engelen et al., 2018). However, some of these results have been criticized as not generalizing to most circumstances, and there have been contradictory results. One study showed that when emotional stimuli are task irrelevant, expected muscular responses to a given emotion are not expressed (Mirabella, 2018). This may suggest that unconscious emotion recognition is not invariable and automatic but depends on the individual’s cognitive state.
The primary somatosensory cortices are engaged during the perception of emotion as well as its contagious spreading (Nummenmaa et al., 2008, 2012), and damage to them impairs the recognition of other’s emotional state (Adolphs et al., 2000). Unconscious body-state simulation of emotion-associated autonomic and visceral changes has recently been reinforced as an important aspect of understanding others’ emotions (Ross and Atkinson, 2020). Common muscular mimicry, thought to be “spillover” from simulating emotions, is abundant when observing others and occurs more to emotional stimuli (Moody et al., 2017). We suggest that respiratory pattern could be an essential mimicry target when simulating others’ emotions and that understanding how the spectrum of such bodily patterns map onto the spectrum of emotions will provide a means to measure unconscious emotion perception and emotional body-state simulation for research purposes.
Somatocentric perspectives such as the Somatic Marker Hypothesis argue that bodily states and feelings mark unconscious cognitive appraisals, which stimulate emotional experience and significantly underlie behavior and decision making. These hypotheses have been criticized, however, for being overly focused on the influence of the periphery and for misinterpretation of evidence (Dunn et al., 2006). A more moderate view we hold is that while bodily states and sensations can significantly influence the emotional mind and can act as emotional stimuli, accurate performance and decision making are not dependent on bodily markers, and the generation of emotional experience is not dependent on bodily feedback. While body states may not induce a waterfall of emotion as personal events or even music may, long-term exposure to such states may significantly alter emotional state. These body rhythms are always present with us, slowly provoking certain mental states, and so they deserve attention from not only those in health care but also the average person who would benefit from the ability to regulate emotional state. We and others have suggested that long-term “negative” body rhythms can keep us trapped in a vicious cycle of poor emotional health, which also leads to physical health declines (Jerath et al., 2019).
Positive and negative emotions differ largely in that positive emotions increase coherence of bodily rhythms, while negative emotions decrease coherence (McCraty and Zayas, 2014). A format of emotional placement based on bodily measurements may allow for the future development of more user-friendly feedback applications than currently exist that allow people to measure their emotional state and respond to it appropriately. Because the respiration–emotion relationship is reciprocal, people may voluntarily influence their emotional state by changing their respiration pattern. While emotions have such a reciprocal relationship with other bodily functions, respiration is special because it can be voluntarily altered.
Breathing Pattern and Emotional State
Autonomic breathing is dictated not only by metabolic demands but also by emotions (Homma and Masaoka, 2008). Although it is debated whether each emotion has its own distinct signature for autonomic functions (and for other physiological mechanisms) (Kreibig, 2010), there is significant support for the idea that there is indeed some physiological specificity across the emotional spectrum (Stemmler, 2004; Nummenmaa et al., 2014), with breathing rhythm frequently labeled as an index of emotional state (Noguchi et al., 2012).
Most real-world events will induce not separate and distinct emotions but, rather, a complex mix of emotions that are most often all positive or negative (Boiten, 1998). This and the fact that full, real-world emotions are often difficult to elicit in laboratory settings make the identification of exact physiological responses and patterns of specific emotions difficult. From the available research, however, it can be concluded that more arousing responses to negative emotions (such as fear, anger, and anxiety) result in shallower, rapid breathing (Boiten, 1998; Masaoka and Homma, 2001). This may result in decreased blood carbon dioxide levels (Kreibig, 2010). Conscious modulation of breathing toward a slower and deeper pattern may strengthen positive emotions when negative emotions are prevalent (Masaoka et al., 2012). Happiness and related positive emotion produces significant respiratory changes, which include increases in the variability of the breathing pattern and decreases in tidal volume and inspiratory time (Boiten, 1998). Positive emotions vary in their effect on respiration depending on how arousing they are, with the arousing ones increasing respiration rate (Kreibig, 2010). Periods of disgust (pathogen-related) lead to suppression and cessation of breathing, likely a natural reaction of avoiding inhalation of noxious contents (Boiten, 1998).
