- 1Independent Researcher, Cali, Colombia
- 2Alliance Bioversity International and CIAT, Cali, Colombia
Household food waste represents one of the main challenges for sustainable development as this directly affects the economy of food consumers, the loss of natural resources and generates additional greenhouse gas emissions. The COVID-19 pandemic and its mitigation strategies caused one of the most serious economic crises in recent decades and could become the worst economic crisis that Latin America has had in its history. The objective of this study is to analyze changes in food waste behavior during the COVID-19 lockdown in Colombia in 2020, applying the Theory of Planned Behavior (TPB). For this purpose, we conducted a survey with 581 Colombian food consumers, which examined the influence of intentions to not waste food, subjective norms, some situational predictors, questions related to the COVID-19 pandemic, and the control of perceived behavior on food waste. The results suggest that the TPB can predict the intention to not waste food and, through it, the actual household food waste behavior, considering the lockdown in Colombia as an external shock. We observe that regarding the intention to not waste food, the most relevant variables are attitudes, subjective norms, control of the perceived behavior, and concerns regarding the Covid-19 pandemic. These variables increase the probability on average by a 0.8 Odds Ratio that the intention not to waste food increases, too. Regarding food waste behavior, whether it is considered ordinal or nominal, we see that the most relevant variables are intention, financial attitudes, and control of perceived behavior, doubling the probability that food waste behavior will improve. Based on the results, we provide recommendations for interested stakeholders that can help in the design of instruments for household food waste reduction.
Introduction
Food waste and loss account for about a third of the global annual food production volume (Gustavsson et al., 2011) and, despite the end consumer being the protagonist (Griffin et al., 2009), 13.8% of the global food leakage occurs at many different stages of the food value chains (FAO, 2017). There is a difference between what is considered food loss and food waste. While food loss occurs throughout the value chains, food waste happens at the retail and consumption levels (FAO, 2019). According to FAO (2011), ~54% of the global annual food leakage corresponds to loss, and 46% to waste.
Combatting food waste makes part of the Sustainable Development Goals (SDG), postulated by the United Nations in the 2030 Agenda for Sustainable Development (i.e., UN-SDG 12: Responsible Consumption and Production) (UN., 2015). Within UN-SDG 12, global food loss and waste are considered by SDG target 12.3, which states that “by 2030, halve global per capita food waste at the retail and consumer level and food losses in production chains and supply, including post-harvest losses” should be reduced (FAO, 2015). Additionally, reducing food waste contributes to the achievement of several other SDG, such as UN-SDG 1: End poverty in all its forms everywhere, and UN-SDG 2: End hunger, achieve food security and improved nutrition, and promote sustainable agriculture. According to the United Nations (UN., 2020b), from 2017 to 2019, 79 countries and the European Union reported on at least one national policy instrument that contributed to the implementation of the 10-Year Framework of Programmes on Sustainable Consumption and Production. Regions such as East Asia, Latin America and the Caribbean, Europe, and North America demonstrate a higher level of sustainability reporting, including water use and waste, than Africa, Central Asia, and Oceania (UN., 2020a).
The global food waste problem has drawn strong focus on research and public policy actions, since it is perceived as a phenomenon with negative effects at different levels, such as (i) the loss of money for both the households and the food value chains (e.g., an unnecessary expenditure of $ 750 billion in 2007; FAO, 2013), (ii) the loss of natural resources, which translates into social costs since food is lost while many people starve (e.g., restricted food security in developing countries; Buchner et al., 2012), and (iii) additional greenhouse gas emissions from the agriculture and livestock sector as well as the involved value chain links (e.g., the global carbon footprint of food waste in 2007 was equivalent to 3.3 gigatons CO2 emissions; FAO, 2013).
Food waste rather results from the lack of routines and planning in terms of production, purchase, and consumption than from intentional rational processes (Stefan et al., 2013), since most people share the idea of not wasting food (Bolton and Alba, 2012). According to the Food and Agriculture Organization of the United Nations (FAO), food waste, at the consumer level, is frequently associated with (i) non-planning regarding food purchase, (ii) overbought, overly large portions and package sizes, and confusion over labels, and (iii) storage problems at home (FAO, 2019).
Since the global spread of COVID-19 in 2020 and its declaration as a pandemic (WHO., 2020), the world is facing one of the worst challenges in modern times, and the high contagiousness of the virus has forced governments and local authorities to apply measures that help controlling it and protect citizens, including e.g., travel bans, temporary closures of public/private establishments, quarantine/confinement of individuals, and nationwide lockdowns, which have caused significant economic downturns. Although the world had faced previous economic recessions, the COVID-19 pandemic and the associated mitigation measures cause changes in employment, income, and health for the entire population (Rodgers et al., 2021). Angelucci et al. (2020) describe that these measures caused disproportionate job losses for those who could not carry out their activities remotely. Nicola et al. (2020) mention that the pandemic, until the publication of their study, has caused around 141 million confirmed cases and more than 3 million deaths at the global level, leading to fears of an imminent economic crisis only comparable with the economic scenarios during World War II and the Crisis of 1929 (World Bank., 2020). Social distancing, mobility restrictions, job losses, panic buying behavior and product shortages in supermarkets, and the overall transformation from what we considered to be normal to a new normality is now at the center of the daily news.
Not only does the pandemic affect public health, employment, or household incomes, but also the food system and its associated value chains, and disruptions are already noticeable and will likely grow over time (FAO, 2020). Ellison and Kalaitzandonakes (2020) mention that the COVID-19 lockdowns caused that some food did not reach the final consumers and is wasted in the different links of the food value chains.
Regarding the food consumers and their households, there are changes in behaviors regarding food waste, which can be grouped into two types (Ellison and Kalaitzandonakes, 2020; Pappalardo et al., 2020; Wang and Hao, 2020): First, panic caused hoarding effects among food consumers and led to food storage in large quantities, i.e., during the first weeks of confinement, since this gave them a sense of security, increasing household food waste considerably. Second, many households perceive income reductions, leading to less food waste since income and food waste are positively related.
There is no single food waste behavior in the face of the pandemic and its mitigation measures, but a heterogeneity in the behaviors linked to household income (Vidal-Mones et al., 2021). In the United Kingdom, for example, a study by Quinn (2020) revealed that the confinement led to considerable reductions in food waste of up to one third when compared to pre-lockdown levels, i.e., in staple foods, such as potatoes, bread, chicken and milk, which is related to the reduced amount of food consumed outside and the higher consumption of homemade food. Amicarelli and Bux (2020), using the food diary methodology, observed food waste trends during the COVID-19 lockdowns in Italy, and describe that they have resulted in an unprecedented situation that changed all the behavior patterns of individuals, including behavior toward food, and that there was a decrease in food waste resulting from the confinement which forced people to stay at home and gave them more time to learn or improve food planning and storage. Rodgers et al. (2021), through a logistic regression model, evaluated the effect of the lockdowns on decision making regarding food consumption and waste in the United States and Italy, and found that confinements decreased domestic food waste because of the general panic COVID-19 caused, which forced individuals to rethink their supply dynamics to reduce outflows of provisions. In this sense, individuals replaced the consumption of perishable food, such as fruits and vegetables, with prepared foods that last longer without deteriorating and are more easily storable.
The economic crisis caused by COVID-19 can become the worst economic crisis in the Latin Americas history. Unlike in Europe, in Latin American countries, the number of people who obey the instruction to stay at home is smaller, since their economic conditions in many cases prohibits such actions, i.e., the lack of unemployment insurances and formal paid and sick leaves. Additionally, many people live from their incomes as day laborers and have insufficient financial means to buy and store food for several days. Likewise, the crisis generates high levels of fiscal spending and indebtedness, which in many cases in Latin America are unsustainable. Considering this, strict COVID-19 measures, such as lockdowns and closures of restaurants, seem to respond better in countries with solid economic models (i.e., Europe), but can have high social costs in countries such as Latin America (Blofield et al., 2020). Considering the socioeconomic effects of the COVID-19 pandemic in Colombia, decreases in food production, reductions in household consumption, and job losses stand out, and according to Mejía (2020), these effects can lead Colombia to its first recession in the 21st century. In the second semester of 2020, Colombia's Gross Domestic Product had a negative growth rate, a situation that had not happened for around 15 years (UNDP., 2021). In Colombia, ~10 million tons of food are lost and wasted every year, which is equivalent to 34% of the total food supply destined for human consumption—an amount that could nourish more than 8 million people a year, which is equivalent to the population of Colombia's capital Bogotá (DNP, 2016). For Colombia, the proportion of food waste that occurs at the consumption level corresponds to only 15.6% of the total wastage, whereas food loss from agricultural production and post-harvest and storage processes represent 40.5 and 19.8%, respectively, and food loss from distribution and retail 20.6% (DNP, 2016).
