- 1Department of Management and Information Systems, College of Business Administration, University of Hail, Ha'il, Saudi Arabia
- 2Ahmed Bin Mohammed Military College, Al Rayyan, Qatar
Innovation resistance research remains in its early stages. Efforts to define and comprehend consumer resistance to innovation necessitate in-depth studies that consider the contextual factors of resistance to innovation. To address this challenge, this research explored consumer resistance to innovation in a low-income population, namely, university students on financial support. The innovation under this study is the productivity applications provided for free by the University of Hail, Saudi Arabia, to all students. This study explores variables such as value barrier, risk barrier, tradition barrier, and image barrier and how they impact consumer resistance to innovation in a low-income population. We extend the theory by investigating the moderating roles of consumer characteristics (motivation, self-efficacy, emotion, and attitude toward existing products). The current study is based on an online survey of low-income students at a Saudi Arabian University; 258 cases were gathered. We found that all the direct effects of the variables (i.e., Value Barrier, Risk Barrier, Tradition Barrier, and Image Barrier) were positively related to consumer resistance to innovation. Importantly, consumer characteristics significantly moderate this relationship, as the relationship is stronger when the measurements for consumer characteristics are high.
Introduction
Companies and governments around the world invest billions of dollars in research and development to produce innovative products and services (Pérez-Luño et al., 2019). However, the question remains: What happens when consumers resist these innovations? The result is that significant investments are lost. Therefore, it is vitally important to research and understand why and how consumers resist innovations and how to design products and services that consumers will not resist. In this pursuit, an emerging research field called consumer resistance to innovation has been developed to investigate the factors that cause such resistance (Ram and Sheth, 1989; Fattah et al., 2021). This study investigates barriers such as value, risk, tradition, and image, and the moderating role of consumer characteristics in resistance to innovation (Pelau et al., 2021).
Ram and Sheth (1989) established the resistance to innovation model, which is based on the characteristics of consumers as well as innovation, and explained why consumers are unable to adopt innovations. Moreover, Ram and Sheth (1989) found that consumers are resistant to innovation because of difficulties in implementing change, together with the ensuing arguments. For consumers, these issues can be divided into psychological and practical barriers. Individuals are unable to accept novel concepts and products because of psychological obstacles, such as image and tradition barriers (Naveed et al., 2022; Zhang et al., 2022). Similarly, a consumer's belief system is often the source of psychological barriers (Ram and Sheth, 1989). Furthermore, other aspects, such as motivation, personality, perception, behavior, attitude, prior experiences with innovations, norms, and users' belief systems, can all be sources of psychological barriers (Ghazali et al., 2022; Li et al., 2022).
With the development of these variables, research on consumer resistance to innovation has proliferated. Researchers have examined a wide variety of innovations in different settings and among different population segments (see Talwar et al., 2020). In their extensive review of the literature, Talwar et al. (2020) found that digital innovations remain understudied in terms of consumer resistance to innovation. Additionally, while the research on this topic spans a wide variety of populations, low-income populations are largely overlooked. This issue of resistance to innovation in low-income populations, therefore, points researchers in this new direction. This study investigates resistance to digital innovation (office productivity applications) among a particular low-income population, namely, university students on financial support.
Little is known about the key variables that impact consumer resistance to innovation, especially in low-income populations. This study aims to analyze the most relevant aspects that affect low-income consumers' resistance to innovation (Uzir et al., 2020). The research focused on examining the link between functional and psychological barriers and consumer resistance to innovation and how consumer characteristics might moderate this relationship (Citrin et al., 2000; Ho and Wu, 2011; Kunz et al., 2011; Chao et al., 2012). Baron and Kenny (1986) proposed using the moderator variable if the associations between the dependent and independent variables were not sufficiently established as either inconsistent/insignificant or consistent/significant. In this regard, consumer characteristics were used as moderators.
The study focused on a particular low-income population, namely, undergraduate university students on financial support in a Saudi Arabian University. Undergraduate students on financial support receive monthly stipends from the government to help them with their university and life expenses. This fact characterizes them as a low-income population. Additionally, the study focused on a specific digital innovation, namely, productivity applications that aim to help students manage their time and tasks in an efficient and streamlined manner. These applications are provided for free by the university to all students to help them manage their studies effectively (Alnoor et al., 2022).
The paper is structured as follows. First, we explore the literature on resistance to innovation in greater depth to develop our hypothesis. Then, the research framework is presented, along with the study's hypotheses. After an exposition of the study's research methodology, the results are presented, followed by a discussion that explains the contributions of the study. The paper concludes with a section that summarizes its results, identifies its limitations, and puts forth future research opportunities.
Literature review
Consumer resistance to innovation
Innovation resistance research is an important field of study for understanding the reasons behind consumers' rejection of technology and, hence, the failure of such technological artifacts. In this pursuit, researchers have sought to investigate and explore the myriad variables that might, in some form or other, lead consumers to resist an innovation (Cornescu and Adam, 2013; Fattah et al., 2021). For example, Mohtar et al. (2015) argued that personal beliefs and cultural norms play a vital role in consumer resistance to innovation. Similarly, Cornescu and Adam (2013) argued that resistance attitudes play a key role in fostering resistance to innovation. It is also important to consider changes in consumption patterns and how they affect consumer resistance to innovation (Gatignon and Robertson, 1991). Such changes in consumption patterns include, but are not limited to, changes in lifestyle and standards of living (Watson, 1971; Zaltman and Duncan, 1977). Additionally, it has been noted that consumers' excitement toward an innovation plays a key role in whether they would resist an innovation (Gold, 1981; Murdock and Franz, 1983; Salerno, 1985). In other words, the lack of excitement toward innovation can be reinterpreted as a form of resistance to that innovation (O'Connor et al., 1990; Ellen et al., 1991).
Few studies have addressed innovation resistance with regard to digital services uptake (Brehm, 1966; Mohtar and Abbas, 2015). Stressing consumer resistance is critical because consumer perception of products plays a vital role in resistance to innovation. As a result, consumer resistance to innovation is crucial because of its positive and negative repercussions, such as the adoption of innovations or the lack thereof (Mohtar et al., 2015; Yu et al., 2015; Andronie et al., 2021). Furthermore, while few studies on consumer resistance to innovation (e.g., Leonard, 2004; Mohtar and Abbas, 2015) have specified factors that positively affected such resistance, some factors remain unexplored (Hoong et al., 2019; Al Halbusi et al., 2022). As a result, to fill a gap in the knowledge, this study aimed to identify the most important determinants of consumer resistance to innovation, with specific attention to low-income populations. In this pursuit, there are few studies that illustrate the relationship between consumer barrier variables (i.e., value barrier, risk barrier, tradition barrier, and image barrier) and consumer resistance to innovation.
Conversely, the degree of consumer resistance is determined by how consumers perceive innovation. The most important component of innovation resistance is the consumer's characteristics and attributes. Variety seekers or innovators like inventing for the sake of new experiences (Barbu et al., 2021); therefore, they are less resistant to new items. Self-efficacy, for example, is a personality feature that influences how consumers react to new products. In the case of innovations, for example, this cannot be verified prior to purchase. A consumer with poor self-efficacy would be more likely to wait until the product's performance has been satisfactorily shown. As a result, consumer resistance to innovation is inversely correlated with self-efficacy (Rokeach, 1973; Hassan et al., 2020).
Motivation is one of the predictors or drivers of consumer resistance to innovation. Consumer buying behavior that is dependent and shaped by ingrained “habits” (Sheth, 1981) is known to cause resistance to innovation. If consumers are content with their current routine and the innovation threatens to disrupt that routine, they are likely to reject the change. As a result, consumer resistance to innovation has a negative association with motivation. Similarly, resistance is influenced by the consumer's receptive attitude toward innovation. Consumers' positive attitudes regarding existing innovation lead to increased resistance toward proposed or new innovative products or technologies (Al Halbusi et al., 2021). If consumers do not feel the need for innovation, they are likely to oppose it (Davis et al., 1992; Lee et al., 2007). Furthermore, resistance to innovation is likely if the consumer's impression of the invention remains positive both before and after it is implemented.