Increasing numbers of studies show not only that emotional state influences respiratory pattern but that respiratory pattern influences and stimulates emotional state, even when one is not aware of the process (Philippot et al., 2002). The mechanism of how the body affects the mind is thought to center around modulation of the ANS and recognition of interoceptive sensation by the brain. However, respiration may act directly on the brain. Evidence is mounting showing the dramatic, seemingly direct effects respiration can have on neural oscillations across diverse brain areas (Kluger and Gross, 2020). This may synchronize neural activity and improve computational efficiency (Kluger and Gross, 2020). The respiratory rhythm has been demonstrated to unify global coordination and tuning of neural firing and dynamics across cortical and subcortical networks (Karalis and Sirota, 2018; Zaccaro et al., 2018). The respiratory rhythm can even dominate local field potentials during quiescence (Karalis and Sirota, 2018). Respiratory synchronized activity in the brain has been shown to modulate cognitive performance depending on the phase properties of the respiratory cycle (Nakamura et al., 2018). Such modulation of neural oscillations has been associated with modulations of emotion (Fumoto et al., 2004; Yu et al., 2011). Respiration can also modulate hemodynamic activity, which has a significant effect on brain activity (Başar, 2008).
Other Responses to Emotion
While breathing is a peripheral rhythm with a special relationship with the mind, other physiological measures have emotional expression. These include cardiovascular indices, temperature, electrodermal, blood oxygenation, photoplethysmographic, and electromyographic measures (Shi et al., 2017). Although potentially invasive, biomarkers such as cortisol can also provide insight into emotional state (Strahler et al., 2017). More uncommon but revealing emotional responses including neural changes revealed by neuroimaging (Critchley and Harrison, 2013), genetic changes (Jonassen and Landrø, 2014), and inflammation (Pace-Schott et al., 2019) could be implemented into future emotion recognition technologies. Heart sounds can even be used in emotion recognition (Xiefeng et al., 2019). Heart rate variability (HRV) is the tiny variations in sinus heartbeats (Shi et al., 2017). Although some have criticized the idea, HRV is widely thought to differentiate a parasympathetic versus a sympathetic state (Balzarotti et al., 2017). HRV could thus be an important tool for identifying ANS imbalances. High HRV is sometimes associated with more positive states of mind, and HRV indices have been used in emotion recognition (Zhu et al., 2019).
Cardiac vagal control (CVC) is commonly measured by HRV, indicates the influence of the cardiovascular system on the parasympathetic nervous system (Kimhy et al., 2013), and may be an important marker for one’s ability to regulate emotion (Balzarotti et al., 2017). HRV indices may also be effective in distinguishing emotional state along with psychiatric emotional disorders (Zhu et al., 2019). Strong CVC indicates stronger vagal reactivity and recovery from stressors. Strong resting CVC is thus connected with greater ANS flexibility, cardiovascular fitness, and ability to respond to stress (Thayer and Fischer, 2009). Strong CVC is also associated with enhanced cognitive abilities such as attention, working memory, and processing speed (Hansen et al., 2003). Low HRV, and thus CVC, is associated with a variety of negative emotions (anger, sadness, and fear) and maladies including anxiety disorder, depression, cardiovascular disease, and increased risk of death (Tsuji et al., 1994; Buccelletti, 2009). Stressful events may actually lower CVC (Balzarotti et al., 2017).
Perhaps a better indicator of emotional valance is not the magnitude of the HRV but the coherence of it. Positive emotions result in a more coherent pattern, possibly providing a renewing effect. Incoherent emotions, on the other hand, produce an incoherent pattern, which is thought to have a depleting effect on health over time (McCraty and Zayas, 2014). One example of a coherence measure is the sine-like shape of heart rate patterns. Wearable devices that aim for emotion recognition should thus focus not only on basic aspects of gathered biometric data but also on patterns within and among data types.