Regarding the epidemiological situation in Colombia, by the end of 2020 1,642,775 cases of COVID-19 were reported, with a mortality rate of ~3% (43,213) (Wordometers., 2021). At that time, ~16,300 new cases were reported daily. By September 2021, Colombia was within the Top-10 countries with both confirmed COVID-19 cases (after the United States, Brazil, France, Argentina, among others) and related deaths (after the United States, India, Mexico, among others) (STATISTA., 2021a,b). To get an idea of the severity of the contagion of COVID-19 in Colombia, the Johns Hopkins University and the World Bank conducted an analysis of deaths per 100,000 inhabitants, and Colombia is ranked number nine worldwide, surpassed only by Peru, Hungary, Bosnia and Herzegovina, North Macedonia, the Czech Republic, Bulgaria, Brazil, and Argentina (RTVE., 2021).
The arrival of the outbreak in Colombia cannot be compared with other countries, not even with those from the same region, since in Colombia there are high rates of poverty, unemployment, low quality of life, and non-optimal economic conditions, which is why the arrival of COVID-19 instantly wreaked havoc. Especially when the proposed mitigation measures by the national government are considered, the above-described panorama even got worse since Colombia has such a high share of informal employment and the lockdown and its related economic downturn left behind those who make their living as day laborers. In addition, the pandemic caused stigmatization toward medical personnel, which responds to fear, mistrust and misinformation (Trejos-Herrera et al., 2020). Another effect of the multiple lockdowns and other measures in Colombia was the increase in incidents of domestic violence, depression, suicide, acute and post-traumatic stress, and anxiety, among others (Garfin et al., 2018; Caballero-Domínguez et al., 2020; Duan and Zhu, 2020; Lima et al., 2020; Pedrozo et al., 2020).
The objective of this article is to analyze changes in food waste behavior during the COVID-19 lockdown in Colombia in 2020. Specifically, we focus on two sub-objectives, namely the effects of the lockdown on (i) changes in the intention to reduce household food waste, and (ii) the actual behavior toward household food waste. We also evaluate whether it is appropriate or not to use the Theory of Planned Behavior (TPB) for predicting the intention and behavior regarding household food waste, based on our results for Colombia as a case study. Data was obtained from a virtual survey conducted during the strict lockdown in July 2020 with 581 households from the four major cities of Colombia: Bogotá, Medellín, Cali, and Barranquilla. With this information and considering the TPB, we apply Random Forest and Ordered Logistic Regression. This article is structured as follows: section 2 postulates the materials and methods, providing information on the theoretical framework, a literature review, data sources, and the description of the different models used. Section 3 presents our findings, section 4 the discussion, and section conclusions and recommendations.
Materials and Methods
Theoretical Framework
There is general agreement among social psychologists that most human behavior is goal-directed. Without this being considered frivolously, human behavior is defined by the realization of structured plans (Ajzen, 1985). As Mankiw (2009) postulates within the 10 principles of economics, individuals respond to incentives because they are rational. Ajzen (1985) posits that there is a difference between intentions and actions, and, although all actions are controlled by intentions, generally not all intentions materialize into actions. Considering this, Ajzen (1985) postulates the Theory of Reasoned Action (TRA) in which beliefs, attitudes, and intentions interact to arrive at a certain behavior.
Ajzen (1985) also points out a difference in the degree of volitional consciousness of individuals and considers internal and external factors that can influence motivation when performing difficult tasks. The Theory of Planned Behavior (TPB) is then the result of considering the reasoned action, plus a measure of control over behavior (Ajzen, 1988, 1991). In this sense, in the TPB, intentions can only be expected to predict a person's attempt to perform a behavior, not necessarily their actual performance. The theory considers all the factors beyond a person's control that prevent him from achieving his goal, even if he intends and thus, the immediate determinant of a person's attempt to conduct is his intention to attempt to do so.
The TPB has been validated in multiple research contexts, for example, in studies related to (i) choices on different transportation methods (Bamberg et al., 2003; Gardner and Abraham, 2010) (ii) recycling patterns and conscious consumption (Boldero, 1995; Taylor and Todd, 1995; Sparks et al., 2014), and (iii) predicting patterns of food consumption and food waste (Stefan et al., 2013; Graham-Rowe et al., 2015; Mallinson et al., 2016; Zhang et al., 2019).
In summary, the TPB reflects attitudes toward behavior, the subjective norms, and behavioral control. It is possible to show that there exists a gap between intention and behavior and that both are conditioned by attitudes, subjective norms, and perceived behavioral control. For this article, we use the TPB to analyze household behavior regarding food waste and the questionnaire design was based on Ajzen (1985), contemplating elements such as subjective norms, intentions, attitudes, perceived behavioral control, financial attitudes, identity of a good supplier, and personal identity.
Literature Review
There is consensus among researchers that people share the idea of not wasting food (e.g., Lyndhurst et al., 2007; Stefan et al., 2013; Jagau and Vyrastekova, 2017). Therefore, it is better to consider that the attitude to waste food is measured as a lack of concern about food waste and not as a right or wrong decision on wasting food (e.g., Lanfranchi et al., 2016). Arvola et al. (2008) and Olsen et al. (2010) considered the influence of moral attitudes on intentions as this improves predictions. In the sense of food waste, people generally feel guilty when they waste or take wasteful attitudes (Bolton and Alba, 2012).
The link of the TPB and food waste was first established by Stefan et al. (2013) to assess if food waste could be related to the food choices and planning within Romanian households. Their results show that moral attitudes toward food waste determine food planning and purchasing routines and, through them, control food waste. In other words, the upright considerations about food waste allow consumers to plan their consumption, hoping they do not have to waste and, therefore, reduce waste.
Graham-Rowe et al. (2015) used the TPB to evaluate fruit and vegetable waste among households in the United Kingdom, applying a linear regression model. They found that attitudes, subjective norms, control of perceived behavior, among others, represented ~64% of the variation of intentions regarding food waste. Regarding changes of behavior, however, they found that only about 5% of the actual behavior changed. As additional predictors to the basic model of planned behavior, they identified consumer identity, early repentance, moral standards, and descriptive norms. Mallinson et al. (2016), also among households in the United Kingdom, identified that household size, packaging format, price awareness, marketing, and behavioral and sociocultural factors influence food waste levels. Stancu et al. (2015) applied the TPB to evaluate the effects of intentions regarding food waste in Denmark, including intention, subjective norms, attitudes toward food waste and perceived behavioral control in their models. They found that intentions, subjective norms, and attitudes contribute to reducing food waste, while perceived behavioral control does not make a significant contribution.
Setti et al. (2018) also postulate the TPB as the indicated option to evaluate aspects related to domestic food waste and based on their study with Italian food consumers suggest that ingrained judgments, values, habits and hunger, the so-called visceral factors (Loewenstein, 1996; Verplanken et al., 1998; Graham-Rowe et al., 2014), influence food decisions, and that there is a gap between food decisions and the results of actual behavior measured as food waste.
Zhang et al. (2019) linked the TPB with consumption behaviors after an outbreak of avian influenza in 2017 in China and found that attitudes, subjective norms, control of perceived behavior, and perceptions about influenza effectively modeled the consumption intention, specifically regarding poultry. This study suggests the suitability of the TPB to model behaviors even when extreme situations like avian influenza or COVID-19 occur.
Data Sources
To analyze the effects of the COVID-19 lockdown on household food waste behavior, and considering the TPB as theoretical construct, we applied a household survey with food consumers from the four principal cities in Colombia: Bogotá, Medellín, Cali, and Barranquilla. A total of 579 people were surveyed, with an age of minimum 18 years, from the socioeconomic strata 2–61, and with knowledge of their household food expenses and participation in the preparation of food at their homes. The survey was carried out virtually (given the restrictions in Colombia at that time) in July 2020 through an online panel by the company Cluster Research2 Below we describe the selected variables and dimensions and their importance.
Explanatory Variables
Within the explanatory variables, we consider four groups, namely (i) situational predictors of food waste behavior (Visschers et al., 2016), (ii) dimensions postulated by an extended version of the TPB (Carrigan et al., 2006; Evans, 2012; Watson and Meah, 2012; Graham-Rowe et al., 2015; Parizeau et al., 2015; Visschers et al., 2016; McCarthy and Liu, 2017), (iii) the COVID-19 lockdown and the effect of fear and the search for normality, and (iv) sociodemographic control variables.
• Situational predictors of food waste behavior:
• Household size: Positively related to the amount of food waste (Koivupuro et al., 2012; Williams et al., 2012; Quested et al., 2013; Parizeau et al., 2015; Schmidt, 2016).
