- 1JSPS International Research Fellow, Research Center for Applied Perceptual Science, Kyushu University, Fukuoka, Japan
- 2Unit of Perceptual Psychology, Dept. Human Science, Research Center for Applied Perceptual Science, Division of Auditory and Visual Perception Research, Research and Development Center for Five-Sense Devices, Kyushu University, Fukuoka, Japan
In the field of psychology, the merge of decision-theory and neuroscientific methods produces an array of scientifically recognized paradigms. For example, by exploring consumer’s eye-movement behavior, researchers aim to deepen the understanding of how patterns of retinal activation are being meaningfully transformed into visual experiences and connected with specific reactions (e.g., purchase). Notably, eye-movements provide knowledge of one’s homeostatic balance and gatekeep information that shape decisions. Hence, vision science investigates the quality of observed environments determined under various experimental conditions. Moreover, it answers questions on how human process visual stimuli and use gained information for a successful strategy to achieve certain goals. While capturing cognitive states with the support of the eye-trackers progresses at a relatively fast pace in decision-making research, measuring the visual performance of real-life tasks, which require complex cognitive skills, is tentatively translated into clinical experiments. Nevertheless, the potential of the human eye as a highly valuable source of biomarkers has been underlined. In this article, we aim to draw readers attention to decision-making experimental paradigms supported with eye-tracking technology among clinical populations. Such interdisciplinary approach may become an important component that will (i) help in objectively illustrating patient’s models of beliefs and values, (ii) support clinical interventions, and (iii) contribute to health services. It is possible that shortly, eye-movement data from decision-making experiments will grant the scientific community a greater understanding of mechanisms underlining mental states and consumption practices that medical professionals consider as obsessions, disorders or addiction.
To consider the state of the decision-maker
translated by the beholder’s eyes is our goal
Introduction
Consumers make decisions at their own pace. Their choices are significantly influenced by personal preferences, situational context of the decision, such as presence of time pressure and size of the opportunity set (a number of given alternatives) as well as the environment of the point of purchase (Baldwin et al., 2012; Venkatraman et al., 2012; Berčík et al., 2016; Spence et al., 2016; Cherubino et al., 2019; Lin et al., 2019; Wolf et al., 2019; Vriens et al., 2020). Importantly, consumers are usually not aware of the steps of simplifying the decision processes by eliminating (ignoring) some information and paying attention (giving more time) to certain, considered options. Hence, technological advances that enable to isolate key processes, which underlay individuals’ preferences and reactions (e.g., buying behavior), attain increasing attention of the media, user analysts and researchers (Hubert and Kenning, 2008; Chynal et al., 2016; Oliveira et al., 2016; Gidlöf et al., 2017; Touchette and Lee, 2017; Spence, 2019).
The application of neurophysiological tools recording brain measures (e.g., electrical brain activity, functional near-infrared spectroscopy, BOLD-contrast imaging used in functional magnetic resonance imaging, fMRI) and non-brain measures (e.g., electrodermal response, heart rate, eye-tracking), as adjuvant instruments to behavioral data in marketing research, is not a new concept (Levy et al., 2011; Berčík et al., 2016; Cherubino et al., 2019). Studies of eye-movements through direct observations were conducted already in the 1800s. An early form of an eye-tracker was built by Edmund Huey in 1908 and first non-intrusive eye-tracker was constructed by a pioneer in experimental educational psychology — Guy Thomas Buswell, known for groundbreaking investigations on recording and analyzing subjects’ eye movements (Buswell, 1935). Buswell’s results indicated that observers often fixated on the same spatial locations in an image, but not necessarily in the same temporal order. Moreover, the viewers’ eyes tended to focus on foreground elements (e.g., faces and people) rather than background elements (e.g., clouds or foliage). In 1945, Brandt, 1945 published a general analysis of eye movement patterns of participants, who looked at advertisements. Similarly to Buswell, Brandt concluded that there are noticeable, individual differences in eye movements, but in general, these behaviors are similar enough in order to formulate “psychological laws” (see Babcock et al., n.d.)
The work on the relationship between eye-movements and the sequence of thought processes has been greatly extended by a Russian psychologist Alfred Lukyanovich Yarbus, who believed that the viewers’ eyes were directed toward areas of the stimulus that were “useful or essential” to perception. Recorded eye-movements of examining Ilya Repin’s painting “An Unexpected Visitor,” provided key findings on the substantial influence of experimental task on viewer’s eye-movements (kindly refer to the book Yarbus, 1967, which is often quoted among researchers investigating the relationship between fixations and interest, Mackworth and Bruner, 1970; Noton and Stark, 1971; Walker Smith et al., 1977; Hayhoe and Ballard, 2005; Unema et al., 2005; Castelhano et al., 2009; Greene et al., 2012; Borji and Itti, 2014; Martinez-Conde and Macknik, 2015; Boisvert and Bruce, 2016; Smith et al., 2018).
“(…) Eye movement reflects the human thought processes; so the observer’s thought may be followed to some extent from records of eye movement (the thought accompanying the examination of the particular object). It is easy to determine from these records which elements attract the observer’s eye (and, consequently, his thought), in what order, and how often.” (Yarbus, 1967, p. 190)
Until now, human eye-positions receive much attention to gain objective insights into how consumers sample information, make decisions under different task-instructions or mind-wander, where the slightest change in gaze allocation reflects a shift in information-prioritization (Yarbus, 1967; Baron-Cohen et al., 1997; Najemnik and Geisler, 2005, 2009; Castelhano et al., 2009; Tatler et al., 2010; Morii and Sakagami, 2015; Boisvert and Bruce, 2016).
Principally, in order to find the optimal outcome, decisional processes such as (i) assessment and formation of preferences, (ii) selection and execution of action(s), (iii) experiencing the outcome, are all orchestrated in human brain systems. A combination of eye-tracking methodology and decision-making (high-level information processing) paradigms can result in understanding one’s cognitive and affective mental state and explain reactions in a real-world context (Bogacz et al., 2006; Bogacz, 2007; Paulus, 2007; Cocker et al., 2012). Moreover, there is growing evidence that decision-making and homeostatic processing are inextricably linked, and that cognitive impairments (e.g., disturbances of memory, attention deficits and difficulty in problem-solving) among clinical populations cannot be understood without the reference to the performance of independent living skills (Brand et al., 2005; Paulus, 2007; Bachman et al., 2010).
Since eye-tracking instruments objectively reflect action selection, based on decision maker’s attention, information processing capabilities, and motivated cognition processes (Deubel and Schneider, 1996; Ernst and Paulus, 2005; Simion and Shimojo, 2006; Glaholt and Reingold, 2009; Markkula, 2015; van der Laan et al., 2015; Gerbella et al., 2017; Onuma et al., 2017; Wolf et al., 2018, 2019), it should not be overlooked that relatively cost-effective and non-invasive eye-tracking devices are becoming vital instruments exposing the mechanism of cognitive and perceptual disturbances among clinical populations (Tseng et al., 2013; Türkan et al., 2016; Sawada et al., 2017; Morita et al., 2020; Wolf et al., 2021).
Visible Impairments in Information Processing
Longitudinal persistence of exhibited deficits in intellectual functioning, i.e., attention, memory and executive function, suggest that information processing abnormalities are related to the core of a vast number of mental disorders. For example, Autism spectrum disorder (ASD) adults and children have been reported to spend less time looking at silent features of face stimuli (mouth, nose, and eyes regions) and more time at non-social stimuli than healthy controls (HC) (Corden et al., 2008; Frazier et al., 2016). Furthermore, while viewing video clips, young ASD adults have been reported to spend more time gazing at objects (instead of people). These observations support a poorer social adjustment among ASD patients, where by fixating on non-social stimuli, ASD patients are predestinated to miss socially important cues (Klin et al., 2002).
In the context of eating disorder group, it has been suggested that the majority of studies investigated the attentional bias toward disorder-relevant stimuli, which was based on reaction times that measured attention indirectly, i.e., without revealing its time course (Shafran et al., 2007; Sperling et al., 2017; Kerr-Gaffney et al., 2019). With the use of eye-tracking technology, however, it is possible to measure attention directly, where for example measurements of the overall viewing time, scan-path length, number of saccades, and the differentiation between early and late stages of attentional processing are achievable (Giel et al., 2011). Jansen and colleagues found that participants with eating disorders, when viewing photos of their own bodies, focused less on their self-defined beautiful body parts and more on their ugly parts than the HC group did (i.e., inspection of the ugly body parts was given priority). However, when viewing pictures of others, the eating disorder group showed the opposite pattern (Jansen et al., 2005).
Recent studies, which investigated food and non-food stimuli under free-viewing task, report that both groups: binge eating disorder (BED) and HC, tend to fixate their gaze longer on non-food images. However, when comparing the total viewing time for food pictures, those with BED visually attended to them for a longer time duration than HC (Schag et al., 2013; Schmidt et al., 2016; Sperling et al., 2017). Interestingly, Baldowski and his team reports that individuals with night eating syndrome show an initial orienting bias toward food stimuli over non-food images (Baldofski et al., 2018).
It has been reported that individuals with social anxiety disorder show a quicker attentional disengagement from the eyes, in line with the vigilance-avoidance theory of attention (Horley et al., 2003, 2004). In two experiments reported by Boll and colleagues, patients with social phobia and HC were compared regarding their gaze behavior toward displayed photos of human faces (Boll et al., 2016). The results from performed paradigms (emotion classification paradigm and gaze-cueing paradigm) indicate that in comparison to HC, patients reflexively orient their attention toward the eyes of emotional faces in the emotion classification paradigm. This initial hypervigilance for the eye region can be observed at very early attentional stages and persists for a longer duration of time. Moreover, Boll and colleagues reported that individuals with social phobia exhibit a delayed attentional orienting into the direction of eye gaze. This observation may suggest a differential time course of eye gaze processing in individuals with social phobia and HC.
The fundamental issue of eye behavior reflecting attention and its deficits (Yarbus, 1967; Braff, 1993; Rommelse et al., 2008; DeAngelus and Pelz, 2009; Borji and Itti, 2014) attracts the scientific community as a measurable indicator of one’s sequence of thoughts (Bird et al., 2012; Thakkar et al., 2018; Wolf et al., 2018, 2019). Therefore, we put forward the statement that eye-movement measurements gathered from visual information-processing paradigms are already supporting the development of integrated eye movement scores (kindly refer to Morita et al., 2017; Obyedkov et al., 2019).
Information processing deficits are an important area of investigation focusing on identifying the key features of life-impoverishing disorders that may allow efficient early intervention and support the discovery of preventive treatments. Moreover, for the reason that almost every area of the brain plays a role related to the ocular motor control system, the implementation of eye-tracking technology to cognitively informative tasks, has proven to be a fruitful approach in uncovering human information processes (Jarodzka et al., 2010; Glaholt and Reingold, 2011; Lai et al., 2013; Pärnamets et al., 2015; Roberts et al., 2018). However, mental health professionals avoid capturing eye-data through tasks that may have “too unusual demands” or could be labeled as “too complicated.” This may explain why real-life oriented decision-making paradigms are hesitantly used to discover the mechanisms that underlie non-optimal (non-homeostatic) behavior among clinical populations.
Loss of Social Autonomy Due to Cognitive Impairments
Unequivocally, scientists need to be thoughtful about patients, who suffer from psychiatric disorders such as schizotypal personality disorder (STPD), schizophrenia (SZ), major depressive disorder (MDD), bipolar disorder (BD), and ASD (which entails a spectrum of disorders, namely autism, Asperger’s disorder, and pervasive developmental disorder-not otherwise specified, PDD-NOS). While experiencing various cognitive impairments, clinical populations may face disinterest in social contact, discomfort in interpersonal situations, decreased ability to feel pleasure (physical anhedonia), and/or diminished experience of reward (Cocker et al., 2012). Notably, some patients are being unable to independently execute instrumental activities of daily living, e.g., managing money, commuting, preparing meals, shopping. For example, BP patients may excessively involve in activities with high potential for painful consequences (e.g., engaging in unrestrained buying sprees or impulsive business investments), being therefore at a higher risk of gambling and compulsive spending. Overall, clinical populations are either omitted as decision-makers, facing a loss of social autonomy (Mottron et al., 2006; Sablier et al., 2009), or taken advantage of due to the inability to control their consumption practices (Jones et al., 2015).
Therefore, it is crucial to identify the relationship between cognitive deficits and specific activities among people with psychiatric diseases. In order to do so, few research groups considered designing experimental paradigms to resemble a real-life tasks (Hamera and Brown, 2000; Rempfer et al., 2003; Zayat et al., 2011; Laloyaux et al., 2012, 2013). In 2002 Hamera and colleagues conducted a grocery shopping skill test, measuring the performance of shopping ability, which requires complex cognitive skills (Hamera et al., 2002). In this particular study, the performance of two groups has been compared, i.e., individuals with significant cognitive impairments (SZ and BD patients) and a normative population of HC. In particular, the researchers reported that individuals with SZ and BD took longer time in task completion. Besides, the group with significant cognitive impairments showed higher redundancy in performing the shopping task than the healthy control group.