The cardiac nervous system can act independently and is complex enough to be thought of as a “little brain” with short-term and long-term memory functions (Shaffer et al., 2014). A great portion of the fibers connecting this system to the brain are afferents, more so than any other bodily organ (Cameron, 2002). The heart is thus an intricate processing and encoding center (Armour and Ardell, 2004), which also releases its own hormones and neurotransmitters (Mukoyama et al., 1991; Huang et al., 1996). The thalamus is thought to be crucial to the formation of an integrated experience and in global cognitive functions due to its dense global networking with the cortex (Jerath et al., 2015). Research has shown that the rhythm of afferent neural information coming from the heart modulates thalamic activity, which can thus have global effects on the brain (Wölk and Velden, 1989). Frontal brain regions of the cortex (McCraty et al., 2004) as well as motor areas (Svensson and Thorén, 1979) show influence by the cardiac nervous system, and these effects include emotional processing influences (Zhang et al., 1986).
A Spectrum of Emotion
Given that emotions have largely distinct bodily patterns and are also influenced by such patterns, it may be possible to accurately map emotions along physiological dimensions. Here we give an example of this alongside dimensions of the ANS and breathing (Figure 1). Emotions are not unitary in the sense that there are different types of sadness, fear, etc., so they may be better classified for personal understanding by a color-coded spectrum. As emotions are currently regarded, single emotions sometimes have different physiological responses. For instance, sadness produces different physiological responses depending on whether it is empathetic or antipathetic (Davydov et al., 2011). Disgust is unique in that it can produce both parasympathetic and sympathetic responses depending on the nature of the reaction (pathogen vs. morality related) (Kreibig, 2010). Pathogen-related disgust can be considered more of a “primordial emotion” like thirst and hunger (Ottaviani et al., 2013). Understanding emotion via such a map will provide visual help in emotional regulation potentially by providing guidance on how to utilize the mind–body response. Mind–body response is a term for the psychophysiological change that occurs due to the interaction between the body and the brain, particularly focusing on the effects body rhythms can have on one’s psychology (Jerath et al., 2014). The development of such a spectrum may reveal embodiments of cognitive processes. Due to the lack of complete response specificity for basic emotions, greater dimensionality in classifying emotions may provide greater insight into their nature, reveal relationships between them, and help produce “navigational” tools for those looking to regulate their own emotions. Emotions may be regulated in a variety of means, from biofeedback methods to mind–body response techniques.
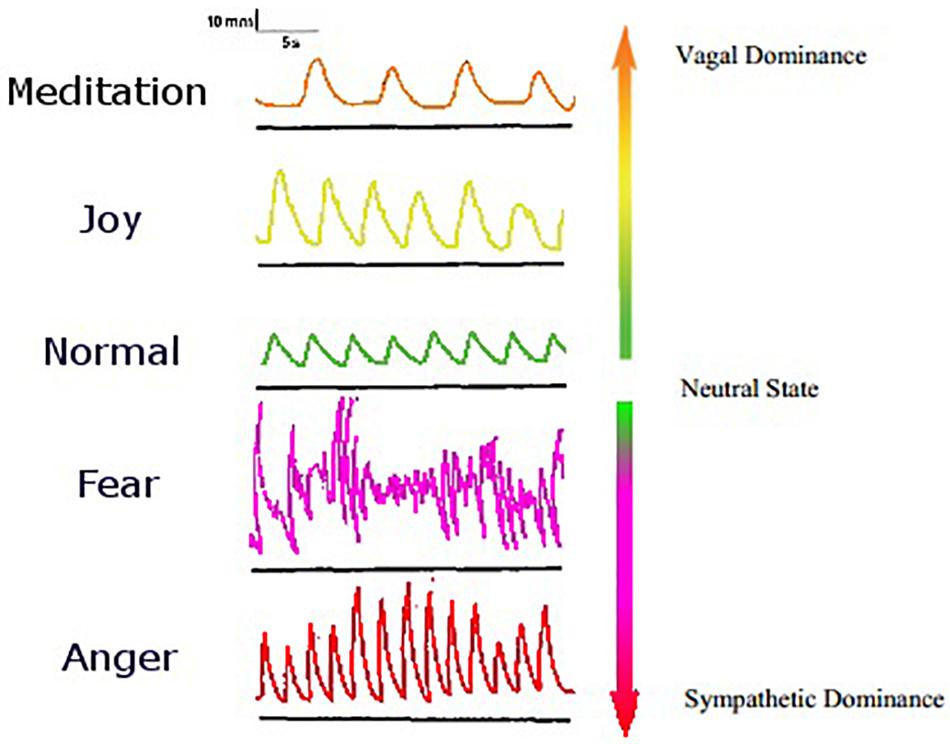
Figure 1. A spectrum of emotion. Affective states are shown here alongside the dimension of respiratory rhythm. The respiratory tracings shown have time on the X-axis and displacement on the Y-axis. Each respiratory state and its corresponding emotion are shown color-coded with their associated autonomic state. This spectrum is only a basic one-dimensional prototype, and future developments using the same fundamental thought could include many other biometrics for highly accurate emotion recognition. A spectrum of color is used to illustrate how biometric data may be translated into user-friendly interfaces for users of related applications and devices to quickly and easily understand their emotional and/or bodily state. A user interface that is powerful in its ability to translate biometric data into an understandable format in relation to emotion and other psychological factors will be crucial in fostering widespread and fruitful use of mind–body applications. Adapted from Kalawski (2003).