• Age of the person in charge of food preparation: The older a person is, the less food waste results (Lyndhurst et al., 2007; Watson and Meah, 2012; Thyberg and Tonjes, 2016).
• The budget available for food purchase (household income): The more is spent on food, the higher the food waste is (Watson and Meah, 2012; Quested et al., 2013; Neff et al., 2015; Parizeau et al., 2015).
(ii) Extended version of the TPB:
Regarding the dimensions mentioned by the extended version of the TPB, we constructed the survey considering the basic and most relevant dimensions to make the survey easily accessible for the interviewees. During the data cleaning process, we considered the averages of the items that make up each of the dimensions and constructed eight variables from 22 items, namely (i) Attitudes about food waste, (ii) Subjective norms, (iii) Control of perceived behavior, (iv) Moral standards, (v) Financial attitudes, (vi) Identity of a good supplier, (vii) Intention to not waste food, and (viii) Declared behavior regarding food waste (Table 1).
(iii) COVID-19 lockdown questions that show the effect of fear and the search for normality:
During extreme situations such as a pandemic, individuals tend to take a self-protective behavior and change their consumption patterns whenever they feel that this will keep them more protected (Lau et al., 2006; Bish and Michie, 2010). The Theory of Protective Action, usually used to describe behaviors in the face of disasters and environmental hazards, postulates that the perceived risk of the situation is the main factor that generates protective behaviors, including behaviors toward consumption (Yeung and Morris, 2001; Pennings et al., 2002; Hornibrook et al., 2005; Lobb et al., 2007; Lindell and Perry, 2012). For this reason, we consider as additional predictors two variables regarding COVID-19 and food consumption:
• The return to the pre-COVID-19-normality: How long do you think it will need until the country will return to normality, i.e., the free movement and gathering of persons at anytime and anywhere in the national territory?
• Concerns regarding the COVID-19 pandemic: How concerned are you about the COVID-19 situation?
(iv) Sociodemographic control variables:
The behavior toward food waste, whether this is about reduction, reuse, or recycling, responds to personal situations, sociodemographic characteristics, and knowledge/experiences (Barr, 2007). In this sense and following previous studies (e.g., Hines et al., 1987; Barr, 2007; Stancu et al., 2015; Thyberg and Tonjes, 2016) we selected the following sociodemographic control variables:
• Gender: There is evidence that women have more pronounced attitudes toward recycling than men (e.g., Barr, 2007; Principato et al., 2015).
• Educational level: There is a positive relationship between education and good practices regarding food waste, monthly household income and income reductions due to the COVID-19 pandemic, as households with higher incomes may recognize the importance of not wasting food and have the means not to do so (e.g., Koivupuro et al., 2012; Principato et al., 2015; Setti et al., 2016).
• City of residence: There are effects inherent to cities such as cultural aspects (e.g., Stuart, 2009).
Figure 1 summarizes the dimensions that were considered in this study, which are consistent with an extended version of the TPB.
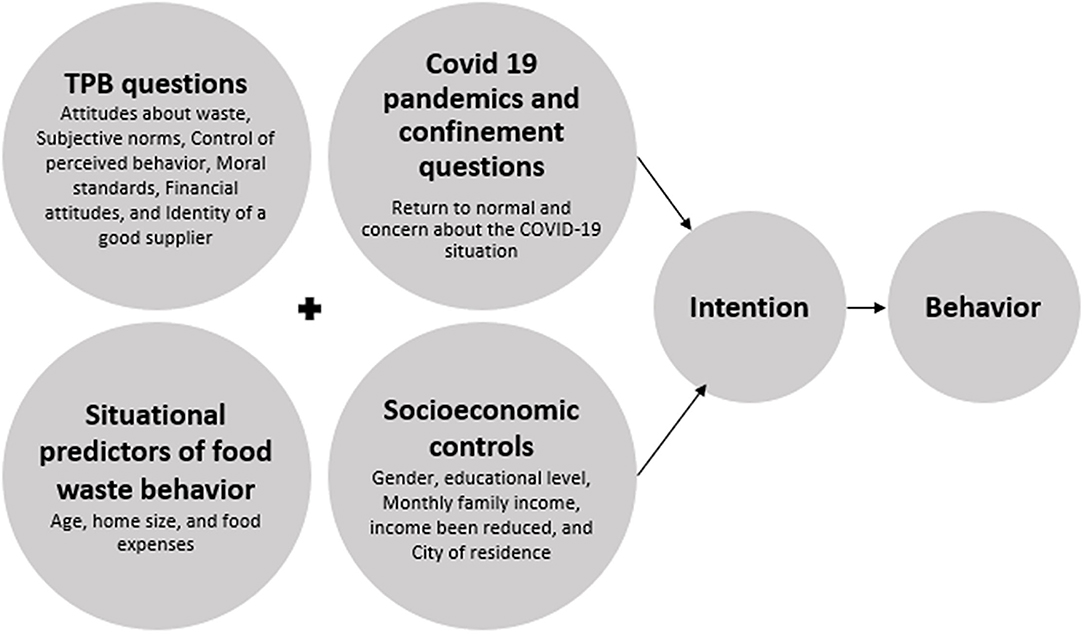
Figure 1. Overview on the dimensions used to predict food waste intention and behavior. Source: own elaboration.
Methodological Approach: Supervised Learning-Random Forest
We consider that supervised learning is a good option to use machine learning since our study counts on a set of labeled data. In supervised learning, a part of the data is used to train the models, so that the algorithm can generalize behaviors and correctly assign classifications. The objective of using supervised learning in this document is to generate models that can classify whether a person would change his behavioral patterns (or intentions) regarding household food waste, given information about their attitudes, subjective norms, and perceived behavioral control, in addition to sociodemographic control variables. The Random Forest joint learning algorithm consists of many decision trees, and, in this sense, it manages to average the classifications/predictions of the individual trees, considerably improving the initial algorithm (Breiman, 2001). One of the desirable characteristics of this algorithm is that it works well regardless of whether the target variable has balancing problems (Breiman, 2001; Liaw and Wiener, 2002).
Methodological Approach: Ordered and Multinomial Logistic Regression
An ordered logistic regression is an extension of the dichotomous logistic regression model and a regression model for ordinal dependent variables. Considering that the dependent variable related to food waste intention is ordinal, we consider this empirical strategy as appropriate.
The model only applies to data that meet the proportional Odds assumption, meaning that the number added to each of these logarithms to get the next is the same in every case. In other words, these logarithms form an arithmetic sequence.
For this article, the scale used is a Likert-scale from 1 to 5, with 5 being the maximum value for each question. We observe that for this set of ordered categories, the behavior of the responses coincides with that of continuous variables, since the increase between the categories occurs by one unit. In this sense, in addition to ordered logistic regression and as a robustness check, we also use a linear regression (considering the variables as continuous) to see the effect of the dependent variables on the intention or declared behavior regarding food waste. We estimate that the effect of moving from a lower to a higher category coincides with the effect of increasing one unit on the propensity to change. As Grace-Martin (2009) mentions, if the predictor is categorical and fictionally coded, a difference of one unit coincides with the change from one category to another.
An important caveat is that the continuous approach is only being considered as an additional robustness check, since we recognize that this treatment of categories as continuous data may have weaknesses. Thus, we estimate the following equation for food waste intention:
Where Intentioni refers to the average intention rating of individual i (1 being the lowest intention and 5 the highest). The SituacionalPredictors, ConfinementQuestions, and TPB are the variables of these categories (see section Explanatory Variables) whose descriptor engineering considers them relevant.
Logistic regression models have many variants in which the dependent variable can have more than two categories (multinomial) or is of ordinal (ordered) character (Long, 1997; Hosmer and Lemeshow, 2000; Peña, 2002; Llaugel and Fernández, 2011). When the dependent variable is the declared behavior of the treatment, it ceases to be ordinal and becomes nominal. In this case, we use a multinomial logistic regression, since the dependent variable responds to independent categories (increased, decreased, and stayed the same). This method corresponds to a generalization of binary logistic regression and is used to model elections, for example. The equation to estimate food waste behavior in this case is:
Where Behaviori has two possible presentations: ordinal and multinomial. In the ordinal analysis, Behaviori refers to the average assessment of individual i's behavior (1 being a negative and 5 a positive assessment). In the multinomial analysis, Behaviori can take three values (increase, decrease, or stay the same). In this sense, multinomial behavior can be considered a declared behavior while ordinal behavior will only present a potential behavior. Like for Equation (1), The SituacionalPredictors, ConfinementQuestions, and TPB are the variables of these categories (see section Explanatory Variables) whose descriptor engineering considers them relevant.