Risk, use, and value barriers, often known as functional barriers, prevent people from embracing new ideas. These functional barriers may develop, for example, if consumers notice major changes as a result of adopting an innovative product (Ram and Sheth, 1989). In addition, resistance to innovation theory incorporates innovative features, such as relative advantage, better product adoption, complexity, and perceived risk, as elements affecting technology acceptance or key grounds for rejection. Likewise, the degree of consumer resistance is determined by the characteristics of innovation as perceived by consumers. Ram and Sheth (1989) also supported Rogers's (2003) assertion that innovation features, such as comparative advantages over an innovation, may be seen from the standpoint of economic benefits or cost savings. In addition to increasing value, innovation may offer superior performance at comparatively cheap prices. Consumers are more likely to oppose innovation if it has a poor competitive advantage over already accessible substitutes. Furthermore, perceived risk is linked to innovation uptake. The perceived amount of risk varies depending on the type of innovation. Consumers regard continual or small improvements as having a lower perceived risk (Gatignon and Robertson, 1991).
Intermittent or large innovations, on the other hand, pose a danger of disrupting consumer habitual behavior; the bigger the perceived risk, the greater the resistance to innovation. The degree to which the invention is seen as somewhat difficult to understand and operate is another aspect of innovation. It might be complicated or tough to be an early adopter or prospective early adopter of some inventions or fresh concepts. Because the phrase adoption rate implies that innovation can be adopted or rejected, the price of the innovation can also be a predictor of the rate of adoption (Abbas et al., 2017). This means that when the cost of new items is high, the rate of adoption falls, thus increasing consumer resistance to innovation (Rogers et al., 2014). Consumers' inventiveness is also based on innovation theory. According to Rogers and Shoemaker (1971), consumer innovativeness is defined as the degree to which consumers are early adopters of innovation compared to their social group. Consumers with a high level of innovativeness are defined by Blackwell et al. (2006) as being ready to make changes in goods and ideas (see also Bartels and Reinders, 2011).
As a result, Ram and Sheth (1989) proposed that rejection is the most powerful type of consumer resistance to innovation, as opposed to other results, such as postponement and delay, which are primarily influenced by situational or innovation variables. For instance, product perceived difficulties influence whether an innovation is adopted or rejected. Furthermore, Yadav and Varadarajan (2005) found that consumer rejection is a critical predictor of resistance to innovation by consumers. In this study, we examined the hurdles that may cause resistance to innovation. There were a variety of barriers to consider; however, the research concentrated on the barriers of value, risk, tradition, and image. In addition, the research examined the moderating role. Furthermore, consumer characteristics, such as motivation, self-efficacy, emotion, and attitude, were investigated as moderators.
Research model and hypothesis development
Value barriers
Value barriers stem from a mismatch with preexisting values, especially with regard to weighing the cost of adoption and innovation against the invention's benefits (Aggarwal and Prasad, 1998; Lian and Yen, 2013). To possess low-value barriers, innovations must deliver greater value to users in exchange for their labor in learning and adapting to such systems (Kliestik et al., 2022).
User behavior influences the resistance, adoption, and usage of innovations. Most previous research suggests that there is a negative association between value barriers and user intentions in diverse settings, such as shopping online (Lian and Yen, 2013; Uzir et al., 2021a), mobile shopping (Moorthy et al., 2017), and mobile services (Joachim et al., 2018). User resistance to m-banking (Yu and Chantatub, 2015) and e-tourism has also been connected to value barriers (Jansukpum and Kettem, 2015). For instance, Sivathanu (2018) observed a positive correlation between value barriers and user resistance.
Hypothesis 1: Value barriers have a positive effect on consumer resistance to innovation.
Risk barriers
Risk barriers relate to the resistance that occurs due to uncertainties, which are an unavoidable part of every innovative product. Dunphy and Herbig (1995) and Aldás-Manzano et al. (2009) argued that an innovation's acceptance is determined by the degree of uncertainty it causes. Ram and Sheth (1989) identified four categories of risk: physical, economic, functional, and social. With respect to mobile banking, for instance, users may be at risk of fraud, money loss, poor Internet access, or dwindling smartphone battery life. Risk barriers have already been found to have an adverse influence on users' intentions and behavior in the research. Risk barriers, for example, have a negative effect on usage intention in a multitude of fields, such as commerce conducted through mobile phones (Al-Jabri and Sohail, 2012; Moorthy et al., 2017), shopping using the internet (Lian and Yen, 2013; Lian et al., 2013), using mobile phone applications to shop for new products (Gupta and Arora, 2017), m-banking as a medium for banking service delivery (Laukkanen, 2016), and mobile gaming (Moorthy et al., 2017). In other words, when risk barriers become high, they result in unfavorable user behaviors, such as resistance. Therefore, prior research has revealed that resistance to various digitization programs, such as m-banking (Yu and Chantatub, 2015) and e-tourism, results in greater risk barriers (Jansukpum and Kettem, 2015).
Risk barriers may become prospective hurdles to mobile online applications' acceptance, usage, and plans to recommend them as a result of the uncertainties they bring. Concerns about safety, confidentiality, and trust abound with mobile applications (Marett et al., 2015; Uzir et al., 2021b; Hassan et al., 2022). Two such concerns are the loss of sensitive information and the creation of security breaches (Ediriweera and Wiewiora, 2021). According to the literature, risk barriers have a negative correlation with a user's intention to utilize and embrace digital services (El-Haddadeh, 2020). A lack of knowledge of the security and privacy consequences of digitalized services among potential and current users might lead to the establishment of barriers related to risk (Luo et al., 2010; Ullah et al., 2021).
Hypothesis 2: Risk barriers have a positive effect on consumer resistance to innovation.
Tradition barriers
Any product or service's success is generally influenced by traditions that guide a user's behavior. Scholars say that traditions are profoundly embedded in the lives of people and that any potential conflict with them results in a significant consumer reaction in the form of unfavorable public shaming and ostracization (Kaur et al., 2020). The impediments that any innovation produces when it disturbs a user's established habit, culture, or behavior are known as tradition barriers (Kumar et al., 2022). Adoption hopes for any new breakthrough are also hampered by traditional restrictions (Antioco and Kleijnen, 2010).
Tradition barriers are linked to mobile application resistance (Jansukpum and Kettem, 2015; Yu et al., 2015). The adoption of mobile payments in India, for instance, has resulted in a significant shift in how consumers make payments. Traditionally, consumers used cash to make payments, but today, they utilize mobile devices to make cashless purchases (Patil et al., 2020). Furthermore, a previous study looking at the adoption of mobile payment applications in India immediately after the demonetization crisis in November 2016 discovered that user resistance to such applications is linked to tradition barriers (Sivathanu, 2018; Mishra et al., 2022).
Hypothesis 3: Tradition barriers have a positive effect on consumer resistance to innovation.
Image barriers
Image barriers refer to the identity associated with an innovation. Such identities might stem from different sources, such as the innovation's country of manufacture, the cultural logic that surrounds a specific innovation, or even the way it is marketed and communicated to the public (Ram and Sheth, 1989). Image barriers are used to combat a negative view of innovation as a result of the presumed amount of complexity associated with its use (Lian and Yen, 2013). Consumers, for example, rarely consider mobile applications secure, resulting in a poor image (Kaur et al., 2020). According to a prior study, when it comes to numerous digitization activities, image is a barrier that has a negative influence on consumers' behavior. For example, image is adversely connected with users' adoption-related intentions toward mobile applications (Laukkanen, 2016; Joachim et al., 2018). Furthermore, client resistance to mobile banking is caused by image barriers (Yu and Chantatub, 2015).
Hypothesis 4: Image barriers have a positive effect on consumer resistance to innovation.
Consumer characteristics
It is critical to assess the impact of the characteristics of both the consumer and the innovation on consumer resistance to innovation because these characteristics have been proven to be determining factors for such resistance (Tornatzky and Klein, 1982; Gatignon and Robertson, 1991; Veryzer, 1998). Additionally, it has been shown that resistance to innovation is dependent on the consumer in that it changes from consumer to consumer, further buttressing the importance of studying consumer characteristics (Ram and Sheth, 1989). The same can also be said about the innovation itself, in that the characteristics of the innovation affect whether consumers resist it (Barczak et al., 1997; Wang et al., 2003).