We assert that the most powerful method for emotion regulation that can be practiced by the layman pertains to aspects of the mind–body response, the most effective method being breathing techniques such as pranayama. Respiration is special in that it is not only a powerful influence on psychology and physiology but also a body rhythm that is controlled voluntarily. This puts it at the forefront of mind–body techniques.
Conclusion
By revealing the potential nature of the intimate relationship between the body and the mind, specifically regarding emotion, we hope to pave the way for the development of new technologies and interfaces for laymen to monitor and influence their bodily state and thus mental state. Future wearable devices may utilize various physiological signs including respiration, heart rate and HRV indices, electrodermal activity, and more to recognize emotion without disrupting ongoing activities. When used in combination with machine learning techniques, personal and highly advanced recognition could be achieved. We have discussed and reviewed the nature of the emotion–body connection in order to spark innovation and insight and gave a basic example of a physiological spectrum of emotion for use in wearable devices to provide user-friendly and easily understandable interfaces for consumers to assist them in identifying, regulating, and modifying their emotional state.
Author Contributions
RJ developed the theory. CB wrote the manuscript. Both authors did the literature review and contributed to the article and approved the submitted version.
Funding
All funding provided by Charitable Medical Healthcare Foundation.
Conflict of Interest
The authors declare that the research was conducted in the absence of any commercial or financial relationships that could be construed as a potential conflict of interest.
References
Adolphs, R., Damasio, H., Tranel, D., Cooper, G., and Damasio, A. R. (2000). A role for somatosensory cortices in the visual recognition of emotion as revealed by three-dimensional lesion mapping. J. Neurosci. 20, 2683–2690. doi: 10.1523/jneurosci.20-07-02683.2000
Armour, J. A., and Ardell, J. L. (2004). Basic and Clinical Neurocardiology. Oxford: Oxford University Press.
Balzarotti, S., Biassoni, F., Colombo, B., and Ciceri, M. R. (2017). Cardiac vagal control as a marker of emotion regulation in healthy adults: a review. Biol. Psychol. 130, 54–66. doi: 10.1016/j.biopsycho.2017.10.008
Barrett, L. F., Mesquita, B., Ochsner, K. N., and Gross, J. J. (2007). The experience of emotion. Annu. Rev. Psychol. 58, 373–403.
Başar, E. (2008). Oscillations in “brain–body–mind”—A holistic view including the autonomous system. Brain Res. 1235, 2–11. doi: 10.1016/j.brainres.2008.06.102
Boiten, F. A. (1998). The effects of emotional behaviour on components of the respiratory cycle. Biol. Psychol. 49, 29–51. doi: 10.1016/s0301-0511(98)00025-8
Buccelletti, E. (2009). Heart rate variability and myocardial infarction: systematic literature review and metanalysis. Eur. Rev. Med. Pharmacol. Sci. 13, 299–307.
Bulut, N. S., Würz, A., Yorguner Küpeli, N., Çarkaxhıu Bulut, G., and Sungur, M. Z. (2018). Heart rate variability response to affective pictures processed in and outside of conscious awareness: three consecutive studies on emotional regulation. Int. J. Psychophysiol. 129, 18–30. doi: 10.1016/j.ijpsycho.2018.05.006
Cameron, O. G. (2002). Visceral Sensory Neuroscience: Interoception. New York, NY: Oxford University Press.