Moreover, in another shopping task investigating buying performance, where participants were required to purchase grocery store items from a shopping list, Laloyaux et al. (2013) significantly differentiated BD patients from HC for two variables, namely (1) the total time to complete the shopping and (2) the mean time spent to consult the grocery list. The same research group reported that the performance of a shopping task correlated significantly with individual’s cognitive functioning (i.e., processing speed, verbal episodic memory, planning, and cognitive flexibility) and with clinical variables, such as duration of illness and functioning in an authentic life context (Laloyaux et al., 2013).
Described examples provide evidences of reliability and validity of context-based tests related to purchase intentions and shopping performance measurement (Manee, 1997; Hamera and Brown, 2000; Rempfer et al., 2003). Also, current advances in eye-tracking technology and data analysis (McGrath et al., 2018; Zommara et al., 2018) allow the scientific community to conduct follow-up experiments (and/or build new ones) in order to provide a deeper knowledge concerning how cognitive deficits impact clinical population in a real-world functioning context.
Future decision-making paradigms may provide promising reaction-derived data, disclosing repressed feelings, unconscious reactions/habits. This may help to clarify the basis of high heritability, associated with psychiatric disorders, and promoting transdiagnostic research. Moreover, it may refine the diagnosis procedure, which is highly challenging, especially in heterogeneous disorders such as schizophrenia (see Koychev et al., 2011). The implementation of eye-tracking methodology appears to be a straightforward undertaking. Through the detected position of the viewer’s pupil, gaze points can be easily determined and further analyzed with the use of mathematical algorithms. Hence, the implementation of eye-tracking technology fits perfectly the aim to non-invasively disclose valuable information regarding individual’s behavior, which cannot be as precisely discovered through written nor verbal approaches (Riby and Hancock, 2008; Dewhurst et al., 2012; Chawarska et al., 2013; Maruta et al., 2014; Diwakar et al., 2015; Fujioka et al., 2016; Crawford et al., 2017; Oyama et al., 2019).
Revealing One’s Moment-by-Moment Focus of Attention
It has been stated that eye-movement technology provides a moment-by-moment measure of the focus of attention and reveals what cognitive strategies (interplay of top-down and bottom-up processing) are employed to solve a particular task (Eckstein et al., 2017). In the past years, experts have used pupil dilation data to reveal for example the subjective difficulty of cognitive tasks and their intensity (mental effort). The choice of this parameter has been supported with a common knowledge that pupil dilation provides a window into the brain’s locus coeruleus-norepinephrine (LC-NE) system (Laeng et al., 2012; Burkhouse et al., 2015; Chevalier et al., 2015; Bast et al., 2020). Neurophysiological findings provide significant insights to the meaning of pupillary responses for mental activity. Given that pupillary responses can be easily measured in a non-invasive manner in each stage of life, and can occur in the absence of conscious processes, they constitute a promising tool for the study of preverbal (e.g., infants) and non-verbal participants.
Furthermore, spontaneous eyeblink rate (sEBR), as a proxy of dopaminergic activity (Jongkees and Colzato, 2016; Eckstein et al., 2017; Gotlieb et al., 2021) has been used to study cognition, interest, and predict stress levels in humans (Chermahini and Hommel, 2010; DeYoung, 2013). Since spontaneous blinks are uniquely different from voluntary and reflexive eye-blinks, they represent a range of information processing functions spanning attention and working memory (Müller et al., 2007; Schumacher et al., 2013; Groman et al., 2014). For example, humans subjected to stressful stimuli (through social and emotional recollection tests) were reported to exhibit an increase in spontaneous blink rate. Several studies reported that increased eyeblink rate reflected an increasing level of fatigue (Beatty, 1982; Borghini et al., 2014; Gergelyfi et al., 2015). On the other hand, spontaneous blink rate has been found to decrease when the subjects are most attentive in performing demanding tasks associated with memory operations and attentive behaviors (see Hirokawa et al., 2004; Brefczynski-Lewis et al., 2011; Paprocki and Lenskiy, 2017). Moreover, eye blink parameters enable to investigate the influence of specific stressors that initiate emotional anxiety or neurological levels of arousal (van de Groep et al., 2017).
Area of interest (AOI) that is not categorized as a gaze metric by itself but defines regions by which gaze metrics may be calculated, has been reported to help in studying reasoning on account of integrating several pieces of information and reflecting the process of comparing specific locations of presented stimuli (Dewhurst et al., 2012; Hunter et al., 2020). Recently, to assess cognitive function using high-performance eye-tracking technology, Oyama and colleagues developed a novel cognitive assessment tool (Oyama et al., 2019), which has been reported as brief and practical since the subject simply views a series of short movies and pictures displayed on a monitor. In each task, multiple stimuli, including the target image (correct answer) and non-target images (distractors), are presented on the monitor. The subject is instructed to identify and focus her gaze on the correct answer. The AOI is set on the correct answer, and the cognitive score is determined from the eye-movement data by measuring the fixation time on the region of the correct answer (kindly refer to Figure 1). Oyama and colleagues concluded that the cognitive score correlated well with the scores from neuropsychological tests, showing an outstanding diagnostic performance in detecting patients with mild cognitive impairment (MCI) and dementia (Oyama et al., 2019).
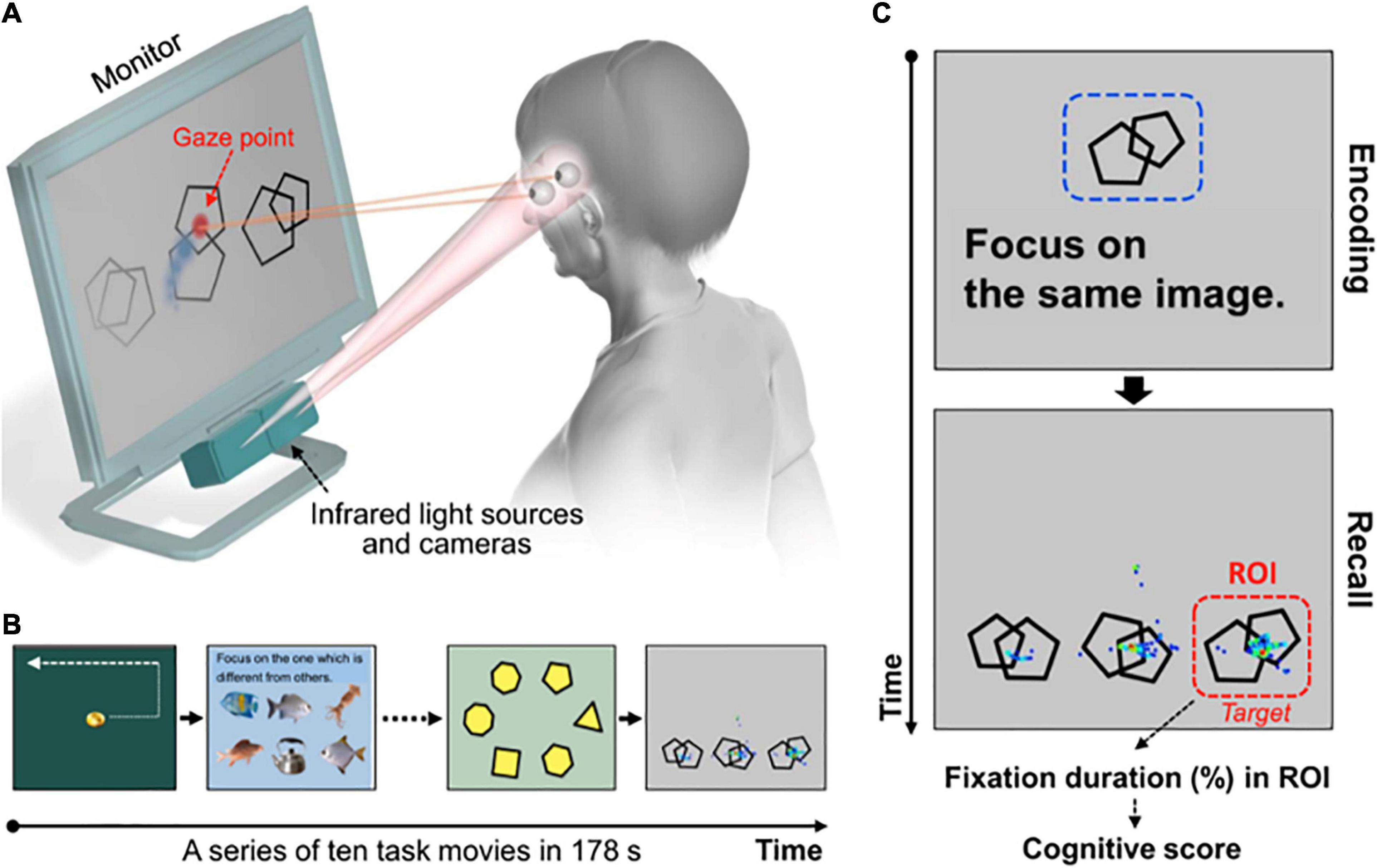
Figure 1. Rapid cognitive assessment using an eye-tracking system and tasks, obtained from Oyama et al. (2019). (A) Participant’s gaze points are recorded. (B) Ten tasks are displayed one by one on the monitor. (C) An example of a working memory task and representative gaze plots with a duration-based heatmap obtained from a control subject. Gaze plots represent the location and time spent looking at the objects. A cue object (e.g., a set of double pentagons) is presented for 10 s (encoding phase), followed by three distinct objects with the same one as the cue object (right bottom on the monitor, target). In the next phase (recall) the participant is asked to remember and gaze at the target object. Fixation duration within the region of interest (ROI) set on the target object is used to obtain the cognitive score.
Next, scanning patterns have been widely covered in the scientific literature, giving insight into one’s exploration behavior, directly related to the nature of the task (Kojima et al., 1992; Bestelmeyer et al., 2006; Castelhano et al., 2009; Risko et al., 2012; Sprenger et al., 2013; Helo et al., 2014; Dowiasch et al., 2016; Yazdan-Shahmorad et al., 2020). Recently, exercising the differences between subjects’ visual scanning patterns Chung et al. (2018) could disambiguate bipolar and unipolar patients with high accuracy (Chung et al., 2018). Since misdiagnosing BD as MDD is relatively common, the introduction of biomarkers to improve diagnostic accuracy, in the earliest course of the illness, plays an important role in the clinical field. With the use of recurrent neural network (RNN), the differences between fixation sequences provided a classifier that disambiguated BD from MDD patients with a remarkably high accuracy.
Finally, gaze duration (viewing time) that reflects (i) the quality of the available visual information, (ii) the contextual background with which objects can be recognized and understood, (iii) individual goals of the observer, and (iv) information-search strategies (Underwood et al., 2008; Najemnik and Geisler, 2009; Schotter et al., 2010; Foulsham and Kingstone, 2011, 2013; Pierce et al., 2016; Roux et al., 2016; Saito et al., 2017) has been pointed out to be a useful index of the extent of information processing (Bird et al., 2012; Wolf et al., 2018, 2019). Moreover, Wolf and colleagues suggested that uncertainty can prolong the effort of visual processing (Wolf et al., 2019).
Taken together, data commonly captured by eye-trackers such as (i) eye opening and closure (e.g., blink duration, blink frequency), (ii) gaze parameters (e.g., number of fixations, saccades, viewing time), (iii) pupil properties (e.g., pupil dilation, pupil size), give valuable insights into how a viewer (un)consciously filters information, undertakes the decision strategy, and determines the subjective hierarchy of perception. Moreover, human eye-movements have illustrated valuable insights regarding the information processing patterns, translated into attentional landscapes, fixation sequences, or heat maps, and served as measurable outputs of the extent of undergoing cognitive processes (Bird et al., 2012; Pärnamets et al., 2016; Zommara et al., 2018). In conclusion, recordings of the eye-movements provide answers to important questions of when and how visual information is being captured and processed in scientifically controlled as well as ecologically valid environments (for outstanding work on implementing ecologically valid paradigms to understand psychiatric disorders, refer to Scholl and Klein-Flügge, 2018).