Limitations of This Study
The database used in this document has several limitations that must be considered.33ee The most important one is that the measure of household food waste, and all dimensions considered, were self-reported by the survey participants through a virtual survey and collected at a specific point in time (July 2020). Sheen et al. (2020) mention the lack of consensus regarding measures of food waste. Additionally, as mentioned before, the dimensions can respond to prosocial norms with answers that may be biased motivated by the feelings of anguish typical at the beginning of the COVID-19 pandemic (e.g., Quested et al., 2011; Krumpal, 2013). Finally, the sample considered in this study is not necessarily representative as we only obtained data from households in the four major cities of Colombia, which cannot necessarily be generalized for the entire country, since the socioeconomic conditions and cultural habits vary, i.e., among rural areas and cities. For this reason, although the results are relevant and shed light on food waste behavior during the COVID-19 lockdown, which can be used for designing policy responses, the real food waste behavior of the country, might be different.
Additionally, because the responses related to food waste intention and behavior, among other dimensions of the TPB, are located between the maximum values (4–5) of the scale, the database is unbalanced. This imbalance can bias the results according to the methodological approach used. In this sense, this weakness might be affecting our results (e.g., Geurts et al., 2006; Strobl et al., 2007; Yousefi et al., 2010).
Although logistic regression models work relatively well, they have some weaknesses, namely they can suffer from (i) overfitting, meaning that if the logistic regression is performed on big data sets, the selection of the variable will take a long time (Geng, 2006), and (ii) influence values, which have an undue influence on the overall fit of the model, meaning that the logistic estimates are consistent and asymptotically efficient before a balanced data set only and tend to be biased if there are balancing problems.
In summary, there exist gaps in both approaches (e.g., Colombet et al., 2000; Lee et al., 2005; Steyerberg et al., 2010; Boulesteix et al., 2013), which can be filled by combining them, which is the reason why we decided to implement both algorithms to provide robust results that respond to classical and modern techniques.
Results
Descriptive Statistics and First Considerations
Table 2 shows the descriptive statistics of the variables under analysis. We use intention to not waste food and behavior regarding food waste as our main dependent variables. As independent variables, we include sociodemographic control variables in the econometric models.
We observe that ~84% of the surveyed households perceived a reduction in their incomes, and data shows that behavior has a strong tendency toward decreasing food waste. Specifically, 55% of the households declared to have reduced food waste, and for 31 and 14%, food waste remained at the pre-pandemic levels or increased, respectively. This finding is preliminary evidence of the positive relationship between household income and household food waste during the COVID-19 lockdown.
Furthermore, the logistic regression between the decrease in household food waste and the decrease in household income reveals that if the household challenges income decreases, the Odds Ratio of decreasing household waste triples. Specifically, if the household income declines by more than 25%, the Odds Ratio of reducing household food waste ranges between 60 and 75%.
The TPB postulates that there is a gap between intention and behavior. In this sense, the results are first presented with intention as the dependent variable, and then the variable behavior is considered.
Figure 2 shows that most of the surveyed households agree that they have an intention to not waste food. Specifically, 61% fully agree with having an attitude that reduces food waste. Figure 3 shows the distribution of the respondents' declared behavior. The comparison of Figures 2, 3 allows to observe the existing gap between food waste intention and behavior: Although 61% of the respondents fully agree on the intention to reduce food waste, only 22% fully agree with having a behavior that reduces food waste.
Consumer Intention to Not Waste Food
For intention as dependent variable, the accuracy data are 0.65 for Random Forest, The Random Forest classification model indicates that the total percentage of items classified correctly regarding the intention to not waste food is 63.79%. One of the main advantages of this model is that it allows observing the importance of the attributes (independent variables) that compose it, which is the increase in the prediction error if the attribute disappears. This allows us to observe a summary and global vision of the behavior of the model. Table 3 shows the results of the Random Forest model for the variable intention.
The results show that the most significant variables in the classification for the variable intention to not waste food are food waste attitudes, the return to normality (no lockdown), household income, reduction in household income, financial attitudes, and control of perceived behavior. This indicates in a first step that the TPB is a valid framework for our analysis.
We implemented an ordered logistic regression (see robustness check with linear regression in Annex 1), using the most relevant independent variables to strengthen our analysis. The variables selected for the variable intention to not waste food are return to normality (no lockdown), household income, food waste attitudes, reduction in household income, financial attitudes, control of perceived behavior, educational level, concerns about the COVID-19 situation, subjective norms, gender, food expenses, moral standards, and identity of a good supplier.
The results of the ordered logistic regression (Table 4) suggest that only the variables concern about the COVID-19 situation, food waste attitudes, financial attitudes, subjective norms, and control of perceived behavior are significant in explaining changes in the intention regarding food waste. We observe that in both the Random Forest model and the logistic models, the variables related to the TPB effectively explain changes in the intention to not waste food. The logistic regression uses Odds Ratio as standardized measure to compare the effect of the independent variables on the dependent variable. Thus, an increase in the food waste attitude (which means that a person is more in agreement with not wasting food) more than doubles (2.2896) the Odds Ratio that the intention to not waste food increases, keeping all other variables constant. Likewise, an increase in the control of perceived behavior (which means that a person feels that he has more control over the level of waste) increases the Odds Ratio by 1.8649 that the intention to not waste food will increase.
The cut points shown at the bottom of the output indicate where the dependent variable is cut to form the five groups we observed in our data, having in mind that this latent variable is continuous.
Behavior Regarding Food Waste
In a first step, we evaluate behavior under a Likert scale in which the survey participants had to define from 1 to 5 how much they agree with the following statements: (i) I have given away food that I do not eat, (ii) I prepare and/or keep the remaining food for an upcoming opportunity, (iii) I buy more food than necessary as a precaution (inverted question), and (iv) Before I prepare the meal, I think about how much I need to prepare. In this sense, behavior is evaluated against many fronts that reduce household food waste, but not against the actual reduction of food waste. In a second step, we evaluate the declared behavior against the actual reduction of household food waste.
Potential Behavior
Using potential behavior as dependent variable, the accuracy data are 0.48 for Random Forest, Table 5 shows the results of the Random Forest model for the variable ordinal declared behavior.
This finding suggests that the TPB framework responds well to analyzing the decision to reduce household food waste during the COVID-19 lockdown. It also shows that financial attitudes play a significant role, highlighting once again that there exists a relationship between household income and behavior toward food waste.
Additionally, we performed an ordered logistic regression (see robustness check in Annex 2) to check our results (Table 6). The variables selected for behavior are financial attitudes, intention, control of perceived behavior, and gender.
For the variable declared behavior, the logistic regression shows that only the variables intention and control of perceived behavior are significant, which coincides with what is established by the TPB. An increase by one unit in the variable intention (which means that a person has a higher intention to not waste food) increases the Odds Ratio by 2.5 that the declared behavior increases. In the same way, if the control of perceived behavior increases by one unit, the Odds Ratio of the declared behavior increases by 2.1.
Declared Behavior
Using declared behavior as dependent variable, the accuracy data are 0.47 for Random Forest, Table 7 shows the results of the Random Forest model for the variable nominal declared behavior.
Like for declared behavior, this finding suggests that the TPB framework responds well to analyzing the decision to reduce household food waste during the COVID-19 lockdown, and that financial attitudes play a significant role, highlighting again that there is a relationship between household income and behavior toward food waste.
Additionally, we performed an ordered logistic regression to check the results. The variables selected for behavior are financial attitudes, intention, control of perceived behavior, gender, reduction in household income, and changes in food expenses.
For declared behavior, the multinomial logistic regression (Table 8) shows that only the variables reduction in household income and changes in food expenses are significant, revealing an incompatibility with what is established by the TPB. The reason for this is that the COVID-19 pandemic and the resulting mitigation strategies (such as lockdowns) caused one of the most serious economic crises in recent years, which led to reductions in household incomes, and, although the TPB manages to predict the intention to not waste food, when it comes to materializing this intention, the effects of the economic variables (reduction in household income and changes in food expenses) overshadow the effects of other variables.
Discussion
Household Food Waste During the COVID-19 Pandemic
The results of our study are in line with other research on food waste. Although the TPB explains the variable intention to not waste food to be important for predicting declared behavior or actual behavior, other variables, such as socioeconomic controls, become relevant, too, supporting the findings of Conner and Armitage (1998). As mentioned by Stefan et al. (2013) and Graham-Rowe et al. (2015), the intention to reduce household food waste can be predicted by considering the variables attitudes, subjective norms, and control of perceived behavior.