Hypothesis 5: Consumer characteristics moderate the relationship between value barriers and consumer resistance to innovation, such that the relationship is stronger when the measurements for consumer characteristics are high.
Hypothesis 6: Consumer characteristics moderate the relationship between risk barriers and consumer resistance to innovation, such that the relationship is stronger when consumer characteristics are high.
Hypothesis 7: Consumer characteristics moderate the relationship between traditional barriers and consumer resistance to innovation, such that the relationship is stronger when consumer characteristics are high.
Hypothesis 8: Consumer characteristics moderate the relationship between image barriers and consumer resistance to innovation, such that the relationship is stronger when consumer characteristics are high.
Methods, sample size, and procedure
The respondents for this study were students on financial support studying at the College of Business Administration, University of Hail. The total population size was 1,800 low-income students. With respect to specifying an appropriate sample that could yield reliable results for the study, we chose this particular sample, as most students were taking courses related to business and innovation and were receiving financial support from the university. Poverty is a consequence of the digital divide, which is the disparity in access to and use of technology and the Internet. Digital resources are more likely to be accessible to the well-educated and affluent, whereas nonwhites and people of lower income are more likely to lack access to them (Kezar et al., 2022). Therefore, a specific digital innovation such as productivity applications in this case aimed at helping students in managing their time and tasks in an efficient and streamlined manner. Thus, they were the best sample for this research.
It was crucial to have a sample size that was precise and adequate. Therefore, Hair et al. (2011) suggested that an appropriate sample size conducive to statistical analysis must be at least 10–20 times greater than the required variables. Before we circulated the survey, the registrar of the college was approached to ask permission. Once permission was granted, the online survey was distributed through emails and WhatsApp groups. Thus, out of 280 distributed questionnaires, 258 responses were returned. Uncompleted responses were excluded, and data from 258 were used for further analysis. This resulted in a response rate of 92%. Among the respondents, 67.4% were men, and 33.56% were women. The age of 60% of the respondents was 18–24 years old, followed by 25–29 (9.2%) and 30–39 (30.8%) years old.
Measurement of the variables
All measures were derived from prior reliable studies. Before the beginning of the data collection stage, the questionnaire was verified by three academic experts in related fields. After the English version was verified, the items were translated from the English version to the Arabic version because the targeted respondents were native Arabic speakers. The translation process was based on the double-blinded principle, where the original English version of the scales was translated into Arabic, and the Arabic version was back-translated by two professional researchers (Brislin, 1970) to assure their validity.
We measured the Value barrier (VB) with 2 items that were slightly adapted from Laukkanen (2016). The Risk barrier (RB) was assessed with 4 items taken from Laukkanen (2016). The Tradition barrier (TB) was measured with 5 items adapted from Laukkanen (2016). To measure the Image barrier (IB), 2 items were slightly adapted from past studies (Laukkanen, 2016). Consumer characteristics were measured with subdimensions involving attitude toward existing products (three items), motivation (four items), social influence (five items), and emotions (six items). These items were taken from Schwartz and Sagiv (1995), Richins (1997), Wang et al. (2003), Walczuch and Lundgren (2004), Agosto and Hughes-Hassell (2005), Reynolds et al. (2006), Park and Chen (2007), and Carayannis et al. (2013). Finally, consumer resistance to innovation was evaluated with seven items adapted from Sheth (1981), Szmigin and Foxall (1998), and Yang (2005). All measures were assessed on a 5-point Likert scale (1 = Strongly Disagree and 5 = Strongly Agree).
Data analysis and results
For several reasons, structural equation modeling (SEM) using partial least squares (PLS) with the Smart PLS 3.3.3 software (Ringle et al., 2005) was used to evaluate the given hypotheses. This thorough, rigorous, and systematic technique is suitable for complex causal analyses, including first- and second-order constructs, and it does not require strict assumptions regarding the underpinning variables (Henseler and Sarstedt, 2013; Hair et al., 2017). Additionally, we used the 5,000-subsample approach to construct bootstrap t-statistics with n-1 degree of freedom to test the significance of the path coefficients (where n is the number of subsamples).
Common method bias
As the independent and dependent variables were gathered using the same questionnaire, the issue of common method bias (CMB) might have occurred. To address this issue, we adopted a two-pronged strategy of procedural and statistical techniques (Podsakoff et al., 2012; Tehseen et al., 2017). On a procedural level, we employed numerous measurement scales in the survey instrument. We also reminded respondents that there were no correct or incorrect responses and that their names would be considered anonymous.
In terms of statistics, we used two methods: Harman's single-factor analysis and the complete collinearity test, depending on Variance Inflation Factors (VIFs). The findings of Harman's single-factor analysis indicated that a single component accounted for just 13.82% of the overall variance. Second, we employed VIFs to conduct a thorough collinearity test (Kock, 2015). (Kock and Lynn, 2012) advised performing such a test to assess both vertical and lateral collinearity. According to (Kock and Lynn, 2012), when the VIF is more than 3.3, it implies pathological collinearity, implying that the model is affected by Common Method Variance (CMV). Nevertheless, as indicated in Table 1, this trial was CMV-free.
Confirmatory factor analysis
Before attempting to utilize a structural model, its properties (item reliability, internal consistency reliability, convergent validity, and discriminant validity) had to be demonstrated (Hair et al., 2017). Table 2 shows that the bulk of the items scored greater than the 0.707 criterion (Hair et al., 2017, 2019). Cronbach's alpha and composite reliability were employed to assess the internal consistency of the constructs. Table 2 shows that both strategies produced good results that were greater than the cutoff of 0.70 (Hair et al., 2017, 2019). In terms of convergent validity, the average variance extracted (AVE) was likewise above the 0.5 criterion (Hair et al., 2017, 2019) (see Table 2).
Two methods were used with regard to discriminant validity: Fornell–Larcker and Heterotrait-Monotrait ratio (HTMT). No issues were revealed using Fornell–Larcker's method. Every construct's AVE was larger than the variance shared by each construct with the other latent variables (Hair et al., 2017) (see Table 3). The HTMT ratio of correlations was based on the Multitrait–Multimethod Matrix (Henseler and Sarstedt, 2013). As a result, when the HTMT value is more than 0.85, there is an issue with discriminant validity based on these criteria (Kline, 2010). Table 4 shows that the HTMT values are all below the threshold of 0.85, thus confirming the discriminant validity of each pair of constructs (Kline, 2010; Henseler and Sarstedt, 2013).
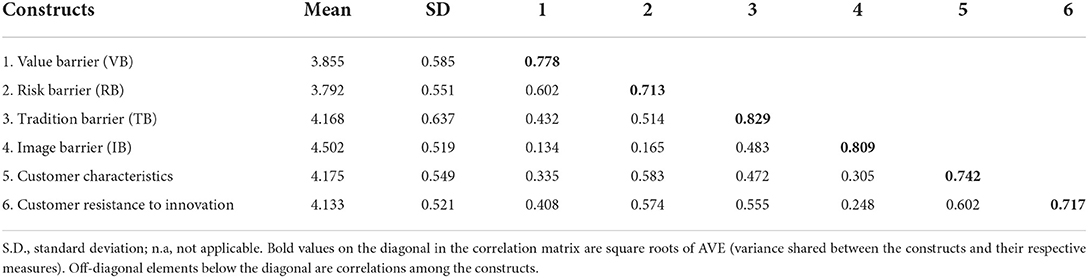
Table 3. Descriptive statistics, correlation matrix, and discriminant validity via Fornell and Larcher.