Thanks to the neuroscientific tools, neurological representations of the brain and neural activity can be generated. The purpose is straightforward, to have real-time insights into specific responses in the brain and nervous system, resulting from the presentation of a stimulus (Lim, 2018). Especially, eye-tracking technique provides a relatively low-cost and sensitive indicator for initial orienting, shift, and maintenance of attention (Caseras et al., 2007; Kou et al., 2016; Wolf et al., 2021). Since eye-movements provide realistic evidence of where participants are likely to look at Bird et al. (2012); Morii and Sakagami (2015); van der Laan et al. (2015); Wolf et al. (2018, 2019), this might be a valid reason why some research groups choose precisely the eye-tracking technique to study attentional biases among individuals with body dysmorphic disorder (BDD) (Kou et al., 2016), which is characterized by repetitive behaviors and/or mental acts occurring in response to preoccupations with perceived defects or flaws in physical appearance (American Psychiatric Association, 2013). For instance, to explore attentional bias toward body-related pictures among females with weight dissatisfaction Gao and colleagues employed the eye-movement technique. The researchers reported an orienting-maintenance pattern of attention toward fatness-related pictures among weight-dissatisfied women, implying that this group preferentially attended to body-related/fatness-related stimuli (Gao et al., 2012). In addition, results presented by Greenberg and her team also suggest that individuals with BDD “overfocus on negative attributes” (Greenberg et al., 2014). “While current treatments generally show moderate effectiveness in the short-term, those with BDD can have high relapse rates, as they still “see” their flaws or defects” (Beilharz et al., 2018). Therefore, elucidating the role of attention (e.g., negative/positive bias) may help to identify risk and maintenance factors among BDD patients (Grocholewski et al., 2012; Greenberg et al., 2014; Kollei et al., 2017). For example, in 2018, Beilharz and her colleagues proposed a visual training program (encompassing basic visual processing, face and emotion recognition, and self-perception), which has been designed to remediate visual abnormalities and reduce symptom severity among individuals with BDD (Beilharz et al., 2018).
In another elegant experiment, supported with the eye-tracking methodology, Toh and colleagues investigated facial affect recognition in BDD and obsessive-compulsive disorder patients. Relative to OCD patients and HCs, patients with BDD demonstrated (i) significantly poorer facial affect perception (misidentifying emotional expressions as angry, i.e., BDD participants misinterpreted more faces as angry in comparison to OCD patients and HCs); (ii) atypical scanning strategy characterized by significantly more blinks, fewer but longer fixations, and less visual attention devoted to the salient facial features (Toh et al., 2015). With this in mind, in the future, researchers may consider developing interventions that will reduce distress for individuals with high body image dissatisfaction (for future directions for positive body image research refer to Halliwell, 2015).
The scientific community is already witnessing a considerable rise in gaze metrics models targeting to unveil one’s values (goals, tradeoffs, objectives) and beliefs (facts, opinions, uncertainties), tapping into unconscious processes governed by individuals’ internal state (homeostasis). Clinicians have actively grasped the technological advances in vision science, demonstrating characteristic eye-movement distortions in patients with psychiatric disorders or individuals being potentially at risk of them (kindly refer to an illustrative example in Figure 2). An extensive number of clinical findings reported abnormal gaze parameters among clinical populations (e.g., prolongation of saccadic latency, abnormal smooth pursuit, ocular drift (glissades), square wave jerks, and impaired vestibulo-ocular reflex) (see Silverstein et al., 2015).
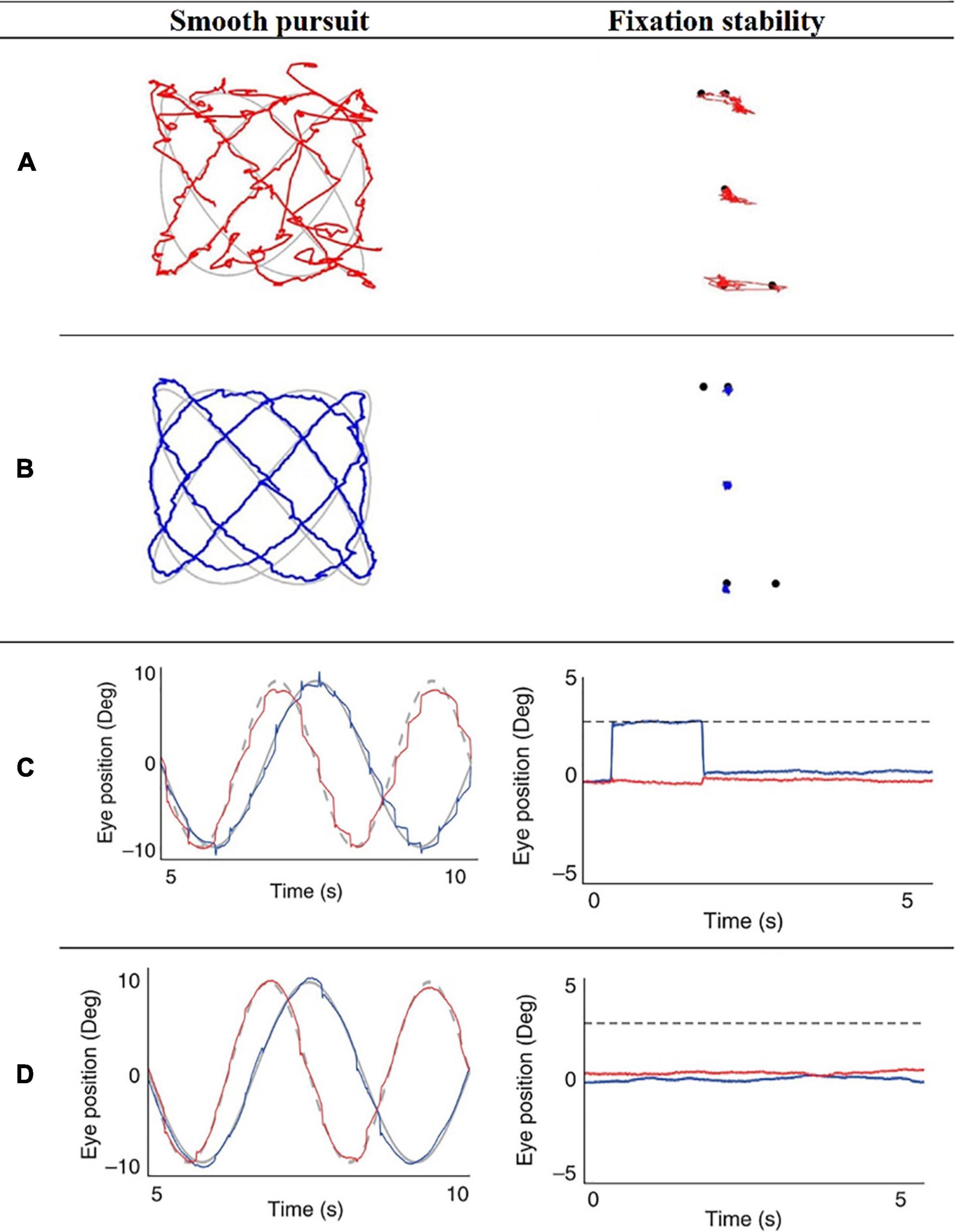
Figure 2. Ilustrative eye-movement recordings of pursuit and fixation stability tests of schizophrenia (SZ) patients and healthy controls (HC), obtained from Benson et al. (2012) and Morita et al. (2017). Importantly, the reader should bear in mind that not every participant will exhibit “normal/abnormal” eye-movements on every trial nor on each task. Illustrative Lissajous pursuit and fixation stability tests of SZ patient (A) and HC (B), obtained from Benson et al. (2012). Representative eye-movement recordings of a patient with SZ (C) and one HC (D), during the smooth pursuit eye movement test (fast Lissajous paradigm) and the fixation stability test (far distractor paradigm), obtained from Morita et al. (2017).
A great number of the clinical results (especially in the schizophrenia research domain) refers to now-classic paradigms, i.e., smooth pursuit test (where participants are required to track a moving target with their gaze) and fixation stability test (where participants are asked to maintain their gaze on a defined fixation point). Both paradigms are known for their overwhelming evidence in the scientific literature (Holzman et al., 1973, 1974, 1980; Holzman, 1985; Koychev et al., 2011), and are highly important to study attentional deficits. For example, it has been reported that SZ patients (and their biological family members) perform the smooth pursuit test inaccurately. Moreover, gaze maintenance on a single target (fixation) is unsteady among SZ patients. Besides, their first-degree relatives have been reported to be deficient in fixation maintenance as well (Benson et al., 2012).
To disclose one’s exploratory eye movement behavior, free-viewing paradigms are also widely applied in clinical research domain (Kogata and Iidaka, 2018; van Renswoude et al., 2018; Lakhlifi et al., 2020) (Figure 3). Abnormalities in visual scanning have been reported to afford an impressive accuracy of specificity and sensitivity. First, by demonstrating usefulness in quantitative scoring and sensitive detection of cognitive impairments and next, by tapping the subtype of mental illness (Beedie et al., 2011; Li et al., 2016, 2020a; Oyama et al., 2019).
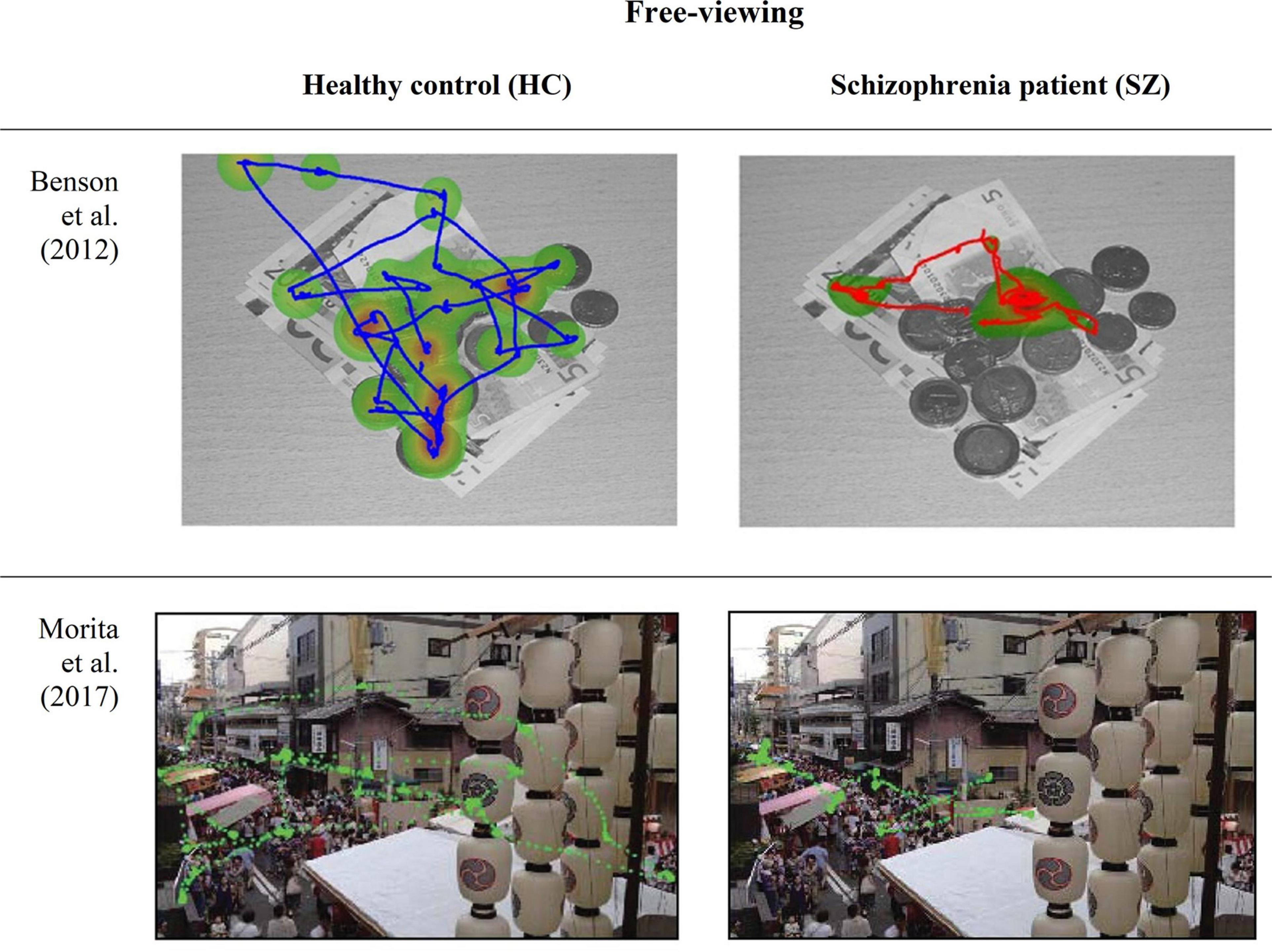
Figure 3. Eye-movement recordings of a patients with schizophrenia (SZ) and healthy controls (HC) during a free-viewing paradigm, obtained from Benson et al. (2012) and Morita et al. (2017).
Although now-classic (i.e., smooth pursuit and fixation stability) and free-viewing tests are highly visible in clinical experiments as routine screenings, simple and sufficiently short real-life decision-making paradigms supported with an eye-tracking technology, are scarcely being applied in a form of a screening tool for the early detection of cognitive decline (and related with it disorders). It is not in the scope of our article to list the benefits of an early diagnosis; however, we find it crucial to mention that it can give individuals at risk the opportunity to benefit from symptomatic treatments (Barnett et al., 2014, cited in Oyama et al., 2019). Additionally, the early detection of cognitive impairments can increase the efficiency of patient recruitment for clinical trials of drug development, which has shifted to focus on the early stage of some diseases (Graham et al., 2017; Carl et al., 2020).