Celeghin, A., Diano, M., Bagnis, A., Viola, M., and Tamietto, M. (2017). Basic emotions in human neuroscience: neuroimaging and beyond. Front. Psychol. 8:1432. doi: 10.3389/fpsyg.2017.01432
Critchley, H. D. (2002). Review: electrodermal responses: what happens in the brain. Neuroscientist 8, 132–142. doi: 10.1177/107385840200800209
Critchley, H. D., and Harrison, N. A. (2013). Visceral influences on brain and behavior. Neuron 77, 624–638. doi: 10.1016/j.neuron.2013.02.008
Damasio, A., and Carvalho, G. B. (2013). The nature of feelings: evolutionary and neurobiological origins. Nat. Rev. Neurosci. 14, 143–152. doi: 10.1038/nrn3403
Davydov, D. M., Zech, E., and Luminet, O. (2011). Affective context of sadness and physiological response patterns. J. Psychophysiol. 25, 67–80. doi: 10.1027/0269-8803/a000031
Denton, D. A., Mckinley, M. J., Farrell, M., and Egan, G. F. (2009). The role of primordial emotions in the evolutionary origin of consciousness. Conscious. Cogn. 18, 500–514. doi: 10.1016/j.concog.2008.06.009
Dunn, B. D., Dalgleish, T., and Lawrence, A. D. (2006). The somatic marker hypothesis: a critical evaluation. Neurosci. Biobehav. Rev. 30, 239–271. doi: 10.1016/j.neubiorev.2005.07.001
Ekman, P., Levenson, R., and Friesen, W. (1983). Autonomic nervous system activity distinguishes among emotions. Science 221, 1208–1210. doi: 10.1126/science.6612338
Engelen, T., Zhan, M., Sack, A. T., and Gelder, B. D. (2018). The influence of conscious and unconscious body threat expressions on motor evoked potentials studied with continuous flash suppression. Front. Neurosci. 12:480. doi: 10.3389/fnins.2018.00480
Fumoto, M., Sato-Suzuki, I., Seki, Y., Mohri, Y., and Arita, H. (2004). Appearance of high-frequency alpha band with disappearance of low-frequency alpha band in EEG is produced during voluntary abdominal breathing in an eyes-closed condition. Neurosci. Res. 50, 307–317. doi: 10.1016/j.neures.2004.08.005
Hansen, A. L., Johnsen, B. H., and Thayer, J. F. (2003). Vagal influence on working memory and attention. Int. J. Psychophysiol. 48, 263–274. doi: 10.1016/s0167-8760(03)00073-4
Homma, I., and Masaoka, Y. (2008). Breathing rhythms and emotions. Exp. Physiol. 93, 1011–1021. doi: 10.1113/expphysiol.2008.042424
Huang, M. H., Friend, D. S., Sunday, M. E., Singh, K., Haley, K., Austen, K. F., et al. (1996). An intrinsic adrenergic system in mammalian heart. J. Clin. Invest. 98, 1298–1303. doi: 10.1172/jci118916
Jerath, R., Barnes, V. A., and Crawford, M. W. (2014). Mind-body response and neurophysiological changes during stress and meditation: central role of homeostasis. J. Biol. Regul. Homeost. Agents 28, 545–554.
Jerath, R., Beveridge, C., and Barnes, V. A. (2019). Self-regulation of breathing as an adjunctive treatment of insomnia. Front. Psychiatry 9:780. doi: 10.3389/fpsyt.2018.00780
Jerath, R., Crawford, M. W., and And Barnes, V. A. (2015). A unified 3D default space consciousness model combining neurological and physiological processes that underlie conscious experience. Front. Psychol. 6:1204. doi: 10.3389/fpsyg.2015.01204
Jonassen, R., and Landrø, N. I. (2014). Serotonin transporter polymorphisms (5-HTTLPR) in emotion processing: implications from current neurobiology. Prog. Neurobiol. 117, 41–53. doi: 10.1016/j.pneurobio.2014.02.003
Kalawski, J. P. (2003). Effects of Tenderness on Problem Solving. Denton, TX: University Of North Texas.