Structural model: Hypotheses testing
In this study, the model comprises the direct hypotheses from H1 to H4 that are described in this section. The hypothesis testing provided the first indication of the direct effect (H1), namely, that VB significantly predicted consumer resistance to innovation. Hence, H1 was accepted with values of β = 0.366, t = 6.467, and p < 0.000. The second direct effect (H2) of the relationship between RB and consumer resistance to innovation was positively significant, with values of β = 0.174, t = 3.019, and p < 0.001. Similarly, for H3, TB was significantly related to consumer resistance to innovation, so that β = 0.288, t = 3.990, and p < 0.000. Finally, IB also positively influenced consumer resistance to innovation, so that β = 0.311, t = 4.881, and p < 0.000. The results are shown in Table 5.
According to the main goals of this study, the moderation test was the key contributor to determining whether consumer characteristics moderate the relationship between the independent elements (i.e., VB, RB, TB, and IB) and the dependent variable (i.e., consumer resistance to innovation) (Figure 1). Therefore, the first interaction, between VB and consumer characteristics toward consumer resistance to innovation, showed a significant interaction, such that β = 0.142, t = 4.640, and p < 0.000. Therefore, H5 is supported. For the second interaction, the relationship between RB and consumer characteristics was insignificant, as the statistical analysis displayed β = 0.045, t = 1.024, and p < 0.153. As such, H6 is not supported. The third interaction, between TB and consumer characteristics toward the consumer resistance to innovation, showed a substantial interaction, with values of β = 0.232, t = 3.166, and p < 0.001. Therefore, H7 is accepted. The final interaction presented the relationship between IB and consumer characteristics toward consumer resistance to innovation. The statistical analysis revealed a positive interaction with values of β = 0.158, t = 2.988, and p < 0.000. Therefore, H8 is supported. Table 6 displays all the results described above.
According to Dawson (2014), this may be followed by an interaction plot. As a result, to examine the gradient of the slopes, this study used an interaction plot for all interactions. As shown in Figure 2, the line labeled “High Consumer Characteristics” for the first interaction has a steeper gradient if contrasted with “Low Consumer Characteristics”, which indicates that when consumer characteristics are higher, the positive relationship between VB and consumer resistance to innovation is stronger (see Figure 2). The second interaction, between TB and consumer characteristics toward consumer resistance to innovation, showed that the positive relationship between TB and consumer resistance to innovation is greater when consumer characteristics are higher rather than lower (see Figure 3). Figure 4 presents the interaction between IB and consumer characteristics on consumer resistance to innovation. As can be seen from the interaction, the consumer characteristics value strengthens the positive relationship between IB and consumer resistance to innovation, such that the relationship is stronger when the consumer characteristics are higher (see Figure 4).
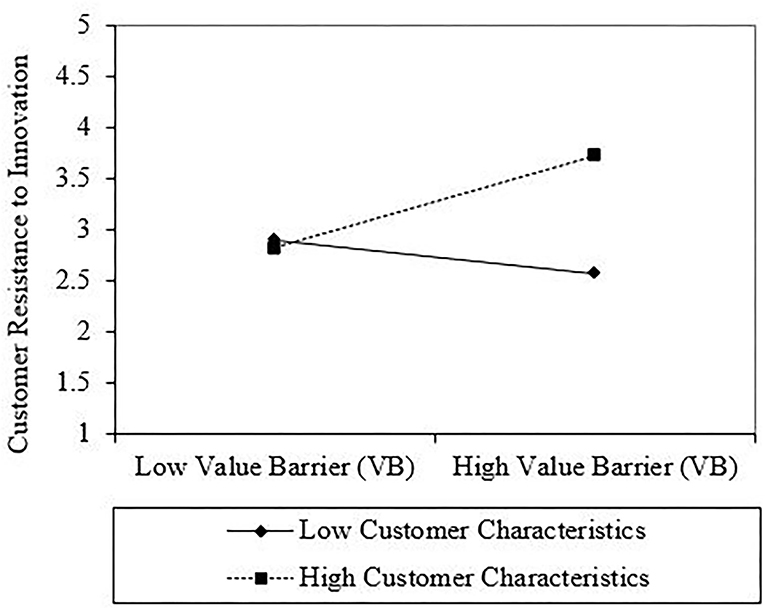
Figure 2. Interaction plot of value barrier (VB) and customer characteristics interaction on the customer resistance to innovation.
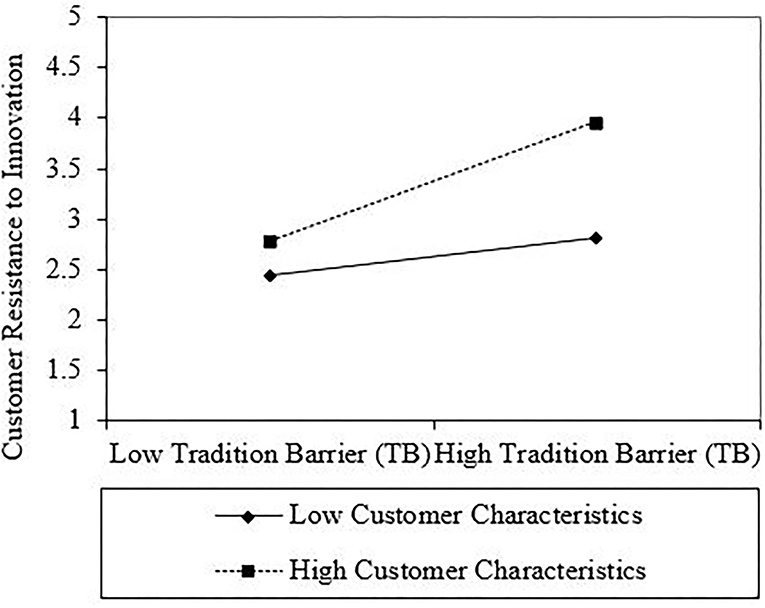
Figure 3. Interaction plot of tradition barrier (TB) and customer characteristics interaction on the customer resistance to innovation.
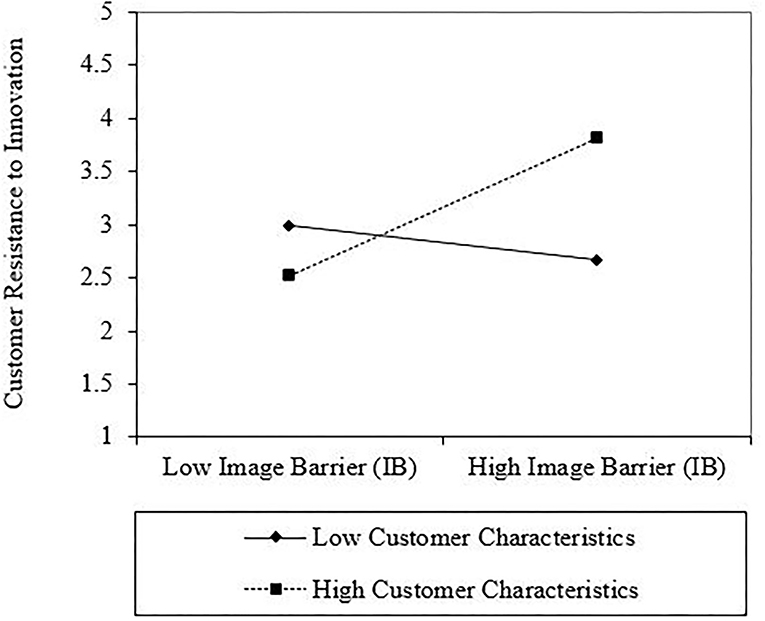
Figure 4. Interaction plot of tradition barrier (IB) and customer characteristics interaction on the customer resistance to innovation.
In terms of the overall explanatory power of the model, R2 = 0.571 for consumer resistance to innovation can be classified as having a moderate-to-substantial effect (Hair et al., 2017). In addition, the Stone–Geisser blindfolding sample reuse technique reveals Q2 values larger than zero, thus indicating that the research model in this study is good for predicting consumer resistance to innovation (Q2 = 0.211) (Hair et al., 2017). Finally, with respect to the overall goodness-of-fit (GoF), the residual index of the standardized root mean square (SRMR) yielded a value of 0.042, which was significantly below the 0.08 cutoff (Henseler and Sarstedt, 2013). Likewise, SRMR's 95% bootstrap quantile is 0.059 and is, therefore, higher than the SRMR value (0.042), indicating that the model possesses a good fit (Hair et al., 2017). Lastly, the discrepancy indexes unweighted least squares discrepancy (dULS) and geodesic discrepancy (dG) are also below the bootstrap-based 95% percentile (dULS = 1.371 < HI 95 of dULS = 2.852; dG = 0.462 < HI 95 of dG = 0.881) (Hair et al., 2017). Therefore, the discrepancy between the model-implied correlation matrix and the empirical study is not significant, thus suggesting that there is no reason to reject the model and that the model is likely to be true (Henseler, 2017).