Hence, to address the investigation on how clinical populations approach real-life functioning tasks, new research questions require analysis of decision-making paradigms that may provide informative attentional scan-paths and explorative eye-movements, beyond the quantification of fixations and saccades (Wolf et al., 2021). Furthermore, since decision-making paradigms fundamentally relate to the individual’s homeostatic processing, it seems straightforward to actively translate them into clinically performed experiments, supported with non-invasive eye-tracking devices.
Translational Practice
Reflecting cognitive states of the viewer, eye-movement measurements have been reported to find potential application in the translational clinical practices. For instance, in the form of biomarkers, they may be used to identify individuals at risk, allowing efficient management of the process of early intervention. Therefore, in this part or the article, we anticipate how the knowledge from decision-making (information processing) paradigms that implement eye-tracking technology, can provide a beneficial platform for economists, psychiatrists, neurologists, and social and clinical psychologists to develop a common language for studying context-laden behavior in psychiatric disorders (Paulus, 2007). Such an approach may broaden current knowledge of mechanisms, which underlie illnesses and maladies (e.g., eating disorder, obsessive-compulsive personality disorder, pathological gambling) characterized by individuals’ inability to control their consumption practices (Javor et al., 2013).
Misconception of Integrating Neuromarketing Into Clinical Experiments
It is important to solve the misconception of integrating neuromarketing into clinical experiments. In neuromarketing research, physiological and neuroscientific methods are being actively used to study consumer behavior to better understand the underlying neurobiology of psychological phenomena of decision-making processes, as well as to provide a more comprehensive assessment of the efficacy of marketing strategies such as advertising campaign planning or product positioning (for an excellent description on the most pertinent neuroscientific methods recommended for neuromarketing, kindly refer to Lim, 2018). Thus, neuromarketing may enclose a two-way interpretation of potential application: (1). scientific, focusing on consumer’s decision-making (action selection, from a set of available options) and (2). commercial (for-profit), requiring scientific findings in order to establish the most profitable business strategies for consumers to purchase a certain product (Gidlöf et al., 2013, 2017; for a comprehensive debate on neuromarketing and consumer neuroscience, see Javor et al., 2013).
In recent years, due to sensational journalism, concerns related to subliminal advertising as a form of external purchase manipulations have emerged (for an insightful discussion on “Consumer Surveillance and Ethical Concerns” see Nemorin and Gandy, 2017). These misconceptions (i.e., scientifically unsupported controversial anecdotes) influence the academic efficacy and practical utility of neuroscientific measurement techniques in understanding the human decision-making processes (Pop et al., 2014; Thomas et al., 2016; Lim, 2018). Although Pop et al. (2014) clearly stated that “One of the most important challenges for companies who offer neuromarketing services is to stick to ethical principles when performing the investigations. This is an obligation they have both toward the beneficiaries – the companies providing products or services – and toward their consumers as well (…),” the dispute between the advocates and critics of neuromarketing remains present until today (Foscht and Swoboda, 2011; Pop et al., 2014).
Not to distract this highly important discussion, consumer neuroscience (that enriches understanding of consumer psychology and behavior) and neuroeconomics (that refers to sensemaking of economic problems through the analysis of neural correlates of decision making) should be studied, among healthy controls and clinical populations (Rahman et al., 2001; Nemorin and Gandy, 2017; Wolf et al., 2021). The decisive advantage is that with the support of neuromarketing and its neuroscientific methods the reasons behind individuals’ decisions can be investigated (Foscht and Swoboda, 2011).
Translational practices (of behavioral economics frameworks) have been mentioned to be useful to evaluate the dysregulation of reward-oriented behavior (Kobayashi et al., 2019). For example, patients with orbitofrontal cortex (OFC) damage have been reported to make poor decisions about day-to-day activities and engage in risk-taking behaviors (Bechara et al., 1994; Shiv et al., 2005; Floden et al., 2008), which may have negative consequences for their wellbeing and overall mental health (Rahman et al., 2001). Therefore, carrying out decision-making paradigms may help licensed therapists to validate the therapy directed toward patients with impulsive-compulsive disorders, such as for example pathological gamblers (frequently reported among individuals with Parkinson’s disease, frontotemporal dementia, and Huntington’s disease) and compulsive buyers (characterized with impulse control disorder, which co-occurs with depression) (De Marchi et al., 1998; Javor et al., 2013; Kalkhoven et al., 2014). In essence, neuromarketing studies, conducted in accordance with the Ethical Code of Conduct issued by the Neuromarketing Science and Business Association, would be of significant benefit to the general social progress (Pop et al., 2014).
Eye-Trackers Offset Traditional Self-Reporting Assessments
We also need to address that in clinical research, mental disorders are mainly diagnosed through observations made by highly specialized psychiatrists that are based on a patient’s overall behavior and self-reports. This means that for a patient to receive a diagnosis of a mental disorder, standardized criteria such as alterations in behavioral and cognitive development, laid out by the Diagnostic and Statistical Manual of Mental Disorders (DSM-V) (American Psychiatric Association, 2013), must be visible to the clinician. However, similarly as in marketing research, traditionally used subjective tools (e.g., rating scales that allow respondents to provide nuanced answers, questionnaires, and tests) are characterized with well-known limitations (Connors et al., 2016; Bell et al., 2018), lacking credibility due to self-report biases that are present when responding to sensitive questions related to addictive behaviors and criminal or sexual experiences (Tourangeau and Yan, 2007). By offsetting weaknesses associated with traditional self-reporting assessments that are usually designed for one disorder or limited in their examination of phenomenological features, eye-tracking generates considerable interest in terms of consumer research not as a complementary but as a standing-alone technology, revealing one’s moment-by-moment internal state (homeostasis) (Ernst and Paulus, 2005; Paulus, 2007; Wolf et al., 2021).
Not restricted to economic disciplines only, the eye-tracking methodology represents a category of interdisciplinary research instrumentation that has successfully intermingled with various research questions/tasks and exercised human eye-behavior with numerous stimuli categories (geometrical figures, computerized and real human faces, naturalistic food images, haptic and pictorial illusions as well as advertisement videos). These compelling arguments stand for eye-tracking technology being actively used in interdisciplinary laboratories, generating behavioral experimental paradigms that integrate contributions from psychology, philosophy, and affective and computer sciences (Borji et al., 2013; Spinks and Mortimer, 2016; Vu et al., 2016; Gidlöf et al., 2017; Wolf et al., 2018; Zommara et al., 2018; Vriens et al., 2020).
Future paradigms, which incorporate a decisional context (homeostatic regulation), may generate findings of significant importance to all behavioral sciences (including medical sciences such as neurology and psychiatry). Although the current trend in clinical research does not aim to replace the diagnosis criteria that rely on clinical observations and self-report, recent studies started to test the possibility of gaze metrics to distinguish patients suffering from mental disorders from healthy participants, hoping for a successful diagnosing tool, and those potentially at risk, aiming for an early intervention chance (Benson et al., 2012).
Bridging decision-making science with medical science may support clinical interventions and contribute to health services research agenda, to improve population health outcomes. Ideally, scientists develop cognitively informative paradigms, aiming to understand behavior among HC, which can be then applied to individuals suffering from mental illnesses, in order to identify biomarkers that point to one’s brain integrity (mechanism of information processing that requires a synchronized activity of lower and higher-order brain structures). Clinical paradigms, however, tend not to implement higher-order cognitive components related to decision-making paradigms, based on neurobiologically informed economic theories and mathematical choice psychology. Thus, a gap is being created where the translational approach of decision-making paradigms, that involves testing of the potential biomarkers in clinical trials, is scarcely reached. If not cued by cognitive scientists, eye-tracking technology may remain a theoretical recommendation for clinical practices (Wolf et al., 2021).
Discussion
To capture and investigate an interplay between cognition (and its deficits) and eye-movements, information-processing paradigms that reveal one’s gaze-patterns are needed. Following the global trend of the so-called digital pharma (or beyond the pill) strategy (Rutkowski et al., 2020) there is a need for technologies that support early diagnostics for cognitive interventions and monitor individual’s mental wellbeing. In our opinion, eye-movement measurements come in as a relatively low-cost measurable indicator (biomarker) of one’s homeostatic (mental) state. It has been reported that cognitive dysfunctions detected through gaze analysis may indicate or even predict mental disease processes (Fujioka et al., 2016; Almubark et al., 2020; Wolf et al., 2021). Moreover, studies of significant importance have produced preliminary findings that show that gaze-metrics parameters such as fixations (their location, number and duration), saccades (their number and amplitudes) and the scan-path length, are abnormal in a great number of neurological diseases (Beedie et al., 2011; Benson et al., 2012; Türkan et al., 2016; Li et al., 2020b; Morita et al., 2020).
However, cognitively informative paradigms are needed to draw further implications for medical experts and clarify aspects of visual impairments that manifest among clinical populations. Such paradigms may provide additional knowledge regarding attention, engagement and memory retention, all together combined as the foundation of an undertaken decision (Scholl and Klein-Flügge, 2018). When taking into consideration that approximately 95% of human decision-making processes happen at the subconscious level (Zaltman, 2003; Pop et al., 2014; Nyoni and Bonga, 2017), neuroscience opens the possibility of getting closer to the invisible part of neuronal connections, overcoming limitations related to self-reported methodologies (Connors et al., 2016; Bell et al., 2018).
Since numerous clinical works call for an etiology-based diagnosis (i.e., to understand the brain processes of individuals, who suffer from mental disorders), the development of reliable biomarkers (improving the diagnosis, identifying at-risk-individuals, and providing novel targets for therapeutic interventions), is not only in highly demand but it is the main purpose of modern clinical research (Kim et al., 2011; Daglas et al., 2015; Yahata et al., 2017; Wolf et al., 2021).
Eye-behavior tests that discriminate clinical cases from control subjects, have mounted in recent years. Shiino and colleagues reported significant differences between subjects with ASD and SZ in 5 selected eye-movement characteristics that were obtained from free-viewing and smooth pursuit tests (Shiino et al., 2020). Some other research groups modernized the clinical development process by integrating digital methods, based on machine learning (ML) approach (Benson et al., 2012; Tseng et al., 2013). For example, Tseng et al. (2013) extracted quantitative features from gaze data with the support of automated ML. Following this procedure, the authors were able to differentiate patients with attention deficit hyperactivity disorder (ADHD) and fetal alcohol spectrum disorder (FASD) with overlapping behavioral phenotypes from age-matched healthy participants (Tseng et al., 2013). Therefore, ML techniques extracting disorder-specific features for an automatic classification should be considered in prospective clinical applications (Huys et al., 2016; Yahata et al., 2017).
It is important to mention that eye-trackers can be utilized as a new intervention training tool for exposure therapies or attention redirection training. In the context of obsessive-compulsive disorder (OCD), Bradley and colleagues reported that eye-tracking, attentional control, and severity of attentional bias can indicate the therapeutic progress of implemented treatment plan, e.g., exposure response prevention — ERP (Bradley et al., 2016). Therefore, monitoring obsessions, compulsions, and attentional biases in a real-life context may determine therapy outcomes more accurately.
An implementation of real-life inspired paradigms related to general as well as social cognitive impairments will allow a better understanding of the homeostatic processing abnormalities among clinical populations (Ernst and Paulus, 2005; Paulus, 2007; Billeke and Aboitiz, 2013). At the same time, the support of eye-tracking technology will help to objectively illustrate and measure how and when information processing goes away from the expected course. For comprehensive and interdisciplinary models to be built in the future, experimental paradigms resembling real-life activities should be conducted, to have a clearer and disorder-specific picture of how converging as well as diverging tasks are processed inside patient’s mind and interpreted by her eyes.
Limitations
Since gaze metrics, gathered from visual information processing tasks, have recently started to support the development of non-invasive and relatively inexpensive biological markers in the clinical research domain, the presented article aimed to draw readers attention to one particular neuroscientific tool, namely the eye-tracking technology. Other neuroscientific methods were omitted due to the intentionally narrowed scope of this article.
Presented work is likely generalizable to journal articles and systematic reviews acquired through the Kyushu University Open Access Policy. Furthermore, to refrain from any unintended bias (caused by the authors’ research background), while addressing the aim of the perspective article, AW and KU have included a great number of articles, which relate to now-classic and modern clinical studies. The protocol was drafted using PRISMA guidelines, revised by the authors and lab members to solicit additional feedback. Articles published between 2010 and 2020, reporting cognitive impairments or abnormal eye-movements patterns among clinical populations, were identified by Mendeley and PubMed searches (search terms were: “eye-tracking”, “shopping task,” “cognitive deficits,” “clinical application,” and “cognitive impairments”). Reference lists of all in-scope articles have been additionally screened for relevant publications. Notably, to interlock the trend of decision-making paradigms, non-clinical open access articles were included in this work as well.