Karalis, N., and Sirota, A. (2018). Breathing coordinates limbic network dynamics underlying memory consolidation. bioRxiv [Preprint]. doi: 10.1101/392530
Keysers, C., Kaas, J. H., and Gazzola, V. (2010). Somatosensation in social perception. Nat. Rev. Neurosci. 11, 417–428. doi: 10.1038/nrn2833
Kimhy, D., Crowley, O. V., Mckinley, P. S., Burg, M. M., Lachman, M. E., Tun, P. A., et al. (2013). The association of cardiac vagal control and executive functioning–findings from the MIDUS study. J. Psychiatr. Res. 47, 628–635. doi: 10.1016/j.jpsychires.2013.01.018
Kluger, D. S., and Gross, J. (2020). Tidal volume and respiration phase modulate cortico-muscular communication. bioRxiv [Preprint]. doi: 10.1101/2020.01.13.904524
Kövecses, Z. (2000). Metaphor and Emotion: Language, Culture, and Body in Human Feeling. Cambridge: Cambridge University Press.
Kreibig, S. D. (2010). Autonomic nervous system activity in emotion: a review. Biol. Psychol. 84, 394–421. doi: 10.1016/j.biopsycho.2010.03.010
LeDoux, J. (2012). Rethinking the emotional brain. Neuron 73, 653–676. doi: 10.1016/j.neuron.2012.02.004
LeDoux, J. E. (1998). The Emotional Brain: The Mysterious Underpinnings of Emotional Life. New York, NY: Simon & Schuster.
Levenson, R. W. (2006). Blood, sweat, and fears: the autonomic architecture of emotion. Ann. N. Y. Acad. Sci. 1000, 348–366. doi: 10.1196/annals.1280.016
Levenson, R. W., Ekman, P., and Friesen, W. V. (1990). Voluntary facial action generates emotion-specific autonomic nervous system activity. Psychophysiology 27, 363–384. doi: 10.1111/j.1469-8986.1990.tb02330.x
Lewis, J. A., and Cuppari, M. (2009). The polygraph: the truth lies within. J. Psychiatry Law 37, 85–92. doi: 10.1177/009318530903700107
Masaoka, Y., and Homma, I. (2001). The effect of anticipatory anxiety on breathing and metabolism in humans. Respir. Physiol. 128, 171–177. doi: 10.1016/s0034-5687(01)00278-x
Masaoka, Y., Sugiyama, H., Katayama, A., Kashiwagi, M., and Homma, I. (2012). Remembering the past with slow breathing associated with activity in the parahippocampus and amygdala. Neurosci. Lett. 521, 98–103. doi: 10.1016/j.neulet.2012.05.047
McCraty, R., Atkison, M., and Bradley, R. T. (2004). Electrophysiological evidence of intuition: part 1. the surprising role of the heart. J. Altern. Complement. Med. 10, 133–143. doi: 10.1089/107555304322849057
McCraty, R., and Zayas, M. A. (2014). Cardiac coherence, self-regulation, autonomic stability, and psychosocial well-being. Front. Psychol. 5:1090. doi: 10.3389/fpsyg.2014.01090
Mirabella, G. (2018). The weight of emotions in decision-making: how fearful and happy facial stimuli modulate action readiness of goal-directed actions. Front. Psychol. 9:1090. doi: 10.3389/fpsyg.2014.01090.
Moody, E. J., Reed, C. L., Van Bommel, T., App, B., and Mcintosh, D. N. (2017). Emotional Mimicry Beyond the Face?: rapid face and body responses to facial expressions. Soc. Psychol. Pers. Sci. 9, 844–852. doi: 10.1177/1948550617726832
Mukoyama, M., Nakao, K., Hosoda, K., Suga, S., Saito, Y., Ogawa, Y., et al. (1991). Brain natriuretic peptide as a novel cardiac hormone in humans. engineered grafts from human decellularized extracellular matrices: a systematic review and future perspectives. Evidence for an exquisite dual natriuretic peptide system, atrial natriuretic peptide and brain natriuretic peptide. J. Clin. Invest. 87, 1402–1412. doi: 10.1172/jci115146
Nakamura, N. H., Fukunaga, M., and Oku, Y. (2018). Respiratory modulation of cognitive performance during the retrieval process. PLoS One 13:e0204021. doi: 10.1371/journal.pone.0204021
Noguchi, K., Masaoka, Y., Satoh, K., Katoh, N., and Homma, I. (2012). Effect of music on emotions and respiration. Showa Univ. J. Med. Sci. 24, 69–75.