Regarding declared behavior, the intention not to waste food has no significant effects on the variable nominal declared behavior since pandemic-related economic factors overshadow the effects of other factors, which coincides with Stefan et al. (2013) who described that although the planning and purchasing routines manage to explain food waste behavior, the intention seems not to be significant. This result reinforces the existing gap between intention and behavior (e.g., Armitage and Conner, 2001; Sheeran, 2002). Graham-Rowe et al. (2015) found that the variation of intentions with respect to food waste explained by the dimensions of the TPB is ~64%; however, with respect to behavioral changes, they found that only about 5% of the actual behavior changed.
The circumstances of the COVID-19 pandemic must be considered within the predictions of intention and subsequently of behavior (as ordinal and nominal variable), which is in line with the findings of Rodgers et al. (2021), who describe that the pandemic has created circumstances which have resulted in both changes in attitudes and behavior when it comes to household food waste.
The findings of this paper support the first hypothesis that, according to the TPB model (Ajzen, 1988, 1991), attitude, subjective norms, and perceived behavioral control can predict the intention to reduce household food waste. Households in favor of food waste reduction, feel that other people also approve the reduction of food waste, leading to a higher likelihood of developing an intention to reduce household waste, which was also shown by (Arvola et al., 2008; Olsen et al., 2010; Stefan et al., 2013; Stancu et al., 2015). Additionally, we found that financial attitudes, concerns regarding COVID-19 and the return to normality (no lockdown) are also significant variables for the intention to reduce household food waste, which is in line with the study presented by Zhang et al. (2019).
Regarding ordinal behavior, it can be predicted considering the variables intention and control of perceived behavior, which is in line with what was proposed by the TPB. Both predictors double the probabilities of behavioral changes. For nominal behavior, the results are, however, not in line with what was proposed by the TPB, since it suggests that only the variables reduction in household income and changes in food expenditures predict it. As mentioned in the results, we consider that this might be due to the strong impacts the COVID-19 pandemic had on household incomes in Colombia.
As Grace-Martin (2019) mentions, logistic regression is a good tool to have in the statistical tool belt. Within the logistic regressions, the most used for categorical results with more than two categories are the multinomial and ordinal varieties. As they have different assumptions, however, the results are also different (e.g., Pedhazur, 1997; Schwab, 2002). Leadbeater (2019) shows that one of the differences between ordered and multinomial models (in addition to their assumptions) is that the ordinal model appears to be more useful as a generalization and “top view” procedure. Otherwise, multinomial models are more appropriate for a more detailed view of group-level comparisons.
We find it interesting to consider both behaviors (ordinal and nominal), since although the TPB can predict ordinal behavior, it seems to fail predicting nominal behavior. We emphasize that although there have been more economic crises, the crisis generated by the COVID-19 pandemic had and has an unprecedented effect since it changed each of the activities that defined our daily lives in the pre-pandemic era. This result is consistent with the literature which states that in a crisis in which people feel threatened, their behaviors will be anticipated to take measures to avoid suffering the negative effects (Chapman and Coups, 2006; Leppin and Aro, 2009; Rivis et al., 2009). Furthermore, as mentioned above, the results of the multinomial analysis are more detailed and may account for a more specific effect.
Conclusions
This document examined the effectiveness of the Theory of Planned Behavior to predict the intention and behavior regarding household food waste in the four major cities of Colombia, considering the COVID-19 lockdown as a case study. This research seeks to provide robust results regarding strategies that allow reducing household waste considering the consumers' preferences and their attitudes toward external shocks and, therefore, is a contribution to the existing empirical evidence, which allows authorities and decision-makers considering and understanding consumer preferences and developing more effective and efficient policies regarding food waste and responsible production and consumption.
Considering that the COVID-19 pandemic is perhaps the most critical event for humanity in recent history, our literature review revealed that research is being conducted that seeks to assess the economic and social effects of the crisis. Since the pandemic is an unprecedented event, the findings are, however, ambiguous. This study, by using modern and conventional techniques of analysis, therefore contributes to the literature by evaluating the effects of COVID-19 on an issue as intrinsic as food waste and consumption patterns and seeks to provide a point of comparison for future research.
The results found in this study respond to a specific point of time and may vary as personal values, preferences, and the information available about COVID-19 change. The results provide, however, information that shows the suitability of the Theory of Planned Behavior for analyzing food waste intentions and behavior, even when considering a critical event of shock for the households such as the COVID-19 lockdown.
Our results allow for providing policy recommendations that achieve behavioral modifications to reduce food waste at home. Although personal considerations about household food waste (i.e., intention, attitudes, and some other TPB dimensions) are relevant predictors, the differential factor between the willingness to not to waste food and effectively not doing it lies in the economic conditions of the households. This highlights the need for strategies aimed at reducing household food waste that consider the different existing income and food expenditure levels, and, above all, the reductions in household incomes caused by the COVID-19 pandemic (Ruiz-Roso et al., 2020; Vidal-Mones et al., 2021). Additionally, fear and concern about are also significant predictors (e.g., Lobb et al., 2007; Stefani et al., 2008), highlighting the need of accurate communication strategies regarding the COVID-19 pandemic and its impacts on society, e.g., by providing correct numbers on infections, unemployment, deaths, and mitigation measures, for reducing panic-driven consumption behaviors.
Data Availability Statement
The raw data supporting the conclusions of this article will be made available by the authors, without undue reservation.
Ethics Statement
The studies involving human participants were reviewed and approved by Internal Review Board of the Alliance of Bioversity International and CIAT. The patients/participants provided their written informed consent to participate in this study.
Author Contributions
AC, KE, MD, and SB: conceptualization and resources. DM, AC, and MD: methodology. DM: formal analysis. DM, MD, and SB: writing the original draft and review and editing. SB: supervision, funding acquisition, and project administration. All authors contributed to the article and approved the submitted version.
Funding
This work was undertaken as part of the CGIAR Research Program (CRP) on Livestock.
Conflict of Interest
The authors declare that the research was conducted in the absence of any commercial or financial relationships that could be construed as a potential conflict of interest.
Publisher's Note
All claims expressed in this article are solely those of the authors and do not necessarily represent those of their affiliated organizations, or those of the publisher, the editors and the reviewers. Any product that may be evaluated in this article, or claim that may be made by its manufacturer, is not guaranteed or endorsed by the publisher.
Acknowledgments
We thank all donors that globally support the work of the CRP programs through their contributions to the CGIAR system.
Footnotes
1. ^Socioeconomic stratification in Colombia categorizes housing units in a scale of one to six according to their physical characteristics, immediate surroundings and rural or urban context. Higher strata (five and six) and lower strata (one, two and three) [Departamento Administrativo Nacional de Estadística (DANE), 2021]. Retrieved from: https://www.dane.gov.co/index.php/servicios-al-ciudadano/servicios-informacion/estratificacion-socioeconomica
References
Ajzen, I. (1985). “From intentions to actions: a theory of planned behavior,” in Action Control. SSSP Springer Series in Social Psychology, eds J. Kuhl and J. Beckmann (Berlin; Heidelberg: Springer). doi: 10.1007/978-3-642-69746-3_2
Ajzen, I. (1991). The theory of planned behavior. Org. Behav. Hum. Decis. Process. 50, 179–211. doi: 10.1016/0749-5978(91)90020-T
Amicarelli, V., and Bux, C. (2020). Food waste in Italian households during the Covid-19 pandemic: a self-reporting approach. Food Secur. 13, 25–37. doi: 10.1007/s12571-020-01121-z
Angelucci, M., Angrisani, M., Bennett, D., Kapteyn, A., and Schaner, S. (2020). Remote work and the heterogeneous impact of COVID-19 on employment and health. Cambridge, MA: National Bureau of Economic Research.