Finally, despite the absence of empirical data, best efforts have been undertaken to produce an objective, academic article that refrains from scientifically unsupported sensational claims concerning neuromarketing and its neuroscientific methods. It is hoped that the gathered examples will propel a greater interest and insightful research in consumer behavior and neuroeconomics, among individuals with mental disorders.
Data Availability Statement
The original contributions presented in the study are included in the article/supplementary material, further inquiries can be directed to the corresponding author.
Author Contributions
AW wrote the manuscript with a critical revision from KU. Both authors contributed to the article and approved the submitted version.
Funding
This work was funded by the KAKENHI grant ID JP19F19307 under the Postdoctoral Fellowship scholarship (AW) from the generous Japan Society for the Promotion of Science (JSPS).
Conflict of Interest
The authors declare that the research was conducted in the absence of any commercial or financial relationships that could be construed as a potential conflict of interest.
Acknowledgments
Both authors would like to thank Yoji Hirano (Kyushu University, Fukuoka, Japan) for the valuable, first-hand insights regarding clinical practices.
References
Almubark, I., Chang, L.-C., Shattuck, K. F., Nguyen, T., Turner, R. S., and Jiang, X. (2020). A 5-min Cognitive Task With Deep Learning Accurately Detects Early Alzheimer’s Disease. Front. Aging Neurosci. 12:603179. doi: 10.3389/fnagi.2020.603179
American Psychiatric Association (2013). Diagnostic and Statistical Manual of Mental Disorders. America: American Psychiatric Publishing, Inc.
Bachman, P., Reichenberg, A., Rice, P., Woolsey, M., Chaves, O., Martinez, D., et al. (2010). Deconstructing processing speed deficits in schizophrenia: application of a parametric digit symbol coding test. Schizophr. Res. 118, 6–11. doi: 10.1016/j.schres.2010.02.1029
Baldofski, S., Lüthold, P., Sperling, I., and Hilbert, A. (2018). Visual Attention to Pictorial Food Stimuli in Individuals With Night Eating Syndrome: an Eye-Tracking Study. Behav. Ther. 49, 262–272. doi: 10.1016/j.beth.2017.07.005
Baldwin, C. L., Spence, C., Bliss, J. P., Brill, J. C., Wogalter, M. S., Mayhorn, C. B., et al. (2012). “Multimodal cueing: the relative benefits of the auditory, visual, and tactile channels in complex environments,” in Proceedings of the Human Factors and Ergonomics Society, (United States: SAGE Publications). 1431–1435. doi: 10.1177/1071181312561404
Barnett, J. H., Lewis, L., Blackwell, A. D., and Taylor, M. (2014). Early intervention in Alzheimer’s disease: a health economic study of the effects of diagnostic timing. BMC Neurol. 14:101. doi: 10.1186/1471-2377-14-101
Baron-Cohen, S., Wheelwright, S., and Jolliffe, T. (1997). Is there a “language of the eyes”? Evidence from normal adults, and adults with autism or Asperger Syndrome. Vis. Cogn. 4, 311–331. doi: 10.1080/713756761
Bast, N., Mason, L., Freitag, C. M., Smith, T., Portugal, A. M., Poustka, L., et al. (2020). Saccade dysmetria indicates attenuated visual exploration in autism spectrum disorder. J. Child Psychol. doi: 10.1111/jcpp.13267
Beatty, J. (1982). Task-evoked pupillary responses, processing load, and the structure of processing resources. Psychol. Bull. 91, 276–292. doi: 10.1037//0033-2909.91.2.276
Bechara, A., Damasio, A. R., Damasio, H., and Anderson, S. W. (1994). Insensitivity to future consequences following damage to human prefrontal cortex. Cognition 50, 7–15. doi: 10.1016/0010-0277(94)90018-3
Beedie, S. A., St.Clair, D. M., and Benson, P. J. (2011). Atypical scanpaths in schizophrenia: evidence of a trait- or state-dependent phenomenon? J. Psychiatry Neurosci. 36, 150–164. doi: 10.1503/jpn.090169
Beilharz, F., Castle, D. J., Phillipou, A., and Rossell, S. L. (2018). Visual training program for body dysmorphic disorder: protocol for a novel intervention pilot and feasibility trial. Pilot Feasibility Stud. 4:189. doi: 10.1186/s40814-018-0384-3
Bell, L., Vogt, J., Willemse, C., Routledge, T., Butler, L. T., and Sakaki, M. (2018). Beyond self-report: a review of physiological and neuroscientific methods to investigate consumer behavior. Front. Psychol. 9:1655. doi: 10.3389/fpsyg.2018.01655
Benson, P. J., Beedie, S. A., Shephard, E., Giegling, I., Rujescu, D., and St. Clair, D. (2012). Simple viewing tests can detect eye movement abnormalities that distinguish schizophrenia cases from controls with exceptional accuracy. Biol. Psychiatry 72, 716-24. doi: 10.1016/j.biopsych.2012.04.019
Berčík, J., Horská, E., Wang, R. W. Y., and Chen, Y. C. (2016). The impact of parameters of store illumination on food shopper response. Appetite 106, 101–109. doi: 10.1016/j.appet.2016.04.010
Bestelmeyer, P. E. G., Tatler, B. W., Phillips, L. H., Fraser, G., Benson, P. J., and St.Clair, D. (2006). Global visual scanning abnormalities in schizophrenia and bipolar disorder. Schizophr. Res. 87, 212–222. doi: 10.1016/j.schres.2006.06.015
Billeke, P., and Aboitiz, F. (2013). Social cognition in schizophrenia: from social stimuli processing to social engagement. Front. Psychiatry 4:4. doi: 10.3389/fpsyt.2013.00004
Bird, G. D., Lauwereyns, J., and Crawford, M. T. (2012). The role of eye movements in decision making and the prospect of exposure effects. Vision Res. 60, 16–21. doi: 10.1016/j.visres.2012.02.014
Bogacz, R. (2007). Optimal decision-making theories: linking neurobiology with behaviour. Trends Cogn. Sci. 11, 118–125. doi: 10.1016/j.tics.2006.12.006
Bogacz, R., Brown, E., Moehlis, J., Holmes, P., and Cohen, J. D. (2006). The physics of optimal decision making: a formal analysis of models of performance in two-alternative forced-choice tasks. Psychol. Rev. 113, 700–765. doi: 10.1037/0033-295X.113.4.700
Boisvert, J. F. G., and Bruce, N. D. B. (2016). Predicting task from eye movements: on the importance of spatial distribution, dynamics, and image features. Neurocomputing 207, 653–668. doi: 10.1016/j.neucom.2016.05.047
Boll, S., Bartholomaeus, M., Peter, U., Lupke, U., and Gamer, M. (2016). Attentional mechanisms of social perception are biased in social phobia. J. Anxiety Disord. 40, 83–93. doi: 10.1016/j.janxdis.2016.04.004
Borghini, G., Astolfi, L., Vecchiato, G., Mattia, D., and Babiloni, F. (2014). Measuring neurophysiological signals in aircraft pilots and car drivers for the assessment of mental workload, fatigue and drowsiness. Neurosci. Biobehav. Rev. 44, 58–75. doi: 10.1016/j.neubiorev.2012.10.003
Borji, A., and Itti, L. (2014). Defending yarbus: eye movements reveal observers’ task. J. Vis. 14:29. doi: 10.1167/14.3.29
Borji, A., Sihite, D. N., and Itti, L. (2013). Quantitative analysis of human-model agreement in visual saliency modeling: a comparative study. IEEE Trans. Image Process. 22, 55–69. doi: 10.1109/TIP.2012.2210727
Bradley, M. C., Hanna, D., Wilson, P., Scott, G., Quinn, P., and Dyer, K. F. W. (2016). Obsessive-compulsive symptoms and attentional bias: an eye-tracking methodology. J. Behav. Ther. Exp. Psychiatry 50, 303–308. doi: 10.1016/j.jbtep.2015.10.007
Braff, D. L. (1993). Information processing and attention dysfunctions in schizophrenia. Schizophr. Bull. 19, 233-59. doi: 10.1093/schbul/19.2.233
Brand, M., Fujiwara, E., Borsutzky, S., Kalbe, E., Kessler, J., and Markowitsch, H. J. (2005). Decision-making deficits of Korsakoff patients in a new gambling task with explicit rules: associations with executive functions. Neuropsychology 19, 267–277. doi: 10.1037/0894-4105.19.3.267
Brefczynski-Lewis, J. A., Berrebi, M. E., McNeely, M. E., Prostko, A. L., and Puce, A. (2011). In the Blink of an Eye: neural Responses Elicited to Viewing the Eye Blinks of Another Individual. Front. Hum. Neurosci. 5:68. doi: 10.3389/fnhum.2011.00068
Burkhouse, K. L., Siegle, G. J., Woody, M. L., Kudinova, A. Y., and Gibb, B. E. (2015). Pupillary reactivity to sad stimuli as a biomarker of depression risk: evidence from a prospective study of children. J. Abnorm. Psychol. 124, 498–506. doi: 10.1037/abn0000072
Buswell, G. T. (1935). How People Look at Pictures. UK: Informa UK Limited. doi: 10.1080/00043079.1936.11408852
Carl, E., Liskiewicz, A., Rivard, C., Alberico, R., Belal, A., Mahoney, M. C., et al. (2020). Dosing parameters for the effects of high-frequency transcranial magnetic stimulation on smoking cessation: study protocol for a randomized factorial sham-controlled clinical trial. BMC Psychol. 8:42. doi: 10.1186/s40359-020-00403-7
Caseras, X., Garner, M., Bradley, B. P., and Mogg, K. (2007). Biases in Visual Orienting to Negative and Positive Scenes in Dysphoria: an Eye Movement Study. J. Abnorm. Psychol. 116, 491–497. doi: 10.1037/0021-843X.116.3.491
Castelhano, M. S., Mack, M. L., and Henderson, J. M. (2009). Viewing task influences eye movement control during active scene perception. J. Vis. 9, 1–15. doi: 10.1167/9.3.6
Chawarska, K., Macari, S., and Shic, F. (2013). Decreased Spontaneous Attention to Social Scenes in 6-Month-Old Infants Later Diagnosed with Autism Spectrum Disorders. Biol. Psychiatry 74, 195–203. doi: 10.1016/j.biopsych.2012.11.022
Chermahini, S. A., and Hommel, B. (2010). The (b)link between creativity and dopamine: spontaneous eye blink rates predict and dissociate divergent and convergent thinking. Cognition 115, 458–465. doi: 10.1016/j.cognition.2010.03.007
Cherubino, P., Martinez-Levy, A. C., Caratù, M., Caratù, C., Cartocci, G., Flumeri, G., et al. (2019). Consumer Behaviour through the Eyes of Neurophysiological Measures: state-of-the-Art and Future Trends. Comput Intell Neurosci. 2019:1976847. doi: 10.1155/2019/1976847
Chevalier, N., Martis, S. B., Curran, T., and Munakata, Y. (2015). Metacognitive processes in executive control development: the case of reactive and proactive control. J. Cogn. Neurosci. 27, 1125–1136. doi: 10.1162/jocn_a_00782
Chung, J., Eizenman, M., Rakita, U., Mcintyre, R., and Giacobbe, P. (2018). Learning Differences between Visual Scanning Patterns Can Disambiguate Bipolar and Unipolar Patients. Proc. AAAI Conf. Artif. Intell. 32, 1.