Discussion
Based on the results for hypothesis H1, VB significantly predicts consumer resistance to innovation. The second direct effect, H2, of the relationship between RB and consumer resistance to innovation was positively significant. Similarly, for H3, TB was significantly related to consumer resistance to innovation. IB also positively influenced consumer resistance to innovation H4 was supported.
In regard to the interactions, the effect found that H5 is supported where the interaction between value barrier and consumers' characteristics was significantly related to customer resistance to innovation, thus H5 is supported. The second interaction between the risk barrier and consumers' characteristics were insignificant; as the statistical analysis indicated, H6 is not supported. The third interaction, between TB and consumer characteristics toward consumer resistance to innovation, revealed a significant interaction. Therefore, H7 is confirmed. The final interaction presents the relationship between IB and consumer characteristics toward consumer resistance to innovation. The statistical analysis showed a positive interaction; therefore, H8 is supported. Above all, most of the variables are true predictors of consumer resistance to innovation.
This study makes substantial contributions to the existing literature by expanding the set of positive outcomes to be expected among factors (i.e., value barrier, risk barrier, tradition barrier, and image barrier) and resistance to innovation in a low-income population. Thus, this study makes a critical contribution by examining these elements in a single research model. Importantly, this tries to extend the theory by investigating the moderating roles of consumer characteristics on the relationships among value barrier, risk barrier, tradition barrier, image barrier, and resistance to innovation. Consumer characteristics greatly influence the demand and uptake of innovations, especially digital innovations (Oh et al., 2022).
Productivity applications in the education industry are among the best tools for managing tasks. This is because they give users access to both personal and educational digital resources. However, productivity applications also face challenges in the educational setting, such as consumer resistance to innovation (Al Halbusi et al., 2020). As a result, this research has several implications based on this study's findings that can be useful in assisting students and universities in increasing the adoption of productivity applications. It also provides deep insight into the factors that significantly influence low-income students' resistance to innovation (Al-Gahtani, 2003).
The insights that can be gained from these results are of vital importance to research on resistance to innovation among low-income populations and for policymakers. Being of low income invites certain conditions that might affect how and when innovations might be resisted. Policy interventions, for instance, might be designed to address the issues revealed by the results of this study. The significance of these results and their use to help overcome resistance to innovation are vital not only to the targeted population group but also to national economic imperatives. The competitiveness of nations and businesses hinges greatly on their ability to generate value through utilizing digital innovations. In contrast, economic competitiveness and the welfare benefits of digital innovations are greater when nations and businesses diverge significantly from utilizing cutting-edge technology (Curzi et al., 2019; El-Haddadeh, 2020). Research such as this on resistance to innovation, especially among underresearched populations, is of vital importance not only to scholars but also to policymakers.
Limitations and suggestions for future research
Just as there are limitations in any research, this study has several limitations. First, because students were the sample used to collect data, the findings cannot be applied to a nonstudent population. As a consequence, future studies should evaluate the sample size, including individuals who are not students, for the findings to be more generally applicable. Moreover, the majority of the respondents were between the ages of 20 and 30. This again limits the generalizability of the results. Thus, it is recommended that future studies encompass a broader age range. This survey, on the other hand, solely includes students from public universities. Future research should include students from both public and private universities in Saudi Arabia. The next limitation of this study relates to the fact that it was a cross-sectional study. As noted, a cross-sectional survey design limits the researcher's ability to identify causality. In other words, a cross-sectional survey design deduces a single data collection point, which is not sufficient to pinpoint causal relations. Thus, the quantitative cross-sectional design aims to identify the mutual occurrence that links antecedents and consequences instead of identifying causality. Thus, since the data that relied on the analysis of the current study were cross-sectional, a longitudinal approach is recommended for future research to provide a better position for researchers to draw causal conclusions, as the Kingdom of Saudi Arabia is huge and has a good number of public universities to study. Lastly, as mentioned previously, most studies on resistance to innovation and its consequences were conducted in the United States and European countries, and there was a need to establish this kind of research in Middle Eastern countries. This is why this study was conducted in Saudi Arabia. As this study is limited to Saudi universities, however, the results cannot be generalized to other Middle Eastern countries due to geographical, political, cultural, and other differences. Therefore, it is recommended to do the same research in other Middle Eastern countries (Kuwait, UAE, Bahrain, Oman, and others), which may enable generalization of the existing findings.
Conclusion
The purpose of this study was to investigate the factors that influence low-income populations' resistance to innovation. Specifically, the case of undergraduate students on financial support and resistance to utilizing digital productivity applications was examined. This study constructed a model of consumer resistance to innovation based on resistance to innovation theory to analyze the variables affecting consumer resistance to innovation (Arnold, 1960; Ram and Sheth, 1989). This framework and the results of this study help to provide a comprehensive understanding of the variables influencing low-income consumer resistance to innovation. This research sheds light on a unique and largely overlooked consumer segment, namely, low-income students, and its findings can be applicable to other low-income population segments. The results of this study reveal a greater need to explore this topic further. For example, it has been identified that low-income populations might be perceived as having a lower value and thus suffer from both a value barrier and a tradition barrier. There remains much to unravel regarding how and why such populations might perceive these barriers differently. Future research could explore these barriers and conduct a comparative study to examine how low-income and high-income students might perceive these barriers differently regarding the same digital innovations. Learning about such issues will greatly inform policy design and interventions to help bring the benefits of such innovations to low-income populations.
Data availability statement
The raw data supporting the conclusions of this article will be made available by the authors, without undue reservation.
Ethics statement
The studies involving human participants were reviewed and approved by University of Hail. Written informed consent for participation was not required for this study in accordance with the national legislation and the institutional requirements.
Author contributions
MAl contributed to research problem formulation, study design, data collection, and overall write up. HAH contributed to questionnaire design, data analysis, and results section write up. MAb contributed to the literature review and discussion section write up. HA contributed to implications, conclusion sections, final proofreading, and quality checks. All authors contributed to the article and approved the submitted version.
Conflict of interest
The authors declare that the research was conducted in the absence of any commercial or financial relationships that could be construed as a potential conflict of interest.
Publisher's note
All claims expressed in this article are solely those of the authors and do not necessarily represent those of their affiliated organizations, or those of the publisher, the editors and the reviewers. Any product that may be evaluated in this article, or claim that may be made by its manufacturer, is not guaranteed or endorsed by the publisher.
References
Abbas, M., Shahid Nawaz, M., Ahmad, J., and Ashraf, M. (2017). The effect of innovation and consumer related factors on consumer resistance to innovation. Cog. Bus. Manag. 4, 1312058. doi: 10.1080/23311975.2017.1312058
Agarwal, R., and Prasad, J. (1998). A conceptual and operational definition of personal innovativeness in the domain of information technology. Infm. Syst. Res. 9, 204–215.
Agosto, D. E., and Hughes-Hassell, S. (2005). People, places, and questions: An investigation of the everyday life information-seeking behaviors of urban young adults. Libr. Inf. Sci. Res. 27, 141–163. doi: 10.1016/j.lisr.2005.01.002
Al Halbusi, H., Al-Sulaiti, K., Abbas, J., and Al-Sulaiti, I. (2022). Assessing factors influencing technology adoption for online purchasing amid COVID-19 in Qatar: moderating role of word of mouth. Front. Environ. Sci. 13, 942527. doi: 10.3389/fenvs.2022.942527
Al Halbusi, H., Hassani, A., and Mosconi, E. (2021). “Social media technologies' use for competitive information and informational trust and their effects on innovation in industrial SMES,” in 2021 IEEE International Conference on Technology Management, Operations and Decisions (ICTMOD) (IEEE), 1–6.