Armitage, C. J., and Conner, M. (2001). Efficacy of the theory of planned behaviour: a meta-analytic review. Br. J. Soc. Psychol. 40, 471–499. doi: 10.1348/014466601164939
Arvola, A., Vassallo, M., Dean, M., Lampila, P., Saba, A., Lähteenmäki, L., et al. (2008). Predicting intentions to purchase organic food: the role of affective and moral attitudes in the theory of planned behaviour. Appetite 50, 443–454. doi: 10.1016/j.appet.2007.09.010
Bamberg, S., Ajzen, I., and Schmidt, P. (2003). Choice of travel mode in the theory of planned behavior: the roles of past behavior, habit, and reasoned action. Basic Appl. Soc. Psychol. 25, 175–187. doi: 10.1207/S15324834BASP2503_01
Banco de la República. (2021). Tasa Representativa del Mercado (TRM - Peso por dólar). Retrieved online at: https://www.banrep.gov.co/es/estadisticas/trm
Barr, S. (2007). Factors influencing environmental attitudes and behaviors: a U.K. Case study of household waste management. Environ. Behav. 39, 435–473. doi: 10.1177/0013916505283421
Bish, A., and Michie, S. (2010). Demographic and attitudinal determinants of protective behaviours during a pandemic: a review. Br. J. Health Psychol. 15, 797–824. doi: 10.1348/135910710X485826
Blofield, M., Hoffmann, B., and Llanos, M. (2020). Assessing the Political and Social Impact of the Covid-19 Crisis in Latín America. Hamburg: GIGA German Institute of Global and Area Studies - Leibniz-Institut für Globale und Regionale Studien, Institut für Lateinamerika-Studien. Available online at: https://nbn-resolving.org/urn:nbn:de:0168-ssoar-67260-7
Boldero, J. (1995). The prediction of household recycling of newspapers: the role of attitudes, intentions and situational factors. J. Appl. Soc. Psychol. 25, 440–462. doi: 10.1111/j.1559-1816.1995.tb01598.x
Bolton, L. E., and Alba, J. W. (2012). When less is more: consumer aversion to unused utility. J. Consum. Psychol. 22, 369–383. doi: 10.1016/j.jcps.2011.09.002
Boulesteix, A.-L., Lauer, S., and Eugster, M. J. (2013). A plea for neutral comparison studies in computational sciences. PLoS One. (2013) 8:e61562. doi: 10.1371/journal.pone.0061562
Buchner, B., Fischler, C., Gustafso, E., Reilly, J., Riccardi, G., and Ricordi, C. (2012). Food Waste: Causes, Impacts and Proposals. Parma: Barilla Center for Food & Nutrition. Available online at: https://www.barillacfn.com/m/publications/food-waste-causes-impact-proposals.pdf (accessed October 11, 2021).
Caballero-Domínguez, C. C., Jimenez, M. P., and Campo-Arias, A. (2020). Suicide risk during the lockdown due to coronavirus disease (COVID-19) in Colombia. Death Stud. (2020) 1–6. doi: 10.1080/07481187.2020.1784312
Carrigan, M., Szmigin, I., and Leek, S. (2006). Managing routine food choices in UK families: the role of convenience consumption. Appetite 47, 372–383. doi: 10.1016/j.appet.2006.05.018
Chapman, G. B., and Coups, E. J. (2006). Emotions and preventive health behavior: worry, regret, and influenza vaccination. Health Psychol. 25, 82–90. doi: 10.1037/0278-6133.25.1.82
Colombet, I., Ruelland, A., Chatellier, G., Gueyffier, F., Degoulet, P., and Jaulent, M. C. (2000). Models to predict cardiovascular risk: comparison of CART, multilayer perceptron, and logistic regression. Proc AMIA Symp. 156–160. Available online at: https://www.ncbi.nlm.nih.gov/pmc/articles/PMC2244093/
Conner, M., and Armitage, C. J. (1998). Extending the theory of planned behavior. A review and avenues for further research. J. Appl. Soc. Psychol. 28, 1429–1464. doi: 10.1111/j.1559-1816.1998.tb01685.x
Departamento Administrativo Nacional de Estadística (DANE). (2021). Socioeconomic Stratification for Home Public Services. Retrieved from: https://www.dane.gov.co/index.php/servicios-al-ciudadano/servicios-informacion/estratificacion-socioeconomica
DNP (2016). Pérdida y Desperdicio de Alimentos en Colombia: Estudio de la Dirección de Seguimiento y Evaluación de Políticas Públicas. Bogotá: DNP. Available online at: https://mrv.dnp.gov.co/Documentos%20de%20Interes/Perdida_y_Desperdicio_de_Alimentos_en_colombia.pdf
Duan, L., and Zhu, G. (2020). Psychological interventions for people affected by the COVID-19 epidemic. Lancet Psychiatry 7, 300–302. doi: 10.1016/S2215-0366(20)30073-0
Ellison, B., and Kalaitzandonakes, M. (2020). Food Waste and Covid-19: Impacts along the Supply Chain. farmdoc daily (10):164, Department of Agricultural and Consumer Economics, University of Illinois at Urbana-Champaign. Available online at: https://farmdocdaily.illinois.edu/2020/09/food-waste-and-covid-19-impacts-along-the-supply-chain.html
Evans, D. (2012). Beyond the throwaway society: ordinary domestic practice and a sociological approach to household food waste. Sociology 46:41e56. doi: 10.1177/0038038511416150
FAO (2011). Global Food Losses and Food Waste. Extent Causes and Prevention. Rome: Food and Agriculture Organisation of the United Nations (FAO). Available online at: http://www.fao.org/docrep/014/mb060e/mb060e00.htm (accessed October 11, 2021).
FAO (2013). Food Balance Sheets. Rome: Food and Agriculture Organisation of the United Nations (FAO). Available online at: http://faostat3.fao.org/download/FB/FBS/E (accessed October 11, 2021)
FAO (2015). Pérdidas y desperdicios de alimentos en América Latina y el Caribe. Oficina regional de la FAO para América Latina y el Caribe. Boletín, 2–31. Rome: Food and Agriculture Organisation of the United Nations (FAO). Available online at: https://www.tec.ac.cr/sites/default/files/media/doc/perdidas_y_desperdicios_de_alimenticios_en_america_latina_y_caribe.pdf (accessed October 11, 2021).
FAO (2017). Pérdidas y desperdicios de alimentos en América Latina y el Caribe. Oficina regional de la FAO para América Latina y el Caribe. Boletín 4. Rome: Food and Agriculture Organisation of the United Nations (FAO). Available online at: https://www.fao.org/publications/card/es/c/64d64217-88c3-450e-88fa-e670d5b19e2c/ (accessed October 11, 2021).
FAO (2019). The State of Food and Agriculture. Moving Forward on Food Loss and Waste Reduction. Rome. Licence: CC BY-NC-SA 3.0 IGO. Retrieved from: http://www.fao.org/3/ca6030en/ca6030en.pdf
FAO (2020). Nueva Enfermedad por Coronavirus (COVID-19). Preguntas frecuentes: Pandemia Del COVID-19, su Impacto en la Alimentación y la Agricultura. Available online at: shar.es/aHRYip
Gardner, B., and Abraham, C. (2010). Going green? Modelling the impact of environmental concerns and perceptions of transportation alternatives on decisions to drive. J. Appl. Psychol. 40, 813–849. doi: 10.1111/j.1559-1816.2010.00600.x
Garfin, D. R., Thompson, R. R., and Holman, E. A. (2018). Acute stress and subsequent health outcomes: a systematic review. J Psychosom Res. 112, 107–113. doi: 10.1016/j.jpsychores.2018.05.017
Geng, M. (2006). A Comparison of Logistic Regression to Random Forests for Exploring Differences in Risk Factors Associated with Stage Atdiagnosis Between Black and White Colon Cancer Patients. Master's Thesis, University of Pittsburgh. Retrieved from: http://d-scholarship.pitt.edu/id/eprint/7034
Geurts, P., Ernst, D., and Wehenkel, L. (2006). Extremely randomized trees. Mach. Learn. 63, 3–42. doi: 10.1007/s10994-006-6226-1
Grace-Martin, K. (2009). Likert Scale Items as Predictor Variables in Regression. Retrieved from: https://www.theanalysisfactor.com/likert-scale-items-as-predictor-variables-in-regression/
Grace-Martin, K. (2019). How to Decide Between Multinomial and Ordinal Logistic Regression Models. Retrieved from: https://www.theanalysisfactor.com/decide-between-multinomial-and-ordinal-logistic-regression-models/
Graham-Rowe, E., Jessop, D., and Sparks, P. (2015). Predicting household food waste reduction using an extended theory of planned behaviour. Resour. Conserv. Recycl. 101, 194–202. doi: 10.1016/j.resconrec.2015.05.020
Graham-Rowe, E., Jessop, D. C., and Sparks, P. (2014). Identifying motivations and barriers to minimising household food waste. Resour. Conserv. Recycl. 84, 15–23. doi: 10.1016/j.resconrec.2013.12.005
Griffin, M., Sobal, J., and Lyson, T. A. (2009). An analysis of a community food waste stream. Agric. Hum. Values 26, 67–81. doi: 10.1007/s10460-008-9178-1
Gustavsson, J., Cederberg, C., Sonesson, U., van Otterdijk, R., and Meybeck, A. (2011). Global Food Losses and Food Waste: Extent, Causes and Prevention. Rome: Food and Agriculture Organisation of the United Nations (FAO). Available online at: https://www.fao.org/3/i2697e/i2697e.pdf (accessed October 11, 2021)