Chynal, P., Sobecki, J., Rymarz, M., and Kilijanska, B. (2016). “Shopping behaviour analysis using eyetracking and EEG,” in Proceedings 2016 9th International Conference on Human System Interactions, HSI 2016, (United States: Institute of Electrical and Electronics Engineers Inc), doi: 10.1109/HSI.2016.7529674
Cocker, P. J., Hosking, J. G., Benoit, J., and Winstanley, C. A. (2012). Sensitivity to cognitive effort mediates psychostimulant effects on a novel rodent cost/benefit decision-making task. Neuropsychopharmacology 37, 1825–1837. doi: 10.1038/npp.2012.30
Connors, B. L., Rende, R., and Colton, T. J. (2016). Beyond self-report: emerging methods for capturing individual differences in decision-making process. Front. Psychol. 7:1–5. doi: 10.3389/fpsyg.2016.00312
Corden, B., Chilvers, R., and Skuse, D. (2008). Avoidance of emotionally arousing stimuli predicts social-perceptual impairment in Asperger’s syndrome. Neuropsychologia 46, 137–147. doi: 10.1016/j.neuropsychologia.2007.08.005
Crawford, T. J., Smith, E. S., and Berry, D. M. (2017). Eye gaze and aging: selective and combined effects of working memory and inhibitory control. Front. Hum. Neurosci. 11:563. doi: 10.3389/fnhum.2017.00563
Daglas, R., Yücel, M., Cotton, S., Allott, K., Hetrick, S., and Berk, M. (2015). Cognitive impairment in first-episode mania: a systematic review of the evidence in the acute and remission phases of the illness. Int. J. Bipolar Disord. 3:9. doi: 10.1186/s40345-015-0024-2
De Marchi, N., Morris, M., Mennella, R., La Pia, S., and Nestadt, G. (1998). Association of obsessive-compulsive disorder and pathological gambling with Huntington’s disease in an Italian pedigree: possible association with Huntington’s disease mutation. Acta Psychiatr. Scand. 97, 62–65. doi: 10.1111/j.1600-0447.1998.tb09964.x
DeAngelus, M., and Pelz, J. B. (2009). Top-down control of eye movements: yarbus revisited. Vis. Cogn. 17, 790–811. doi: 10.1080/13506280902793843
Deubel, H., and Schneider, W. X. (1996). Saccade target selection and object recognition: evidence for a common attentional mechanism. Vision Res. 36, 1827–1837. doi: 10.1016/0042-6989(95)00294-4
Dewhurst, R., Nyström, M., Jarodzka, H., Foulsham, T., Johansson, R., and Holmqvist, K. (2012). It depends on how you look at it: scanpath comparison in multiple dimensions with MultiMatch, a vector-based approach. Behav. Res. Methods 44, 1079–1100. doi: 10.3758/s13428-012-0212-2
DeYoung, C. G. (2013). The neuromodulator of exploration: a unifying theory of the role of dopamine in personality. Front. Hum. Neurosci. 7:762. doi: 10.3389/fnhum.2013.00762
Diwakar, M., Harrington, D. L., Maruta, J., Ghajar, J., El-Gabalawy, F., Muzzatti, L., et al. (2015). Filling in the gaps: anticipatory control of eye movements in chronic mild traumatic brain injury. NeuroImage Clin. 8, 210–223. doi: 10.1016/j.nicl.2015.04.011
Dowiasch, S., Backasch, B., Einhäuser, W., Leube, D., Kircher, T., and Bremmer, F. (2016). Eye movements of patients with schizophrenia in a natural environment. Eur. Arch. Psychiatry Clin. Neurosci. 266, 43–54. doi: 10.1007/s00406-014-0567-8
Eckstein, M. K., Guerra-Carrillo, B., Miller Singley, A. T., and Bunge, S. A. (2017). Beyond eye gaze: what else can eyetracking reveal about cognition and cognitive development? Dev. Cogn. Neurosci. 25, 69–91. doi: 10.1016/j.dcn.2016.11.001
Ernst, M., and Paulus, M. P. (2005). Neurobiology of decision making: a selective review from a neurocognitive and clinical perspective. Biol. Psychiatry 58, 597–604. doi: 10.1016/j.biopsych.2005.06.004
Floden, D., Alexander, M. P., Kubu, C. S., Katz, D., and Stuss, D. T. (2008). Impulsivity and risk-taking behavior in focal frontal lobe lesions. Neuropsychologia 46, 213–223. doi: 10.1016/j.neuropsychologia.2007.07.020
Foscht, T., and Swoboda, B. (2011). Käuferverhalten Grundlagen - Perspektiven - Anwendungen, 4th Edn. Germany: Springer Gabler.
Foulsham, T., and Kingstone, A. (2011). Look at my poster! Active gaze, preference and memory during a poster session. Perception 40, 1387–1389. doi: 10.1068/p7015
Foulsham, T., and Kingstone, A. (2013). Where have eye been? Observers can recognise their own fixations. Perception 42, 1085–1089. doi: 10.1068/p7562
Frazier, T., Klingemier, E., and Beukemann, M. (2016). Development of an objective autism risk index using remote eye tracking. J. Am. Acad. Child Adolesc. Psychiatry 55, 301–309.
Fujioka, T., Inohara, K., Okamoto, Y., Masuya, Y., Ishitobi, M., Saito, D. N., et al. (2016). Gazefinder as a clinical supplementary tool for discriminating between autism spectrum disorder and typical development in male adolescents and adults. Mol. Autism. 7:19. doi: 10.1186/s13229-016-0083-y
Gao, X., Wang, Q.-C., Chen, H., Wang, B.-Y., and Zhao, G. (2012). Time Course of Attentional Bias Components Toward Body-shape Related Pictures Among Women with Fat Negative Physical Self: an Eye Movement Study. Acta Psychol. Sin. 44, 498–519. doi: 10.3724/sp.j.1041.2012.00498
Gerbella, M., Rozzi, S., and Rizzolatti, G. (2017). The extended object-grasping network. Exp. Brain Res. 235, 2903–2916. doi: 10.1007/s00221-017-5007-3
Gergelyfi, M., Jacob, B., Olivier, E., and Zénon, A. (2015). Dissociation between mental fatigue and motivational state during prolonged mental activity. Front. Behav. Neurosci. 9:176. doi: 10.3389/fnbeh.2015.00176
Gidlöf, K., Anikin, A., Lingonblad, M., and Wallin, A. (2017). Looking is buying. How visual attention and choice are affected by consumer preferences and properties of the supermarket shelf. Appetite 116, 29–38. doi: 10.1016/j.appet.2017.04.020
Gidlöf, K., Wallin, A., Dewhurst, R., and Holmqvist, K. (2013). Using eye tracking to trace a cognitive process: gaze behaviour during decision making in a natural environment. J. Eye Mov. Res. 6:1. doi: 10.16910/jemr.6.1.3
Giel, K. E., Teufel, M., Friederich, H. C., Hautzinger, M., Enck, P., and Zipfel, S. (2011). Processing of pictorial food stimuli in patients with eating disorders-A systematic review. Int. J. Eat. Disord. 44, 105–117. doi: 10.1002/eat.20785
Glaholt, M. G., and Reingold, E. M. (2009). Stimulus exposure and gaze bias: a further test of the gaze cascade model. Atten. Percept. Psychophys 71, 445–450. doi: 10.3758/APP.71.3.445
Glaholt, M. G., and Reingold, E. M. (2011). Eye Movement Monitoring as a Process Tracing Methodology in Decision Making Research. J. Neurosci. Psychol. Econ. 4, 125–146. doi: 10.1037/a0020692
Gotlieb, R. J. M., Yang, X.-F., and Immordino-Yang, M. H. (2021). Measuring Learning in the Blink of an Eye: adolescents’ Neurophysiological Reactions Predict Long-Term Memory for Stories. Front. Educ. 5:594668. doi: 10.3389/feduc.2020.594668
Graham, W. V., Bonito-Oliva, A., and Sakmar, T. P. (2017). Update on Alzheimer’s Disease Therapy and Prevention Strategies. Annu. Rev. Med. 68, 413–430. doi: 10.1146/annurev-med-042915-103753
Greenberg, J. L., Reuman, L., Hartmann, A. S., Kasarskis, I., and Wilhelm, S. (2014). Visual hot spots: an eye tracking study of attention bias in body dysmorphic disorder. J. Psychiatr. Res. 57, 125–132. doi: 10.1016/j.jpsychires.2014.06.015
Greene, M. R., Liu, T., and Wolfe, J. M. (2012). Reconsidering Yarbus: a failure to predict observers’ task from eye movement patterns. Vision Res. 62, 1–8. doi: 10.1016/j.visres.2012.03.019
Grocholewski, A., Kliem, S., and Heinrichs, N. (2012). Selective attention to imagined facial ugliness is specific to body dysmorphic disorder. Body Image 9, 261–269. doi: 10.1016/j.bodyim.2012.01.002
Groman, S. M., James, A. S., Seu, E., Tran, S., Clark, T. A., Harpster, S. N., et al. (2014). In the blink of an eye: relating positive-feedback sensitivity to striatal dopamine d2-like receptors through blink rate. J. Neurosci. 34, 14443–14454. doi: 10.1523/JNEUROSCI.3037-14.2014
Halliwell, E. (2015). Future directions for positive body image research. Body Image 14, 177–189. doi: 10.1016/j.bodyim.2015.03.003
Hamera, E., and Brown, C. E. (2000). Developing a context-based performance measure for persons with schizophrenia: the test of grocery shopping skills. Am. J. Occup. Ther. 54, 20–25. doi: 10.5014/ajot.54.1.20
Hamera, E. K., Brown, C., Rempfer, M., and Davis, N. C. (2002). Test of Grocery Shopping Skills: discrimination of People with and without Mental Illness. Psychiatr. Rehabil. Ski. 6, 296–311. doi: 10.1080/10973430208408440
Hayhoe, M., and Ballard, D. (2005). Eye movements in natural behavior. Trends Cogn. Sci. 9, 188–194. doi: 10.1016/j.tics.2005.02.009
Helo, A., Pannasch, S., Sirri, L., and Rämä, P. (2014). The maturation of eye movement behavior: scene viewing characteristics in children and adults. Vision Res. 103, 83–91. doi: 10.1016/j.visres.2014.08.006
Hirokawa, K., Yagi, A., and Miyata, Y. (2004). Comparison of blinking behavior during listening to and speaking in Japanese and English. Percept. Mot. Skills 98, 463–472. doi: 10.2466/pms.98.2.463-472
Holzman, P. S. (1985). Eye Movement Dysfunctions and Psychosis. Int. Rev. Neurobiol. 27, 179–205. doi: 10.1016/S0074-7742(08)60558-9
Holzman, P. S., Kringlen, E., Levy, D. L., and Haberman, S. J. (1980). Deviant Eye Tracking in Twins Discordant for Psychosis: a Replication. Arch. Gen. Psychiatry 37, 627–631. doi: 10.1001/archpsyc.1980.01780190025002
Holzman, P. S., Proctor, L. R., and Hughes, D. W. (1973). Eye-Tracking Patterns in Schizophrenia. Science 181, 179–81.
Holzman, P. S., Proctor, L. R., Levy, D. L., Yasillo, N. J., Meltzer, H. Y., and Hurt, S. W. (1974). Eye-Tracking Dysfunctions in Schizophrenic Patients and Their Relatives. Arch. Gen. Psychiatry 31, 143–151. doi: 10.1001/archpsyc.1974.01760140005001
Horley, K., Williams, L. M., Gonsalvez, C., and Gordon, E. (2004). Face to face: visual scanpath evidence for abnormal processing of facial expressions in social phobia. Psychiatry Res. 127, 43–53.