Al Halbusi, H., Jimenez Estevez, P., Eleen, T., Ramayah, T., and Hossain Uzir, M. U. (2020). The roles of the physical environment, social servicescape, co-created value, and customer satisfaction in determining tourists' citizenship behavior: Malaysian cultural and creative industries. Sustainability 12, 3229. doi: 10.3390/su12083229
Aldás-Manzano, J., Lassala-Navarré, C., Ruiz-Mafé, C., and Sanz-Blas, S. (2009). The role of consumer innovativeness and perceived risk in online banking usage. Int. J. Bank Market. 27, 53–75. doi: 10.1108/02652320910928245
Al-Gahtani, S. S. (2003). Computer technology adoption in Saudi Arabia: correlates of perceived innovation attributes. Inf. Technol. Dev. 10, 57–69. doi: 10.1002/itdj.1590100106
Al-Jabri, I. M., and Sohail, M. S. (2012). Mobile banking adoption: application of diffusion of innovation theory. J. Elect. Comm. Res. 13, 379–391. Available online at: https://papers.ssrn.com/sol3/papers.cfm?abstract_id=2523623
Alnoor, A., Al-Abrrow, H., Al Halbusi, H., Khaw, K. W., Chew, X., Al-Maatoq, M., et al. (2022). Uncovering the antecedents of trust in social commerce: an application of the non-linear artificial neural network approach. Compet. Rev. Int. Bus. J. 32, 1–32. doi: 10.1108/CR-04-2021-0051
Andronie, M., Lǎzǎroiu, G., Ştefǎnescu, R., Ionescu, L., and Cocoşatu, M. (2021). Neuromanagement decision-making and cognitive algorithmic processes in the technological adoption of mobile commerce apps. Oecon. Coper. 12, 1033–1062. doi: 10.24136/oc.2021.034
Antioco, M., and Kleijnen, M. (2010). Consumer adoption of technological innovations: Effects of psychological and functional barriers in a lack of content versus a presence of content situation. Euro. J. Market.. 44, 1–26.
Barbu, C. M., Florea, D. L., Dabija, D. C., and Barbu, M. C. (2021). Customer experience in fintech. J. Theoret. Appl. Electron. Commer. Res. 16, 1415–1433. doi: 10.3390/jtaer16050080
Barczak, G., Ellen, P. S., and Pilling, B. K. (1997). Developing typologies of consumer motives for use of technologically based banking services. J. Bus. Res. 38, 131–139. doi: 10.1016/S0148-2963(96)00032-X
Baron, R. M., and Kenny, D. A. (1986). The moderator–mediator variable distinction in social psychological research: Conceptual, strategic, and statistical considerations. J. Pers. Soc. Psychol. 51, 1173. doi: 10.1037/0022-3514.51.6.1173
Bartels, J., and Reinders, M. J. (2011). Consumer innovativeness and its correlates: a propositional inventory for future research. J. Bus. Res. 64, 601–609. doi: 10.1016/j.jbusres.2010.05.002
Blackwell, R. D., Miniard, P. W., and Engel, J. F. (2006). Consumer Behavior. Mason, OH: Thomson/South-Western.
Brislin, R. W. (1970). Back-translation for cross-cultural research. J. Cross Cult. Psychol. 1, 185–216. doi: 10.1177/135910457000100301
Carayannis, E. G., Clark, S. C., and Valvi, D. E. (2013). Smartphone affordance: achieving better business through innovation. J. Knowl. Econ. 4, 444–472. doi: 10.1007/s13132-012-0091-x
Chao, C. W., Reid, M., and Mavondo, F. T. (2012). Consumer innovativeness influence on really new product adoption. Aust. Market. J. 20, 211–217. doi: 10.1016/j.ausmj.2012.02.001
Citrin, A. V., Sprott, D. E., Silverman, S. N., and Stem, D. E. (2000). Adoption of Internet shopping: the role of consumer innovativeness. Ind. Manag. Data Syst.
Cornescu, V., and Adam, C. R. (2013). The consumer resistance behavior towards innovation. Proc. Econ. Finance 6, 457–465. doi: 10.1016/S2212-5671(13)00163-9
Curzi, Y., Fabbri, T., Scapolan, A. C., and Boscolo, S. (2019). Performance appraisal and innovative behavior in the digital era. Front. Psychol. 10, 1659. doi: 10.3389/fpsyg.2019.01659
Davis, F. D., Bagozzi, R. P., and Warshaw, P. R. (1992). Extrinsic and intrinsic motivation to use computers in the workplace 1. J. Appl. Soc. Psychol. 22, 1111–1132. doi: 10.1111/j.1559-1816.1992.tb00945.x
Dawson, J. F. (2014). Moderation in management research: What, why, when, and how. J. Bus. Psychol. 29, 1–19.
Dunphy, S., and Herbig, P. A. (1995). Acceptance of innovations: the customer is the key!. J. High Technol. Manag. Res. 6, 193–209. doi: 10.1016/1047-8310(95)90014-4
Ediriweera, A., and Wiewiora, A. (2021). Barriers and enablers of technology adoption in the mining industry. Resour. Policy 73, 102188. doi: 10.1016/j.resourpol.2021.102188
El-Haddadeh, R. (2020). Digital innovation dynamics influence on organisational adoption: the case of cloud computing services. Inf. Syst. Front. 22, 985–999. doi: 10.1007/s10796-019-09912-2
Ellen, P. S., Bearden, W. O., and Sharma, S. (1991). Resistance to technological innovations: an examination of the role of self-efficacy and performance satisfaction. J. Acad. Market. Sci. 19, 297–307. doi: 10.1007/BF02726504
Fattah, A. M., Al Halbusi, H., Dahleez, K. A., Al Sinawi, S., and Al Wahibi, G. (2021). An investigation of components deriving entrepreneurial activities in Duqm city: a special economic zone in Oman. Int. J. Entrepreneurship. 25, 1–14. Available online at: https://www.abacademies.org/articles/an-investigation-of-components-deriving-entrepreneurial-activities-in-duqm-city-a-special-economic-zone-in-oman.pdf
Gatignon, H., and Robertson, T. S. (1991). “Innovative decision processes,” in Handbook of Consumer Behavior, eds T. S. Robertson, and H. H. Kassarjian (Englewood Cliffs, NJ: Prentice Hall), 316–348.
Ghazali, E.M., Al Halbusi, H., Fattah, F.A., Uzir, M.U.H., Mutum, D.S., and Tan, F.L. (2022). A study of player behavior and motivation to purchase Dota 2 virtual in game items. Kybernetes. 78, 1–25. doi: 10.1108/K-08-2021-0678
Gold, B. (1981). Technological diffusion in industry: research needs and shortcomings. J. Ind. Econ. 247–269. doi: 10.2307/2098302
Gupta, A., and Arora, N. (2017). Understanding determinants and barriers of mobile shopping adoption using behavioral reasoning theory. J. Retail. Consum. Serv. 36, 1–7. doi: 10.1016/j.jretconser.2016.12.012
Hair, J. F., Hult, G. T., Ringle, C. M., Sarstedt, M., and Thiele, K. O. (2017). Mirror, mirror on the wall: a comparative evaluation of composite-based structural equation modeling methods. J. Acad. Market. Sci. 45, 616–632. doi: 10.1007/s11747-017-0517-x
Hair, J. F., Ringle, C. M., and Sarstedt, M. (2011). PLS-SEM: indeed a silver bullet. J. Market. Theory Pract. 19, 139–152. doi: 10.2753/MTP1069-6679190202
Hair, J. F., Risher, J. J., Sarstedt, M., and Ringle, C. M. (2019). When to use and how to report the results of PLS-SEM. Eur. Bus. Rev. 31, 2–24. doi: 10.1108/EBR-11-2018-0203
Hassan, M. M., Jambulingam, M., Alagas, E. N., Uzir, M. U., and Halbusi, H. A. (2020). Necessities and ways of combating dissatisfactions at workplaces against the Job-Hopping Generation Y employees. Global Bus. Rev. 0972150920926966. [Epub ahead of print].