Hines, J. M., Hungerford, H. R., and Tomera, A. N. (1987). Analysis and synthesis of research on responsible environmental behavior: a meta-analysis. J. Environ. Educ. 18, 1–8. doi: 10.1080/00958964.1987.9943482
Hornibrook, S. A., McCarthy, M., and Fearne, A. (2005). Consumers' perception of risk: the case of beef purchases in Irish supermarkets. Int. J. Retail. Distrib. 33, 701–715. doi: 10.1108/09590550510622263
Jagau, H. L., and Vyrastekova, J. (2017). Behavioral approach to food waste: an experiment. Br. Food J. 119, 882–894. doi: 10.1108/BFJ-05-2016-0213
Koivupuro, H.-K., Hartikainen, H., Silvennoinen, K., Katajajuuri, J.-M., Heikintalo, N., Reinikainen, A., et al. (2012). Influence of socio-demographical, behavioural and attitudinal factors on the amount of avoidable food waste generated in Finnish households. Int. J. Consum. Stud. 36, 183–191. doi: 10.1111/j.1470-6431.2011.01080.x
Krumpal, I. (2013). Determinants of social desirability bias in sensitive surveys: a literature review. Qual. Quant. 47, 2025–2047. doi: 10.1007/s11135-011-9640-9
Lanfranchi, M., Calabro, G., De Pascale, A., Fazio, A., and Gianneto, C. (2016). Household food waste and eating behavior: empirical survey. Br. Food J. 118, 3059–3072. doi: 10.1108/BFJ-01-2016-0001
Lau, J. T. F., Tsui, H. Y., and Kim, J. H. (2006). Perceptions about status and modes of H5N1 transmission and associations with immediate behavioural responses in the Hong Konggeneral population. Prev. Med. 43, 406–410. doi: 10.1016/j.ypmed.2006.06.007
Leadbeater, C. (2019). Ordinal vs. Multinomial. Does Model Selection Make a Difference? Paper 215-2019. Retrieved from: https://www.lexjansen.com/wuss/2019/215_Final_Paper_PDF.pdf
Lee, J. W., Lee, J. B., Park, M., and Song, S. H. (2005). An extensive comparison of recent classification tools applied to microarray data. Comput. Stat. Data Anal. 48, 869–885. doi: 10.1016/j.csda.2004.03.017
Leppin, A., and Aro, A. R. (2009). Risk perceptions related to SARS and avian influenza: theoretical foundations of current empirical research. Int. J. Behav. Med. 16, 7–29. doi: 10.1007/s12529-008-9002-8
Liaw, A., and Wiener, M. (2002). Classification and regression by randomForest. R News. 2, 18–22. Available online at: http://cogns.northwestern.edu/cbmg/LiawAndWiener2002.pdf
Lima, C. K. T., Medeiros, P., Silva, I., Oliveira, J., Steves, J., De Souza, R., et al. (2020). The emotional impact of coronavirus 2019-nCoV. Psychiatry Res. 287:112915. doi: 10.1016/j.psychres.2020.112915
Lindell, M. K., and Perry, R. W. (2012). The protective action decision model: theoretical modifications and additional evidence. Risk Anal. 32, 616–632. doi: 10.1111/j.1539-6924.2011.01647.x
Llaugel, F. A., and Fernández, A. I. (2011). Evaluación del uso de modelos de regresión logística para el diagnóstico de instituciones financieras. Ciencia Soc. 36, 590–627. doi: 10.22206/cys.2011.v36i4.pp590-627
Lobb, A. E., Mazzocchi, M., and Traill, W. B. (2007). Modelling risk perception and trust in food safety information within the theory of planned behaviour. Food Qual. Prefer. 18, 384–395. doi: 10.1016/j.foodqual.2006.04.004
Loewenstein, G. (1996). Out of control: visceral influences on behavior. Org. Behav. Hum. Decis. Process. 65, 272–292. doi: 10.1006/obhd.1996.0028
Long, J. S. (1997), Regresión Models for Categorical and Limited Dependent Variables. Thousand Oaks, CA: Sage Publications.
Lyndhurst, B., Cox, J., and Downing, P. (2007). Food Behaviour Consumer Research: Quantitative Phase. Banbury: Waste and Resources Action Programme (WRAP).
Mallinson, L. J., Russell, J. M., and Barker, M. E. (2016). Attitudes and behaviour towards convenience food and food waste in the United Kingdom. Appetite 103, 17–28. doi: 10.1016/j.appet.2016.03.017
McCarthy, B., and Liu, H. B. (2017). Food waste and the ‘green’ consumer. Austr. Mark. J. (2017) 25, 126–132. doi: 10.1016/j.ausmj.2017.04.007
Mejía, L. (2020). Actualización de Las Perspectivas Económicas 2020-2021. Retrieved from: http://dams.fedesarrollo.org.co/tendenciaeconomica/publicaciones/208/
Neff, R.A., Spiker, M. L., and Truant, P. L. (2015). Wasted food: US Consumers reported awareness, attitudes, and behaviors. PLoS One 10:e0127881. doi: 10.1371/journal.pone.0127881
Nicola, M., Alsafi, Z., Sohrabi, C., Kerwan, A., Al-Jabir, A., Iosifidis, C., et al. (2020). The socio-economic implications of the coronavirus pandemic (COVID-19): a review. Int. J. Surg. 78, 185–193. doi: 10.1016/j.ijsu.2020.04.018
Olsen, N. V., Sijtsema, S. J., and Hall, G. (2010). Predicting consumers' intention to consume ready-to-eat meals. The role of moral attitude. Appetite 55, 534–539. doi: 10.1016/j.appet.2010.08.016
Pappalardo, G., Cerroni, S., Nayga, R. M. Jr., and Yang, W. (2020). Impact of Covid-19 on Household Food Waste: the Case of Italy. Front. Nutr. 7:585090. doi: 10.3389/fnut.2020.585090
Parizeau, K., von Massow, M., and Martin, R. (2015). Household-level dynamics of food waste production and related beliefs, attitudes, and behaviors in Guelph, Ontario. Waste Manag. 35, 207–217. doi: 10.1016/j.wasman.2014.09.019
Pedhazur, E. J. (1997). Multiple Regression in Behavioral Research: Explanation and Prediction, 3rd Edn. New York, NY: Harcourt Brace.
Pedrozo, J. C., Pedrozo, M. J., and Campo-Arias, A. (2020). Pandemic-Related Perceived Stress Scale of COVID-19: an exploration of online psychometric performance. Rev. Colomb. Psiquiatr. 49, 229–230. doi: 10.1016/j.rcp.2020.05.005
Pennings, J. M. E., Wansink, B., and Meulenberg, M. T. G. (2002). A note on modeling consumer reactions to a crisis: the case of the mad cow disease. Int. J. Res. Mark. 19, 91–100. doi: 10.1016/S0167-8116(02)00050-2
Principato, L., Secondi, L., and Pratesi, C. A. (2015). Reducing food waste: an investigation on the behaviour of Italian youths. Br. Food J. 117, 731–748. doi: 10.1108/BFJ-10-2013-0314
Quested, T. E., Marsh, E., Stunell, D., and Parry, A. D. (2013). Spaghetti soup: the complex world of food waste behaviors. Resour. Conserv. Recycl. 79, 43–51. doi: 10.1016/j.resconrec.2013.04.011
Quested, T. E., Parry, A. D., Easteal, S., and Swannell, R. (2011). Food and drink waste from households in the UK. Nutr. Bull. 36, 460e467. doi: 10.1111/j.1467-3010.2011.01924.x
Quinn, I. (2020). What's Happened to Food Waste in a Covid-19 World? Retrieved from: https://www.thegrocer.co.uk/food-waste/whats-happened-to-food-waste-in-a-covid-19-world/649031.article
Rivis, A., Sheeran, P., and Armitage, C. J. (2009). Expanding the affective and normative components of the theory of planned behavior: a meta-analysis of anticipated affect and moral norms. J. Appl. Soc. Psychol. 39, 2985–3019. doi: 10.1111/j.1559-1816.2009.00558.x
Rodgers, R. F., Lombardo, C., Cerolini, S., Franko, D. L., Omori, M., Linardon, J., et al. (2021). Waste not and stay at home - evidence of decreased food waste during the COVID-19 pandemic from the U.S. and Italy. Appetite 160:105110. doi: 10.1016/j.appet.2021.105110
RTVE. (2021). Muertes por COVID-19 en el Mundo:¿Qué País Cuenta Con Más Fallecidos por Habitante? Retreived from: https://www.rtve.es/noticias/20210604/paises-muertos-coronavirus-poblacion/2012350.shtml
Ruiz-Roso, M. B., Padilha, P. d. C, Mantilla-Escalante, D. C., Ulloa, N., Brun, P., et al. (2020). Covid-19 confinement and changes of adolescent's dietary trends in Italy, Spain, Chile, Colombia, and Brazil. Nutrients 12:1807. doi: 10.3390/nu12061807
Schmidt, K. (2016). Explaining and promoting household food waste-prevention by an environmental psychological based intervention study. Resour. Conserv. Recycl. 111, 53–66. doi: 10.1016/j.resconrec.2016.04.006
Setti, M., Banchelli, F., Falasconi, L., Segrè, A., and Vittuari, M. (2018). Consumers' food cycle and household waste. When behaviors matter. J. Clean. Prod. 185, 1–1032. doi: 10.1016/j.jclepro.2018.03.024
Setti, M., Falasconi, L., Segrè, A., Cusano, I., and Vittuari, M. (2016). Italian consumers' income and food waste behaviour. Br. Food J. 118, 1731–1746. doi: 10.1108/BFJ-11-2015-0427
Sheen, F., Hardman, C. A., and Robinson, E. (2020). Food waste concerns, eating behaviour and body weight. Appetite 151:104692. doi: 10.1016/j.appet.2020.104692
Sheeran, P. (2002). “Intention behavior relations: a conceptual and empirical review, in European Review of Social Psychology, eds. W. Stroebe, and M. Hewstone (Chichester: Wiley), 1–36.