Horley, K., Williams, L. M., Gonsalvez, C., and Gordon, E. (2003). Social phobics do not see eye to eye: a visual scanpath study of emotional expression processing. J. Anxiety Disord. 17, 33–44. doi: 10.1016/S0887-6185(02)00180-9
Hubert, M., and Kenning, P. (2008). A current overview of consumer neuroscience. J. Consum. Behav. 7, 272–292. doi: 10.1002/cb.251
Hunter, L., Roland, L., and Ferozpuri, A. (2020). Emotional Expression Processing and Depressive Symptomatology: eye-Tracking Reveals Differential Importance of Lower and Middle Facial Areas of Interest. Depress. Res. Treat. 2020:1049851. doi: 10.1155/2020/1049851
Huys, Q. J. M., Maia, T. V., and Frank, M. J. (2016). Computational psychiatry as a bridge from neuroscience to clinical applications. Nat. Neurosci. 19, 404–413. doi: 10.1038/nn.4238
Jansen, A., Nederkoorn, C., and Mulkens, S. (2005). Selective visual attention for ugly and beautiful body parts in eating disorders. Behav. Res. Ther. 43, 183–196. doi: 10.1016/j.brat.2004.01.003
Jarodzka, H., Scheiter, K., Gerjets, P., and van Gog, T. (2010). In the eyes of the beholder: how experts and novices interpret dynamic stimuli. Learn. Instr. 20, 146–154. doi: 10.1016/j.learninstruc.2009.02.019
Javor, A., Koller, M., Lee, N., Chamberlain, L., and Ransmayr, G. (2013). Neuromarketing and consumer neuroscience: contributions to neurology. BMC Neurol. 13:13. doi: 10.1186/1471-2377-13-13
Jones, L., Metcalf, A., Gordon-Smith, K., Forty, L., Perry, A., Lloyd, J., et al. (2015). Gambling problems in bipolar disorder in the UK: prevalence and distribution. Br. J. Psychiatry 207, 328–333. doi: 10.1192/bjp.bp.114.154286
Jongkees, B. J., and Colzato, L. S. (2016). Spontaneous eye blink rate as predictor of dopamine-related cognitive function—A review. Neurosci. Biobehav. Rev. 71, 58–82. doi: 10.1016/j.neubiorev.2016.08.020
Kalkhoven, C., Sennef, C., Peeters, A., and van den Bos, R. (2014). Risk-taking and pathological gambling behavior in Huntington’s disease. Front. Behav. Neurosci. 8:103. doi: 10.3389/fnbeh.2014.00103
Kerr-Gaffney, J., Harrison, A., and Tchanturia, K. (2019). Eye-tracking research in eating disorders: a systematic review. Int. J. Eat. Disord. 52, 3–27. doi: 10.1002/eat.22998
Kim, J., Park, S., and Blake, R. (2011). Perception of Biological Motion in Schizophrenia and Healthy Individuals: a Behavioral and Fmri Study. PLoS One 6:e19971. doi: 10.1371/journal.pone.0019971
Klin, A., Jones, W., Schultz, R., Volkmar, F., and Cohen, D. (2002). Defining and quantifying the social phenotype in autism. Am. J. Psychiatry 159, 895–908. doi: 10.1176/appi.ajp.159.6.895
Kobayashi, S., Asano, K., Matsuda, N., and Ugawa, Y. (2019). Dopaminergic influences on risk preferences of Parkinson’s disease patients. Cogn. Affect. Behav. Neurosci. 19, 88–97. doi: 10.3758/s13415-018-00646-3
Kogata, T., and Iidaka, T. (2018). A review of impaired visual processing and the daily visual world in patients with schizophrenia. Nagoya J. Med. Sci. 80, 317–328. doi: 10.18999/nagjms.80.3.317
Kojima, T., Matsushima, E., Ando, K., Ando, H., Sakurada, M., Ohta, K., et al. (1992). Exploratory eye movements and neuropsychological tests in schizophrenic patients. Schizophr. Bull. 18, 85–94. doi: 10.1093/schbul/18.1.85
Kollei, I., Horndasch, S., Erim, Y., and Martin, A. (2017). Visual selective attention in body dysmorphic disorder, bulimia nervosa and healthy controls. J. Psychosom. Res. 92, 26–33. doi: 10.1016/j.jpsychores.2016.11.008
Kou, H., Su, Y., Bi, T., Gao, X., and Chen, H. (2016). Attentional Biases toward Face-Related Stimuli among Face Dissatisfied Women: orienting and Maintenance of Attention Revealed by Eye-Movement. Front. Psychol. 7:919. doi: 10.3389/fpsyg.2016.00919
Koychev, I., El-Deredy, W., and William Deakin, J. F. (2011). New visual information processing abnormality biomarkers for the diagnosis of schizophrenia. Expert Opin. Med. Diagn. 5, 357–368. doi: 10.1517/17530059.2011.586029
Laeng, B., Sirois, S., and Gredebäck, G. (2012). Pupillometry: a window to the preconscious? Perspect. Psychol. Sci. 7, 18–27. doi: 10.1177/1745691611427305
Lai, M. L., Tsai, M. J., Yang, F. Y., Hsu, C. Y., Liu, T. C., Lee, S. W. Y., et al. (2013). A review of using eye-tracking technology in exploring learning from 2000 to 2012. Educ. Res. Rev. 10, 90–115. doi: 10.1016/j.edurev.2013.10.001
Lakhlifi, M., Laprevote, V., Schwan, R., and Schwitzer, T. (2020). Free viewing exploration in schizophrenia: review of evidence from laboratory settings to natural environment. Encephale 46, 115–122. doi: 10.1016/j.encep.2019.11.012
Laloyaux, J., Michel, C., Mourad, H., Bertrand, H., Domken, M. A., Van der, L. M., et al. (2012). Performance on an everyday life activity in persons diagnosed with alcohol dependency compared to healthy controls: relations between a computerized shopping task and cognitive and clinical variables. Alcohol Alcohol. 47, 240–247. doi: 10.1093/alcalc/ags014
Laloyaux, J., Pellegrini, N., Mourad, H., Bertrand, H., Domken, M. A., Van der Linden, M., et al. (2013). Performance on a computerized shopping task significantly predicts real world functioning in persons diagnosed with bipolar disorder. Psychiatry Res. 210, 465–471. doi: 10.1016/j.psychres.2013.06.032
Levy, I., Lazzaro, S. C., Rutledge, R. B., and Glimcher, P. W. (2011). Choice from non-choice: predicting consumer preferences from blood oxygenation level-dependent signals obtained during passive viewing. J. Neurosci. 31, 118–125. doi: 10.1523/JNEUROSCI.3214-10.2011
Li, X., Bin, Jiang, W. L., Wen, Y. J., Wang, C. M., Tian, Q., et al. (2020a). The attenuated visual scanpaths of patients with schizophrenia whilst recognizing emotional facial expressions are worsened in natural social scenes. Schizophr. Res. 220, 155-163. doi: 10.1016/j.schres.2020.03.040
Li, J., Zhong, Y., Han, J., Ouyang, G., Li, X., and Liu, H. (2020b). Classifying ASD children with LSTM based on raw videos. Neurocomputing 390, 226–238. doi: 10.1016/j.neucom.2019.05.106
Li, Y., Xu, Y., Xia, M., Zhang, T., Wang, J., Liu, X., et al. (2016). Eye Movement Indices in the Study of Depressive Disorder. Shanghai Arch. Psychiatry 28, 326–334. doi: 10.11919/j.issn.1002-0829.216078
Lim, W. M. (2018). Demystifying neuromarketing. J. Bus. Res. 91, 205–220. doi: 10.1016/j.jbusres.2018.05.036
Lin, Y. H. T., Hamid, N., Shepherd, D., Kantono, K., and Spence, C. (2019). Environmental sounds influence the multisensory perception of chocolate gelati. Foods 8:124. doi: 10.3390/foods8040124
Mackworth, N. H., and Bruner, J. S. (1970). How adults and children search and recognize pictures. Hum. Dev. 13, 149–177. doi: 10.1159/000270887
Manee, F. S. (1997). Cognitve Impairments And Grocery Shopping Performance In Individuals With TBI. Unite States: University of Hartford.
Markkula, G. (2015). Answering questions about consciousness by modeling perception as covert behavior. Front. Psychol. 6:803. doi: 10.3389/fpsyg.2015.00803
Martinez-Conde, S., and Macknik, S. L. (2015). From Exploration to Fixation: an Integrative View of Yarbus’s Vision. Perception 44, 884–899. doi: 10.1177/0301006615594963
Maruta, J., Heaton, K. J., Maule, A. L., and Ghajar, J. (2014). Predictive visual tracking: specificity in mild traumatic brain injury and sleep deprivation. Mil. Med. 179, 619–625. doi: 10.7205/MILMED-D-13-00420
McGrath, D. S., Meitner, A., and Sears, C. R. (2018). The specificity of attentional biases by type of gambling: an eye-tracking study. PLoS One 13:e0190614. doi: 10.1371/journal.pone.0190614
Morii, M., and Sakagami, T. (2015). The effect of gaze-contingent stimulus elimination on preference judgments. Front. Psychol. 6:1351. doi: 10.3389/fpsyg.2015.01351
Morita, K., Miura, K., Fujimoto, M., Yamamori, H., Yasuda, Y., Iwase, M., et al. (2017). Eye movement as a biomarker of schizophrenia: using an integrated eye movement score. Psychiatry Clin. Neurosci. 71, 104–114. doi: 10.1111/pcn.12460
Morita, K., Miura, K., Kasai, K., and Hashimoto, R. (2020). Eye movement characteristics in schizophrenia: a recent update with clinical implications. Neuropsychopharmacol. Reports 40, 2–9. doi: 10.1002/npr2.12087
Mottron, L., Dawson, M., Souličres, I., Hubert, B., and Burack, J. (2006). Enhanced perceptual functioning in autism: an update, and eight principles of autistic perception. J. Autism Dev. Disord. 36, 27–43. doi: 10.1007/s10803-005-0040-7
Müller, J., Dreisbach, G., Brocke, B., Lesch, K. P., Strobel, A., and Goschke, T. (2007). Dopamine and cognitive control: the influence of spontaneous eyeblink rate, DRD4 exon III polymorphism and gender on flexibility in set-shifting. Brain Res. 1131, 155–162. doi: 10.1016/j.brainres.2006.11.002
Najemnik, J., and Geisler, W. S. (2005). Optimal eye movement strategies in visual search. Nature 434, 387–391. doi: 10.1038/nature03390
Najemnik, J., and Geisler, W. S. (2009). Simple summation rule for optimal fixation selection in visual search. Vision Res. 49, 1286–1294. doi: 10.1016/j.visres.2008.12.005
Nemorin, S., and Gandy, O. H. (2017). Exploring neuromarketing and its reliance on remote sensing: social and ethical concerns. Int. J. Commun. 11, 4824–4844.
Noton, D., and Stark, L. (1971). Scanpaths in saccadic eye movements while viewing and recognizing patterns. Vision Res. 11, 929-42. doi: 10.1016/0042-6989(71)90213-6
Obyedkov, I., Skuhareuskaya, M., Skugarevsky, O., Obyedkov, V., Buslauski, P., Skuhareuskaya, T., et al. (2019). Saccadic eye movements in different dimensions of schizophrenia and in clinical high-risk state for psychosis. BMC Psychiatry 19:110. doi: 10.1186/s12888-019-2093-8
Oliveira, D., Machín, L., Deliza, R., Rosenthal, A., Walter, E. H., Giménez, A., et al. (2016). Consumers’ attention to functional food labels: insights from eye-tracking and change detection in a case study with probiotic milk. LWT - Food Sci. Technol. 68, 160-167. doi: 10.1016/j.lwt.2015.11.066
Onuma, T., Penwannakul, Y., Fuchimoto, J., and Sakai, N. (2017). The effect of order of dwells on the first dwell gaze bias for eventually chosen items. PLoS One 12:e0181641. doi: 10.1371/journal.pone.0181641
Oyama, A., Takeda, S., Ito, Y., Nakajima, T., Takami, Y., Takeya, Y., et al. (2019). Novel Method for Rapid Assessment of Cognitive Impairment Using High-Performance Eye-Tracking Technology. Sci. Rep. 9:12932. doi: 10.1038/s41598-019-49275-x
Paprocki, R., and Lenskiy, A. (2017). What does eye-blink rate variability dynamics tell us about cognitive performance? Front. Hum. Neurosci. 11:620. doi: 10.3389/fnhum.2017.00620
Pärnamets, P., Johansson, P., Hall, L., Balkenius, C., Spivey, M. J., and Richardson, D. C. (2015). Biasing moral decisions by exploiting the dynamics of eye gaze. Proc. Natl. Acad. Sci. U. S. A. 112, 4170–4175. doi: 10.1073/pnas.1415250112
Pärnamets, P., Johansson, R., Gidlöf, K., and Wallin, A. (2016). How Information Availability Interacts with Visual Attention during Judgment and Decision Tasks. J. Behav. Decis. Mak 29, 218–231. doi: 10.1002/bdm.1902
Paulus, M. P. (2007). Decision-making dysfunctions in psychiatry - Altered homeostatic processing? Science 318, 602–606. doi: 10.1126/science.1142997
Pierce, K., Marinero, S., Hazin, R., McKenna, B., Barnes, C. C., and Malige, A. (2016). Eye tracking reveals abnormal visual preference for geometric images as an early biomarker of an autism spectrum disorder subtype associated with increased symptom severity. Biol. Psychiatry 79, 657–666. doi: 10.1016/j.biopsych.2015.03.032
Pop, N., Al, Dabija, D. C., and Iorga, A. M. (2014). Ethical responsibility of neuromarketing companies in harnessing the market research - A global exploratory approach. Amfiteatru Econ. 16, 26–40.