Nummenmaa, L., Glerean, E., Hari, R., and Hietanen, J. K. (2014). Bodily maps of emotions. Proc. Natl. Acad. Sci. U.S.A. 111, 646–651.
Nummenmaa, L., Glerean, E., Viinikainen, M., Jääskeläinen, I. P., Hari, R., and Sams, M. (2012). Emotions promote social interaction by synchronizing brain activity across individuals. Proc. Natl. Acad. Sci. U.S.A. 109, 9599–9604. doi: 10.1073/pnas.1206095109
Nummenmaa, L., Hirvonen, J., Parkkola, R., and Hietanen, J. K. (2008). Is emotional contagion special? An fMRI study on neural systems for affective and cognitive empathy. NeuroImage 43, 571–580. doi: 10.1016/j.neuroimage.2008.08.014
Ottaviani, C., Mancini, F., Petrocchi, N., Medea, B., and Couyoumdjian, A. (2013). Autonomic correlates of physical and moral disgust. Int. J. Psychophysiol. 89, 57–62. doi: 10.1016/j.ijpsycho.2013.05.003
Pace-Schott, E. F., Amole, M. C., Aue, T., Balconi, M., Bylsma, L. M., Critchley, H., et al. (2019). Physiological feelings. Neurosci. Biobehav. Rev. 103, 267–304.
Philippot, P., Chapelle, G., and Blairy, S. (2002). Respiratory feedback in the generation of emotion. Cogn. Emot. 16, 605–627. doi: 10.1080/02699930143000392
Purves, D., Augustine, G. J., Fitzpatrick, D., Hall, W. C., Lamantia, A.-S., Platt, M., et al. (2018). Neuroscience. New York, NY: Sinauer Associates.
Rangel, A., Camerer, C., and Montague, P. R. (2008). A framework for studying the neurobiology of value-based decision making. Nat. Rev. Neurosci. 9, 545–556. doi: 10.1038/nrn2357
Rosenfeld, J. P. (1995). Alternative Views of Bashore and Rapp’s (1993) alternatives to traditional polygraphy: a critique. Psychol. Bull. 117, 159–166. doi: 10.1037/0033-2909.117.1.159
Ross, P., and Atkinson, A. P. (2020). Expanding simulation models of emotional understanding: the case for different modalities, body-state simulation prominence, and developmental trajectories. Front. Psychol. 11:309. doi: 10.3389/fpsyg.2020.00309
Shaffer, F., Mccraty, R., and Zerr, C. L. (2014). A healthy heart is not a metronome: an integrative review of the heart’s anatomy and heart rate variability. Front. Psychol. 5:1040. doi: 10.3389/fpsyg.2014.01040
Shariff, A. F., and Tracy, J. L. (2011). What are emotion expressions for? Curr. Dir. Psychol. Sci. 20, 395–399.
Shi, H., Yang, L., Zhao, L., Su, Z., Mao, X., Zhang, L., et al. (2017). Differences of heart rate variability between happiness and sadness emotion states: a pilot study. J. Med. Biol. Eng. 37, 527–539. doi: 10.1007/s40846-017-0238-0
Stemmler, G. (2004). “Physiological processes during emotion,” in The Regulation of Emotion, eds P. Philippot and R. S. Feldman (Mahwah, NJ: Lawrence Erlbaum Associates Publishers), 33–70.
Strahler, J., Skoluda, N., Kappert, M. B., and Nater, U. M. (2017). Simultaneous measurement of salivary cortisol and alpha-amylase: application and recommendations. Neurosci. Biobehav. Rev. 83, 657–677. doi: 10.1016/j.neubiorev.2017.08.015
Svensson, T. H., and Thorén, P. (1979). Brain noradrenergic neurons in the locus coeruleus: inhibition by blood volume load through vagal afferents. Brain Res. 172, 174–178. doi: 10.1016/0006-8993(79)90908-9
Thayer, J. F., and Fischer, J. E. (2009). Heart rate variability, overnight urinary norepinephrine and C-reactive protein: evidence for the cholinergic anti-inflammatory pathway in healthy human adults. J. Intern. Med. 265, 439–447. doi: 10.1111/j.1365-2796.2008.02023.x
Tsuji, H., Vendittijr, F. J., Manders, E. S., Evans, J. C., Larson, M. G., Feldman, C. L., et al. (1994). Reduced heart rate variability and mortality risk in an elderly cohort. The Framingham heart study. Circulation 90, 878–883. doi: 10.1161/01.cir.90.2.878
Valderas, M. T., Bolea, J., Laguna, P., Vallverdú, M., and Bailón, R. (2015). “Human emotion recognition using heart rate variability analysis with spectral bands based on respiration,” in Proceedings of the 2015 37th Annual International Conference of the IEEE Engineering in Medicine and Biology Society (EMBC), Milano, 6134–6137.