Sparks, P., Hinds, J., Curnock, S., and Pavey, L. J. (2014). Connectedness and its consequences: a study of relationships with the natural environment. J. Appl. Soc. Psychol. 44, 166–174. doi: 10.1111/jasp.12206
Stancu, V., Haugaard, P., and Lähteenmäki, L. (2015). Determinants of consumer food waste behaviour: two routes to food waste. Appetite 96, 7–17. doi: 10.1016/j.appet.2015.08.025
STATISTA. (2021a). Annual per capita household food waste of selected countries worldwide as of 2020. Retrieved from https://www.statista.com/statistics/933059/per-capita-food-waste-of-selected-countries/
STATISTA. (2021b). Número de casos confirmados de coronavirus en el mundo a fecha de 10 de septiembre de 2021 por País. Retreived from: https://es.statista.com/estadisticas/1091192/paises-afectados-por-el-coronavirus-de-wuhan-segun-los-casos-confirmados/
Stefan, V., van Herpen, E., Tudoran, A. A., and Lähteenmäki, L. (2013). Avoiding food waste by Romanian consumers: the importance of planning and shopping routines. Food Qual. Prefer. 28, 375–381. doi: 10.1016/j.foodqual.2012.11.001
Stefani, G., Cavicchi, A., Romano, D., and Lobb, A. E. (2008). Determinants of intention to purchase chicken in Italy: the role of consumer risk perception and trust in different information sources. Agribusiness 24, 523–537. doi: 10.1002/agr.20177
Steyerberg, E. W., Vickers, A. J., Cook, N. R., Gerds, T., Gonen, M., Obuchowski, N., et al. (2010). Assessing the performance of prediction models: a framework for some traditional and novel measures. Epidemiology 21:128. doi: 10.1097/EDE.0b013e3181c30fb2
Strobl, C., Boulesteix, A.-L., Zeileis, A., and Hothorn, T. (2007). Bias in random forest variable importance measures: Illustrations, sources and a solution. BMC Bioinf. 8:25. doi: 10.1186/1471-2105-8-25
Taylor, S., and Todd, P. (1995). An integrated model of waste management behavior. A test of household recycling and composting intentions. Environ. Behav. 27, 603–630. doi: 10.1177/0013916595275001
Thyberg, K. L., and Tonjes, D. J. (2016). Drivers of food waste and their implications for sustainable policy development. Resour. Conserv. Recycl. 106, 110–123. doi: 10.1016/j.resconrec.2015.11.016
Trejos-Herrera, A. M., Vinaccia, S., and Bahamón, M. J. (2020). Coronavirus in Colombia: Stigma and quarantine. J. Glob. Health 10:020372. doi: 10.7189/jogh.10.020372
UN. (2015). The 17 Goals. New York, NY: United Nations - Department of Economic and Social Affairs, Sustainable Development. Available online at: https://sdgs.un.org/goals
UN. (2020a). Businesses Must Address Gaps in the Quality of Sustainability Reporting. Retrieved from: https://unstats.un.org/sdgs/report/2020/goal-12/
UN. (2020b). Countries Now Need to Operationalize the Principles of Sustainable Economic Growth. Retrieved from: https://unstats.un.org/sdgs/report/2020/goal-12/
UNDP. (2021). One Year of Pandemic: Socioeconomic Impact of COVID-19 in Colombia. Retrieved from: https://www.co.undp.org/content/colombia/es/home/-sabias-que-/un-ano-de-pandemia–impacto-socioeconomico-de-la-covid-19-en-col.html
Verplanken, B., Aarts, H., van Knippenberg, A., and Moonen, A. (1998). Habit versus planned behaviour: a field experiment. Br. J. Soc. Psychol. 37, 111–128. doi: 10.1111/j.2044-8309.1998.tb01160.x
Vidal-Mones, B., Barco, H., Diaz-Ruiz, R., and Fernandez-Zamudio, M.-A. (2021). Citizens' food habit behavior and food waste consequences during the first COVID-19 Lockdown in Spain. Sustainability 13:3381. doi: 10.3390/su13063381
Visschers, V. H., Wickli, N., and Siegrist, M. (2016). Sorting out food waste behaviour: a survey on the motivators and barriers of self-reported amounts of food waste in households. J. Environ. Psychol. 45, 66–78. doi: 10.1016/j.jenvp.2015.11.007
Wang, H. H., and Hao, N. (2020). Panic buying? Food hoarding during the pandemic period with city lockdown. J. Integr. Agric. 19, 2916–2925. doi: 10.1016/S2095-3119(20)63448-7
Watson, M., and Meah, A. (2012). Food, waste and safety: negotiating conflicting social anxieties into the practices of domestic provisioning. Sociol. Rev. 60, 102–120. doi: 10.1111/1467-954X.12040
WHO. (2020). WHO Director-General's Opening Remarks at the Media Briefing on COVID-19 - 11 March 2020. Available online at: bit.ly/2B6xj0w
Williams, H., Wilkström, F., Otterbring, T., Löfgren, M., and Gustafsson, A. (2012). Reasons for household food waste with special attention to packaging. J. Clean. Prod. 24, 141–148. doi: 10.1016/j.jclepro.2011.11.044
Wordometers. (2021). Coronavirus - Colombia. Retrieved from: https://www.worldometers.info/coronavirus/country/colombia/ (accessed September 28, 2021).
World Bank. (2020). COVID-19 (coronavirus) Plunges the World Economy Into the Worst Recession Since World War II. Retrieved from: https://www.bancomundial.org/es/news/press-release/2020/06/08/covid-19-to-plunge-global-economy-into-worst-recession-since-world-war-ii (Press Release June 08, 2020).
Yeung, R. M., and Morris, J. (2001). Food safety risk: consumer perception and purchase behaviour. Br. Food J. 103, 170–187. doi: 10.1108/00070700110386728
Yousefi, M. R., Hua, J., Sima, C., and Dougherty, E. R. (2010). Reporting bias when using real data sets to analyze classification performance. Bioinformatics 26, 68–76. doi: 10.1093/bioinformatics/btp605
Zhang, Y., Yang, H., Cheng, P., and Luqman, A. (2019). Predicting consumers' intention to consume poultry during an H7N9 emergency: an extension of the theory of planned behavior model. Hum. Ecol. Risk Assess. 26, 190–211. doi: 10.1080/10807039.2018.1503931
ANNEX
Keywords: COVID-19, food waste, pandemic, consumer behavior, logistic regression, random forest
Citation: Mejia D, Diaz M, Charry A, Enciso K, Ramírez O and Burkart S (2021) “Stay at Home”: The Effects of the COVID-19 Lockdown on Household Food Waste in Colombia. Front. Psychol. 12:764715. doi: 10.3389/fpsyg.2021.764715
Received: 25 August 2021; Accepted: 30 September 2021;
Published: 28 October 2021.
Edited by:
Boris C. Rodríguez-Martín, Proyecto PlaniFive, SpainReviewed by:
Yunier Broche-Pérez, Universidad Central Marta Abreu de Las Villas, CubaNathaniel James Siebert Ashby, Technion Israel Institute of Technology, Israel
Copyright © 2021 Mejia, Diaz, Charry, Enciso, Ramírez and Burkart. This is an open-access article distributed under the terms of the Creative Commons Attribution License (CC BY). The use, distribution or reproduction in other forums is permitted, provided the original author(s) and the copyright owner(s) are credited and that the original publication in this journal is cited, in accordance with accepted academic practice. No use, distribution or reproduction is permitted which does not comply with these terms.
*Correspondence: Stefan Burkart, cy5idXJrYXJ0QGNnaWFyLm9yZw==