Rahman, S., Sahakian, B. J., Cardinal, R. N., Rogers, R. D., and Robbins, T. W. (2001). Decision making and neuropsychiatry. Trends Cogn. Sci. 5, 271–277. doi: 10.1016/S1364-6613(00)01650-8
Rempfer, M. V., Hamera, E. K., Brown, C. E., and Cromwell, R. L. (2003). The relations between cognition and the independent living skill of shopping in people with schizophrenia. Psychiatry Res. 117, 103–112. doi: 10.1016/S0165-1781(02)00318-9
Riby, D. M., and Hancock, P. J. B. (2008). Viewing it differently: social scene perception in Williams syndrome and Autism. Neuropsychologia 46, 2855–2860. doi: 10.1016/j.neuropsychologia.2008.05.003
Risko, E. F., Anderson, N. C., Lanthier, S., and Kingstone, A. (2012). Curious eyes: individual differences in personality predict eye movement behavior in scene-viewing. Cognition 122, 86–90. doi: 10.1016/j.cognition.2011.08.014
Roberts, H., Soto, V., Tyson-Carr, J., Kokmotou, K., Cook, S., Fallon, N., et al. (2018). Tracking Economic Value of Products in Natural Settings: a Wireless EEG Study. Front. Neurosci. 12:910. doi: 10.3389/fnins.2018.00910
Rommelse, N. N. J., Van der Stigchel, S., and Sergeant, J. A. (2008). A review on eye movement studies in childhood and adolescent psychiatry. Brain Cogn. 68, 391–414. doi: 10.1016/j.bandc.2008.08.025
Roux, P., Brunet-Gouet, E., Passerieux, C., and Ramus, F. (2016). Eye-tracking reveals a slowdown of social context processing during intention attribution in patients with schizophrenia. J. Psychiatry Neurosci. 41, E13-21. doi: 10.1503/jpn.150045
Rutkowski, T. M., Abe, M. S., Koculak, M., and Otake-Matsuura, M. (2020). “Classifying Mild Cognitive Impairment from Behavioral Responses in Emotional Arousal and Valence Evaluation Task - AI Approach for Early Dementia Biomarker in Aging Societies - AI A,” in Proceedings of the Annual International Conference of the IEEE Engineering in Medicine and Biology Society, EMBS. (United States: Institute of Electrical and Electronics Engineers Inc). doi: 10.1109/EMBC44109.2020.9175805
Sablier, J., Stip, E., and Franck, N. (2009). [Cognitive remediation and cognitive assistive technologies in schizophrenia]. Encephale. 35, 160–167. doi: 10.1016/j.encep.2008.02.010
Saito, T., Nouchi, R., Kinjo, H., and Kawashima, R. (2017). Gaze Bias in Preference Judgments by Younger and Older Adults. Front. Aging Neurosci. 9:285. doi: 10.3389/fnagi.2017.00285
Sawada, K., Kanehara, A., Sakakibara, E., Eguchi, S., Tada, M., Satomura, Y., et al. (2017). Identifying neurocognitive markers for outcome prediction of global functioning in individuals with first-episode and ultra-high-risk for psychosis. Psychiatry Clin. Neurosci. 71, 318–327. doi: 10.1111/pcn.12522
Schag, K., Teufel, M., Junne, F., Preissl, H., Hautzinger, M., Zipfel, S., et al. (2013). Impulsivity in Binge Eating Disorder: food Cues Elicit Increased Reward Responses and Disinhibition. PLoS One 8:e76542. doi: 10.1371/journal.pone.0076542
Schmidt, R., Lüthold, P., Kittel, R., Tetzlaff, A., and Hilbert, A. (2016). Visual attentional bias for food in adolescents with binge-eating disorder. J. Psychiatr. Res. 80, 22–29. doi: 10.1016/j.jpsychires.2016.05.016
Scholl, J., and Klein-Flügge, M. (2018). Understanding psychiatric disorder by capturing ecologically relevant features of learning and decision-making. Behav. Brain Res. 355, 56–75. doi: 10.1016/j.bbr.2017.09.050
Schotter, E. R., Berry, R. W., McKenzie, C. R. M., and Rayner, K. (2010). Gaze bias: selective encoding and liking effects. Vis. Cogn. 18, 1113–1132. doi: 10.1080/13506281003668900
Schumacher, S., Schnyder, U., Furrer, M., Mueller-Pfeiffer, C., Wilhelm, F. H., Moergeli, H., et al. (2013). Startle reactivity in the long-term after severe accidental injury: preliminary data. Psychiatry Res. 210, 570–574. doi: 10.1016/j.psychres.2013.06.034
Shafran, R., Lee, M., Cooper, Z., Palmer, R. L., and Fairburn, C. G. (2007). Attentional bias in eating disorders. Int. J. Eat. Disord. 40, 369–380. doi: 10.1002/eat.20375
Shiino, T., Miura, K., Fujimoto, M., Kudo, N., Yamamori, H., Yasuda, Y., et al. (2020). Comparison of eye movements in schizophrenia and autism spectrum disorder. Neuropsychopharmacol. Rep. 40, 92–95. doi: 10.1002/npr2.v40.1
Shiv, B., Loewenstein, G., Bechara, A., Damasio, H., and Damasio, A. R. (2005). Investment behavior and the negative side of emotion. Psychol. Sci. 16, 435–439. doi: 10.1111/j.0956-7976.2005.01553.x
Silverstein, S., Keane, B. P., Blake, R., Giersch, A., Green, M., and Kéri, S. (2015). Vision in schizophrenia: why it matters. Front. Psychol. 6:41. doi: 10.3389/fpsyg.2015.00041
Simion, C., and Shimojo, S. (2006). Early interactions between orienting, visual sampling and decision making in facial preference. Vision Res. 46, 3331–3335. doi: 10.1016/j.visres.2006.04.019
Smith, K. G., Schmidt, J., Wang, B., Henderson, J. M., and Fridriksson, J. (2018). Task-Related Differences in Eye Movements in Individuals With Aphasia. Front. Psychol. 9:2430. doi: 10.3389/fpsyg.2018.02430
Spence, C. (2019). Neuroscience-Inspired Design: from Academic Neuromarketing to Commercially Relevant Research. Organ. Res. Methods 22, 275–298. doi: 10.1177/1094428116672003
Spence, C., Okajima, K., Cheok, A. D., Petit, O., and Michel, C. (2016). Eating with our eyes: from visual hunger to digital satiation. Brain Cogn. 110, 53–63. doi: 10.1016/j.bandc.2015.08.006
Sperling, I., Baldofski, S., Lüthold, P., and Hilbert, A. (2017). Cognitive food processing in binge-eating disorder: an eye-tracking study. Nutrients 9:904. doi: 10.3390/nu9080903
Spinks, J., and Mortimer, D. (2016). Lost in the crowd? Using eye-tracking to investigate the effect of complexity on attribute non-attendance in discrete choice experiments Clinical decision-making, knowledge support systems, and theory. BMC Med. Inform. Decis. Mak. 16:14. doi: 10.1186/s12911-016-0251-1
Sprenger, A., Friedrich, M., Nagel, M., Schmidt, C. S., Moritz, S., and Lencer, R. (2013). Advanced analysis of free visual exploration patterns in schizophrenia. Front. Psychol. 4:737. doi: 10.3389/fpsyg.2013.00737
Tatler, B. W., Wade, N. J., Kwan, H., Findlay, J. M., and Velichkovsky, B. M. (2010). Yarbus, eye movements, and vision. i-Perception. 1, 7–27. doi: 10.1068/i0382
Thakkar, K. N., Brascamp, J. W., Ghermezi, L., Fifer, K., Schall, J. D., and Park, S. (2018). Reduced pupil dilation during action preparation in schizophrenia. Int. J. Psychophysiol. 128, 111-118. doi: 10.1016/j.ijpsycho.2018.03.012
Thomas, A. R., Pop, N. A., Iorga, A. M., and Ducu, C. (2016). Ethics and Neuromarketing: implications for Market Research and Business Practice. Germany: Springer International Publishing. doi: 10.1007/978-3-319-45609-6
Toh, W. L., Castle, D. J., and Rossell, S. L. (2015). Facial affect recognition in body dysmorphic disorder versus obsessive-compulsive disorder: an eye-tracking study. J. Anxiety Disord. 35, 49–59. doi: 10.1016/j.janxdis.2015.08.003
Touchette, B., and Lee, S. E. (2017). Measuring Neural Responses to Apparel Product Attractiveness: an Application of Frontal Asymmetry Theory. Cloth. Text. Res. J. 35, 3–15. doi: 10.1177/0887302X16673157
Tourangeau, R., and Yan, T. (2007). Sensitive Questions in Surveys. Psychol. Bull. 133, 859–883. doi: 10.1037/0033-2909.133.5.859
Tseng, P. H., Cameron, I. G. M., Pari, G., Reynolds, J. N., Munoz, D. P., and Itti, L. (2013). High-throughput classification of clinical populations from natural viewing eye movements. J. Neurol. 260, 275–284. doi: 10.1007/s00415-012-6631-2
Türkan, B. N., Amado, S., Ercan, E. S., and Perçinel, I. (2016). Comparison of change detection performance and visual search patterns among children with/without ADHD: evidence from eye movements. Res. Dev. Disabil. 4, 205–215. doi: 10.1016/j.ridd.2015.12.002
Underwood, G., Templeman, E., Lamming, L., and Foulsham, T. (2008). Is attention necessary for object identification? Evidence from eye movements during the inspection of real-world scenes. Conscious. Cogn. 17, 159–170. doi: 10.1016/j.concog.2006.11.008
Unema, P. J. A., Pannasch, S., Joos, M., and Velichkovsky, B. M. (2005). Time course of information processing during scene perception: the relationship between saccade amplitude and fixation duration. Vis. Cogn. 12, 473–494. doi: 10.1080/13506280444000409
van de Groep, I. H., de Haas, L. M., Schutte, I., and Bijleveld, E. (2017). Spontaneous eye blink rate (EBR) predicts poor performance in high-stakes situations. Int. J. Psychophysiol. 119, 50–57. doi: 10.1016/j.ijpsycho.2017.01.009
van der Laan, L. N., Hooge, I. T. C., De Ridder, D. T. D., Viergever, M. A., and Smeets, P. A. M. (2015). Do you like what you see? The role of first fixation and total fixation duration in consumer choice. Food Qual. Prefer 39, 46–55. doi: 10.1016/j.foodqual.2014.06.015
van Renswoude, D. R., Raijmakers, M. E. J., Koornneef, A., Johnson, S. P., Hunnius, S., and Visser, I. (2018). Gazepath: an eye-tracking analysis tool that accounts for individual differences and data quality. Behav. Res. Methods 50, 834–852. doi: 10.3758/s13428-017-0909-3
Venkatraman, V., Clithero, J. A., Fitzsimons, G. J., and Huettel, S. A. (2012). New scanner data for brand marketers: how neuroscience can help better understand differences in brand preferences. J. Consum. Psychol. 22, 143–153. doi: 10.1016/j.jcps.2011.11.008
Vriens, M., Vidden, C., and Schomaker, J. (2020). What I see is what I want: top-down attention biasing choice behavior. J. Bus. Res. 111, 262–269. doi: 10.1016/j.jbusres.2019.09.001
Vu, T. M. H., Tu, V. P., and Duerrschmid, K. (2016). Design factors influence consumers’ gazing behaviour and decision time in an eye-tracking test: a study on food images. Food Qual. Prefer. 47, 130–138. doi: 10.1016/j.foodqual.2015.05.008
Walker Smith, G. J., Gale, A. G., and Findlay, J. M. (1977). Eye movement strategies involved in face perception. Perception 6, 313–326. doi: 10.1068/p060313
Wolf, A., Ounjai, K., Takahashi, M., Kobayashi, S., Matsuda, T., and Lauwereyns, J. (2018). Evaluative processing of food images: a conditional role for viewing in preference formation. Front. Psychol. 9:936. doi: 10.3389/fpsyg.2018.00936
Wolf, A., Ounjai, K., Takahashi, M., Kobayashi, S., Matsuda, T., and Lauwereyns, J. (2019). Evaluative processing of food images: longer viewing for indecisive preference formation. Front. Psychol. 10:608. doi: 10.3389/fpsyg.2019.00608
Wolf, A., Ueda, K., and Hirano, Y. (2021). Recent updates of eye movement abnormalities in patients with schizophrenia: a scoping review. Psychiatry Clin. Neurosci. 75, 82–100. doi: 10.1111/pcn.13188
Yahata, N., Kasai, K., and Kawato, M. (2017). Computational neuroscience approach to biomarkers and treatments for mental disorders. Psychiatry Clin. Neurosci. 71, 215–237. doi: 10.1111/pcn.12502
Yarbus, A. L. (1967). Eye Movements and Vision. Unite States: Springer, doi: 10.1007/978-1-4899-5379-7
Yazdan-Shahmorad, P., Sammaknejad, N., and Bakouie, F. (2020). Graph-Based Analysis of Visual Scanning Patterns: a Developmental Study on Green and Normal Images. Sci. Rep. 10, 1–11. doi: 10.1038/s41598-020-63951-3
Zaltman, G. (2003). How Customers Think: essential Insights into the Mind of the Market, 1st Edn. United States: Harvard Business School Press.
Zayat, E., Rempfer, M., Gajewski, B., and Brown, C. E. (2011). Patterns of association between performance in a natural environment and measures of executive function in people with schizophrenia. Psychiatry Res. 187, 1–5. doi: 10.1016/j.psychres.2010.11.011
Keywords: clinical research, cognitive impairments, consumer science, eye-tracking, neuromarketing, translational practice
Citation: Wolf A and Ueda K (2021) Contribution of Eye-Tracking to Study Cognitive Impairments Among Clinical Populations. Front. Psychol. 12:590986. doi: 10.3389/fpsyg.2021.590986
Received: 03 August 2020; Accepted: 07 May 2021;
Published: 07 June 2021.
Edited by:
Eirini Mavritsaki, Birmingham City University, United KingdomReviewed by:
Henri Leinonen, University of California, Irvine, United StatesJoanne Harris, Swinburne University of Technology, Australia
Copyright © 2021 Wolf and Ueda. This is an open-access article distributed under the terms of the Creative Commons Attribution License (CC BY). The use, distribution or reproduction in other forums is permitted, provided the original author(s) and the copyright owner(s) are credited and that the original publication in this journal is cited, in accordance with accepted academic practice. No use, distribution or reproduction is permitted which does not comply with these terms.
*Correspondence: Alexandra Wolf, b2xhb2thbWlAZ21haWwuY29t