Verschuuren, J., Chuang, L., Rosenblum, M., Lieberman, F., Pryor, A., Posner, J. B., et al. (1996). Inflammatory infiltrates and complete absence of Purkinje cells in anti-Yo-associated paraneoplastic cerebellar degeneration. Acta Neuropathol. 91, 519–525. doi: 10.1007/s004010050460
Williams, M. A., and Mattingley, J. B. (2004). Unconscious perception of non-threatening facial emotion in parietal extinction. Exp. Brain Res. 154, 403–406. doi: 10.1007/s00221-003-1740-x
Wölk, C., and Velden, M. (1989). “Revision of the baroreceptor hypothesis on the basis of the new cardiac cycle effect,” in Psychobiology: Issues and Applications, eds N. W. Bond and D. Siddle (North-Holland: Elsevier Science), 371–379.
Wood, A., Rychlowska, M., Korb, S., and Niedenthal, P. (2016). Fashioning the face: sensorimotor simulation contributes to facial expression recognition. Trends Cogn. Sci. 20, 227–240. doi: 10.1016/j.tics.2015.12.010
Wu, N., Jiang, H., and Yang, G. (2012). “Emotion recognition based on physiological signals,” in Advances in Brain Inspired Cognitive Systems, eds H. Zhang, A. Hussain, D. Liu, and Z. Wang (Berlin: Springer), 311–320.
Xiefeng, C., Wang, Y., Dai, S., Zhao, P., and Liu, Q. (2019). Heart sound signals can be used for emotion recognition. Sci. Rep. 9:6486.
Yu, X., Fumoto, M., Nakatani, Y., Sekiyama, T., Kikuchi, H., Seki, Y., et al. (2011). Activation of the anterior prefrontal cortex and serotonergic system is associated with improvements in mood and EEG changes induced by Zen meditation practice in novices. Int. J. Psychophysiol. 80, 103–111. doi: 10.1016/j.ijpsycho.2011.02.004
Zaccaro, A., Piarulli, A., Laurino, M., Garbella, E., Menicucci, D., Neri, B., et al. (2018). How breath-control can change your life: a systematic review on psycho-physiological correlates of slow breathing. Front. Hum. Neurosci. 12:353. doi: 10.3389/fnhum.2018.00353
Zhang, J.-X., Harper, R. M., and Frysinger, R. C. (1986). Respiratory modulation of neuronal discharge in the central nucleus of the amygdala during sleep and waking states. Exp. Neurol. 91, 193–207. doi: 10.1016/0014-4886(86)90037-3
Keywords: respiration, respiratory rhythm, emotion, emotion recognition, autonomic nervous system, health monitoring
Citation: Jerath R and Beveridge C (2020) Respiratory Rhythm, Autonomic Modulation, and the Spectrum of Emotions: The Future of Emotion Recognition and Modulation. Front. Psychol. 11:1980. doi: 10.3389/fpsyg.2020.01980
Received: 26 April 2020; Accepted: 16 July 2020;
Published: 14 August 2020.
Edited by:
Sonja A. Kotz, Max Planck Institute for Human Cognitive and Brain Sciences, GermanyReviewed by:
Paddy Ross, Durham University, United KingdomGiovanni Mirabella, University of Brescia, Italy
Copyright © 2020 Jerath and Beveridge. This is an open-access article distributed under the terms of the Creative Commons Attribution License (CC BY). The use, distribution or reproduction in other forums is permitted, provided the original author(s) and the copyright owner(s) are credited and that the original publication in this journal is cited, in accordance with accepted academic practice. No use, distribution or reproduction is permitted which does not comply with these terms.
*Correspondence: Ravinder Jerath, Umo2MDVyQGFvbC5jb20=