Hassan, M. S., Al Halbusi, H., Razali, A., Ariffin, R. N., and Williams, K. A. (2022). The Swedish gamble: trust in the government and self-efficacy in the battle to combat COVID-19. Curr. Psychol. 1–16. doi: 10.1007/s12144-022-02947-w [Epub ahead of print].
Henseler, J. (2017). Bridging design and behavioral research with variance-based structural equation modeling. J. Advertis. 46, 178–192. doi: 10.1080/00913367.2017.1281780
Henseler, J., and Sarstedt, M. (2013). Goodness-of-fit indices for partial least squares path modeling. Comput. Stat. 28, 565–580. doi: 10.1007/s00180-012-0317-1
Ho, C. H., and Wu, W. (2011). Role of innovativeness of consumer in relationship between perceived attributes of new products and intention to adopt. Int. J. Electron. Bus. Manag. 9, 258–266.
Hoong, C. W., Qureshi, Z. H., Sajilan, S., and Al Halbusi, H. A. (2019). “Study on the factors influencing social entrepreneurial intention among undergraduates,” in 2019 13th International Conference on Mathematics, Actuarial Science, Computer Science and Statistics (MACS) (IEEE), 1–7.
Jansukpum, K., and Kettem, S. (2015). “Applying innovation resistance theory to understand consumer resistance of using online travel in Thailand,” in 2015 14th International Symposium on Distributed Computing and Applications for Business Engineering and Science (DCABES) (IEEE), 139–142.
Joachim, V., Spieth, P., and Heidenreich, S. (2018). Active innovation resistance: an empirical study on functional and psychological barriers to innovation adoption in different contexts. Ind. Market. Manag. 71, 95–107. doi: 10.1016/j.indmarman.2017.12.011
Kaur, P., Dhir, A., Singh, N., Sahu, G., and Almotairi, M. (2020). An innovation resistance theory perspective on mobile payment solutions. J. Retail. Consum. Serv. 55, 102059. doi: 10.1016/j.jretconser.2020.102059
Kezar, A., Perez, R. J., and Swanson, E. (2022). The potential of and mechanisms for a hub of innovation on campus to support changes for low-income, first generation, and racially minoritized college students. Res. High. Educ. 1–24. doi: 10.1007/s11162-022-09690-y [Epub ahead of print].
Kliestik, T., Zvarikova, K., and Lăzăroiu, G. (2022). Data-driven machine learning and neural network algorithms in the retailing environment: Consumer engagement, experience, and purchase behaviors. Econ. Manag. Finan. Markets 17, 57–69. doi: 10.22381/emfm17120224
Kline, R. B. (2010). Principles and Practice of Structural Equation Modeling. New York, NY: The Guilford Press.
Kock, N. (2015). Common method bias in PLS-SEM: a full collinearity assessment approach. Int. J. e-Collaboration 11, 1–10. doi: 10.4018/ijec.2015100101
Kock, N., and Lynn, G. (2012). Lateral collinearity and misleading results in variance-based SEM: An illustration and recommendations. J. Assoc. Infm. Syst. 13. doi: 10.17705/1jais.00302
Kumar, P., Chauhan, S., and Jaiswal, M. P. (2022). An innovation resistance theory perspective on home service applications: The moderating role of country context. Int. J. Consum. Stud. doi: 10.1111/ijcs.12787 [Epub ahead of print].
Kunz, W., Schmitt, B., and Meyer, A. (2011). How does perceived firm innovativeness affect the consumer? J. Bus. Res. 64, 816–822. doi: 10.1016/j.jbusres.2010.10.005
Laukkanen, T. (2016). Consumer adoption versus rejection decisions in seemingly similar service innovations: the case of the Internet and mobile banking. J. Bus. Res. 69, 2432–2439. doi: 10.1016/j.jbusres.2016.01.013
Lee, M. K., Cheung, C. M., and Chen, Z. (2007). Understanding user acceptance of multimedia messaging services: an empirical study. J. Am. Soc. Inf. Sci. Technol. 58, 2066–2077. doi: 10.1002/asi.20670
Leonard, K. J. (2004). Critical success factors relating to healthcare's adoption of new technology: a guide to increasing the likelihood of successful implementation. Elect. Healthcare. 2, 72–81. Available online at: https://papers.ssrn.com/sol3/papers.cfm?abstract_id=2523623
Li, H. X., Hassan, K., Malik, H. A., Anuar, M. M., Khan, T. I., Yaacob, M. R., et al. (2022). Impulsive and compulsive buying tendencies and consumer resistance to digital innovations: the moderating role of perceived threat of COVID-19. Front. Psychol. 13, 912051. doi: 10.3389/fpsyg.2022.912051
Lian, J. W., and Yen, D. C. (2013). To buy or not to buy experience goods online: perspective of innovation adoption barriers. Comput. Human Behav. 29, 665–672. doi: 10.1016/j.chb.2012.10.009
Lian, X., Zhang, J., Azarin, S. M., Zhu, K., Hazeltine, L. B., Bao, X., et al. (2013). Directed cardiomyocyte differentiation from human pluripotent stem cells by modulating Wnt/β-catenin signaling under fully defined conditions. Nat. Protoc. 8, 162–175. doi: 10.1038/nprot.2012.150
Luo, Y., Xue, Q., and Han, B. (2010). How emerging market governments promote outward FDI: experience from China. J. World Bus. 45, 68–79. doi: 10.1016/j.jwb.2009.04.003
Marett, K., Pearson, A. W., Pearson, R. A., and Bergiel, E. (2015). Using mobile devices in a high risk context: the role of risk and trust in an exploratory study in Afghanistan. Technol. Soc. 41, 54–64. doi: 10.1016/j.techsoc.2014.11.002
Mishra, V., Walsh, I., and Srivastava, A. (2022). Merchants' adoption of mobile payment in emerging economies: the case of unorganised retailers in India. Eur. J. Inf. Syst. 31, 74–90. doi: 10.1080/0960085X.2021.1978338
Mohtar, S., and Abbas, M. (2015). Consumer resistance to innovation due to perceived risk: relationship between perceived risk and consumer resistances to innovation. J. Technol. Operat. Manag. 10, 1–13. Available online at: http://stmlportal.net/jtom/wp-content/uploads/2015/12/CONSUMER-RESISTANCE-TO-INNOVATION-DUE-TO-PERCEIVED-RISK.pdf
Mohtar, S. B., Abbas, M., and Baig, M. N. A. (2015). conceptual investigation of consumer resistance to innovations. Middle East J. Sci. Res. 23, 339–346.
Moorthy, K., Ling, C. S., Fatt, Y. W., Yee, C. M., Yin, E. C., Yee, K. S., et al. (2017). Barriers of mobile commerce adoption intention: perceptions of generation X in Malaysia. J. Theoret. Appl. Electron. Commer. Res. 12, 37–53. doi: 10.4067/S0718-18762017000200004
Murdock, G., and Franz, L. (1983). Habit and perceived risk as factors in the resistance to the use of ATMs. J. Retail Bank., 5, 20–29.
Naveed, R. T., Alhaidan, H., Al Halbusi, H., and Al-Swidi, A. K. (2022). Do organizations really evolve? The critical link between organizational culture and organizational innovation toward organizational effectiveness: pivotal role of organizational resistance. J. Innovat. Knowl. 7, 100178. doi: 10.1016/j.jik.2022.100178
O'Connor, E. J., Parsons, C. K., Liden, R. C., and Herold, D. M. (1990). Implementing new technology: management issues and opportunities. J. High Technol. Manag. Res. 1, 69–89. doi: 10.1016/1047-8310(90)90014-U
Oh, G. E., Aliyev, M., Kafouros, M., and Au, A. K. (2022). The role of consumer characteristics in explaining product innovation performance: evidence from emerging economies. J. Bus. Res. 149, 713–727. doi: 10.1016/j.jbusres.2022.05.060
Park, Y., and Chen, J. V. (2007). Acceptance and adoption of the innovative use of smartphone. Ind. Manag. Data Syst. 107, 1349–1365. doi: 10.1108/02635570710834009
Patil, P., Tamilmani, K., Rana, N. P., and Raghavan, V. (2020). Understanding consumer adoption of mobile payment in India: extending Meta-UTAUT model with personal innovativeness, anxiety, trust, and grievance redressal. Int. J. Inf. Manage. 54, 102144. doi: 10.1016/j.ijinfomgt.2020.102144
Pelau, C., Dabija, D. C., and Ene, I. (2021). What makes an AI device human-like? The role of interaction quality, empathy and perceived psychological anthropomorphic characteristics in the acceptance of artificial intelligence in the service industry. Comput. Human Behav. 122, 106855. doi: 10.1016/j.chb.2021.106855
Pérez-Luño, A., Alegre, J., and Valle-Cabrera, R. (2019). The role of tacit knowledge in connecting knowledge exchange and combination with innovation. Technol. Anal. Strat. Manag. 31, 186–198. doi: 10.1080/09537325.2018.1492712
Podsakoff, P. M., MacKenzie, S. B., and Podsakoff, N. P. (2012). Sources of method bias in social science research and recommendations on how to control it. Annu. Rev. Psychol. 63, 539–569. doi: 10.1146/annurev-psych-120710-100452
Ram, S., and Sheth, J. N. (1989). Consumer resistance to innovation: the marketing problem and its solution. J. Consum. Market. 6, 5–14. doi: 10.1108/EUM0000000002542
Reynolds, K. E., Folse, J. A. G. M.A., and Jones (2006). Search Regret: Antecedents and Consequences. J. Retail., 82, 339–348. doi: 10.1016/j.jretai.2006.08.007
Richins, M. L. (1997). Measuring emotions in the consumption experience. J. Consum. Res. 24, 127–146. doi: 10.1086/209499
Ringle, C. M., Wende, S., and Will, A. (2005). SmartPLS 2.0 (software). Hamburg: SmartPLS. Available online at: www.smartpls.de (accessed January 7, 2014).
Rogers, E. M., and Shoemaker, F. F. (1971). Communication of Innovations; A Cross-Cultural Approach. New York, NY: Free Press.
Rogers, E. M., Singhal, A., and Quinlan, M. M. (2014). “Diffusion of innovations,” in An Integrated Approach to Communication Theory and Research (Routledge), 432–448.
Schwartz, S. H., and Sagiv, L. (1995). Identifying culture-specifics in the content and structure of values. J. Cross Cult. Psychol., 26, 92–116.
Sheth, J. N. (1981). Psychology of Innovation Resistance: The Less Developed Concept in Diffusion Research. Vol. 4.
Sivathanu, B. (2018). Adoption of digital payment systems in the era of demonetization in India: an empirical study. J. Sci. Technol. Policy Manag. doi: 10.1108/JSTPM-07-2017-0033
Szmigin, I., and Foxall, G. (1998). Three forms of innovation resistance: the case of retail payment methods. Technovation 18, 459–468. doi: 10.1016/S0166-4972(98)00030-3
Talwar, S., Talwar, M., Kaur, P., and Dhir, A. (2020). Consumers' resistance to digital innovations: a systematic review and framework development. Aust. Market. J. 28, 286–299. doi: 10.1016/j.ausmj.2020.06.014
Tehseen, S., Ramayah, T., and Sajilan, S. (2017). Testing and controlling for common method variance: a review of available methods. J. Manag. Sci. 4, 142–168. doi: 10.20547/jms.2014.1704202
Tornatzky, L. G., and Klein, K. J. (1982). Innovation characteristics and innovation adoption-implementation: a meta-analysis of findings. IEEE Transact. Eng. Manag. 1, 28–45. doi: 10.1109/TEM.1982.6447463
Ullah, F., Sepasgozar, S. M., Thaheem, M. J., and Al-Turjman, F. (2021). Barriers to the digitalisation and innovation of Australian Smart Real Estate: a managerial perspective on the technology non-adoption. Environ. Technol. Innovat. 22, 101527. doi: 10.1016/j.eti.2021.101527
Uzir, M. U., Al Halbusi, H., Lim, R., Jerin, I., Hamid, A. B., Ramayah, T., et al. (2021a). Applied Artificial Intelligence and user satisfaction: smartwatch usage for healthcare in Bangladesh during COVID-19. Technol. Soc. 67, 101780. doi: 10.1016/j.techsoc.2021.101780
Uzir, M. U., Al Halbusi, H., Thurasamy, R., Hock, R. L., Aljaberi, M. A., Hasan, N., et al. (2021b). The effects of service quality, perceived value and trust in home delivery service personnel on customer satisfaction: evidence from a developing country. J. Retail. Consum. Serv. 63, 102721. doi: 10.1016/j.jretconser.2021.102721
Uzir, M. U., Jerin, I., Al Halbusi, H., Hamid, A. B., and Latiff, A. S. (2020). Does quality stimulate customer satisfaction where perceived value mediates and the usage of social media moderates?. Heliyon 6, e05710. doi: 10.1016/j.heliyon.2020.e05710
Veryzer, R. W. Jr. (1998). Discontinuous innovation and the new product development process. J. Prod. Innovat. Manag. 15, 304–321. doi: 10.1111/1540-5885.1540304
Walczuch, R., and Lundgren, H. (2004). Psychological antecedents of institution-based consumer trust in e-retailing. Inf. Manag. 42, 159–177. doi: 10.1016/j.im.2003.12.009
Wang, Y. S., Wang, Y. M., Lin, H. H., and Tang, T. I. (2003). Determinants of user acceptance of Internet banking: an empirical study. Int. J. Serv. Ind. Manag. doi: 10.1108/09564230310500192
Watson, G. (1971). Resistance to change. Am. Behav. Sci. 14, 745–766. doi: 10.1177/000276427101400507
Yadav, M. S., and Varadarajan, P. R. (2005). Understanding product migration to the electronic marketplace: a conceptual framework. J. Retail. 81, 125–140. doi: 10.1016/j.jretai.2005.03.006
Yang, K. C. (2005). Exploring factors affecting the adoption of mobile commerce in Singapore. Telemat. Informat. 22, 257–277. doi: 10.1016/j.tele.2004.11.003
Yu, C. S., and Chantatub, W. (2015). Consumer's resistance to using mobile banking: evidence from Thailand and Taiwan. Int. J. Electron. Commer. Stud. 7, 21–38. doi: 10.7903/ijecs.1375
Yu, C. S., Li, C. K., and Chantatub, W. (2015). Analysis of consumer E-lifestyles and their effects on consumer resistance to using mobile banking: empirical surveys in Thailand and Taiwan. Int. J. Bus. Inf. 10, 21–38.
Keywords: consumer resistance, innovation resistance theory, adoption of innovation, resistance to innovation, consumer behavior, consumers' characteristics
Citation: Alshallaqi M, Al Halbusi H, Abbas M and Alhaidan H (2022) Resistance to innovation in low-income populations: The case of university students' resistance to using digital productivity applications. Front. Psychol. 13:961589. doi: 10.3389/fpsyg.2022.961589
Received: 04 June 2022; Accepted: 18 August 2022;
Published: 07 October 2022.
Edited by:
Shahid Nawaz, Islamia University of Bahawalpur, PakistanReviewed by:
Abd Aziz Othman, Universiti Utara Malaysia, MalaysiaAndreia de Bem Machado, Federal University of Santa Catarina, Brazil
Luigi Aldieri, University of Salerno, Italy
Usama Awan, Lappeenranta University of Technology, Finland
Elvira Nica, Bucharest Academy of Economic Studies, Romania
Copyright © 2022 Alshallaqi, Al Halbusi, Abbas and Alhaidan. This is an open-access article distributed under the terms of the Creative Commons Attribution License (CC BY). The use, distribution or reproduction in other forums is permitted, provided the original author(s) and the copyright owner(s) are credited and that the original publication in this journal is cited, in accordance with accepted academic practice. No use, distribution or reproduction is permitted which does not comply with these terms.
*Correspondence: Mohammad Alshallaqi, shelaqy@gmail.com